the Creative Commons Attribution 4.0 License.
the Creative Commons Attribution 4.0 License.
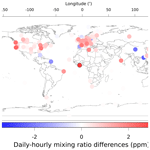
To what extent does the CO2 diurnal cycle impact flux estimates derived from global and regional inversions?
Christian Rödenbeck
Michał Gałkowski
Frank-Thomas Koch
Kai U. Totsche
Santiago Botía
Christoph Gerbig
Ignoring the diurnal cycle in surface-to-atmosphere CO2 fluxes leads to a systematic bias in CO2 mole fraction simulations sampled at daytime because the daily mean flux systematically misses the CO2 uptake during the daytime hours. In an atmospheric inversion using daytime-selected CO2 measurements at most continental sites and not resolving diurnal cycles in the flux, this leads to systematic biases in the estimates of the annual sources and sinks of atmospheric CO2. This study focuses on quantifying the impact of this diurnal cycle effect on the annual carbon fluxes estimated with the CarboScope (CS) atmospheric inversion at regional, continental, and global scales for the period of time 2010–2020. Our analysis is based on biogenic fluxes of hourly net ecosystem exchange (NEE) obtained from the data-driven FLUXCOM-X estimates, together with global and regional atmospheric transport models. Differences between CO2 mixing ratios simulated with daily averaged and hourly NEE from FLUXCOM-X range between around −2.5 and 7 ppm averaged annually throughout a site network across the world. These differences lead to systematic biases in CO2 flux estimates from the atmospheric inversions. Although the impact on the global total flux is negligible (around 2 % of the overall land flux of −1.79 Pg C yr−1), we find significant biases in the annual flux budgets at continental and regional scales. For Europe, the annual mean difference in the fluxes arising indirectly from the diurnal cycle of CO2 through the boundary condition amounts to around 48 % of the annual posterior fluxes (0.31 Pg C yr−1) estimated with CarboScope-Regional (CSR). Furthermore, the differences in NEE estimates calculated with CS increase the magnitude of the flux budgets for some regions such as North American temperate forests and northern Africa by a factor of about 1.5. To the extent that FLUXCOM-X diurnal cycles are realistic at all latitudes and for the station set including many continental stations as used in our inversions here, we conclude that ignoring the diurnal variations in the land CO2 flux leads to overestimation of both CO2 sources in the tropical lands and CO2 sinks in the temperate zones.
- Article
(3685 KB) - Full-text XML
- BibTeX
- EndNote
Accurate estimation of the carbon budget is necessary for verifying the reduction of global carbon emissions in line with climate adaptation policies adopted in the Paris Climate Agreement (Glanemann et al., 2020). From a scientific perspective, it is also of high interest to extend our understanding about physical and biogeochemical dynamics of the carbon cycle in the Earth's climate system. As reported in the Sixth Assessment Report (AR6) of the Intergovernmental Panel on Climate Change (Canadell et al., 2023), the cumulative carbon budget in the atmosphere recently exceeded 279 Pg C by 2019, mainly due to fossil fuel combustion, cement production, and net emissions associated with land-use change since the industrial era began in 1850. The consequence is global warming, with a global mean temperature excess by around 1.1 °C between 1850–1900 and 2011–2020. Recently, the annual fossil-fuel-related emission of CO2 increased to 10.9 Pg C yr−1 over the past decade (2010–2019), with 5.1 Pg C yr−1 accumulated in the atmosphere, 3.4 Pg C yr−1 taken up by the terrestrial biosphere, and 2.5 Pg C yr−1 counted as an ocean sink (Canadell et al., 2023). Fixing the largest amount of atmospheric CO2 in the biosphere via photosynthesis, the terrestrial biosphere sink consequently increases in response to CO2 concentration growth, which is thought to result from three effects: (1) fertilization by the rise in CO2 abundance in the atmosphere, (2) increase in nitrogen inputs into soil used by agriculture to enhance plants growth, and (3) prolongation of growing seasons (Friedlingstein et al., 2022). It is important to note, however, that interannual variability in the land sink is large (about 1 Pg C yr−1), making it difficult to pin down small emission variations in global atmospheric CO2 concentrations (Baker et al., 2006). Moreover, due to the heterogeneity in terrestrial ecosystems, the atmosphere-to-land CO2 fluxes are also largely variable at temporal and regional subscales (Marcolla et al., 2017), as regularly demonstrated by both dynamic global vegetation models (DGVMs) and data-based global inversion models, e.g. within the framework of the Global Carbon Project (Friedlingstein et al., 2022, 2023).
Atmospheric inverse modelling has increasingly been used at global (Ciais et al., 2010; Kaminski et al., 1999; Peylin et al., 2002; Rödenbeck et al., 2003) and regional scales (see Berchet et al., 2021; Chevallier et al., 2014; Gerbig et al., 2003; Monteil and Scholze, 2021; Munassar et al., 2022; Rivier et al., 2010) to constrain the surface–atmosphere carbon fluxes and their variabilities based on atmospheric measurements sampled through surface in situ networks and airborne-based monitoring instruments. Although the observational constraint is meant to drive the solution of the inverse problem, uncertainties and biases in atmospheric transport together with prescribed flux components such as anthropogenic emissions and ocean fluxes can diminish the capability of the inversions in terms of finding the optimal values of the state parameters (Engelen, 2002; Gurney et al., 2003). The atmospheric transport uncertainty especially remains a genuine challenge in inverse modelling to estimate carbon fluxes (Deng et al., 2017; Gerbig et al., 2008; Munassar et al., 2023), specifically over regions where observations either do not exist or are relatively sparse, such as over the tropics (Gurney et al., 2003; Botía, 2022). In fact, already in 2007, Stephens et al. (2007) found inconsistencies in the vertical atmospheric CO2 distributions between aircraft measurements and atmospheric models that transfer large amounts of terrestrial carbon from the tropics towards northern latitudes, leading to overestimation of tropical sources and stronger sinks in northern terrestrial land, an effect necessary to maintain the mass balance in the carbon budget.
The interhemispheric gradient of the annual mean CO2 concentration evident by global atmospheric data represents one of the primary atmospheric constraints of the global carbon budget in all global atmospheric tracer inversions (Tans et al., 1990). Originally, this gradient was suggested to result primarily from higher emissions of fossil fuels over the Northern Hemisphere as compared to those in the Southern Hemisphere. Denning et al. (1995), however, found a significant meridional gradient imposed by the seasonal CO2 exchange of terrestrial biota, amounting to half of the gradient imposed by fossil fuel emissions, suggesting a stronger sink in the Northern Hemisphere than previously assumed. This effect has been referred to as “the atmospheric rectifier”, in which CO2 uptake and vertical mixing are both driven by solar radiation (Larson and Volkmer, 2008; Denning et al., 1999). That is, in the early afternoon on any sunny day of the growing season, the uptake of CO2 by photosynthesis and the dilution due to deep atmospheric vertical mixing attenuate the level of atmospheric CO2 concentrations (Stephens et al., 2007). By contrast, during the nighttime, CO2 concentrations accumulate near the Earth's surface, owing to ecosystem respiration and the atmospheric stratification under the shallow boundary layer. On daily average, the covariance between the atmospheric transport and terrestrial biospheric fluxes results in a vertical gradient of CO2 distributions with a surplus near the surface. In addition, an interhemispheric gradient is imposed, with a higher concentration near northern polar regions and a lower concentration in the tropics and the Southern Hemisphere (Chan et al., 2008). This effect becomes uncertain at regional scales along the latitude bands. Therefore, misrepresentation of biosphere fluxes and vertical transport affects the simulations of CO2 mole fractions (Gurney et al., 2003). Although the ability of transport models to represent the atmospheric variability is essential to reconstruct the observations of CO2 mole fractions, Patra et al. (2008) found that the representation of CO2 terrestrial input fluxes is crucial to capture CO2 synoptical variations, with the best agreement with observations achieved when diurnally varying terrestrial fluxes are used. Increasing both the horizontal resolution and vertical levels in transport models improves the performance of transport models to retrieve CO2 diurnal variations observed at different locations, especially over coastal and mountain terrains (Law et al., 2008).
As biases in simulated CO2 mole fractions arise from both diurnal and seasonal variations of the vertical transport and biosphere CO2 fluxes, information of these variations should be accounted for in inverse modelling. While the seasonal effect can be reconciled in atmospheric inversions by the seasonal variations of CO2, already included in the observational constraint, the diurnal variations are commonly missing in the observational constraint because in inversions the use of observations is typically restricted to times when the lower atmosphere is well mixed (i.e. during local noon to afternoon times) (Gerbig et al., 2008). An accurate representation of the such variations is then dependent on the performance of atmospheric transport models to represent the seasonal and diurnal cycles. Therefore, reconciliation of the diurnal effect in atmospheric inversions is achievable by including diurnal variations of the biogenic terrestrial fluxes in the prior fluxes, provided that the vertical transport diurnal variations are accounted for. However, not all atmospheric inversions contributing to the Global Carbon Project (GCP) account for the diurnal cycle in biosphere–atmosphere exchange of CO2, at least in their current setups such as CarboScope and the Carbon in Ocean-Land-Atmosphere inversion (COLA; Liu et al., 2022). On the other hand, there are a number of inversions using biosphere flux models that resolve diurnal variations, such as the Copernicus Atmosphere Monitoring Service inversion (CAMS; Chevallier et al., 2017) assimilating 3-hourly biosphere–atmosphere fluxes from ORCHIDEE (Krinner et al., 2005), the Global ObservatioN-based system for monitoring Greenhouse GAses inversion (GONGGA; Jin et al., 2024) from ORCHIDEE-MICT (Guimberteau et al., 2018), the Global Carbon Assimilation System (GCAS) from the Boreal Ecosystems Productivity Simulator (BEPS) (Jiang et al., 2021), and both Carbon Tracker Europe (CTE; van der Laan-Luijkx et al., 2017) and the inversion algorithm THU (Kong et al., 2022) using 3-hourly net ecosystem exchange (NEE) from the Simple Biosphere model (SiB4; Haynes et al., 2019). Additionally, several GCP inversions utilize biogenic prior fluxes estimated by biosphere models without a direct inclusion of the CO2 diurnal cycle (i.e. run at daily or coarser time steps). However, the diurnal cycle of CO2 is included through temporal downscaling of gross flux components to hourly time steps using surface radiation and temperature from meteorological fields to derive the diurnal variations of gross primary productivity (GPP) and ecosystem respiration following the approach established by Olsen and Randerson (2004). Of those inversion models are Carbon Tracker (CT; Jacobson et al., 2023), Carbon Monitoring System Flux (CMS-Flux; Liu et al., 2021), Model for Interdisciplinary Research on Climate version 4 (MIROC4-ACTM; Chandra et al., 2022), NICAM-based Inverse Simulation for Monitoring CO2 (NISMON-CO2; Niwa et al., 2022), and the Institute of Atmospheric Physics Carbon dioxide retrieval Algorithm for satellite remote sensing (IAPCAS; Yang et al., 2021).
Our study aims to investigate the impact of the diurnal cycle on global and regional CO2 flux estimates using CarboScope inversion (CS), which so far (version v2023) has not accounted for diurnal variations in the CO2 flux. In the standard global CS framework, the posterior biogenic fluxes are dominantly driven by the atmospheric data constraint, and the control vector represents deviations from zero biogenic fluxes used as a priori estimate. Unfortunately, the diurnal flux variability cannot be constrained by the atmospheric data because the atmospheric measurements are only used during daytime hours for surface stations and nighttime hours for mountain stations, reflecting that the transport model is expected to have particularly large errors outside these time periods. In the global total carbon flux, the impact of the diurnal cycle effect is attenuated because it is well constrained by the linear rise of CO2 from year to year, due to the mass conservation of CO2 in the atmosphere. However, for the fluxes at local scales, the diurnal cycle effect can lead to biases in the estimates.
