the Creative Commons Attribution 3.0 License.
the Creative Commons Attribution 3.0 License.
Effects of model resolution and parameterizations on the simulations of clouds, precipitation, and their interactions with aerosols
Zhanqing Li
Yuwei Zhang
Hyelim Yoo
Seungbum Kim
Byung-Gon Kim
Yong-Sang Choi
Jungbin Mok
Junshik Um
Kyoung Ock Choi
Danhong Dong
This study investigates the roles played by model resolution and microphysics parameterizations in the well-known uncertainties or errors in simulations of clouds, precipitation, and their interactions with aerosols by the numerical weather prediction (NWP) models. For this investigation, we used cloud-system-resolving model (CSRM) simulations as benchmark simulations that adopt high-resolution and full-fledged microphysical processes. These simulations were evaluated against observations, and this evaluation demonstrated that the CSRM simulations can function as benchmark simulations. Comparisons between the CSRM simulations and the simulations at the coarse resolutions that are generally adopted by current NWP models indicate that the use of coarse resolutions as in the NWP models can lower not only updrafts and other cloud variables (e.g., cloud mass, condensation, deposition, and evaporation) but also their sensitivity to increasing aerosol concentration. The parameterization of the saturation process plays an important role in the sensitivity of cloud variables to aerosol concentrations. while the parameterization of the sedimentation process has a substantial impact on how cloud variables are distributed vertically. The variation in cloud variables with resolution is much greater than what happens with varying microphysics parameterizations, which suggests that the uncertainties in the NWP simulations are associated with resolution much more than microphysics parameterizations.
- Article
(9628 KB) - Full-text XML
- BibTeX
- EndNote
It is well known that there are errors in NWP simulations of the water and energy cycles, and the treatment of clouds and precipitation and their interactions with aerosols in the NWP models is likely a major source of those errors (Sundqvist et al., 1989; Randall et al., 2006; Seifert et al., 2012). Thus, the NWP community has recognized that the accurate representation of clouds, precipitation, and cloud–aerosol–precipitation interaction (CAPI) is important for the improvement of NWP models, and some of these models have started to improve the representation by considering CAPI (Morcrette et al., 2011; Dipu et al., 2017).
CAPI may not have a substantial impact on the total precipitation amount, but it does affect the temporal and spatial variabilities of precipitation (Li et al., 2011; van den Heever et al., 2011; Seifert et al., 2012; Lee and Feingold, 2013; Fan et al., 2013; Lee et al., 2014), the importance of which increases as the temporal and/or spatial scales of the forecast decrease. The distribution of extreme precipitation events such as droughts and floods, closely linked to the spatiotemporal variability, has important social and economic implications.
In recent years, resolution in NWP models has increased to the point that traditional cumulus parameterization schemes may no longer work properly. Motivated by this, scale-aware cumulus parameterization schemes (e.g., Bogenschutz and Krueger, 2013; Thayer-Calder et al., 2015; Griffin and Larson, 2016) are being implemented into these models with different resolutions for better representation of clouds and precipitation. These scale-aware schemes, which represent sub-grid-scale dynamic processes (e.g., cloud-scale updrafts and downdrafts) that are associated with cloud convection as in traditional cumulus parameterizations, are designed to be applied to the increased resolution in the NWP models.
The uncertainties or the errors in simulations of clouds, precipitation, and CAPI in the NWP models may be incurred both from microphysics parameterizations and from model resolution. The implementation of two-moment cloud microphysics (e.g., Morrison and Gettelman, 2008; Morrison et al., 2009) and scale-aware schemes is intended to reduce these uncertainties. It is important to first understand and quantify the uncertainties associated with the two-moment scheme and how model resolution creates the uncertainties; it is also important to understand the relative significance between the uncertainties associated with the two-moment scheme and those created by resolution. This understanding and quantification can provide us with a guideline on how to represent microphysics in two-moment schemes and sub-grid processes in scale-aware schemes for the efficient reduction of the uncertainties in NWP models. Note that the representation of sub-grid processes requires information on the contribution of resolution to the uncertainties, and in this study, we focus on the two-moment scheme developed by Morrison and Gettelman (2008) and Morrison et al. (2009), which is referred to as the MG scheme henceforth.
Fan et al. (2012) and Khain et al. (2015) have shown that the parameterizations of three key microphysical processes (i.e., saturation, collection, and sedimentation) in microphysical schemes act as a main source of errors in the simulation of clouds, precipitation, and CAPI. We try to identify and quantify the errors or the uncertainties through comparisons between simulations with parameterizations of the three key processes in the MG scheme and the CSRM simulations with full-fledged microphysical processes. Regarding the understanding of the uncertainties arising from the choice of resolution, we also perform comparisons between high-resolution CSRM simulations and low-resolution simulations. This helps gain an understanding of how the microphysical representation and coarse resolution in NWP models contribute to the uncertainties in their simulations of clouds and precipitation by accounting for CAPI. Here, the CSRM simulations act as benchmark simulations by representing microphysical processes with high-level sophistication and by resolving cloud-scale physical and dynamic processes with a high resolution.
The Advanced Research Weather Research and Forecasting (ARW) model, a non-hydrostatic compressible model, is the CSRM selected for use in this study. A fifth-order monotonic advection scheme is used for the advection of cloud variables (Wang et al., 2009). The ARW model considers radiation processes by adopting the Rapid Radiation Transfer Model for General Circulation Models (RRTMG; Fouquart and Bonnel, 1980; Mlawer et al., 1997). The effective sizes of hydrometeors, which vary with varying aerosol properties, are calculated in a microphysics scheme that is adopted by this study and described below; the calculated sizes are transferred to the RRTMG. Then, the effects of the effective sizes of hydrometeors on radiation are calculated in the RRTMG. The ARW model considers sub-grid-scale turbulence by adopting 1.5-order turbulence kinetic energy closure (Basu et al., 1998).
For an assessment of the uncertainties in the MG scheme, which is a type of bulk scheme, we need to use microphysics schemes that are much more sophisticated than the MG scheme. Through extensive comparisons between various types of bin schemes and bulk schemes, Fan et al. (2012) and Khain et al. (2015) have concluded that the use of bin schemes or bin–bulk schemes is desirable for reasonable simulations of clouds, precipitation, and their interactions with aerosols. This is because these schemes do not use a saturation adjustment, a mass-weight mean terminal velocity, or constant collection efficiencies that have been used in bulk schemes. Instead, bin schemes use predicted supersaturation levels and terminal velocities and collection efficiencies that vary with the sizes of hydrometeors. Based on the work by Fan et al. (2012) and Khain et al. (2015), this study considers bin schemes to be full-fledged microphysics schemes against which the uncertainties in the MG scheme can be assessed. Hence, a bin scheme is adopted in the CSRM used here.
The bin scheme adopted by the CSRM is based on the Hebrew University Cloud Model described by Khain and Lynn (2009). The bin scheme solves a system of kinetic equations for the size distribution functions of water drops, ice crystals (plate, columnar, and branch types), snow aggregates, graupel and hail, and cloud condensation nuclei. Each size distribution is represented by 33 mass-doubling bins; i.e., the mass of a particle mk in the kth bin is .
As stated in the Introduction, this study focuses on the uncertainties or errors in the simulations of clouds, precipitation, and CAPI. This means that the examination of the uncertainties in the simulations of aerosol physics and chemistry is out of the scope of this study. Instead of simulating aerosol physics and chemistry explicitly, initial aerosol physical and chemical properties (i.e., aerosol chemical composition and size distribution) are prescribed. Then, aerosol size distribution (or aerosol number concentration in each size bin) evolves only through cloud processes (as described below) but not through aerosol physical and chemical processes. During the evolution, the prescribed aerosol composition is assumed not to vary.
In this study, it is assumed that aerosol particles are composed of ammonium sulfate. The aerosol size distribution evolves prognostically with sinks and sources, which include advection, droplet nucleation, and aerosol regeneration from droplet evaporation (Fan et al., 2009). Aerosol activation is calculated according to the Köhler theory; i.e., aerosol particles with radii exceeding the critical value at a grid point are activated to become droplets based on predicted supersaturation, and the corresponding bins of the aerosol spectra are emptied. After activation, the aerosol mass is transported within hydrometeors by collision–coalescence and removed from the atmosphere once hydrometeors that contain aerosols reach the surface. Aerosol particles return to the atmosphere upon evaporation or the sublimation of the hydrometeors that contain them.
