the Creative Commons Attribution 4.0 License.
the Creative Commons Attribution 4.0 License.
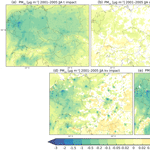
Impact of urban canopy meteorological forcing on aerosol concentrations
Michal Belda
Jan Karlický
Tatsiana Bardachova
Tomas Halenka
Petr Pisoft
The regional climate model RegCM4 extended with the land surface model CLM4.5 was coupled to the chemistry transport model CAMx to analyze the impact of urban meteorological forcing on surface fine aerosol (PM2.5) concentrations for summer conditions over the 2001–2005 period, focusing on the area of Europe. Starting with the analysis of the meteorological modifications caused by urban canopy forcing, we found a significant increase in urban surface temperatures (up to 2–3 K), a decrease of specific humidity (by up to 0.4–0.6 gkg−1), a reduction of wind speed (up to −1 ms−1) and an enhancement of vertical turbulent diffusion coefficient (up to 60–70 m2s−1).
These modifications translated into significant changes in surface aerosol concentrations that were calculated by a “cascading” experimental approach. First, none of the urban meteorological effects were considered. Then, the temperature effect was added, then the humidity and the wind, and finally, the enhanced turbulence was considered in the chemical runs. This facilitated the understanding of the underlying processes acting to modify urban aerosol concentrations. Moreover, we looked at the impact of the individual aerosol components as well. The urbanization-induced temperature changes resulted in a decrease of PM2.5 by −1.5 to −2 µg m−3, while decreased urban winds resulted in increases by 1–2 µg m−3. The enhanced turbulence over urban areas resulted in decreases of PM2.5 by −2 µg m−3. The combined effect of all individual impact depends on the competition between the partial impacts and can reach up to −3 µg m−3 for some cities, especially when the temperature impact was stronger in magnitude than the wind impact. The effect of changed humidity was found to be minor. The main contributor to the temperature impact is the modification of secondary inorganic aerosols, mainly nitrates, while the wind and turbulence impact is most pronounced in the case of primary aerosol (primary black and organic carbon and other fine particle matter). The overall as well as individual impacts on secondary organic aerosol are very small, with the increased turbulence acting as the main driver. The analysis of the vertical extent of the aerosol changes showed that the perturbations caused by urban canopy forcing, besides being large near the surface, have a secondary maximum for turbulence and wind impact over higher model levels, which is attributed to the vertical extent of the changes in turbulence over urban areas. The validation of model data with measurements showed good agreement, and we could detect a clear model improvement in some areas when including the urban canopy meteorological effects in our chemistry simulations.
- Article
(12005 KB) - Full-text XML
- BibTeX
- EndNote
Among the many types of impacts of urban areas on the environment, the impact on the atmospheric environment is regarded to be the “most important and most far-reaching” (Folberth et al., 2015). A major component of this impact is the direct influence of the urban canopy on meteorological conditions: cities are largely covered by artificial surfaces and they affect the physical properties of the air above in a very specific way resulting in an increase in temperatures, a reduction of winds, an increase in turbulence and other meteorological modifications (Lee et al., 2011; Huszar et al., 2014, 2018; Karlický et al., 2018).
The most known aspect of urban influence on meteorological conditions is the formation of the urban heat island (UHI), which has been the subject of a large number of studies since the early 1980s (Oke, 1982). UHI and other related meteorological effects were studied by many authors, and impacts on temperature, wind speed, turbulence and structure of the boundary layer were identified (Basara et al., 2008; Gaffin et al., 2008; Roth, 2000; Kastner-Klein et al., 2001; Hou et al., 2013; Angevine et al., 2003). Cities further influence humidity, precipitation and the hydrological cycle in general (Richards, 2004; Rozoff et al., 2003). With the introduction of urban canopy parameterizations and models of different complexity, modeling approaches describing the urban effects on meteorology and climate became widespread, examining both local (Wouters et al., 2013; Hou et al., 2013) and regional scales (Feng et al., 2013; Trusilova et al., 2008; Struzewska and Kaminski, 2012; Huszar et al., 2014; Karlický et al., 2018). Huszar et al. (2014) showed that urbanization can contribute to regional warming (e.g. Huszar et al., 2014) and determine the climate of whole regions (Květoň and Žák, 2007), as the impact usually exceeds the geographical location of the city itself and propagates to larger scales.
Over urban areas, the meteorological conditions are thus largely perturbed. Consequently, as air chemistry is strongly linked to meteorological conditions, it is expected that modifications in meteorological parameters will result in modifications in species concentrations as well, as has already been shown by many regarding the climate-change-related meteorological changes and their impact on air quality (Huszar et al., 2011; Katragkou et al., 2011; Juda-Rezler et al., 2012).
In particular, the UHI triggers higher urban temperatures, modifies chemical reaction rates and particle nucleation and also influences dry deposition velocities and wet scavenging rates (Seinfeld and Pandis, 1998). Further, Hidalgo et al. (2010) and Ryu et al. (2013b), e.g., showed that the UHI can generate urban breeze circulation, resulting in pollutant transport from and to cities depending on the time of day but also on the surrounding orography and/or the presence of coasts (Ganbat et al., 2015; Li et al., 2017b). Urban surfaces, however, act in the opposite direction a well: higher drag induces wind stilling and thus suppresses the dispersion of urban emissions and secondary pollutants into regional scales. Surface heterogeneities in urban areas further enhance turbulence, and increased eddy transport helps pollutant transport to upper layers of the urban boundary layer (UBL; Stutz et al., 2004). In general, a very strong link is identified between the state of the UBL and pollution (Masson et al., 2008).
The above-listed effects act, however, simultaneously in a rather complex manner, requiring coupled modeling approaches. Most of the work done focused on gas-phase chemistry, especially ozone (O3) and nitrogen oxide (NOx) changes due to urban land surface forcing. Martilli et al. (2003) and Sarrat et al. (2006) each focused on one single city, Athens and Paris, and found a significant impact on pollutant concentrations, mainly due to changed turbulence when urban surfaces are considered. Civerolo et al. (2007), using a bulk approach for the description of urban surfaces, predicted a considerable increase in the episode maximum 8 h average O3 concentration due to future urbanization. Struzewska and Kaminski (2012) looked at the southern Poland region and found a reduction of primary pollutants (NOx and CO) due to enhanced vertical mixing. Recently, Fallmann et al. (2016) analyzed ozone changed after urban greening utilization and found O3 reduction due to temperature mitigation, but increases of primary pollutants due to the associated decreased mixing. A large number of authors focused on Chinese cities and urban areas (Wang et al., 2007, 2009; Xie et al., 2016a; Zhu et al., 2017). They calculated an ozone concentration increase due to urbanization which has a similar magnitude to future emission changes and changed climate, emphasizing the importance of considering urban canopy effects in air quality modeling. Liao et al. (2014) investigated how different urban canopy parameterizations influence the air quality prediction for a Chinese agglomeration. Sulfur dioxide (SO2) changes due to urbanization were modeled by Chen et al. (2014) who found significant decreases of this important primary pollutant over urban areas, caused mainly by increased vertical mixing over the urban canopy. Ryu et al. (2013a) and Ryu et al. (2013b) modeled the urbanization impact on ozone concentrations over Seoul, South Korea, and identified a strong urban breeze circulation greatly affecting ozone concentrations, and this was found to be further modulated by the effect of anthropogenic heat released from the city. Regarding aerosols, Zhu et al. (2017) investigated the impact of the change of land use from natural to artificial due to urban expansion on coarse particle matter (PM10) and found decreases driven mainly by enhanced vertical eddy transport. Increases of planetary boundary layer (PBL) height and turbulence over urban areas were the main reason for decreases of primary pollutant concentration near the surface in Xie et al. (2016b) and Li et al. (2017a). Over Toulouse, France, Masson et al. (2008) analyzed how fine-mode aerosols interact with the urban boundary layer and found a strong role of vertical dispersion in modulating their concentration. Recently, de la Paz et al. (2016) found that the application of urban canopy models instead of simple “bulk” approaches has a positive impact on model accuracy for both gaseous species (NO2 and O3) and PM2.5. For fine aerosol they found that the main driver for changes is the lower wind in urban areas that is closer to observed values than that modeled with simple approaches or without considering urban surfaces at all. For Paris, France, Kim et al. (2015) showed that when using an urban canopy model that triggers stronger vertical mixing in the model over urban areas, PM2.5 concentrations are lower and agree better with observations. The important consequences that land use changes and, particularly, urbanization have on PM concentrations were noted by Tao et al. (2013) too.
The listed studies point to different meteorological changes occurring over urban areas that influence local and regional air pollution, often in an opposite manner. Indeed, when evaluating the integrated chemical effect of urban canopy meteorological forcing, one encounters a number of difficulties. First of all, the individual meteorological components (like temperature changes, modifications of turbulence etc.) often counteract each other. Enhanced urban temperatures trigger higher reaction rates for production as well as chemical destruction of secondary pollutants. Decreases in wind speeds block pollutants from dispersing into larger scales; however this is also true for their precursors which stay close to sources and can trigger their destruction, like in the case of ozone–NOx interaction. However, at the same time, enhanced vertical mixing contributes to their removal, which decreases the concentrations. Another complexity in the urban meteorology–air chemistry interaction is presented by the fact that the urbanization-induced meteorological features as well as emissions are not uniformly distributed in time and their daily cycles have specific features. Maximums and minimums in their peaks sometimes occur simultaneously, leading to the amplifications of the effects. In the case of secondary aerosols, the situation is even more complicated as their concentrations are influenced not only directly via the urban meteorological effects, but also via changes in their gas-phase precursors. The overall impact on aerosol concentration is then the complex combination of the impact on individual aerosol components, which, in the case of secondary aerosols, is largely modulated by the impact on the source precursors. It is clear that an integrated meteorology and air quality modeling framework is an inevitable tool to analyze the changes of air pollutants due to urban canopy land surface forcing in detail.
Here, we introduce a model estimate of the impact of the urban canopy meteorological forcing on fine aerosol (PM2.5) concentrations over European urban areas. This study is a follow-up to Huszar et al. (2018) in which the impact was analyzed from the perspective of gas-phase chemistry, focusing especially on NOx and ozone. Here, we make a further step and look at aerosols' concentrations and as a novelty, our study, as one of the first to do so, will investigate each component of PM2.5, as it is expected that different components of aerosol respond differently to urban meteorological forcing. Another novelty of the study is that instead of modeling selected short periods (although interesting from a meteorological perspective), we perform continuous long-term simulations in order to capture the long-term average impact. Our study is motivated by the appreciation that urban fine aerosol still represents a substantial threat to the public in large cities (Cheng et al., 2016) and represents an important aspect of cities' air pollution (Huszar et al., 2016a). In order to implement measures for its reduction, proper knowledge of the contributors to pollution levels is crucial and this has to include the potential contribution from land use changes related to urbanization as well.
