the Creative Commons Attribution 4.0 License.
the Creative Commons Attribution 4.0 License.
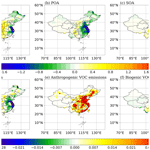
Understanding the long-term trend of organic aerosol and the influences from anthropogenic emission and regional climate change in China
Wenxin Zhang
Yaman Liu
Kan Huang
Minghuai Wang
Organic aerosol (OA) is a major type of fine particulate matter. OA shows a large variability influenced by anthropogenic emissions, vegetation, and meteorological changes. Understanding OA trends is crucial for air quality and climate studies, yet changes in OA over time in China are poorly documented. This study applied the Community Atmosphere Model version 6 with comprehensive tropospheric and stratospheric chemistry (CAM6-Chem) to investigate long-term OA trends in China from 1990 to 2019 and identify the driving factors. The simulations agreed well with ground-based measurements of OA from 151 observational sites and the CAQRA reanalysis dataset. Although OA trends showed a modest 5.6 % increase, this resulted from a significant −8.1 % decrease in primary organic aerosols (POAs) and a substantial 32.3 % increase in secondary organic aerosols (SOAs). Anthropogenic emissions of POA and volatile organic compounds (VOCs) were the dominant contributors to these trends. While biogenic VOCs (BVOCs) played a secondary role in SOA formation, significant changes were observed in specific subspecies: isoprene-derived SOA decreased by −18.8 % due to anthropogenic sulfate reduction, while monoterpene-derived SOA increased by 12.3 % driven by enhanced emissions from rising temperatures. Through sensitivity experiments, our study found a negligible response of monoterpene-derived SOA to changes in anthropogenic nitrogen oxide (NOx) emissions as a net effect of changes in multiple pathways. This study highlights the complex interplay between POA reduction and SOA growth, revealing notable OA trends in China and the varying roles of both anthropogenic and biogenic emissions.
- Article
(4142 KB) - Full-text XML
-
Supplement
(11690 KB) - BibTeX
- EndNote
PM2.5 (particulate matter less than or equal to 2.5 µm in diameter) is a standard air pollutant and attracts numerous research attention during the past decade. Ground level mass concentration of PM2.5 was found to gradually increase during 2000–2013, and it has decreased since 2014 in China (An et al., 2019; Lin et al., 2018; Ma et al., 2016). The trend of PM2.5 is believed to be driven primarily by China's emission control policies (Lu et al., 2020; Tong et al., 2020) and regional climate change over east Asia, which affects the dispersion conditions (Xu et al., 2022). Organic aerosol (OA) is an important component of PM2.5 as it can contribute up to 77 % of total fine-mode particles during haze pollution episode (An et al., 2019; Zhong et al., 2021). Despite its significance, there have been limited studies involving long-term continuous observations of OA. Unlike PM2.5, the observational gaps restrict our understanding of historical trends in OA, making it challenging to access air quality changes accurately and formulate effective environmental policies. While a few existing modeling studies have explored recent changes in OA in China, they often cover only limited time periods or specific years (Chen et al., 2024a; Zheng et al., 2023b).
OA consists of primary organic aerosols (POAs) and secondary organic aerosols (SOAs). POA is largely emitted from anthropogenic sources such as vehicle emissions, residential biofuel usage, and industrial activities, as well as biomass burning. Consequently, POA emissions are usually intensive (Fadel et al., 2021; Kanellopoulos et al., 2021) in urban areas (Liu et al., 2023), with their impact being primarily localized due to dependence on anthropogenic sources. Control of POA emissions has been effective in reducing PM2.5 concentrations, as observed in regions like the western United States (Pye et al., 2019). In China, studies have also reported a significant contribution of POA to PM2.5. Huang et al. (2019) discovered that POA emerges as the primary constituent during pollution episodes in the North China Plain region (Huang et al., 2019). Zheng et al. (2023a) found a decrease of 11.8 µg m−3 in OA concentrations at Beijing over 2005–2018, with most of the decrease coming from POA (Zheng et al., 2023a).