3.1 The Seoul case
A mesoscale convective system (MCS) was observed over Seoul, Korea (37.57∘ N, 126.97∘ E; 09:00 local solar time (LST) on 26 July 2011–09:00 LST 27 July 2011). This case, referred to as the Seoul case, involved heavy rainfall with a maximum precipitation rate of ∼150 mm h−1. This heavy rainfall caused flash floods and landslides on a mountain at the southern flank of the city, leading to the deaths of 60 people.
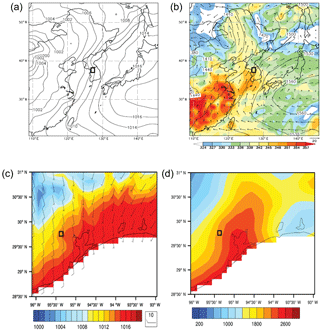
Figure 1(a) Sea-level pressure (hPa) and (b) 850 hPa wind (m s−1; arrows) geopotential height (m; contours) and equivalent potential temperature (K; shaded) at 09:00 LST on 26 July 2011 over northeast Asia. The rectangles in the Korean Peninsula in panels (a) and (b) mark the center of Seoul. (c) Sea-level pressure (hPa; shaded) and wind at 10 (m s−1; barbs) and (d) convective available potential energy (J kg−1) at 05:00 LST on 18 July 2013 in and around Houston. The rectangles in panels (c) and (d) mark the center of Houston.
At 09:00 LST 26 July 2011, favorable synoptic-scale features for the development of heavy rainfall over Seoul were observed. The western Pacific subtropical high (WPSH) was located over the southeast of Korea and Japan, and there was a low-pressure trough over north China (Fig. 1a). Low-level jets between the flank of the WPSH and the low-pressure system brought warm, moist air from the Yellow Sea to the Korean Peninsula (Fig. 1b). The transport of warm and moist air by the southwesterly low-level jet is an important condition for the development of heavy rainfall events over Seoul (Hwang and Lee, 1993; Sun and Lee, 2002).
3.2 The Houston case
An MCS was observed over Houston, Texas (29.42∘ N, 94.45∘ W; 07:00 LST 18 July 2013–04:00 LST 19 July 2013). The Houston case involved moderate rainfall with a maximum precipitation rate of ∼50 mm h−1.
At 05:00 LST, 2 hours before the initiation of convection, the low-level wind in and around Houston was southerly (Fig. 1c), favoring the transport of water vapor from the Gulf of Mexico to the Houston area. Associated with this, the environmental convective available potential energy (CAPE) (Fig. 1d) in and around Houston along the coastline was high (as represented by red areas in Fig. 1d). The high CAPE provided a favorable condition for the development of the MCS.
Using the ARW model and its bin scheme, a three-dimensional CSRM simulation of the observed MCS was performed over the MCS period for each of the cases.
Initial and boundary conditions, which represent the synoptic features, for the control run are derived from the National Centers for Environmental Prediction Global Forecast System final (FNL) analysis. The open lateral boundary condition is adopted in the control run. This enables the advection of the synoptic condition into and out of a domain in the CSRM simulations to occur through the boundary of the domain. All experiments employ a prognostic surface skin temperature scheme (Zeng and Beljaars, 2005) and a revised roughness length formulation (Donelan et al., 2004).
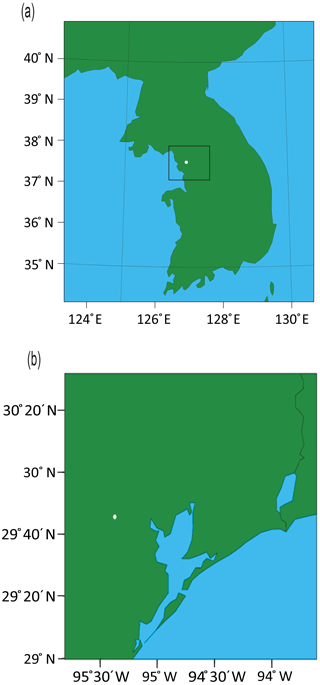
Figure 2(a) The domain (marked by the rectangle) used in simulations for the Seoul case. The small white circle marks the center of Seoul. (b) The domain used in simulations for the Houston case. The small white circle marks the center of Houston.
The control run for each of the cases consists of a domain with a Lambert conformal map projection. The domain is marked by the rectangle for the Seoul case in Fig. 2a and the domain for the Houston case is shown in Fig. 2b. The control run for the Seoul case is referred to as “the control-Seoul run” and the control run for the Houston case is referred to as “the control-Houston run” henceforth. The domain for the Seoul (Houston) case covers the Seoul (Houston) area; to resolve cloud-scale processes, a 500 m horizontal resolution is applied to the domain. The domain has 41 vertical layers with a vertical resolution ranging from 70 m near the surface to 800 m at the model top (∼50 hPa). Note that the cumulus parameterization scheme is not used in this domain where cloud-scale convection and associated convective rainfall generation are assumed to be explicitly resolved. Based on observations, the aerosol concentration at the surface at the first time step is set at 5500 (1500) cm−3 for the Seoul (Houston) case. Above the top of the planetary boundary layer around 2 km, the aerosol concentration is reduced exponentially.
To examine and isolate CAPI, i.e., the effect of increasing the loading of aerosols on clouds and precipitation, the control run is repeated with the aerosol concentration at the first time step reduced by a factor of 10. This factor is based on observations showing that reduction in aerosol loading between polluted days and clean days is generally tenfold over Seoul and Houston (Lance et al., 2009; Kim et al., 2014). This simulation is referred to as the low-aerosol-Seoul run for the Seoul case and the low-aerosol-Houston run for the Houston case. Since the control-Seoul run and the control-Houston run involve higher aerosol concentrations than the low-aerosol-Seoul run and the low-aerosol-Houston run, respectively, for naming purposes the control-Seoul run and the control-Houston run are also referred to as the high-aerosol-Seoul run and the high-aerosol-Houston run, respectively.
In addition to the simulations described above, more simulations were performed to fulfill the goals of this study (Table 1). Details of those simulations are given in the following sections.
5.1 Test on the effects of resolution on the simulations of clouds, precipitation, and CAPI
5.1.1 Cloud mass
To test the effects of resolution on the simulations of clouds, precipitation, and their interactions with aerosols, we repeat the standard CSRM runs at the 500 m resolution (i.e., the high-aerosol-Seoul run, the low-aerosol-Seoul run, the high-aerosol-Houston run, and the low-aerosol-Houston run) by using 15 and 35 km resolutions instead. These resolutions are similar to those generally adopted by current NWP models. To isolate the effects of resolution on the simulations of clouds, precipitation, and their interactions with aerosols, only resolution varies among the CSRM runs at the fine resolution and the repeated runs at the coarse resolutions here, and these runs have an identical model setup except for resolution. For the identical setup, as an example, we do not apply the convection parameterizations (e.g., cumulus parameterizations) to the repeated runs, since the convection parameterizations are not applied to the CSRM runs. Hence, cloud variables (e.g., the updraft speed) are not diagnosed by convection parameterizations but predicted in both the CSRM runs and the repeated runs. With the identical setup except for resolution, the comparisons between the CSRM simulations and the repeated simulations can isolate the pure effects of the use of coarse resolution on clouds, precipitation, and their interactions with aerosol.
The repeated simulations at the 15 km resolution are referred to as the high-aerosol-15-Seoul run, the low-aerosol-15-Seoul run, the high-aerosol-15-Houston run, and the low-aerosol-15-Houston run, while the repeated simulations at the 35 km resolution are referred to as the high-aerosol-35-Seoul run, the low-aerosol-35-Seoul run, the high-aerosol-35-Houston run, and the low-aerosol-35-Houston run. In this study, simulations with names that include “high-aerosol” represent the polluted scenario, while those with names including “low-aerosol” represent the clean scenario. In the following, we describe results from the standard and repeated simulations. For the Houston case, no clouds form at the 35 km resolution, so the description of results is only for results at the 15 km resolution.