In studies focusing on specific land regions, like Europe, it is essential to make use of as many continental measurements as possible. As these measurements are typically located within complex atmospheric circulation patterns, the relatively coarse global transport model is not well suited to represent these measurement locations. Therefore, variations in transport and in fluxes need to be resolved on finer spatial and temporal scales. Unfortunately, due to computational limitations, the transport model, and thus the inversion calculation, needs to be confined to the regional domain of interest. As the inversion problem is intrinsically global, however, such a regional inversion needs to be nested into a global inversion. Here, we consider the regional inversion for Europe using CarboScope-Regional (CSR) as described in Munassar et al. (2023, 2022), which uses the two-step scheme (Rödenbeck et al., 2009) to provide boundary conditions from a global inversion to the regional inversion. Even though the setup of the regional inversion does include the diurnal cycle in the a priori fluxes, it is nevertheless prone to biases passed on through the lateral boundary conditions calculated by the global inversion currently not taking the diurnal cycle of CO2 fluxes into account. In addition, the inversion is affected by imperfect representation of diurnal cycle in the transport model.
In this study, we focus on the impact of not having the diurnal cycle in the current global CarboScope setup, both the impact on the result of the global inversion and the inherited impact on the CSR results for Europe. Differences in CO2 mole fractions simulated with and without diurnal variations in NEE are calculated by forward runs of the global transport model. These differences are then inverted by a global inversion to address the impact on flux estimates. We analysed the flux differences on the global scale, in coarse latitude bands, as well as over a set of regions covering the whole globe. After that, the indirect effect of diurnal variations passed to regional inversions via the far-field influences was evaluated using CSR in the regional domain of Europe, employing a mesoscale transport model.
The organization of the paper is as follows: the next section describes the methods applied in this experiment elucidating the inversion setup, the prior information obtained from the biosphere flux model, and the atmospheric transport models (Sect. 2). Results of CO2 mixing ratio differences calculated with biosphere fluxes through forward model simulations across the site network are presented in Sect. 3, as well as differences in NEE estimates due to the impact of the diurnal cycle effect. Section 4 is devoted to the discussion of the results analysed at global and regional scales, highlighting the implications for carbon budget estimates. We summarize the key findings and relating perspectives of this study in Sect. 5.
2.1 Simulating the diurnal rectification of atmospheric CO2
Modelled CO2 mixing ratios are calculated for an atmospheric station network distributed around the world, as typically used for a global atmospheric inversion (Fig. 1). The CS global inversion uses atmospheric data collected from 169 stations around the world. Some of the stations provide continuous measurements, typically at hourly time intervals, while others provide flask samples collected at discrete times (typically weekly). According to the design of this experiment, we chose a subset containing 78 stations that consistently provide data from 2010 onwards, as in the CarboScope inversion run s10oc_v2022 (http://www.bgc-jena.mpg.de/CarboScope/?ID=s10oc_v2022, last access: 9 January 2025). The transport model TM3 (Heimann and Körner, 2003) is run with biogenic terrestrial fluxes from FLUXCOM-X (Bodesheim et al., 2018; Jung et al., 2019; Nelson et al., 2024). As land NEE has the largest diurnal cycle, only the biogenic flux component is taken into account to simulate CO2 dry mole fractions. Two forward runs are performed to generate simulations of CO2 dry mole factions: one with diurnal variations in NEE and one without diurnal variations (using daily averaged fluxes). Afterwards, the differences between simulations based on daily averaged NEE and simulations based on hourly NEE were calculated for each monitoring site across the whole network. These differences in simulated CO2 dry mole fractions are inverted in CS to quantify the impact on NEE estimates. This was done for practical considerations, as inverting a mole fraction difference is equivalent to performing two inversions and then obtaining the difference between the retrieved fluxes, due to the linearity of atmospheric tracer transport and inverse estimation.
2.2 Inversion setups
CarboScope (CS) is used at the global scale using the transport model TM3 (Heimann and Körner, 2003) at a spatial resolution of 5° × 4°. As the standard CarboScope CO2 inversion uses fixed ocean CO2 fluxes from an interpolation of surface-ocean pCO2 data, the state space vector is confined to the biogenic terrestrial flux component that is corrected spatially and temporally based on Bayesian inference (Enting, 2002). As the fluxes are resolved on a daily time step, the diurnal cycle in terrestrial ecosystems is not accounted for. The spatial and temporal autocorrelations of the prior error are exponentially decaying functions with 1200 km spatial correlation length and 30 d temporal correlation length. The inversion algorithm searches for the optimal flux adjustment as an additive correction based on the constraints guided by observations and a priori fluxes. For more details of the mathematical setup of CS, the reader is referred to Rödenbeck (2005).
Table 1CS and CSR inversion setups.

∗ Resolution of the driving meteorological fields; STILT is a Lagrangian particle model simulating subgrid-scale vertical mixing at effectively higher spatial scales.
To investigate the effect of the CO2 diurnal cycle on regional fluxes, CSR is used to optimize NEE at 0.5° × 0.5° spatial and 3-hourly temporal resolutions over specific regional domains, such as Europe, in this study. A spatial correlation length of 66 km is defined for prior flux error in CSR covariance matrices, while the temporal error structure remains identical to the configuration of CS. The spatial correlation decay follows a hyperbolic function, with the decay being faster in the meridional direction than in the zonal direction by a factor of 2. By comparison with CS, the TM3 transport model is replaced with the regional atmospheric transport model STILT with 0.25° horizontal resolution. It is important to note that a priori biogenic fluxes used in CSR do account for the diurnal cycle. Thus, our investigation focuses on the influence of the CO2 diurnal cycle as passed into the regional domain through initial and boundary conditions. The lateral boundary conditions are provided to the regional inversion by the two-step inversion scheme explained in Rödenbeck et al. (2009). Details of CS and CSR configurations, including prior uncertainty prescription, are listed in Table 1. For this experiment setup, ocean fluxes and anthropogenic emissions are omitted in both CS and CSR because these cancel out in the difference.
The uncertainty of the model–data mismatch is defined similarly in CS and CSR. It comprises a combination of the uncertainties arising from measurements, atmospheric transport, and spatial representation. Weekly values of the errors are assigned to stations based on a classification regarding the ability of atmospheric transport model to represent atmospheric dynamics over the locations of stations. For instance, tall towers, mountain sites, and stations located at/near shores and aircraft samples have an error of 1.5 ppm, while surface sites that represent complex circulations are assigned a relatively larger error (2.5 ppm). For hourly measurements, the error value is inflated depending on the number of data points assimilated per week such that the hourly error becomes the weekly error times the square root of the number of weekly hours (e.g. 42 h in the case that a time window of 6 h per day is chosen and if there are no data gaps).
2.3 Transport models
TM3 is an Eulerian transport model that solves the continuity equation (and parametrizations of boundary layer and convective mixing) for atmospheric tracers in a three-dimensional grid over the globe (Heimann and Körner, 2003). The model is driven by meteorological fields, such as wind velocity, air temperature, surface pressure, and specific humidity, obtained from NCEP reanalysis data (Kalnay et al., 1996). The tracer advection is determined by the mixing ratio and gradient of the tracer in grid boxes based on the slope scheme developed by Russell and Lerner (1981). In addition, the vertical transport is resolved by vertical diffusion and cumulus cloud transport deduced through evaporation fluxes, which are taken from meteorological fields. TM3 is run here at 5° × 4° spatial resolution, with 19 vertical levels spanning the troposphere and the stratosphere. Since the model is initialized with a homogeneous background of the tracer concentration, the model is run for at least 1 year before the period of interest to avoid any impact resulting from the model spin-up.
In the regional inversion CSR, the Stochastic Time-Inverted Lagrangian Transport model (STILT; Lin et al., 2003) is used at a finer horizontal resolution of 0.25° × 0.25° to resolve the atmospheric mesoscale variability via tracking the dispersion of tracers backward in time from starting locations called “receptors”. Forecasted meteorological data obtained from the ECMWF Integrated Forecasting System (IFS) drive an ensemble of virtual particles at receptor locations, normally over stations where atmospheric data are sampled. The particles are transported 10 d backward, and the surface influence functions (“footprints”) are stored at 0.25° horizontal resolution and hourly time steps. A daytime window of 6 h (11:00 to 16:00 LT) is chosen for low-elevation stations. For high-altitude sites such as mountain stations, a nighttime window of 23:00 to 04:00 LT is used to select free-tropospheric conditions. Note that the vertical resolution of the underlying meteorological data is much higher for STILT (89 levels up to about 20 km height) compared to TM3.
2.4 Biogenic terrestrial fluxes
In Bayesian inversion formalism, an a priori knowledge of the control parameters is essential to regularize the solution of the underdetermined system (Enting, 2002). Typically, prior fluxes of CO2 representing the exchange between the surface and the atmosphere are taken from bottom-up estimation. As we aim to assess the impact of CO2 diurnal cycle on the flux estimate, the fluxes are confined to the biogenic terrestrial component. The experiment was designed to invert the differences in mole fractions simulated with two variants of biosphere fluxes (i.e. with and without the diurnal cycle in the biosphere fluxes), rather than the formal way of implementing two inversions that would account for such variations in the prior biosphere fluxes. Hourly NEE calculated from FLUXCOM-X (Nelson et al., 2024), a data-driven upscaled flux product developed based on FLUXCOM (Bodesheim et al., 2018), is used as a variant that includes information of the CO2 diurnal cycle in CS. In addition, another variant of these fluxes was created by averaging these fluxes from hourly to daily. Moreover, the dataset used in this study is produced at 0.05° spatial resolution as a new product (labelled X-BASE) of the new modelling framework FLUXCOM-X, which has been developed to allow for applying different methodological choices with machine-learning-based gradient regression algorithms (Nelson et al., 2024). FLUXCOM-X mainly targets the estimations of four predicted variables (NEE, gross primary productivity (GPP), evapotranspiration (ET), and transpiration (ETT)) using 12 predictor variables obtained from global meteorology and satellite observations of daily surface reflectance and land surface temperature from the MODerate Imaging Spectroradiometer (MODIS). Hourly ERA-5 reanalysis data including several parameters such as air temperature, incoming shortwave radiation, and vapour pressure deficit are used at 0.25° spatial resolution. A total of 294 eddy covariance stations distributed across the world were used to provide observed fluxes for training and cross-validation. Quite a good performance of FLUXCOM-X was exceptionally noticed in retrieving the diurnal variations of the predicted variables, evident by comparing the model predictions with observations withheld for validations throughout the globe. This promotes the validity of using such products in our analysis to quantify the impact of CO2 diurnal cycle in inverse modelling. For detailed information about X-BASE products and their setup, the reader is referred to Nelson et al. (2024).
To quantify the impact of CO2 diurnal cycle, first, two forward runs using the global model TM3 were performed. The differences in CO2 simulations, extracted for a global set of measurement locations, were calculated by subtraction between the simulations based on daily and hourly data. To derive the impact on retrieved fluxes, these differences in CO2 simulations were then subsequently inverted using the standard CS configuration, except that all the prior fluxes and the initial CO2 mole fraction in the model atmosphere were set to zero (while the prior uncertainty remained identical to that of the standard inversion). This procedure is thus equivalent to using the difference of the results from two hypothetical inversions performed with and without diurnal cycle in the priors. That is, the linearity of the inversion operator is maintained in the inverted differences that represent the effect resulting from the hourly variability (deviations around daily means) required to be added to a daily mean, so as to reconstruct the diurnal cycle. The difference between the two inversions is thus determined by the transport operator and these hourly deviations, which lead to non-zero daily mean of simulated CO2 mole fractions due to the rectifier effect. Therefore, a correction of the magnitude of such a difference is required to be added to the daily a posteriori flux estimated with missing hourly flux variations to reconcile the diurnal effect. These corrections are applied to CS flux estimates in this study as diagnostics. To find out the indirect impact on the regional flux estimates in the regional inversion CSR, a two-step inversion was done using the inverted differences in the “far-field contribution” from the global inversion.