On the other hand, SOA is mainly produced through complex transformations of volatile organic compounds (VOCs) emitted from both anthropogenic and biogenic sources (Qin et al., 2018; Shrivastava et al., 2017; Zhang et al., 2007). These VOCs undergo multiple oxidation processes in the atmosphere, making SOA formation sensitive to chemical reactions, as well as meteorological conditions (An et al., 2019; Fan et al., 2020; Hu et al., 2017). In southern China, biogenic VOCs (BVOCs), primarily monoterpenes and isoprene, are significant contributors to SOA formation, particularly during summer due to warm temperatures and extensive vegetation (Guenther et al., 2012). Given that SOA formation is affected by both anthropogenic and natural factors, the response of OA to changing emission and climate conditions is likely nonlinear.
In the context of global warming, China has made considerable efforts to reduce anthropogenic emissions through a range of climate policies (Cai et al., 2017; Cui et al., 2020; Feng et al., 2019; Zheng et al., 2018). These measures have led to reductions in both POA and SOA concentrations. However, biogenic SOA (BSOA) also plays an important role in determining OA trends as it may change due to anthropogenic emission change. For example, studies indicate that BSOA produced from isoprene epoxydiols (IEPOX) has significantly decreased in the southeast United States due to reductions in anthropogenic SO2 emissions (Hoyle et al., 2011; Liu et al., 2021b; Qin et al., 2018; Shilling et al., 2013; Shrivastava et al., 2019), and a similar response was also reported in China (Dong et al., 2022). Likewise, monoterpene-derived SOA (SOAMT) is sensitive to nitrogen oxide (NOx) concentrations, and interactions between NOx and BVOCs can alter the oxidation pathways and ultimately affect BSOA formation (Jo et al., 2019; Xu et al., 2021; Zhang et al., 2018).
The abovementioned studies suggest that the long-term trends in OA might be a net result of the opposing trends in its subspecies, driven by multiple anthropogenic and natural factors. Nevertheless, existing modeling studies largely attribute OA trends to emission changes without detailed consideration of different subspecies. Moreover, the interactions between BVOCs and changing climate conditions complicate predictions of BSOA responses, hindering accurate forecasts of future OA trends. Diagnostically investigating OA trends and driving factors is therefore crucial to ensure a more comprehensive assessment of air quality changes. Given the limited availability of long-term observational data, this study employs a modeling tool along with available measurements from observation sites and a reanalysis dataset to explore the long-term trends of OA in China from 1990 to 2019, considering contributions from different subspecies. This analysis aims to support future air pollution control strategies by providing a better understanding of OA and its driving factors, thereby enabling more effective management of air quality in the face of ongoing climate change.
2.1 Model configuration
This study uses the Community Atmospheric Model version 6 with comprehensive tropospheric and stratospheric chemistry (CAM6-Chem) from the Community Earth System Model version 2.1.0 (CESM2.1.0). The gas-phase chemistry is represented by the Model for Ozone and Related chemical Tracers (MOZART) chemical mechanism (MOZART-TS2) including comprehensive isoprene and monoterpene chemistry (Schwantes et al., 2020). The aerosol model utilizes the four-mode version of the Modal Aerosol Module (MAM4) (Liu et al., 2016) and employs the volatility basis set (VBS) approach (Donahue et al., 2006; Hodzic et al., 2016) to simulate SOA formation. VOCs (isoprene, glyoxal, monoterpenes, sesquiterpene, benzene, toluene, lumped xylenes, intermediate volatile organic compounds, and semi-volatile organic compounds) are oxidized to produce five different types of volatile SOA gaseous precursors, with volatilities corresponding to effective saturation concentrations (C∗) of 0.01, 0.1, 1.0, 10.0, and 100.0 µg m−3 at 300 K, respectively (Tilmes et al., 2019). Heterogeneous production of isoprene-epoxydiol-derived SOA (SOAIE) is represented within the coupled Model for Simulating Aerosol Interactions and Chemistry (MOSAIC) (Jo et al., 2019, 2021; Zaveri et al., 2008, 2021) mechanism. The photolysis rate of monoterpene-derived SOA is updated based on our previous work (Liu et al., 2023). The aerosol wet removal scheme uses the Cloud Layers Unified By Binormals (CLUBB) scheme to unify shallow convective and stratiform clouds, coupled with the two-moment cloud microphysics scheme by Gettelman and Morrison (2015) for aerosol activation and removal (Gettelman and Morrison, 2015). For deep convective clouds, the scheme employs the parameterization by Zhang and McFarlane (1995) and relies on empirical parameters for estimating aerosol wet removal processes (Zhang and McFarlane, 1995).
