the Creative Commons Attribution 4.0 License.
the Creative Commons Attribution 4.0 License.
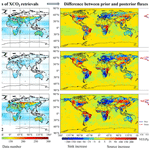
The role of OCO-3 XCO2 retrievals in estimating global terrestrial net ecosystem exchanges
Xingyu Wang
Hengmao Wang
Zhengqi Zhang
Mousong Wu
Wei He
Weimin Ju
Jing M. Chen
Satellite-based column-averaged dry-air CO2 mole fraction (XCO2) retrievals are frequently used to improve the estimates of terrestrial net ecosystem exchanges (NEEs). The Orbiting Carbon Observatory 3 (OCO-3) satellite, launched in May 2019, was designed to address important questions about the distribution of carbon fluxes on Earth, but its role in estimating global terrestrial NEE remains unclear. Here, using the Global Carbon Assimilation System, version 2, we investigate the impact of OCO-3 XCO2 on the estimation of global NEE by assimilating the OCO-3 XCO2 retrievals alone and in combination with the OCO-2 XCO2 retrievals. The results show that when only the OCO-3 XCO2 is assimilated (Exp_OCO3), the estimated global land sink is significantly lower than that from the OCO-2 experiment (Exp_OCO2). The estimate from the joint assimilation of OCO-3 and OCO-2 (Exp_OCO3&2) is comparable on a global scale to that of Exp_OCO2. However, there are significant regional differences. Compared to the observed global annual CO2 growth rate, Exp_OCO3 has the largest bias and Exp_OCO3&2 shows the best performance. Furthermore, validation with independent CO2 observations shows that the biases of Exp_OCO3 are significantly larger than those of Exp_OCO2 and Exp_OCO3&2 at middle and high latitudes. The reasons for the poor performance of assimilating OCO-3 XCO2 alone include the lack of observations beyond 52° S and 52° N, the large fluctuations in the number of data, and the varied observation time. Our study indicates that assimilating OCO-3 XCO2 retrievals alone leads to an underestimation of land sinks at high latitudes and that a joint assimilation of OCO-2 XCO2 and the OCO-3 XCO2 retrievals observed in the afternoon is required for a better estimation of global terrestrial NEE.
- Article
(6275 KB) - Full-text XML
-
Supplement
(848 KB) - BibTeX
- EndNote
The rising carbon dioxide (CO2) concentration in the Earth's atmosphere in recent decades, which is mainly caused by human activities, such as the burning of fossil fuels, deforestation, and land-use change, has become a global concern (Hansen et al., 2013). Terrestrial ecosystems and oceans together absorb about 56 % of anthropogenic CO2 emissions (Friedlingstein et al., 2023). Among them, terrestrial ecosystems play a crucial role in regulating the atmospheric CO2 concentration. However, the carbon uptake capacity of terrestrial ecosystems varies considerably globally and regionally (Bousquet et al., 2000; Takahashi et al., 2009; Piao et al., 2020). Therefore, accurate quantification of global and regional terrestrial net ecosystem exchange (NEE) is very important to understand these ecosystems' role and potential in regulating changes in the atmospheric CO2 concentration.
Atmospheric inversion is a major method for estimating surface carbon fluxes from observations of atmospheric CO2 concentration (Enting and Newsam, 1990; Gurney et al., 2002; Thompson et al., 2016; Jiang et al., 2021), but it is more effective at the global scale than at the regional scale. A large number of previous studies have shown that different atmospheric inversion models can produce relatively consistent global estimates of carbon fluxes, but their performance at regional scales is variable. In regions such as the tropics, Southern Hemisphere oceans, and most continental interiors (South America, Africa, and boreal Asia), the reliability of atmospheric inversions varies considerably due to the heterogeneous distribution of in situ observations, leading to an increase in the uncertainty in carbon flux estimates (Peylin et al., 2013; Wang et al., 2019). The use of satellite observations to constrain atmospheric inversions can be effective in improving carbon flux estimates because of their better spatial coverage (Basu et al., 2013; Byrne et al., 2020; Jiang et al., 2021; Wang et al., 2022; He et al., 2023a). The National Aeronautics and Space Administration (NASA) launched the Orbiting Carbon Observatory 2 (OCO-2) satellite in 2014 (Crisp et al., 2017; Eldering et al., 2012, 2017), followed by the Orbiting Carbon Observatory 3 (OCO-3) satellite in 2019 (Eldering et al., 2019; Taylor et al., 2020). The OCO satellites have a high sensitivity to the column-averaged dry-air CO2 mole fraction (XCO2), a fine footprint, and good spatial coverage, and they can therefore be used to better constrain surface carbon flux estimates. In previous studies, many atmospheric inversion models have used the XCO2 from the OCO-2 satellite to estimate global (e.g. Crowell et al., 2019; Peiro et al., 2022; Byrne et al., 2023) and regional (e.g. Palmer et al., 2019; Byrne et al., 2021; Philip et al., 2022; He et al., 2022; He et al., 2023a) surface carbon fluxes. For example, Miller et al. (2018) evaluated the effectiveness of OCO-2 observations in constraining regional biospheric CO2 fluxes. Their findings indicate that OCO-2 observations are most effective at continental and hemispheric scales. Byrne et al. (2022) utilized OCO-2 data to fill a gap in station observations at high latitudes. Their study confirmed the presence of significant and widely distributed early cold-season CO2 emissions in the northeastern region of Eurasia. Furthermore, several studies have utilized OCO-2 XCO2 data to investigate the impact of climate extremes on terrestrial NEE, such as El Niño (e.g. Liu et al., 2017) and droughts (He et al., 2023b; Chen et al., 2024). OCO-3 introduces new technologies and observational methods to monitor CO2 on Earth, offering the same spatial resolution as OCO-2. It is aimed at detecting mid-latitude regions where human CO2 emissions are concentrated. However, few studies have used the OCO-3 XCO2 retrievals to constrain global and regional surface carbon fluxes until now. Therefore, it is important to investigate the impact of assimilating OCO-3 observations on the estimates of global and terrestrial carbon sinks.
In this study, we used both OCO-2 and OCO-3 XCO2 retrievals to invert global and regional carbon fluxes for the period of 2020–2022 with the Global Carbon Assimilation System, version 2 (GCASv2) (Jiang et al., 2021). The XCO2 retrievals from OCO-2 and OCO-3 were assimilated separately and together in order to disentangle the effect of OCO-3 XCO2 retrievals on the estimates of global and regional terrestrial carbon sinks.
2.1 Inversion method
The Global Carbon Assimilation System, version 2 (GCASv2) (Jiang et al., 2021; Wang et al., 2021), designed primarily for assimilating satellite XCO2 retrievals, was adopted in this study to invert surface carbon fluxes. The system uses the Model for Ozone and Related chemical Tracers, version 4 (MOZART-4; Emmons et al., 2010) to simulate three-dimensional atmospheric CO2 concentrations and an ensemble square root filter (EnSRF; Whitaker and Hamill, 2002) to implement the inversion of surface fluxes. MOZART-4 is an offline global chemical transport model developed by the National Center for Atmospheric Research (NCAR). It can be driven by essentially any meteorological dataset and with any emissions inventory, so there is not a unique standard simulation (Emmons et al., 2010). We turned off all gas-phase, heterogeneous chemical reactions and aerosol and deposition processes in the MOZART-4 model and added a corresponding number of CO2 tracers according to the ensemble number in GCASv2 in order to allow the model to run more quickly. EnSRF assimilates observations in a sequential way and obviates the need to perturb the observations. It shows good performance as long as the observation errors are uncorrelated (Houtekamer and Mitchell, 2001). GCASv2 is an upgrade of GCAS (Zhang et al., 2015), the latter of which was established in 2015. The main upgrades include (1) the addition of an assimilation module for satellite observations, (2) a change in the assimilation algorithm (i.e. EnSRF), (3) a change in the operational flow of the assimilation system, (4) the addition of a “super-observation” scheme, (5) inversion of fluxes at the grid scale, and (6) an improvement in the localization scheme.
GCASv2 runs cyclically, with a two-step optimization strategy in each assimilation window (1 week). In the first step, the prior fluxes () in each grid are independently perturbed with a random number (δi) drawn from a Gaussian distribution that has a mean of 0 and standard deviation of 1 and with a scaling factor (λ) that represents the uncertainty in each prior flux (Eq. 1).
Then the perturbed fluxes are put into the MOZART-4 model to simulate ensembles of CO2 concentrations. The CO2 profiles are sampled according to the locations and times of XCO2 observations and converted into the simulated ensembles of XCO2 (XCO) according to prior XCO2 (XCO), prior XCO2 profiles (ya,j), a pressure weighting function (hj), and the averaging kernel (aj) of the XCO2 retrievals (Eq. 2).
Subsequently, the perturbed fluxes (), the simulated XCO2 ensembles, and the observed XCO2 (y) are used in EnSRF to optimize the carbon fluxes () (Eqs. 3–5). The background error covariance matrix (Pb) is calculated based on according to Eq. (3), where is the mean of . The posterior flux () is a correction to the prior flux using the bias between simulated and observed XCO2 () and the Kalman gain matrix (K) (Eq. 4). K is calculated according to Eq. (5), which is a function of the model–data mismatch error covariance matrix (R) and the background error covariance matrix.
In the second step, the optimized carbon fluxes are put into the MOZART-4 model to obtain the initial field of the next assimilation window. This scheme allows the compensation of inversion results between neighbouring windows and mass conservation between flux adjustments and concentration changes.
In order to reduce the effects of horizontal observation error correlation and representativeness error, based on the optimal estimation theory (Miyazaki et al., 2012), the system also performs a super-observation scheme, which combines multiple observations located within the same model grid into a single high-precision super-observation. In this method, it first calculates the simulated XCO2 corresponding to each observed XCO2 based on the observation time and location, and then it performs a retrieval error-weighted average for all the simulated and observed XCO2, respectively, falling within the same model grid in the data assimilation window.