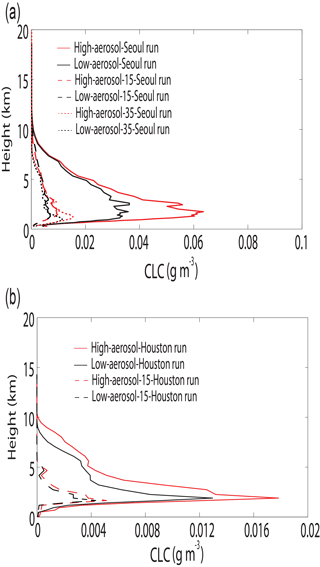
Figure 3Vertical distributions of the time- and domain-averaged cloud liquid content (CLC) for (a) the Seoul case and (b) the Houston case. Solid lines represent simulations at the 500 m resolution, while dashed lines represent those at the 15 km resolution. Dotted lines represent simulations at the 35 km resolution.
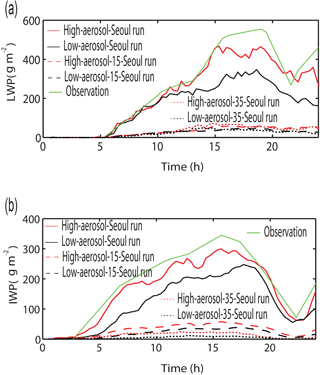
Figure 5Time series of the domain-averaged (a) liquid water path (LWP) and (b) ice water path (IWP) for the Seoul case. Solid lines represent simulations at the 500 m resolution, while dashed and dotted lines represent those at the 15 and 35 km resolutions, respectively. Green lines represent observed LWP and IWP.
Figure 3a and b show the vertical distributions of the time- and domain-averaged cloud liquid content (CLC) in the simulations for the Seoul case and the Houston case, respectively. Figure 4a and b show the vertical distributions of the time- and domain-averaged cloud ice content (CIC) in the simulations for the Seoul case and the Houston case, respectively. There are increases in the cloud mass (represented by CLC and CIC) with increasing aerosol concentration between the polluted scenario and the clean scenario, not only for both the Seoul and Houston cases but also at all resolutions considered. The cloud mass is substantially less at the 15 and 35 km resolutions compared to that in the simulations at the 500 m resolution. In addition, increases in the cloud mass with increasing aerosol concentration are reduced substantially as resolution coarsens. At the 500 m resolution, on average, there is about a ∼30–50 % increase in cloud mass, while at the 15 or 35 km resolutions there is only a ∼2–5 % increase in cloud mass in both cases.
Figure 5a and b show the time series of the domain-averaged liquid water path (LWP) and ice water path (IWP) for the Seoul case, while Fig. 6a and b show the same for the Houston case. Note that LWP and IWP are the vertical integrals of CLC and CIC, respectively. Consequently, the same behavior as CLC and CIC is seen: there are increases in LWP and IWP with increasing aerosol concentrations between the polluted and clean scenarios at all resolutions, while there are less LWP and IWP with the use of the 15 and 35 km resolutions compared to using the 500 m resolution. Also, the sensitivity of LWP and IWP to increasing aerosol concentrations is reduced significantly as resolution coarsens.
In Figs. 5 and 6, satellite-observed LWP and IWP for both cases follow their CSRM-simulated counterparts reasonably well for the polluted scenario. This shows that the CSRM simulations, which are performed with the 500 m resolution, perform well and can thus represent benchmark simulations. Taking the CSRM simulations as benchmark simulations, we see that the ARW simulations at the coarse resolutions of 15 and 35 km underestimate the cloud mass and its sensitivity to increasing aerosol concentrations compared to the CSRM simulations due to coarse resolution.
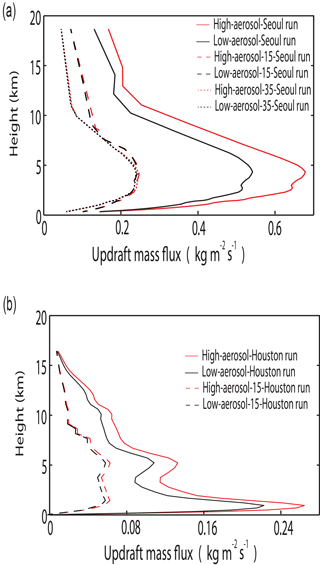
Figure 7Vertical distributions of the time- and domain-averaged updraft mass fluxes for (a) the Seoul case and (b) the Houston case. Solid lines represent simulations at the 500 m resolution, while dashed lines represent those at the 15 km resolution. Dotted lines represent simulations at the 35 km resolution.
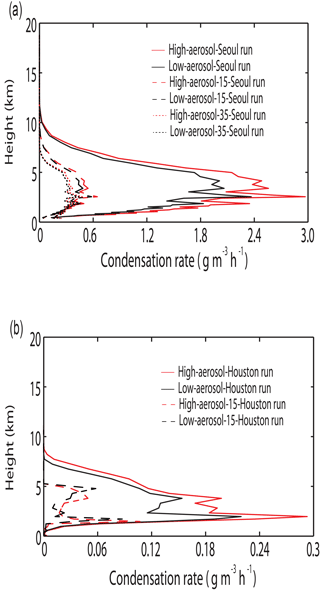
Figure 8Vertical distributions of the time- and domain-averaged condensation rates for (a) the Seoul case and (b) the Houston case. Solid lines represent simulations at the 500 m resolution, while dashed lines represent those at the 15 km resolution. Dotted lines represent simulations at the 35 km resolution.
5.1.2 Updrafts, condensation, and deposition
To understand the response of the cloud mass to increasing aerosol concentrations and the variation in the cloud mass and its response to increasing aerosol concentrations with varying resolution, as shown in Figs. 3–6, we calculate updraft mass fluxes since these fluxes control supersaturation that in turn controls condensation and deposition as key determination factors for the cloud mass. Updraft mass fluxes are obtained by multiplying the predicted updraft speed by air density. Since there are negligible differences in air density among the ARW simulations, most of differences in updraft mass fluxes among the simulations are caused by differences in the updraft speed or updrafts. Those differences in air density are in general ∼ 2 orders of magnitude smaller than those in the updraft speed or updrafts. We also obtain condensation and deposition rates. The vertical distributions of the time- and domain-averaged updraft mass fluxes, condensation rates, and deposition rates for the Seoul and Houston cases are shown in Figs. 7–9, respectively. Here, condensation and deposition rates are defined as the rates of changes in liquid mass and ice mass in a unit volume of air and for a unit time due to condensation and deposition on the surface of hydrometeors, respectively.
As seen for the cloud mass, updraft mass fluxes and condensation and deposition rates increase with increasing aerosol concentrations between the polluted scenario and the clean scenario at all resolutions and for all cases considered. Increasing aerosol concentrations alter cloud microphysical properties such as drop size and autoconversion. Aerosol-induced changes in autoconversion in turn increase cloud liquid mass as a source of evaporation and freezing. Numerous studies (e.g., Khain et al., 2005; Seifert and Beheng, 2006; Tao et al., 2007, 2012; van den Heever and Cotton, 2007; Storer et al., 2010; Lee et al., 2013, 2017) have shown that aerosol-induced increases in cloud liquid mass and associated increases in the freezing of cloud liquid enhance the freezing-related latent heating and thus parcel buoyancy, and this invigorates convection or increases updraft mass fluxes. Studies have also shown that the aerosol-induced increases in cloud liquid mass and associated increases in the evaporation of cloud liquid enhance the evaporation-related latent cooling and thus negative buoyancy. This intensifies downdrafts, and after reaching the surface, the intensified downdrafts spread out toward the surrounding warm air to form intensified gust fronts and then uplift the warm air more strongly. More strongly uplifted warm air leads to invigorated convection or increased updraft mass fluxes. These freezing- and evaporation-related invigoration mechanisms are operative to induce the aerosol-induced enhancement of updraft mass fluxes, condensation, and deposition in this study.