We conducted long-term simulations covering the period from 1990 to 2019. The horizontal resolution of the simulations is set to 0.95° for latitude and 1.25° for longitude, with 32 vertical layers extending to approximately 40 km. The simulation has a spin-up time of 1 year and a relaxation time of 50 h to investigate surface OA trends. Natural emissions are calculated online using the Model of Emissions of Gases and Aerosol from Nature version 2.1 (MEGAN2.1), which is coupled to CESM (Emmons et al., 2020; Guenther et al., 2012). Anthropogenic emissions from 1990 to 2019 were sourced from the Multi-resolution Emission Inventory for China (MEIC; http://www.meicmodel.org, last access: 10 September 2024) (Li et al., 2017). Intermediate volatile organic compound (IVOC) and semi-volatile organic compound (SVOC) emissions were scaled based on POA emissions and non-methane VOC emissions (Chang et al., 2022; Tilmes et al., 2019), with specific formulas provided in the Supplement. We used the Modern-Era Retrospective analysis for Research and Applications (MERRA2) reanalysis data (Gelaro et al., 2017) for meteorological constraints.
To distinguish the effects of biogenic emissions and anthropogenic NOx emissions on SOAMT, we conducted additional sensitivity simulations by applying scaling factors for monoterpene and NOx emissions, respectively. Monoterpene emissions are higher in summer (Zhang et al., 2018), and 2013 saw the peak monoterpene emissions (Fig. 8a), while NOx concentrations reached a secondary peak (Fig. 8b). Therefore, we have selected July 2013 for sensitivity simulations to better capture SOAMT's response to both emission types. A benchmark simulation was conducted and denoted as 100nudging, which has the same configuration as the long-term simulation but with a 0.5 h relaxation time to minimize the impact of meteorological fields (Liu et al., 2021a; Tilmes et al., 2019). One type of sensitivity experiment was monoterpene emission experiments, and the two sets of experiments were named 0.5MTERP and 2MTERP. Their model configurations were the same as those of 100nudging, but the monoterpene emissions were, respectively, set to 0.5 and 2 times the 100nudging emissions. Their differences relative to 100nudging indicated the impact of monoterpene emissions disturbance on SOAMT formation. The other type of sensitivity experiment was for NOx emissions, and the two sets of experiments were named 0.5 NOx and 2 NOx. Their model configurations were also the same as those of 100nudging, but the NOx emissions were respectively set to 0.5 and 2 times the 100nudging emissions. Their differences relative to 100nudging indicated the impact of NOx emissions disturbance on SOAMT formation.
The model used in this study classifies monoterpenes into four categories: α-pinene, β-pinene, limonene, and myrcene. In the following sections, these will be collectively referred to as monoterpenes. In this study we constrained BSOA as the summary of SOAMT and SOAIE to focus on these two most important contributors. It shall be mentioned that isoprene and monoterpenes make the most contributions to BVOCs in China (Ding et al., 2016). For this reason, BVOC emissions in this study are constrained to the sum of isoprene and monoterpene emissions, allowing for a more focused analysis of the primary contributors. This study specifically focuses on the impact of aerosols derived from these compounds on OA trends.