There are inevitably spurious correlations in the ensemble Kalman filter (EnKF) method; to reduce the effect of spurious correlations, a two-layer localization scale was adopted in GCASv2, which is used to select which observations can be used for the flux analysis for each grid. The localization technique is based on the correlation coefficient between the simulated XCO2 ensembles (XCO) in each observation location and the perturbed fluxes () in the current model grids and their distances. The observations will be accepted for assimilation if the distance is less than 500 km and the correlation coefficient is greater than 0 and if the distance is greater than or equal to 500 km and less than 3000 km and the correlation coefficient is significant (p<0.05). Otherwise, the observations are not accepted. The reason for this scheme is that considering the atmospheric horizontal diffusion, we believe that there must be a correlation between the flux of one grid and the concentrations in its neighbouring grids, and therefore observations are accepted as long as this correlation coefficient is greater than zero. In contrast, at distant locations (>500 km), where the effect of atmospheric horizontal diffusion is essentially negligible, the relationship between source and receptor is mainly due to atmospheric transport, and in order to minimize spurious correlations, we require that such correlations must be significant. More details of the system can be found in Jiang et al. (2021).
2.2 OCO-2 and OCO-3 XCO2 retrievals
In July 2014, the Orbiting Carbon Observatory 2 (OCO-2) satellite was launched by NASA with the primary objective of providing accurate space-based measurements to quantify changes in XCO2. The satellite is equipped with three high-resolution spectrometers that can detect two near-infrared wavelength bands (1.61 and 2.06 µm) of sunlight reflectance spectra to observe CO2. In May 2019, NASA launched OCO-3, mounted to the International Space Station (ISS) to detect CO2 in mid-latitudes, where human emissions are more concentrated. OCO-3 operates in a low-inclination orbit from 52° S to 52° N and is equipped with three high-resolution spectrometers, providing the same spatial resolutions as and an observation mode similar to the OCO-2 satellite (Taylor et al., 2023). However, since OCO-3 is mounted on the ISS, its observation time and frequency for the same place are different from those of OCO-2.
The XCO2 data from OCO-3 and OCO-2 used in this study are bias-corrected products from August 2019 to December 2022 at the image element level. The data are sourced from Version 10.4r Level 2 Lite and Version 11.1r Level 2 Lite, respectively. Before using them in our inversion system, it is essential to pre-process the data. First, both the land (land nadir + land glint, LNLG) and ocean (ocean glint, OG) retrievals were adopted, and they were filtered using the parameter of XCO2_quality_flag, which indicates the quality of the data. Only data with XCO2_quality_flag=0 were selected for assimilation in this study. Then the LNLG and OG retrievals and their corresponding retrieval parameters (namely XCO, ya,j, hj, and aj in Eq. 2) were re-gridded to a spatial resolution of 1° × 1° and 5° × 5°, respectively, using the arithmetic averaging method. For the OG data, we used a coarser re-gridding resolution because the distribution of XCO2 is more homogeneous on sea than on land. Finally, both OCO-3 and OCO-2 XCO2 retrievals were converted into the X2019 scale of the World Meteorological Organization (WMO) following Hall et al. (2021). Figure 1a and c display the distribution and coverage of screened OCO-3 and OCO-2 XCO2 retrievals from 2020 to 2022. Compared to OCO-2, OCO-3 has more observational data in the mid-latitudes of the Northern Hemisphere and Southern Hemisphere, especially in arid and semi-arid regions.
Following Jiang et al. (2022), the model–data mismatch errors were amplified by a factor on top of the XCO2 posterior errors but with the minimum observation error setting at 1 ppm. It needs to be noted that in the OCO-3 and OCO-2 products, the XCO2 posterior errors in OG retrievals (0.48±0.11 and 0.51±0.15 ppm in 2020 for OCO-2 and OCO-3, respectively) are smaller than those for LNLG (0.54±0.12 and 0.64±0.18 ppm in 2020 for OCO-2 and OCO-3, respectively), but in fact, the observational error should be greater at sea than on land (Peiro et al., 2022). Therefore, before multiplying by a uniform factor, we increased the XCO2 posterior errors in OG retrievals by 0.2 ppm. Taylor et al. (2023) reported that the means of the uncertainties for the OCO-2 and OCO-3 quality-filtered and bias-corrected XCO2 are 1.0 and 1.3 ppm, respectively. Considering that the global atmospheric transport model may have an uncertainty of about 1.0 ppm (Lauvaux et al., 2009), in this study, we thus set the amplification factor to be 3.5. Through this treatment, the mean model–data mismatch errors in LNLG and OG are about 1.9 and 2.4 ppm for OCO-2 and 2.3 and 2.5 ppm for OCO-3, respectively.
2.3 Prior carbon fluxes
There are four prior carbon fluxes used in this study, which are terrestrial NEE, ocean–atmosphere (OCN) carbon exchanges, fossil fuel and cement production (FOSSIL) carbon emissions, and biomass combustion (FIRE) carbon emissions. NEE was simulated using the BEPS model (Chen et al., 2019). The OCN fluxes were derived from the mean of the JMA Ocean CO2 Map (Iida et al., 2021), which contains a global product with 1° × 1° resolution (Globe, v2022) and another product for the Northwest Pacific region with a resolution of 0.25° × 0.25° (The western North Pacific, v2023). These two products were integrated before they were used in this study. The FOSSIL carbon emissions were obtained from GCP-GridFEDv2023.1 (Jones et al., 2021), which contains monthly global carbon emissions from fossil fuels, cement production, and cement product weathering carbon sequestration at a spatial resolution of 0.1° × 0.1°. The FIRE carbon emissions were obtained directly from the Global Fire Emissions Database, Version 4.1 (GFED4.1s; Randerson et al., 2017). All four prior fluxes cover the entire time period of this study (i.e. August 2019 to December 2022), and they were re-gridded to a unified spatial resolution of 1° × 1° before being used in the GCASv2 system.
2.4 Evaluation data and methods
Due to the significant spatial-scale discrepancy between the inverted fluxes and the in situ-observed fluxes, direct validation of the posterior net ecosystem exchange (NEE) using observed data is typically unattainable. However, we are able to indirectly evaluate the posterior fluxes by comparing the atmospheric CO2 concentrations, simulated with the posterior fluxes, against independent CO2 measurements. (e.g. Jin et al., 2018; Wang et al., 2019; Feng et al., 2020; Jiang et al., 2021). In this study, we used surface flask observations at 66 sites from the ObsPack dataset (CO2 GLOBALVIEWplus v9.1 ObsPack; Schuldt et al., 2023) to independently assess the posterior fluxes. The screening of the 66 sites followed the methodology of Jiang et al. (2022). The distribution of the 66 flask sites is shown in Fig. 2. The specific metrics assessed were the statistics of mean bias (BIAS), mean absolute error (MAE), and root mean square error (RMSE). We calculated annual BIAS, MAE, and RMSE globally for different latitudinal zones and for different land areas.
The GCASv2 system was run from 1 August 2019 to 31 December 2022. The initial 5 months was designated as the spin-up stage, and the results from January 2020 to December 2022 were analysed in this study. Three inversion experiments were conducted: (1) assimilation of OCO-3 XCO2 (all inversion experiments use OG+LNLG data) retrievals alone (Exp_OCO3), (2) assimilation of OCO-2 XCO2 retrievals alone (Exp_OCO2), and (3) simultaneous assimilation of OCO-3 and OCO-2 XCO2 retrievals (Exp_OCO3&2). In each experiment, the methodology employed was consistent with that of previous studies (Peters et al., 2007; Jiang et al., 2021, 2022); only the NEE and OCN fluxes were optimized, and the FIRE and FOSSIL emissions were prescribed. According to Eq. (1), the prior NEE and OCN fluxes were perturbed using Eq. (6).
where , , , and represent the prior fluxes of NEE, OCN, FIRE, and FOSSIL, respectively; δi denotes random perturbation samples, which is independent between grids; and λNEE and λocn are the scaling factors for prior NEE and OCN fluxes, which were set to be 6 and 10 in this study, respectively. As described above, the prior fluxes have a spatial resolution of 1° × 1°; for δi, NEE and δi,ocn, we adopted a spatial resolution of 3° × 3°, and the outputs of the posterior fluxes have the same spatial resolution as the prior fluxes, meaning that in each 3° × 3° grid, the prior fluxes were adjusted with the same factor.
Additionally, two forward simulations were conducted to obtain the prior and posterior CO2 concentrations, which were then compared with the independent CO2 observations to assess the posterior carbon fluxes. Following Jiang et al. (2022), MOZART-4 is driven by the 1.9° × 2.5° grid version of the GEOS5 Global Atmosphere Forcing Data (Tilmes, 2016). It has a vertical level of 72 layers, and MOZART-4 uses the lowest 56 vertical levels of GEOS5 and the same spatial resolution as GEOS5 data.