Aerosol-induced percentage increases in updraft mass fluxes and deposition and condensation rates at the 500 m resolution between the polluted scenario and the clean scenario are approximately 1 order of magnitude greater than those at the 15 and 35 km resolutions. Stated differently, the sensitivity of updraft mass fluxes to increasing aerosol concentrations is reduced substantially with coarsening resolution, due to this, the sensitivity of deposition and condensation rates, and thus the cloud mass, to increasing aerosol concentrations is also reduced substantially with coarsening resolution. Updraft mass fluxes at the 15 and 35 km resolutions are much smaller than those at the 500 m resolution (Fig. 7). This induces deposition and condensation rates, and thus the cloud mass, to be much smaller at the 15 and 35 km resolutions than at the 500 m resolution. Hence, taking the CSRM simulations as benchmark simulations, the updraft mass fluxes (and thus the cloud mass) are underestimated in the ARW simulations at the 15 and 35 km resolutions due to the coarse resolutions. Taking the sensitivity of updraft mass fluxes to increasing aerosol concentrations in the CSRM simulations as the benchmark sensitivity, the ARW simulations at the 15 and 35 km resolutions also underestimate the sensitivity due to the coarse resolutions.
Sub-grid updrafts or updrafts that are not resolved by the coarse resolutions in the NWP models are to be represented by cumulus parameterizations or scale-aware cumulus parameterizations in those models. Comparisons between the CSRM simulations at the fine resolution and the ARW simulations at the coarse resolutions (which are generally adopted by current NWP models) here suggest that with no cumulus parameterizations or scale-aware cumulus parameterizations to represent sub-grid updrafts, coarse resolutions induce the underestimation of updrafts and their sensitivity to increasing aerosol concentrations. This in turn suggests that cumulus parameterizations or scale-aware cumulus parameterizations should represent sub-grid updrafts in NWP models in such a way that sub-grid updrafts correct and prevent the coarse-resolution-induced underestimation of updrafts. The comparisons also suggest that cumulus parameterizations or scale-aware cumulus parameterizations, with pathways through which increasing aerosol concentrations interact with updrafts, should represent interactions between sub-grid updrafts and varying aerosol concentrations in NWP models in such a way that the interactions correct and prevent the coarse-resolution-induced underestimation of the sensitivity of updrafts to varying aerosol concentrations.
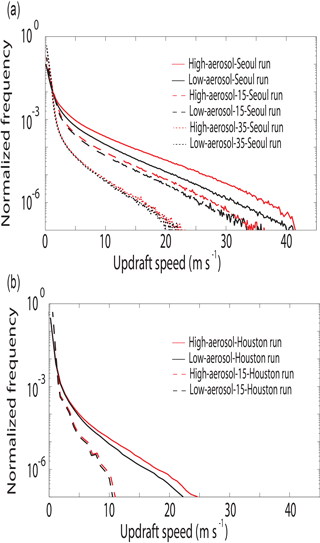
Figure 10Distributions of normalized updraft frequency over updraft speeds for (a) the Seoul case and (b) the Houston case. Solid lines represent simulations at the 500 m resolution, while dashed lines represent those at the 15 km resolution. Dotted lines represent simulations at the 35 km resolution.
Figure 10 shows the frequency distribution of updrafts over the updraft speed, which is normalized over the domain and the simulation period. We first calculate the frequency over the domain at each time step and in each discretized updraft bin. The frequency in each bin and at each time step is then divided by the total number of grid points in the whole domain. The normalized frequency at each time step is summed over all of the time steps in each updraft bin. This sum is divided by the total number of time steps as the final step in the normalization process. With coarsening resolution, the normalized frequency of weak updrafts with speeds less than ∼2 m s−1 increases for both scenarios in both cases. However, the normalized frequency of strong updrafts with speeds greater than ∼2 m s−1 is reduced with coarsening resolution. The frequency shift from high-level updraft speeds to low-level speeds leads to a reduction in the mean updrafts with coarsening resolution for both scenarios in both cases.
The updraft frequency is greater in the polluted scenario than in the clean scenario at all resolutions and for all cases. The overall difference in the frequency between the scenarios is reduced with coarsening resolution. This is associated with the reduction in the sensitivity of the averaged updrafts to increasing aerosol concentrations with coarsening resolution. In particular, the difference in the frequency for weak updrafts (speeds less than ∼2 m s−1) between the scenarios does not vary much with coarsening resolution. On average, the percentage difference for weak updrafts is less than 2–3 % at all resolutions. However, the difference for strong updrafts varies significantly with varying resolution. The mean difference for strong updrafts varies from ∼30–60 % for the 500 m resolution to less than ∼5–6 % for the 15 and 35 km resolutions. Analyses of the updraft frequency here suggest that strong updrafts are more sensitive to the aerosol-induced invigoration of convection than weak updrafts. Variation in the sensitivity of the averaged updrafts to increasing aerosol concentrations at varying resolution is associated more with variation in the response of strong updrafts to aerosol-induced invigoration at varying resolution than with that of weak updrafts. Another point to make here is that the frequency of weak updrafts is overestimated, while that of strong updrafts is underestimated at coarse resolution compared to the frequencies in the fine-resolution CSRM simulations.
5.1.3 Evaporation and precipitation distributions
Aerosol-induced increases in evaporation and associated cooling affect downdrafts, and changes in downdrafts in turn affect gust fronts. Aerosol-induced changes in the intensity of gust fronts affect the organization of cloud systems, which is characterized by cloud-cell spatiotemporal distributions. In general, aerosol-induced greater increases in evaporation result in aerosol-induced greater changes in the intensity of gust fronts and in cloud system organization (Tao et al., 2007, 2012; van den Heever and Cotton, 2007; Storer et al., 2010; Lee et al., 2013, 2017).
Considering that individual cloud cells act as individual sources of precipitation, aerosol-induced changes in the cloud system organization can alter precipitation spatiotemporal distributions, which play an important role in hydrological circulations. It is thus important to examine how the response of evaporation to increasing aerosol concentrations varies with varying resolution, i.e., to see how coarse resolution affects the quality of simulations of aerosol effects on hydrological circulations. Motivated by this, evaporation rates are obtained and are shown in Fig. 11. Here, the evaporation rate is defined as the rate of change in liquid mass in a unit volume of air and for a unit time due to evaporation on the surface of hydrometeors.
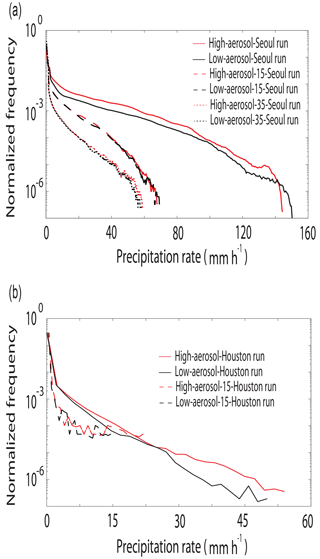
Figure 12Distributions of normalized precipitation frequency over precipitation rates for (a) the Seoul case and (b) the Houston case. Solid lines represent simulations at the 500 m resolution, while dashed lines represent those at the 15 km resolution. Dotted lines represent simulations at the 35 km resolution.
As seen in the above-described variables, evaporation rates increase as the aerosol concentration increases, and the sensitivity of the evaporation rate to increasing aerosol concentrations is reduced with coarsening resolution among the ARW simulations. This suggests that the sensitivities of the cloud system organization and precipitation distributions to increasing aerosol concentrations are likely also reduced with coarsening resolution, as reported in previous studies (e.g., Tao et al., 2007, 2012; van den Heever and Cotton, 2007; Storer et al., 2010; Lee et al., 2013, 2017). This is confirmed by the distribution of normalized precipitation frequency over precipitation rates shown in Fig. 12. Similar to the normalization for the updraft frequency, we first calculate the frequency of surface precipitation rates at each time step and in each discretized precipitation rate bin. The frequency in each bin and at each time step is then divided by the total number of grid points at the surface. The normalized frequency at each time step is summed over all of the time steps. This sum is divided by the total number of time steps as the final step in the normalization process. Figure 12 shows that due to the reduction in the sensitivity of evaporative cooling to the increasing aerosol concentration as resolution coarsens, differences in the distribution of precipitation frequency between the polluted scenario and the clean scenario are reduced substantially as resolution coarsens. Taking the 500 m resolution CSRM simulations as benchmark simulations, this demonstrates that the coarse-resolution ARW simulations underestimate the sensitivity of evaporative cooling, cloud system organization, and precipitation distributions to increasing aerosol concentrations.