3.1 Differences in CO2 mole fractions
We present results from the difference between the two forward runs performed with the transport model TM3 over a set of atmospheric stations distributed throughout the globe for the period of time 2010–2020 (the transport model was coupled with hourly and daily averaged biogenic fluxes that are obtained from FLUXCOM-X; see Sect. 2.4). The time-averaged differences in CO2 mole fractions between daily and hourly simulations range from −2.49 to 6.97 ppm (1 ppm = 1 µmol mol−1), Fig. 1. Most of the sites (113) show positive differences with a mean of 0.67 ppm, while the remaining 34 sites resulted in negative differences with a mean of −0.41 ppm. These positive differences are found over sites that are more representative of the terrestrial land signal during the daytime. On the other hand, the negative differences are dominated by some mountain sites where simulations are confined to a nighttime window, resulting in a lagged land signal compared to daytime simulations. A portion of 27 sites of those 34 with negative difference are flask sites located either in remote islands or at shores. Therefore, such sites are affected by a large-scale ocean background with little terrestrial influence as well as by areas that contain mixed footprints from land and ocean, albeit with small influences. This implies that ignoring the diurnal cycle of CO2 leads to an excess of CO2 mole fraction over sites that are dominated by land footprints and to lower mole fractions over some mountain and ocean sites due to a lagged land signal in comparison with the simulations done with the diurnal cycle included.
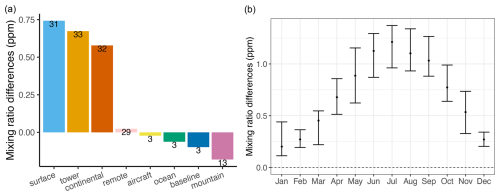
Figure 2Differences of CO2 dry mole fractions between simulations calculated with NEE based on daily and hourly data over specific site classifications and over months. Panel (a) shows the mean differences averaged over site-specific classifications (on x axis) for the analysed years (2010–2020), and the numbers mentioned in bars are the number of sites per classification. Panel (b) denotes monthly differences computed for the Northern Hemisphere and averaged over 88 sites representing towers, surface, and continental stations that dominate the impact over northern hemispheric lands (note that error bars refer to the range of differences over the target years (2010–2020)).
The differences due to the CO2 diurnal cycle effect are also assessed separately for the different types of sites to distinguish their representativeness of land and ocean backgrounds but also daytime and nighttime land signals. A large number of sites are located in lands and thus can have a good representativeness of the biosphere signal. Most of these sites are tall towers (33), continental sites (32), and surface sites (31), over which the largest positive mean differences in simulated CO2 mole fractions occur (0.70, 0.63, and 0.77 ppm, respectively; see Fig. 2, left panel). A total of 29 remote sites, mostly situated in islands, show a very weak impact with a 0.02 ppm mean difference. A total of 13 mountain sites demonstrate a mean difference of −0.22 ppm, indicating the dominant nighttime land signal as simulations are restricted to a local nighttime window over mountain locations. In addition, some sites that poorly represent the biosphere footprints such as baseline, ocean, and aircraft observing locations have quite small negative differences in CO2 mole fraction simulations amounting to −0.10, −0.09, and −0.01 ppm, respectively. It should be noted that there are only three sites for each of these types, which makes it statistically difficult to draw conclusions, even though these small differences are expected over such types of locations where the biosphere signal is generally weak.
A large impact is observed during the growing season as the amplitude of the diurnal cycle reaches its maximum due to the strong uptake of CO2 occurring under the best conditions of light availability and soil water content. Figure 2 (right panel) shows monthly differences of CO2 dry mole fractions averaged for 11 years (2010–2020) over 88 stations that dominate the diurnal impact in the Northern Hemisphere. All of these stations are towers, surface, or continental sites, which have a reliable representativeness of land footprints. The differences amid the analysed years range between 0.11 and 1.37 ppm, with a mean difference of 0.71 ppm. The median computed over these years is 0.70 ppm, confirming a consistency with the mean. The large spreads of the monthly differences across years indicate a remarkable interannual variability of the diurnal impact. In June–July–August the mean difference amounts to 1.33 ppm, larger than the rest of the months, while the smallest differences were found in January–February–December with a mean of 0.38 ppm. The transition periods (March–April–May and September–October–November), close to the onset and termination of carbon uptake period, have moderate and relatively similar differences in CO2 mixing ratios (0.85 and 1.0 ppm, respectively).
3.2 Differences in NEE estimates
To outline the impact of CO2 diurnal cycle on the flux estimates, we next focus on analysing the differences in terrestrial NEE derived by the global CS inversion from the differences in CO2 mixing ratios for the period 2010–2020. The diurnal cycle effect in the global annual budget of terrestrial biogenic fluxes estimated in the CS inversion results in a difference of 0.04 Pg C yr−1 averaged over the analysed years, with a standard deviation of 0.13 Pg C yr−1. This difference in the global scale is rather small and equivalent to about 2 % of the mean annual terrestrial flux (−1.79 Pg C yr−1) and only to around 1 % of the prior uncertainty assumed for the biogenic fluxes. These findings suggest that the diurnal cycle of CO2 does not have a significant impact on the annual global flux budget as, due to the fact that global CO2 flux estimates are well constrained by the growth rate of atmospheric CO2 mole fractions, the diurnal cycle effect has to be compensated for between subregions and months. Even though there are noticeable seasonal variations in the impact of the CO2 diurnal cycle effect (Fig. 3), the negative differences during June–July–August are compensated for by the positive differences during January–February–December and March–April–May when accounting for the impact on the annual scale. Differences during September–October–November remain around zero.
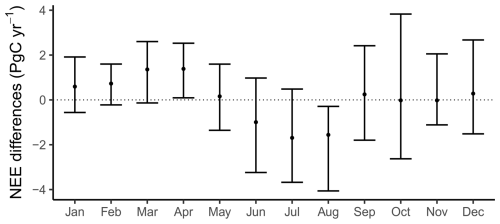
Figure 3Monthly mean differences in global NEE estimates resulting from the CO2 diurnal cycle, averaged over 2010–2020. Error bars refer to min and max differences among all the analysed years.
We next quantified the impact on latitudinal bands. Despite the negligible impact of the diurnal cycle at the global scale, the results indicate quite large differences in these bands (confined to NEE over lands, as ocean fluxes are not adjusted in the inversion setup used here). Figure 4 illustrates that the largest differences are estimated between 90–15° S (0.39 Pg C yr−1) and between 15° S–15° N (−0.46 Pg C yr−1), on an order of magnitude similar to the original flux. In the bands 15–45° N and 45–90° N, the differences are smaller than the flux estimates but still non-negligible, with −0.17 and 0.27 Pg C yr−1 change, respectively. As noted before, the overall difference over global land is quite small, owing to the symmetry of differences in sign and magnitude along the latitude bands. The negative differences found in the bands that extend from equatorial to subtropical areas in the north (15° S–15° N and 15–45° N) are translated to stronger sinks in the corrected flux budgets. On the other hand, the bands containing temperate and boreal zones (90–15° S and 45–90° N) show positive differences, which imply additional sources in the corrected flux budgets.
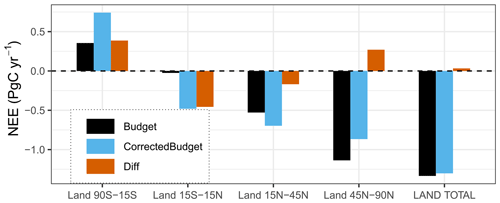
Figure 4Integrated annual differences of NEE along latitude bands; NEE estimates calculated with CS are also shown before and after the corrections due to the diurnal cycle.
Note that the size and even the sign of the effects quantified here may depend on the size and distribution of diurnal variations in the flux dataset employed in the forward runs, as well as on the inversion setup and choice of stations. Therefore, they are meant as diagnostics for the diurnal effect of CO2. Even if the corrections of the flux budgets applied in this paper are denoted in figures as “CorrectedBudget”, they are tentative changes based on the specific diurnal cycles from FLUXCOM-X and the specific inversion setup and station set used here.
The excess of simulated CO2 mole fractions resulting from using daily NEE makes the inversion adjust to stronger sinks as compensatory fluxes, while the underestimation of simulated CO2 mole fractions (seen at only a few sites) is compensated for by increasing sources. Basically, the correction to be added to the inversion using daily mean priors is an inversion of the difference of daily − hourly mean prior fluxes. Therefore, the positive differences shown in Fig. 4 lead to a weaker sink (or additional CO2 sources) in the posterior fluxes and vice versa for the negative differences.
To investigate the aforementioned compensation effect in a set of subregions across the globe, we analysed the differences calculated with CS over the set of regions used in the TransCom experiment (Gurney et al., 2003). Figure 5 indicates large changes in the annual flux budgets over most of the regions. As expected, the results exhibit positive and negative differences over land, leading to the compensation in the annual mean difference and flux estimates over the globe. In this context, negative differences imply either an underestimation of CO2 uptake or an overestimation of CO2 release by inversions when neglecting the diurnal cycle in the flux. Negative differences are found for northern and southern Africa, Eurasian temperate forests, North American boreal forests, and tropical Asia. On the other hand, positive differences are found over North American temperate forests, South American tropics and temperate forests, Eurasia boreal, Australia, and Europe. This implies that additional CO2 sources are suggested in the flux estimates for the regions exhibiting positive differences, while flux estimates of the regions with positive differences should be corrected by allocating more sinks. This, to some extent, modifies the dipoles (source–sink compensation) persistent in the inversion estimates reported in the literature, particularly the northern extratropics versus southern and tropical lands (Friedlingstein et al., 2023; Kondo et al., 2020; Peylin et al., 2002). In this case, the inversions tend to allocate stronger uptake in northern extratropical lands, relying on the gradient of observations distributed across the latitude bands at the expense of CO2 source allocations placed in the tropics and southern lands, where observational coverage is currently poor, to maintain the global atmospheric CO2 growth. Based on the results shown in Fig. 5, the large increase in sinks due to the diurnal effect suggested in Africa corresponds to reallocating large sources in North and South American temperate forests, South American tropics, and Australia. Although such additional sources are distributed over the Southern and Northern Hemisphere, the stronger uptake in Africa moderates the dipole effect in the results of CS. Additionally, the opposing flux corrections calculated between Eurasian boreal and temperate forests cancel out the effect on dipoles if the flux estimates over both regions are aggregated together.
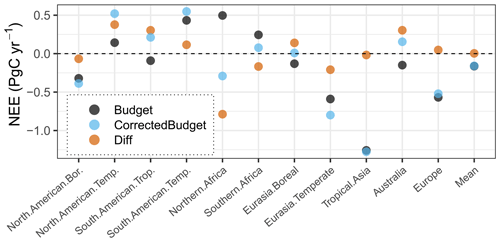
Figure 5Annual NEE estimates and their respective corrected estimates based on differences due to the diurnal cycle effect integrated over TransCom regions, averaged over the time period 2010–2020.
Moreover, the largest negative difference (−0.79 Pg C yr−1) among the TransCom regions is found in northern Africa, which would turn the flux budget from a net source of 0.50 Pg C yr−1 to a net sink of −0.29 Pg C yr−1. Over temperate North America, a large positive difference of 0.38 Pg C yr−1 is found, pushing the flux budget from a net source (about 0.15 Pg C yr−1) to a larger net source (0.53 Pg C yr−1). Given the positive and negative differences seen in “Diff” in Fig. 5, the mean annual difference for the global scale stays roughly around zero globally (see above). Generally, the posterior fluxes estimated by CS without the diurnal cycle of CO2 include biases that require corrections by additional sinks over regions that show negative differences and of additional sources over regions characterized by positive differences. These findings demonstrate that the systematic biases in the annual flux budgets get larger when disaggregating the total land to the continental scale.