4.1 Global carbon budget
Table 1 presents the prior and the posterior annual global carbon budgets from the three inversion experiments during 2020–2022. The global terrestrial NEEs obtained from the Exp_OCO3, Exp_OCO2, and Exp_OCO3&2 experiments are , , and PgC yr−1, respectively. The global NEE inferred from Exp_OCO3 is significantly weaker than the global NEEs from Exp_OCO2 and Exp_OCO3&2, and the latter two are comparable. For the OCN carbon sink, Exp_OCO3 has the strongest sink but is closest to the a priori result, while Exp_OCO2 and Exp_OCO3&2 have essentially the same sink. Combined with the FOSSIL and FIRE carbon emissions, the global net carbon fluxes are 4.74±0.77, 5.55±0.67, 4.90±0.63, and 4.93±0.60 PgC yr−1 for the a priori, Exp_OCO3, Exp_OCO2, and Exp_OCO3&2, respectively. In comparison with the average atmospheric CO2 growth rate of 4.93 PgC yr−1 for 2020–2022 given by the Global Carbon Budget 2023 (Friedlingstein et al., 2023), the results of Exp_OCO3&2 are the closest, with a mean bias of 0.0 PgC yr−1, whereas Exp_OCO3 has the largest bias, with a deviation of 0.62 PgC yr−1. This indicates that the carbon sinks in Exp_OCO3 may be significantly underestimated, and joint assimilation of OCO-2 and OCO-3 XCO2 retrievals gives the best performance on a global scale.
4.2 Regional NEE
Figure 3 shows the spatial distribution of annual mean posterior terrestrial fluxes and oceanic fluxes from Exp_OCO3, Exp_OCO2, and Exp_OCO3&2 and their differences from the a priori fluxes. Overall, the spatial distribution of carbon sources and sinks in terrestrial ecosystems obtained from different experiments is basically the same, with sources in western North America (N America), eastern Amazonia, parts of Siberia, parts of northwestern China, central and western Australia, and the Sahel region and eastern parts of Africa, while other areas are carbon sinks. However, the carbon sources and sinks obtained from Exp_OCO3 exhibit a markedly different strength compared to those derived from the other two experiments. Compared with the prior flux, the terrestrial carbon sinks in northeastern China, most of Europe, northern Siberia, the central and northeastern United States (USA), and southern Africa increased significantly in all three experiments. However, the increase in terrestrial carbon sinks in regions other than northeastern China in Exp_OCO2 and Exp_OCO3&2 was greater than that in Exp_OCO3. Meanwhile, in southern Canada, the western and southern USA, eastern Brazil and northern South America (S America), and the Sahel region and eastern parts of Africa, all three inversion experiments show a significant decrease in the terrestrial carbon sink. The degree of change in the inversion results is more pronounced in Exp_OCO2 and Exp_OCO3&2 than in Exp_OCO3. Figure 3 also show the distribution of terrestrial carbon fluxes along latitudes. The posterior and prior fluxes have a similar distribution trend along the latitude, with a significant peak of carbon sink near 60° N, and the strongest sinks of Exp_OCO2 and Exp_OCO3&2 are comparable and are significantly stronger than the a priori, while Exp_OCO3 has the weakest peak in carbon sink and is close to the a priori. In addition, it could also be found that the terrestrial carbon sinks obtained from Exp_OCO3 are also significantly smaller than those from Exp_OCO2 and Exp_OCO3&2 near 30° S.
In order to better understand and compare the differences among different inversion experiments, we have aggregated the prior and the posterior NEEs into the 11 TransCom 3 land regions (Fig. 2), as shown in Table 2. It is clear that almost all terrestrial regions behave as carbon sinks, both prior and posterior fluxes. Among the experiments, only the terrestrial NEE in northern Africa obtained by Exp_OCO3&2 shows a weak carbon source. There is relatively good agreement between all the inversion experiments on whether the land carbon flux is a source or sink, but there is a significant difference in the NEE values. In all regions except temperate N America, northern Africa, temperate Asia, and Australia, Exp_OCO3 shows a weaker carbon sink than Exp_OCO2. Comparing Exp_OCO3 with Exp_OCO3&2, Exp_OCO3&2 shows stronger carbon sinks in temperate N America, southern Africa, Australia, and Europe and weaker sinks in tropical S America, northern Africa, and boreal Asia; elsewhere Exp_OCO3&2 shows intermediate sinks compared to the other two experiments.
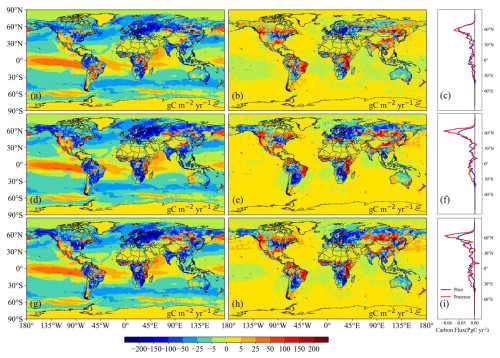
Figure 3Spatial distribution of annual mean posterior terrestrial and oceanic carbon fluxes from 2020 to 2022, the difference between posterior and prior fluxes, and the distribution of terrestrial NEEs at different latitudes (a–c: Exp_OCO3; d–f: Exp_OCO2; g–i: Exp_OCO3&2).
The regions with more pronounced differences among experiments are temperate S America and Europe. In Europe, the posterior fluxes of each inversion experiment show a pronounced carbon sink, which is significantly larger than the prior flux, but the results of different experiments vary to some extent, with NEEs ranging from to PgC yr−1 (Table 2) and Exp_OCO3&2 having the largest sink. In the temperate part of S America, Exp_OCO3 exhibits a very weak carbon sink, whereas both Exp_OCO2 and Exp_OCO3&2 show a moderate carbon sink. One potential explanation for this discrepancy is that the XCO2 concentration observed by OCO-3 in the temperate part of South America is higher than that observed by OCO-2 for the duration of the study period (by ∼ 0.55 ppm). Consequently, assimilating the OCO-3 observations yields a weaker carbon sink. Compared with the prior flux, the posterior NEE in the tropical part of S America shows a significant discrepancy: the prior flux show a very strong carbon sink of 0.23 PgC yr−1, whereas the subsequent application of constraints from satellite observations results in an approximate 2–3-fold reduction in the carbon sinks, with values ranging from to PgC yr−1.
Following the imposition of constraints derived from satellite observations, the carbon sinks on the Northern Hemisphere land are all enhanced, with the largest enhancement of 0.59 PgC yr−1 in Exp_OCO3&2, followed by 0.19 and 0.36 PgC yr−1 in Exp_OCO3 and Exp_OCO2, respectively. While in the tropics, the carbon sinks were all weakened, with Exp_OCO3 being weakened most, by 0.67 PgC yr−1, and Exp_OCO2 and Exp_OCO3&2 being weakened by 0.37 and 0.59 PgC yr−1, respectively; on Southern Hemisphere land, in Exp_OCO3, the sinks were weakened by 0.2 PgC yr−1, whereas in Exp_OCO2 and Exp_OCO3&2, they were enhanced by 0.08 and 0.05 PgC yr−1, respectively.
4.3 Seasonal cycle of NEE
Figure 4 illustrates the seasonal cycle of NEE for each TransCom 3 region. The posterior NEEs of different experiments are in good agreement on the seasonal cycle in most regions. In the Northern Hemisphere, the seasonal cycles of NEE in boreal N America, temperate N America, boreal Asia, temperate Asia, and Europe show relatively consistent trends. Carbon sinks in these regions generally occur from May to September and carbon sources from October to April. Large differences are evident in the strength of the carbon sinks observed in different regions, with different months in which the strongest carbon sinks occur. Boreal N America, temperate N America, and boreal Asia have the strongest carbon sinks in July; temperate Asia has the peak in July or August; and Europe has the strongest sinks in June. In the Southern Hemisphere, the southern Africa and temperate S America have more consistent seasonal cycles, with their carbon sources occurring roughly from July to December and sinks from January to June. The strongest carbon sources all occur in October, and the strongest sinks occur around March. In Australia, carbon sinks occur mainly from March to October, with the peak occurring in August. In the tropics, southern Africa shows a seasonal cycle that is opposite to that of northern Africa, and carbon sinks occur from January to July, with the strongest carbon sinks occurring near March. Tropical Asia shows a carbon sink in most months, with the strongest sink in September. The seasonal cycle in tropical S America is more complex, with the strongest carbon source in October. In general, seasonal amplitudes are small in the tropics and large in the northern regions. The averaged seasonal amplitudes of the three inversion experiments in boreal Asia, Europe, and temperate N America are 1.17, 0.97, and 0.72 PgC yr−1, respectively, while the seasonal amplitudes in tropical Asia and S America are about 0.10 PgC yr−1.
The regions where the difference between the prior and posterior NEEs is particularly pronounced are tropical S America, southern Africa, Australia, and Europe. In the tropical part of S America, the prior NEE is a significant sink from May to July, but after constraints from satellite observations, the carbon sink decreases significantly, even approaching neutral in June and July, and furthermore, in September and October, the sink also decreases significantly compared to the a priori. In southern Africa, the carbon sink is significantly stronger from January to March compared to the a priori, and conversely, the carbon source is significantly stronger in October and November. In Australia, the carbon sink is significantly increased from January to August and decreased in October and November compared to the a priori. In Europe, there is a significant increase in the carbon sinks from May to June compared to the a priori.
As described in Sect. 4.2, in temperate N America, northern Africa, temperate Asia, and Australia, Exp_OCO3 shows a stronger sink than Exp_OCO2, and this sink mainly occurs in May and June in temperate N America, in August and September in northern Africa, and from April to September in temperate Asia and Australia (except for July for Australia). In other regions, Exp_OCO3 has weaker sinks than Exp_OCO2. In the high latitudinal regions, on the one hand, the carbon sinks in June and July of Exp_OCO3 are generally smaller than those of Exp_OCO2, and on the other hand, the carbon source in October is significantly higher than that of Exp_OCO2, while in the tropics, the carbon sink is lower than that of Exp_OCO2 almost all year round. Compared to Exp_OCO3, Exp_OCO3&2 shows stronger carbon sinks in temperate N America, southern Africa, Australia, and Europe, mainly in summer, and weaker sinks in tropical S America, northern Africa, and boreal Asia, mainly in autumn. Elsewhere Exp_OCO3&2 shows intermediate carbon sinks compared to the other two experiments.
