the Creative Commons Attribution 4.0 License.
the Creative Commons Attribution 4.0 License.
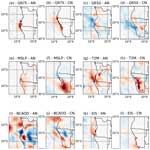
A satellite-based analysis of semi-direct effects of biomass burning aerosols on fog and low-cloud dissipation in the Namib Desert
Hendrik Andersen
Jan Cermak
Paola Formenti
Eva Pauli
Julian Quinting
In the Namib Desert, fog is the only regular water input and, thus, is a crucial water source for its fauna and flora. Each year, between June and October, absorbing biomass burning aerosols (BBAs) overlie the stratocumulus clouds in the adjacent Southeast Atlantic. In some synoptic settings, this layer of BBAs reaches Namibia and its desert, where it interacts with coastal fog and low clouds (FLCs). In this study, a novel 15-year data set of geostationary satellite observations of FLC dissipation time in the Namib Desert is used, along with reanalysis data, to better understand the potential semi-direct effects of BBAs on FLC dissipation in the Namib Desert, i.e., through adjustments of atmospheric stability and thermodynamics via the interaction of aerosols with radiation. This is done by investigating both the time of day when FLCs dissolve and the synoptic-scale meteorology depending on BBA loading. It is found that FLC dissipation time is significantly later on high-BBA-loading days. BBAs are transported to the Namib along moist free-tropospheric air by a large-scale anticyclonic recirculation pattern. At the surface, the associated longwave heating strengthens a continental heat low, which modifies the circulation and boundary layer moisture along the coastline, complicating the attribution of BBA effects. During high-BBA days, the vertical profiles of the temporal development of air temperatures highlight contrasting daytime and nighttime processes modifying the local inversion. These processes are thought to be driven by greenhouse warming as a result of the moisture in the BBA plumes and BBA absorption (only during the daytime). A statistical learning framework is used to quantify meteorological and BBA influences on FLC dissipation time. The statistical model is able to reproduce the observed differences in FLC dissipation time between high- and low-BBA days and attributes these differences mainly to differences in circulation, boundary layer moisture and near-surface air temperature along the coastline. However, the model is prone to underfitting and is not able to reproduce the majority of the FLC dissipation variability. While the model does not suggest that BBA patterns are important for FLC dissipation, the findings show how the moist BBA plumes modify local thermodynamics, to which FLC dissipation is shown to be sensitive. The findings highlight the challenges of disentangling meteorological and aerosol effects on cloud development using observations and invite detailed modeling analyses of the underlying processes, for example, with large-eddy simulations.
- Article
(8688 KB) - Full-text XML
- BibTeX
- EndNote
Fog is the most relevant water source in the hyperarid Namib Desert and is the key to the survival of many species (Louw and Holm, 1972; Seely and Henschel, 1998; Ebner et al., 2011; Warren-Rhodes et al., 2013; Gottlieb et al., 2019; Wang et al., 2019). Regional climate simulations of southern Africa suggest a warmer and drier climate in the future (James and Washington, 2013; Maúre et al., 2018), such that fog might become even more relevant as a water source for local ecosystems. However, weather and climate models struggle to adequately represent fog in general (Vautard et al., 2009; Price et al., 2018), and a lack of understanding of the processes involved in the formation, maintenance and dissipation of fog in the Namib limits robust projections of possible future developments of this system.
The diurnal coastal circulation in the region features sea breezes during the day as cooler ocean air moves inland and land breezes at night as cooler air flows from land to sea (Lindesay and Tyson, 1990), facilitating the advection of fog, which is thought to predominantly form in the stable marine boundary layer over the cool Benguela Current, following which it is advected inland (Olivier and Stockton, 1989; Seely and Henschel, 1998; Cermak, 2012; Andersen et al., 2019, 2020; Spirig et al., 2019). Fog most frequently occurs along the coastline but can extend more than 100 km inland (Olivier, 1995; Cermak, 2012; Andersen et al., 2019). The seasonal cycle of fog occurrence varies with latitude, as well as with distance to the coast. The maximum fog occurrence in low-lying coastal locations is during austral winter, whereas, during austral summer, primarily lifted stratus clouds are advected and only manifest as fog further inland, where they are intercepted by the land (Lancaster, 1984; Seely and Henschel, 1998; Andersen et al., 2019). The day-to-day variability of fog and low clouds (FLCs) in the central Namib Desert has been found to be associated with distinct synoptic-scale patterns that determine the large-scale transport of free-tropospheric moisture, controlling fog occurrence in two main ways. (1) Dry free-tropospheric air is advected from the southwest over the marine coastal regions where FLCs typically form, facilitating a strong cloud top radiative cooling that helps sustain the low-cloud layer. (2) At the same time, tropical moist air is advected over continental southern Africa, causing surface heating and the development of a heat low which drives the inland advection of the cloudy marine air masses (Andersen et al., 2020). While these findings help understand links between synoptic-scale variability and fog occurrence, the understanding of Namibian fog processes is still limited. In particular, possible influences of atmospheric aerosol particles on the life cycle of fog and its properties have not been studied to date.
A seasonally recurrent feature of central Africa is the large-scale burning of biomass that emits large amounts of biomass burning aerosols (BBAs) into the atmosphere between June and October (Zuidema et al., 2016). Lifted into the free troposphere over the continent, these BBAs are frequently transported over the South Atlantic Ocean by mid-tropospheric easterly winds between 5 and 15° S (Adebiyi et al., 2015). As wet scavenging is negligible in this region, the BBA plumes frequently persist for a relatively long time above the marine boundary layer clouds (Wilcox, 2010; Adebiyi and Zuidema, 2016). Recent international projects have focused on the BBA effects on low clouds over the Southeast Atlantic Ocean, particularly ORACLES (ObseRvations of Aerosols above CLouds and their intEractionS), SEALS-sA (Sea Earth Atmosphere Linkages Study in southern Africa), AEROCLO-sA (AErosol, RadiatiOn and CLOuds in southern Africa), LASIC (Layered Atlantic Smoke Interactions with Clouds) and CLARIFY (CLoud–Aerosol–Radiation Interaction and Forcing: Year 2017) (Zuidema et al., 2016; Formenti et al., 2019; Haywood et al., 2021; Redemann et al., 2021). One of the overall findings of these projects and related research is the important role of BBAs in marine low-cloud development via semi-direct and indirect effects (e.g., Fuchs et al., 2017; Adebiyi and Zuidema, 2018; Diamond et al., 2018; Gordon et al., 2018; Deaconu et al., 2019; Herbert et al., 2020; Zhang and Zuidema, 2019).
The absorbing BBA layer leads to local heating, mostly in the free troposphere, but also reduces the downward solar radiation received at the surface, leading to a cooling there. As a consequence, BBAs exert a strong positive direct radiative effect and change the local temperature profile (Deaconu et al., 2019; De Graaf et al., 2020). Recent studies have shown that BBA layers tend to increase the stability of the lower troposphere, leading to stronger, lower-lying inversions with less entrainment of free-tropospheric air into the boundary layer and, thus, an increased cloudiness and cloud thickness (Wilcox, 2010; Sakaeda et al., 2011; Gordon et al., 2018; Deaconu et al., 2019). However, BBAs are frequently transported within an anomalously moist atmospheric layer (Adebiyi et al., 2015) that modifies the longwave cooling rate of surfaces below (Adebiyi et al., 2015; Adebiyi and Zuidema, 2018; Deaconu et al., 2019), thereby complicating the attribution of BBA effects. BBA-induced atmospheric heating can also lead to a reduction in cloudiness if the heating occurs within the cloud layer due to increased evaporation (Hansen et al., 1997). Most of the research in this field is focused on the marine environment of the Southeast Atlantic, where the majority of the BBA plumes are transported. However, under different weather systems, a substantial number of BBA plumes circulate to the free troposphere of the southwestern African coastline (Flamant et al., 2022), leading to situations of high aerosol optical depth (AOD) in the central Namib Desert (Adebiyi and Zuidema, 2016; Adesina et al., 2019). Potential atmospheric effects and, in particular, semi-direct effects of BBAs on Namib-region FLCs are highly likely in such situations. Semi-direct effects in this study refer to the large-scale semi-direct effects, as defined in Diamond et al. (2022), involving atmospheric thermodynamic, stability and circulation adjustments resulting from the absorption or scattering of solar radiation by aerosols. As Namib-region FLCs primarily occur during the nighttime and typically dissipate shortly after sunrise (Andersen and Cermak, 2018; Andersen et al., 2019; Spirig et al., 2019) and because semi-direct effects of the absorbing BBAs are expected to be most pronounced during the daytime, the strongest effects of BBAs may be expected during the dissipation of FLCs.
The goals of this study are, thus, to better understand possible BBA semi-direct effects on the dissipation of FLCs in the Namib Desert and to attempt to disentangle the BBA effects from other meteorological covariates. To this end, a 15-year time series of geostationary satellite observations of FLCs in the Namib Desert is analyzed together with reanalysis data to characterize situations under contrasting BBA loading and is used in a statistical learning framework to quantify and partially disentangle meteorological and BBA influences on FLC dissipation time. The guiding hypothesis is that, during the biomass burning season, BBA plumes lead to a stronger, lower-lying inversion and slower early-morning planetary boundary layer development, resulting in a longer FLC lifetime.
This study uses multiple data sets from different space-borne sensors, reanalysis products and statistical analysis methods to characterize the dissipation of FLCs and its potential links to BBAs in the Namib region. As the study focuses on the interactions between FLCs and BBAs, all analyses are conducted during the BBA season from June to October (De Graaf et al., 2014) over a 15-year period (2004–2015). The spatial domain of this study is the western coastline of southern Africa (5–30° S, 0–25° E). Two regions with a high frequency of FLC occurrence were delineated by Andersen and Cermak (2018) and are the particular focus of this study: the Central Namib (CN; 22–24° S) and the Angolan Namib (AN; 15–17° S) (Fig. 1). Both regions have a similar topography with a relative narrow coastal plain and a central plateau further inland. The two regions are mostly flat and arid, except in the northernmost part of the AN, which features low cliffs. The biggest difference lies in the distance to the BBA emission sources, with the AN being closer. Within each region, FLCs are advected inland, and, therefore, their life cycle characteristics (i.e., time of advection and dissipation) are dependent on the distance to the coastline (Andersen and Cermak, 2018; Andersen et al., 2019). To control for the influence of the coastal proximity over FLC life cycle characteristics, FLCs are considered only within the first 25 km from the coastline in the coastal plain.