2.1 Models
Models used in this study were introduced and used with a very similar setup to that used in Huszar et al. (2016a, b, 2018). Here we provide a rather brief but self-consistent description of them. The regional climate model RegCM (version 4.4) was used as a meteorological driver (Giorgi et al., 2012). For convective- and large-scale precipitation, the Grell and SUBEX schemes were invoked, respectively (Grell, 1993; Pal et al., 2000). The planetary boundary layer processes were modeled using the Holtslag scheme (Holtslag et al., 1990). Radiative transfer calculations were conducted using the NCAR Community Climate Model Version 3 (CCM3; Kiehl et al., 1996).
The Community Land Model version 4.5 (CLM4.5; Lawrence et al., 2011; Oleson et al., 2013) was chosen to describe the land-cover processes. CLM4.5 provides a more comprehensive description of land surface processes compared to the simple BATS land surface model which is originally included in the RegCM model (Dickinson et al., 1993). CLM4.5 further contains the CLMU (Community Land Model Urban) urban canopy scheme (Oleson et al., 2008a) based on the traditional canyon representation of urban areas. The canyon consists of roofs, walls and the canyon floor. Trapping of solar and long-wave radiation within the canyon is taken into account. Momentum fluxes are calculated for the urban land unit using roughness lengths and displacement heights typical for the canyon. Anthropogenic heat from air conditioning and heating is computed online within the CLMU from the heat conduction equation, with interior boundary conditions represented by the interior temperature of the building. Another heat flux is added to this anthropogenic heat flux that accounts for the waste heat from air heating/conditioning. It is parameterized directly from the amount of energy required to keep the internal building temperature between prescribed maximum and minimum values, assuming 50 % efficiency of the heating/cooling systems (Oleson et al., 2008b).
We have chosen to use the CLM4.5 scheme as it produces a stronger and more realistic UHI compared to the BATS scheme and gives a more emphasized wind speed decrease (see Huszar et al., 2014, 2018). It further reduces the overestimation of evaporation in summer seen in the BATS scheme and hence models precipitation with a higher accuracy, which was already concluded by Wang et al. (2015) who compared these two schemes.
For chemical simulations, the chemistry transport model (CTM) CAMx version 6.30 ENVIRON (2016) was coupled offline to the regional climate model. CAMx is an Eulerian photochemical CTM implementing multiple gas-phase chemistry mechanism options (CBV, CB6, SAPRC07TC). In this study, the CBV scheme (Yarwood et al., 2005) is invoked. CAMx further implements static two-mode treatment as well as multisectional particle size treatment and wet and dry deposition of gases and particles, and it calculates the composition and phase state of the ammonia–sulfate–nitrate–chloride–sodium–water inorganic aerosol system in equilibrium with gas-phase precursors using the ISORROPIA thermodynamic equilibrium model (Nenes and Pandis, 1998) activated in our setup. For aerosol, we invoked the two-mode particle size treatment, and the semi-volatile equilibrium scheme called SOAP (Strader et al., 1999) for the formation of secondary organic aerosol (SOA) was used.
As an offline couple, no feedbacks from the simulated species concentration changes on RegCM radiation/microphysical processes (cloud/rain) were taken into account. Huszar et al. (2016b) showed minor effects of these feedbacks in long-term averages, so we consider this to be a reasonable simplification. The RegCM-generated meteorological fields are translated into CAMx input using the RegCM2CAMx preprocessor (Huszar et al., 2012). In RegCM2CAMx, we replaced the O'Brien (1970) method for calculating the vertical eddy diffusion coefficients (required by CAMx) with the more advanced Byun (1999) scheme. This leads to a better match of model results with measurements (Eben et al., 2005). With this choice, an inconsistency is introduced to the modeling setup as the methods calculating vertical diffusion coefficient differ between the climate and chemistry models. To achieve the highest degree of coupling between the driving climate model and a chemistry transport model, the Holtslag et al. (1990) scheme produced vertical diffusion (Kv) parameters that should have been used directly to drive the vertical diffusion in CAMx. As RegCM4 does not support the output of these parameters (in the versions and configurations used), it was technically much simpler to take the vertical profiles of wind speed and temperature as well as the PBL height from the driving model and apply a diagnostic method to calculate the Kv for CAMx (provided by Byun, 1999). Applying a “inconsistent” method in calculating Kv for CTMs, however, does not implicate less accurate results than directly coupling the PBL parameters, as shown by Lee et al. (2011).
2.1.1 Data and simulations
The models were applied for the same domain and configuration as in Huszar et al. (2018); i.e., 10 km × 10 km horizontal resolution centered over Prague, Czech Republic, with grid boxes in x, y, and z directions. It is clear that with such a horizontal resolution, the fine-scale structure of chemical transformation of emissions from cities cannot be resolved. With this resolution, emissions from cities enter the atmosphere through a few grid boxes while considering instant dilution on the model scale. It is clear that due to the nonlinearity of chemical processes, this can lead to some errors in the final species concentrations. This effect is considered, however, to be small in the case of city emissions (Markakis et al., 2015). Many previous studies also agreed that resolutions similar to ours are suitable to model the regional impact of urban emissions (Varghese et al., 2011; Tie et al., 2010) although some overestimation of secondary species is documented when using a coarser model resolution (Karlický et al., 2017).
The top model levels correspond to 50 hPa for the climate model. CAMx was only set up on the lowermost 18 levels (up to approximately 10 km). Lateral boundary conditions for the regional climate calculations were taken from a 50 km × 50 km resolution experiment carried out using the RegCM4 model within the EURO-CORDEX initiative (Vautard et al., 2013) driven by the ERA-Interim reanalysis (Simmons et al., 2010).
The TNO emissions prepared in the framework of the FP7 MEGAPOLI project were used (Kuenen et al., 2010) as the anthropogenic emissions' source. They provide high-resolution ( longitude, latitude, roughly 7 km × 7 km) European data of annual emission estimates for NOx, SO2, non-methane volatile organic compounds (NMVOCs), methane (CH4), ammonia (NH3), carbon monoxide (CO) and particulates (PM10 and PM2.5) in 10 activity sectors. For each sector, specific temporal disaggregation factors and NMVOC speciation profiles were applied to decompose the annual sums into hourly emissions following Winiwarter and Zueger (1996). For chemical initial and boundary conditions (ICBCs), a 30 km × 30 km domain run was performed covering the whole of Europe. This large domain run was, in turn, driven by time–space-invariant chemical ICBCs. This choice of chemical ICBCs resulted in some model biases listed in Huszar et al. (2016a), especially regarding ozone. Meteorology-dependent biogenic emissions of isoprene and monoterpenes (BVOCs) were considered in the study following Guenther et al. (1993).
Land use was extracted from the USGS data, while the urban land unit percentage was derived from the resolution LandScan2004 dataset based on census, nighttime light satellite observations and road proximity (Jackson et al., 2010). They define 132 regional categories (the world is divided into 33 regions with similarities in urban characteristics, and each category is subdivided into 4 subcategories representing different urban intensities – tall building district (TBD), high density (HD), medium density (MD) and low density (LD)). For each bottom category, average building heights (H), urban canyon height-to-width ratios (H : W), and fractions of pervious surface (e.g., vegetation), roof area and impervious surfaces (e.g., roads and sidewalks) are defined, among other parameters. The urban land unit within CLM4.5 is represented as a fraction in percentages of three (of the four in Jackson et al.) urban intensities (HD, MD and LD). This gives a reasonable description of urban coverage at 10 km resolution, and even small cities well below 10 km in diameter are accounted for. For chemical simulations, the land use was kept the same for all experiments in order to separate the effect of meteorological changes only.
The experiments cover a period 2001–2005, with the year 2000 as a spin-up. Summer months (JJA) are analyzed in our study when urban effects are the most pronounced. With the regional climate model, one pair of experiments was carried out: (i) a reference simulation (NOURBAN experiment), which does not consider the urban land unit, meaning that urban grid boxes are replaced with the one that is most typical for the surrounding grid boxes, most often crops; (ii) and a simulation that considers urban surfaces and that is parameterized with the urban canopy model CLMU (URBAN experiment).
Within the impact of the simulated meteorological changes on chemistry, the following effects are considered: (1) modified temperature (t impact); (2) modified absolute humidity (q impact); (3) modified wind field (uv impact) and (4) modified turbulence (via changes in the vertical eddy diffusion coefficient; Kv impact). A number of experiments with the chemistry model CAMx were carried out depending on which effect is ex-/included. The reference CAMx simulation is driven by the reference climate simulation and is denoted in the same way: NOURBAN. Afterwards, a “full” experiment was carried out whereby all the listed effects were considered (the URB_t+q+uv+Kv experiment). To obtain a more detailed picture about the role each process plays, additional experiments were carried out by turning on the individual components of the overall meteorological effect one by one following the methodology in Huszar et al. (2018). Accordingly, the URB_t experiment only considered the urban temperature effects. In the URB_t+q experiment, both temperature and humidity effects were accounted for. The effect of modified wind was added to the URB_t+q+uv experiment. Finally, in the full experiment, the effect of modified eddy diffusion coefficients on chemistry was considered. With such a “cascading” approach, one can analyze the separate impact of individual meteorological parameters and their contribution to the total impact. Although this experimental approach must lead to some inconsistency in the meteorological driving fields, e.g., the vertical layer structure (layer interface heights) defined in the CAMx input will be not consistent with the increased temperature in the URB_t experiment, these simulations serve only to explain how the chemical changes are built up from summing up of the meteorological influences, and an assumption is made according to which of the possible effects of these inconsistencies is small when averaged over a long period. Further, the role of the ordering of individual impacts was investigated. Short runs were carried out for summer 2001 (not shown here) for which the temperature, humidity, wind and turbulence changes are considered in different orders and the results for individual components were practically the same. The effect of temperature-driven BVOC changes is not accounted for as it is rather negligible (Huszar et al., 2018).