5.2 Test on the effects of microphysics parameterizations on the simulations of clouds, precipitation, and CAPI
As mentioned previously, among microphysical processes, saturation, sedimentation, and collection processes are those whose parameterizations are a main cause of errors in the simulation of clouds, precipitation, and CAPI. Motivated by this, we focus on these three microphysical processes for testing the effects of microphysics parameterizations on the simulations of clouds, precipitation, and CAPI. As a preliminary step to this test, we first focus on the effects of microphysics parameterizations on the simulation of the cloud mass, which plays a key role in cloud radiative properties and precipitation. Based on Figs. 3 and 4, we focus on the CLC, which accounts for the bulk of the total cloud mass.
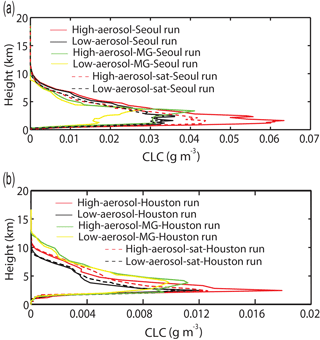
Figure 13Vertical distributions of the time- and domain-averaged cloud liquid content (CLC) for (a) the Seoul case and (b) the Houston case. Solid red and black lines represent simulations with the bin scheme and at the 500 m resolution, while dashed red and black lines represent the bin-scheme simulations with the saturation adjustment. Solid yellow and green lines represent simulations with the MG scheme.
Table 2Rates of the different processes that are associated with saturation, collection, and sedimentation for the Seoul case. The cloud liquid condensation and collection (i.e., autoconversion plus accretion of cloud liquid) rates as shown here are averaged over the whole domain and simulation period. The sedimentation rates as shown here are averaged over the whole simulation period and over each of the following three layers with different altitude ranges: a layer between the surface and 2.5 km, between 2.5 and 5.0 km, and above 5.0 km.
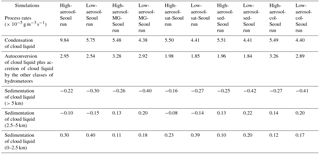
Figure 13 shows the vertical distributions of the time- and domain-averaged CLC. In Fig. 13a, solid red and black lines represent the high-aerosol-Seoul run and the low-aerosol-Seoul run, respectively, while in Fig. 13b, those lines represent the high-aerosol-Houston run and the low-aerosol-Houston run, respectively. Note that the runs shown in the figure are performed using the bin scheme and the 500 m resolution. These simulations were repeated with the Morrison two-moment scheme. These repeated simulations using the MG scheme, referred to as the high-aerosol-MG-Seoul run, the low-aerosol-MG-Seoul run, the high-aerosol-MG-Houston run, and the low-aerosol-MG-Houston run, are represented by solid yellow and green lines in Fig. 13. Between the high-aerosol and low-aerosol runs using the MG scheme for the two cases, there is an increase in CLC with increasing aerosol concentration. However, this increase is much smaller than that between the high-aerosol and low-aerosol runs using the bin scheme for the two cases. As seen in Tables 2 and 3, differences in the time- and domain-averaged rate of condensation, which is the primary source of cloud liquid or CLC, are greater between the polluted and clean scenarios using the bin scheme (e.g., the high-aerosol run and the low-aerosol run) than using the MG scheme (e.g., the high-aerosol-MG run and the low-aerosol-MG run) for the two cases. This contributes to the greater increase in CLC with increasing aerosol concentration between the high-aerosol run and the low-aerosol run than between the high-aerosol-MG run and the low-aerosol-MG run for the two cases.
In addition, there is a significant difference in the shape of the vertical profile of CLC between the simulations with the MG scheme and those with the bin scheme for both cases. Here, the shape is represented by the peak value of CLC and the altitude of the peak value in the vertical profile. The peak value is higher in the simulations with the bin scheme than in the simulations with the MG scheme for each of the polluted and clean scenarios. The altitude of the peak value is lower in the simulations with the bin scheme than in the simulations with the MG scheme. For the Seoul (Houston) case, the altitude is ∼2 km in the simulations with the bin scheme, while it is ∼4 km in those with the MG scheme.
We next test how the parameterization of saturation processes, which determine phase transition processes such as condensation, affects the simulations by comparing the supersaturation prediction in the bin scheme to the saturation adjustment in the MG scheme. To do this, the simulations with the bin scheme are repeated after replacing the supersaturation prediction in the bin scheme with the saturation adjustment in the MG scheme. These repeated simulations are referred to as the high-aerosol-sat-Seoul run, the low-aerosol-sat-Seoul run, the high-aerosol-sat-Houston run, and the low-aerosol-sat-Houston run. The high-aerosol-sat-Seoul run and the low-aerosol-sat-Seoul run for the Seoul case and the high-aerosol-sat-Houston run and the low-aerosol-sat-Houston run for the Houston case are represented by dashed lines in Fig. 13. As in the other simulations, there is an increase in CLC with increasing aerosol concentrations between the high-aerosol-sat and the low-aerosol-sat runs for the two cases. However, this increase is much smaller than that between the high-aerosol and low-aerosol runs for the two cases, but it is similar to that between the high-aerosol-MG and low-aerosol-MG runs for the two cases. As seen in Tables 2 and 3, differences in the time- and domain-averaged condensation rate between the high-aerosol-sat and the low-aerosol-sat runs are much smaller than those between the high-aerosol and low-aerosol runs for the two cases. These differences between the high-aerosol-sat and the low-aerosol-sat runs are similar to those between the high-aerosol-MG and low-aerosol-MG runs for the two cases. This contributes to the smaller CLC increase between the high-aerosol-sat and the low-aerosol-sat runs than between the high-aerosol and low-aerosol runs. It also contributes to the similarity in the CLC increase between the pair of the high-aerosol-sat and the low-aerosol-sat runs and that of the high-aerosol-MG and low-aerosol-MG runs for the two cases. Here, we see that the sensitivity of the CLC and associated condensation to increasing aerosol concentrations is affected by the parameterization of the saturation process and that the use of the saturation adjustment reduces the sensitivity compared to using the supersaturation prediction.
The high-aerosol-sat-Seoul run, the low-aerosol-sat-Seoul run, the high-aerosol-sat-Houston run, and the low-aerosol-sat-Houston run are repeated by replacing the bin-scheme sedimentation with the sedimentation from the MG scheme as a way of testing the effects of the parameterization of sedimentation on the simulations. These repeated runs are referred to as the high-aerosol-sed-Seoul run, the low-aerosol-sed-Seoul run, the high-aerosol-sed-Houston run, and the low-aerosol-sed-Houston run. These runs are identical to the high-aerosol-Seoul run, the low-aerosol-Seoul run, the high-aerosol-Houston run, and the low-aerosol-Houston run, respectively, except for the parameterization of the saturation and sedimentation processes. As mentioned previously, terminal velocities vary as hydrometeor sizes vary in the bin scheme, while the MG scheme adopts mass-weight mean terminal velocities for the calculation of the sedimentation process.
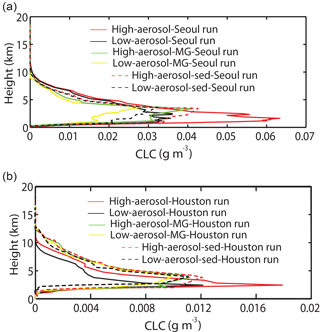
Figure 14Vertical distributions of the time- and domain-averaged cloud liquid content (CLC) for (a) the Seoul case and (b) the Houston case. Solid red and black lines represent simulations with the bin scheme and at the 500 m resolution, while dashed red and black lines represent the bin-scheme simulations with the saturation adjustment and the MG-scheme sedimentation process. Solid yellow and green lines represent simulations with the MG scheme.