3.3 Analysis of the diurnal cycle effect of CO2 over the European continent
This section will address results from the global CS and from CSR for the domain used for the regional inversion covering most of Europe (33° S–73° N, 15° W–35° E). It should be noted that this CSR domain is different than the one used for Europe in the TransCom set of regions in CS in Fig. 5, and therefore NEE differences and flux estimates are expected to be slightly different over both domains. The differences for this domain estimated with CS due to missing the CO2 diurnal cycle in CS amounts to 0.12 Pg C yr−1, averaged over the period 2010–2020. It represents about 25 % of the prior NEE uncertainty assumed for annual fluxes for this area but also exceeds the posterior uncertainty by a factor of 2. Furthermore, the mean flux budget computed for the respective years was −0.37 Pg C yr−1. Thus, this diurnal effect leads to a bias of around 32 % in the annual flux estimates in Europe. There are also slight monthly variations of the impact seen among years (Fig. 6, “CS”).
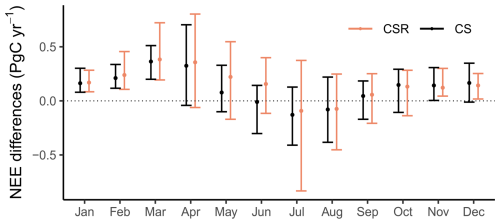
Figure 6Monthly mean NEE differences resulting from the CO2 diurnal cycle with CSR (red) and CS (black) estimated over Europe using the differences in mixing ratios for the period of time 2010–2020. Error bars mark the range of monthly differences over the analysed years.
To assess the diurnal effect on the regional inversion for Europe, CSR was used to estimate the differences in NEE due to the diurnal cycle effect passed on from the global inversion via the far-field contributions calculated by CS. The mean differences over all the years appear to be consistent in both the magnitude and temporal patterns between CSR and CS estimates, albeit larger in CSR. The mean difference calculated with CSR results in 0.15 Pg C yr−1, representing 32 % of the prior uncertainty assumed in CSR. When relating the differences to posterior uncertainty calculated with CSR over Europe, the impact even exceeds the magnitude of posterior uncertainty by a factor of around 2.5. Additionally, the CO2 diurnal effect leads to a bias of around 48 % in CO2 estimates (−0.31 Pg C yr−1) that were calculated as the mean annual flux budget for the respective years by CSR.
Although there are notable variations in monthly differences of CSR over years shown in the error bars reflecting the spread over years (see Fig. 6, “CSR”), they agree in the magnitude and in month-to-month variations with those of CS. The seasonality in differences suggests a compensation effect when integrating NEE estimates to the annual scale. Additionally, the findings demonstrate a smaller year-to-year variability of the CO2 diurnal effect during winter compared to the variability during spring and summer. This leads to dominant contributions of spring and summer fluxes to the interannual variability of NEE estimates resulting from the CO2 diurnal cycle.
3.4 How much does the CO2 diurnal cycle affect interannual variability?
Figure 7 illustrates the annual flux differences due to the diurnal cycle effect of CO2 and the corresponding flux estimates. The findings indicate how much the lack of the CO2 flux diurnal cycle affects annual CO2 flux budgets for the individual years, globally and regionally, over the CSR-Europe domain. Including the diurnal cycle of CO2 generally shifted estimates towards sources over all the years, albeit in different magnitudes. The analysis here confirms that the interannual variability (IAV) of flux estimates is less sensitive to the diurnal variations at the global scale (“Global”; see Fig. 7). Although the mean annual flux differences between daily and hourly NEE-based inversions stay around zero over the analysed years, they can be slightly larger in individual years (standard deviation of 0.13 Pg C yr−1). Furthermore, the similarity of IAV for the annual flux estimates and the corrected estimates (0.82 and 0.88 Pg C yr−1, respectively) indicates the negligible influence of CO2 diurnal variations on the IAV of global estimates. By contrast, the results demonstrate a larger impact on the IAV of the regional flux estimates over Europe. The NEE differences calculated by CS resulted in an IAV amplitude of −0.06 Pg C yr−1, about half the IAV amplitude of the estimated fluxes (−0.14 Pg C yr−1).
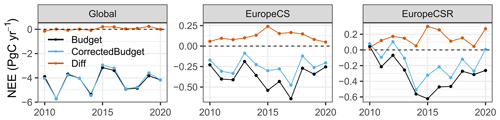
Figure 7Annual flux budgets (before and after corrections due to the CO2 diurnal cycle) estimated using CS over the globe and Europe using CS and over Europe using CSR for 2010–2020.
In terms of the indirect effect of diurnal variations passed to the regional inversion through far-field influences, CSR suggests a slightly weaker uptake (−0.16 Pg C yr−1) than CS does (−0.25 Pg C yr−1) after taking the diurnal cycle effect into consideration. The IAV of the differences over Europe calculated with CSR amounts to 0.09 Pg C yr−1, suggesting a significant impact when comparing it with the IAV of the annual flux estimates 0.20 Pg C yr−1. It is noteworthy that CS and CSR differ in their atmospheric transport models, and the spatial correlation of prior uncertainty is chosen differently. Consequently, such different setups likely result in the discrepancies between CS and CSR in evaluating the impact of the CO2 diurnal cycle.
To show the spatial patterns of the differences in the domain of Europe, posterior NEE estimated in a regional (CSR) inversion is analysed together with the differences due to the diurnal variations in CS and the corrected estimates. Figure 8 depicts the annual mean of NEE without and with the diurnal cycle of CO2 taken into account and the differences, averaged over 11 years. Positive corrections to NEE fluxes occur generally along the Mediterranean coast (southern and western Europe), as well as north-western Europe (southern UK, Benelux, northern Germany). On the other hand, over Scandinavia, over the northern UK, and in central Europe, smaller negative corrections (meaning higher CO2 uptake in the respective regions) are persistent. These findings refer to a notable impact of CO2 diurnal cycle on NEE estimated over smaller local domains, particularly over regions where CO2 exchange between the atmosphere and terrestrial ecosystems is more active. It should be noted that because of the partial compensation between subregions with positive and negative differences, the annual mean difference in NEE fluxes will also become smaller as larger areas are aggregated, underlining the increasing importance of the CO2 diurnal effect as finer spatiotemporal scales are analysed.
The seasonal features of the covariation between atmospheric transport convection and terrestrial CO2 fluxes discussed in Denning et al. (1995, 1996b, a) hold true for the diurnal variability of both the atmospheric dynamics and biota. Ignoring the diurnal cycle of CO2 in the biosphere fluxes used as priors in inversions results in significant biases in CO2 mole fraction simulations calculated with atmospheric transport models. In this study, we quantified the effect on the CO2 mole fraction and estimated NEE arising from the diurnal cycle of CO2 at global and regional scales. We find that CO2 mole fractions simulated during the daytime at sites that are dominated by land footprints with the inclusion of the biogenic diurnal variability tend to be lower than those simulated with daily averaged biogenic fluxes; the opposite effect is found for the simulations calculated during nighttime over mountain sites, as well as over sites that are more representative for ocean backgrounds where the biosphere signal is generally weak and lagged. Also, the impact found over locations with dominant land backgrounds that also have a larger number of sites was much larger in magnitude than that found over locations with ocean backgrounds. This typically points to the prevalence of the diurnal effect over lands, particularly in the biogenic terrestrial ecosystems in the Northern Hemisphere.
The differences in simulated CO2 mixing ratios inevitably lead to differences in NEE estimates derived from atmospheric inversions. Regarding the global total flux, the impact of ignoring the diurnal cycle is compensated for by the seasonal and spatial variations, as the inversion constrains reasonably well the long-term mean CO2 flux irrespective of when and where the flux adjustments are allocated. This is evident by the small annual difference of 2 % of the global land flux estimate (−1.37 Pg C yr−1), which lies within the range of total atmosphere-to-land sinks (1.10 and 1.70 Pg C yr−1) estimated by inversions as reported to the Global Carbon Budget (GCB) in Friedlingstein et al. (2022), to which CS contributes. Consequently, neither IAV nor the magnitude of global flux estimates significantly changed after applying corrections of the CO2 diurnal effect over the analysed period of time, indicating a negligible impact on the integrated global flux budget.
However, the diurnal cycle of CO2 does influence NEE estimates calculated at regional domains. For example, results analysed over the land region within the CSR domain covering Europe yield an NEE difference of 48 % and 32 % of the annual estimates calculated by CSR-Europe and the global CS, respectively. The annual mean differences represent a shift of flux estimates towards higher CO2 sources in both inversions. Even though the diurnal cycle is taken into account in CSR within the regional domain using biogenic prior fluxes obtained from biosphere flux models at hourly time intervals, the differences from the global CS are passed to CSR estimates as an indirect effect through the lateral boundary conditions. By comparing two products of lateral boundary conditions obtained from CS and TM5-4DVar global inversions, Munassar et al. (2023) also found a non-negligible sensitivity of regional inversions to systematic biases in lateral boundary conditions. They found an impact of 0.40 Pg C yr−1 resulting from the lateral boundary conditions over the CSR domain of Europe for 2018. As the diurnal cycle of CO2 was missing in the setup of CS used in that study, part of this impact can potentially be attributed to the diurnal cycle effect. This suggests that the systematic biases in regional inversions due to lateral boundary conditions would be attenuated to approximately 0.25 Pg C yr−1 if taking into account a bias of 0.15 Pg C yr−1 arising from the diurnal cycle effect found in CS via this study.
The problem of the seasonal rectifier considered by Denning et al. (1995) could be solved by explicitly estimating seasonal variations from the atmospheric data. Unlike this, unfortunately, the diurnal cycle cannot be deduced from the atmospheric data, primarily owing to the limitation of the assimilated data to either daytime or nighttime. Even though a weak variability may emerge due to the diurnal variability of atmospheric vertical transport, it cannot reproduce a realistic rectification observed in atmospheric CO2 concentrations (Yi et al., 2000) without coupling the transport variability with a priori information of terrestrial flux variability. In an experiment done at a site level with and without diurnal biosphere variations, Denning et al. (1996a) found that diurnal CO2 concentrations simulated with diurnal flux variations were more realistic in phase when compared to observations, while those simulated with mean prescribed biogenic fluxes follow the phase of the planetary boundary layer (PBL) depth. This indicates the importance of accounting for the diurnal variations when retrieving atmospheric CO2 dry mole fractions. Further assessment of the uncertainty of such variations produced from biosphere models will benefit the characterization of estimated CO2 flux errors among the inversions contributing to the Global Carbon Project (GCP) but also extend the understanding of biogenic terrestrial variability. Notwithstanding, the FLUXCOM-X model, used in our experiment, is thought to have a good ability to resolve the diurnal cycle of CO2 because the model is trained against observations, meteorology, and remote sensing data using different prediction approaches to reproduce GPP at half-hourly timescales (Bodesheim et al., 2018; Nelson et al., 2024). This is supported by a validation analysis by Nelson et al. (2024) using the FLUXCOM-X dataset. The outcome of this analysis suggests that FLUXCOM-X provides good pieces of information regarding the diurnal variations, in spite of some shortcomings concerning the seasonal and interannual variabilities. Such flux products can thus be beneficial for global inversions to provide a better constraint for CO2 diurnal cycle, provided that seasonal and interannual variations are already constrained by CO2 observations. Nevertheless, the eddy covariance measurements needed to estimate and validate diurnal cycles in upscaling products like FLUXCOM-X are known to represent only a certain part of the biosphere; thus the representativity of the upscaled fluxes on continental scales is hard to assess.