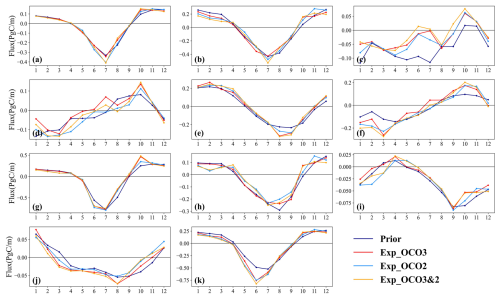
Figure 4Averaged prior and posterior seasonal cycle of NEE in different TransCom 3 regions during 2020–2022: (a) boreal N America, (b) temperate N America, (c) tropical S America, (d) temperate S America, (e) northern Africa, (f) southern Africa, (g) boreal Asia, (h) temperate Asia, (i) tropical Asia, (j) Australia, and (k) Europe.
4.4 Evaluation against independent observations
As shown in Fig. 5, observations from 66 surface flask sites were used to evaluate the posterior fluxes. The prior and posterior CO2 concentrations were simulated by the MOZART-4 model using the corresponding prior and posterior fluxes, as described in Sect. 3. The overall assessment results of the individual inversion experiments on a global scale are shown in Table 3. The results show that the BIAS, MAE, and RMSE between the prior CO2 concentrations and surface flask observations are −1.82, 3.27, and 5.01 ppm, respectively. The prior BIAS shows a pronounced negative bias, which can be attributed to the fact that the prior NEE in 2019 (generated by the spin-up stage) was, on average, approximately 3.5 PgC less than the posterior NEE. This part of the NEE has an impact on the subsequent inversion. After constraints using the XCO2 retrievals, the biases of the three experiments are reduced significantly compared to the a priori, indicating that the surface carbon fluxes have been improved. A comparison of the three inversion experiments reveals that Exp_OCO3 exhibits the largest BIAS, while Exp_OCO3&2 exhibits the lowest MAE and RMSE.
Table 3Error statistics between the simulated CO2 concentrations and surface flask observations (ppm).
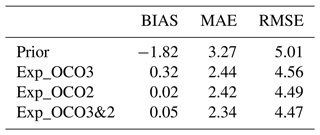
Figure 5a and b illustrate the BIAS of the individual inversion experiments at different latitudinal zones and in different TransCom 3 land regions. In all latitudinal bands and all land regions, the CO2 concentrations modelled by the a priori fluxes have the largest negative BIAS, which is greater than −1.2 ppm in all cases. Across latitudinal zones, in the Southern Hemisphere, and south of 30° N latitude, Exp_OCO3 had the smallest BIAS, which is smaller than that of Exp_OCO2 and comparable to the results of Exp_OCO3&2. However, in the middle to high latitudes of the Northern Hemisphere, the BIAS of Exp_OCO3 is higher than that of Exp_OCO2 and Exp_OCO3&2. Especially in the region north of 60° N latitude, Exp_OCO3 exhibits a significant positive BIAS, while Exp_OCO2 and Exp_OCO3&2 both exhibit small negative BIAS. This suggests that the carbon sinks at middle to high latitudes were underestimated. We also find that the OCO-3 retrievals help with the lack of space-based XCO2 observations in the tropics compared to OCO-2. The BIAS of Exp_OCO3&2 is smaller than Exp_OCO2 in the region from 30° S to 30° N. Meanwhile, the BIAS of Exp_OCO3&2 is also smaller than Exp_OCO2 in southern Africa, northern Africa, and tropical Asia. Furthermore, we can find that the BIAS can be further reduced in the middle to high latitudes of the Northern Hemisphere after the addition of assimilated OCO-3 observations compared to Exp_OCO2. In different TransCom 3 land regions, the BIAS of the three inversion experiments is less than ±0.6 ppm, except in the temperate part of Asia. In Africa, temperate S America, tropical Asia, and Australia, Exp_OCO3 has the smallest BIAS, while the BIAS of Exp_OCO3&2 is between the values of Exp_OCO3 and Exp_OCO2. However, in temperate N America and Europe, Exp_OCO3 has the largest BIAS, followed by that of Exp_OCO2, while Exp_OCO3&2 has the smallest BIAS.
4.5 Discussion
In most of the previous studies that used OCO-2 XCO2 to invert surface carbon fluxes, the OG data were not used (e.g. Peiro et al., 2022; Byrne et al., 2023); the reason for this is that the OG XCO2 may have larger uncertainties, meaning inversions assimilating OCO-2 OG retrievals produced unrealistic results of annual global ocean sinks (Peiro et al., 2022). In addition to its large uncertainties, we believe that another reason for the poor assimilation performance of OG is the relatively homogeneous distribution of XCO2 for ocean, causing a large correlation of the model–data biases among different XCO2 observations within the same region, which leads to observations in the same region having the same direction of adjustment for surface fluxes and thus to a significant overestimation or underestimation of the ocean carbon sink. Because of this, some assimilation algorithms (e.g. EnSRF) can only achieve better assimilation results when the model–data biases between observations have relatively small correlation or are uncorrelated. Therefore, in this study, we set the OG data to have larger uncertainties than the LNLG data and re-gridded them at a coarser spatial resolution of 5° × 5°. The results show that under this scheme, the inverted ocean sink is reasonable, with a value of −2.6 PgC yr−1 (Table 1). In addition, in order to compare the scheme that we have adopted in this study with the previous schemes that do not assimilate the OG, we added three additional inversion experiments in which only the LNLG data were assimilated (Table S1 in the Supplement). It was found that all three inversion experiments without OG observations place smaller constraints on the ocean fluxes compared to the original experiments, with the posterior ocean fluxes remaining almost identical to the prior ocean fluxes. Correspondingly, the inverted global land sink as well as the sinks in most regions shows a slight decrease (Tables S2 and S3). Evaluations in comparison with in situ observations showed that there are some increases in the a posteriori concentration biases for all three experiments after removing OG. For example, for the experiments assimilating OCO-2 data, the mean bias increased from 0.02 to 0.14 ppm (Table S4). This suggests that assimilating OG data with our method can improve the inversions somewhat compared to removing OG.
Since OCO-3 has similar observation uncertainties in XCO2 to OCO-2 (Taylor et al., 2023), the poor performance of assimilating OCO-3 XCO2 retrievals (Exp_OCO3) may be related to (1) OCO-3 lacking observations beyond 52° N and 52° S latitudes (Fig. 1a), (2) the observation time being different from OCO-2, and (3) OCO-3 spatial coverage being between 52° S and 52° N. We first examined weekly changes in the number of data of OCO-3 using the re-gridded data as described in Sect. 2.3 and found that there are very significant cyclical fluctuations in the number of data from OCO-3 (Fig. S4a). Every 8 weeks or so, there is a trough in the number of data. There is an approximately 5-fold difference between the weeks with the highest and the lowest number of data, and in the weeks with the lower number of data, there were essentially no observations in the Northern Hemisphere (Fig. S4b). This implies that the surface carbon fluxes are largely unconstrained in the Northern Hemisphere, especially at middle to high latitudes, during the weeks with a low number of observational data, resulting in poorer assimilation performance than for OCO-2. For the observation time, all observations of OCO-2 were at 13:30 local time (LST), whereas those of OCO-3 were variable, with only about 14 % of the observations close to 13:30 LST and about 54 % in the morning or after 16:00 LST (Fig. S1 in the Supplement). For reasons such as a coarser model resolution, the global atmospheric chemical transport models generally simulate atmospheric concentrations better only in the afternoon, when boundary layer heights are at their highest and atmospheric mixing is at its best, so assimilating these observations in the morning and after 16:00 LST may result in poorer inversions due to the greater simulation bias of the atmospheric transport models at these times of day.
In order to quantify these effects, we added another three inversion experiments, which were named Exp_OCO2r, Exp_OCO3tc, and Exp_OCO2ts (Table S1). In Exp_OCO2r, only the OCO-2 XCO2 retrievals located between the 52° S and 52° N retrievals were assimilated; in Exp_OCO3tc, all the observation times of the OCO-3 XCO2 retrievals were changed to 13:30 LST; and in Exp_OCO3ts, only OCO-3 data with observation times between 12:00 and 15:00 LST were assimilated. When the OCO-2 data beyond 52° N and 52° S latitudes were also removed (Exp_OCO2r), the NEE estimates, both globally and for individual regions, are close to those of the Exp_OCO3 experiment, especially in the high-latitude region of Europe and boreal North America; the inverted NEEs are almost identical to those of the Exp_OCO3 experiment (Tables S2 and S3); and the bias of a posteriori concentrations from observations at high latitudes is close to that of the OCO-3 experiment (Fig. S3). However, globally, compared to the OCO-3 experiment, the Exp_OCO2r experiment still shows smaller deviation between the global net flux and the observed annual growth rate (Table S2) and a smaller global mean bias in the posterior concentrations (Table S4). This suggests that the lack of observations of OCO-3 beyond 52° N and 52° S latitudes does have a significant impact on the inversion results. In addition, it can also be noted that at mid-latitudes, the bias in Exp_OCO2r is also smaller than that in the OCO-3 experiment, which may be caused by the significant fluctuations in the number of data of OCO-3 (Fig. S4). When we changed all the observation times of the OCO-3 XCO2 retrievals to 13:30 LST (Exp_OCO3tc), although we are not actually able to do so, the inversion does show a significant improvement compared to Exp_OCO3. However, if we only select the data with an observation time between 12:00 and 15:00 LST (Exp_OCO3ts), the deviation between the global net flux and the observed annual growth rate and the mean biases of the posterior concentrations at most latitudes are larger than those of Exp_OCO3 (Table S2 and Fig. S3), indicating a poorer performance than Exp_OCO3. The probable reason for this is that the number of observations is substantially reduced at this time (Fig. S2), which leads to a substantial weakening of the observational constraints on surface carbon fluxes (Fig. S5).