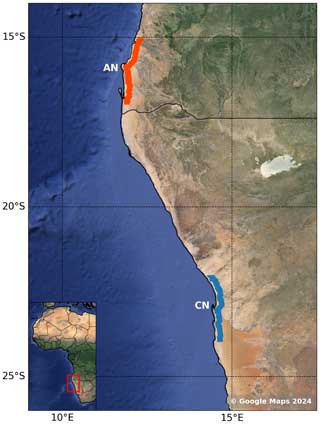
Figure 1Overview of the study area, with the Angolan Namib region in orange and the Central Namib region in blue. The two regions are defined by excluding the two first pixels after the coastline and within 25 km of it.
2.1 Satellite observations of FLC dissipation time
The data set of FLC dissipation time is created in two steps. First, the FLC detection algorithm developed by Andersen and Cermak (2018) is used on data from the Spinning Enhanced Visible and Infrared Imager (SEVIRI) sensor, mounted on the geostationary Meteosat Second Generation (MSG) satellites. SEVIRI provides spatiotemporally coherent observations of clouds. It features a spatial resolution of 3 km at nadir and a repeat rate of 15 min (Schmetz et al., 2002). The FLC detection algorithm is able to identify FLCs consistently at all times of the day by using infrared observations and a combination of threshold tests and image analysis techniques. Extensive validation against surface observations has shown a good performance (probability of detection of 94 %, a false-alarm rate of 12 % and an overall correctness of classification of 97 %). It should be noted that this satellite technique does not discriminate between fog and lifted-stratus situations.
In the second step, a statistical method is applied to objectively detect the dissipation time of FLCs from the initial binary FLC data set produced in step 1. This method, as described by Pauli et al. (2022), employs logistic regression to predict the probability of a data point belonging to one of two binary classes: FLC or no FLC. By defining the transition between classes when this probability exceeds 50 %, it becomes possible to determine the time of the transition from one class to the other and, as a result, to determine the formation and dissipation times of each individual FLC event. A minor adjustment to the initial algorithm was implemented: the number of consecutive 15 min time steps with the presence (or absence) of FLCs required to define an event is 4 instead of 10 because, in Namibia, the FLC events are more stable, and the satellite view is less frequently interrupted by high-cloud advection than in Europe, where the method was originally developed.
The resulting data set provides the daily UTC time of FLC dissipation from 2004 to 2018, with a 15 min temporal step (allowing for 96 possible dissipation times from 00:00 to 23:45) and a spatial resolution of 3×3 km. In both regions, dissipation begins shortly after sunrise, reaching a maximum at 08:00 UTC, which can be attributed to stronger solar irradiance (Andersen and Cermak, 2018). This is followed by a decrease until a daily minimum is observed around 12:00 UTC. It should be noted that the two pixels closest to the coastline are excluded in this analysis as they tend to be noisy; FLCs can hover along the coastline, intermittently covering coastal locations, leading to uncertain estimations of dissipation time. Additionally, any absence of FLC events, such as during clear-sky days, is discarded and treated as missing data.
2.2 CAMS global reanalysis
To quantify the atmospheric BBA load, the Copernicus Atmosphere Monitoring Service (CAMS) reanalysis data from the ECMWF are used. This product features a spatial resolution of 0.75°×0.75° and a temporal resolution of 3 h (Inness et al., 2019). While more observational-based products exist, such as aircraft measurements from field campaigns in the region, which are very useful in case studies, a reanalysis data set is preferred in this study to avoid issues related to missing data and cloud interference and to facilitate combination with meteorological reanalysis data in a statistical framework. Additionally, the AOD data were extensively validated using observations from Gueymard and Yang (2020), where the authors found a small but existing tendency in CAMS to underestimate AOD across Africa. However, they conclude that, for many applications, this data set offers significant advantages over customary observationally based products.
The data for black carbon aerosol optical depth (BCAOD) at 550 nm are used as a proxy for the BBA loading as several aircraft campaigns have shown that southern African BBA fractional black carbon content is high in terms of both the number and mass of total particles (Taylor et al., 2020; Denjean et al., 2020). Half-day averages from 00:00 to 12:00 UTC are used to capture BBAs that may influence the morning dissipation of FLCs, which is the most frequent dissipation time (Andersen and Cermak, 2018). To study possible BBA effects on FLC dissipation, two groups of days are created for each region: days with high BBA loading (BCAOD ≥ 75th percentile) and days with low BBA loading (BCAOD ≤ 25th percentile). They are referred to as “high-BBA days” and “low-BBA days”, each containing around 300 d after discarding those where at least one pixel of the region is outside the defined thresholds.
In the CN, the first quartile is characterized by a high density, followed by an almost exponential decrease into and throughout the third quartile. In contrast, the AN exhibits a more linear increase in density within the first quartile, followed by a gradual decline as BCAOD increases (see Appendix A). These differences are attributed to the distance from emission sources.
2.3 ERA5 reanalysis
Large-scale meteorological conditions are represented in the ERA5 reanalysis data from the European Centre for Medium-Range Weather Forecasts (ECMWF). ERA5 features a 0.25°×0.25° spatial resolution and an hourly temporal resolution (Hersbach et al., 2020). To characterize large-scale dynamic and thermodynamic conditions, fields of mean sea level pressure (MSLP), geopotential height at 650 hPa (Z650), 2 m air temperature (T2M), sea surface temperature (SST), specific humidity at 650 hPa (Q650) and 975 hPa (Q975), and u and v components of wind at 650 hPa and 10 m above the surface are analyzed. Additionally, atmospheric temperature at all ERA5 pressure levels between 1000 and 500 hPa is used. Estimated inversion strength (EIS) is computed based on the method developed by Wood and Bretherton (2006). If not explicitly indicated, 08:00 UTC fields are selected to represent conditions during the typical time of FLC dissipation (Andersen and Cermak, 2018). Additionally, the high- and low-BBA days defined with CAMS are used to average ERA5 fields to obtain the meteorological situations on these days. However, aerosol effects are not explicitly represented in ERA5. The model is forced by climatological distributions of optical depth from sea salt, soil and/or dust, organic and/or black carbon, and sulfate (Hersbach et al., 2015). Therefore, it is important to keep in mind that this prescribed forcing constrains the model's radiative environment by limiting the effects of aerosol variability. An additional limitation of the data is related to the assimilation scheme of ERA5, which uses 12 h windows from 09:00 to 21:00 UTC (Hersbach et al., 2020). In regions with sparse observations, such as the Namib Desert, the reanalysis relies heavily on satellite data and the underlying numerical weather model, which can result in discontinuities during these times.
2.4 CALIPSO
To characterize the cloud and aerosol layers, as well as their relative positions, data from the active-sensor platform of the Cloud-Aerosol Lidar and Infrared Pathfinder Satellite Observation (CALIPSO) is used and is presented in Fig. 2. CALIPSO is equipped with the Cloud-Aerosol Lidar with Orthogonal Polarization (CALIOP), which features a vertical resolution of 30 m and a horizontal resolution of 333 m (Vaughan et al., 2004). Here, the level-2 CALIPSO 5 km merged cloud and aerosols layer product (version 4.20) is used for the period 13 June 2006–31 December 2018 based on both nighttime and daytime overpasses. In contrast to all other data in this study, 13 June 2006 is the earliest available date because CALIPSO was launched in April 2006. The FLC layers were derived using a similar approach to that presented in Cermak (2018). First, the cloud layer altitude was calculated by subtracting the terrain altitude from the observed feature altitude. Then all cloud layers with a cloud top height equal to or smaller than 2 km were defined as FLC. To characterize the vertical distribution of BBAs, smoke layers were derived using the Feature Classification Flags product (Vaughan et al., 2023). It should be noted that, for aerosol layers located below 1 km above the surface, the instrument cannot differentiate between smoke and urban pollution, but, in this region, urban pollution is not expected to be relevant.
2.5 Ridge regression
In this study, statistical modeling of FLC dissipation times is done by using meteorological fields from ERA5 and atmospheric BBA loading from CAMS as predictors. Typically, local meteorological fields are used as predictors for the statistical modeling of FLCs and Namibian stratocumulus clouds (e.g., Adebiyi and Zuidema, 2018; Fuchs et al., 2018; Zipfel et al., 2022). However, the Namibian FLC system is largely controlled by regional wind systems and their modulation through synoptic-scale variability (Andersen et al., 2020), and approximating such large-scale dynamics using local pressure or wind fields is difficult. Spatial neural networks would be an ideal tool for this; however, they require a large number of data points, and their interpretation and sensitivity estimation are more challenging. Therefore, ridge regression, a regularized linear model (James et al., 2021), is used to predict FLC occurrence based on large-scale spatial meteorological fields, as in Andersen et al. (2020). The regularization helps deal with the high number of correlated predictors which could lead to a high-variance (overfitting) problem if a classical statistical model were used. In ridge regression, the regularization is controlled (λ), which shrinks the coefficients of the model towards zero using the L2 penalty: the squared magnitudes of the coefficient value are added as a penalty term to the loss function (Friedman et al., 2010). Usually, the optimal value of the tuning parameter λ is defined using cross-validation, which derives a value for λ which is high enough to reduce overfitting issues while simultaneously not impairing the predictive skill of the model.
Here, to reduce model variance and increase the robustness of model predictions, an ensemble of 50 ridge regression models is used. Each regression model is trained on 80 % of the data, which are randomly selected, withholding 20 % for testing. To ensure comparability between the individual models, λ is set to a fixed value of 10 000, a stronger regularization than determining λ through cross-validation (optimal values range from 3000 to 7000). However, analyses of the training–test results showed only a marginal loss of predictive skill while substantially reducing overfitting when setting λ to 10 000.
The ridge regression method is applied to predict FLC dissipation times for the days with high and low BBA derived from CAMS. The predictors are the spatial fields of MSLP, Z650, T2M, SST, Q650, Q975 and EIS (as defined in Sect. 2.3) from ERA5 and of BCAOD from CAMS. For the different predictors to be comparable, standardization of the features was done by removing the mean and scaling to unit variance. To harmonize the spatial resolution of all predictors, the ERA5 fields (0.25°×0.25°) are upscaled to match the coarser resolution of CAMS (0.75°×0.75°). However, the rescaling of the predictand is unnecessary because a spatial median of dissipation times is calculated for each region at each time step. Because FLC events are highly dependent on the synoptic-scale meteorological processes in the region (Andersen et al., 2020), the spatial domain of the predictors is set to be centered on the zone of interest (the CN or AN) but is large enough to capture the synoptic processes. For the AN, the latitudinal extent is set between 5 and 25° S, while, for the CN, it is between 10 and 30° S. The longitudinal extent is the same for both regions: 5 and 22.5° E. Spatial patterns of 08:00 UTC (representative of maximum FLC dissipation; see Sect. 2.3) for ERA5 fields and half-day time averages for CAMS fields are used. Model estimation was performed using the scikit-learn package in Python (Pedregosa et al., 2011).