The vertical distributions of the CLC in the high-aerosol-sed-Seoul run, the low-aerosol-sed-Seoul run, the high-aerosol-sed-Houston run, and the low-aerosol-sed-Houston run are represented by dashed lines in Fig. 14. Comparisons between the pair of high-aerosol-sed and low-aerosol-sed runs and the pair of high-aerosol-MG and low-aerosol-MG runs for the two cases show that not only the increases in the CLC with increasing aerosol concentrations but also the shapes of the vertical distribution of the CLC in the high-aerosol-sed and low-aerosol-sed runs are similar to those in the high-aerosol-MG and low-aerosol-MG runs for the two cases. As seen in Tables 2 and 3, in the high-aerosol run and low-aerosol runs, the averaged sedimentation-induced increase in CLC is only in the low altitudes between the surface and 2.5 km. However, in the high-aerosol-MG and the low-aerosol-MG runs, the averaged sedimentation-induced increase in CLC occurs at both the mid-altitudes between 2.5 and 5.0 km and the low altitudes below 2.5 km; the greater increase occurs in the mid-altitudes rather than in the low altitudes. This contributes to the lower altitude of the CLC peak in the high-aerosol and low-aerosol runs than that in the high-aerosol-MG and low-aerosol-MG runs for the two cases. In the high-aerosol-sed and low-aerosol-sed runs, due to the use of the sedimentation from the MG scheme, the altitudes at which the sedimentation-induced increase in CLC occurs are all below 5 km, and there is a greater sedimentation-induced increase in CLC in the mid-altitudes than in the low altitudes as in the high-aerosol-MG and low-aerosol-MG runs. This contributes to the similarity in the altitude of the CLC peak between a pair of the high-aerosol-sed and low-aerosol-sed runs and a pair of the high-aerosol-MG and low-aerosol-MG runs for the two cases. The results here demonstrate that differences in the shape of the vertical profile of CLC between the bin-scheme simulations and the MG-scheme simulations are not explained by differences in the representation of the saturation process alone. The results here also demonstrate that the representation of the sedimentation process plays an important role in generating the differences in the shape of the vertical profile of CLC.
In Fig. 14, we still see remaining differences in the vertical profiles of CLC between the high-aerosol-sed-Seoul and high-aerosol-MG-Seoul runs, between the low-aerosol-sed-Seoul and low-aerosol-MG-Seoul runs, between the high-aerosol-sed-Houston and high-aerosol-MG-Houston runs, and between the low-aerosol-sed-Houston and low-aerosol-MG-Houston runs. To understand the cause of these differences, the high-aerosol-sed-Seoul run, the low-aerosol-sed-Seoul run, the high-aerosol-sed-Houston run, and the low-aerosol-sed-Houston run are repeated again with the MG-scheme collection process. These repeated runs are referred to as the high-aerosol-col-Seoul run, the low-aerosol-col-Seoul run, the high-aerosol-col-Houston run, and the low-aerosol-col-Houston run. These runs are identical to the high-aerosol-Seoul run, the low-aerosol-Seoul run, the high-aerosol-Houston run, and the low-aerosol-Houston run, respectively, except for the parameterization of the saturation, sedimentation, and collection processes. As mentioned previously, collection efficiencies vary as hydrometeor sizes vary in the bin scheme, while the MG scheme uses constant collection efficiencies.
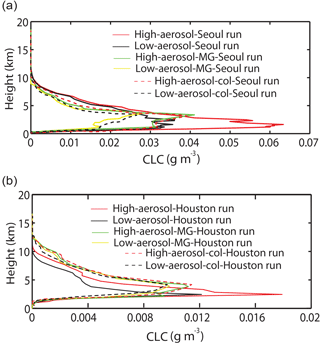
Figure 15Vertical distributions of the time- and domain-averaged cloud liquid content (CLC) for (a) the Seoul case and (b) the Houston case. Solid red and black lines represent simulations with the bin scheme and at the 500 m resolution, while dashed red and black lines represent the bin-scheme simulations with the saturation adjustment and the MG-scheme sedimentation and collection processes. Solid yellow and green lines represent simulations with the MG scheme.
As seen in Fig. 15, the remaining differences between the high-aerosol-col-Seoul and high-aerosol-MG-Seoul runs, between the low-aerosol-col-Seoul and low-aerosol-MG-Seoul runs, between the high-aerosol-col-Houston and high-aerosol-MG-Houston runs, and between the low-aerosol-col-Houston and low-aerosol-MG-Houston runs nearly disappear. As seen in Tables 2 and 3, in the high-aerosol-sed (low-aerosol-sed) run with the bin-scheme collection process, the rate of conversion of cloud liquid to rain via autoconversion and accretion of cloud liquid is lower than in the high-aerosol-MG (low-aerosol-MG) run. This contributes to the higher CLC in the high-aerosol-sed run than in the high-aerosol-MG run and in the low-aerosol-sed run than in the low-aerosol-MG run for the two cases. When the high-aerosol-col and low-aerosol-col runs adopt the collection process from the MG scheme, the conversion rate becomes similar between the high-aerosol-col (low-aerosol-col) run and the high-aerosol-MG (low-aerosol-MG) run. This contributes to the disappearance of the abovementioned remaining differences as shown in Fig. 15. Here, with fairly good confidence, it is demonstrated that differences between the high-aerosol-Seoul run (the high-aerosol-Houston run) and the high-aerosol-MG-Seoul run (the high-aerosol-MG-Houston run) or between the low-aerosol-Seoul run (the low-aerosol-Houston run) and the low-aerosol-MG-Seoul run (the low-aerosol-MG-Houston run) are explained by differences in the parameterizations of the saturation, sedimentation, and collection processes between the bin scheme and the MG scheme.
5.3 Relative importance of resolution and parameterizations
Comparisons between ARW simulations with different resolutions and those with different microphysics parameterizations as shown in Figs. 3 and 13 demonstrate that the variation in cloud variables is much greater with respect to the variation in resolution than with the variation in microphysics parameterizations. For example, comparisons between Figs. 3 and 13 show that the variation in the time- and domain-averaged cloud mass is ∼2–4 times greater as resolution varies than when the microphysics parameterizations vary. This suggests that as a first step toward reducing the first-order errors in the NWP simulations, we first need to focus on the reduction in errors that are associated with the use of coarse resolution in the NWP models.
This study examines the uncertainties in simulations of clouds, precipitation, and CAPI in NWP models. Here, we focus on uncertainties that are created by the microphysics parameterizations and by the model resolution chosen. In particular, for the examination of the uncertainties associated with microphysics parameterizations, we investigate the contributions of the parameterizations of three key microphysical processes, i.e., saturation, collection, and sedimentation, to the uncertainties.
As a way of examining the uncertainties created by the microphysics parameterizations, we compare the MG scheme (a representative bulk scheme) to the bin scheme, which acts as a benchmark scheme. The vertical distribution of the cloud mass simulated by the MG scheme deviates substantially from that simulated by the bin scheme. In particular, there is a substantial discrepancy in the peak value of the distribution and the altitude of the peak value between the schemes. Also, there is a substantial discrepancy between the schemes in the sensitivity of the cloud mass to increasing aerosol concentrations.
The discrepancy in the sensitivity is closely linked to the discrepancy in the parameterization of the saturation processes between the schemes. The use of the saturation adjustment in the bulk scheme reduces the sensitivity by a factor of ∼2 compared to the use of the supersaturation prediction in the bin scheme. The discrepancy in the peak value and its altitude between the schemes is strongly linked to the parameterization of sedimentation in the schemes. The use of identical parameterizations of saturation and sedimentation makes the sensitivity and the peak value and its altitude similar between the schemes, although there still remains a slight difference in the magnitude of the cloud mass. This remaining difference is explained by the discrepancy in the parameterization of the collection process. When the two schemes use identical parameterizations of saturation, sedimentation, and collection processes, the sensitivity and the peak value and its altitude become nearly identical between the two schemes. This confirms that differences in the parameterizations of the three key processes (i.e., saturation, sedimentation, and collection) are the main cause of the differences in the simulations of clouds between the schemes as indicated by Fan et al. (2012) and Khain et al. (2015).
