the Creative Commons Attribution 4.0 License.
the Creative Commons Attribution 4.0 License.
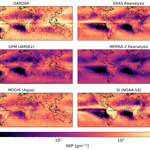
An update on global atmospheric ice estimates from satellite observations and reanalyses
David Ian Duncan
Patrick Eriksson
This study assesses the global distribution of mean atmospheric ice mass from current state-of-the-art estimates and its variability on daily and seasonal timescales. Ice water path (IWP) retrievals from active and passive satellite platforms are analysed and compared with estimates from two reanalysis data sets, ERA5 (European Centre for Medium-range Weather Forecasts Reanalysis 5, ECMWF) and MERRA-2 (Modern-Era Retrospective Analysis for Research and Applications 2). Large discrepancies in IWP exist between the satellite data sets themselves, making validation of the model results problematic and indicating that progress towards a consensus on the distribution of atmospheric ice has been limited. Comparing the data sets, zonal means of IWP exhibit similar shapes but differing magnitudes, with large IWP values causing much of the difference in means. Diurnal analysis centred on A-Train overpasses shows similar structures in some regions, but the degree and sign of the variability varies widely; the reanalyses exhibit noisier and higher-amplitude diurnal variability than borne out by the satellite estimates. Spatial structures governed by the atmospheric general circulation are fairly consistent across the data sets, as principal component analysis shows that the patterns of seasonal variability line up well between the data sets but disagree in severity. These results underscore the limitations of the current Earth observing system with respect to atmospheric ice, as the level of consensus between observations is mixed. The large-scale variability of IWP is relatively consistent, whereas disagreements on diurnal variability and global means point to varying microphysical assumptions in retrievals and models alike that seem to underlie the biggest differences.
The value of the satellite data record for atmospheric science can be separated into three main groups. Operational meteorology relies on satellite data to power numerical weather prediction (NWP) models and inform forecasters (Bauer et al., 2015). Secondly, the satellite data record's global perspective can address questions too large in scale for other observing systems, such as concerning the global distribution of precipitation (Hou et al., 2014) or how much solar radiation is reflected back to space (Vonder Haar and Suomi, 1971). This is inherently valuable for climatology but also crucially important as a check on global models to verify that their output indeed mimics observed reality (Jiang et al., 2012; Wielicki et al., 1995). Lastly, satellite data have proven invaluable for research on atmospheric phenomena and processes, ranging from cloud scales to the atmospheric general circulation, especially where other observations are sparse or non-existent, such as over the oceans and polar regions.
Ice clouds and their effects on Earth's radiative balance are significant at weather and climate timescales – for instance, the planetary albedo and solar energy production are both affected by the coverage, distribution, and properties of ice clouds. Additionally, accounting for atmospheric ice is significant when attempting to close the observed global hydrological cycle. Ice clouds are the most significant emitter of long-wave radiation out to space, and thus uncertainty about their properties impacts the global energy balance. Yet common prognostic variables such as ice water content (IWC) or its integral, usually known as ice water path (IWP), exhibit great spread in both global models and observational data sets derived from satellite instruments (Eliasson et al., 2011; Waliser et al., 2009). This signals a weakness in the meteorological satellite data record, as the limited sensitivity and high uncertainties result in an insufficient constraint on models at weather and climate timescales.
Nearly a decade ago, Waliser et al. (2009) identified cloud ice as a great challenge for modellers and observationalists alike in a landmark study. That paper was optimistic that there were “expectations of progress” on this tough problem; this progress was expected to be driven by more sophisticated models and greater utilization of then recently launched satellite sensors. The two main questions that the current study endeavours to answer are as follows: how much progress has been made, and how much consensus is there on atmospheric ice between models and observations?
Quantifying ice clouds has proven difficult from satellite-borne instruments due to the physics concerning atmospheric ice. Ice clouds can be quite reflective or relatively transparent at visible wavelengths, depending on their thickness and particle size distribution (Baran and Francis, 2004; Zhang et al., 1999). In the infrared, ice clouds can act as nearly perfect black bodies, with clouds too thin to be detectable by the eye, evincing measurable signal at infrared wavelengths (Jensen et al., 1996). Microwave radiation has complex and varied interactions with ice clouds. These range from essentially no interaction at lower-frequency “window” channels to multiple scattering that is highly dependent on ice particle size and shape at higher frequencies (Buehler et al., 2007). Due to this increasing sensitivity with frequency, the platforms best suited for sensing atmospheric ice are higher-frequency (e.g. >85 GHz) passive microwave radiometers and higher-frequency radars. While optical and infrared sensors can detect ice clouds with great sensitivity, the attenuation of these signals means that mainly cloud-top information is obtained from such observations. Thus, for quantification of column integrated atmospheric ice, microwave-based methods are preferred because they have sensitivity to the whole atmospheric column.
The history of IWP estimates can logically be divided into eras before and after the advent of CloudSat (Stephens et al., 2002), which was launched in 2006. Prior to CloudSat, little was known about the vertical structure of clouds on the global scale. Geostationary and polar orbiting satellites had provided data on cloud fraction and cloud-top temperatures since the 1970s using visible and infrared sensors, but the vertical structure of clouds was a relative unknown. In spite of these limitations, global IWP was estimated from various satellite retrievals in the pre-CloudSat era (Bauer and Schlüssel, 1993; Lin and Rossow, 1994; Zhao and Weng, 2002), albeit with retrieval errors typically admitted to be large. Explicit vertical information requires active sensors with profiling capability, such as radar and lidar, whereas existing passive sensors can at best provide implicit vertical information.
Due to CloudSat's high-sensitivity 94 GHz radar and its pairing with the CALIPSO lidar in the A-Train constellation, vertical profiles of atmospheric ice ranging from pristine cirrus ice particles to precipitating ice were now retrievable (Kulie et al., 2016; Sassen et al., 2009). The synergy of CALIPSO with CloudSat permitted greater sensitivity to very thin clouds that CloudSat might otherwise miss (Stephens et al., 2018). Comparisons with output from climate models showed that the magnitude and vertical distribution of ice in models were often far from those of observational data sets (Jiang et al., 2012; Waliser et al., 2009), while IWP compared poorly as well (Eliasson et al., 2011). Microwave limb sounders launched before CloudSat can also act as a check on models due to their sensitivity to IWC (Eriksson et al., 2008; Wu et al., 2009), though their profiling capability is limited to the upper troposphere. Waliser et al. (2009) pointed to this combination of A-Train sensors and limb sounders as a tool with which to bring observed and modelled atmospheric ice estimates closer together.
While CloudSat did provide a quantum leap in observing atmospheric ice profiles, it is ultimately a single-frequency radar, and atmospheric retrievals using CloudSat are solving a multivariate problem given only one reflectivity measurement. CloudSat retrievals must therefore make myriad assumptions about the properties of particles within the volume sampled (Austin et al., 2009; Delanoë and Hogan, 2008; Deng et al., 2010). By one estimate, these assumptions can translate into ±50 % error uncertainties for IWC within a given range gate (Heymsfield et al., 2008). Systematic biases in IWP retrieval from CloudSat are harder to quantify on a global scale, with the microphysical assumptions – namely the shapes and size distribution of particles – perhaps being the biggest cause for retrieval uncertainty. Separating hydrometeors into frozen, mixed-phase, and liquid is another major cause for IWP retrieval uncertainty.
Put bluntly, the global observing system as it stands is not well suited to quantifying atmospheric ice (Birman et al., 2017). The difficulty of quantifying ice mass has been helped greatly by A-Train sensors, but the highly limited spatio-temporal sampling of CloudSat–CALIPSO leaves many open questions regarding the variability of atmospheric ice, from diurnal to intraseasonal timescales (Hong and Liu, 2015). Extant passive sensors provide excellent spatio-temporal coverage but possess channel suites with limited sensitivity to IWP, intended instead to measure humidity or precipitation. This limited sensitivity has not deterred investigators from using existing passive sensors to study IWC and IWP (Gong et al., 2018; Huang et al., 2006; Sun and Weng, 2012; Weng and Grody, 2000), though many are strongly tied to A-Train retrievals (Gong and Wu, 2014; Holl et al., 2014; Strandgren et al., 2017) to ameliorate the limited signal that is available. Satellite simulators offer an alternative perspective (Masunaga et al., 2010), in that the retrieval of physical quantities may be viewed as having secondary importance if signals at various wavelengths are well simulated and heating rates are consistent; but, in the context of developing further physical understanding, this study focuses on the retrieved quantities.