In Huszar et al. (2016a), an extensive validation is provided for the chemistry simulated by the RegCM4–CAMx couple for the 2001–2010 period. Here, the same configuration and input data are used except that the CLM4.5 surface model is used instead of the older BATS scheme. We only expect a minor impact on the simulated species concentrations, as lateral boundary conditions and emissions were identified as the main sources of model bias, and these are the same in this study. We only provide a spatial comparison of modeled PM2.5 concentrations with observations (see the following), mainly to examine possible model improvement when urban canopy effects are considered in the driving meteorology. Regarding the meteorology, we use the same pair of (URBAN–NOURBAN) experiments to drive CAMx as in Huszar et al. (2018). They compared temperature and precipitation fields with observational data and found an underestimation of modeled temperatures by 2–3 K and both under- and overestimation of precipitation by ±30 % depending on the location. In this study, we make the assumption that the simulated impact of the urban canopy on climate is only influenced by these biases in a minor way, as the impact is always calculated as a difference, and thus the biases are partly eliminated.
3.1 Impact on meteorological conditions
Huszar et al. (2018) provided both the spatial impact of the urban canopy on meteorological conditions and the diurnal cycle of the impact over selected cities. Here we limit our presentation to the diurnal cycles extended with the impact on the absolute humidity. If not specified otherwise, the diurnal cycles are plotted in sundial time (approximately UTC+1 h for almost the entire domain). We analyzed a large number of cities; however the results showed a high degree of similarity between them. Therefore, here we present only two cities, Berlin and Prague. For the humidity, we also show the spatial impact presented for day- and nighttime, considering 11:00–16:00 and 00:00–05:00, respectively. Shaded areas mean a statistically significant difference at the 98 % level using the t test.
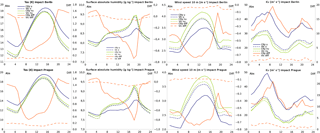
Figure 1Diurnal cycle of the modeled near-surface temperature in ∘C, absolute humidity in g kg−1, 10 m wind speed in ms−1 and the eddy diffusion coefficient in the fifth model level (about 700–800 m) in m2s−1 in local time for two selected cities, Berlin and Prague: blue – URBAN experiment, green – NOURBAN experiment, red – difference of URBAN and NOURBAN simulations, solid line – city averaged values, dashed line – city vicinity.
In Fig. 1, the JJA average diurnal cycle of (panels a–e) the near-surface temperature, humidity, 10 m wind speed and vertical eddy diffusion coefficient over selected cities (solid lines) and their vicinities (dashed lines) is plotted. The pattern regarding the temperature impact is very similar for both cities. As expected, the experiment with urban surfaces predicts higher temperatures over urban areas, while the difference reaches almost zero during morning hours. Maximum temperatures are enhanced by about 0.5 to 1 ∘C when urban land use type is considered. The largest impact occurs during the late afternoon and evening, peaking around 20:00. The plots further reveal an almost negligible impact over the city vicinities (increase up to 0.2 ∘C). The diurnal temperature range is reduced by 1.5 to 2 ∘C.
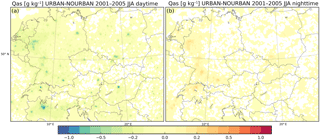
Figure 2Impact of urban canopy on the near-surface absolute humidity for 2001–2005 JJA in g kg−1 for daytime (a) and nighttime (b). Shaded areas represent a statistically significant impact at the 98 % level using the t test.
The diurnal cycle of absolute humidity is plotted in Fig. 1, second column. In absolute values, maximum values are reached during late afternoon hours, when the evaporation from the surface is highest, reaching 9–10 g kg−1. As expected, humidity is decreased due to the presence of urban surfaces, caused mainly by decreased evaporation and increased runoff. The decrease is largest simultaneously with the largest absolute values occurring during 19:00–20:00. It can reach −0.7 to −0.8 g kg−1. The plot also reveals that during nighttime, the urban impact on absolute humidity can be positive. Similarly, the humidity values from the urban vicinity are slightly lower than over the city (seen in the URBAN experiment). This is probably connected to higher capacity of urban air to hold water vapor (due to higher nighttime temperatures), or in other words, rural air cools more quickly during the night and more vapor is removed by condensation on the surface. Higher humidity during nighttime due to the urban surfaces is also seen on the spatial distribution of the impact in Fig. 2, where it can reach 0.2 g kg−1, especially for cities over the western part of the domain, probably due to the more humid maritime climate that they have. On the other hand, during daytime, a clear decrease of absolute moisture is modeled, with peaks over cities often exceeding −1 g kg−1 (e.g., for Milan, or Budapest).
In the case of the impact on wind, as expected, the wind speeds during the day are higher compared to those during the night. The decreases can reach about −0.6 to −0.8 ms−1 and their timings match the timing of the highest absolute wind speeds during daytime. This is expected as the change is proportional to the absolute values. The impact on wind is smallest around 19:00–20:00, when the evening PBL transition occurs (Huszar et al., 2018).
The last column of Fig. 1 shows the diurnal cycle of the vertical maximum of the turbulent diffusion coefficient. A systematic increase by 10–20 m2s−1 is modeled due to urban surfaces throughout the day, with a maximum occurring during morning hours. The minimum is modeled during afternoon to late evening hours. The impact on the city vicinities is practically negligible.
3.2 Impact on aerosols
Regarding the impact of urban canopy meteorological forcing on aerosol concentration, we will start with the impact on the PM2.5 surface concentrations. Next, the impact on its components, i.e., primary and secondary (in)organic aerosols, will be evaluated. For all aerosol types, besides the total impact, results will also be provided for the individual components (t, q, uv and Kv impact). These aerosol components are considered: sulfates (PSO4), nitrates (PNO3), ammonium (PNH4), primary organic aerosol (POA), primary elemental carbon (PEC), other fine particulate matter (FPRM) and secondary organic aerosol (SOA). Further, for each component, both the spatial distribution of the impact and also the diurnal cycle over selected cities is presented. In the spatial distribution figures, shaded areas represent statistically significant differences at the 98 % level using the t test.
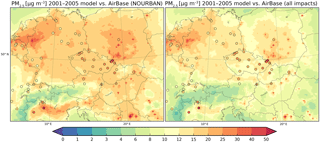
Figure 3Absolute surface PM2.5 concentrations in µg m−3 averaged over the 2001–2005 JJA period for the NOURBAN experiment (i.e., without urban surface meteorological effects) and with the experiment for which all urban meteorological effects are considered. Circles represent measured values from the AirBase database.
3.2.1 PM2.5
Figure 3 shows the modeled 2001–2005 average surface concentration of PM2.5 for the NOURBAN experiment (left) and for the experiment with all meteorological effects included (URB_t+q+uv+Kv; right). Colored dots denote measured averages extracted from the European Environment Agency AirBase data background stations. Largest PM2.5 values are modeled over highly populated large agglomerations like the Ruhr area in Germany, the Po valley in northern Italy or southern Poland, where summer average values can reach 30–50 µg m−3. The comparison to measured data shows some model underestimation, especially over central European countries and northern Italy, where model values are lower by 5–10 µg m−3. On the other hand, over large parts of Germany, model results agree with measurements or are slightly positively biased. If considering the urban canopy meteorological effects, PM2.5 is clearly decreased by about 3 µg m−3 over urban areas. This leads to better model results over parts of western Europe, but over areas where PM2.5 is underestimated, the model bias is increasing.
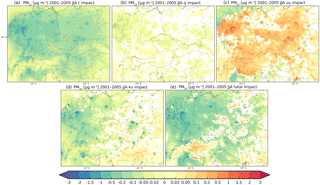
Figure 4Impact of urban canopy meteorological forcing on PM2.5 surface concentrations in µg m−3 for the 2001–2005 JJA period for (a) temperature (t), (b) humidity (q), (c) wind (uv), (d) turbulence (Kv) and (e) total impact. Shaded areas represent a statistically significant impact at the 98 % level using the t test.
The perturbation of PM2.5 surface concentrations due to urban canopy meteorological changes is plotted in Fig. 4 for the temperature, humidity, wind and turbulence impact as well as the combined total impact (from left to right from top to bottom). Regarding the temperature impact, there is a domain-wide decrease peaking over cities with values from −1.5 to −2 µg m−3, especially over the western part of the domain (e.g., over the Ruhr area in Germany). Smaller decreases are modeled for central and eastern European cities (up to −0.5 µg m−3 decrease). The impact is not only seen over urban areas but propagates to rural ones as well, with statistically significant decreases around −0.1 to −0.2 µg m−3. Changes in moisture content (decrease on average) only cause a slight decrease of PM2.5 over most of the western part of the domain up to −0.1 µg m−3. Over other parts, small increases are modeled. The urbanization-induced wind stilling causes PM2.5 to increase over most of the domain, with peaks, as expected, over cities up to 2 µg m−3. Statistically significant increases are modeled even over rural areas, with less urban fraction often exceeding 0.5 µg m−3. PM2.5 responds to the increased vertical mixing due to urban surfaces with decreases over cities by up to −2 µg m−3, again mainly over the western part of the region in focus. Above some rural areas, statistically significant increases are modeled; however these are very small, not exceeding 0.1 µg m−3 and most often below 0.05 µg m−3. The total impact of all the considered components shows spatial similarities with the turbulence impact – it is enhanced or suppressed depending on the competition between the temperature (decreases) and wind impact (increases). However, over most of the cities, the temperature impact is stronger in magnitude than the wind impact, causing the total impact to be enhanced, often reaching −3 µg m−3 decrease.