By selecting the simulations with the bin scheme as benchmark simulations, we see that the use of the saturation adjustment, as done in most current NWP models, can lead to an underestimation of the sensitivity of the cloud mass to increasing aerosol concentrations. Fan et al. (2012) and Khain et al. (2015) have also shown that the sensitivity of the cloud mass to increasing aerosol concentrations is lower in the bulk scheme than in the bin scheme. This study shows that the lower sensitivity in the bulk scheme is closely linked to the use of the saturation adjustment in the bulk scheme.
It is well known that the shape of the vertical profile of the cloud mass (i.e., the peak value of the cloud mass and its altitude) or how the cloud mass is distributed in the vertical domain has substantial implications for cloud radiative forcing and precipitation processes. This study demonstrates that the different parameterizations of the sedimentation process between the schemes lead to different shapes of the cloud mass profiles and thus different cloud radiative forcings and precipitation processes. The use of a mass-weight mean terminal velocity for sedimentation as used in bulk schemes can lead to misleading shapes, cloud radiative forcings, and precipitation processes compared to those in the benchmark bin-scheme simulations in which terminal velocities vary as hydrometeor sizes vary.
This study shows that the use of the coarse resolutions generally used in current NWP models can cause an underestimation of the updraft intensity and thus condensation and deposition, which leads to an underestimation of the cloud mass. Also, the use of coarse resolution results in the underestimation of the sensitivity of updrafts and cloud mass and of the sensitivity of evaporation to increasing aerosol concentrations. The underestimation of the sensitivity of evaporation leads to that of the sensitivity of cloud system organization and precipitation distributions to increasing aerosol concentrations.
Through the examination of the sensitivity of the results to the resolution chosen, we find that updrafts, other associated cloud variables, and their sensitivity to increasing aerosol concentrations are strongly controlled by small-scale updrafts. When they are resolved with the use of high-resolution models, there are high-value-averaged updrafts and variables and their strong sensitivity; but when they are not resolved in low-resolution models, there are low-value-averaged updrafts and variables and their weak sensitivity. This means that small-scale updrafts not resolved with coarse resolution play an important role in the simulation of the correct magnitude of updrafts, other associated variables, and their sensitivity to increasing aerosol concentrations.
The frequency distributions of updrafts simulated in this study show that the frequency of weak updrafts is overestimated, while that of strong updrafts is underestimated in the simulations with coarse resolution compared to those in the CSRM simulations. Hence, the updraft speed shifts toward lower values with coarsening resolution. We see that not resolving small-scale updrafts results in the underestimation of strong updrafts and the overestimation of weak updrafts for both the polluted and clean scenarios. This suggests that sub-grid parameterizations (e.g., cumulus parameterizations and scale-aware cumulus parameterizations) in NWP models should be able to compensate for the overestimation and underestimation of weak updrafts and strong updrafts, respectively, due to coarse resolution.
The difference in the frequency distributions of updrafts between the polluted and clean scenarios is reduced substantially, particularly for strong updrafts, with coarsening resolution. Not resolving small-scale updrafts results in a reduced difference in strong updrafts between the scenarios. This is why the sensitivity of updrafts to increasing aerosol concentrations is reduced with coarsening resolution. In general, parameterizations that represent sub-grid updrafts do not have pathways through which increasing aerosol concentrations affect updrafts. However, recent studies by Lim et al. (2014), Thayer-Calder et al. (2015), and Griffin and Larson (2016) have attempted to consider interactions among microphysical processes, their variations with varying aerosol concentrations, and sub-grid dynamic (e.g., updrafts and downdrafts) and thermodynamic (e.g., temperature) variables in those parameterizations. These efforts should focus on countering the variation in the sensitivity of updrafts, particularly strong updrafts to increasing aerosol concentrations with coarsening resolution. Interactions between varying aerosol concentrations, thermodynamic variables, and downdrafts in parameterizations should be able to counter the variation in the sensitivity of cloud-system organization and precipitation distributions to increasing aerosol concentrations with coarsening resolution. While the pattern of the sensitivity and its variation shown in this study provide valuable information useful for aiding these efforts, results may be different for different cloud types and environments given the strong dependence of aerosol–cloud interactions on cloud type and environmental conditions. To aid the efforts in a generalized way, future studies with more cases that involve various types of aerosol–cloud interactions are needed.
The data used are currently private and stored in our private computer system. Opening the data to the public requires approval from the funding sources, which are NOAA, ETRI, and KMA. Since the funding project associated with this work is still ongoing, opening the data to the public is currently not viable; 2–3 years after the project ends, the data can be open to the public.
The authors declare that they have no conflict of interest.
This study is supported by NOAA (grant NOAA-NWS-NWSPO-2015-2004117) and the Korea Environmental Industry & Technology Institute
funded by the Korea Ministry of Environment under the Climate Change Correspondence Program. This study is also supported by
the Development of Climate and Atmospheric Environmental Applications project funded by ETRI, which is a subproject of the program Development
of Geostationary Meteorological Satellite Ground Segment (NMSC-2017-01) funded by NMSC of
KMA.
Edited by: Jianping Huang
Reviewed by: three anonymous referees
Basu, S., Begum, Z. N., and Rajagopal, E. N.: Impact of boundary-layer parameterization schemes on the prediction of the Asian summer monsoon, Bound.-Layer Meteorol., 86, 469–485, 1998.
Bogenschutz, P. A. and Krueger, S. K.: A simplified PDF parameterization of subgrid-scale clouds and turbulence for cloud-resolving models, J. Adv. Model. Earth Sy., 5, 195–211, https://doi.org/10.1002/jame.20018, 2013.
Dipu, S., Quaas, J., Wolke, R., Stoll, J., Mühlbauer, A., Sourdeval, O., Salzmann, M., Heinold, B., and Tegen, I.: Implementation of aerosol–cloud interactions in the regional atmosphere–aerosol model COSMO-MUSCAT(5.0) and evaluation using satellite data, Geosci. Model Dev., 10, 2231–2246, https://doi.org/10.5194/gmd-10-2231-2017, 2017.
Donelan, M. A., Haus, B. K., Reul, N., Plant, W. J., Stiassnie, M., Graber, H. C., Brown, O. B., and Saltzman, E. S.: On the limiting aerodynamic roughness of the ocean in very strong winds, Geophys. Res. Lett., 31, L18306, https://doi.org/10.1029/2004GL019460, 2004.
Fan, J., Yuan, T., Comstock, J. M., Ghan, S., Khain, A., Leung, L. R., Li, Z., Martins, V. J., and Ovchinnikov, M.: Dominant role by vertical wind shear in regulating aerosol effects on deep convective clouds, J. Geophys. Res.-Atmos., 114, D22206, https://doi.org/10.1029/2009JD012352, 2009.
Fan, J., Leung, L. R., Li, Z., Morrison, H., Chen, H., Zhou, Y., Qian, Y., and Wang, Y.: Aerosol impacts on clouds and precipitation in eastern China: results from bin and bulk microphysics, J. Geophys. Res.-Atmos., 117, D00K36, https://doi.org/10.1029/2011JD016537, 2012.
Fan, J., Leung, L. R., Rosenfeld, D., Chen, Q., Li, Z., Zhang, J., and Yan, H.: Microphysical effect determine macrophysical response for aerosol impact on deep convective clouds, P. Natl. Acad. Sci. USA, 110, E4581–E4590, https://doi.org/10.1073/pnas.1316830110, 2013.
Fouquart, Y. and Bonnel, B.: Computations of solar heating of the Earth's atmosphere: a new parameterization, Beitr. Phys. Atmos., 53, 35–62, 1980.
Griffin, B. M. and Larson, V. E.: Parameterizing microphysical effects on variances and covariances of moisture and heat content using a multivariate probability density function: a study with CLUBB (tag MVCS), Geosci. Model Dev., 9, 4273–4295, https://doi.org/10.5194/gmd-9-4273-2016, 2016.