The study of atmospheric ice is expected to gain operational prominence and observational capacity with the second generation of orbiting European meteorological satellites, MetOp-SG. The Ice Cloud Imager (ICI) on MetOp-SG will feature high-frequency microwave channels better suited to ice cloud mass observation (Brath et al., 2018; Buehler et al., 2007; Evans et al., 2012) than those of current meteorological satellite sensors. ICI will be the first operational sensor purpose-built for observing atmospheric ice, and as such may constitute an inflection point in its study. Specifically, the agreement between models and observational data sets is important for operational sensors, as satellite radiances need to be modelled with veracity to be assimilated and positively impact the forecast. In the microwave spectrum, successful data assimilation is most challenging when hydrometeors have a large impact on radiances, though there has been significant progress in recent years with cloud- and precipitation-affected radiance assimilation, with such observations now constituting one of the most important data streams for modern NWP (Geer et al., 2017).
At the end of the CloudSat era of observations, a decade on from Waliser et al. (2009) and prior to the era of operational ice cloud monitoring expected with ICI, this study assesses the state of knowledge for atmospheric ice by probing state-of-the-art satellite and reanalysis data sets. The comparisons will be separated into global mean IWP (Sect. 3), zonal mean profiles of IWC (Sect. 4), interseasonal variability of IWP (Sect. 5), and diurnal variability of IWP (Sect. 6). These are prefaced by brief descriptions of the sensors, algorithms, and data sets employed, and followed by a discussion on the findings.
2.1 Satellite data sets
CloudSat was launched in 2006 for the purpose of characterizing the vertical structure of clouds on a global scale, carrying a W-band (94 GHz) nadir-pointing radar (Stephens et al., 2018). Its effective field of view has a width of 1.4 km on the Earth's surface, with a vertical resolution of approximately 500 m oversampled down to 240 m. CALIPSO is a 532 nm lidar that flies in tight formation with CloudSat, observing at a 60 m vertical resolution with an approximately 1 km horizontal footprint. These A-Train sensors operate in a sun-synchronous low Earth orbit with an ascending node at approximately 13:30 local solar time (LST).
This study uses two CloudSat-based data sets for IWC and IWP, both of which include CALIPSO lidar data to improve sensitivity to thin clouds. These are the DARDAR (Delanoë and Hogan, 2008) and 2C-ICE (Deng et al., 2010) products. Both retrievals use an optimal estimation methodology to retrieve IWC in each range gate of observed reflectivities. This study primarily uses DARDAR data, as both analysis herein as well as Deng et al. (2013) found 2C-ICE to perform very similarly to DARDAR in a global sense. DARDAR has a longer publicly available data record and was thus chosen as the primary CloudSat ice retrieval used here. CloudSat has been operating in daytime-only mode since 2011 due to a battery issue, a fact that prompted some of the comparison choices that will be mentioned later. The specific versions used are 2C-ICE PR04 and DARDAR CLOUD.v2.1.1, with L2 data averaged and gridded at 2.5∘ resolution. The coarse grid is necessary for CloudSat data given its narrow beam and the repeat cycle of its orbit track (Kulie et al., 2016).
Three passive-only data sets provide IWP estimates for the comparison. The Moderate Resolution Imaging Spectroradiometer (MODIS) is an instrument flown on two NASA satellites, Aqua and Terra, that provides high spatial and spectral resolution data on clouds at visible and infrared wavelengths (Platnick et al., 2003). Only data from MODIS on the Aqua satellite are considered here, as Aqua flies in the A-Train behind CloudSat and thus offers the closest point of comparison with respect to sampling. L3 daily (MYD08D3) version C06 data were used (Hubanks et al., 2016; Platnick et al., 2015, 2017), an aggregation of pixel-level MODIS retrievals performed at a 1∘ resolution. These data are weighted by the retrieved cloud fraction as done elsewhere in the literature. C06 represents a large update for MODIS products, including significant changes in the treatment of ice microphysics and pixel averaging, which affects ice optical thickness and effective radius results relative to previous releases (Platnick et al., 2017). The IWP retrieval from MODIS relies on a band combination using absorbing and non-absorbing bands to retrieve cloud optical thickness and effective radius, with the path value proportional to their product (Platnick et al., 2003).
The Global Precipitation Measurement (GPM) mission coordinates a constellation of passive microwave radiometers of various origins and characteristics, harmonized via the GPM Core Observatory to produce precipitation estimates globally at relatively high spatial and temporal resolutions (Hou et al., 2014). While IWP is not a primary focus of GPM, the Bayesian retrieval algorithm utilized by GPM to derive precipitation computes profiles of hydrometeor species (Kummerow et al., 2015), and IWP is an output found in L2 and L3 products. To offer the best comparison with other A-Train sensors, GPM V05 L2 data are taken from the Advanced Microwave Scanning Radiometer 2 (AMSR2), a member of the GPM constellation which has flown ahead of CloudSat in the A-Train since its launch in 2012 (GPM Science Team, 2016). AMSR2 is a 14-channel microwave imager observing from 6.9 to 89 GHz. The L2 IWP values from AMSR2 were gridded at 2.5∘ resolution. The a priori database used by the GPM retrieval consists of simulated radiances through hydrometeor profiles observed by GPM's Dual-frequency Precipitation Radar, which is sensitive to a range of precipitating hydrometeors but not cloud ice. This is by virtue of the lower frequencies and lower receiver sensitivity of its radar, at Ku- and Ka-bands, whereas CloudSat observes at W-band, with 13.6 and 35.5 GHz versus 94 GHz. The GPM results are thus expected to provide an effective estimate of precipitating IWP only, as significant retrieved IWP values are only present in pixels with non-negligible probabilities of precipitation (not shown), indicative of the Bayesian weighting scheme.
The Synergistic Passive Atmospheric Retrieval Experiment-ICE (SPARE-Ice, herein abbreviated SI) represents a passive-only retrieval data set that employs both microwave and infrared radiances to derive IWP (Holl et al., 2014). This is accomplished via a neural network retrieval that was trained on the CloudSat 2C-ICE data set co-located with data from operational microwave and infrared sensors. The only data available were from the NOAA-18 satellite, using radiances from the Microwave Humidity Sounder (MHS) and Advanced Very High Resolution Radiometer (AVHRR), so those L2 data are used in this study, gridded at 2.5∘ resolution. While there are no data from the A-Train, NOAA-18's equatorial crossing time of 18:30 LST is not drastically different from the 13:30 LST ascending node of CloudSat.
2.2 Reanalysis data sets
Reanalysis refers to a modelling approach that endeavours to provide the best estimate of past atmospheric conditions that is consistent with the applied NWP model and all observations used in its data assimilation scheme. Now a widely used tool for atmospheric research, reanalysis uses the same principles as model initialization for NWP forecasting, synthesizing observations as disparate as radiances, sondes, and buoys to yield a complete picture of the atmosphere as it was. It is worth stressing that reanalyses are not climate models and are not an observational record either, but occupy a space in between. However, reanalyses are driven by the observational record and run on models fundamentally similar to climate models, so their interpretation can be instructive about both.
Two reanalysis data sets are used in this study. The first is the European Centre for Medium-range Weather Forecasts (ECMWF) Reanalysis 5, known as ERA5. The other is the Modern-Era Retrospective Analysis for Research and Applications version 2 (MERRA-2), a data set produced by NASA's Global Modeling and Assimilation Office (Gelaro et al., 2017). Both reanalyses provide profiles of ice water content, and both were downloaded at 0.5∘ resolution.
Differentiating between precipitating and non-precipitating ice is problematic for models and observations alike, as noted in previous studies (Jiang et al., 2012; Waliser et al., 2009). ERA5 is different from all other data sets used in this study, as it differentiates between precipitating and non-precipitating ice, classifying them as snow water and cloud ice water, respectively. Because all other data sets queried treat all ice together, ERA5 values reported here are combined unless otherwise stated. Additionally, a caveat to ERA5 IWP values discussed herein is that ERA5 does not output convective ice (Geer et al., 2018), which is parameterized in the model as a convective flux, and thus not included in total IWP here. In contrast, MERRA-2 parameterizes all precipitating ice and outputs only non-precipitating ice.