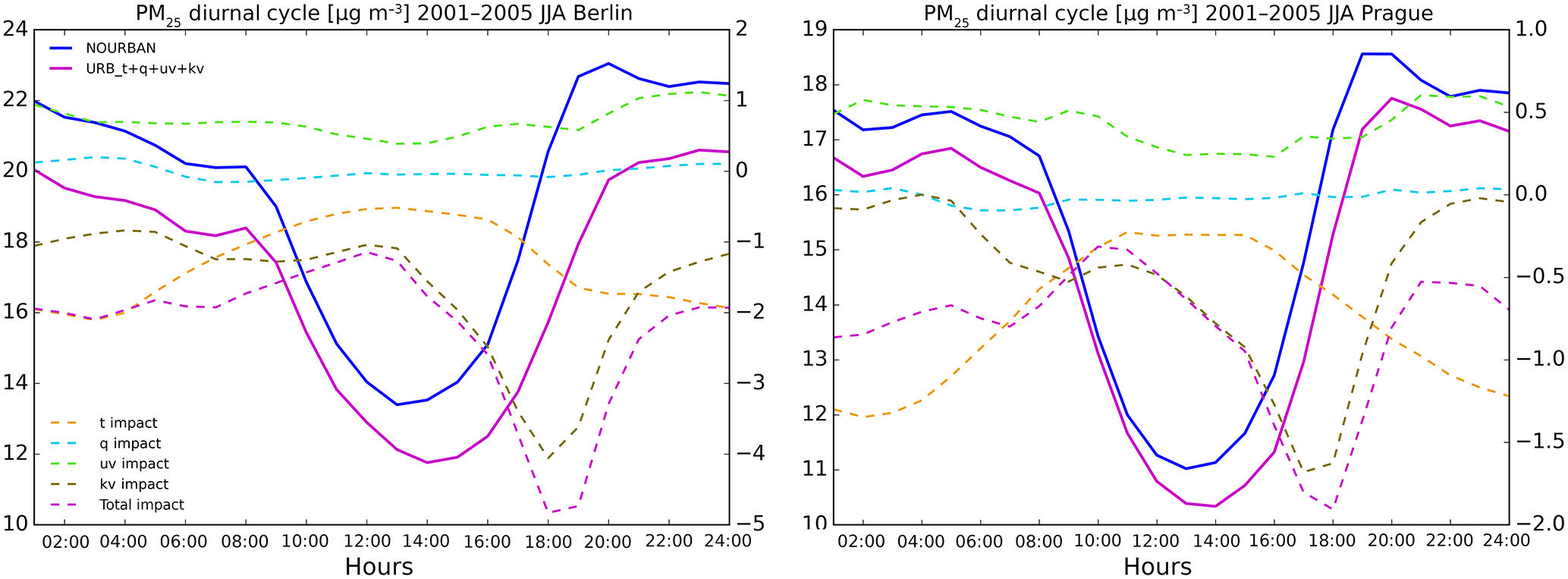
Figure 5Impact of urban canopy meteorological forcing on PM2.5 average diurnal cycle of surface concentrations for the 2001–2005 JJA period for two selected cities (Berlin and Prague) in µg m−3. Bold lines represent the absolute concentrations (left y axis) for the NOURBAN run (blue) and the total impact URB_t+q+uv+Kv run (purple). Dashed lines (right y axis) show the change due to changes of individual meteorological components (temperature – orange, humidity – aquamarine, wind – green, turbulence – olive and total impact – purple).
As has already been pointed out, the urban meteorological phenomenon has a well defined diurnal cycle. Thus, it can be expected that the above-presented impacts on aerosol are not uniformly distributed across the day but will have a specific diurnal cycle too. In Fig. 5 the diurnal variation of the PM2.5 absolute surface concentrations and their change over two representative cities, Berlin and Prague, is presented. The impact over other cities is qualitatively the same in shape, only with different magnitudes. The absolute values (solid lines) are plotted for the NOURBAN case and for the experiment with all urban meteorological effects considered (URB_t+uv+q+Kv). The changes due to individual and combined urban meteorological effects are plotted with dashed lines. Fine particulates' concentrations for the selected cities have a clear diurnal cycle with maxima (minima) around 18:00–22:00 (10:00–14:00) µg m−3 occurring around 19:00–20:00 (13:00-14:00). Regarding the diurnal cycle of the effect of individual meteorological components, there are, however, substantial differences. Temperature (orange) causes decreases by up to 1.5–2 µg m−3, with a maximum during nighttime, while the minimum occurs around noon (around −0.5 µg m−3 change). As already seen in the spatial figure, the changes due to modified humidity (aquamarine) are very low, not exceeding 0.05 µg m−3. A wind decrease causes an increase in urban PM2.5 concentrations (green) by around 0.5–1 µg m−3 throughout the day, while changes are higher when the absolute values are higher too, i.e., during the evening and nighttime. A very clear daily cycle is modeled for the turbulence-induced concentration change (olive), with a profound maximum (of the absolute change) occurring at 18:00–19:00, with values around −4 and −1.6 µg m−3 for Berlin and Prague, respectively. The daily cycle of the total impact (purple) has a similar shape to the turbulence impact, with a maximum occurring around 18:00–19:00, reaching −5 and −2 µg m−3 for Berlin and Budapest, respectively. A smaller secondary peak occurs during nighttime, when the temperature component is strong. In summary, the most emphasized change is caused by increased turbulence; thus the total impact is dominated by this component too.
It is clear that, in general, different components of the fine aerosol will contribute differently to the absolute concentrations as well as to the changes presented above. To see what the relative importance of each aerosol type is, in Fig. 6 we plotted the relative composition of the average summer aerosol for six selected cities. The largest contribution is made by sulfates and nitrates, being around 50 %, while sulfates dominate, especially over eastern European cities. Ammonium constitutes about 15 % of the total fine aerosol. In the emission database used (TNO), emissions of SO+2 over eastern European countries are much higher than over western Europe, while the opposite is true for the NOx emissions. As ammonium emissions are also slightly higher over western Europe, it is clear that over eastern Europe, sulfate ions will prefer to stabilize nitrate ions, resulting in ammonium sulfate formation, while over western Europe, the emissions ratios will favor the formation of ammonium nitrates (Schaap et al., 2004). Primary organic aerosol, primary elemental carbon (black carbon) and other fine particulate matter constitutes around 10 %, 5 % and 10 %, respectively. Secondary organic aerosol has the lowest contribution of around a few percent. In the following, the impact of each component of the urban meteorological forcing on the individual aerosol type will be analyzed, starting with the primary aerosols. Note that we ignored the humidity effect, as its contribution to the total PM2.5 change turned out to be minor.
3.2.2 Primary aerosols
Figure 7 shows the spatial distribution of urban meteorological forcing on the surface concentration of primary aerosols, POA, PEC and FPRM. In the chosen model configuration, these primary aerosols do no interact with chemistry, and their emissions as well as their contribution to the total fine aerosol over urban areas are comparable (see Fig. 6). Therefore, their response to different meteorological modifications is comparable. The impact of temperature changes is minor, reaching only a few −0.01 µg m−3 change over urban areas. A more emphasized impact is modeled due to urban wind changes reaching 0.3–0.4 µg m−3 over many cities. Increased turbulence leads to statistically significant changes almost over the entire domain, with decreases up to −0.5 µg m−3. The total impact reflects the opposite sign of the wind and turbulence changes and is somewhat lower reaching −0.3 µg m−3, but over some areas where the wind impact dominates, it can be even positive up to 0.2 µg m−3. Due to lower values, the statistically significant changes occupy smaller areas, mainly limited around large cities.
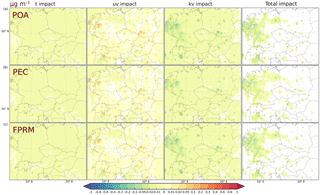
Figure 7Impact of urban canopy meteorological forcing on (a–c) POA, PEC, FPRM average 2001–2005 JJA surface concentrations in µg m−3. The columns from left to right correspond to temperature, wind, turbulence and the total impact. Shaded areas represent a statistically significant impact at the 98 % level using the t test.
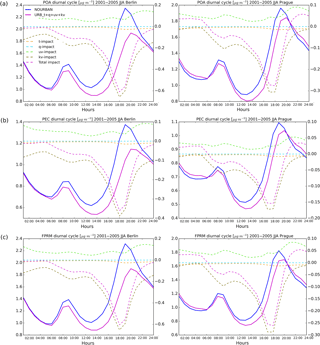
Figure 8Impact of urban canopy meteorological forcing on (a–c) POA, PEC and FPRM average diurnal cycle of surface concentrations for the 2001–2005 JJA period for two selected cities (Berlin and Prague) in µg m−3. Bold lines represent the absolute concentrations (left y axis) for the NOURBAN run (blue) and the total impact URB_t+q+uv+Kv run (purple). Dashed lines (right y axis) show the change due to changes of individual meteorological components (temperature – orange, humidity – aquamarine, wind – green, turbulence – olive and total impact – purple).
The diurnal cycle of the absolute urban primary aerosol concentration as well as changes due to urban meteorological forcing is shown in Fig. 8. The pattern is very similar for both selected cities, with two maxima for the absolute values mainly caused by the diurnal cycle of urban emissions (mainly morning and evening heavy traffic). As seen in the spatial figures, the temperature impact is almost zero, with a tiny negative peak during evening hours when the absolute values are highest. The wind-induced changes are similar in magnitude throughout the day, with a maximum again during evening hours reaching 0.1–0.2 µg m−3 for the selected cities. The strongest impact is modeled for the turbulence effects, with a very well expressed peak occurring during evening hours (around 18:00). The strong Kv impact determines the cycle of the total impact as well, which is slightly smaller due to the positive impact of the wind changes. These results are similar to the diurnal cycle of PM2.5 values except that the t impact is much stronger in the case of PM2.5. This gives us a hint that the reason for this difference will probably lie in the contribution of secondary aerosol. In the following, we will look at their contribution to the overall final aerosol modifications.
3.2.3 Secondary aerosols
Figure 9 plots the spatial distribution of the urban meteorological forcing on the surface concentration of secondary inorganic aerosols, PSO4, PNO3 and PNH4, as well as of secondary organic aerosols. The urban temperature changes cause a decrease of each aerosol type, while the strongest decrease is modeled for nitrates, up to −1 µg m−3. Sulfates decrease over urban areas by up to 0.3 µg m−3 and ammonium is decreased by up to 0.4–0.5 µg m−3. The smallest decrease is modeled for the SOA. Consequently, from secondary aerosols, nitrates contribute the most to the temperature-induced PM2.5 changes. In the case of the wind impact, there is an evident increase in concentration due to suppressed dilution from sources for all the examined aerosol components. Here again, the strongest increases are modeled for nitrates, up to 0.6–0.8 µg m−3 (especially over western Europe), while for sulfates and ammonium, the change reaches 0.3–0.4 µg m−3. For SOA, being a relatively minor modeled aerosol component, the wind-induced changes encompass very small increases up to 0.015 µg m−3. A more complicated response of surface aerosol concentrations is modeled for changes in the vertical eddy diffusion. In the western part of the domain and over large cities, an increase in the diffusion coefficient leads to a decrease of surface aerosols up to −0.4 µg m−3, especially for sulfates and ammonium. Over eastern Europe, a slight increase in secondary aerosol is modeled, reaching 0.05 µg m−3 over larger areas. The SOA is an exception here, where concentrations are suppressed all over the domain, peaking over urban areas up to −0.04 µg m−3.
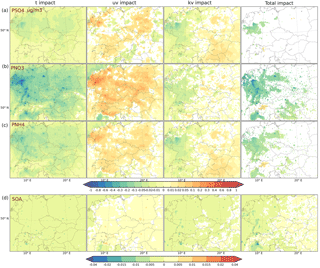
Figure 9Impact of urban canopy meteorological forcing on (a–d) PSO4, PNO3, PNH4 and SOA average 2001–2005 JJA surface concentrations in µg m−3. The columns from left to right correspond to temperature, wind, turbulence and the total impact. Shaded areas represent a statistically significant impact at the 98 % level using the t test.