2.2 Observations
We used observations from a number of different sources to evaluate the simulation performance of major aerosol species as well as key intermediates. We used ground-based measurements compiled by Miao et al. (2021) and Chen et al. (2024), which provided mean mass concentrations of surface OA, POA, and SOA in China from 2013 to 2019, along with the corresponding station locations (Chen et al., 2024a; Miao et al., 2021). A total of 151 measurements were included by removing duplicate values. We also used the high-resolution simulation dataset of PM2.5 composition over China (CAQRA-aerosols) provided by the National Natural Science Foundation Air Pollution Complex Major Research Plan Data Integration Project (project number: 92044303, https://www.capdatabase.cn, last access: 20 June 2024). The CAQRA-aerosols data were developed using emission inversion and high-resolution numerical simulation techniques (Kong et al., 2021). The CAQRA-aerosols data provided mass concentrations of organic carbon (OC) rather than OA (Kong et al., 2021). The root mean square error of OC on the monthly average concentration scale was 12.0 µg m−3, with a mean bias of 0.03 µg m−3 (0.17 %) (Kong et al., 2021). We used OA / OC ratios (1.19–3.04) to convert OC to OA concentrations (Malm and Hand, 2007) to facilitate comparison with simulation results. Since ratios vary with site and season, we used the mean value of 1.8 recommended by Malm and Hand (2007) as the conversion factor (Malm and Hand, 2007). To understand the model performance for simulating air pollutants, we also analyzed them by comparing them with the output values at the corresponding times and locations in the model. The 24 h average PM2.5 and ozone (O3) data from the China Environmental Monitoring Terminal (CEMT) National Urban Air Quality Real-Time Distribution Platform (NUAQRDP) (https://air.cnemc.cn:18007/, last access: 20 April 2024) were used to analyze changes in surface aerosol concentrations over the period 2014–2019.
Moreover, we used MODIS Level3 Collection 6.1 monthly aerosol optical depth (AOD) data as an indicator for column density of fine size aerosols. The 550 nm AOD data were retrieved using the combined Dark Target–Deep Blue algorithm (Levy et al., 2013), with monthly averaged data covering the years 2000–2019 at a spatial resolution of 1°. Performance of NOx simulation was also assessed using nitrogen dioxide (NO2) column concentrations monitored by the OMI (Ozone Monitoring Instrument) Level-3 data on board NASA's AURA satellite (https://disc.gsfc.nasa.gov/datasets?keywords=OMI&page=1, last access: 20 April 2024), with daily averaged data covering the period 2000–2019 at a spatial resolution of 0.25°.
3.1 Evaluation of model performance
Performance of the model was evaluated by comparing simulation results with ground-based observations, the CAQRA-aerosols dataset, and satellite products mentioned in Sect. 2. The results of the baseline simulation were compared with observations from multiple sources. Overall, the model can generally reproduce the spatial distributions and mass concentrations of OA and its components (Fig. S1 in the Supplement). By validation with observations from Miao et al. (2021) and Chen et al. (2024), the modeled normalized mean bias (NMB) of surface OA, POA, and SOA was −34.5 %, −7.4 %, and −64.8 %, respectively. Although there was a general underestimation of surface OA by the model, the simulations showed close agreement with measurements, with a coefficient of determination (R2) by 0.8. The capability of CAM-Chem in simulating OA over China was generally very consistent with other modeling studies. For example, Qin et al. (2018) utilized the Community Multiscale Air Quality (CMAQ) model (v5.0.2) to evaluate BSOA and reported an NMB of −70 % (Qin et al., 2018); Zheng et al. (2023b) employed the Weather Research and Forecasting model (WRF, v3.9)-CMAQ/2D-VBS modeling system and observed an NMB of −20 % for POA (Zheng et al., 2023b).
Our model-simulated spatial distribution of OA was in good agreement with the CAQRA-aerosols dataset. Both our model and the dataset indicated relatively higher OA concentrations in eastern China and lower concentrations in western China (Fig. S2 in the Supplement). The CAQRA-aerosols dataset showed that OA has strong seasonality in China, with the highest average mass concentrations in winter and the lowest in summer. Our model simulations reproduced this seasonal characteristic well (Fig. S3 in the Supplement). The results of both the model and the CAQRA-aerosols dataset indicated a decreasing trend in OA concentrations in China (Fig. 1b). Notably, there was good spatial consistency, with a significant decrease observed in eastern China, particularly in the North China Plain region (Fig. 1c and f). This decreasing trend was especially pronounced in Beijing. Given that only the Beijing site had continuous ground-based observations over multiple years from Miao et al. (2021) and Chen et al. (2024), we further evaluated the long-term simulation results for this site. The simulation values showed good agreement with the observed values, reflecting a consistent trend over the years (Fig. 1e).