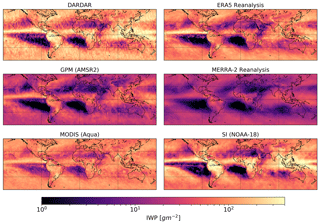
Figure 1Global mean IWP maps from six data sets: four satellite data sets (DARDAR, GPM, MODIS, SI) and two reanalyses (ERA5, MERRA-2). 2015 data are used for all data sets except SI (2013) and DARDAR (2008–2015) due to data availability and desire for greater sampling, respectively. Daytime data were used exclusively to improve comparability with MODIS and the extended CloudSat record. Note that MERRA-2 constitutes non-precipitating ice only, whereas the other panels represent total ice.
A noted difference between ERA5 and MERRA-2 is that ERA5 assimilates cloud- and precipitation-affected radiances from microwave sensors at a higher rate and for more channels (Geer et al., 2017, 2018; McCarty et al., 2016). Since these channels are the most sensitive to columnar atmospheric ice, it is hypothesized that ERA5 should represent a better estimate than MERRA-2. ERA5 assimilates a portion of all-sky radiances from both the AMSR2 sensor and the NOAA-18 satellite, and MERRA-2 assimilates MHS radiances from NOAA-18, so the reanalysis estimates are not entirely independent of the GPM and SI estimates given.
Figure 1 shows the near-global maps of mean IWP from each of the data sets mentioned above. Limited by the data sets' time periods of availability, 2015 was the best common year of availability, though 2013 was used for SI. DARDAR is an exception, displaying data spanning 2008 to 2015, so as to yield a map that is well populated. For all of the data sets, a daytime-only subset is employed to match CloudSat for the fairest comparison in light of the sampling differences. This also permits a better comparison with MODIS, which performs cloud retrievals only during the daytime. The reanalyses were sampled according to A-Train crossing times, which consistently occur near 13:30 LST: i.e. grid points around 0∘ E longitude are averages of the 12Z and 15Z time steps, etc. These are not co-locations with A-Train data, but gridded data sampled daily at about the satellites' crossing time with a width of 45∘ longitude. The maps presented in Fig. 1 are from 60∘ N to 60∘ S, as inclusion of the polar regions would introduce skewed sampling given the daytime-only constraint, in addition to CloudSat not observing beyond about 82∘ latitude.
High IWP values are found in common features across the data sets sampled and include the intertropical convergence zone (ITCZ), Pacific warm pool, and storm track regions. The data sets also largely agree on regions of limited ice cloud mass, such as the stratocumulus regions or the Sahara and Arabian deserts. Some more localized features are also visible, such as enhanced IWP values along ridges that may cause orographic uplift like the coast of Alaska, the southwestern coast of South America, or the edge of the Tibetan plateau, though these features are more present in some estimates than others.
ERA5 is something of an outlier, in that it displays local maxima and minima that are more extreme than those in the other data sets, especially in the eastern Pacific. Notable too is the more dappled appearance of the mean field, the result of high IWP values preferentially occurring at some grid points. While these may be physically reasonable, perhaps caused by periodic behaviour exposed by the diurnal sampling of this analysis, none of the satellite data sets exhibit these tendencies. ERA5 features a more skewed distribution of IWP, with 10 to 20 kg m−2 not uncommon. In contrast, the level 2 MODIS retrieval has a maximum reportable IWP of 5.5 kg m−2, while GPM and DARDAR rarely retrieve values greater than 10 kg m−2 at their native resolutions, though higher values are occasionally retrieved. This raises an interesting theoretical question, namely how much ice mass can be contained in a strong updraught or grid cell; however, practically, the frequency of very large IWP values can also have a sizable effect on the global distribution of IWP, skewing the mean and having an impact on the final interpretation. The standard deviation of IWP from ERA5 reflects much greater variability than the other data sets (not shown), a consequence of more frequent extreme values (see Fig. 2).
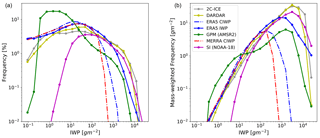
Figure 2Probability distribution function of IWP values from native resolution level 2 data and 0.5∘ reanalysis data. This is shown as a percentage (a) and weighted by mass (b); i.e. summing values in the right panel yields approximately the near-global mean. These data include daytime values spanning 60∘ N–60∘ S from 2015, with 2C-ICE (2008) and SI (2013) being the exceptions due to availability. DARDAR data were treated with a running mean of 6 pixels to approximate a passive microwave footprint. The frequencies shown account for zero values, which are given in the text.
Figure 2 presents a frequency-based perspective on the IWP values that make up the means shown in Fig. 1. The data sets are sampled in the same way, with ERA5 data separated into non-precipitating (cloud) ice and total ice to be more comparable with MERRA-2 and the other data sets, respectively. DARDAR data are treated with a running mean of 6 pixels long to roughly simulate the larger field of view of the passive measurements. For this same reason, the MODIS data are excluded from this analysis because the L3 data are too different in spatial scale. Included in Fig. 2 but not Fig. 1 are CloudSat 2C-ICE data, which are treated like DARDAR; as can be seen, the behaviour of 2C-ICE is similar to DARDAR but differences exist between the retrievals, contributing to a 4 % global mean difference (not shown).
Zeros are accounted for in the calculated frequencies but not shown in Fig. 2 due to the logarithmic scale. True zero values differ significantly among all the data sets, more than may be expected based on sampling resolution or sensitivity alone. Zeros constitute 34 % and 40 % of all ERA5 and MERRA-2 data points, while DARDAR and 2C-ICE display 48 % and 51 % of all points as zero IWP. The passive microwave data sets' contrasting retrieval methods are manifest in divergent behaviour, with GPM containing less than 1 % zero values while SI shows 77 %. If instead of true zeros we expand to values less than 1 g m−2, the reanalyses and DARDAR come closer together with frequencies ranging between 46 % to 59 %, while GPM and SI remain separate at 28 % and 77 %. For perspective, the detectability limit of CloudSat–CALIPSO is roughly 1 g m−2, whereas for passive microwave less than 190 GHz the detectability limit is more like 100 g m−2 (Holl et al., 2014).
The middle of the distribution in Fig. 2 shows relatively similar frequencies of occurrence for DARDAR and the reanalyses between about 1 and 100 g m−2. In fact, ERA5 and MERRA-2 non-precipitating ice data track each other closely from very low values up to 200 g m−2. The importance of zeros to the given frequencies is clearest for the GPM and SI results, as GPM exhibits an order of magnitude higher occurrence around 1.0 g m−2, a consequence of its Bayesian averaging despite AMSR2 possessing no sensitivity for such thin ice clouds; in contrast, SI sees far lower frequencies of occurrence for all but the highest IWP values, which are more representative of the sensitivity range from a passive microwave sensor (Birman et al., 2017; Buehler et al., 2007).
The right panel of Fig. 2 features mass-weighted frequencies, showing which IWP bins contribute most to each data set's mean. Integrating each curve in the right panel would approximate the near-global mean values presented in Fig. 3 but without latitudinal weighting. This view shows that the main cause for discrepancies in mean IWP comes from the high end – magnitudes in excess of 800 g m−2 – with the peak contribution for 2C-ICE and DARDAR coming from IWP values of 1 to 16 kg m−2. Notably, 2C-ICE and DARDAR diverge for very high IWP values, indicating the importance of microphysical or retrieval assumptions for these cases, since these observations use the same data. ERA5 and SI overestimate the prevalence of very high IWP values relative to the CloudSat–CALIPSO-based estimates, with ERA5 reporting IWP values greater than 16 kg m−2 significantly more often than either GPM or DARDAR. These large IWP values have an outsize influence on the means despite their low frequencies of occurrence. For instance, DARDAR retrieves >4 kg m−2 less than 0.5 % of the time but this accounts for almost a fourth of the global mean IWP; in contrast, ERA5 estimates such cases at less than 0.1 % frequency, contributing to about a ninth of its global mean.