In this study, we constrained terrestrial NEEs for the period from 1 August 2019 to 31 December 2022 using the OCO-2 and OCO-3 XCO2 retrievals and the GCASv2 system, and we analysed the inversion results from 2020 to 2022. We conducted three inversion experiments for separately and jointly assimilating the OCO-2 and OCO-3 XCO2 retrievals to explore the impact of the OCO-3 XCO2 retrievals on the constraints of global terrestrial NEEs. The prior and posterior CO2 mixing ratios obtained from forward simulations using the prior and posterior fluxes are analysed in comparison with observations from 66 surface flask sites.
Globally, the terrestrial carbon sink from Exp_OCO3 is smaller than the prior, while the terrestrial carbon sinks from the other two inversion experiments are slightly larger than the prior, but the difference is small. The global net carbon flux from Exp_OCO3&2 is very close to the observed atmospheric CO2 growth rate. Regionally, the posterior NEEs for most terrestrial regions show a carbon sink, with Europe showing a very strong sink and North Africa being close to carbon neutrality. In the Northern Hemisphere, the carbon sinks are enhanced, with Exp_OCO3&2 being the most enhanced by 0.59 PgC yr−1 and Exp_OCO3 and Exp_OCO2 being enhanced by 0.19 and 0.36 PgC yr−1, respectively. In the tropics, the carbon sinks are weakened, with Exp_OCO3 being the most weakened by 0.67 PgC yr−1 and the Exp_OCO2 and Exp_OCO3&2 sinks being weakened by 0.37 and 0.59 PgC yr−1, respectively; in the southern land, the sink inverted in Exp_OCO3 is weakened by 0.2 PgC yr−1, whereas those sinks inverted in Exp_OCO2 and Exp_OCO3&2 are enhanced by 0.08 and 0.05 PgC yr−1, respectively.
On a global scale, the BIAS between the prior CO2 concentrations and surface flask observations is −1.82 ppm, with an MAE of 3.27 ppm and an RMSE of 5.01 ppm. The deviations between the posterior CO2 concentrations and surface flask observations for all three inversions are reduced to different degrees from the prior, especially for the BIAS, which decreased to 0.32, 0.02, and 0.05 ppm for Exp_OCO3, Exp_OCO2, and Exp_OCO3&2, respectively. The reasons for the poor performance of assimilating OCO-3 XCO2 alone are, firstly, the fact that it is only available between 52° S and 52° N, which leads to a lack of observational constraints on the carbon sinks at high latitudes, and the large fluctuations in the number of observational data, which lead to significant differences in observational constraints at mid-latitudes at different times; secondly, the varied observation time also affect the inversions, but even choosing afternoon observations does not improve the inversions because the number of observed data drops significantly. Therefore, a better option for the future would be to jointly assimilate the OCO-2 XCO2 data and the OCO-3 XCO2 retrievals observed in the afternoon (12:00 to 16:00 LST).
The code of the GCASv2 system is available to the community and can be accessed upon request from Fei Jiang (jiangf@nju.edu.cn) at Nanjing University.
The OCO-2 and OCO-3 data used in this study are available at https://doi.org/10.5067/8E4VLCK16O6Q (OCO-2 Science Team et al., 2022b) and https://doi.org/10.5067/970BCC4DHH24 (OCO-3 Science Team et al., 2022a), respectively. The FOSSIL carbon emissions of GCP-GridFEDv2023.1 are available at https://doi.org/10.5281/zenodo.8386803 (Jones et al., 2023). The FIRE carbon emissions from GFED4.1s are available at https://www.geo.vu.nl/~gwerf/GFED/GFED4/ (van der Werf et al., 2017). The ObsPack dataset is available at https://doi.org/10.25925/20231201 (Schuldt et al., 2023). The results of the three inversion experiments and evaluation are publicly available at https://doi.org/10.5281/zenodo.11239535 (Wang, 2024).
The supplement related to this article is available online at: https://doi.org/10.5194/acp-25-867-2025-supplement.
XW and FJ designed the research. XW ran the model, analysed the results, and wrote the paper. HW and ZZ collected the OCO-2 and OCO-3 XCO2 retrievals. MW, JW, WH, WJ, and JC participated in the discussion of the inversion results and provided revisions before the paper was submitted.
The contact author has declared that none of the authors has any competing interests.
Publisher's note: Copernicus Publications remains neutral with regard to jurisdictional claims made in the text, published maps, institutional affiliations, or any other geographical representation in this paper. While Copernicus Publications makes every effort to include appropriate place names, the final responsibility lies with the authors.
The OCO-2 and OCO-3 data are produced by the OCO project at the Jet Propulsion Laboratory, California Institute of Technology, and were obtained from the data archive at the NASA Goddard Earth Science Data and Information Services Center. We acknowledge all atmospheric data providers to obspack_co2_1_GLOBALVIEWplus_v9.1_2023-12-08. We are also grateful to the High-Performance Computing Center (HPCC) of Nanjing University for doing the numerical calculations in this paper on its blade cluster system.
This work is supported by the National Key R&D Program of China (grant no. 2023YFB3907404), the National Natural Science Foundation of China (grant no. 42377102), and the Fengyun Application Pioneering Project (grant no. FY-APP-2022.0505).
This paper was edited by Chris Wilson and reviewed by three anonymous referees.
Basu, S., Guerlet, S., Butz, A., Houweling, S., Hasekamp, O., Aben, I., Krummel, P., Steele, P., Langenfelds, R., Torn, M., Biraud, S., Stephens, B., Andrews, A., and Worthy, D.: Global CO2 fluxes estimated from GOSAT retrievals of total column CO2, Atmos. Chem. Phys., 13, 8695–8717, https://doi.org/10.5194/acp-13-8695-2013, 2013.
Botta, A., Ramankutty, N., and Foley, J. A.: LBA-ECO LC-04 IBIS Model Simulations for the Amazon and Tocantins Basins, 1921–1998, https://doi.org/10.3334/ORNLDAAC/1139, 2012.
Bousquet, P., Peylin, P., Ciais, P., Le Quéré, C., Friedlingstein, P., and Tans, P. P.: Regional Changes in Carbon Dioxide Fluxes of Land and Oceans Since 1980, Science, 290, 1342–1346, https://doi.org/10.1126/science.290.5495.1342, 2000.
Byrne, B., Liu, J., Lee, M., Baker, I., Bowman, K. W., Deutscher, N. M., Feist, D. G., Griffith, D. W. T., Iraci, L. T., Kiel, M., Kimball, J. S., Miller, C. E., Morino, I., Parazoo, N. C., Petri, C., Roehl, C. M., Sha, M. K., Strong, K., Velazco, V. A., Wennberg, P. O., and Wunch, D.: Improved constraints on northern extratropical CO2 fluxes obtained by combining surface-based and space-based atmospheric CO2 measurements, J. Geophys. Res.-Atmos., 125, e2019JD032029, https://doi.org/10.1029/2019JD032029, 2020.
Byrne, B., Liu, J., Lee, M., Yin, Y., Bowman, K. W., Miyazaki, K., Norton, A. J., Joiner, J., Pollard, D. F., Griffith, D. W. T., Velazco, V. A., Deutscher, N. M., Jones, N. B., and Paton-Walsh, C.: The carbon cycle of southeast Australia during 2019–2020: Drought, fires, and subsequent recovery, AGU Adv., 2, e2021AV000469, https://doi.org/10.1029/2021AV000469, 2021.
Byrne, B., Liu, J., Yi, Y., Chatterjee, A., Basu, S., Cheng, R., Doughty, R., Chevallier, F., Bowman, K. W., Parazoo, N. C., Crisp, D., Li, X., Xiao, J., Sitch, S., Guenet, B., Deng, F., Johnson, M. S., Philip, S., McGuire, P. C., and Miller, C. E.: Multi-year observations reveal a larger than expected autumn respiration signal across northeast Eurasia, Biogeosciences, 19, 4779–4799, https://doi.org/10.5194/bg-19-4779-2022, 2022.
Byrne, B., Baker, D. F., Basu, S., Bertolacci, M., Bowman, K. W., Carroll, D., Chatterjee, A., Chevallier, F., Ciais, P., Cressie, N., Crisp, D., Crowell, S., Deng, F., Deng, Z., Deutscher, N. M., Dubey, M. K., Feng, S., García, O. E., Griffith, D. W. T., Herkommer, B., Hu, L., Jacobson, A. R., Janardanan, R., Jeong, S., Johnson, M. S., Jones, D. B. A., Kivi, R., Liu, J., Liu, Z., Maksyutov, S., Miller, J. B., Miller, S. M., Morino, I., Notholt, J., Oda, T., O'Dell, C. W., Oh, Y.-S., Ohyama, H., Patra, P. K., Peiro, H., Petri, C., Philip, S., Pollard, D. F., Poulter, B., Remaud, M., Schuh, A., Sha, M. K., Shiomi, K., Strong, K., Sweeney, C., Té, Y., Tian, H., Velazco, V. A., Vrekoussis, M., Warneke, T., Worden, J. R., Wunch, D., Yao, Y., Yun, J., Zammit-Mangion, A., and Zeng, N.: National CO2 budgets (2015–2020) inferred from atmospheric CO2 observations in support of the global stocktake, Earth Syst. Sci. Data, 15, 963–1004, https://doi.org/10.5194/essd-15-963-2023, 2023.