3.1 Characterization of FLCs and BBAs during the biomass burning season
The intensity of semi-direct effects is sensitive to the vertical layering of aerosols and clouds (Herbert et al., 2020). Figure 2 shows the CALIPSO vertical profiles of smoke and cloud layers over the Namib region. The vertical profiles were realized in two configurations. The first one is a latitudinal cross-section between 5 and 30° S within a 1° band of the coastline (Fig. 2a). The second configuration is a longitudinal cross-section between 5 and 20° E, where all latitudes between the AN and the CN (15–24° S) are taken (Fig. 2b). The cross-sections show the well-known features of BBAs and low clouds of the region (Adebiyi et al., 2015; Redemann et al., 2021). The CALIPSO observations show that, typically, the smoke layers are above the low-cloud layers. Most frequently, smoke layers are detected over the tropical African continent where BBAs are emitted and then transported over the South Atlantic Ocean between 550 and 750 hPa. In both regions, the aerosol plumes are located higher during high-BBA days, around 550 hPa, whereas, on low-BBA days, they are situated around 750 hPa. This pattern is likely due, for the most part, to the large-scale atmospheric processes responsible for the transport of the aerosol plumes into these regions. Both cross-sections suggest that cloud and smoke layers can be intermingling even though CALIPSO tends to overestimate the distance between the layers (Rajapakshe et al., 2017). This is particularly the case in the AN, where the BBA loading is higher and where the low clouds tend to be higher up. In this situation, potential indirect aerosol effects on FLC microphysics (Costantino and Bréon, 2013; Che et al., 2022; Gupta et al., 2022) may be more relevant in the AN, even though indirect effects have also been shown further south when BBAs are mixed into the marine boundary layer (Diamond et al., 2018). At the same time, mixing of BBAs into the low-cloud layer can also lead to the dissipation of clouds when the absorption of solar radiation leads to a local heating (Hansen et al., 1997).
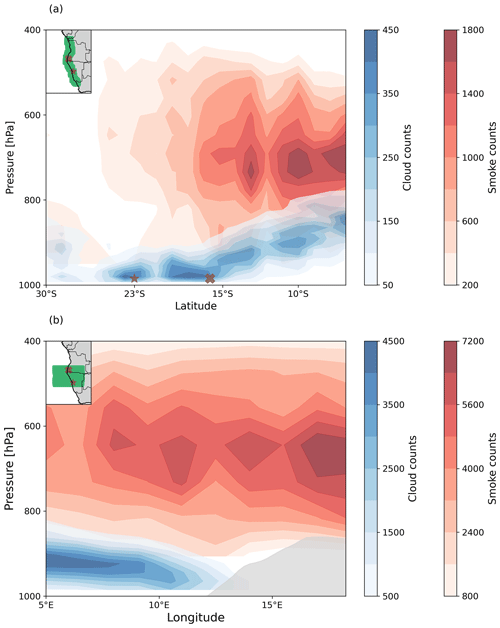
Figure 2BBA season mean climatology (2004–2018) for (a) latitudinal cross-section of CALIOP smoke and cloud counts with longitude averages within 1° after the coastline. The brown cross (respectively, star) represents the center of the AN (respectively, the CN). (b) Longitudinal cross-section of CALIOP smoke and cloud counts with latitude averages between 15–24° S. The gray area is the mean orography within the latitude range. Only clouds below 2 km are considered. The green areas represent the ground tracks selected in each cross-section.
As outlined in the Introduction, the strongest potential semi-direct BBA effects may be expected during the dissipation of FLCs. Figure 3 shows the observed dissipation times in the AN (Fig. 3a) and the CN (Fig. 3b) during the biomass burning season over the 2004–2018 period. To have one dissipation time per day and region, the median dissipation time is computed over all pixels for each region. Then, the data are separated into two groups of high and low BBA loading (see Sect. 2.2), with around 200 d in each group after discarding days without FLC events, which had no data on FLC dissipation time. In agreement with Andersen and Cermak (2018), FLC dissipation is observed to mainly occur between 06:00 and 09:00 UTC. However, there is a significant difference (p<0.01) between the FLC dissipation time on high- and low-BBA days, with dissipation occurring later on high-BBA days, in line with the guiding hypothesis (the median dissipation time is 30 min later in the AN and 75 min later in the CN). While the variability of dissipation times, measured by the interquartile range, does not change with BBA in the AN, it is 27 % smaller during high-BBA days in the CN, where dissipation before 07:00 UTC becomes rare. The lower number of days in the low-BBA group of the CN certainly impacts the increased variability and earlier dissipation time compared to the other groups. Additionally, the dissipation of FLCs generally occurs later in the AN than in the CN, regardless of the BBA loading.
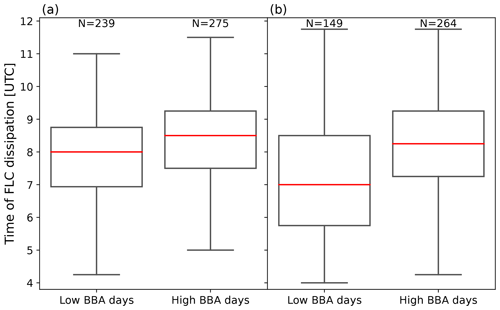
Figure 3Observed time of dissipation of FLC events for the high- and low-BBA days in the AN (a) and the CN (b) during the biomass burning season in the 2004–2018 period. The red lines represent the median dissipation times, and the whiskers extend to the highest (lowest) data points still within 1.5× the interquartile range from the third (first) quartile. FLC dissipation is significantly later (p<0.01) on high-BBA days in both regions. N indicates the number of days per group.
One should note that it is possible that separating high-BBA days from low-BBA days in the AN and CN may lead to sampling different meteorological situations as well because BBA occurrence over the Namibian coastline is associated with a specific recirculating pattern (Adebiyi and Zuidema, 2016). Such meteorological sampling biases would complicate the attribution of BBA effects and are thus analyzed in the following.
3.2 Possible meteorological sampling biases
Figure 4 shows large-scale patterns of the mean differences in Z650 and winds at 650 hPa (a, b), the typical altitude of the BBA plumes; Q650 (c, d); and BCAOD (e, f) between high- and low-BBA days in the AN (a, c, e) and in the CN (b, d, f). Additionally, Fig. 5 shows these differences for MSLP at 975 hPa and for 10 m winds (a, b), Q975 (c, d), T2M (e, f) and EIS (g, h) in the AN (a, c, e) and the CN (b, d, f). Both figures are based on 15 years of BBA season ERA5 and CAMS (BCAOD) data. It is important to understand that the differences between the composites are temporal and not geographical: the selection of high and low BBA days is region-specific. Z650 and winds at 650 hPa (Fig. 4a and b) show systematic differences between high- and low-BBA days. The continental anticyclone is stronger on high-BBA days in the AN (a), leading to a clear northwesterly circulation anomaly. In the CN (b), this circulation pattern is even more pronounced, where the continental anticyclone is stronger, whereas the South Atlantic anticyclone is weaker. This circulation pattern is responsible of the large-scale advection of moist air masses leading to marked positive Q650 anomalies on high-BBA days in both regions (Fig. 4c and d). As the circulation is stronger during the CN (d) high-BBA days, the Q650 anomalies extend further southward than during high-BBA days in the AN (c). The BBAs are also transported to the AN and CN by the same circulation, leading to similar anomaly patterns. This is coherent with the literature as BBA plumes are known to be transported in moist air masses (Adebiyi et al., 2015). The local BBA anomalies (Fig. 4e and f) on high-BBA days are stronger in the AN (e), possibly because the AN is closer to the emission sources (Swap et al., 1996), but extend further southward on high-BBA days in the CN (f). The geographic locations of the AN and CN may also explain the difference in the magnitude of the circulation anomaly: the proximity of the AN to the emission sources means that a smaller shift in the circulation is necessary for the BBA plumes to be transported there.
Differences in MSLP (Fig. 5a and b) show negative pressure anomalies of about 1–2 hPa in the AN (a) and of 4–6 hPa in the CN (b) over land during high-BBA days. The near-surface wind speed anomalies show that these pressure anomalies lead to a change in the regional coastal circulation in both regions and, overall, to an onshore flow anomaly. MSLP differences are of opposite sign compared to the Z650 differences, indicating that an anticyclonic anomaly at 650 hPa is associated with a strengthened heat low at the surface. The positive Q975 anomalies on high-BBA days along the coastline in both regions are also a signal of an onshore flow of moist air masses or, rather, a lack of dry offshore flow (Fig. 5c and d). Figure 4 showed an advection of moist air masses in the free troposphere. These moist air masses can cause a longwave heating at the surface, which can explain the observed negative pressure anomalies (Alamirew et al., 2018). Indeed, positive T2M (Fig. 5e and f) anomalies of about 4–5 K in the AN (e) and of 7–8 K in the CN (f) are observed, and their spatial patterns are clearly related to the free-tropospheric moisture anomalies. As such, the observed negative pressure anomalies are a clear sign of a heat low anomaly, which is defined as an area of low atmospheric pressure caused by intense surface heating. This phenomenon seems to be mainly driven by the greenhouse warming of the moist free-tropospheric air masses. Northerly advection of warm air masses is also observed and likely contributes to the development of the heat low anomaly as well. It should be noted that these processes have been associated with fog occurrence in the Namib region before (Andersen et al., 2020). Differences in EIS (Fig. 5g and h) show strong positive anomalies up to 6 K near the coastline in both regions. The stronger inversion during high-BBA days could be related to BBA absorption and is in line with the guiding hypothesis.