Zonal means from 1 year of data are given in Fig. 3. The data that make up these means are the same as in Fig. 1 with the exception of DARDAR, which was limited to 2015 as sampling is less of a concern for zonal means. In contrast to the exponential scale that is useful for gauging spatial patterns of IWP, zonal means are instructive as to the relative magnitudes of each data set and provide a sense of the Hadley cell's influence on the global distribution of atmospheric ice. To ensure that 2015 is not an outlier for IWP and that interannual IWP variability is not a big concern, ERA5 global means were analysed and found to vary by about 1 % from year to year, with 2015 being a typical year.
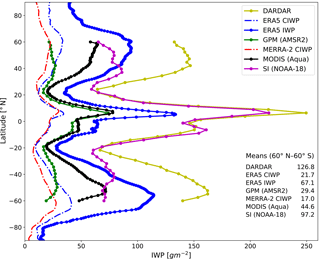
Figure 3Zonal means of IWP, centred on A-Train daytime observations. 2015 is used for all data sets except for SI (2013). ERA5 zonal means are presented for both non-precipitating cloud ice (CIWP) and total ice (CIWP + SIWP = IWP) for a better comparison with MERRA-2 and the observations, respectively. The observational data sets are cut off at 60∘ latitude to mitigate relative sampling biases, with near-global mean values displayed.
The overall shape of the IWP zonal means is fairly consistent across the data sets, in line with the atmospheric general circulation: high IWP values north of the equator characterized by the ITCZ, relative minima in the subtropics, and higher IWP values indicative of the storm tracks at midlatitudes. However, large discrepancies exist in the overall magnitude of IWP, including between the observational data sets; these discrepancies in magnitude are especially stark at midlatitudes, where differentiation of supercooled water and graupel is a predominant concern (Hu et al., 2010). The satellite data sets use ancillary data to either explicitly or implicitly separate hydrometeor types via the freezing level height, and different freezing level assumptions could have an especially large impact on precipitating ice mass values.
The zonal means of IWP shown in Fig. 3 provide a sense of the spread in current state-of-the-art estimates of atmospheric ice. The spatial patterns of global ice mass distribution point to a relative agreement concerning the regions of frequent convection and subsidence, while the spread in magnitudes in these regions demonstrates a lack of consensus. Though this study analyses different data sets, the spread in magnitudes between satellite platforms does not appear to be appreciably better than that reported by Eliasson et al. (2011) or Waliser et al. (2009).
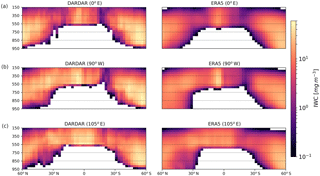
Figure 4Annual mean IWC profiles through three longitude slices centred at 0∘ E (a), 90∘ W (b), and 105∘ E (c). ERA5 values are a sum of cloud and precipitating ice. Data are limited to daytime in 2015 as before. Values below 0.1 mg m−3 are in white. The y axis is given as pressure levels in units of hPa, a convention that is maintained in Figs. 5 and 6 as well.
Comparisons of the vertical distribution of atmospheric ice are limited here to active remote sensing techniques and model data, as existing passive observations contain little to no information content regarding profiles. The analysis is thus limited to DARDAR and the two reanalyses. As the globally averaged zonal profiles of IWC have been explored in Hong and Liu (2015) and to some extent in Waliser et al. (2009), this analysis focuses on the zonal distribution of IWC along three different longitude bands (centred at 0∘ E, 105∘ E, and 90∘ W) to explore the consistency of IWC estimates across regions and regimes. The longitude slices are each 45∘ wide; e.g. the 0∘ E slice includes data from 22.5∘ E to 22.5∘ W. As with the global IWP analysis above, the data are centred on A-Train daytime observations. The DARDAR data were converted from height to pressure coordinates by assuming the international standard atmosphere.
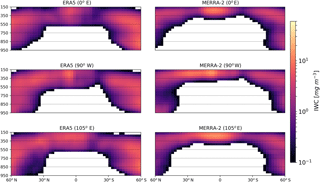
Figure 6As in Fig. 4 but for non-precipitating cloud ice from the ERA5 and MERRA-2 reanalyses. Values below 0.1 mg m−3 are in white.
Figure 4 shows the zonal mean profiles of total IWC from DARDAR and ERA5 for 2015, centred on three longitudes and displayed in pressure coordinates. The differences in IWC between ERA5 and DARDAR are given in Fig. 5 to visualize the subtler differences found in Fig. 4. Displayed differences are not especially smooth due to the limited sampling afforded by a year of DARDAR data, but some salient features are visible. In almost all regions with significant IWC values, DARDAR exhibits higher IWC. ERA5 underestimates IWC relative to DARDAR at most levels, except at pressure levels where precipitating ice dominates, including at middle levels in the deep tropics. At high latitudes some of this difference could be explained by ground clutter, which affects CloudSat measurements in the lowest kilometre of the troposphere. Another explanation for differences near the melting layer is that CloudSat attenuates in strong precipitation and may underestimate precipitating ice due to the signal being absent. Further, in middle to upper levels – essentially above the freezing level – ERA5 typically exhibits IWC values 20 % to 80 % lower than DARDAR, largely independent of IWC magnitude or region. For instance, while the Southern Ocean yields negative differences larger in magnitude than those in the subtropics, the percent difference in IWC is similar for these regions. This points to a fundamental difference in ice representation at upper levels between ERA5 and DARDAR. The consistent low bias of ERA5 with respect to DARDAR for clouds well above the freezing level signals significant and systematic differences in the treatment of cloud ice microphysics in the upper troposphere.
Whereas Fig. 4 examines total IWC, Fig. 6 offers the same view but for non-precipitating ice alone, thus allowing a direct comparison between ERA5 and MERRA-2. As mentioned in Sect. 2.2, MERRA-2 parameterizes frozen precipitation and outputs non-precipitating IWC only, precluding direct comparison with DARDAR, which senses total ice. MERRA-2 exhibits more ice near the tropopause and less at middle levels when compared to ERA5, in contrast to the general agreement on placement of total ice seen in Fig. 4. In fact, almost all MERRA-2 cloud ice occurs at less than 400 hPa, except for latitudes beyond about 45∘, whereas ERA5 shows significant IWC values at middle levels as well. Overall, MERRA-2 IWP is only about 20 % lower than ERA5 non-precipitating IWC globally (Fig. 3), but Fig. 6 shows that the vertical distribution of IWC varies significantly between MERRA-2 and ERA5 and is relatively independent of region.
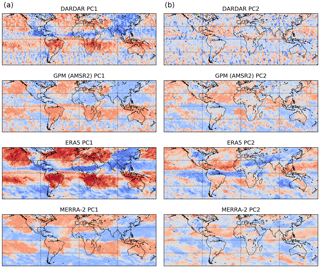
Figure 7First (a) and second (b) principal components of IWP interseasonal variability, with seasons defined as DJF, MAM, JJA, and SON. The colour scale is nominal, linear, dimensionless, of arbitrary sign, and consistent between data sets. The time period comprises December 2014 to November 2015 for GPM, ERA5, and MERRA-2; DARDAR data span 2008–2015 to improve sampling.
The atmospheric general circulation governs the distribution of clouds on long timescales, with the seasonal cycle shifting ice-laden clouds north and south to varying extents, and IWP essentially following large-scale convection (Boucher et al., 2013). To examine the degree to which the observational data sets and reanalyses agree on interseasonal variability of atmospheric ice, mean IWP was calculated for four seasons (DJF, MAM, JJA, SON). Principal component (PC) analysis is employed to differentiate the dominant modes of variability. Because of the sparse sampling of DARDAR, multiple years of data were used in an attempt to better resolve the seasonal cycle. Daytime data from 2008 to 2015 were gridded at 2.5∘ resolution as in the previous analyses. GPM (AMSR2) is the other observational data set analysed. The reanalyses are sampled in according with A-Train centred daytime observations as before.
Results from the principal component analysis are seen in Fig. 7. The gridded time series for each data set were standardized, so the PC magnitudes represent deviations that are comparable between the data sets in spite of their relative biases in IWP magnitudes. The first two PCs are shown, with PC1 representing 54 %, 48 %, 43 %, and 53 % of annual variability for DARDAR, GPM, ERA5, and MERRA-2; the second PC represents 23 %, 23 %, 14 %, and 27 % of the variability of the same data sets, but is not significantly different from the third PC for the observational data sets, as noise seems to dominate the signal.