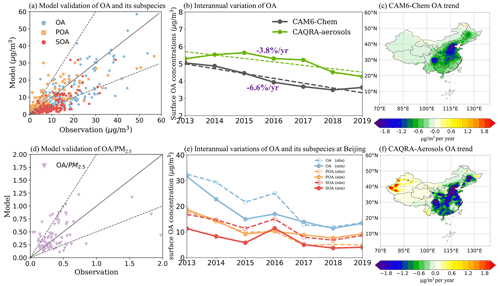
Figure 1(a) Validation of modeled organic aerosols (OAs), primary organic aerosols (POAs), and secondary organic aerosols (SOAs) based on ground-based measurements compiled by Miao et al. (2021) and Chen et al. (2024) (unit: µg m−3). (b) The 2013–2019 interannual variation of average surface organic aerosol (OA) concentrations in CAM6-Chem (dark gray) and the CAQRA-aerosols dataset (green) (unit: µg m−3). The trend lines (dotted line) are based on linear regression fitting. (c) The 2013–2019 CAM6-Chem modeled annual long-term trend of surface OA concentrations (unit: ). The trend is calculated by linear regression on an annual scale over 1990–2019. (d) Validation of modeled OA / PM2.5 based on OA measurements compiled by Miao et al. (2021) and Chen et al. (2024) and PM2.5 observations from the China Environmental Monitoring Terminal National Urban Air Quality Real-time Distribution Platform. (e) Interannual variation of average surface OA, POA, and SOA concentrations (µg m−3) at the Beijing site from 2013–2019 in CAM6-Chem (solid line; sim) and ground-based measurements compiled by Miao et al. (2021) and Chen et al. (2024) (dashed line; obs). (f) The 2013–2019 annual long-term trend of surface OA concentrations (unit: ) in the CAQRA-aerosols dataset. The trend is calculated by linear regression on an annual scale over 1990–2019.
In terms of PM2.5 simulation, the model slightly underestimated observation by −19.2 % (Fig. S6a in the Supplement). This might be at least partially because of coarse model grid resolution, while CEMT observational sites are mostly within urban area. We then compared the simulation with observation for the contribution of OA to PM2.5, showing good performance with an NMB of 5.6 %. The observed OA / PM2.5 ratio was calculated by paring OA measurements reported in Miao et al. (2021) and Chen et al. (2024) with CEMT PM2.5 observations during the same period, which fall into the same CAM6-Chem model grid. Due to lack of synergic collected observations of aerosol subspecies, we did not validate the contributions from other subspecies but focus on OA only. The moderate underestimations of OA and PM2.5 but relatively better performance for simulating OA / PM2.5 ratio suggested that although the model may have deficiencies in reproducing the absolute concentrations of OA, it was able to capture the contribution from OA correctly. It also implied that there might be systematic bias within the modeling system affecting underestimations of aerosol mass concentrations such as coarse grid resolution. Our recent study thoroughly evaluated the CAM-Chem simulation of PM2.5 in China and reported that a finer grid (∼0.25°) would substantially lower the modeling bias, especially over complex terrains during haze episodes (Yue et al., 2023). The fine grid version was not employed in this study as it is not compatible with the MOSAIC module yet, and we consider the heterogeneous chemistry of SOA and thermodynamic equilibrium of nitrate represented by MOSAIC as more important for this study to focus on the long-term trend of OA and subspecies.
3.2 Trend and attribution of surface OA
In this section we first briefly introduced the general characteristics (e.g., concentrations, main subspecies, spatial distribution) of simulated OA, and then investigated the overall change in OA through the study period.