As it combines both decreases (temperature and turbulence impact) and increases (wind and partly the turbulence impact), the total impact of all meteorological effects often tends to be smaller and the areas of statistical changes occupy a smaller fraction of the domain. This is clearly seen in the case of PSO4, where due to the combined effects of the urban meteorological forcing, statistically significant effects are only modeled for the Ruhr area in northwestern Germany, controlled by the temperature- and turbulence-induced decrease reaching −0.5 µg m−3. For PNO3 the combined meteorological impact manifests itself as a statistically significant decrease of concentrations over large cities, while the highest values (up to −0.8 µg m−3) are modeled over the largest cities. A similar picture is obtained for ammonium, for which statistically significant decreases are modeled for the western part of the domain. In each case above, statistically significant effects are controlled by the combined effect of temperature and turbulence, while the wind-induced increase is too small to counterbalance them. For SOA, the situation is similar: the total impact is dominated by decreases due to temperature- and turbulence-induced decreases, reaching a −0.04 µg m−3 decrease over urban areas.
In order to better understand the modeled response of secondary inorganic aerosol to changed meteorological forcing, we plot the response of the precursor species as well. In Huszar et al. (2018, Fig. 6), the response of NOx to urbanization-induced meteorological changes was presented and increases of species surface concentrations were modeled due to decreased wind up to 2 ppbv. Due to turbulence increase, NOx responded with a decrease up to −4 ppbv. Here, in Fig. 10, the responses of sulfur dioxide (SO2) and ammonia (NH3) surface concentrations are presented as precursors of sulfates and particulate ammonium (ammonium sulfates and ammonium nitrates). For both aerosol types, surface concentrations decrease due to increased urban temperatures, by up to −0.2 to −0.5 ppbv, and this is probably connected to increased dry deposition. Lower urban winds cause reduced horizontal dilution, resulting in higher concentrations: this is seen for both SO2 and NH3 of which concentrations are usually enhanced around cities by up to 2–4 ppbv. However, in the case of sulfur dioxide, some rural areas encounter decreases, probably due to the fact that transport is suppressed to these areas due to reduced wind speeds. The same holds for ammonia; however, the decrease here is very small. Enhanced urban turbulence leads to a clear decrease of SO2 surface concentrations over and near urban areas, by up to −4 ppbv. In the case of NH3, this decrease reaches −1 to −1.5 ppbv. A more diverse picture is obtained for the combined effect of urban meteorological changes, and both decreases and increases are encountered over and around cities between −3 and 3 ppbv (somewhat smaller values for ammonia). This is probably caused by the competing effect of wind-induced increase and decrease due to increased vertical eddy transport. In summary, primary precursors respond to increased urban temperatures, decreased urban winds and increased turbulence by a slight decrease, larger decrease and increase, respectively.
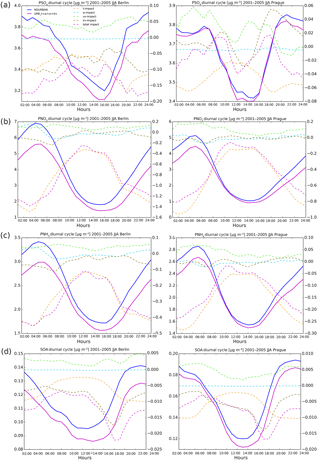
Figure 11Impact of urban canopy meteorological forcing on (a–d) PSO4, PNO3, PNH4 and SOA average diurnal cycle of surface concentrations for the 2001–2005 JJA period for two selected cities (Berlin and Prague) in µg m−3. Bold lines represent the absolute concentrations (left y axis) for the NOURBAN run (blue) and the total impact URB_t+q+uv+Kv run (purple). Dashed lines (right y axis) show the change due to changes of individual meteorological components (temperature – orange, humidity – aquamarine, wind – green, turbulence – olive and total impact – purple).
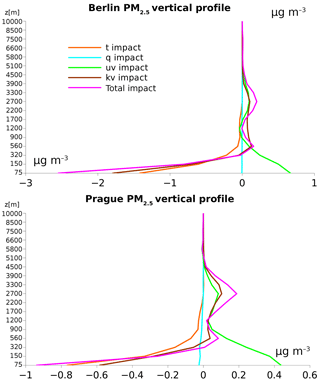
Figure 12Impact of urban canopy meteorological forcing on the vertical profile of PM2.5 concentrations for two selected cities, Berlin and Prague, in µg m−3 averaged over the 2001–2005 JJA period (temperature impact – orange, humidity impact – aquamarine, wind impact – green, turbulence – brown and total impact – purple). Vertical axis shows the layer interface heights in meters for the 18 CTM layers.
The diurnal cycle of the impact of urban meteorological forcing on secondary (in)organic aerosol is shown in Fig. 11 for two selected cities, Berlin and Prague. As the formation of these, beginning with uptake in the water phase and nucleation, is highly temperature-dependent and lower temperatures favor gas–particle partitioning, the absolute values (taken from the NOURBAN and URB_t+q+uv+Kv runs) are lower during daytime, as expected. This behavior is evident from the t impact (orange): it follows the cycle of the urban impact on temperature, being largest during nighttime hours when the UHI is the strongest. It is clearly seen that the temperature impact is the dominant impact for nitrates and ammonium (note the total impact curve in purple). The changes of aerosol concentrations due to modified moisture content are negligible, as already seen for PM2.5. The impact of wind changes follows the spatial result seen in Fig. 9 and is characterized by increases: it is usually lower during daytime, when absolute values are also low compared to nighttime values.
The impact of increased turbulence is evident in the case of SOA, which seems to be the main contributor to the total impact for both cities. It only causes small changes for nitrates and ammonium. In the case of PSO4, however, the two cities differ: while in Berlin, increased vertical mixing removes some sulfates from the surface layer, reducing its concentrations, for Prague, the behavior is more complicated, and concentrations can even increase due to higher vertical mixing. The change is relatively small, however, in the case of Prague, and the concentrations can vary as a function of some secondary nonlinear effects like transport from higher model levels to lower ones due to residual turbulence during nighttime.
In order to obtain an idea of the vertical extent of these effects that take place, in Fig. 12 we plotted the vertical profile of individual impacts on PM2.5 concentrations for Berlin and Prague. As expected, the temperature, turbulence and the total impact reduce surface concentrations while the wind increases them. At around 900–1200 m the changes quickly become very small, and for the temperature effect, almost negligible. At higher levels, the turbulence impact changes sign and shows a strong maximum around 2700 m. The same secondary maximum occurs for the wind impact. Consequently, the combined impact also has this large positive maximum, reaching 0.2–0.3 µg m−3.
The modeled meteorological response due to introduction of urban canopy shows expected features. The impact on temperature and its daily cycle has a very similar magnitude as well as shape to those in numerous previous studies examining European cities (Pichierri et al., 2012; Giannaros et al., 2013; Struzewska and Kaminski, 2012; Sarrat et al., 2006) but similar diurnal variations of the UHI (which is close to the impact of urban land surface on temperature) were obtained for cities over other continents (Gaffin et al., 2008; Ryu et al., 2013a). Due to a notable increase in nighttime temperature and only a slight enhancement of the daytime ones, a strong decrease of diurnal temperature range is modeled, with values comparable to previous works (e.g. Trusilova et al., 2008). The simulated wind speed changes are also consistent with previous model experiments performed over Europe (Struzewska and Kaminski, 2012; Vautard et al., 2013) or over Chinese urban areas (Hou et al., 2013; Zhu et al., 2017). The sudden drop of wind impact to almost zero during evening hours is caused by relatively high downward momentum flux due to enhanced turbulence (Huszar et al., 2018) during the evening transition period (Lapworth, 2003). Concerning the urban impact on the turbulence, our simulated eddy diffusion coefficient and their changes are in line with what was previously modeled over urban areas (Kim et al., 2015) or in general over complex terrain (ENVIRON, 2011), although they are slightly smaller. This is probably caused by the inconsistencies introduced by the diagnostic calculation of vertical diffusivity values rather than directly taking them from the PBL scheme of the driving model used.
Due to the urban canopy, humidity decreased on average in our simulations, which is in line with expectations. Urban areas are covered with materials that have a low evapotranspiration and are covered with minimal vegetation. Further, due to high runoff, the precipitated water is transported by sewer system away from urban areas. In summary, sources of moisture over urban areas are limited compared to rural surfaces with a much higher fraction of vegetation (Richards, 2004). However, during nighttime a slight increase in the absolute humidity is observed in the model. In general, this can be a result of both the increased source of moisture and the decreased sink. The first possible reason can be dismissed. Regarding the reduced sinks of moisture, this is a straight consequence of high urban temperatures. Compared to rural environments, urban areas can hold a larger quantity of moisture as a consequence of the Clausius–Clapeyron equation. In rural areas during the night, the temperature often drops below the dew point, resulting in condensation, which acts as a sink. This does not occur, however, to such an extent in urban areas, where a reduced dew deposition has been observed in correspondence with expectations (Richards and Oke, 2002). Hence, urban nighttime absolute humidity can be slightly higher than the rural one, at least near the surface.
It has been shown that the urban canopy meteorological forcing decreases fine aerosol concentrations, and this decrease is strongest during late afternoon hours. The component analysis of the response revealed that the most important contributor to this change is the enhanced turbulence over cities, which facilitates the removal of both the aerosol itself and also its precursors (as seen for SO2 and NH3 and noted for NO2). The diurnal change caused by all the considered meteorological changes has an almost identical shape to the change caused purely by the turbulence enhancement, especially at high absolute values. Our results confirm the strong connection between aerosol concentration and vertical turbulent transport that has been confirmed already by many authors (e.g. Kim et al., 2015; Zhu et al., 2017). These authors revealed, in line with our results, that urbanization-triggered enhanced turbulence reduces the modeled aerosol concentrations. It is mainly the primary aerosol (organics, black carbon and other fine particulate matter) which has the most pronounced responses to the turbulence changes.
Regarding the wind impact, our expectation has been confirmed – there is a clear relationship between urban wind speeds and air pollution: reduced wind causes reduction in pollutant dispersion, leading to increased concentration near the sources or vice versa. This is in line with the recent findings of Jandaghian and Akbari (2018), who showed that albedo-induced increases in urban wind speeds result in a decrease of PM2.5 concentrations. The wind-induced aerosol increase has a clear diurnal cycle that reflects the cycle of the absolute values: an approximately 2 times higher increase is modeled for nighttime (from early evening to early morning) than for daytime.