All four data sets observe dominant modes of interseasonal variability with strikingly similar spatial patterns. As with the general pattern of annual mean IWP, there is broad agreement between observations and reanalyses regarding the seasonal movement of atmospheric ice. The first PC is most strongly defined by the gradient north of the equator, indicative of the ITCZ shifting with the season. The second PC is more difficult to interpret, though its similarity across data sets is again notable. GPM and MERRA-2 exhibit more muted seasonal signals of IWP variability when compared to DARDAR, whereas ERA5 is more pronounced. This would suggest that, while the data sets largely agree on the movement of atmospheric ice seasonally in a spatial sense, this signal differs in magnitude between data sets. For PC1 there exist a few anomalous regions for individual data sets, such as GPM in mainland Europe and northern Africa, and the reanalyses disagree with GPM and DARDAR in eastern North America. However, given the GPM emphasis on precipitating ice, this sort of discrepancy is understandable and relatively minor.
In summary, the interseasonal variability analysed in Fig. 7 displays surprisingly good agreement between observations and reanalyses, with spatial patterns that are generally well matched for the first two principal components from time series of seasonal mean IWP values. Interannual variability, e.g. ENSO, has not been controlled due to the limited sampling of DARDAR data and the limited overlap of the data sets. This analysis appears to show, however, that large-scale variability of atmospheric ice is similarly represented across models and satellite observations. The relative strength of this variability does vary, however, with ERA5 displaying stronger seasonal variations than either DARDAR or GPM.
In contrast to variability on seasonal timescales, there is no reason to expect consistent behaviour amongst the data sets when it comes to shorter timescales. Models tend to create precipitation too quickly and too lightly relative to CloudSat (King et al., 2015), and observed diurnal cycles of precipitation are not well represented (Dai, 2006). Since atmospheric ice is dominated by precipitating ice signals in many regions, most notably in ERA5, the expectation is that IWP variability on short timescales will manifest little consistency between data sets. When comparing satellite and model data, this has been found for IWC in relation to deep convection specifically (Johnston et al., 2014), as well as for clouds more generally (Yin and Porporato, 2017). Additionally, there is observational evidence that cloud ice microphysics vary diurnally (Gong et al., 2018) and that upper tropospheric ice mass varies diurnally (Eriksson et al., 2014). To analyse IWP diurnal variability, data were divided into daytime and night-time A-Train observations. While this is not ideal, as two points per day cannot resolve, say, a sinusoidal pattern in diurnal IWP variability, it is the only approach available when relying on data from sun-synchronous satellites.
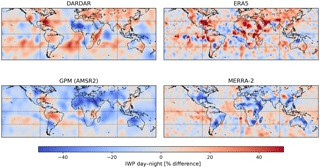
Figure 8Daytime minus night-time differences in IWP at A-Train crossing times, shown as percent differences. Fields have been smoothed to aid comparison. Due to availability, data from 2015 are used for all except DARDAR, for which the data are from 2008 to 2009.
The GPM and reanalysis data are from 2015 as in the previous sections. Due to the availability of night-time CloudSat observations, DARDAR data come from 2008–2009. The daytime and night-time data from the reanalyses are centred on 13:30 and 01:30 LST to maintain consistency with DARDAR and GPM (AMSR2) observations. Unlike in previous sections, the gridded data were smoothed before differencing. Due to limited sampling and the resultant noisy spatial patterns, the 2.5∘ gridded GPM and DARDAR data have been smoothed using a Gaussian filter of 5∘ width, while the reanalyses at 0.5∘ resolution were smoothed using a 2∘ wide Gaussian filter. This was done to aid comparison between the data sets.
Figure 8 displays daytime minus night-time differences in IWP. Unlike the similar patterns observed in Fig. 7, the diurnal cycle variations show a low degree of agreement across the data sets examined. Some common features are observed by GPM, DARDAR, and ERA5, such as increased daytime IWP over the Caribbean and Amazon, pointing to diurnally forced convection that is captured by each data set. And while some regions display behaviour common to a few of the data sets, the overall picture is one of disagreement. GPM observes more IWP at night-time over much of the Earth, mostly independent of the surface type, whereas the reanalyses see stronger land–sea differences but with opposite polarity. ERA5 witnesses noisier diurnal variability than the other data sets and often with larger magnitudes in both absolute and relative terms. As noted in Sect. 3, the means and standard deviations of ERA5 data in some regions are dominated by a few large IWP values, causing the chaotic appearance of diurnal differences seen in the equatorial eastern Pacific and the tropical Atlantic.
This study has endeavoured to assess the current state of atmospheric ice estimates and the progress made since Waliser et al. (2009). Two state-of-the-art reanalyses and five satellite data sets informed this analysis that examined mean IWP as well as the vertical distribution and temporal variability of atmospheric ice. The overall conclusion is that IWP estimates vary substantially among the various data sets. Large-scale spatial and temporal patterns are fairly consistent between estimates, but the relative magnitudes differ significantly and the analysis of diurnal variability shows limited commonality between the various estimates. By including reanalyses and analysing the diurnal and seasonal variability of atmospheric ice, this study builds on and adds to previous studies such as Eliasson et al. (2011) and Hong and Liu (2015).
Causes of the observed IWP differences are not necessarily any different from those of a decade ago, with sensors' differing sensitivities determining which parts of the IWP distribution are observed. Microphysical assumptions in any particular retrieval or model – for both ice particle habit and size distribution – are problematic to fault because of the large natural variability observed. In comparisons with in situ data, Heymsfield et al. (2017) showed that validations of satellite and model estimates of IWC depend greatly on location and regime, with two CloudSat retrievals performing very differently in low temperature ranges, surely caused by microphysical assumptions in the retrievals. It stands to reason that if IWC retrievals from one sensor can vary substantially due to microphysics, this is a predominant concern for models and other observational platforms too. That study also showed 2C-ICE underestimating mean IWC in comparison to in situ data, which may suggest that the CloudSat–CALIPSO means shown here are biased low in many cases.
Differentiating between cloud and precipitating ice remains an issue when comparing model output with observations. Deng et al. (2018) argue that the majority of IWC at all atmospheric levels measured by 2C-ICE is particles of maximum diameter less than 800 µm, which are presumably non-precipitating and might indicate that ERA5 has too much precipitating ice, since SWP dominates CIWP in most regimes for ERA5. This differentiation is important, but depends on a fall speed to designate precipitating ice, and even CloudSat–CALIPSO has very limited information on this. If the GPM estimate is taken to represent a lower bound for precipitating ice due to its sensitivity primarily to large hydrometeors, this is not too far off the magnitude of SWP in ERA5. It is troubling that the CloudSat–CALIPSO global means of estimates are driven by high IWP cases (Fig. 2), where attenuation and partitioning into ice and mixed-phase are significant potential error sources for CloudSat. If there were a systematic high bias in retrievals of very high IWP from CloudSat, then the spread between estimates would shrink considerably.
Determining the progress made since Waliser et al. (2009) or assessing the relative agreement between models and observations is dependent on how the results are framed and the metrics employed. The spatial distribution of IWP and its order of magnitude globally has moved slowly towards a consensus, undoubtedly aided by the A-Train sensors. Vertical profiles of IWC show reasonable agreement between DARDAR and ERA5 on the location of ice in the atmospheric column in a mean sense (Fig. 5). However, with differences in magnitude that approach a factor of 2 in the tropical mid-troposphere, and strong disagreements at cloud base, this comparison signals that work remains. Encouraging progress has been made overall, evidenced by increasing agreement on the spatial distribution and seasonal variability of atmospheric ice. In contrast, the near-total disagreement on diurnal variability of IWP indicates that finer scales are a concern, potentially caused by microphysics that are seen differently by different platforms. For instance, Gong et al. (2018) found that the ice particle axis ratio displays a distinct diurnal cycle over land and thus impacts polarimetric microwave measurements. These microphysical issues also affect the reanalyses examined due to their assimilation of satellite radiances, though less directly as model parameterizations also come into play.