In a further assessment, the differences in NEE due to the CO2 diurnal effect were analysed (over three latitude land bands representing the Southern Hemisphere, the tropics, and the Northern Hemisphere) using biosphere fluxes from the Carnegie–Ames–Stanford Approach (CASA) (Potter et al., 1993) instead of FLUXCOM-X (Fig. 9). The biogenic flux products of CASA are used by – and provided through – the Carbon Tracker inversion CT2022 (Jacobson et al., 2023), where the temporal downscaling of monthly net primary productivity (NPP) and ecosystem respiration is performed using surface solar radiation and temperature from ERA5 reanalysis following the methodology by Olsen and Randerson (2004) to include the diurnal cycle of CO2. The differences in NEE calculated with CASA-based fluxes were estimated by CarboScope in the same way as the differences estimated with FLUXCOM-X, as described in the Methods section. Quite small differences were found between daily and hourly fluxes calculated with CASA compared to those calculated with FLUXCOM-X over the three latitude bands. This implies that the CO2 diurnal cycle retrieved using the Olsen–Randerson method is much weaker than that calculated with FLUXCOM-X. It is noteworthy that the Olsen–Randerson method is used by several global inversions to produce diurnal variations for the biogenic fluxes if not yet represented by the biosphere flux models themselves used as priors. Therefore, such discrepancies in resolving the CO2 diurnal cycle among biosphere models suggest that more work is needed regarding the best methodology to be applied to generate the diurnal variations.
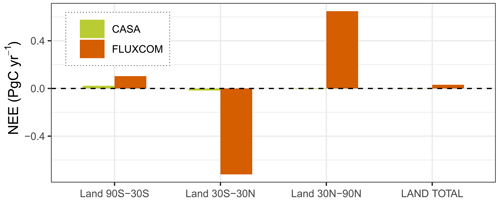
Figure 9Differences in NEE resulting from the diurnal cycle effect of CO2 estimated by CarboScope using two biosphere models (CASA-based biogenic fluxes in green bars and FLUXCOM-X in red bars), averaged over 2010–2020 across a set of latitude land bands indicated on the x axis.
In the tropics (30° S–30° N) the difference was found to be −0.65 Pg C yr−1 over land, proposing a much stronger sink (−1.48 Pg C yr−1) than CS estimated (−0.83 Pg C yr−1). Friedlingstein et al. (2022) found that atmospheric inversions suggested the land tropics to be close to neutral over the past decade ranging between −0.90 and 0.70 Pg C yr−1, with high uncertainty. After considering the impact of the diurnal cycle, CS estimates for the tropical lands will tend to result in even stronger CO2 sinks. Conversely, latitude bands containing temperate forest zones show weaker CO2 uptake when the correction due to the diurnal cycle is taken into account. That is, the land sink over the northern extra-tropics (30–90° N) amounts to −0.53 Pg C yr−1 after correcting for the diurnal cycle effect compared to −1.12 Pg C yr−1 estimated with CS for 2010–2020. Hence, the underestimation of CO2 uptake in the tropics and the overestimation of CO2 uptake in temperate zones constitute the trade-off that maintains the CO2 mass balance in the global carbon budgets derived by CS.
Given the non-negligible impact of the diurnal cycle of CO2 over the latitude bands, the impact is quantified in the context of annual flux budgets at continental and regional scales using CS results over the globe. NEE differences were analysed over a set of regions, encompassing the whole land area around the world, as used in the TransCom experiment (Gurney et al., 2003), and are shown in Fig. 5. The differences over most of the land regions exhibit large biases in the posterior annual fluxes over the analysed regions. This has been outlined by applying flux corrections that suggest additional sources in the posterior annual fluxes over some regions such as the temperate North America region, while stronger sinks are suggested over other regions, as is the case for northern Africa. The differences of the two largest cases in northern Africa and in temperate North America (−0.79 and 0.38 Pg C yr−1, respectively) exceed the corresponding uncertainties (0.54 and 0.22 Pg C yr−1, respectively) calculated as the spread among different model estimates reported in Gurney et al. (2004) within the TransCom Experiment. The global mean difference is, however, small and consistent with the global total uncertainty. These findings indicate that misallocations of flux adjustments in different regions occur as a result of lacking diurnal information in the atmospheric inversions. It is noteworthy that some regions that show large differences such as Africa are repeatedly characterized by a larger uncertainty in inversion estimates by previous studies (e.g. Gurney et al., 2004; Stephens et al., 2007). These studies attribute the compensatory fluxes over such regions to the lack of observational constraints and thus may be allocated with the surplus of flux budgets remaining from the well-constrained regions. Moreover, estimates of terrestrial CO2 fluxes by inversions reported in other studies generally suffer from larger uncertainties over North and South America and Africa. This is in principle attributed partially to the lack of observational constraint, as reported by Byrne et al. (2023), in which several global inversions were utilized with different setups of atmospheric dataset sources obtained from in situ and OCO-2 satellite measurements in the Model Intercomparison Project (MIP) to estimate net carbon exchange (NCE). However, the inversions in that study included prior biosphere fluxes from various models that have different prescriptions for estimating the diurnal variations such as CASA, SiB-4, ORCHIDEE, and CARDAMOM. The posterior estimates are likely to be driven by prior flux models, especially over tropical regions as a consequence of not having enough observations. This is reflected in the increase in spatial uncertainty estimated by Byrne et al. (2023) across tropical and subtropical regions, where substantial differences in NEE estimates are found in our study due to the diurnal CO2 effect. Therefore, the substantial discrepancies in posterior CO2 fluxes calculated using global inversions over regional scales, e.g. North and South America and Africa, as reported by Friedlingstein et al. (2023), not only are caused by transport and lack of observations but also involve a contribution from inaccuracies in representing CO2 diurnal variations estimated by bottom-up biosphere models used as priors in Bayesian inversions.
NEE differences due to the diurnal cycle effect show year-to-year variations that affect IAV of the flux estimates. This is evident by the sensitivity of NEE IAV to the diurnal cycle of CO2 assessed over the TransCom land regions (Table 2). Regions with a large mean difference in NEE also tend to have a larger IAV of the diurnal cycle impact, such as northern Africa, North American temperate forests, and South American tropics, but also the rest of the land regions suggest a large IAV in NEE differences. This finding implies that both the magnitude of NEE estimates and IAV are affected by the neglect of the CO2 diurnal cycle at regional scales. It is noteworthy that this analysis is performed with a global in situ site network using 147 sites, representing the total number of stations available to provide measurements for normal CS inversions. Hence, the IAV can be subject to changes if the analysis was done with a different set of stations and with different temporal coverage of the dataset over the analysed period 2010–2020. Even though evaluating the uncertainty of diurnal variations remains an important target for future work, the inclusion of such variations in biogenic prior fluxes used in inversions should generally decrease biases in CO2 mole fraction simulations and thus reduce biases in estimated CO2 fluxes by inversions.
Table 2Sensitivity of IAV to the impact of CO2 diurnal cycle. “Diff. IAV” corresponds to the IAV of the impact of diurnal cycle on retrieved fluxes, “Flux IAV” corresponds to the IAV of the estimated fluxes themselves, and “Flux IAV corr.” corresponds to IAV of estimated fluxes after corrections.
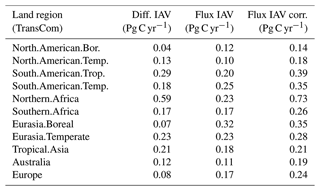
Based on the results, the stronger uptake dominant in the Northern Hemisphere attributed to the CO2 fertilization effect and deposition of nitrogen (Ciais et al., 2019; Sarmiento et al., 2010) cannot be predicted accurately by inversions that do not include diurnal cycle of CO2 in their prior fluxes. The greatest impact of the CO2 diurnal effect obviously appears over regions with strong biological activities, as seen from a diagnostic map of CO2 mole fractions simulated at the model surface level with the inverted response of the diurnal effect across the world (Fig. 10). Averaging out the diurnal variations in the biosphere flux model FLUXCOM-X has led to overestimation of CO2 mole fractions, largely over the temperate areas where a large amount of carbon is stored in the temperate forests in North America, Europe, and eastern Asia in the Northern Hemisphere as well as over the rainforests of South America and Australia in the Southern Hemisphere (Erb et al., 2018).
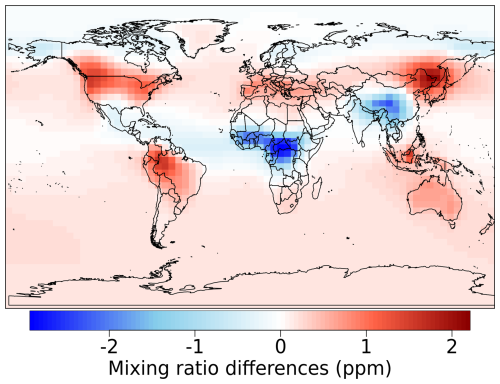
Figure 10The 2-D fields of CO2 mole fractions simulated using the inverted response of the diurnal cycle (i.e. inverted differences of mole fractions calculated with daily and hourly NEE data) for local daytime at the model surface level for the period 2010–2020 over the globe with TM3.
By contrast, CS tends to underestimate CO2 mole fractions as the CO2 diurnal cycle of FLUXCOM-X is flattened in the tropical areas, in particular central Africa and south-eastern Asia. This pattern is also, to a lesser extent, seen over the boreal forests in the further north. These results outline the discrepancies in the annual flux budgets computed over the TransCom regions and over the latitude bands. It should be noted that these corrections are used in this study as diagnostics as the diurnal cycle is subject to uncertainty depending on the accuracy of the biosphere model to reproduce diurnal variations. Additionally, the spatial patterns of mole fractions over Europe in Fig. 10 correspond quite well to the spatial patterns of posterior flux differences estimated by CSR (Fig. 8).
Ignoring the diurnal cycle in surface-to-atmosphere CO2 fluxes leads to a systematic bias in CO2 mole fraction simulations sampled at daytime because the daily mean flux systematically misses the CO2 uptake during the daytime hours. In an atmospheric inversion using daytime-selected CO2 measurements at most continental sites and not resolving diurnal cycles in the flux (as is the case for the 60-year global CarboScope CO2 inversion that operates on a daily flux time step due to limitation in computer memory), this leads to systematic biases in the estimates of the annual sources and sinks of atmospheric CO2. In the case of the absence of diurnal variations in the prior inputs, correcting the flux estimates is essential to reduce these systematic biases. Such a correction can be applied either to the mole fractions before performing the inversions or to the optimized fluxes after performing the inversions. In this paper, we diagnosed how large such a flux correction would need to be in annual carbon flux budgets derived from CS and CSR for 2010–2020 at global and regional scales. NEE containing the diurnal cycle of CO2 flux was obtained from the data-driven biosphere flux estimate by FLUXCOM-X (Nelson et al., 2024) at hourly timescales over the globe. These fluxes were transported by the TM3 model to simulate CO2 mole fractions. Removing the diurnal cycle of NEE using daily fluxes leads to an increase in simulated CO2 mole fractions in comparison with simulations done with hourly NEE because of the diurnal effect described above. The difference between the simulations was inverted to obtain the impact of this effect on the inversion results. Regarding the net fluxes of CO2 estimated by the global CarboScope (CS) inversion, the difference in the flux budget due to the diurnal cycle was negligible at the global scale, which is expected as the global annual trend of CO2 is well constrained by the observations. However, based on FLUXCOM-X diurnal cycles, the impact at the regional and local scales amounts to up to about 51 % relative to the sum of differences and flux estimates, depending on region. The analysis of NEE differences across latitudinal bands and for the set of regions used in the TransCom experiment points to larger differences exceeding the magnitude of the estimated annual flux budgets in some regions such as North American temperate and northern Africa. The overall NEE differences in the TransCom land regions suggest a weaker sink in temperate zones (particularly in the north) and a stronger sink in parts of the tropics than estimated when ignoring the diurnal cycle. This illustrates a compensation effect between the regions to retain the global mass balance as discussed above. In addition to the mean flux, the IAV of flux estimates is also affected by the diurnal variations. This indicates that it may be unrealistic to use climatological diurnal variations to correct the atmospheric CO2 inversions, though further investigations are required to look into the origin of IAV in the NEE differences (e.g. to determine how much of the biases comes from the amplitude of the diurnal cycle of CO2 in the biosphere model and from the PBL in the transport model).