The simulated changes due to urban temperature increase are only evident in the case of secondary aerosols. This is expected as temperature strongly controls the gas–particle partitioning and nucleation of aerosols with high temperatures, reducing the tendency to form particles. In this regard, it is not surprising that temperature-induced changes are strong in the case of secondary aerosols and are negligible for primary ones. To support our finding about the temperature-driven decrease of secondary aerosol concentrations, Juda-Rezler et al. (2012) and Dawson et al. (2007) earlier reached the conclusion that secondary inorganic aerosol and SOA included in PM decrease as they shift from the particle to the gas phase with increasing temperature. In our simulations, this is most evident for nitrates, in line with Myhre et al. (2006) and Dawson et al. (2007).
An almost negligible impact on PM2.5 is modeled due to changes in moisture content. It has a slight effect on dry deposition velocities of gases via increasing stomatal and cuticle resistance due to higher relative humidity (Zhang et al., 2003). Further, reduced moisture negatively affects the hydroxyl radical concentrations, reducing the oxidative capacity of the air above urban areas. However, both processes are probably of minor importance as the moisture variations are small.
The simulated impacts on secondary organic aerosol are in line with expectations, although they are very small in magnitude. Higher urban temperatures negatively affect the nucleation rates of organic vapors, leading to the reduced SOA concentrations. Decreased urban winds reduce the dispersion of SOA, leading to a slight increase in condensation. Thirdly, enhanced urban turbulence favors the removal of both SOA and its precursors (semi-volatile hydrocarbons), resulting in lower concentrations, which seems to dominate the overall urban impact. This stands in contrast with inorganic aerosols, for which the urbanization-induced turbulence increase resulted in both a concentration decrease and increase. This can be explained by the different emission ratios of the primary precursor species across the domain. Thus, turbulence impacts them with different magnitudes, and the competition between sulfates, nitrates and ammonium ions leads to different inorganic aerosol responses.
Our results showed that the effects of urban canopy meteorological forcing are getting weaker with increasing height, which is not surprising, as the meteorological changes are often limited to the first few model layers (Huszar et al., 2014). The turbulence here is a different case, and Huszar et al. (2018) showed that it can be significantly perturbed over cities at higher model layers as well (up to 2200 m). This can explain the vertical secondary maximum occurring for the turbulence impact: it transports pollutants from the surface to higher model layers; however, at certain heights the turbulence is no longer affected by the urban areas and aerosol can thus accumulate, increasing the upper level concentrations. For the wind impact, the situation can be similar. Higher surface concentrations result in more PM transported to higher model layers.
An important question is whether the inclusion of urban canopy effects in the driving meteorological fields reduces the simulated aerosol bias. This is not clear from the results. In areas where fine aerosol was overestimated by the model, especially over western Europe, a decrease of PM2.5 reduced the model bias. On the other hand, over eastern Europe, the model error is enhanced as aerosol was underpredicted in our model here. In general, the effort of more realistic representation of different processes in models does not always imply better model performance. However, this should not discourage the improvement of models, and in our case, it is evident that accounting for the urbanization-induced meteorological changes in air quality simulations leads to modified concentrations that cannot be disregarded in future urban air quality oriented studies. Especially the temperature, wind and eddy diffusion fields must be correct and reflect urban conditions.
There are some further documented effects of the urban canopy on meteorology, for example, the urban breeze circulation (Hidalgo et al., 2010), which affects pollutant transport over city scales (Ryu et al., 2013a). Another meteorological phenomenon occurring over urban areas is the impact on convection: the UHI-triggered convergence zone over urban areas favors the development of convection and increases the frequency of extreme precipitation (Zhong et al., 2017), directly influencing the air quality by decreased solar radiation (due to cloud cover) and increased wet removal of pollutants. These effects, however, act over scales that were not resolved in our modeling setup; thus applying higher resolutions for investigating the urbanization–meteorology–air quality interactions is essential in future research.
All data modeled and analyzed in this study are available upon request. Please contact peter.huszar@mff.cuni.cz.
PH and TH designed the basic idea and organized the project team. PH and JK performed the model simulations. MB configured the models and maintained the hardware the experiments were run on. TB and PP prepared the input and validation data. PH wrote the paper with contributions from all other authors.
The authors declare that they have no competing interests.
This work has been funded by the OP-PPR project (Operation Programme Prague
– Growth Pole) CZ.07.1.02/0.0/0.0/16_040/0000383 “URBI PRAGENSI – Urbanization of weather forecast, air quality prediction and climate
scenarios for Prague”, by the PROGRESS Q47/Q16 – Programmes of Charles
University project, by the SVV 2018 project of Charles University and by the UNCE 2040202013
project. We further acknowledge the TNO MEGAPOLI emissions
dataset from the EU-FP7 project MEGAPOLI (http://megapoli.dmi.dk/, last access: 31 August 2018) and the
providers of the AirBase European Air Quality data
(https://www.eea.europa.eu/data-and-maps/data/aqereporting-8, last access: 31 August 2018).
Edited by: Alex B. Guenther
Reviewed by: three anonymous referees
Angevine, W. M., White, A. B., Senff, C. J., Trainer, M., Banta, R. M., and Ayoub, M. A.: Urban-rural contrasts in mixing height and cloudiness over Nashville in 1999, J. Geophys. Res., 108, 4092, https://doi.org/10.1029/2001JD001061, 2003. a
Basara, J. B., Hall Jr., P. K., Schroeder, A. J., Illston, B. G., and Nemunaitis, K. L.: Diurnal cycle of the Oklahoma City urban heat island, J. Geophys. Res., 113, D20109, https://doi.org/10.1029/2008JD010311, 2008. a
Byun, D. W. and Ching, J. K. S.: Science Algorithms of the EPA Model-3 Community Multiscale Air Quality (CMAQ) Modeling System, Office of Research and Development, U.S. EPA, North Carolina, 1999. a, b
Chen, B., Yang, S., Xu, X.D., and Zhang, W.: The impacts of urbanization on air quality over the Pearl River Delta in winter: roles of urban land use and emission distribution, Theor. Appl. Climatol., 117, 29–39, 2014. a
Cheng, Z., Luo, L., Wang, S., Wang, Y., Sharma, S., Shimadera, H., Wang, K., Bressi, M., de Miranda, R. M., Jiang, J., Zhou, W., Fajardo, O., Yan, N., and Hao, J.: Status and characteristics of ambient PM2.5 pollution in global megacities, Environment International, 89–90, 212–221, 2016. a
Civerolo, K., Hogrefe, C., Lynn, B., Rosenthal, J., Ku, J.-Y., Solecki, W., Cox, J., Small, C., Rosenzweig, C., Goldberg, R., Knowlton, K., and Kinney, P.: Estimating the effects of increased urbanization on surface meteorology and ozone concentrations in the New York City metropolitan region, Atmos. Environ., 41, 1803–1818, 2007. a
Dawson, J. P., Adams, P. J., and Pandis, S. N.: Sensitivity of PM2.5 to climate in the Eastern US: a modeling case study, Atmos. Chem. Phys., 7, 4295–4309, https://doi.org/10.5194/acp-7-4295-2007, 2007. a, b
de la Paz, D., Borge, R., and Martilli, A.: Assessment of a high resolution annual WRF-BEP/CMAQ simulation for the urban area of Madrid (Spain), Atmos. Environ., 144, 282–296, 2016. a
Dickinson, R. E., Henderson-Sellers, A., and Kennedy, P.: Biosphere–atmosphere transfer scheme (BATS) version 1 as coupled to the NCAR community climate model, Tech Rep, National Center for Atmospheric Research Tech Note NCAR.TN387 + STR, NCAR, Boulder, CO, 1993. a
Eben, K., Jurus, P., Resler, J., Belda, M., Pelikán, E., Krüger, B. C., and Keder, J.: An ensemble Kalman filter for short-term forecasting of tropospheric ozone concentrations, Q. J. Roy. Meteor. Soc., 131, 3313–3322, https://doi.org/10.1256/qj.05.110, 2005. a
ENVIRON: Dallas-Fort Worth Modeling Support: Improving the Representation of Vertical Mixing Processes in CAMx – Final Report, ENVIRON International Corporation, Novato, California, 2011. a
ENVIRON: CAMx User's Guide Comprehensive Air Quality model with Extentions, version 6.30, available at: http://www.camx.com/ (last access: 31 August 2018), Novato, California, 2016. a
Fallmann, J., Forkel, R., and Emeis, S.: Secondary effects of urban heat island mitigation measures on air quality, Atmos. Environ., 25, 199–211, 2016. a
Feng, J.-M., Wang, Y.-L., and Ma, Z.-G.: Long-term simulation of large-scale urbanization effect on the East Asian monsoon, Clim. Change, 1–13, https://doi.org/10.1007/s10584-013-0885-2, 2013. a
Folberth, G. A., Butler, T. M., Collins, W. J., and Rumbold, S. T.: Megacities and climate change – A brief overview, Environ. Pollut., 203, 235–242, https://doi.org/10.1016/j.envpol.2014.09.004, 2015. a
Gaffin, S. R., Rosenzweig, C., Khanbilvardi, R., Parshall, L., Mahani, S., Glickman, H., Goldberg, R., Blake, R., Slosberg, R. B., and Hillel, D.: Variations in New York City's urban heat island strength over time and space, Theor. Appl. Climatol., 94, 1–11, https://doi.org/10.1007/s00704-007-0368-3, 2008. a, b
Ganbat, G., Baik, J. J., and Ryu, Y. H.: A numerical study of the interactions of urban breeze circulation with mountain slope winds, Theor. Appl. Climatol., 120, 123–135, 2015. a
Giannaros, T. M. and Melas, D.: Study of the urban heat island in a coastal Mediterranean city: the case study of Thessaloniki, Greece, Atmos. Res., 118, 103–120, https://doi.org/10.1016/j.atmosres.2012.06.006, 2012.