It is difficult to pinpoint the main cause of discrepancies in IWP described herein. Figure 2 clearly shows that the data sets diverge for large IWP values, whereas overlapping areas of IWP sensitivity such as 10–100 g m−2 show comparable frequencies of occurrence. Temporal sampling is not expected to be a main driver of observed IWP discrepancies – the large differences between DARDAR, GPM (AMSR2), and MODIS (Aqua) reflect this, as they are all in the A-Train. Similarly, sensor resolution will affect such comparisons, especially for frequencies of occurrence, but the zonal mean IWP in Fig. 3 indicates that temporal sampling and sensor spatial resolution are likely secondary concerns. For instance, neither sampling nor resolution can explain the factor of 3 separating global mean IWP from GPM and SI or the factor of nearly 3 separating MODIS and DARDAR.
For the reanalyses, MERRA-2 seems to underestimate ice mass systematically, since non-precipitating ice should dominate total IWP (Deng et al., 2018). MERRA-2 also distributes cloud ice markedly differently from both DARDAR and ERA5, which may be a consequence of assimilating relatively few all-sky channels that are sensitive to scattering. ERA5 exhibits greater magnitudes of non-precipitating ice, but seems to possess both too much precipitating ice overall and high frequencies of very large IWP values (Fig. 2) that may not be physical. However, ERA5 provides what appears to be a reasonable estimate of atmospheric ice at all but near-instantaneous scales (Fig. 8), especially when considering the caveat that convective ice flux is not included in this analysis. ERA5 captures large-scale variability well in comparison with satellite estimates, and matches the vertical distribution of mean ice mass reasonably well, if not the magnitude.
For the observations, variations in sensors' sensitivity to atmospheric ice and retrieval microphysical assumptions appear most to blame for the persistent spread in IWP estimates from satellite data sets. The current Earth observing system was not optimized to sense atmospheric ice, and thus the relatively poor observational constraints on models have remained despite advances in understanding. Better constraints for modelling atmospheric ice microphysics may be aided by future multispectral microwave sensors like ICI on MetOp-SG observing at higher frequencies (Buehler et al., 2007; Jiang et al., 2017), where scattering properties are more sensitive to particle size.
The code used for all analysis given herein is available (Duncan, 2018). The data used in this study were culled from the following sources. ERA5 data constitute modified Copernicus Climate Change Service Information [2018], accessed via their Python API; GPM data come from NASA's Precipitation Processing System, accessed via http://pps.gsfc.nasa.gov (last access: 3 December 2017; GPM Science Team, 2016); SI data were accessed via University of Hamburg; MERRA-2 data were accessed via NASA's Goddard Earth Services Data and Information Services Center (GES DISC), found at https://gmao.gsfc.nasa.gov/reanalysis/MERRA-2/ (last access: 5 December 2017), specifically the datasets GMAO (2015a, b); 2C-ICE data were accessed via the CloudSat Data Processing Center (DPC) at Colorado State University, found at http://www.cloudsat.cira.colostate.edu (last access: 19 November 2017); DARDAR data were accessed via the ICARE Data and Services Center, http://www.icare.univ-lille1.fr/projects/dardar (last access: 19 November 2017); MODIS data were accessed via http://modis-atmos.gsfc.nasa.gov (last access: 19 November 2017; Platnick et al., 2015).
DID and PE conceived the study together, with DID performing the analysis and writing the manuscript.
The authors declare that they have no conflict of interest.
The authors would like to acknowledge support from the Swedish National Space
Agency. Thanks as well to the anonymous referees for helpful criticism and the
data providers upon whom studies such as this rely.
Edited by: Michael Pitts
Reviewed by: two anonymous referees
Austin, R. T., Heymsfield, A. J., and Stephens, G. L.: Retrieval of ice cloud microphysical parameters using the CloudSat millimeter-wave radar and temperature, J. Geophys. Res.-Atmos., 114, D00A23, https://doi.org/10.1029/2008JD010049, 2009. a
Baran, A. J. and Francis, P. N.: On the radiative properties of cirrus cloud at solar and thermal wavelengths: A test of model consistency using high-resolution airborne radiance measurements, Q. J. Roy. Meteor. Soc., 130, 763–778, https://doi.org/10.1256/qj.03.151, 2004. a
Bauer, P. and Schlüssel, P.: Rainfall, total water, ice water, and water vapor over sea from polarized microwave simulations and Special Sensor Microwave/Imager data, J. Geophys. Res.-Atmos., 98, 20737–20759, https://doi.org/10.1029/93JD01577, 1993. a
Bauer, P., Thorpe, A., and Brunet, G.: The quiet revolution of numerical weather prediction, Nature, 525, 47–55, https://doi.org/10.1038/nature14956, 2015. a
Birman, C., Mahfouf, J. F., Milz, M., Mendrok, J., Buehler, S. A., and Brath, M.: Information content on hydrometeors from millimeter and sub-millimeter wavelengths, Tellus A, 69, 1271562, https://doi.org/10.1080/16000870.2016.1271562, 2017. a, b
Boucher, O., Randall, D., Artaxo, P., Bretherton, C., Feingold, G., Forster, P., Kerminen, V.-M., Kondo, Y., Liao, H., Lohmann, U., Rasch, P., Satheesh, S. K., Sherwood, S., Stevens, B., and Zhang, X.-Y.: Clouds and aerosols, in: Climate change 2013: the physical science basis. Contribution of Working Group I to the Fifth Assessment Report of the Intergovernmental Panel on Climate Change, 571–657, Cambridge University Press, Cambridge, UK and New York, USA, 2013. a
Brath, M., Fox, S., Eriksson, P., Harlow, R. C., Burgdorf, M., and Buehler, S. A.: Retrieval of an ice water path over the ocean from ISMAR and MARSS millimeter and submillimeter brightness temperatures, Atmos. Meas. Tech., 11, 611–632, https://doi.org/10.5194/amt-11-611-2018, 2018. a
Buehler, S. A., Jiménez, C., Evans, K. F., Eriksson, P., Rydberg, B., Heymsfield, A. J., Stubenrauch, C. J., Lohmann, U., Emde, C., John, V. O., Sreerekha, T. R., and Davis, C. P.: A concept for a satellite mission to measure cloud ice water path, ice particle size, and cloud altitude, Q. J. Roy. Meteor. Soc., 133, 109–128, https://doi.org/10.1002/qj.143, 2007. a, b, c, d
Dai, A.: Precipitation characteristics in eighteen coupled climate models, J. Climate, 19, 4605–4630, 2006. a
Delanoë, J. and Hogan, R. J.: A variational scheme for retrieving ice cloud properties from combined radar, lidar, and infrared radiometer, J. Geophys. Res.-Atmos., 113, D07204, https://doi.org/10.1029/2007JD009000, 2008. a, b
Deng, M., Mace, G. G., Wang, Z., and Okamoto, H.: Tropical Composition, Cloud and Climate Coupling Experiment validation for cirrus cloud profiling retrieval using CloudSat radar and CALIPSO lidar, J. Geophys. Res.-Atmos., 115, 1014–1030, 2010. a, b