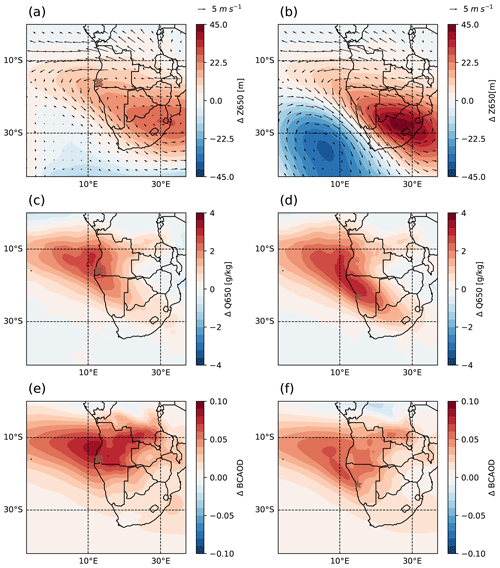
Figure 4BBA season mean climatological (2004–2018) differences (high-BBA days – low-BBA days) for (a) ERA5 geopotential height and winds at 650 hPa in the AN, (c) ERA5 specific humidity at 650 hPa in the AN, (e) CAMS black carbon AOD at 550 nm in the AN. Panels (b), (d), and (f) are the same as (a), (c), and (e), respectively, but in the CN. The u and v components of winds are bilinearly interpolated to a 2.5°×2.5° grid for clarity. ERA5 data are sampled at 08:00 UTC, while half-day averages from 00:00 to 12:00 UTC are used for CAMS. The brown cross (respectively, star) represents the center of the AN (respectively, the CN).
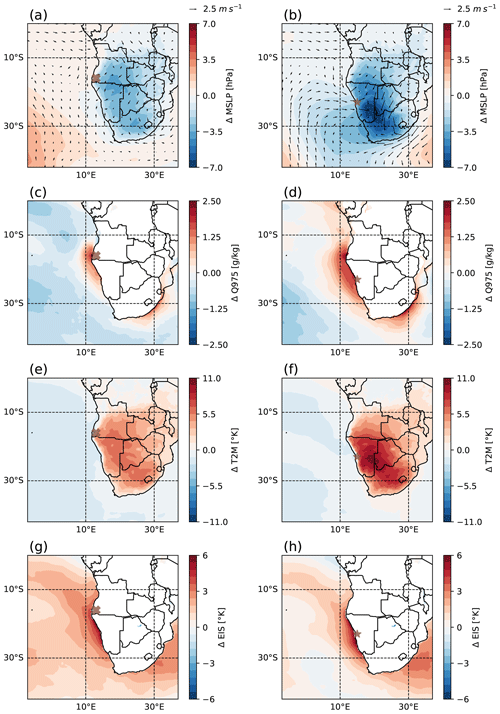
Figure 5BBA season mean climatological (2004–2018) differences (high-BBA days − low-BBA days) for (a) ERA5 mean sea level pressure at 975 hPa and 10 m winds in the AN, (c) ERA5 specific humidity at 975 hPa in the AN with subsurface regions masked, (e) ERA5 2 m air temperature in the AN, (g) ERA5 estimated inversion strength in the AN. Panels (b), (d), (f), and (h) are the same as (a), (c), (e), and (g), respectively, but in the CN. The u and v components of winds are bilinearly interpolated to a 2.5°×2.5° grid for clarity. Data are sampled at 08:00 UTC. The brown cross (respectively, star) represents the center of the AN (respectively, the CN).
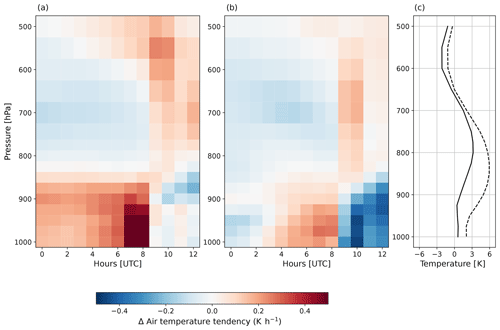
Figure 6Mean differences (high-BBA days − low-BBA days) of the hourly tendency of air temperature (K h−1) at each time step for 16 pressure levels in the AN (a) and in the CN (b) during the BBA season (2004–2018). (c) Vertical profiles of the mean differences (high-BBA days − low-BBA days) of air temperature (K) at 08:00 UTC for 16 pressure levels in the AN (solid line) and the CN (dashed line).
So far, the results indicate that at least part of the observed later FLC dissipation on high-BBA days may be caused by the transport of moist free-tropospheric air masses strengthening a continental heat low, which modifies the coastal circulation and boundary layer moisture along the coastline. In the following, possible effects of BBAs on the vertical profiles of air temperature and heating rates are analyzed. Figure 6 shows differences in the temporal air temperature tendency between high- and low-BBA days, averaged for the BBA season over the 2004–2018 period for all pressure levels from the surface to 500 hPa for the AN (Fig. 6a) and the CN (Fig. 6b). This can be interpreted as the differences of the first derivative of the temperature. A positive air temperature–development difference means that the air at a specific time and altitude is cooling less (during the nighttime) or warming more (during the daytime) on high-BBA days. A negative difference means that the air temperature in the grid cell is cooling less and/or warming more on low-BBA days. In both regions, high-BBA days between 04:00 and 08:00 UTC feature a weaker cooling in the boundary layer but a stronger cooling in the free troposphere. Conversely, shortly after sunrise, around 08:00 UTC, the free troposphere features a stronger warming on high-BBA days, whereas the boundary layer warming is less pronounced, particularly in the CN. A sign change of aerosol effects between night and daytime has been described before in Herbert et al. (2020). These observed differences in the vertical profiles of cooling and/or heating rates may be explained by the warm, moist absorbing aerosol-laden air masses in the free troposphere. During the nighttime, the warmer free-tropospheric air loses more heat and heats the surface via stronger greenhouse warming and/or reduces cloud top radiative cooling, as described in Adebiyi and Zuidema (2018). After sunrise, the absorbing BBAs lead to a stronger heating in the free troposphere but may also slow down the surface heating by blocking incoming solar radiation. These differences in the temporal development of the vertical temperature structure are in line with our hypothesis and may contribute to the observed differences in FLC dissipation. One should note, though, that the FLC layer also feeds back to the surface temperatures and the boundary layer development via its radiative effects. In particular, the observed later FLC dissipation on high-BBA days may contribute to the initially weaker boundary layer heating rates after sunrise. However, the later FLC dissipation cannot explain the weaker daytime warming of the boundary layer on high-BBA days, especially pronounced in the CN. But air temperatures are not only driven by radiation – there are advective contributions as well. The latter are likely to differ, particularly as coastal circulation is also changed, and cause this weaker warming.
The vertical profiles of the mean differences in air temperature between high- and low-BBA days at 08:00 UTC in the AN and CN (Fig. 6c) show that, above 650 hPa, the temperatures are slightly warmer on low-BBA days, but the differences are small. Below 650 hPa, the temperatures are higher on high-BBA days, with a maximum of 3 K at 800 hPa in the AN and 6 K at 850 hPa in the CN; thus, the inversion is stronger and lower-lying in the CN compared to in the AN on high-BBA days. This may be a potential reason for the 45 min difference in the later median dissipation time between the CN and AN in Fig. 3
Additionally, the morning development of the boundary layer height (see Appendix B) indicates that the planetary boundary layer (PBL) deepens slightly more until noon on low-BBA days. In the CN, the PBL is marginally lower on high-BBA days; however, the differences are minimal, as shown by the largely overlapping standard deviation areas.
There are distinct meteorological differences between high- and low-BBA days in the two regions, which are associated with the BBA transport and its local loading in the considered regions. However, by comparing situations averaged over hundreds of days, this study does not effectively capture out-of-the-ordinary events, such as mid-latitude intrusion events (Zhang and Zuidema, 2021), which can significantly impact BBA transport and distribution on specific days. While detailed case studies using back trajectories could analyze the variability of such events, they are beyond the scope of this study.
To quantify and attempt to disentangle the contributions of the relevant meteorological parameters and BBAs to the observed differences in FLC dissipation time, a statistical approach is used.
3.3 Statistical estimation of partial contributions to FLC dissipation
As described in Sect. 2.5, spatial data of meteorology and BBAs are used to predict FLC dissipation time with an ensemble of ridge regression models. The ensemble average skill in the AN is R2=0.34, with a standard deviation of 0.04, and in the CN, it is R2=0.30, with a standard deviation of 0.03. The plot of actual versus predicted dissipation times (see Appendix C) exhibits a relatively large spread around the line of perfect agreement, with a tendency for over-prediction in both the AN and CN. Nevertheless, the highest density of points broadly follows the diagonal line. The comparatively low predictive skill likely means that important information for the prediction of FLC dissipation time is not captured by the selected predictors. For example, variability in downwelling shortwave radiation (driven by, e.g., sub-seasonal variability in solar geometry and day-to-day variability in higher-level clouds), the temporal evolution of meteorological fields and precise vertical information regarding BBAs would likely increase the skill of the model. Additionally, it is also possible to use individual members from the ensemble data assimilation of ERA5 as predictors to potentially increase the variability. An additional limitation of the model is that the coarse resolution of the predictors can make it difficult for the model to capture local aerosol effects. Figure 7 shows ensemble mean predicted FLC dissipation times on low- and high-BBA days in the AN (a) and CN (b) as boxplots, similarly to the observations shown in Fig. 3. The variability in the predicted FLC dissipation times is lower than observed, a typical sign that the statistical model is underfitting. Despite the limitations of the statistical model ensemble, the majority of the observed significant differences (p<0.01) in the FLC dissipation time between high- and low-BBA days can be reproduced. Dissipation of FLCs is predicted to occur later during high-BBA days. The median dissipation time is 35 min later in the AN and 44 min later in the CN, and so the model reproduces exactly the observed difference in the AN but underestimates the observed differences in the CN. In the following, the mean coefficient fields of the model ensemble are used to estimate the contributions of the meteorological and BBA predictors to the predicted differences in the FLC dissipation time and, therefore, to quantify the influences of the processes outlined in Sect. 3.2.
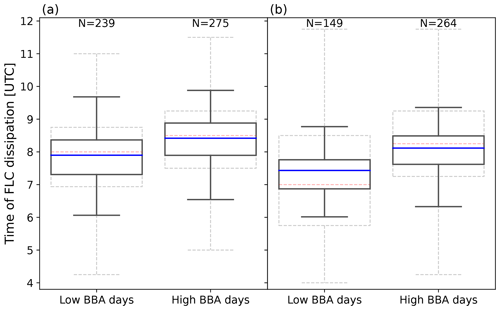
Figure 7Ensemble mean predicted time of dissipation of FLC events in the AN (a) and CN (b) during the biomass burning season during the 2004–2018 period. The blue lines represent the median dissipation times, and the whiskers extend to the highest (lowest) data points still within 1.5× the interquartile range from the third (first) quartile. FLC dissipation is significantly later (p<0.01) on high-BBA days in both regions. Observed dissipation times are outlined with dashed lines in the background. N indicates the number of days per group.