Spatial distributions of annual average concentrations of OA and related subspecies are presented in Fig. 2. The concentrations of OA show prominent regional differences, with its high value () areas concentrated in the North China Plain and Sichuan Basin area, while the vast remote areas in western China such as Tibet and Xinjiang show lower OA concentrations (). Spatial distribution of total OA at national scale is predominantly determined by POA, as shown in Fig. 2b. In the densely populated North China Plain urban area, contribution of POA to OA can reach up to 75 %. SOA was relatively lower in concentration (Fig. 2c) and was found to have a consistent spatial distribution pattern like POA, indicating a dominate contribution from anthropogenic-VOC-derived SOA (ASOA) to total SOA. Spatial distributions of POA and SOA are generally well consistent with anthropogenic POA and VOC emissions which also concentrated over urban clusters as shown in Fig. 2d and e, respectively. Pearl River Delta is found to be unique as it has relatively low POA emissions but high anthropogenic VOC emission, probably due to vehicle exhausts (Lee et al., 2002; Liu et al., 2024). It is also important to note that southern China has a non-negligible level of SOA where biogenically produced BSOA was found to play an important role due to extensive vegetation coverages with excessive BVOC emissions as shown in Fig. 2f. For example, contributions of BSOA to OA can reach up to 27 % over the Yunnan–Guizhou Plateau for climatological summer averages.
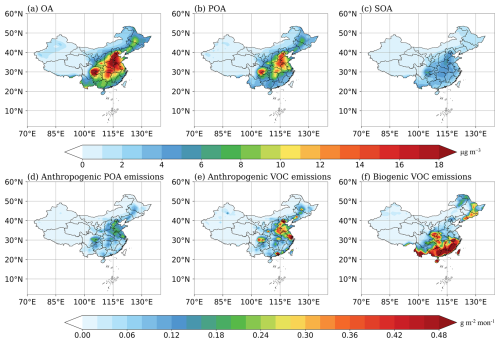
Figure 2The 1990–2019 annual average of surface organic aerosols (OAs; a; unit: µg m−3), primary organic aerosols (POAs; b; unit: µg m−3), secondary organic aerosols (SOAs; c; unit: µg m−3), anthropogenic POA emissions (d; unit: g m−2 per month), anthropogenic volatile organic compound emissions (e; unit: g m−2 per month), and biogenic volatile organic compound emissions (f; unit: g m−2 per month) concentrations.
General trends of OA and subspecies are demonstrated in Fig. 3, with more details such as time series plots (Figs. S7 and S13 in the Supplement) and trends at the seasonal scale (Figs. S16 and S17 in the Supplement) in the Supplement. All trends are calculated by linear regression on an annual scale over 1990–2019. In general, trend of surface OA concentrations showed a regional difference as decreasing over eastern coastal provinces and increasing over the western inland provinces. For example, the annual average surface OA concentrations showed a significant decreasing trend over Yangtze River Delta by around −1.4 µg m−3 per decade (−13.8 % per decade), while in the Sichuan Basin area, surface OA shows an increasing trend by 0.4 µg m−3 per decade (7.3 % per decade). Trends in different seasons were consistent with the annual trend mentioned above but with stronger changes, as the increase in OA over the Sichuan Basin area in summer was more prominent, and so was the decrease over Yangtze River Delta in winter (Fig. S16).
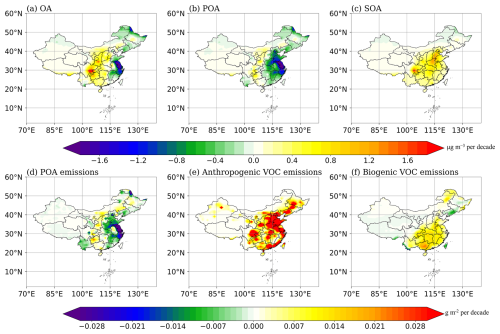
Figure 3The 1990–2019 annual average long-term trend of surface organic aerosols (OAs; a; unit: µg m−3 per decade), primary organic aerosols (POAs; b; unit: µg m−3 per decade), secondary organic aerosols (SOAs; c; unit: µg m−3 per decade), primary organic aerosols emissions (d; unit: g m−2 per decade), anthropogenic volatile organic compound emissions (e; unit: g m−2 per decade), and biogenic volatile organic compound emissions (f; unit: g m−2 per decade).