Deng, M., Mace, G. G., Wang, Z., and Lawson, R. P.: Evaluation of several A-Train ice cloud retrieval products with in situ measurements collected during the SPARTICUS campaign, J. Appl. Meteorol. Clim., 52, 1014–1030, https://doi.org/10.1175/JAMC-D-12-054.1, 2013. a
Deng, M., Mace, G. G., Wang, Z., Li, J.-L. F., and Luo, Y.: Partitioning Ice Water Content from Retrievals and Its Application in Model Comparison, J. Atmos. Sci., 75, 1105–1120, https://doi.org/10.1175/JAS-D-17-0017.1, 2018. a, b
Duncan, D. I.: An update on global atmospheric ice estimates from observations and reanalyses, Zenodo, https://doi.org/10.5281/zenodo.1342367, 2018. a
Eliasson, S., Buehler, S. A., Milz, M., Eriksson, P., and John, V. O.: Assessing observed and modelled spatial distributions of ice water path using satellite data, Atmos. Chem. Phys., 11, 375–391, https://doi.org/10.5194/acp-11-375-2011, 2011. a, b, c, d
Eriksson, P., Ekström, M., Rydberg, B., Wu, D. L., Austin, R. T., and Murtagh, D. P.: Comparison between early Odin-SMR, Aura MLS and CloudSat retrievals of cloud ice mass in the upper tropical troposphere, Atmos. Chem. Phys., 8, 1937–1948, https://doi.org/10.5194/acp-8-1937-2008, 2008. a
Eriksson, P., Rydberg, B., Sagawa, H., Johnston, M. S., and Kasai, Y.: Overview and sample applications of SMILES and Odin-SMR retrievals of upper tropospheric humidity and cloud ice mass, Atmos. Chem. Phys., 14, 12613–12629, https://doi.org/10.5194/acp-14-12613-2014, 2014. a
Evans, K. F., Wang, J. R., O'C Starr, D., Heymsfield, G., Li, L., Tian, L., Lawson, R. P., Heymsfield, A. J., and Bansemer, A.: Ice hydrometeor profile retrieval algorithm for high-frequency microwave radiometers: application to the CoSSIR instrument during TC4, Atmos. Meas. Tech., 5, 2277–2306, https://doi.org/10.5194/amt-5-2277-2012, 2012. a
Geer, A., Baordo, F., Bormann, N., Chambon, P., English, S., Kazumori, M., Lawrence, H., Lean, P., Lonitz, K., and Lupu, C.: The growing impact of satellite observations sensitive to humidity, cloud and precipitation, Q. J. Roy. Meteor. Soc., 143, 3189–3206, https://doi.org/10.1002/qj.3172, 2017. a, b
Geer, A. J., Lonitz, K., Weston, P., Kazumori, M., Okamoto, K., Zhu, Y., Liu, E. H., Collard, A., Bell, W., Migliorini, S., Chambon, P., Fourrié, N., Kim, M.-J., Köpken-Watts, C., and Schraff, C.: All-sky satellite data assimilation at operational weather forecasting centres, Q. J. Roy. Meteor. Soc., https://doi.org/10.1002/qj.3202, accepted, 2018. a, b
Gelaro, R., McCarty, W., Suárez, M. J., Todling, R., Molod, A., Takacs, L., Randles, C. A., Darmenov, A., Bosilovich, M. G., Reichle, R., Wargan, K., Coy, L., Cullather, R., Draper, C., Akella, S., Buchard, V., Conaty, A., da Silva, A. M., Gu, W., Kim, G. K., Koster, R., Lucchesi, R., Merkova, D., Nielsen, J. E., Partyka, G., Pawson, S., Putman, W., Rienecker, M., Schubert, S. D., Sienkiewicz, M., and Zhao, B.: The modern-era retrospective analysis for research and applications, version 2 (MERRA-2), J. Climate, 30, 5419–5454, https://doi.org/10.1175/JCLI-D-16-0758.1, 2017. a
Global Modeling and Assimilation Office (GMAO): MERRA-2 inst3_3d_asm_Np: 3d,3-Hourly,Instantaneous,Pressure-Level,Assimilation,Assimilated Meteorological Fields V5.12.4, Greenbelt, MD, USA, Goddard Earth Sciences Data and Information Services Center (GES DISC), https://doi.org/10.5067/QBZ6MG944HW0, 2015a. a
Global Modeling and Assimilation Office (GMAO): MERRA-2 inst1_2d_int_Nx: 2d,1-Hourly,Instantaneous,Single-Level,Assimilation,Vertically Integrated Diagnostics V5.12.4, Greenbelt, MD, USA, Goddard Earth Sciences Data and Information Services Center (GES DISC), https://doi.org/10.5067/G0U6NGQ3BLE0, 2015b. a
Gong, J. and Wu, D. L.: CloudSat-constrained cloud ice water path and cloud top height retrievals from MHS 157 and 183.3 GHz radiances, Atmos. Meas. Tech., 7, 1873–1890, https://doi.org/10.5194/amt-7-1873-2014, 2014. a
Gong, J., Zeng, X., Wu, D. L., and Li, X.: Diurnal Variation of Tropical Ice Cloud Microphysics: Evidence from Global Precipitation Measurement Microwave Imager (GPM-GMI) Polarimetric Measurements, Geophys. Res. Lett., 45, 1185–1193, https://doi.org/10.1002/2017GL075519, 2018. a, b, c
GPM Science Team: GPM AMSR-2 on GCOM-W1 (GPROF) Radiometer Precipitation Profiling L2A 1.5 hours 10 km V05, Greenbelt, MD, Goddard Earth Sciences Data and Information Services Center (GES DISC), https://doi.org/10.5067/GPM/AMSR2/GCOMW1/GPROF/2A/05 (last accessed: 20 December 2017), 2016. a, b
Heymsfield, A. J., Protat, A., Austin, R. T., Bouniol, D., Hogan, R. J., Delanoe, J., Okamoto, H., Sato, K., van Zadelhoff, G. J., Donovan, D. P., and Wang, Z.: Testing IWC retrieval methods using radar and ancillary measurements with in situ data, J. Appl. Meteorol. Clim., 35, 135–163, https://doi.org/10.1175/2007JAMC1606.1, 2008. a
Heymsfield, A., Krämer, M., Wood, N. B., Gettelman, A., Field, P. R., and Liu, G.: Dependence of the ice water content and snowfall rate on temperature, globally: Comparison of in situ observations, satellite active remote sensing retrievals, and global climate model simulations, J. Appl. Meteorol. Clim., 56, 189–215, https://doi.org/10.1175/JAMC-D-16-0230.1, 2017. a
Holl, G., Eliasson, S., Mendrok, J., and Buehler, S.: SPARE-ICE: Synergistic ice water path from passive operational sensors, J. Geophys. Res.-Atmos., 119, 1504–1523, 2014. a, b, c
Hong, Y. and Liu, G.: The characteristics of ice cloud properties derived from CloudSat and CALIPSO measurements, J. Climate, 28, 3880–3901, https://doi.org/10.1175/JCLI-D-14-00666.1, 2015. a, b, c
Hou, A. Y., Kakar, R. K., Neeck, S., Azarbarzin, A. A., Kummerow, C. D., Kojima, M., Oki, R., Nakamura, K., and Iguchi, T.: The Global Precipitation Measurement Mission, B. Am. Meteorol. Soc., 95, 701–722, https://doi.org/10.1175/BAMS-D-13-00164.1, 2014. a, b
Hu, Y., Rodier, S., Xu, K.-m., Sun, W., Huang, J., Lin, B., Zhai, P., and Josset, D.: Occurrence, liquid water content, and fraction of supercooled water clouds from combined CALIOP/IIR/MODIS measurements, J. Geophys. Res.-Atmos., 115, D00H34, https://doi.org/10.1029/2009JD012384, 2010. a
Huang, J., Minnis, P., Lin, B., Yi, Y., Fan, T. F., Sun-Mack, S., and Ayers, J. K.: Determination of ice water path in ice-over-water cloud systems using combined MODIS and AMSR-E measurements, Geophys. Res. Lett., 33, L21801, https://doi.org/10.1029/2006GL027038, 2006. a
Hubanks, P., Platnick, S., King, M., and Ridgway, B.: MODIS Atmosphere L3 gridded product algorithm theoretical basis document (ATBD) & users guide, Collection 006, Version 4.2, Tech. rep., NASA, available at: http://modis-atmos.gsfc.nasa.gov/MOD08_M3/atbd.html (last access: 14 January 2018), 2016. a
Jensen, E. J., Toon, O. B., Selkirk, H. B., Spinhirne, J. D., and Schoeberl, M. R.: On the formation and persistence of subvisible cirrus clouds near the tropical tropopause, J. Geophys. Res.-Atmos., 101, 21361–21375, https://doi.org/10.1029/95JD03575, 1996. a