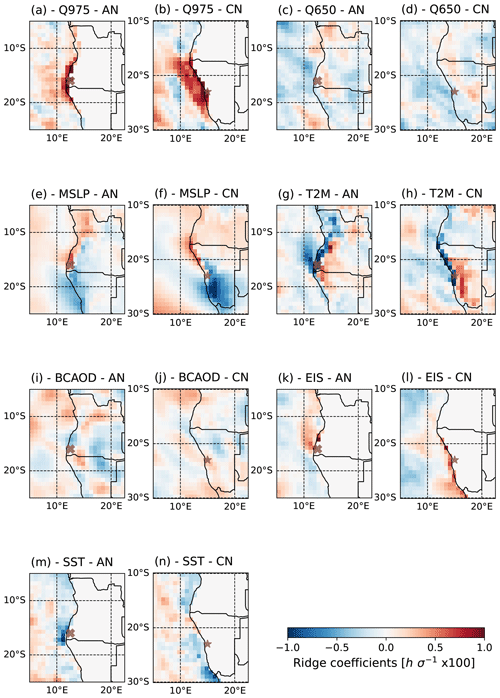
Figure 8Ensemble mean spatial coefficient fields (hours per standard deviation × 100) for each predictor: (a, b) specific humidity at 975 hPa with subsurface regions masked, (c, d) specific humidity at 650 hPa, (e, f) mean sea level pressure, (g, h) 2 m temperature, (i, j) black carbon AOD, (k, l) estimated inversion strength and (m, n) sea surface temperature in the AN (a, c, e, g, i, k, m, o) and the CN (b, d, f, h, j, l, n, p). The brown cross (respectively, star) represents the center of the AN (respectively, the CN).
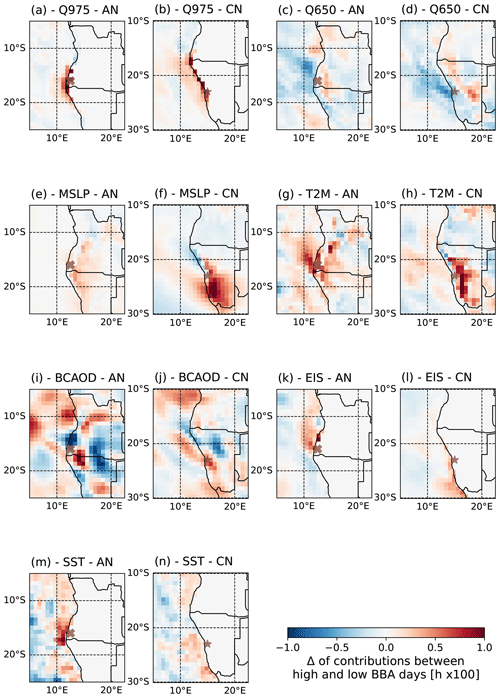
Figure 9Ensemble mean differences (high-BBA days − low-BBA days) of spatial contributions (hours × 100) for each predictor: (a, b) specific humidity at 975 hPa with subsurface regions masked, (c, d) specific humidity at 650 hPa, (e, f) mean sea level pressure, (g, h) 2 m temperature, (i, j) black carbon AOD, (k, l) estimated inversion strength and (m, n) sea surface temperature in the AN (a, c, e, g, i, k, m, o) and the CN (b, d, f, h, j, l, n, p). The brown cross (respectively, star) represents the center of the AN (respectively, the CN).
Figure 8 shows the mean spatial coefficient fields for each predictor used in the model ensemble: Q975 (a, b), Q650 (c, d), MSLP (e, f), T2M (g, h), BCAOD (i, j), EIS (k, l) and SST (m, n) in the AN and the CN. A clear coefficient pattern is apparent in the regional coastal Q975 (Fig. 8a and b) in both regions. FLC dissipation time is shown to be sensitive to boundary layer moisture exactly where the anomalies on high-BBA days are observed (Fig. 5). Coefficient fields of MSLP (Fig. 8e and f) show that the coastal circulation (onshore advection of moist air masses in the boundary layer) is important not only for the occurrence of FLCs (Andersen et al., 2020) but also for its lifetime. Near-surface air temperatures (Fig. 8g and h) also show a clear spatial coefficient pattern, with negative coefficients along the coastline and positive coefficients further inland. These patterns may describe the radiative feedback of FLCs on near-surface air temperatures along the coast and the contribution to the heat low further inland. For BCAOD (Fig. 8i and j), the coefficient pattern is fairly noisy and does not give a clear signal. Nevertheless, there is a very localized positive sensitivity of FLC dissipation time to EIS (Fig. 8k and i), which, in turn, may be partially driven by BBA absorption (Fig. 6). The coefficient pattern of SST (Fig. 8o and p) suggests that colder temperatures in isolated coastal regions may be related to delayed FLC dissipation times.
By multiplying the ensemble mean coefficients shown in Fig. 8 by the mean predictor fields on high- and low-BBA days, the spatial contributions of each predictor to the predicted FLC dissipation times (Fig. 7) are obtained. Subtracting the contributions on low-BBA days from those on high-BBA days results in the contributions to later dissipation times during high-BBA days. This is shown in Fig. 9 for the same predictors used in Fig. 8 in the AN and the CN.
A strong positive contributor to later dissipation times is Q975 in both regions (Fig. 9a and b) due to strong positive sensitives of the model (Fig. 8) and positive Q975 anomalies (Fig. 5). Despite positive anomalies in the PBL, the coefficient field for Q650 (Fig. 9c and d) is noisy in both regions and so are the contributions. Nevertheless, there are positive contributions over land, where a potential greenhouse warming may lead to strong warming contributing to the heat low and, thus, to the onshore circulation anomaly. Over the ocean, the negative contributions could be attributed to a reduction in the cloud top radiative cooling by the moist free troposphere (Zheng et al., 2018), which reduces the mixing of surface moisture with the FLCs (Caldwell et al., 2005). For MSLP, there is a strong positive contribution in the CN (Fig. 9f) and positive but weaker contributions in the AN (Fig. 9e). The difference in the magnitude of contributions between the AN and the CN can be explained by the stronger anomalies observed in the CN in Sect. 3.2 and the stronger circulation needed due to the fact that the CN is farther away from BBA sources than the AN. T2M is related to moisture in the free troposphere via greenhouse warming. In the case of the AN model (Fig. 9g) along the coastline, the negative sensitives coupled with the negative T2M anomalies result in positive contributions. Whereas, in the case of CN (Fig. 9g), the model's stronger inland positive T2M anomalies lead to weaker coastline negative anomalies and result in negative contributions. On the other hand, in both regions, a heat low leads to positive inland T2M contributions. BCAOD contributions are strong but noisy (Fig. 9i and j). Additionally, in this study, BCAOD is the parameter used to create the high- and low-BBA groups; therefore, the relative differences between the groups are particularly large, amplifying the noisy coefficient fields. For these reasons, the robustness of this contribution is questionable. On the other hand, the very localized but strong positive contribution of EIS in the AN (Fig. 9k) could be related to absorbing BBA layers leading to local heating in the free troposphere and, thus, to a stronger inversion. From this perspective, the weaker contributions in the CN (Fig. 9i) could be explained by the greater distance of the region from the BBA sources and the smaller concentration of smoke layers, as seen in Fig. 2a. Finally, SST has positive contributions in the AN (Fig. 9m). These contributions are the product of a negative coefficient field and a negative SST anomaly near the coastline (not shown). This could be partly explained by reduced incoming solar radiation due to absorption in the free troposphere. However, since the ocean surface only responds slowly, other factors, such as the changed circulation, possibly contribute as well. Mallet et al. (2024), in a recent model-based study, found that an SST decrease, associated with solar heating in the free troposphere, is responsible for a positive feedback of BBA radiative effects on low-level clouds. In the CN (Fig. 9n), contributions are weaker and noisier, and the SST anomaly is spatially removed from the CN and the BBA anomaly. Overall, according to the statistical model, increased moisture in the PBL and changes in coastal circulation, with an onshore anomaly, are the main causes of later FLC dissipation, which may be a bit counterbalanced by the moist free-troposphere air masses initiating FLC dissipation by reducing cloud top radiative cooling over the ocean.
The central objective of this study was to investigate to what extent the circulation of the seasonally occurring biomass burning aerosols (BBAs) may influence the dissipation time of fog and low clouds (FLCs) in the Namib region and, more precisely, in two sub-regions with a high frequency of FLC events: the Central Namib and the Angolan Namib. To do this, a novel satellite-based data set of FLC dissipation times was used. In addition, the ERA5 reanalysis of meteorological fields and CAMS black carbon AOD products were analyzed. For each of the two regions, two groups of days with high and low BBA loading were created to analyze possible BBA effects on FLC dissipation time. The main findings of this study are as follows:
-
During the BBA season (June–October) of the investigated 15-year period (2004–2018), FLC dissipation time is significantly later on high-BBA days in both regions. This is consistent with our guiding hypothesis and with recent studies, which have shown that BBA layers tend to increase cloudiness (Wilcox, 2010; Sakaeda et al., 2011; Gordon et al., 2018; Deaconu et al., 2019). However, these studies were focused on the South Atlantic; here, this knowledge is extended over land. However, the grouping of high- and low-BBA days has been shown to lead to meteorological sampling biases, complicating the separation of meteorological effects from the “large-scale” semi-direct BBA effects.
-
BBAs are shown to be transported along moist free-tropospheric air by a large-scale circulation pattern. The associated longwave heating causes a continental heat low, which modifies the coastal circulation and boundary layer humidity along the coastline. A longwave heating modulating coastal circulation has been shown to drive fog occurrence (Adebiyi et al., 2015; Adebiyi and Zuidema, 2018; Andersen et al., 2020). Here, the understanding of the system is expanded by showing that it is also important for the dissipation of FLCs. Additionally, this study shows that, similarly to the South Atlantic (Adebiyi et al., 2015; Adebiyi and Zuidema, 2018; Deaconu et al., 2019), longwave heating impacts FLCs over land as well.
-
The temporal development of vertical temperatures shows a stronger nighttime cooling and surface and boundary layer warming during high-BBA days. After sunrise, the free troposphere features stronger warming, whereas the boundary layer does not warm as quickly during high-BBA days, strengthening the local inversion. Because aerosol effects have been shown to change sign after sunrise (Herbert et al., 2020), the differences in temporal temperature evolution are thought to be driven by the greenhouse effect of moisture in the BBA plumes and BBA absorption. These factors may contribute to the observed differences in FLC dissipation.