When replacing FLUXCOM-X diurnal cycles by diurnal cycles according to the Olsen–Randerson method, our analysis results in a substantially smaller impact on the inversion results. The Olsen–Randerson method is used in several global inversions taking part in the Global Carbon Budget of Friedlingstein et al. (2023). Hence, a more comprehensive assessment of the uncertainty of the diurnal cycle effect among atmospheric inversions is planned in a follow-up study.
NEE estimates derived from a regional inversion, even if using a diurnal cycle in its regional prior, are still prone to an indirect impact of the diurnal cycle effect through the lateral boundary conditions if coming from a global inversion that is missing diurnal variations. In our case of CarboScope-Regional (CSR) for Europe using boundary conditions from the global CS, such an impact amounts to approximately 50 % of the mean annual flux estimates calculated over Europe.
We conclude that incorporating diurnal variations into the prior fluxes, directly or via a suitable correction, is important in global and regional atmospheric CO2 inversions. Further work is needed to assess the uncertainty of the effect and to develop a suitable implementation of the diurnal cycle effect into the global CarboScope CO2 inversion.
The simulations of CO2 mole fractions, NEE differences derived from the inversions, and codes used for the analysis can be made available upon request to the corresponding author.
SM, CG, and CR designed the study. SM performed the simulations and analysis and drafted the paper with contributions from CR. CG, KUT, MG, FTK, and SB provided support. All the authors revised the paper and edited the text.
At least one of the (co-)authors is a member of the editorial board of Atmospheric Chemistry and Physics. The peer-review process was guided by an independent editor, and the authors also have no other competing interests to declare.
Publisher's note: Copernicus Publications remains neutral with regard to jurisdictional claims made in the text, published maps, institutional affiliations, or any other geographical representation in this paper. While Copernicus Publications makes every effort to include appropriate place names, the final responsibility lies with the authors.
The authors thank Sönke Zaehle for his valuable comments on the manuscript in the internal review. We also acknowledge the use of hourly NEE data calculated with FLUXCOM-X provided by Jake Nelson and Sophia Walter as well as CarbonTracker CT2022 and CT-NRT.v2023-3 results provided by NOAA GML, Boulder, Colorado, USA from the website at http://carbontracker.noaa.gov (last access: 11 January 2025). This work used resources of the Deutsches Klimarechenzentrum (DKRZ) granted by its Scientific Steering Committee (WLA) under project ID bm1400.
This research has been supported by the German Federal Ministry of Education and Research (BMBF) project “Integrated Greenhouse Gas Monitoring System for Germany – Modellierung (ITMS M)” (grant no. 01 LK2102A).
The article processing charges for this open-access publication were covered by the Max Planck Society.
This paper was edited by Tuukka Petäjä and reviewed by two anonymous referees.
Baker, D. F., Law, R. M., Gurney, K. R., Rayner, P., Peylin, P., Denning, A. S., Bousquet, P., Bruhwiler, L., Chen, Y. H., Ciais, P., Fung, I. Y., Heimann, M., John, J., Maki, T., Maksyutov, S., Masarie, K., Prather, M., Pak, B., Taguchi, S., and Zhu, Z.: TransCom 3 inversion intercomparison: Impact of transport model errors on the interannual variability of regional CO2 fluxes, 1988–2003, Global Biogeochem. Cy., 20, GB1002, https://doi.org/10.1029/2004gb002439, 2006.
Berchet, A., Sollum, E., Thompson, R. L., Pison, I., Thanwerdas, J., Broquet, G., Chevallier, F., Aalto, T., Berchet, A., Bergamaschi, P., Brunner, D., Engelen, R., Fortems-Cheiney, A., Gerbig, C., Groot Zwaaftink, C. D., Haussaire, J.-M., Henne, S., Houweling, S., Karstens, U., Kutsch, W. L., Luijkx, I. T., Monteil, G., Palmer, P. I., van Peet, J. C. A., Peters, W., Peylin, P., Potier, E., Rödenbeck, C., Saunois, M., Scholze, M., Tsuruta, A., and Zhao, Y.: The Community Inversion Framework v1.0: a unified system for atmospheric inversion studies, Geosci. Model Dev., 14, 5331–5354, https://doi.org/10.5194/gmd-14-5331-2021, 2021.
Bodesheim, P., Jung, M., Gans, F., Mahecha, M. D., and Reichstein, M.: Upscaled diurnal cycles of land–atmosphere fluxes: a new global half-hourly data product, Earth Syst. Sci. Data, 10, 1327–1365, https://doi.org/10.5194/essd-10-1327-2018, 2018.
Botía, S.: Greenhouse gas exchange in the Amazon: Carbon dioxide and methane insights from the Amazon Tall Tower Observatory, PhD, Wageningen University, Wageningen, the Netherlands, https://doi.org/10.18174/573967, 2022.
Byrne, B., Baker, D. F., Basu, S., Bertolacci, M., Bowman, K. W., Carroll, D., Chatterjee, A., Chevallier, F., Ciais, P., Cressie, N., Crisp, D., Crowell, S., Deng, F., Deng, Z., Deutscher, N. M., Dubey, M. K., Feng, S., García, O. E., Griffith, D. W. T., Herkommer, B., Hu, L., Jacobson, A. R., Janardanan, R., Jeong, S., Johnson, M. S., Jones, D. B. A., Kivi, R., Liu, J., Liu, Z., Maksyutov, S., Miller, J. B., Miller, S. M., Morino, I., Notholt, J., Oda, T., O'Dell, C. W., Oh, Y.-S., Ohyama, H., Patra, P. K., Peiro, H., Petri, C., Philip, S., Pollard, D. F., Poulter, B., Remaud, M., Schuh, A., Sha, M. K., Shiomi, K., Strong, K., Sweeney, C., Té, Y., Tian, H., Velazco, V. A., Vrekoussis, M., Warneke, T., Worden, J. R., Wunch, D., Yao, Y., Yun, J., Zammit-Mangion, A., and Zeng, N.: National CO2 budgets (2015–2020) inferred from atmospheric CO2 observations in support of the global stocktake, Earth Syst. Sci. Data, 15, 963–1004, https://doi.org/10.5194/essd-15-963-2023, 2023.
Canadell, J. G., Monteiro, P. M. S., Costa, M. H., Cunha, L. C. d., Cox, P. M., Eliseev, A. V., Henson, S., Ishii, M., Jaccard, S., Koven, C., Lohila, A., Patra, P. K., Piao, S., Rogelj, J., Syampungani, S., Zaehle, S., and Zickfeld, A. K.: Global Carbon and Other Biogeochemical Cycles and Feedbacks, in: Climate Change 2021 – The Physical Science Basis: Working Group I Contribution to the Sixth Assessment Report of the Intergovernmental Panel on Climate Change, edited by: Masson-Delmotte, V., Zhai, P., Pirani, A., Connors, S. L., Péan, C., Berger, S., Caud, N., Chen, Y., Goldfarb, L., Gomis, M. I., Huang, M., Leitzell, K., Lonnoy, E., Matthews, J. B. R., Maycock, T. K., Waterfield, T., Yelekçi, O., Yu, R., and Zhou, B., Cambridge University Press, Cambridge, 673–816, https://doi.org/10.1017/9781009157896.007, 2023.
Chan, D., Ishizawa, M., Higuchi, K., Maksyutov, S., and Chen, J.: Seasonal CO2rectifier effect and large-scale extratropical atmospheric transport, J. Geophys. Res., 113, D17309, https://doi.org/10.1029/2007jd009443, 2008.
Chandra, N., Patra, P. K., Niwa, Y., Ito, A., Iida, Y., Goto, D., Morimoto, S., Kondo, M., Takigawa, M., Hajima, T., and Watanabe, M.: Estimated regional CO2 flux and uncertainty based on an ensemble of atmospheric CO2 inversions, Atmos. Chem. Phys., 22, 9215–9243, https://doi.org/10.5194/acp-22-9215-2022, 2022.
Chevallier, F., Palmer, P. I., Feng, L., Boesch, H., O'Dell, C. W., and Bousquet, P.: Toward robust and consistent regional CO2 flux estimates from in situ and spaceborne measurements of atmospheric CO2, Geophys. Res. Lett., 41, 1065–1070, https://doi.org/10.1002/2013gl058772, 2014.
Chevallier, F., Broquet, G., Pierangelo, C., and Crisp, D.: Probabilistic global maps of the CO2 column at daily and monthly scales from sparse satellite measurements, J. Geophys. Res.-Atmos., 122, 7614–7629, https://doi.org/10.1002/2017JD026453, 2017.
Ciais, P., Rayner, P., Chevallier, F., Bousquet, P., Logan, M., Peylin, P., and Ramonet, M.: Atmospheric inversions for estimating CO2 fluxes: methods and perspectives, Climatic Change, 103, 69–92, https://doi.org/10.1007/s10584-010-9909-3, 2010.
Ciais, P., Tan, J., Wang, X., Roedenbeck, C., Chevallier, F., Piao, S.-L., Moriarty, R., Broquet, G., Le Quéré, C., and Canadell, J.: Five decades of northern land carbon uptake revealed by the interhemispheric CO2 gradient, Nature, 568, 221–225, 2019.
Deng, A. J., Lauvaux, T., Davis, K. J., Gaudet, B. J., Miles, N., Richardson, S. J., Wu, K., Sarmiento, D. P., Hardesty, R. M., Bonin, T. A., Brewer, W. A., and Gurney, K. R.: Toward reduced transport errors in a high resolution urban CO2 inversion system, Elem.-Sci. Anth., 5, 20, https://doi.org/10.1525/elementa.133, 2017.
Denning, A. S., Fung, I. Y., and Randall, D.: Latitudinal gradient of atmospheric CO2 due to seasonal exchange with land biota, Nature, 376, 240–243, https://doi.org/10.1038/376240a0, 1995.
Denning, A. S., Randall, D. A., Collatz, G. J., and Sellers, P. J.: Simulations of terrestrial carbon metabolism and atmospheric CO2 in a general circulation model, Tellus B, 48, 543–567, https://doi.org/10.1034/j.1600-0889.1996.t01-1-00010.x, 1996a.
Denning, A. S., Randall, D. A., Collatz, G. J., and Sellers, P. J.: Simulations of terrestrial carbon metabolism and atmospheric CO2 in a general circulation model: Part 2: Simulated CO2 concentrations, Tellus B, 48, 543–567, 1996b.
Denning, A. S., Takahashi, T., and Friedlingstein, P.: Can a strong atmospheric CO2 rectifier effect be reconciled with a “reasonable” carbon budget? Keynote Perspective, Tellus B, 51, 249–253, 1999.
Engelen, R. J.: On error estimation in atmospheric CO2inversions, J. Geophys. Res., 107, 4635, https://doi.org/10.1029/2002jd002195, 2002.
Enting, I. G.: Inverse problems in atmospheric constituent transport, Cambridge University Press, Cambridge, New York, xv, 392 pp., ISBN 978-0-521-81210-8, 2002.