Hwang, S.-O. and Lee, D.-K.: A study on the relationship between heavy rainfalls and associated low-level jets in the Korean peninsula, J. Korean Meteorol. Soc., 29, 133–146, 1993.
Khain, A. and Lynn, B.: Simulation of a supercell storm in clean and dirty atmosphere using weather research and forecast model with spectral bin microphysics, J. Geophys. Res.-Atmos., 114, D19209, https://doi.org/10.1029/2009JD011827, 2009.
Khain, A. P., Rosenfeld, D., and Pokrovsky, A.: Aerosol impact on the dynamics and microphysics of deep convective clouds, Q. J. Roy. Meteor. Soc., 131, 2639–2663, https://doi.org/10.1256/qj.04.62, 2005.
Khain, A. P., Beheng, K. D., Heymsfield, A., Korolev, A., Krichak, S. O., Levin, Z., Pinsky, M., Phillips, V., Prabhakaran, T., Teller, A., van den Heever, S. C., and Yano, J.-I.: Representation of microphysical processes in cloudresolving models: spectral (bin) microphysics versus bulk parameterization, Rev. Geophys., 53, 247–322, https://doi.org/10.1002/2014RG000468, 2015.
Kim, J. H., Yum, S. S., Shim, S., Kim, W. J., Park, M., Kim, J.-H., Kim, M.-H., and Yoon, S.-C.: On the submicron aerosol distributions and CCN number concentrations in and around the Korean Peninsula, Atmos. Chem. Phys., 14, 8763–8779, https://doi.org/10.5194/acp-14-8763-2014, 2014.
Lance, S., Nenes, A., Mazzoleni, C., Dubey, M. K., Gates, H., Varutbangkul, V., Rissman, T. A., Murphy, S. M., Sorooshian, A., Flagan, R. C., Seinfeld, J. H., Feingold, G., and Jonsson, H. H.: Cloud condensation nuclei activity, closure, and droplet growth kinetics of Houston aerosol during the Gulf of Mexico Atmospheric Composition and Climate Study (GoMACCS), J. Geophys. Res.-Atmos., 114, D00F15, https://doi.org/10.1029/2008JD011699, 2009.
Lee, S.-S. and Feingold, G.: Aerosol effects on the cloud-field properties of tropical convective clouds, Atmos. Chem. Phys., 13, 6713–6726, https://doi.org/10.5194/acp-13-6713-2013, 2013.
Lee, S. S., Tao, W.-K., and Jung, C. H.: Aerosol effects on instability, circulations, clouds and precipitation, Adv. Meteorol., 2014, 683950, https://doi.org/10.1155/2014/683950, 2014.
Lee, S. S., Li, Z., Mok, J., Ahn, M.-H., Kim, B.-G., Choi, Y.-S., Jung, C.-H., and Yoo, H. L.: Interactions between aerosol absorption, thermodynamics, dynamics, and microphysics and their impacts on a multiple-cloud system, Clim. Dynam., 49, 3905–3921, https://doi.org/10.1007/s00382-017-3552-x, 2017.
Li, Z., Niu, F., Fan, J., Liu, Y., Rosenfeld, D., and Ding, Y.: Long-term impacts of aerosols on the vertical development of clouds and precipitation, Nat. Geosci., 4, 888–894, https://doi.org/10.1038/NGEO1313, 2011.
Lim, K.-S. S., Fan, J., Leung, L. R., Ma, P.-L., Singh, B., Zhao, C., Zhang, Y., Zhang, G., and Song, X.: Investigation of aerosol indirect effects using a cumulus microphysics parameterization in a regional climate model, J. Geophys. Res.-Atmos., 119, 906–926, 2014.
Mlawer, E. J., Taubman, S. J., Brown, P. D., Iacono, M. J., and Clough, S. A.: RRTM, a validated correlated-k model for the longwave, J. Geophys. Res.-Atmos., 102, 16663–16682, 1997.
Morcrette, J.-J., Benedetti, A., Ghelli, A., Kaiser, J. W., and Tompkins, A. M.: Aerosol-cloud-radiation interactions and their Impact on ECMWF/MACC forecasts, Technical Memorandum, Reading, England, 660, 35 pp., 2011.
Morrison, H. and Gettelman, A.: A new two-moment bulk stratiform cloud microphysics scheme in the Community Atmosphere Model, version 3 (CAM3). Part I: Description and numerical tests, J. Climate, 21, 3642–3659, https://doi.org/10.1175/2008JCLI2105.1, 2008.
Morrison, H., Thompson, G., and Tatarskii, V.: Impact of cloud microphysics on the development of trailing stratiform precipitation in a simulated squall line: comparison of one- and two- moment schemes, Mon. Weather Rev., 137, 991–1007, 2009.
Randall, D. A., Schlesinger, M. E., Galin, V., Meleshko, V., Morcrette, J.-J., and Wetherald, R.: Cloud feedbacks, in: Frontiers in the Science of Climate Modeling, edited by: Kiehl, J. T., Ramanathan, V., Cambridge University Press, https://doi.org/10.1017/CBO9780511535857.009, 217–250, 2006.
Seifert, A. and Beheng, D.: A two-moment cloud microphysics parameterization for mixed-phase clouds. Part 2: Maritime vs. continental deep convective storms, Meteorol. Atmos. Phys., 92, 67–82, 2006.
Seifert, A., Köhler, C., and Beheng, K. D.: Aerosol-cloud-precipitation effects over Germany as simulated by a convective-scale numerical weather prediction model, Atmos. Chem. Phys., 12, 709–725, https://doi.org/10.5194/acp-12-709-2012, 2012.
Storer, R. L., van den Heever, S. C., and Stephens, G. L.: Modeling aerosol impacts on convective storms in different environments, J. Atmos. Sci., 67, 3904–3915, 2010.
Sun, J. and Lee, T.-Y.: A numerical study of an intense quasistationary convection band over the Korean peninsula, J. Meteorol. Soc. Jpn., 80, 1221–1245, 2002.
Sundqvist, H., Berge, E., and Kristjansson, J. E.: Condensation and cloud parameterization studies with a mesoscale numerical weather prediction model, Mon. Weather Rev., 117, 1641–1657, 1989.
Tao, W.-K., Li, X., Khain, A., Matsui, T., Lang, S., and Simpson, J.: The role of atmospheric aerosol concentration on deep convective precipitation: cloud-resolving model simulations, J. Geophys. Res.-Atmos., 112, D24S18, https://doi.org/10.1029/2007JD008728, 2007.
Tao, W.-K., Chen, J. P., Li, Z., and Zhang, C.: Impact of aerosols on convective clouds and precipitation, Rev. Geophys., 50, RG2001, https://doi.org/10.1029/2011RG000369, 2012.
Thayer-Calder, K., Gettelman, A., Craig, C., Goldhaber, S., Bogenschutz, P. A., Chen, C.-C., Morrison, H., Höft, J., Raut, E., Griffin, B. M., Weber, J. K., Larson, V. E., Wyant, M. C., Wang, M., Guo, Z., and Ghan, S. J.: A unified parameterization of clouds and turbulence using CLUBB and subcolumns in the Community Atmosphere Model, Geosci. Model Dev., 8, 3801–3821, https://doi.org/10.5194/gmd-8-3801-2015, 2015.
van den Heever, S. C. and Cotton, W. R.: Urban aerosol impacts on downwind convective storms, J. Appl. Meteorol. Clim., 46, 828–850, 2007.
van den Heever, S. C., Stephens, G. L., and Wood, N. B.: Aerosol indirect effects on tropical convection characteristics under conditions of radiative-convective equilibrium, J. Atmos. Sci., 68, 699–718, 2011.
Wang, H., Skamarock, W. C., and Feingold, G.: Evaluation of scalar advection schemes in the Advanced Research WRF model using large-eddy simulations of aerosol-cloud interactions, Mon. Weather Rev., 137, 2547–2558, 2009.
Zeng, X. and Beljaars, A.: A prognostic scheme of sea surface skin temperature for modeling and data assimilation, Geophys. Res. Lett, 32, L14605, https://doi.org/10.1029/2005GL023030, 2005.