Chen, H., He, W., Liu, J., Nguyen, N. T., Chevallier, F., Yang, H., Lv, Y., Huang, C., Rödenbeck, C., Miller, S., Jiang, F., Liu, J., Johnson, M., Philip, S., Liu, Z., Zeng, N., Basu, S., and Baker, D.: Satellite-detected large CO2 release in southwestern North America during the 2020–2021 drought and associated wildfires, Environ. Res. Lett., 19, 054047, https://doi.org/10.1088/1748-9326/ad3cf7, 2024.
Chen, J. M., Ju, W., Ciais, P., Viovy, N., Liu, R., Liu, Y., and Lu, X.: Vegetation structural change since 1981 significantly enhanced the terrestrial carbon sink, Nat. Commun., 10, 4259, https://doi.org/10.1038/s41467-019-12257-8, 2019.
Crisp, D., Pollock, H. R., Rosenberg, R., Chapsky, L., Lee, R. A. M., Oyafuso, F. A., Frankenberg, C., O'Dell, C. W., Bruegge, C. J., Doran, G. B., Eldering, A., Fisher, B. M., Fu, D., Gunson, M. R., Mandrake, L., Osterman, G. B., Schwandner, F. M., Sun, K., Taylor, T. E., Wennberg, P. O., and Wunch, D.: The on-orbit performance of the Orbiting Carbon Observatory-2 (OCO-2) instrument and its radiometrically calibrated products, Atmos. Meas. Tech., 10, 59–81, https://doi.org/10.5194/amt-10-59-2017, 2017.
Crowell, S., Baker, D., Schuh, A., Basu, S., Jacobson, A. R., Chevallier, F., Liu, J., Deng, F., Feng, L., McKain, K., Chatterjee, A., Miller, J. B., Stephens, B. B., Eldering, A., Crisp, D., Schimel, D., Nassar, R., O'Dell, C. W., Oda, T., Sweeney, C., Palmer, P. I., and Jones, D. B. A.: The 2015–2016 carbon cycle as seen from OCO-2 and the global in situ network, Atmos. Chem. Phys., 19, 9797–9831, https://doi.org/10.5194/acp-19-9797-2019, 2019.
Eldering, A., Boland, S., Solish, B., Crisp, D., Kahn, P., and Gunson, M.: High precision atmospheric CO2 measurements from space: The design and implementation of OCO-2, 2012 IEEE Aerospace Conference, 3–10 March 2012, 1–10, https://doi.org/10.1109/AERO.2012.6187176, 2012.
Eldering, A., O'Dell, C. W., Wennberg, P. O., Crisp, D., Gunson, M. R., Viatte, C., Avis, C., Braverman, A., Castano, R., Chang, A., Chapsky, L., Cheng, C., Connor, B., Dang, L., Doran, G., Fisher, B., Frankenberg, C., Fu, D., Granat, R., Hobbs, J., Lee, R. A. M., Mandrake, L., McDuffie, J., Miller, C. E., Myers, V., Natraj, V., O'Brien, D., Osterman, G. B., Oyafuso, F., Payne, V. H., Pollock, H. R., Polonsky, I., Roehl, C. M., Rosenberg, R., Schwandner, F., Smyth, M., Tang, V., Taylor, T. E., To, C., Wunch, D., and Yoshimizu, J.: The Orbiting Carbon Observatory-2: first 18 months of science data products, Atmos. Meas. Tech., 10, 549–563, https://doi.org/10.5194/amt-10-549-2017, 2017.
Eldering, A., Taylor, T. E., O'Dell, C. W., and Pavlick, R.: The OCO-3 mission: measurement objectives and expected performance based on 1 year of simulated data, Atmos. Meas. Tech., 12, 2341–2370, https://doi.org/10.5194/amt-12-2341-2019, 2019.
Emmons, L. K., Walters, S., Hess, P. G., Lamarque, J.-F., Pfister, G. G., Fillmore, D., Granier, C., Guenther, A., Kinnison, D., Laepple, T., Orlando, J., Tie, X., Tyndall, G., Wiedinmyer, C., Baughcum, S. L., and Kloster, S.: Description and evaluation of the Model for Ozone and Related chemical Tracers, version 4 (MOZART-4), Geosci. Model Dev., 3, 43–67, https://doi.org/10.5194/gmd-3-43-2010, 2010.
Enting, I. G. and Newsam, G. N. Atmospheric constituent inversion problems: Implications for baseline monitoring, J. Atmos. Chem., 11, 69–87, https://doi.org/10.1007/BF00053668, 1990.
Feng, S., Jiang, F., Wu, Z., Wang, H., Ju, W., and Wang, H.: CO Emissions Inferred From Surface CO Observations Over China in December 2013 and 2017, J. Geophys. Res.-Atmos., 125, https://doi.org/10.1029/2019jd031808, 2020.
Friedlingstein, P., O'Sullivan, M., Jones, M. W., Andrew, R. M., Bakker, D. C. E., Hauck, J., Landschützer, P., Le Quéré, C., Luijkx, I. T., Peters, G. P., Peters, W., Pongratz, J., Schwingshackl, C., Sitch, S., Canadell, J. G., Ciais, P., Jackson, R. B., Alin, S. R., Anthoni, P., Barbero, L., Bates, N. R., Becker, M., Bellouin, N., Decharme, B., Bopp, L., Brasika, I. B. M., Cadule, P., Chamberlain, M. A., Chandra, N., Chau, T.-T.-T., Chevallier, F., Chini, L. P., Cronin, M., Dou, X., Enyo, K., Evans, W., Falk, S., Feely, R. A., Feng, L., Ford, D. J., Gasser, T., Ghattas, J., Gkritzalis, T., Grassi, G., Gregor, L., Gruber, N., Gürses, Ö., Harris, I., Hefner, M., Heinke, J., Houghton, R. A., Hurtt, G. C., Iida, Y., Ilyina, T., Jacobson, A. R., Jain, A., Jarníková, T., Jersild, A., Jiang, F., Jin, Z., Joos, F., Kato, E., Keeling, R. F., Kennedy, D., Klein Goldewijk, K., Knauer, J., Korsbakken, J. I., Körtzinger, A., Lan, X., Lefèvre, N., Li, H., Liu, J., Liu, Z., Ma, L., Marland, G., Mayot, N., McGuire, P. C., McKinley, G. A., Meyer, G., Morgan, E. J., Munro, D. R., Nakaoka, S.-I., Niwa, Y., O'Brien, K. M., Olsen, A., Omar, A. M., Ono, T., Paulsen, M., Pierrot, D., Pocock, K., Poulter, B., Powis, C. M., Rehder, G., Resplandy, L., Robertson, E., Rödenbeck, C., Rosan, T. M., Schwinger, J., Séférian, R., Smallman, T. L., Smith, S. M., Sospedra-Alfonso, R., Sun, Q., Sutton, A. J., Sweeney, C., Takao, S., Tans, P. P., Tian, H., Tilbrook, B., Tsujino, H., Tubiello, F., van der Werf, G. R., van Ooijen, E., Wanninkhof, R., Watanabe, M., Wimart-Rousseau, C., Yang, D., Yang, X., Yuan, W., Yue, X., Zaehle, S., Zeng, J., and Zheng, B.: Global Carbon Budget 2023, Earth Syst. Sci. Data, 15, 5301–5369, https://doi.org/10.5194/essd-15-5301-2023, 2023.
Gurney, K. R., Law, R. M., Denning, A. S., Rayner, P. J., Baker, D., Bousquet, P., Bruhwiler, L., Chen, Y.-H., Ciais, P., Fan, S., Fung, I. Y., Gloor, M., Heimann, M., Higuchi, K., John, J., Maki, T., Maksyutov, S., Masarie, K., Peylin, P., Prather, M., Pak, B. C., Randerson, J., Sarmiento, J., Taguchi, S., Takahashi, T., and Yuen, C.-W.: Towards robust regional estimates of CO2 sources and sinks using atmospheric transport models, Nature, 415, 626–630, https://doi.org/10.1038/415626a, 2002.
Hall, B. D., Crotwell, A. M., Kitzis, D. R., Mefford, T., Miller, B. R., Schibig, M. F., and Tans, P. P.: Revision of the World Meteorological Organization Global Atmosphere Watch (WMO/GAW) CO2 calibration scale, Atmos. Meas. Tech., 14, 3015–3032, https://doi.org/10.5194/amt-14-3015-2021, 2021.
Hansen, J., Sato, M., Russell, G., and Kharecha, P.: Climate sensitivity, sea level and atmospheric carbon dioxide, Philos. Trans. R. Soc., A, 371, 20120294, https://doi.org/10.1098/rsta.2012.0294, 2013.
He, W., Jiang, F., Wu, M., Ju, W., Scholze, M., Chen, J. M., Byrne, B., Liu, J., Wang, H., Wang, J., Wang, S., Zhou, Y., Zhang, C., Nguyen, N. T., Shen, Y., and Chen, Z.: China's Terrestrial Carbon Sink Over 2010–2015 Constrained by Satellite Observations of Atmospheric CO2 and Land Surface Variables, J. Geophys. Res.-Biogeosci., 127, e2021JG006644, https://doi.org/10.1029/2021JG006644, 2022.
He, W., Jiang, F., Ju, W., Chevallier, F., Baker, D. F., Wang, J., Wu, M., Johnson, M. S., Philip, S., Wang, H., Bertolacci, M., Liu, Z., Zeng, N., and Chen, J. M.: Improved Constraints on the Recent Terrestrial Carbon Sink Over China by Assimilating OCO-2 XCO2 Retrievals, J. Geophys. Res.-Atmos., 128, e2022JD037773, https://doi.org/10.1029/2022JD037773, 2023a.
He, W., Jiang, F., Ju, W., Byrne, B., Xiao, J., Nguyen, N. T., Wu, M., Wang, S., Wang, J., Rödenbeck, C., Li, X., Scholze, M., Monteil, G., Wang, H., Zhou, Y., He, Q., and Chen, J. M.: Do State-Of-The-Art Atmospheric CO2 Inverse Models Capture Drought Impacts on the European Land Carbon Uptake?, J. Adv. Model. Earth Syst, 15, e2022MS003150, https://doi.org/10.1029/2022MS003150, 2023b.