Erb, K.-H., Kastner, T., Plutzar, C., Bais, A. L. S., Carvalhais, N., Fetzel, T., Gingrich, S., Haberl, H., Lauk, C., Niedertscheider, M., Pongratz, J., Thurner, M., and Luyssaert, S.: Unexpectedly large impact of forest management and grazing on global vegetation biomass, Nature, 553, 73–76, https://doi.org/10.1038/nature25138, 2018.
Friedlingstein, P., Jones, M. W., O'Sullivan, M., Andrew, R. M., Bakker, D. C. E., Hauck, J., Le Quéré, C., Peters, G. P., Peters, W., Pongratz, J., Sitch, S., Canadell, J. G., Ciais, P., Jackson, R. B., Alin, S. R., Anthoni, P., Bates, N. R., Becker, M., Bellouin, N., Bopp, L., Chau, T. T. T., Chevallier, F., Chini, L. P., Cronin, M., Currie, K. I., Decharme, B., Djeutchouang, L. M., Dou, X., Evans, W., Feely, R. A., Feng, L., Gasser, T., Gilfillan, D., Gkritzalis, T., Grassi, G., Gregor, L., Gruber, N., Gürses, Ö., Harris, I., Houghton, R. A., Hurtt, G. C., Iida, Y., Ilyina, T., Luijkx, I. T., Jain, A., Jones, S. D., Kato, E., Kennedy, D., Klein Goldewijk, K., Knauer, J., Korsbakken, J. I., Körtzinger, A., Landschützer, P., Lauvset, S. K., Lefèvre, N., Lienert, S., Liu, J., Marland, G., McGuire, P. C., Melton, J. R., Munro, D. R., Nabel, J. E. M. S., Nakaoka, S.-I., Niwa, Y., Ono, T., Pierrot, D., Poulter, B., Rehder, G., Resplandy, L., Robertson, E., Rödenbeck, C., Rosan, T. M., Schwinger, J., Schwingshackl, C., Séférian, R., Sutton, A. J., Sweeney, C., Tanhua, T., Tans, P. P., Tian, H., Tilbrook, B., Tubiello, F., van der Werf, G. R., Vuichard, N., Wada, C., Wanninkhof, R., Watson, A. J., Willis, D., Wiltshire, A. J., Yuan, W., Yue, C., Yue, X., Zaehle, S., and Zeng, J.: Global Carbon Budget 2021, Earth Syst. Sci. Data, 14, 1917–2005, https://doi.org/10.5194/essd-14-1917-2022, 2022.
Friedlingstein, P., O'Sullivan, M., Jones, M. W., Andrew, R. M., Bakker, D. C. E., Hauck, J., Landschützer, P., Le Quéré, C., Luijkx, I. T., Peters, G. P., Peters, W., Pongratz, J., Schwingshackl, C., Sitch, S., Canadell, J. G., Ciais, P., Jackson, R. B., Alin, S. R., Anthoni, P., Barbero, L., Bates, N. R., Becker, M., Bellouin, N., Decharme, B., Bopp, L., Brasika, I. B. M., Cadule, P., Chamberlain, M. A., Chandra, N., Chau, T.-T.-T., Chevallier, F., Chini, L. P., Cronin, M., Dou, X., Enyo, K., Evans, W., Falk, S., Feely, R. A., Feng, L., Ford, D. J., Gasser, T., Ghattas, J., Gkritzalis, T., Grassi, G., Gregor, L., Gruber, N., Gürses, Ö., Harris, I., Hefner, M., Heinke, J., Houghton, R. A., Hurtt, G. C., Iida, Y., Ilyina, T., Jacobson, A. R., Jain, A., Jarníková, T., Jersild, A., Jiang, F., Jin, Z., Joos, F., Kato, E., Keeling, R. F., Kennedy, D., Klein Goldewijk, K., Knauer, J., Korsbakken, J. I., Körtzinger, A., Lan, X., Lefèvre, N., Li, H., Liu, J., Liu, Z., Ma, L., Marland, G., Mayot, N., McGuire, P. C., McKinley, G. A., Meyer, G., Morgan, E. J., Munro, D. R., Nakaoka, S.-I., Niwa, Y., O'Brien, K. M., Olsen, A., Omar, A. M., Ono, T., Paulsen, M., Pierrot, D., Pocock, K., Poulter, B., Powis, C. M., Rehder, G., Resplandy, L., Robertson, E., Rödenbeck, C., Rosan, T. M., Schwinger, J., Séférian, R., Smallman, T. L., Smith, S. M., Sospedra-Alfonso, R., Sun, Q., Sutton, A. J., Sweeney, C., Takao, S., Tans, P. P., Tian, H., Tilbrook, B., Tsujino, H., Tubiello, F., van der Werf, G. R., van Ooijen, E., Wanninkhof, R., Watanabe, M., Wimart-Rousseau, C., Yang, D., Yang, X., Yuan, W., Yue, X., Zaehle, S., Zeng, J., and Zheng, B.: Global Carbon Budget 2023, Earth Syst. Sci. Data, 15, 5301–5369, https://doi.org/10.5194/essd-15-5301-2023, 2023.
Gerbig, C., Lin, J. C., Wofsy, S. C., Daube, B. C., Andrews, A. E., Stephens, B. B., Bakwin, P. S., and Grainger, C. A.: Toward constraining regional-scale fluxes of CO2 with atmospheric observations over a continent: 2. Analysis of COBRA data using a receptor-oriented framework, J. Geophys. Res.-Atmos., 108, 4757, https://doi.org/10.1029/2003jd003770, 2003.
Gerbig, C., Körner, S., and Lin, J. C.: Vertical mixing in atmospheric tracer transport models: error characterization and propagation, Atmos. Chem. Phys., 8, 591–602, https://doi.org/10.5194/acp-8-591-2008, 2008.
Glanemann, N., Willner, S. N., and Levermann, A.: Paris Climate Agreement passes the cost-benefit test, Nat Commun, 11, 110, https://doi.org/10.1038/s41467-019-13961-1, 2020.
Guimberteau, M., Zhu, D., Maignan, F., Huang, Y., Yue, C., Dantec-Nédélec, S., Ottlé, C., Jornet-Puig, A., Bastos, A., Laurent, P., Goll, D., Bowring, S., Chang, J., Guenet, B., Tifafi, M., Peng, S., Krinner, G., Ducharne, A., Wang, F., Wang, T., Wang, X., Wang, Y., Yin, Z., Lauerwald, R., Joetzjer, E., Qiu, C., Kim, H., and Ciais, P.: ORCHIDEE-MICT (v8.4.1), a land surface model for the high latitudes: model description and validation, Geosci. Model Dev., 11, 121–163, https://doi.org/10.5194/gmd-11-121-2018, 2018.
Gurney, K. R., Law, R. M., Denning, A. S., Rayner, P. J., Baker, D., Bousquet, P., Bruhwiler, L., Chen, Y. H., Ciais, P., Fan, S. M., Fung, I. Y., Gloor, M., Heimann, M., Higuchi, K., John, J., Kowalczyk, E., Maki, T., Maksyutov, S., Peylin, P., Prather, M., Pak, B. C., Sarmiento, J., Taguchi, S., Takahashi, T., and Yuen, C. W.: TransCom 3 CO2 inversion intercomparison: 1. Annual mean control results and sensitivity to transport and prior flux information, Tellus B, 55, 555–579, https://doi.org/10.1034/j.1600-0889.2003.00049.x, 2003.
Gurney, K. R., Law, R. M., Denning, A. S., Rayner, P. J., Pak, B. C., Baker, D., Bousquet, P., Bruhwiler, L., Chen, Y.-H., Ciais, P., Fung, I. Y., Heimann, M., John, J., Maki, T., Maksyutov, S., Peylin, P., Prather, M., and Taguchi, S.: Transcom 3 inversion intercomparison: Model mean results for the estimation of seasonal carbon sources and sinks, Global Biogeochem. Cy., 18, GB1010, https://doi.org/10.1029/2003GB002111, 2004.
Haynes, K. D., Baker, I. T., Denning, A. S., Stöckli, R., Schaefer, K., Lokupitiya, E. Y., and Haynes, J. M.: Representing Grasslands Using Dynamic Prognostic Phenology Based on Biological Growth Stages: 1. Implementation in the Simple Biosphere Model (SiB4), J. Adv. Model. Earth Sy., 11, 4423–4439, https://doi.org/10.1029/2018MS001540, 2019.
Heimann, M. and Körner, S.: The global atmospheric tracer model TM3 – Model Description and User's Manual Release 3.8a, Technical Report, Max-Planck Institute for Biogeochemistry, 2003.
Jacobson, A. R., Schuldt, K. N., Tans, P., Andrews, A., Miller, J., Oda, T., Mund, J., Weir, B., Ott, L., and Aalto, T.: CarbonTracker CT2022, NOAA Global Monitoring Laboratory, https://doi.org/10.25925/z1gj-3254, 2023.
Jiang, F., Wang, H., Chen, J. M., Ju, W., Tian, X., Feng, S., Li, G., Chen, Z., Zhang, S., Lu, X., Liu, J., Wang, H., Wang, J., He, W., and Wu, M.: Regional CO2 fluxes from 2010 to 2015 inferred from GOSAT XCO2 retrievals using a new version of the Global Carbon Assimilation System, Atmos. Chem. Phys., 21, 1963–1985, https://doi.org/10.5194/acp-21-1963-2021, 2021.
Jin, Z., Tian, X., Wang, Y., Zhang, H., Zhao, M., Wang, T., Ding, J., and Piao, S.: A global surface CO2 flux dataset (2015–2022) inferred from OCO-2 retrievals using the GONGGA inversion system, Earth Syst. Sci. Data, 16, 2857–2876, https://doi.org/10.5194/essd-16-2857-2024, 2024.
Jung, M., Koirala, S., Weber, U., Ichii, K., Gans, F., Camps-Valls, G., Papale, D., Schwalm, C., Tramontana, G., and Reichstein, M.: The FLUXCOM ensemble of global land-atmosphere energy fluxes, Scientific Data, 6, 1–14, 2019.
Kalnay, E., Kanamitsu, M., Kistler, R., Collins, W., Deaven, D., Gandin, L., Iredell, M., Saha, S., White, G., Woollen, J., Zhu, Y., Chelliah, M., Ebisuzaki, W., Higgins, W., Janowiak, J., Mo, K. C., Ropelewski, C., Wang, J., Leetmaa, A., Reynolds, R., Jenne, R., and Joseph, D.: The NCEP/NCAR 40-year reanalysis project, B. Am. Meteorol. Soc., 77, 437–471, https://doi.org/10.1175/1520-0477(1996)077<0437:Tnyrp>2.0.Co;2, 1996.
Kaminski, T., Heimann, M., and Giering, R.: A coarse grid three-dimensional global inverse model of the atmospheric transport: 2. Inversion of the transport of CO2in the 1980s, J. Geophys. Res.-Atmos., 104, 18555–18581, https://doi.org/10.1029/1999jd900146, 1999.
Kondo, M., Patra, P. K., Sitch, S., Friedlingstein, P., Poulter, B., Chevallier, F., Ciais, P., Canadell, J. G., Bastos, A., and Lauerwald, R.: State of the science in reconciling top-down and bottom-up approaches for terrestrial CO2 budget, Glob. Change Biol., 26, 1068–1084, 2020.
Kong, Y., Zheng, B., Zhang, Q., and He, K.: Global and regional carbon budget for 2015–2020 inferred from OCO-2 based on an ensemble Kalman filter coupled with GEOS-Chem, Atmos. Chem. Phys., 22, 10769–10788, https://doi.org/10.5194/acp-22-10769-2022, 2022.