Giannaros, T. M., Melas, D., Daglis, I. A., Keramitsoglou, I., and Kourtidis, K.: Numerical study of the urban heat island over Athens (Greece) with the WRF model, Atmos. Environ., 73, 103–111, https://doi.org/10.1016/j.atmosenv.2013.02.055, 2013. a
Giorgi, F., Coppola, E., Solmon, F., Mariotti, L., Sylla, M., Bi, X., Elguindi, N., Diro, G. T., Nair, V., Giuliani, G., Cozzini, S., Guettler, I., O'Brien, T. A., Tawfi, A. B., Shalaby, A., Zakey, A., Steiner, A., Stordal, F., Sloan, L., and Brankovic, C.: RegCM4: model description and preliminary tests over multiple CORDEX domains, Clim. Res., 52, 7–29, 2012. a
Grell, G.: Prognostic evaluation of assumptions used by cumulus parameterizations, Mon. Weather Rev., 121, 764–787, 1993. a
Guenther, A. B., Zimmermann, P. C., Harley, R., Monson, R. K., and Fall, R.: Isoprene and monoterpene emission rate variability: model evaluations and sensitivity analyses, J. Geophys. Res., 98, 12609–12617, 1993. a
Hidalgo, J., Masson, V., and Gimeno, L.: Scaling the Daytime Urban Heat Island and Urban-Breeze Circulation, J. Appl. Meteorol. Clim., 49, 889–901, 2010. a, b
Holtslag, A. A. M., de Bruijn, E. I. F., and Pan, H.-L.: A high resolution air mass transformation model for shortrange weather forecasting, Mon. Weather Rev., 118, 1561–1575, 1990. a, b
Hou, A., Ni, G., Yang, H., and Lei, Z.: Numerical Analysis on the Contribution of Urbanization to Wind Stilling: an Example over the Greater Beijing Metropolitan Area, J. Appl. Meteorol. Clim., 52, 1105–1115, https://doi.org/10.1175/JAMC-D-12-013.1, 2013. a, b, c
Huszar, P., Juda-Rezler, K., Halenka, T., Chervenkov, H, Syrakov, D., Krüger, B.C., Zanis, P., Melas, D., Katragkou, E., Reizer, M., Trapp, W., and Belda, M: Effects of climate change on ozone and particulate matter over Central and Eastern Europe, Clim. Res. 50, 51–68, https://doi.org/10.3354/cr01036, 2011. a
Huszar, P., Miksovsky, J., Pisoft, P., Belda, M., and Halenka, T.: Interactive coupling of a regional climate model and a chemistry transport model: evaluation and preliminary results on ozone and aerosol feedback, Clim. Res., 51, 59–88, https://doi.org/10.3354/cr01054, 2012. a
Huszar, P., Halenka, T., Belda, M., Zak, M., Sindelarova, K., and Miksovsky, J.: Regional climate model assessment of the urban land-surface forcing over central Europe, Atmos. Chem. Phys., 14, 12393–12413, https://doi.org/10.5194/acp-14-12393-2014, 2014. a, b, c, d, e, f
Huszar, P., Belda, M., and Halenka, T.: On the long-term impact of emissions from central European cities on regional air quality, Atmos. Chem. Phys., 16, 1331–1352, https://doi.org/10.5194/acp-16-1331-2016, 2016a. a, b, c, d
Huszár, P., Belda, M., Karlický, J., Pišoft, P., and Halenka, T.: The regional impact of urban emissions on climate over central Europe: present and future emission perspectives, Atmos. Chem. Phys., 16, 12993–13013, https://doi.org/10.5194/acp-16-12993-2016, 2016b. a, b
Huszar, P., Karlický, J., Belda, M., Halenka, T., and Pisoft, P.: The impact of urban canopy meteorological forcing on summer photochemistry, Atmos. Environ., 176, 209–228, https://doi.org/10.1016/j.atmosenv.2017.12.037, 2018. a, b, c, d, e, f, g, h, i, j, k, l, m
Jackson, T. L., Feddema, J. J., Oleson, K. W., Bonan, G. B., and Bauer, J. T.: Parameterization of Urban Characteristics for Global Climate Modeling, Ann. Assoc. Am. Geogr. 100, 848–865, https://doi.org/10.1080/00045608.2010.497328 , 2010. a
Jandaghian, Z. and Akbari, H.: The Effect of Increasing Surface Albedo on Urban Climate and Air Quality: A Detailed Study for Sacramento, Houston, and Chicago, Climate, 6, 19, https://doi.org/10.3390/cli6020019, 2018. a
Juda-Rezler, K., Reizer, M., Huszar, P., Krueger, B., Zanis, P., Syrakov, D., Katragkou, E., Trapp, W., Melas, D., Chervenkov, H., Tegoulias, I., and Halenka, T.: Modelling the effects of climate change on air quality over central and Eastern Europe: concept, evaluation and projections, Clim. Res., 53, 179–203, https://doi.org/10.3354/cr01072, 2012. a, b
Karlický, J., Huszár, P., and Halenka, T.: Validation of gas phase chemistry in the WRF-Chem model over Europe, Adv. Sci. Res., 14, 181–186, https://doi.org/10.5194/asr-14-181-2017, 2017. a
Karlický, J., Huszár, P., Halenka, T., Belda, M., Žák, M., Pišoft, P., and Mikšovský, J.: Multi-model comparison of urban heat island modelling approaches, Atmos. Chem. Phys., 18, 10655–10674, https://doi.org/10.5194/acp-18-10655-2018, 2018. a, b
Kastner-Klein, P., Fedorovich, E., and Rotach, M. W.: A wind tunnel study of organised and turbulent air motions in urban street canyons, J. Wind Eng. Ind. Aerod., 89, 849–861, 2001. a
Katragkou, E., Zanis, P., Kioutsioukis, I., Tegoulias, I., Melas, D., Kruger, B., and Coppola, C. E.: Future climate change impacts on summer surface ozone from regional climate-air quality simulations over Europe, J. Geophys. Res., 116, D22307, https://doi.org/10.1029/2011JD015899, 2011. a
Kiehl, J., Hack, J., Bonan, G., Boville, B., Breigleb, B., Williamson, D., and Rasch, P.: Description of the NCAR Community Climate Model (CCM3), National Center for Atmospheric Research Tech Note NCAR/TN-420 + STR, NCAR, Boulder, CO, 1996. a
Kim, Y, Sartelet, K., Raut, J.-Ch., and Chazette, P.: Influence of an urban canopy model and PBL schemes on vertical mixing for air quality modeling over Greater Paris, Atmos. Environ., 107, 289–306, https://doi.org/10.1016/j.atmosenv.2015.02.011, 2015 a, b, c
Kuenen, J., Denier van der Gon, H., Visschedijk, A., van der Brugh, H., Finardi, S., Radice, P., d'Allura, A., Beevers, S., Theloke, J., Uzbasich, M., Honor, C., and Perrussel, O.: A Base Year (2005) MEGAPOLI European Gridded Emission Inventory (Final Version), Deliverable D1.6, MEGAPOLI Scientific Report 10-17, MEGAPOLI-20-REP-2010-10, TNO Built Environment and Geosciences, Utrecht, the Netherlands, p. 39, 2010. a
Květoň, V. and Žák, M.: New climate atlas of Czechia, Stud. Geophys. Geod., 51, 345–349 , 2007. a
Lapworth, A.: Factors determining the decrease in surface wind speed following the evening transition, Q. J. Roy. Meteor. Soc., 129, 1945–1968, https://doi.org/10.1256/qj.02.163, 2003. a
Lawrence, D. M., Oleson, K. W., Flanner, M. G., Thornton, P. E., Swenson, S. C., Lawrence, P. J., Zeng, X., Yang, Z.-L., Levis, S., Sakaguchi, K., Bonan, G. B., and Slater, A.G.: Parameterization improvements and functional and structural advances in version 4 of the Community Land Model, J. Adv. Model. Earth Sys., 3, 27, https://doi.org/10.1029/2011MS000045, 2011. a
Lee, S.-H., Kim, S.-W., Angevine, W. M., Bianco, L., McKeen, S. A., Senff, C. J., Trainer, M., Tucker, S. C., and Zamora, R. J.: Evaluation of urban surface parameterizations in the WRF model using measurements during the Texas Air Quality Study 2006 field campaign, Atmos. Chem. Phys., 11, 2127–2143, https://doi.org/10.5194/acp-11-2127-2011, 2011. a, b
Li, M. M., Wang, T. J., Xie, M., Zhuang, B. L., Li, S., Han, Y., Song, Y., and Cheng, N. L.: Improved meteorology and ozone air quality simulations using MODIS land surface parameters in the Yangtze River Delta urban cluster, China, J. Geophys. Res.-Atmos., 122, 3116–3140, https://doi.org/10.1002/2016JD026182, 2017. a
Li, M., Wang, T., Xie, M., Zhuang, B., Li, S., Han, Y., and Cheng, N.: Modeling of urban heat island and its impacts on thermal circulations in the Beijing-Tianjin-Hebei region, China, Theor. Appl. Climatol., 128, 999–1013, 2017b. a
Liao, J., Wang, T., Wang, X., Xie, M., Jiang, Z., Huang, X., and Zhu, J.: Impacts of different urban canopy schemes in WRF/Chem on regional climate and air quality in Yangtze River Delta, China, Atmos. Res., 145–146, 226–243, https://doi.org/10.1016/j.atmosres.2014.04.005, 2014. a
Markakis, K., Valari, M., Perrussel, O., Sanchez, O., and Honore, C.: Climate-forced air-quality modeling at the urban scale: sensitivity to model resolution, emissions and meteorology, Atmos. Chem. Phys., 15, 7703–7723, https://doi.org/10.5194/acp-15-7703-2015, 2015. a
Martilli, A., Roulet, Y.-A., Junier, M., Kirchner, F., Rotach, M. W., and Clappier, A.: On the impact of urban surface exchange parameterisations on air quality simulations: the Athens case, Atmos. Environ., 37, 4217–4231, https://doi.org/10.1016/S1352-2310(03)00564-8, 2003. a
Masson, V., Gomes, L., Pigeon, G., Liousse, C., Pont, V., Lagouarde, J.-P., Voogt, J., Salmond, J., Oke, T. R., Hidalgo, J., Legain, D., Garrouste, O., Lac, C., Connan, O., Briottet, X., Lachérade, S., and Tulet, P.: The Canopy and Aerosol Particles Interactions in TOulouse Urban Layer (CAPITOUL) experiment, Meteorol. Atmos. Phys., 102, 135, https://doi.org/10.1007/s00703-008-0289-4, 2008. a, b
Myhre, G., Grini, A., and Metzger, S.: Modelling of nitrate and ammonium-containing aerosols in presence of sea salt, Atmos. Chem. Phys., 6, 4809–4821, https://doi.org/10.5194/acp-6-4809-2006, 2006. a
Nenes, A., Pandis, S. N., and Pilinis, C.: ISORROPIA: a new thermodynamic equilibrium model for multiphase multicomponent inorganic aerosols, Aquat. Geochem., 4, 123–152, 1998. a