Jiang, J. H., Su, H., Zhai, C., Perun, V. S., Del Genio, A., Nazarenko, L. S., Donner, L. J., Horowitz, L., Seman, C., Cole, J., Gettelman, A., Ringer, M. A., Rotstayn, L., Jeffrey, S., Wu, T., Brient, F., Dufresne, J.-L., Kawai, H., Koshiro, T., Watanabe, M., L'Ecuyer, T. S., Volodin, E. M., Iversen, T., Drange, H., Mesquita, M. D. S., Read, W. G., Waters, J. W., Tian, B., Teixeira, J., and Stephens, G. L.: Evaluation of cloud and water vapor simulations in CMIP5 climate models using NASA “A-Train” satellite observations, J. Geophys. Res.-Atmos., 117, D14105, https://doi.org/10.1029/2011JD017237, 2012. a, b, c
Jiang, J. H., Yue, Q., Su, H., Reising, S. C., Kangaslahti, P. P., Deal, W. R., Schlecht, E. T., Wu, L., and Evans, K. F.: A simulation of ice cloud particle size, humidity, and temperature measurements from the TWICE CubeSat, Earth Space Sci., 4, 574–587, https://doi.org/10.1002/2017EA000296, 2017. a
Johnston, M. S., Eliasson, S., Eriksson, P., Forbes, R. M., Gettelman, A., Räisänen, P., and Zelinka, M. D.: Diagnosing the average spatio-temporal impact of convective systems – Part 2: A model intercomparison using satellite data, Atmos. Chem. Phys., 14, 8701–8721, https://doi.org/10.5194/acp-14-8701-2014, 2014. a
King, J. M., Kummerow, C. D., Van Den Heever, S. C., and Igel, M. R.: Observed and modeled warm rainfall occurrence and its relationships with cloud macrophysical properties, J. Atmos. Sci., 72, 4075–4090, 2015. a
Kulie, M. S., Milani, L., Wood, N. B., Tushaus, S. A., Bennartz, R., and L'Ecuyer, T. S.: A Shallow Cumuliform Snowfall Census Using Spaceborne Radar, J. Hydrometeorol., 17, 1261–1279, https://doi.org/10.1175/JHM-D-15-0123.1, 2016. a, b
Kummerow, C. D., Randel, D. L., Kulie, M., Wang, N.-Y., Ferraro, R., Joseph Munchak, S., and Petkovic, V.: The evolution of the Goddard profiling algorithm to a fully parametric scheme, J. Atmos. Ocean. Tech., 32, 2265–2280, https://doi.org/10.1175/JTECH-D-15-0039.1, 2015. a
Lin, B. and Rossow, W. B.: Observations of cloud liquid water path over oceans: Optical and microwave remote sensing methods, J. Geophys. Res.-Atmos., 99, 20907–20927, 1994. a
Masunaga, H., Matsui, T., kuo Tao, W., Hou, A. Y., Kummerow, C. D., Nakajima, T., Bauer, P., Olson, W. S., Sekiguchi, M., and Nakajima, T. Y.: Satellite Data Simulator Unit, B. Am. Meteorol. Soc., 91, 1625–1632, https://doi.org/10.1175/2010BAMS2809.1, 2010. a
McCarty, W., Coy, L., Gelaro, R., Huang, A., Merkova, D., Smith, E., Sienkiewicz, M., and Wargan, K.: MERRA-2 input observations: Summary and assessment, NASA Tech. Rep. Series on Global Modeling and Data Assimilation, NASA/TM-2016-104606, Greenbelt, MD, USA, available at: https://gmao.gsfc.nasa.gov/pubs/docs/McCarty885.pdf (last access: 14 January 2018), 2016. a
Platnick, S., King, M. D., Ackerman, S. A., Menzel, W. P., Baum, B. A., Riedi, J. C., and Frey, R. A.: The MODIS cloud products: algorithms and examples from Terra, IEEE T. Geosci. Remote, 41, 459–473, https://doi.org/10.1109/TGRS.2002.808301, 2003. a, b
Platnick, S., King, M., and Hubanks, P.: MODIS Atmosphere L3 Daily Product, NASA MODIS Adaptive Processing System, Goddard Space Flight Center, https://doi.org/10.5067/MODIS/MYD08_D3.006, 2015. a, b
Platnick, S., Meyer, K. G., King, M. D., Wind, G., Amarasinghe, N., Marchant, B., Arnold, G. T., Zhang, Z., Hubanks, P. A., Holz, R. E., Yang, P., Ridgway, W. L., and Riedi, J.: The MODIS Cloud Optical and Microphysical Products: Collection 6 Updates and Examples From Terra and Aqua, IEEE T. Geosci. Remote, 55, 502–525, https://doi.org/10.1109/TGRS.2016.2610522, 2017. a, b
Sassen, K., Wang, Z., and Liu, D.: Global distribution of cirrus clouds from CloudSat/cloud-aerosol lidar and infrared pathfinder satellite observations (CALIPSO) measurements, J. Geophys. Res.-Atmos., 113, D00A12, https://doi.org/10.1029/2008JD009972, 2009. a
Stephens, G. L., Vane, D. G., Boain, R. J., Mace, G. G., Sassen, K., Wang, Z., Illingworth, A. J., O'Connor, E. J., Rossow, W. B., Durden, S. L., Miller, S. D., Austin, R. T., Benedetti, A., Mitrescu, C., and the CloudSat Science Team: The CloudSat mission and the A-Train, B. Am. Meteorol. Soc., 83, 1771–1790, https://doi.org/10.1175/BAMS-83-12-1771, 2002. a
Stephens, G., Winker, D., Pelon, J., Trepte, C., Vane, D., Yuhas, C., L'Ecuyer, T., and Lebsock, M.: CloudSat and CALIPSO within the A-Train: Ten years of Actively Observing the Earth System, B. Am. Meteorol. Soc., 99, 569–581, https://doi.org/10.1175/BAMS-D-16-0324.1, 2018. a, b
Strandgren, J., Bugliaro, L., Sehnke, F., and Schröder, L.: Cirrus cloud retrieval with MSG/SEVIRI using artificial neural networks, Atmos. Meas. Tech., 10, 3547–3573, https://doi.org/10.5194/amt-10-3547-2017, 2017. a
Sun, N. and Weng, F.: Retrieval of cloud ice water path from Special Sensor Microwave Imager/Sounder (SSMIS), J. Appl. Meteorol. Clim., 51, 366–379, https://doi.org/10.1175/JAMC-D-11-021.1, 2012. a
Vonder Haar, T. H. and Suomi, V. E.: Measurements of the earth's radiation budget from satellites during a five-year period. Part I: Extended time and space means, J. Atmos. Sci., 28, 305–314, https://doi.org/10.1175/1520-0469(1971)028<0305:MOTERB>2.0.CO;2, 1971. a
Waliser, D. E., Li, J. L. F., Woods, C. P., Austin, R. T., Bacmeister, J., Chern, J., Del Genio, A., Jiang, J. H., Kuang, Z., Meng, H., Minnis, P., Platnick, S., Rossow, W. B., Stephens, G. L., Sun-Mack, S., Tao, W. K., Tompkins, A. M., Vane, D. G., Walker, C., and Wu, D.: Cloud ice: A climate model challenge with signs and expectations of progress, J. Geophys. Res. Atmos., 114, D00A21, https://doi.org/10.1029/2008JD010015, 2009. a, b, c, d, e, f, g, h, i, j
Weng, F. and Grody, N. C.: Retrieval of ice cloud parameters using a microwave imaging radiometer, J. Atmos. Sci., 57, 1069–1081, https://doi.org/10.1175/1520-0469(2000)057<1069:ROICPU>2.0.CO;2, 2000. a
Wielicki, B. A., Harrison, E. F., Cess, R. D., King, M. D., and Randall, D. A.: Mission to planet Earth: Role of clouds and radiation in climate, B. Am. Meteorol. Soc., 76, 2125–2153, https://doi.org/10.1175/1520-0477(1995)076<2125:MTPERO>2.0.CO;2, 1995. a
Wu, D. L., Austin, R. T., Deng, M., Durden, S. L., Heymsfield, A. J., Jiang, J. H., Lambert, A., Li, J. L., Livesey, N. J., McFarquhar, G. M., Pittman, J. V., Stephens, G. L., Tanelli, S., Vane, D. G., and Waliser, D. E.: Comparisons of global cloud ice from MLS, CloudSat, and correlative data sets, J. Geophys. Res.-Atmos., 114, D00A24, https://doi.org/10.1029/2008JD009946, 2009. a
Yin, J. and Porporato, A.: Diurnal cloud cycle biases in climate models, Nat. Commun., 8, 2269, https://doi.org/10.1038/s41467-017-02369-4, 2017. a
Zhang, Y., Macke, A., and Albers, F.: Effect of crystal size spectrum and crystal shape on stratiform cirrus radiative forcing, Atmos. Res., 52, 59–75, https://doi.org/10.1016/S0169-8095(99)00026-5, 1999. a
Zhao, L. and Weng, F.: Retrieval of ice cloud parameters using the Advanced Microwave Sounding Unit, J. Appl. Meteorol., 41, 384–395, https://doi.org/10.1175/1520-0450(2002)041<0384:ROICPU>2.0.CO;2, 2002. a