-
A statistical model (ridge regression) was used to quantify and partially disentangle meteorological and BBA effects. The statistical model is shown to be able to reproduce the observed differences in FLC dissipation time on high- and low-BBA days, with these differences mainly being attributed to differences in circulation, boundary layer humidity and near-surface temperatures along the coastline. With this model, it is not possible to make definitive conclusions about BBA effects as the underfitting makes it hard to capture subtle semi-direct BBA effects on FLC dissipation. Additionally, using this model requires the assumption of linearity in the underlying processes. Despite these limitations, the contributions of EIS, for example, may hint at semi-direct BBA effects.
The findings highlight the difficulties of disentangling meteorological and aerosol effects on low-cloud development through statistical modeling approaches. While most of the differences in FLC dissipation time are likely driven by meteorology, some observational indications of possible semi-direct effects on FLC dissipation are found. To further investigate and fully disentangle meteorological and BBA effects, targeted analyses with large-eddy simulations are essential. Additional approaches to better constrain meteorological influences can be employed, such as selecting air masses with similar hydrologic histories using isotope observations (Henze et al., 2023) and/or computing backward trajectories of air masses with comparable dynamical and thermodynamical conditions (Andersen et al., 2020) but contrasting BBA loadings. Additionally, radiative sensitivity studies, such as that of Obregón et al. (2018), could also be useful in disentangling aerosol direct effects from meteorological covariates. New satellite data from cutting-edge instruments, such as the high-spectral-resolution lidar onboard the EarthCARE satellite (Wehr et al., 2023), will improve our understanding of aerosol and cloud interactions. In a different approach, one could use a statistical model similar to the one developed here, with CMIP6 data as predictors, as done by Ceppi and Nowack (2021), to assess the impacts of climate change on FLCs in the region.
CAMS data were generated using the Copernicus Atmosphere Monitoring Service. ERA5 data were generated using Copernicus Climate Change Service information. CALIPSO data were generated using the CALIPSO Search and Subsetting web application. Satellite FLC data are available at https://doi.org/10.35097/z5adwk39bbfke404 (Pauli et al., 2024). The code for data processing is available from the corresponding author upon reasonable request.
AM, HA and JC had the idea for the analysis. AM obtained and analyzed most of the data sets, conducted the original research, and wrote the paper. EP contributed to the design and creation of the satellite FLC data set. HA, JC, PF, EP and JQ contributed to the article preparation and the interpretation of the findings.
The contact author has declared that none of the authors has any competing interests.
Publisher’s note: Copernicus Publications remains neutral with regard to jurisdictional claims made in the text, published maps, institutional affiliations, or any other geographical representation in this paper. While Copernicus Publications makes every effort to include appropriate place names, the final responsibility lies with the authors.
This article is part of the special issue “New observations and related modelling studies of the aerosol–cloud–climate system in the Southeast Atlantic and southern Africa regions (ACP/AMT inter-journal SI)”. It is not associated with a conference.
Paola Formenti was funded through the AEROCLO-sA project, supported by the French National Research Agency under grant agreement no. ANR-15-CE01-0014-01, the French national program LEFE/INSU, the Programme national de Télédetection Spatiale (grant no. PNTS-2016-14), the French National Agency for Space Studies (CNES), the South African National Research Foundation (NRF) under grant no. UID 105958 and the European Union's Seventh Framework Programme (grant no. FP7/2014-2018) under EUFAR2 contract no. 312609. The contribution of Julian Quinting was funded by the European Union (ERC, ASPIRE, grant no. 101077260). We thank Martina Klose for the discussion and ideas which improved the study. We are also grateful to the editor, Franziska Aemisegger, and the two anonymous reviewers for their careful and constructive feedback, which has helped improve the paper.
This research has been supported by the Deutsche Forschungsgemeinschaft (grant no. 462604610) in the project Namib Fog Life Cycle Analysis – Aerosols and Climate (NaFoLi[CA]2).
The article processing charges for this open-access publication were covered by the Karlsruhe Institute of Technology (KIT).
This paper was edited by Franziska Aemisegger and reviewed by two anonymous referees.
Adebiyi, A. A. and Zuidema, P.: The role of the southern African easterly jet in modifying the southeast Atlantic aerosol and cloud environments, Q. J. Roy. Meteor. Soc., 142, 1574–1589, https://doi.org/10.1002/qj.2765, 2016. a, b, c
Adebiyi, A. A. and Zuidema, P.: Low cloud cover sensitivity to biomass-burning aerosols and meteorology over the southeast Atlantic, J. Clim., 31, 4329–4346, https://doi.org/10.1175/JCLI-D-17-0406.1, 2018. a, b, c, d, e, f
Adebiyi, A. A., Zuidema, P., and Abel, S. J.: The convolution of dynamics and moisture with the presence of shortwave absorbing aerosols over the southeast Atlantic, J. Clim., 28, 1997–2024, https://doi.org/10.1175/JCLI-D-14-00352.1, 2015. a, b, c, d, e, f, g
Adesina, J. A., Piketh, S. J., Formenti, P., Maggs-Kölling, G., Holben, B. N., and Sorokin, M. G.: Aerosol optical properties and direct radiative effect over Gobabeb, Namibia, Clean Air J., 29, 1–11, https://doi.org/10.17159/caj/2019/29/2.7518, 2019. a
Alamirew, N. K., Todd, M. C., Ryder, C. L., Marsham, J. H., and Wang, Y.: The early summertime Saharan heat low: sensitivity of the radiation budget and atmospheric heating to water vapour and dust aerosol, Atmos. Chem. Phys., 18, 1241–1262, https://doi.org/10.5194/acp-18-1241-2018, 2018. a
Andersen, H. and Cermak, J.: First fully diurnal fog and low cloud satellite detection reveals life cycle in the Namib, Atmos. Meas. Tech., 11, 5461–5470, https://doi.org/10.5194/amt-11-5461-2018, 2018. a, b, c, d, e, f, g, h
Andersen, H., Cermak, J., Solodovnik, I., Lelli, L., and Vogt, R.: Spatiotemporal dynamics of fog and low clouds in the Namib unveiled with ground-and space-based observations, Atmos. Chem. Phys., 19, 4383–4392, https://doi.org/10.5194/acp-19-4383-2019, 2019. a, b, c, d, e
Andersen, H., Cermak, J., Fuchs, J., Knippertz, P., Gaetani, M., Quinting, J., Sippel, S., and Vogt, R.: Synoptic-scale controls of fog and low-cloud variability in the Namib Desert, Atmos. Chem. Phys., 20, 3415–3438, https://doi.org/10.5194/acp-20-3415-2020, 2020. a, b, c, d, e, f, g, h, i
Caldwell, P., Bretherton, C. S., and Wood, R.: Mixed-layer budget analysis of the diurnal cycle of entrainment in southeast Pacific stratocumulus, J. Atmos. Sci., 62, 3775–3791, https://doi.org/10.1175/JAS3561.1, 2005. a
Ceppi, P. and Nowack, P.: Observational evidence that cloud feedback amplifies global warming, P. Natl. Acad. Sci. USA, 118, e2026290118, https://doi.org/10.1073/pnas.2026290118, 2021. a
Cermak, J.: Low clouds and fog along the South-Western African coast – Satellite-based retrieval and spatial patterns, Atmos. Res., 116, 15–21, https://doi.org/10.1016/j.atmosres.2011.02.012, 2012. a, b
Cermak, J.: Fog and low cloud frequency and properties from active-sensor satellite data, Remote Sens., 10, 1209, https://doi.org/10.3390/rs10081209, 2018. a
Che, H., Stier, P., Watson-Parris, D., Gordon, H., and Deaconu, L.: Source attribution of cloud condensation nuclei and their impact on stratocumulus clouds and radiation in the south-eastern Atlantic, Atmos. Chem. Phys., 22, 10789–10807, https://doi.org/10.5194/acp-22-10789-2022, 2022. a
Costantino, L. and Bréon, F.-M.: Aerosol indirect effect on warm clouds over South-East Atlantic, from co-located MODIS and CALIPSO observations, Atmos. Chem. Phys., 13, 69–88, https://doi.org/10.5194/acp-13-69-2013, 2013. a
De Graaf, M., Bellouin, N., Tilstra, L., Haywood, J., and Stammes, P.: Aerosol direct radiative effect of smoke over clouds over the southeast Atlantic Ocean from 2006 to 2009, Geophys. Res. Lett., 41, 7723–7730, https://doi.org/10.1002/2014GL061103, 2014. a
De Graaf, M., Schulte, R., Peers, F., Waquet, F., Tilstra, L. G., and Stammes, P.: Comparison of south-east Atlantic aerosol direct radiative effect over clouds from SCIAMACHY, POLDER and OMI–MODIS, Atmos. Chem. Phys., 20, 6707–6723, https://doi.org/10.5194/acp-20-6707-2020, 2020. a
Deaconu, L. T., Ferlay, N., Waquet, F., Peers, F., Thieuleux, F., and Goloub, P.: Satellite inference of water vapour and above-cloud aerosol combined effect on radiative budget and cloud-top processes in the southeastern Atlantic Ocean, Atmos. Chem. Phys., 19, 11613–11634, https://doi.org/10.5194/acp-19-11613-2019, 2019. a, b, c, d, e, f