Houtekamer, P. L. and Mitchell, H. L.: A sequential ensemble Kalman filter for atmospheric data assimilation, Mon. Weather Rev., 129, 123–137, https://doi.org/10.1175/1520-0493(2001)129<0123:ASEKFF>2.0.CO;2, 2001.
Iida, Y., Takatani, Y., Kojima, A., and Ishii, M.: Global trends of ocean CO2 sink and ocean acidification: an observation-based reconstruction of surface ocean inorganic carbon variables, J. Oceanogr., 77, 323–358, https://doi.org/10.1007/s10872-020-00571-5, 2021.
Jiang, F., Wang, H., Chen, J. M., Ju, W., Tian, X., Feng, S., Li, G., Chen, Z., Zhang, S., Lu, X., Liu, J., Wang, H., Wang, J., He, W., and Wu, M.: Regional CO2 fluxes from 2010 to 2015 inferred from GOSAT XCO2 retrievals using a new version of the Global Carbon Assimilation System, Atmos. Chem. Phys., 21, 1963–1985, https://doi.org/10.5194/acp-21-1963-2021, 2021.
Jiang, F., Ju, W., He, W., Wu, M., Wang, H., Wang, J., Jia, M., Feng, S., Zhang, L., and Chen, J. M.: A 10-year global monthly averaged terrestrial net ecosystem exchange dataset inferred from the ACOS GOSAT v9 XCO2 retrievals (GCAS2021), Earth Syst. Sci. Data, 14, 3013–3037, https://doi.org/10.5194/essd-14-3013-2022, 2022.
Jin, J., Lin, H. X., Heemink, A., and Segers, A.: Spatially varying parameter estimation for dust emissions using reduced-tangent-linearization 4DVar, Atmos. Environ., 187, 358–373, https://doi.org/10.1016/j.atmosenv.2018.05.060, 2018.
Jones, M. W., Andrew, R. M., Peters, G. P., Janssens-Maenhout, G., De-Gol, A. J., Ciais, P., Patra, P. K., Chevallier, F., and Le Quéré, C.: Gridded fossil CO2 emissions and related O2 combustion consistent with national inventories 1959–2018, Sci. Data, 8, 2, https://doi.org/10.1038/s41597-020-00779-6, 2021.
Jones, M. W., Andrew, R. M., Peters, G. P., Janssens-Maenhout, G., De-Gol, A. J., Dou, X., Liu, Z., Pickers, P., Ciais, P., Patra, P. K., Chevallier, F., and Le Quéré, C.: Gridded fossil CO2 emissions and related O2 combustion consistent with national inventories (GCP-GridFEDv2023.1), Zenodo [data set], https://doi.org/10.5281/zenodo.8386803, 2023.
Lauvaux, T., Pannekoucke, O., Sarrat, C., Chevallier, F., Ciais, P., Noilhan, J., and Rayner, P. J.: Structure of the transport uncertainty in mesoscale inversions of CO2 sources and sinks using ensemble model simulations, Biogeosciences, 6, 1089–1102, https://doi.org/10.5194/bg-6-1089-2009, 2009.
Liu, J., Bowman, K. W., Schimel, D. S., Parazoo, N. C., Jiang, Z., Lee, M., Bloom, A. A., Wunch, D., Frankenberg, C., Sun, Y., O'Dell, C. W., Gurney, K. R., Menemenlis, D., Gierach, M., Crisp, D., and Eldering, A.: Contrasting carbon cycle responses of the tropical continents to the 2015–2016 El Niño, Science, 358, eaam5690, https://doi.org/10.1126/science.aam5690, 2017.
Miller, S. M., Michalak, A. M., Yadav, V., and Tadić, J. M.: Characterizing biospheric carbon balance using CO2 observations from the OCO-2 satellite, Atmos. Chem. Phys., 18, 6785–6799, https://doi.org/10.5194/acp-18-6785-2018, 2018.
Miyazaki, K., Eskes, H. J., Sudo, K., Takigawa, M., van Weele, M., and Boersma, K. F.: Simultaneous assimilation of satellite NO2, O3, CO, and HNO3 data for the analysis of tropospheric chemical composition and emissions, Atmos. Chem. Phys., 12, 9545–9579, https://doi.org/10.5194/acp-12-9545-2012, 2012.
OCO-3 Science Team (Chatterjee, A. and Payne, V.): OCO-3 Level 2 bias-corrected XCO2 and other select fields from the full-physics retrieval aggregated as daily files, Retrospective processing v10.4r, Greenbelt, MD, USA, Goddard Earth Sciences Data and Information Services Center (GES DISC) [data set], https://doi.org/10.5067/970BCC4DHH24, 2022a.
OCO-2 Science Team (Payne, V. and Chatterjee, A.): OCO-2 Level 2 bias-corrected XCO2 and other select fields from the full-physics retrieval aggregated as daily files, Retrospective processing V11.1r, Greenbelt, MD, USA, Goddard Earth Sciences Data and Information Services Center (GES DISC) [data set], https://doi.org/10.5067/8E4VLCK16O6Q, 2022b.
Palmer, P. I., Feng, L., Baker, D., Chevallier, F., Bösch, H., and Somkuti, P.: Net carbon emissions from African biosphere dominate pan-tropical atmospheric CO2 signal, Nat. Commun., 10, 3344, https://doi.org/10.1038/s41467-019-11097-w, 2019.
Peiro, H., Crowell, S., Schuh, A., Baker, D. F., O'Dell, C., Jacobson, A. R., Chevallier, F., Liu, J., Eldering, A., Crisp, D., Deng, F., Weir, B., Basu, S., Johnson, M. S., Philip, S., and Baker, I.: Four years of global carbon cycle observed from the Orbiting Carbon Observatory 2 (OCO-2) version 9 and in situ data and comparison to OCO-2 version 7, Atmos. Chem. Phys., 22, 1097–1130, https://doi.org/10.5194/acp-22-1097-2022, 2022.
Peters, W., Jacobson, A. R., Sweeney, C., Andrews, A. E., Conway, T. J., Masarie, K., Miller, J. B., Bruhwiler, L. M. P., Pétron, G., Hirsch, A. I., Worthy, D. E. J., van der Werf, G. R., Randerson, J. T., Wennberg, P. O., Krol, M. C., and Tans, P. P.: An atmospheric perspective on North American carbon dioxide exchange: CarbonTracker, P. Natl. Acad. Sci. USA, 104, 1892518930, https://doi.org/10.1073/pnas.0708986104, 2007.
Peylin, P., Law, R. M., Gurney, K. R., Chevallier, F., Jacobson, A. R., Maki, T., Niwa, Y., Patra, P. K., Peters, W., Rayner, P. J., Rödenbeck, C., van der Laan-Luijkx, I. T., and Zhang, X.: Global atmospheric carbon budget: results from an ensemble of atmospheric CO2 inversions, Biogeosciences, 10, 6699–6720, https://doi.org/10.5194/bg-10-6699-2013, 2013.
Philip, S., Johnson, M. S., Baker, D. F., Basu, S., Tiwari, Y. K., Indira, N. K., Ramonet, M., and Poulter, B.: OCO-2 Satellite-Imposed Constraints on Terrestrial Biospheric CO2 Fluxes Over South Asia, J. Geophys. Res.-Atmos., 127, e2021JD035035, https://doi.org/10.1029/2021JD035035, 2022.
Piao, S., Wang, X., Wang, K., Li, X., Bastos, A., Canadell, J. G., Ciais, P., Friedlingstein, P., and Sitch, S.: Interannual variation of terrestrial carbon cycle: Issues and perspectives, Global Change Biol., 26, 300–318, https://doi.org/10.1111/gcb.14884, 2020.
Randerson, J. T., Van Der Werf, G. R., Giglio, L., Collatz, G. J., and Kasibhatla, P. S.: Global Fire Emissions Database, Version 4.1 (GFEDv4), https://doi.org/10.3334/ORNLDAAC/1293, 2017.