O'Brien, J. J.: A note on the vertical structure of the eddy exchange coefficient in the planetary boundary layer, J. Atmos. Sci., 27, 1213–1215, 1970. a
Oke, T. R.: The energetic basis of the urban heat island, Q. J. Roy. Meteor. Soc., 108, 1–24, https://doi.org/10.1002/qj.49710845502, 1982. a
Oleson, K. W., Bonan, G. B., Feddema, J., Vertenstein, M., and Grimmond, C. S. B.: An urban parameterization for a global climate model. 1. Formulation and evaluation for two cities, J. Appl. Meteorol. Clim., 47,1038–1060, 2008. a
Oleson, K. W., Bonan, G.B., Feddema, J., and Vertenstein, M.: An urban parameterization for a global climate model. 2. Sensitivity to input parameters and the simulated urban heat island in offline simulations, J. Appl. Meteorol. Clim., 47, 1061–1076, 2008. a
Oleson, K., Lawrence, D. M., Bonan, G. B., Drewniak, B., Huang, M., Koven, C. D., Levis, S., Li, F., Riley, W. J., Subin, Z. M., Swenson, S. C., Thornton, P. E., Bozbiyik, A., Fisher, R., Heald, C. L., Kluzek, E., Lamarque, J.-F., Lawrence, P. J., Leung, L. R., Lipscomb, W., Muszala, S., Ricciuto, D. M., Sacks, W., Sun, Y., Tang, J., and Yang, Z.-L.: Technical Description of version 4.5 of the Community Land Model (CLM), NCAR Technical Note NCAR/TN-503+STR, Boulder, Colorado, 420 pp., 2013. a
Pal, J. S., Small, E. E., and Eltahir, E. A. B.: Simulation of regional scale water and energy budgets: representation of subgrid cloud and precipitation processes within RegCM, J. Geophys. Res.-Atmos., 105, 29579–29594, 2000. a
Pichierri, M., Bonafoni, S., and Biondi, R.: Satellite air temperature estimation for monitoring the canopy layer heat island of Milan, Remote Sens. Environ., 127, 130–138, https://doi.org/10.1016/j.rse.2012.08.025, 2012. a
Richards, K.: Observation and simulation of dew in rural and urban environments, Prog. Phys. Geogr., 28, 76–94, 2004. a, b
Richards, K. and Oke, T. R.: Validation and results of a scale model of dew deposition in urban environments, Int. J. Climatol., 22, 1915–1933, 2002. a
Roth, M.: Review of atmospheric turbulence over cities, Q. J. Roy. Meteor. Soc., 126, 941–990, 2000. a
Rozoff, C. M., Cotton, W. R., and Adegoke, J. O.: Simulation of St. Louis, Missouri, land use impacts on thunderstorms, J. Appl. Meteorol., 42, 716–738, 2003. a
Ryu, Y.-H., Baik, J.-J., Kwak, K.-H., Kim, S., and Moon, N.: Impacts of urban land-surface forcing on ozone air quality in the Seoul metropolitan area, Atmos. Chem. Phys., 13, 2177–2194, https://doi.org/10.5194/acp-13-2177-2013,, 2013a. a, b, c
Ryu, Y.-H., Baik, J.-J., and Lee, S.-H.: Effects of anthropogenic heat on ozone air quality in a megacity, Atmos. Environ., 80, 20–30, https://doi.org/10.1016/j.atmosenv.2013.07.053, 2013b. a, b
Sarrat, C., Lemonsu, A., Masson, V., and Guedalia, D.: Impact of urban heat island on regional atmospheric pollution, Atmos. Environ., 40, 1743–1758, 2006. a, b
Schaap, M., van Loon, M., ten Brink, H. M., Dentener, F. J., and Builtjes, P. J. H.: Secondary inorganic aerosol simulations for Europe with special attention to nitrate, Atmos. Chem. Phys., 4, 857–874, https://doi.org/10.5194/acp-4-857-2004, 2004. a
Seinfeld, J. H. and Pandis, S. N.: Atmospheric Chemistry and Physics: From Air Pollution to Climate Change, J. Wiley, New York, 1998. a
Simmons, A. J., Willett, K. M., Jones, P. D., Thorne, P. W., and Dee, D. P.: Low-frequency variations in surface atmospheric humidity, temperature and precipitation: inferences from reanalyses and monthly gridded observational datasets, J. Geophys. Res., 115, D01110, https://doi.org/10.1029/2009JD012442, 2010. a
Strader, R. Lurmann, F., and Pandis, S. N.: Evaluation of secondary organic aerosol formation in winter, Atmos. Environ., 33., 4849–4863, 1999. a
Struzewska, J. and Kaminski, J. W.: Impact of urban parameterization on high resolution air quality forecast with the GEM – AQ model, Atmos. Chem. Phys., 12, 10387–10404, https://doi.org/10.5194/acp-12-10387-2012, 2012. a, b, c, d
Stutz, J., Alicke, B., Ackermann, R., Geyer, A., White, A., and Williams, E.: Vertical profiles of NO3, N2O5, O3, and NOx in the nocturnal boundary layer: 1. Observations during the Texas Air Quality Study 2000, J. Geophys. Res., 109, D12306, https://doi.org/10.1029/2003JD004209, 2004. a
Tao, Z., Santanello, J. A., Chin, M., Zhou, S., Tan, Q., Kemp, E. M., and Peters-Lidard, C. D.: Effect of land cover on atmospheric processes and air quality over the continental United States – a NASA Unified WRF (NU-WRF) model study, Atmos. Chem. Phys., 13, 6207–6226, https://doi.org/10.5194/acp-13-6207-2013, 2013. a
Tie, X., Brasseur, G., and Ying, Z.: Impact of model resolution on chemical ozone formation in Mexico City: application of the WRF-Chem model, Atmos. Chem. Phys., 10, 8983–8995, https://doi.org/10.5194/acp-10-8983-2010, 2010. a
Trusilova, K., Jung, M., Churkina, G., Karstens, U., Heimann, M., and Claussen, M.: Urbanization Impacts on the Climate in Europe: Numerical Experiments by the PSU–NCAR Mesoscale Model (MM5), J. Appl. Meteorol. Clim., 47, 1442–1455, https://doi.org/10.1175/2007JAMC1624.1, 2008. a, b
Varghese, S., et al. Varghesea, S., Langmann, B., Ceburnis, D., and O'Dowda, C. D.: Effect of horizontal resolution on meteorology and air-quality prediction with a regional scale model, Atmos. Environ., 101, 574–594, 2011. a
Vautard, R., Gobiet, A., Jacob, D., Belda, M., Colette, A., Deque, M., Fernandez, J., Garcia-Diez, M., Goergen, K., Guttler, I., Halenka, T., Karacostas, T., Katragkou, E., Keuler, K., Kotlarski, S., Mayer, S., van Meijgaard, E., Nikulin, G., Patarcic, M., Scinocca, J., Sobolowski, S., Suklitsch, M., Teichmann, C., Warrach-Sagi, K., Wulfmeyer, V., and Yiou, P.: The simulation of European heat waves from an ensemble of regional climate models within the EURO-CORDEX project, Clim. Dynam., 41, 2555–2575, https://doi.org/10.1007/s00382-013-1714-z, 2013. a, b
Wang, X. M., Lin, W. S., Yang, L. M., Deng, R. R., and Lin, H.: A numerical study of influences of urban land-use change on ozone distribution over the Pearl River Delta region, China, Tellus B, 59, 633–641, 2007. a
Wang, X., Chen, F., Wu, Z., Zhang, M., Tewari, M., Guenther, A., and Wiedinmyer, C.: Impacts of weather conditions modified by urban expansion on surface ozone: Comparison between the Pearl River Delta and Yangtze River Delta regions, Adv. Atmos. Sci., 26, 962–972, 2009. a
Wang, X., Yang, M., and Pang, G.: Influences of Two Land-Surface Schemes on RegCM4 Precipitation Simulations over the Tibetan Plateau, Adv. Meteorol., 2015, 106891, https://doi.org/10.1155/2015/106891, 2015. a
Winiwarter, W. and Zueger, J.: Pannonisches Ozonprojekt, Teilprojekt Emissionen, Endbericht, Report OEFZS-A-3817, Austrian Research Center, Seibersdorf, 1996. a
Wouters, H., De Ridder, K., Demuzere, M., Lauwaet, D., and van Lipzig, N. P. M.: The diurnal evolution of the urban heat island of Paris: a model-based case study during Summer 2006, Atmos. Chem. Phys., 13, 8525–8541, https://doi.org/10.5194/acp-13-8525-2013, 2013. a
Xie, M., Zhu, K., Wang, T., Feng, W., Gao, D., Li, M., Li, S., Zhuang, B., Han, Y., Chen, P., and Liao, J.: Changes in regional meteorology induced by anthropogenic heat and their impacts on air quality in South China, Atmos. Chem. Phys., 16, 15011–15031, https://doi.org/10.5194/acp-16-15011-2016, 2016. 2016a. a
Xie, M., Liao, J., Wang, T., Zhu, K., Zhuang, B., Han, Y., Li, M., and Li, S.: Modeling of the anthropogenic heat flux and its effect on regional meteorology and air quality over the Yangtze River Delta region, China, Atmos. Chem. Phys., 16, 6071–6089, https://doi.org/10.5194/acp-16-6071-2016, 2016b. a
Yarwood, G., Rao, S., Yocke, M., and Whitten, G. Z.: Updates to the Carbon Bond chemical mechanism: CB05, Final Report prepared for US EPA, available at: http://www.camx.com/publ/pdfs/CB05_Final_Report_120805.pdf (last access: 31 August 2018), Novato, NC, USA, 2005. a
Zhang, L., Brook, J. R., and Vet, R.: A revised parameterization for gaseous dry deposition in air-quality models, Atmos. Chem. Phys., 3, 2067–2082, https://doi.org/10.5194/acp-3-2067-2003, 2003. a
Zhong, S., Qian, Y., Zhao, C., Leung, R., Wang, H., Yang, B., Fan, J., Yan, H., Yang, X.-Q., and Liu, D.: Urbanization-induced urban heat island and aerosol effects on climate extremes in the Yangtze River Delta region of China, Atmos. Chem. Phys., 17, 5439–5457, https://doi.org/10.5194/acp-17-5439-2017, 2017. a
Zhong, S., Qian, Y., Sarangi, C., Zhao, C., Leung, R., Wang, H., Yan, H., Yang, T., and Yang, B.: Urbanization effect on winter haze in the Yangtze River Delta region of China, Geophys. Res. Letters, 45, 6710–6718, https://doi.org/10.1029/2018GL077239, 2018.
Zhu, K., Xie, M., Wang, T., Cai, J., Li, S., and Feng, W.: A modeling study on the effect of urban land surface forcing to regional meteorology and air quality over South China, Atmos. Environ., 152, 389–404, https://doi.org/10.1016/j.atmosenv.2016.12.053, 2017. a, b, c, d