Denjean, C., Brito, J., Libois, Q., Mallet, M., Bourrianne, T., Burnet, F., Dupuy, R., Flamant, C., and Knippertz, P.: Unexpected biomass burning aerosol absorption enhancement explained by black carbon mixing state, Geophys. Res. Lett., 47, e2020GL089055, https://doi.org/10.1029/2020GL089055, 2020. a
Diamond, M. S., Dobracki, A., Freitag, S., Small Griswold, J. D., Heikkila, A., Howell, S. G., Kacarab, M. E., Podolske, J. R., Saide, P. E., and Wood, R.: Time-dependent entrainment of smoke presents an observational challenge for assessing aerosol–cloud interactions over the southeast Atlantic Ocean, Atmos. Chem. Phys., 18, 14623–14636, https://doi.org/10.5194/acp-18-14623-2018, 2018. a, b
Diamond, M. S., Saide, P. E., Zuidema, P., Ackerman, A. S., Doherty, S. J., Fridlind, A. M., Gordon, H., Howes, C., Kazil, J., Yamaguchi, T., Zhang, J., Feingold, G., and Wood, R.: Cloud adjustments from large-scale smoke–circulation interactions strongly modulate the southeastern Atlantic stratocumulus-to-cumulus transition, Atmos. Chem. Phys., 22, 12113–12151, https://doi.org/10.5194/acp-22-12113-2022, 2022. a
Ebner, M., Miranda, T., and Roth-Nebelsick, A.: Efficient fog harvesting by Stipagrostis sabulicola (Namib dune bushman grass), J. Arid Environ., 75, 524–531, https://doi.org/10.1016/j.jaridenv.2011.01.004, 2011. a
Flamant, C., Gaetani, M., Chaboureau, J.-P., Chazette, P., Cuesta, J., Piketh, S. J., and Formenti, P.: Smoke in the river: an Aerosols, Radiation and Clouds in southern Africa (AEROCLO-sA) case study, Atmos. Chem. Phys., 22, 5701–5724, https://doi.org/10.5194/acp-22-5701-2022, 2022. a
Formenti, P., D'Anna, B., Flamant, C., Mallet, M., Piketh, S. J., Schepanski, K., Waquet, F., Auriol, F., Brogniez, G., Burnet, F., Chaboureau, J.-P., Chauvigné, A., Chazette, P., Denjean, C., Desboeufs, K., Doussin, J.-F., Elguindi, N., Feuerstein, S., Gaetani, M., Giorio, C., Klopper, D., Mallet, M. D., Nabat, P., Monod, A., Solmon, F., Namwoonde, A., Chikwililwa, C., Mushi, R., Welton, E. J., and Holben, B.: The aerosols, radiation and clouds in southern Africa field campaign in Namibia: Overview, illustrative observations, and way forward, Bull. Am. Meteorol. Soc., 100, 1277–1298, https://doi.org/10.1175/BAMS-D-17-0278.1, 2019. a
Friedman, J., Hastie, T., and Tibshirani, R.: Regularization paths for generalized linear models via coordinate descent, J. Stat. Softw., 33, 1–22, https://doi.org/10.18637/jss.v033.i01, 2010. a
Fuchs, J., Cermak, J., Andersen, H., Hollmann, R., and Schwarz, K.: On the influence of air mass Origin on low-cloud properties in the Southeast Atlantic, J. Geophys. Res.-Atmos., 122, 11076-11091 https://doi.org/10.1002/2017JD027184, 2017. a
Fuchs, J., Cermak, J., and Andersen, H.: Building a cloud in the southeast Atlantic: understanding low-cloud controls based on satellite observations with machine learning, Atmos. Chem. Phys., 18, 16537–16552, https://doi.org/10.5194/acp-18-16537-2018, 2018. a
Gordon, H., Field, P. R., Abel, S. J., Dalvi, M., Grosvenor, D. P., Hill, A. A., Johnson, B. T., Miltenberger, A. K., Yoshioka, M., and Carslaw, K. S.: Large simulated radiative effects of smoke in the south-east Atlantic, Atmos. Chem. Phys., 18, 15261–15289, https://doi.org/10.5194/acp-18-15261-2018, 2018. a, b, c
Gottlieb, T. R., Eckardt, F. D., Venter, Z. S., and Cramer, M. D.: The contribution of fog to water and nutrient supply to Arthraerua leubnitziae in the central Namib Desert, Namibia, J. Arid Environ., 161, 35–46, https://doi.org/10.1016/j.jaridenv.2018.11.002, 2019. a
Gueymard, C. A. and Yang, D.: Worldwide validation of CAMS and MERRA-2 reanalysis aerosol optical depth products using 15 years of AERONET observations, Atmos. Environ., 225, 117216, https://doi.org/10.1016/j.atmosenv.2019.117216, 2020. a
Gupta, S., McFarquhar, G. M., O'Brien, J. R., Poellot, M. R., Delene, D. J., Chang, I., Gao, L., Xu, F., and Redemann, J.: In situ and satellite-based estimates of cloud properties and aerosol–cloud interactions over the southeast Atlantic Ocean, Atmos. Chem. Phys., 22, 12923–12943, https://doi.org/10.5194/acp-22-12923-2022, 2022. a
Hansen, J., Sato, M., and Ruedy, R.: Radiative forcing and climate response, J. Geophys. Res.-Atmos., 102, 6831–6864, https://doi.org/10.1029/96JD03436, 1997. a, b
Haywood, J. M., Abel, S. J., Barrett, P. A., Bellouin, N., Blyth, A., Bower, K. N., Brooks, M., Carslaw, K., Che, H., Coe, H., Cotterell, M. I., Crawford, I., Cui, Z., Davies, N., Dingley, B., Field, P., Formenti, P., Gordon, H., de Graaf, M., Herbert, R., Johnson, B., Jones, A. C., Langridge, J. M., Malavelle, F., Partridge, D. G., Peers, F., Redemann, J., Stier, P., Szpek, K., Taylor, J. W., Watson-Parris, D., Wood, R., Wu, H., and Zuidema., P.: The CLoud–Aerosol–Radiation interaction and forcing: Year 2017 (CLARIFY-2017) measurement campaign, Atmos. Chem. Phys., 21, 1049–1084, https://doi.org/10.5194/acp-21-1049-2021, 2021. a
Henze, D., Noone, D., and Toohey, D.: Detection of dilution due to turbulent mixing vs. precipitation scavenging effects on biomass burning aerosol concentrations using stable water isotope ratios during ORACLES, Atmos. Chem. Phys., 23, 15269–15288, https://doi.org/10.5194/acp-23-15269-2023, 2023. a
Herbert, R. J., Bellouin, N., Highwood, E. J., and Hill, A. A.: Diurnal cycle of the semi-direct effect from a persistent absorbing aerosol layer over marine stratocumulus in large-eddy simulations, Atmos. Chem. Phys., 20, 1317–1340, https://doi.org/10.5194/acp-20-1317-2020, 2020. a, b, c, d
Hersbach, H., Peubey, C., Simmons, A., Berrisford, P., Poli, P., and Dee, D.: ERA-20CM: A twentieth-century atmospheric model ensemble, Q. J. Roy. Meteorol. Soc., 141, 2350–2375, https://doi.org/10.1002/qj.2528, 2015. a
Hersbach, H., Bell, B., Berrisford, P., Hirahara, S., Horányi, A., Muñoz-Sabater, J., Nicolas, J., Peubey, C., Radu, R., Schepers, D., Simmons, A., Soci, C., Abdalla, S., Abellan, X., Balsamo, G., Bechtold, P., Biavati, G., Bidlot, J., Bonavita, M., De Chiara, G., Dahlgren, P., Dee, D., Diamantakis, M., Dragani, R., Flemming, J., Forbes, R., Fuentes, M., Geer, A., Haimberger, L., Healy, S., Hogan, R. J., Hólm, E., Janisková, M., Keeley, S., Laloyaux, P., Lopez, P., Lupu, C., Radnoti, G., de Rosnay, P., Rozum, I., Vamborg, F., Villaume, S., and Thépaut, J.-N.: The ERA5 global reanalysis, Q. J. Roy. Meteorol. Soc., 146, 1999–2049, https://doi.org/10.1002/qj.3803, 2020. a, b
Inness, A., Ades, M., Agustí-Panareda, A., Barré, J., Benedictow, A., Blechschmidt, A.-M., Dominguez, J. J., Engelen, R., Eskes, H., Flemming, J., Huijnen, V., Jones, L., Kipling, Z., Massart, S., Parrington, M., Peuch, V.-H., Razinger, M., Remy, S., Schulz, M., and Suttie, M.: The CAMS reanalysis of atmospheric composition, Atmos. Chem. Phys., 19, 3515–3556, https://doi.org/10.5194/acp-19-3515-2019, 2019. a
James, G., Witten, D., Hastie, T., and Tibshirani, R.: Introduction, 1–14, Springer US, New York, NY, ISBN 978-1-0716-1418-1, https://doi.org/10.1007/978-1-0716-1418-1_1, 2021. a
James, R. and Washington, R.: Changes in African temperature and precipitation associated with degrees of global warming, Climatic Change, 117, 859–872, https://doi.org/10.1007/s10584-012-0581-7, 2013. a
Lancaster, J., L. N. and S. M.: Climate of the central Namib Desert, Madoqua, 14, 5–61, https://hdl.handle.net/10520/AJA10115498_484 (last access: 13 January 2025), 1984. a
Lindesay, J. and Tyson, P.: Climate and near-surface airflow over the central Namib. Regional aeolian dynamics in the Namib, Desert sand dune dynamics: review and prospect, Transvaal Museum Monographs, 7, 1, https://hdl.handle.net/10520/AJA090799001_175 (last access: 13 January 2025), 1990. a
Louw, G. N. and Holm, E.: Physiological, morphological and behavioural adaptations of the ultrapsammophilous, Namib Desert lizard Aporosaura anchietae (Bocage), Madoqua, 1, 67–85, 1972. a
Mallet, M., Voldoire, A., Solmon, F., Nabat, P., Drugé, T., and Roehrig, R.: Impact of Biomass Burning Aerosols (BBA) on the tropical African climate in an ocean-atmosphere-aerosols coupled climate model, EGUsphere [preprint], https://doi.org/10.5194/egusphere-2024-496, 2024. a
Maúre, G., Pinto, I., Ndebele-Murisa, M., Muthige, M., Lennard, C., Nikulin, G., Dosio, A., and Meque, A.: The southern African climate under 1.5 °C and 2 °C of global warming as simulated by CORDEX regional climate models, Environ. Res. Lett., 13, 065002, https://doi.org/10.1088/1748-9326/aab190, 2018. a
Obregón, M., Costa, M., Silva, A., and Serrano, A.: Impact of aerosol and water vapour on SW radiation at the surface: Sensitivity study and applications, Atmos. Res., 213, 252–263, https://doi.org/10.1016/j.atmosres.2018.06.001, 2018. a
Olivier, J.: Spatial distribution of fog in the Namib, J. Arid Environ., 29, 129–138, https://doi.org/10.1016/S0140-1963(05)80084-9, 1995. a
Olivier, J. and Stockton, P.: The influence of upwelling extent upon fog incidence at Lüderitz, southern Africa, Int. J. Climatol., 9, 69–75, https://doi.org/10.1002/joc.3370090106, 1989. a