Schuldt, K. N., Mund, J., Aalto, T., Abshire, J. B., Aikin, K., Allen, G., Andrews, A., Apadula, F., Arnold, S., Baier, B., Bakwin, P., Bartyzel, J., Bentz, G., Bergamaschi, P., Beyersdorf, A., Biermann, T., Biraud, S. C., Blanc, P.-E., Boenisch, H., Bowling, D., Brailsford, G., Brand, W. A., Brunner, D., Bui, T. P., Bäni, L., Calzolari, F., Chang, C. S., Chen, H., Chen, G., Chmura, L., Clark, S., Climadat, S., Colomb, A., Commane, R., Condori, L., Conen, F., Conil, S., Couret, C., Cristofanelli, P., Cuevas, E., Curcoll, R., Daube, B., Davis, K. J., De Mazière, M., De Wekker, S., Dean-Day, J. M., Della Coletta, J., Delmotte, M., Di Iorio, T., DiGangi, E., DiGangi, J. P., Dickerson, R., Elkins, J. W., Elsasser, M., Emmenegger, L., Fang, S., Fischer, M. L., Forster, G., France, J., Frumau, A., Fuente-Lastra, M., Galkowski, M., Gatti, L. V., Gehrlein, T., Gerbig, C., Gheusi, F., Gloor, E., Goto, D., Griffis, T., Hammer, S., Hanisco, T. F., Hanson, C., Haszpra, L., Hatakka, J., Heimann, M., Heliasz, M., Heltai, D., Henne, S., Hensen, A., Hermans, C., Hermansen, O., Hintsa, E., Hoheisel, A., Holst, J., Iraci, L. T., Ivakhov, V., Jaffe, D. A., Jordan, A., Joubert, W., Kang, H.-Y., Karion, A., Kawa, S. R., Kazan, V., Keeling, R. F., Keronen, P., Kim, J., Klausen, J., Kneuer, T., Ko, M.-Y., Kolari, P., Kominkova, K., Kort, E., Kozlova, E., Krummel, P. B., Kubistin, D., Kulawik, S. S., Kumps, N., Labuschagne, C., Lam, D. H., Lan, X., Langenfelds, R. L., Lanza, A., Laurent, O., Laurila, T., Lauvaux, T., Lavric, J., Law, B. E., Lee, C.-H., Lee, H., Lee, J., Lehner, I., Lehtinen, K., Leppert, R., Leskinen, A., Leuenberger, M., Leung, W. H., Levin, I., Levula, J., Lin, J., Lindauer, M., Lindroth, A., Loh, Z. M., Lopez, M., Lunder, C. R., Löfvenius, M. O., Machida, T., Mammarella, I., Manca, G., Manning, A., Manning, A., Marek, M. V., Marklund, P., Marrero, J. E., Martin, D., Martin, M. Y., Martins, G. A., Matsueda, H., McKain, K., Meijer, H., Meinhardt, F., Merchant, L., Metzger, J.-M., Mihalopoulos, N., Miles, N. L., Miller, C. E., Miller, J. B., Mitchell, L., Monteiro, V., Montzka, S., Moore, F., Moossen, H., Morgan, E., Morgui, J.-A., Morimoto, S., Munger, J. W., Munro, D., Mutuku, M., Myhre, C. L., Mölder, M., Müller-Williams, J., Nakaoka, S.-I., Necki, J., Newman, S., Nichol, S., Nisbet, E., Niwa, Y., Njiru, D. M., Noe, S. M., Nojiri, Y., O'Doherty, S., Obersteiner, F., Paplawsky, B., Parworth, C. L., Peischl, J., Peltola, O., Peters, W., Philippon, C., Piacentino, S., Pichon, J. M., Pickers, P., Piper, S., Pitt, J., Plass-Dülmer, C., Platt, S. M., Prinzivalli, S., Ramonet, M., Ramos, R., Ren, X., Reyes-Sanchez, E., Richardson, S. J., Rigouleau, L.-J., Riris, H., Rivas, P. P., Rothe, M., Roulet, Y.-A., Ryerson, T., Ryoo, J.-M., Sargent, M., Sasakawa, M., Scheeren, B., Schmidt, M., Schuck, T., Schumacher, M., Seibel, J., Seifert, T., Sha, M. K., Shepson, P., Shook, M., Sloop, C. D., Smith, P. D., Spain, G., St. Clair, J. M., Steger, D., Steinbacher, M., Stephens, B., Sweeney, C., Sørensen, L. L., Taipale, R., Takatsuji, S., Tans, P., Thoning, K., Timas, H., Torn, M., Trisolino, P., Turnbull, J., Vermeulen, A., Viner, B., Vitkova, G., Walker, S., Watson, A., Weiss, R., Weyrauch, D., Wofsy, S. C., Worsey, J., Worthy, D., Xueref-Remy, I., Yates, E. L., Young, D., Yver-Kwok, C., Zaehle, S., Zahn, A., Zellweger, C., Zimnoch, M., Souza, R. A. de, di Sarra, A. G., van Dinther, D., and van den Bulk, P.: Multi-laboratory compilation of atmospheric carbon dioxide data for the period 1957–2022; obspack_co2_1_GLOBALVIEWplus_v9.1_2023-12-08; NOAA Earth System Research Laboratory, Global Monitoring Laboratory, https://doi.org/10.25925/20231201, 2023.
Takahashi, T., Sutherland, S. C., Wanninkhof, R., Sweeney, C., Feely, R. A., Chipman, D. W., Hales, B., Friederich, G., Chavez, F., Sabine, C., Watson, A., Bakker, D. C. E., Schuster, U., Metzl, N., Yoshikawa-Inoue, H., Ishii, M., Midorikawa, T., Nojiri, Y., Körtzinger, A., Steinhoff, T., Hoppema, M., Olafsson, J., Arnarson, T. S., Tilbrook, B., Johannessen, T., Olsen, A., Bellerby, R., Wong, C. S., Delille, B., Bates, N. R., and de Baar, H. J. W.: Climatological mean and decadal change in surface ocean pCO2, and net sea-air CO2 flux over the global oceans, Deep Sea Res. Pt. II, 56, 554–577, https://doi.org/10.1016/j.dsr2.2008.12.009, 2009.
Taylor, T. E., Eldering, A., Merrelli, A., Kiel, M., Somkuti, P., Cheng, C., Rosenberg, R., Fisher, B., Crisp, D., Basilio, R., Bennett, M., Cervantes, D., Chang, A., Dang, L., Frankenberg, C., Haemmerle, V. R., Keller, G. R., Kurosu, T., Laughner, J. L., Lee, R., Marchetti, Y., Nelson, R. R., O'Dell, C. W., Osterman, G., Pavlick, R., Roehl, C., Schneider, R., Spiers, G., To, C., Wells, C., Wennberg, P. O., Yelamanchili, A., and Yu, S.: OCO-3 early mission operations and initial (vEarly) XCO2 and SIF retrievals, Remote Sens. Environ., 251, 112032, https://doi.org/10.1016/j.rse.2020.112032, 2020.
Taylor, T. E., O'Dell, C. W., Baker, D., Bruegge, C., Chang, A., Chapsky, L., Chatterjee, A., Cheng, C., Chevallier, F., Crisp, D., Dang, L., Drouin, B., Eldering, A., Feng, L., Fisher, B., Fu, D., Gunson, M., Haemmerle, V., Keller, G. R., Kiel, M., Kuai, L., Kurosu, T., Lambert, A., Laughner, J., Lee, R., Liu, J., Mandrake, L., Marchetti, Y., McGarragh, G., Merrelli, A., Nelson, R. R., Osterman, G., Oyafuso, F., Palmer, P. I., Payne, V. H., Rosenberg, R., Somkuti, P., Spiers, G., To, C., Weir, B., Wennberg, P. O., Yu, S., and Zong, J.: Evaluating the consistency between OCO-2 and OCO-3 XCO2 estimates derived from the NASA ACOS version 10 retrieval algorithm, Atmos. Meas. Tech., 16, 3173–3209, https://doi.org/10.5194/amt-16-3173-2023, 2023.
Thompson, R. L., Patra, P. K., Chevallier, F., Maksyutov, S., Law, R. M., Ziehn, T., van der Laan-Luijkx, I. T., Peters, W., Ganshin, A., Zhuravlev, R., Maki, T., Nakamura, T., Shirai, T., Ishizawa, M., Saeki, T., Machida, T., Poulter, B., Canadell, J. G., and Ciais, P.: Top–down assessment of the Asian carbon budget since the mid1990s, Nat. Commun., 7, 10724, https://doi.org/10.1038/ncomms10724, 2016.
Tilmes, S.: GEOS5 Global Atmosphere Forcing Data, Research Data Archive at the National Center for Atmospheric Research, Computational and Information Systems Laboratory [dataset], https://doi.org/10.5065/QTSA-G775, 2016.
van der Werf, G. R., Randerson, J. T., Giglio, L., van Leeuwen, T. T., Chen, Y., Rogers, B. M., Mu, M., van Marle, M. J. E., Morton, D. C., Collatz, G. J., Yokelson, R. J., and Kasibhatla, P. S.: Global fire emissions estimates during 1997–2016, Earth Syst. Sci. Data, 9, 697–720, https://doi.org/10.5194/essd-9-697-2017, 2017 (data available at: https://www.geo.vu.nl/~gwerf/GFED/GFED4/, last access: 20 January 2025).
Wang, H., Jiang, F., Wang, J., Ju, W., and Chen, J. M.: Terrestrial ecosystem carbon flux estimated using GOSAT and OCO-2 XCO2 retrievals, Atmos. Chem. Phys., 19, 12067–12082, https://doi.org/10.5194/acp-19-12067-2019, 2019.
Wang, J., Jiang, F., Wang, H. M., Qiu, B., Wu, M. S., He, W., Ju, W. M., Zhang, Y. G., Chen, J. M., and Zhou, Y. L.: Constraining global terrestrial gross primary productivity in a global carbon assimilation system with OCO–2 chlorophyll fluorescence data, Agr. Forest Meteorol., 304–305, 108424, https://doi.org/10.1016/j.agrformet.2021.108424, 2021.
Wang, H., Jiang, F., Liu, Y., Yang, D., Wu, M., He, W., Wang, J., Wang, J., Ju, W., and Chen, J. M.: Global Terrestrial Ecosystem Carbon Flux Inferred from TanSat XCO2 Retrievals, J. Remote Sens., 2022, 9816536, https://doi.org/10.34133/2022/9816536, 2022.
Wang, X.: The role of OCO-3 XCO2 retrievals in estimating global terrestrial net ecosystem exchanges, Zenodo [data set], https://doi.org/10.5281/zenodo.11239535, 2024.
Whitaker, J. S. and Hamill, T. M.: Ensemble Data Assimilation without Perturbed Observations, Mon. Weather Rev., 130, 1913–1924, https://doi.org/10.1175/1520-0493(2002)130<1913:EDAWPO>2.0.CO;2, 2002.
Zhang, S., Zheng, X., Chen, J. M., Chen, Z., Dan, B., Yi, X., Wang, L., and Wu, G.: A global carbon assimilation system using a modified ensemble Kalman filter, Geosci. Model Dev., 8, 805–816, https://doi.org/10.5194/gmd-8-805-2015, 2015.