Krinner, G., Viovy, N., de Noblet-Ducoudré, N., Ogée, J., Polcher, J., Friedlingstein, P., Ciais, P., Sitch, S., and Prentice, I. C.: A dynamic global vegetation model for studies of the coupled atmosphere-biosphere system, Global Biogeochem. Cy., 19, GB1015, https://doi.org/10.1029/2003GB002199, 2005.
Larson, V. E. and Volkmer, H.: An idealized model of the one-dimensional carbon dioxide rectifier effect, Tellus B, 60, 525–536, 2008.
Law, R. M., Peters, W., Rodenbeck, C., Aulagnier, C., Baker, I., Bergmann, D. J., Bousquet, P., Brandt, J., Bruhwiler, L., Cameron-Smith, P. J., Christensen, J. H., Delage, F., Denning, A. S., Fan, S., Geels, C., Houweling, S., Imasu, R., Karstens, U., Kawa, S. R., Kleist, J., Krol, M. C., Lin, S. J., Lokupitiya, R., Maki, T., Maksyutov, S., Niwa, Y., Onishi, R., Parazoo, N., Patra, P. K., Pieterse, G., Rivier, L., Satoh, M., Serrar, S., Taguchi, S., Takigawa, M., Vautard, R., Vermeulen, A. T., and Zhu, Z.: TransCom model simulations of hourly atmospheric CO2: Experimental overview and diurnal cycle results for 2002, Global Biogeochem. Cy., 22, Gb3009, https://doi.org/10.1029/2007gb003050, 2008.
Lin, J. C., Gerbig, C., Wofsy, S. C., Andrews, A. E., Daube, B. C., Davis, K. J., and Grainger, C. A.: A near-field tool for simulating the upstream influence of atmospheric observations: The Stochastic Time-Inverted Lagrangian Transport (STILT) model, J. Geophys. Res.-Atmos., 108, 4493, https://doi.org/10.1029/2002jd003161, 2003.
Liu, J., Baskaran, L., Bowman, K., Schimel, D., Bloom, A. A., Parazoo, N. C., Oda, T., Carroll, D., Menemenlis, D., Joiner, J., Commane, R., Daube, B., Gatti, L. V., McKain, K., Miller, J., Stephens, B. B., Sweeney, C., and Wofsy, S.: Carbon Monitoring System Flux Net Biosphere Exchange 2020 (CMS-Flux NBE 2020), Earth Syst. Sci. Data, 13, 299–330, https://doi.org/10.5194/essd-13-299-2021, 2021.
Liu, Z., Zeng, N., Liu, Y., Kalnay, E., Asrar, G., Wu, B., Cai, Q., Liu, D., and Han, P.: Improving the joint estimation of CO2 and surface carbon fluxes using a constrained ensemble Kalman filter in COLA (v1.0), Geosci. Model Dev., 15, 5511–5528, https://doi.org/10.5194/gmd-15-5511-2022, 2022.
Marcolla, B., Rödenbeck, C., and Cescatti, A.: Patterns and controls of inter-annual variability in the terrestrial carbon budget, Biogeosciences, 14, 3815–3829, https://doi.org/10.5194/bg-14-3815-2017, 2017.
Monteil, G. and Scholze, M.: Regional CO2 inversions with LUMIA, the Lund University Modular Inversion Algorithm, v1.0, Geosci. Model Dev., 14, 3383–3406, https://doi.org/10.5194/gmd-14-3383-2021, 2021.
Munassar, S., Rödenbeck, C., Koch, F.-T., Totsche, K. U., Gałkowski, M., Walther, S., and Gerbig, C.: Net ecosystem exchange (NEE) estimates 2006–2019 over Europe from a pre-operational ensemble-inversion system, Atmos. Chem. Phys., 22, 7875–7892, https://doi.org/10.5194/acp-22-7875-2022, 2022.
Munassar, S., Monteil, G., Scholze, M., Karstens, U., Rödenbeck, C., Koch, F.-T., Totsche, K. U., and Gerbig, C.: Why do inverse models disagree? A case study with two European CO2 inversions, Atmos. Chem. Phys., 23, 2813–2828, https://doi.org/10.5194/acp-23-2813-2023, 2023.
Nelson, J. A., Walther, S., Gans, F., Kraft, B., Weber, U., Novick, K., Buchmann, N., Migliavacca, M., Wohlfahrt, G., Šigut, L., Ibrom, A., Papale, D., Göckede, M., Duveiller, G., Knohl, A., Hörtnagl, L., Scott, R. L., Zhang, W., Hamdi, Z. M., Reichstein, M., Aranda-Barranco, S., Ardö, J., Op de Beeck, M., Billesbach, D., Bowling, D., Bracho, R., Brümmer, C., Camps-Valls, G., Chen, S., Cleverly, J. R., Desai, A., Dong, G., El-Madany, T. S., Euskirchen, E. S., Feigenwinter, I., Galvagno, M., Gerosa, G. A., Gielen, B., Goded, I., Goslee, S., Gough, C. M., Heinesch, B., Ichii, K., Jackowicz-Korczynski, M. A., Klosterhalfen, A., Knox, S., Kobayashi, H., Kohonen, K.-M., Korkiakoski, M., Mammarella, I., Gharun, M., Marzuoli, R., Matamala, R., Metzger, S., Montagnani, L., Nicolini, G., O'Halloran, T., Ourcival, J.-M., Peichl, M., Pendall, E., Ruiz Reverter, B., Roland, M., Sabbatini, S., Sachs, T., Schmidt, M., Schwalm, C. R., Shekhar, A., Silberstein, R., Silveira, M. L., Spano, D., Tagesson, T., Tramontana, G., Trotta, C., Turco, F., Vesala, T., Vincke, C., Vitale, D., Vivoni, E. R., Wang, Y., Woodgate, W., Yepez, E. A., Zhang, J., Zona, D., and Jung, M.: X-BASE: the first terrestrial carbon and water flux products from an extended data-driven scaling framework, FLUXCOM-X, Biogeosciences, 21, 5079–5115, https://doi.org/10.5194/bg-21-5079-2024, 2024.
Niwa, Y., Ishijima, K., Ito, A., and Iida, Y.: Toward a long-term atmospheric CO2 inversion for elucidating natural carbon fluxes: technical notes of NISMON-CO2 v2021.1, Progress in Earth and Planetary Science, 9, 42, https://doi.org/10.1186/s40645-022-00502-6, 2022.
Olsen, S. C. and Randerson, J. T.: Differences between surface and column atmospheric CO2 and implications for carbon cycle research, J. Geophys. Res.-Atmos., 109, D02301, https://doi.org/10.1029/2003JD003968, 2004.
Patra, P. K., Law, R. M., Peters, W., Rödenbeck, C., Takigawa, M., Aulagnier, C., Baker, I., Bergmann, D. J., Bousquet, P., Brandt, J., Bruhwiler, L., Cameron-Smith, P. J., Christensen, J. H., Delage, F., Denning, A. S., Fan, S., Geels, C., Houweling, S., Imasu, R., Karstens, U., Kawa, S. R., Kleist, J., Krol, M. C., Lin, S.-J., Lokupitiya, R., Maki, T., Maksyutov, S., Niwa, Y., Onishi, R., Parazoo, N., Pieterse, G., Rivier, L., Satoh, M., Serrar, S., Taguchi, S., Vautard, R., Vermeulen, A. T., and Zhu, Z.: TransCom model simulations of hourly atmospheric CO2: Analysis of synoptic-scale variations for the period 2002–2003, Global Biogeochem. Cy., 22, GB4013, https://doi.org/10.1029/2007GB003081, 2008.
Peylin, P., Baker, D., Sarmiento, J., Ciais, P., and Bousquet, P.: Influence of transport uncertainty on annual mean and seasonal inversions of atmospheric CO2 data, J. Geophys. Res.-Atmos., 107, 4385, https://doi.org/10.1029/2001jd000857, 2002.
Potter, C. S., Randerson, J. T., Field, C. B., Matson, P. A., Vitousek, P. M., Mooney, H. A., and Klooster, S. A.: Terrestrial ecosystem production: A process model based on global satellite and surface data, Global Biogeochem. Cy., 7, 811–841, https://doi.org/10.1029/93GB02725, 1993.
Rivier, L., Peylin, P., Ciais, P., Gloor, M., Rödenbeck, C., Geels, C., Karstens, U., Bousquet, P., Brandt, J., and Heimann, M.: European CO2 fluxes from atmospheric inversions using regional and global transport models, Climatic Change, 103, 93–115, https://doi.org/10.1007/s10584-010-9908-4, 2010.
Rödenbeck, C.: Estimating CO2 sources and sinks from atmospheric mixing ratio measurements using a global inversion of atmospheric transport, Technical Report, Max-Planck Institute for Biogeochemistry, 2005.
Rödenbeck, C., Houweling, S., Gloor, M., and Heimann, M.: CO2 flux history 1982–2001 inferred from atmospheric data using a global inversion of atmospheric transport, Atmos. Chem. Phys., 3, 1919–1964, https://doi.org/10.5194/acp-3-1919-2003, 2003.
Rödenbeck, C., Gerbig, C., Trusilova, K., and Heimann, M.: A two-step scheme for high-resolution regional atmospheric trace gas inversions based on independent models, Atmos. Chem. Phys., 9, 5331–5342, https://doi.org/10.5194/acp-9-5331-2009, 2009.
Russell, G. L. and Lerner, J. A.: A new finite-differencing scheme for the tracer transport equation, J. Appl. Meteorol., 20, 1483–1498, 1981.
Sarmiento, J. L., Gloor, M., Gruber, N., Beaulieu, C., Jacobson, A. R., Mikaloff Fletcher, S. E., Pacala, S., and Rodgers, K.: Trends and regional distributions of land and ocean carbon sinks, Biogeosciences, 7, 2351–2367, https://doi.org/10.5194/bg-7-2351-2010, 2010.
Stephens, B. B., Gurney, K. R., Tans, P. P., Sweeney, C., Peters, W., Bruhwiler, L., Ciais, P., Ramonet, M., Bousquet, P., Nakazawa, T., Aoki, S., Machida, T., Inoue, G., Vinnichenko, N., Lloyd, J., Jordan, A., Heimann, M., Shibistova, O., Langenfelds, R. L., Steele, L. P., Francey, R. J., and Denning, A. S.: Weak Northern and Strong Tropical Land Carbon Uptake from Vertical Profiles of Atmospheric CO2, Science, 316, 1732–1735, https://doi.org/10.1126/science.1137004, 2007.
Tans, P. P., Fung, I. Y., and Takahashi, T.: Observational Contrains on the Global Atmospheric CO2 Budget, Science, 247, 1431–1438, https://doi.org/10.1126/science.247.4949.1431, 1990.
van der Laan-Luijkx, I. T., van der Velde, I. R., van der Veen, E., Tsuruta, A., Stanislawska, K., Babenhauserheide, A., Zhang, H. F., Liu, Y., He, W., Chen, H., Masarie, K. A., Krol, M. C., and Peters, W.: The CarbonTracker Data Assimilation Shell (CTDAS) v1.0: implementation and global carbon balance 2001–2015, Geosci. Model Dev., 10, 2785–2800, https://doi.org/10.5194/gmd-10-2785-2017, 2017.
Yang, D., Liu, Y., Feng, L., Wang, J., Yao, L., Cai, Z., Zhu, S., Lu, N., and Lyu, D.: The First Global Carbon Dioxide Flux Map Derived from TanSat Measurements, Adv. Atmos. Sci., 38, 1433–1443, https://doi.org/10.1007/s00376-021-1179-7, 2021.
Yi, C., Davis, K. J., Bakwin, P. S., Berger, B. W., and Marr, L. C.: Influence of advection on measurements of the net ecosystem-atmosphere exchange of CO2 from a very tall tower, J. Geophys. Res., 105, 9991–9999, 2000.