Pauli, E., Cermak, J., and Andersen, H.: A satellite-based climatology of fog and low stratus formation and dissipation times in central Europe, Q. J. Roy. Meteorol. Soc., 148, 1439–1454, https://doi.org/10.1002/qj.4272, 2022. a
Pauli, E., Andersen, H., Cermak, J., and Mass, A.: Satellite-based fog and low stratus cloud formation and dissipation time data set over the Namib Desert, RADAR [data set], https://doi.org/10.35097/z5adwk39bbfke404, 2024. a
Pedregosa, F., Varoquaux, G., Gramfort, A., Michel, V., Thirion, B., Grisel, O., Blondel, M., Louppe, G., Prettenhofer, P., Weiss, R., Dubourg, V., Vanderplas, J., Passos, A., Cournapeau, D., Brucher, M., Perrot, M., and Duchesnay, É.: Scikit-learn: Machine learning in Python, J. Mach. Learn. Res., 12, 2825–2830, 2011. a
Price, J. D., Lane, S., Boutle, I. A., Smith, D. K. E., Bergot, T., Lac, C., Duconge, L., McGregor, J., Kerr-Munslow, A., Pickering, M., and Clark., R.: LANFEX: a field and modeling study to improve our understanding and forecasting of radiation fog, Bull. Am. Meteorol. Soc., 99, 2061–2077, https://doi.org/10.1175/BAMS-D-16-0299.1, 2018. a
Rajapakshe, C., Zhang, Z., Yorks, J. E., Yu, H., Tan, Q., Meyer, K., Platnick, S., and Winker, D. M.: Seasonally transported aerosol layers over southeast Atlantic are closer to underlying clouds than previously reported, Geophys. Res. Lett., 44, 5818–5825, https://doi.org/10.1002/2017GL073559, 2017. a
Redemann, J., Wood, R., Zuidema, P., Doherty, S. J., Luna, B., LeBlanc, S. E., Diamond, M. S., Shinozuka, Y., Chang, I. Y., Ueyama, R., Pfister, L., Ryoo, J.-M., Dobracki, A. N., da Silva, A. M., Longo, K. M., Kacenelenbogen, M. S., Flynn, C. J., Pistone, K., Knox, N. M., Piketh, S. J., Haywood, J. M., Formenti, P., Mallet, M., Stier, P., Ackerman, A. S., Bauer, S. E., Fridlind, A. M., Carmichael, G. R., Saide, P. E., Ferrada, G. A., Howell, S. G., Freitag, S., Cairns, B., Holben, B. N., Knobelspiesse, K. D., Tanelli, S., L'Ecuyer, T. S., Dzambo, A. M., Sy, O. O., McFarquhar, G. M., Poellot, M. R., Gupta, S., O'Brien, J. R., Nenes, A., Kacarab, M., Wong, J. P. S., Small-Griswold, J. D., Thornhill, K. L., Noone, D., Podolske, J. R., Schmidt, K. S., Pilewskie, P., Chen, H., Cochrane, S. P., Sedlacek, A. J., Lang, T. J., Stith, E., Segal-Rozenhaimer, M., Ferrare, R. A., Burton, S. P., Hostetler, C. A., Diner, D. J., Seidel, F. C., Platnick, S. E., Myers, J. S., Meyer, K. G., Spangenberg, D. A., Maring, H., and Gao., L.: An overview of the ORACLES (ObseRvations of Aerosols above CLouds and their intEractionS) project: aerosol–cloud–radiation interactions in the southeast Atlantic basin, Atmos. Chem. Phys., 21, 1507–1563, https://doi.org/10.5194/acp-21-1507-2021, 2021. a, b
Sakaeda, N., Wood, R., and Rasch, P. J.: Direct and semidirect aerosol effects of southern African biomass burning aerosol, J. Geophys. Res.-Atmos., 116, D12205, https://doi.org/10.1029/2010JD015540, 2011. a, b
Schmetz, J., Pili, P., Tjemkes, S., Just, D., Kerkmann, J., Rota, S., and Ratier, A.: An introduction to Meteosat second generation (MSG), Bull. Am. Meteorol. Soc., 83, 977–992, https://doi.org/10.1175/1520-0477(2002)083<0977:AITMSG>2.3.CO;2, 2002. a
Seely, M. K. and Henschel, J. R.: The climatology of Namib fog, in: Proceedings of the First International Conference on Fog and Fog Collection, Vancouver, Canada, 19–24 July 1998, 353–356, https://www.researchgate.net/profile/Joh-Henschel/ (last access: 13 January 2025), 1998. a, b, c
Spirig, R., Vogt, R., Larsen, J. A., Feigenwinter, C., Wicki, A., Franceschi, J., Parlow, E., Adler, B., Kalthoff, N., Cermak, J., Andersen, H., Fuchs, J., Bott, A., Hacker, M., Wagner, N., Maggs-Kölling, G., Wassenaar, T., and Seely, M.: Probing the fog life cycles in the Namib Desert, Bull. Am. Meteorol. Soc., 100, 2491–2507, https://doi.org/10.1175/BAMS-D-18-0142.1, 2019. a, b
Swap, R., Garstang, M., Macko, S., Tyson, P., Maenhaut, W., Artaxo, P., Kållberg, P., and Talbot, R.: The long-range transport of southern African aerosols to the tropical South Atlantic, J. Geophys. Res.-Atmos., 101, 23777–23791, https://doi.org/10.1029/95JD01049, 1996. a
Taylor, J. W., Wu, H., Szpek, K., Bower, K., Crawford, I., Flynn, M. J., Williams, P. I., Dorsey, J., Langridge, J. M., Cotterell, M. I., Fox, C., Davies, N. W., Haywood, J. M., and Coe, H.: Absorption closure in highly aged biomass burning smoke, Atmos. Chem. Phys., 20, 11201–11221, https://doi.org/10.5194/acp-20-11201-2020, 2020. a
Vaughan, M., Pitts, M., Trepte, C., Winker, D., Getzewich, B., Tackett, J., Cai, X., Detweiler, P., Garnier, A., Kar, J., Lambeth, J., Lee, K.-P., Lucker, P., Magill, B., Murray, T., Rodier, S., Ryan, R., Tremas, T., Pelon, J., and Flamant, C.: Cloud-Aerosol LIDAR Infrared Pathfinder Satellite Observations (CALIPSO) data management system data products catalog, Release 4.95, Document pc-sci-503, NASA Langley Research Center, https://www-calipso.larc.nasa.gov/products/CALIPSO_DPC_Rev4x95.pdf (last access: 13 January 2025), 2023. a
Vaughan, M. A., Young, S. A., Winker, D. M., Powell, K. A., Omar, A. H., Liu, Z., Hu, Y., and Hostetler, C. A.: Fully automated analysis of space-based lidar data: An overview of the CALIPSO retrieval algorithms and data products, BBA Lib., 5575, 16–30, https://doi.org/10.1117/12.572024, 2004. a
Vautard, R., Yiou, P., and Van Oldenborgh, G. J.: Decline of fog, mist and haze in Europe over the past 30 years, Nat. Geosci., 2, 115–119, https://doi.org/10.1038/ngeo414, 2009. a
Wang, L., Kaseke, K. F., Ravi, S., Jiao, W., Mushi, R., Shuuya, T., and Maggs-Kölling, G.: Convergent vegetation fog and dew water use in the Namib Desert, Ecohydrology, 12, e2130, https://doi.org/10.1002/eco.2130, 2019. a
Warren-Rhodes, K. A., McKay, C. P., Boyle, L. N., Wing, M. R., Kiekebusch, E. M., Cowan, D. A., Stomeo, F., Pointing, S. B., Kaseke, K. F., Eckardt, F., Henschel, J. R., Anisfeld, A., Seely, M., and Rhodes., K. L.: Physical ecology of hypolithic communities in the central Namib Desert: the role of fog, rain, rock habitat, and light, J. Geophys. Res.-Biogeo., 118, 1451–1460, https://doi.org/10.1002/jgrg.20117, 2013. a
Wehr, T., Kubota, T., Tzeremes, G., Wallace, K., Nakatsuka, H., Ohno, Y., Koopman, R., Rusli, S., Kikuchi, M., Eisinger, M., Tanaka, T., Taga, M., Deghaye, P., Tomita, E., and Bernaerts, D.: The EarthCARE mission – science and system overview, Atmos. Meas. Tech., 16, 3581–3608, https://doi.org/10.5194/amt-16-3581-2023, 2023. a
Wilcox, E.: Stratocumulus cloud thickening beneath layers of absorbing smoke aerosol, Atmos. Chem. Phys., 10, 11769–11777, https://doi.org/10.5194/acp-10-11769-2010, 2010. a, b, c
Wood, R. and Bretherton, C. S.: On the relationship between stratiform low cloud cover and lower-tropospheric stability, J. Clim., 19, 6425–6432, https://doi.org/10.1175/JCLI3988.1, 2006. a
Zhang, J. and Zuidema, P.: The diurnal cycle of the smoky marine boundary layer observed during August in the remote southeast Atlantic, Atmos. Chem. Phys., 19, 14493–14516, https://doi.org/10.5194/acp-19-14493-2019, 2019. a
Zhang, J. and Zuidema, P.: Sunlight-absorbing aerosol amplifies the seasonal cycle in low-cloud fraction over the southeast Atlantic, Atmos. Chem. Phys., 21, 11179–11199, https://doi.org/10.5194/acp-21-11179-2021, 2021. a
Zheng, Y., Rosenfeld, D., and Li, Z.: The relationships between cloud top radiative cooling rates, surface latent heat fluxes, and cloud-base heights in marine stratocumulus, J. Geophys. Res.-Atmos., 123, 11–678, https://doi.org/10.1029/2018JD028579, 2018. a
Zipfel, L., Andersen, H., and Cermak, J.: Machine-Learning Based Analysis of Liquid Water Path Adjustments to Aerosol Perturbations in Marine Boundary Layer Clouds Using Satellite Observations, Atmosphere, 13, 586, https://doi.org/10.3390/atmos13040586, 2022. a
Zuidema, P., Redemann, J., Haywood, J., Wood, R., Piketh, S., Hipondoka, M., and Formenti, P.: Smoke and clouds above the southeast Atlantic: Upcoming field campaigns probe absorbing aerosol's impact on climate, Bull. Am. Meteorol. Soc., 97, 1131–1135, https://doi.org/10.1175/BAMS-D-15-00082.1, 2016. a, b
- Abstract
- Introduction
- Data and methods
- Results and discussion
- Conclusions and outlook
- Appendix A: Dissipation time and AOD distributions
- Appendix B: Morning boundary layer height development
- Appendix C: Prediction versus truth scatterplot
- Code and data availability
- Author contributions
- Competing interests
- Disclaimer
- Special issue statement
- Acknowledgements
- Financial support
- Review statement
- References
- Abstract
- Introduction
- Data and methods
- Results and discussion
- Conclusions and outlook
- Appendix A: Dissipation time and AOD distributions
- Appendix B: Morning boundary layer height development
- Appendix C: Prediction versus truth scatterplot
- Code and data availability
- Author contributions
- Competing interests
- Disclaimer
- Special issue statement
- Acknowledgements
- Financial support
- Review statement
- References