The overall OA trend is the net result of the long-term changes in its subspecies POA (Fig. 3b) and SOA (Fig. 3c). POA generally showed consistent trends with total OA which decreased in the east by up to −8.9 % per decade (−0.24 µg m−3 per decade) and increased in the west by up to 13.2 % per decade (0.08 µg m−3 per decade). SOA showed a clear upward trend throughout the country by 10.8 % per decade (0.16 µg m−3 per decade). The spatial distributions of the long-term trends of POA and SOA were well consistent with the pattern of changes in emissions as shown in Fig. 3d–f.
It is interesting to note that the most significant changes in OA were not over the regions with high OA concentrations. The different patterns of changes in POA and SOA led to various changes of OA over three typical urban cluster areas including the North China Plain, Yangtze River Delta, and Sichuan Basin area. The North China Plain has the highest level of total OA but a minor trend, probably due to the net effect of a minor decrease in POA and an increase in ASOA, both driven by changes in anthropogenic emissions. The Yangtze River Delta has the lowest level of total OA concentrations but the most significant decreasing trend, primarily due to the reduction in POA. Zheng et al. (2023b) suggested that anthropogenic POA emissions were reduced by −65.7 % in the Yangtze River Delta over 2005–2019 as a result of air quality management (Zheng et al., 2023b). The Sichuan Basin area has a relatively high level of OA and also the most significant increasing trend. Changes in emissions suggested that the enhancement was mainly driven by excessive SOA from anthropogenic precursors, as shown in Fig. 3e. A more detailed discussion of anthropogenic and biogenic contributions to this increased SOA over the Sichuan Basin area will be provided in later sections.
Given China's vast area, we used the probability density function (PDF) to analyze the distribution of OA and its components across different concentration ranges for all grid points, complementing the overall trend analysis. Figure 4c shows that the PDF for SOA concentrations flattened out, with higher probability density values, leading to a significant national increase in SOA levels. In contrast, POA trends moved in the opposite direction (Fig. 4b). While western China's vast area may impact arithmetic averages, PDF analysis still shows a significant rise in SOA levels, which is consistent with the annual variations calculated using arithmetic averages. To better understand the impact of low OA concentration areas like Xinjiang, Tibet, and Qinghai (Fig. 2a), we also analyzed trends while excluding these regions (Table S2 in the Supplement). The calculated trends indicate that the impact of low concentration regions is limited, while the overall trend is dominated by changes in high concentration areas.
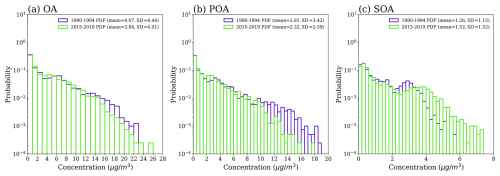
Figure 4Probability density function (PDF) distributions of simulated 5-year average concentrations of surface organic aerosols (OAs; a), primary organic aerosols (POAs; b), and secondary organic aerosols (SOAs; c) for the periods 1990–1994 (purple) and 2015–2019 (green), along with the annual mean values and standard deviations for each species in different time periods.
3.2.1 Attribution of POA trend
We analyzed the decreasing trend of POA and found that both anthropogenic emissions and biomass burning were responsible for it. Anthropogenic emissions contributed 87.4 % and dominated the long-term trend, while biomass burning substantially affected the interannual variation (IAV) although it only contributed by 12.5 % emission on average. As shown in Figs. 5a and S7a, variations in biomass burning are responsible for the large IAV of POA concentrations. Biomass burning usually has a strong seasonality, so we analyzed its contribution across different seasons (Fig. 5b). We found that biomass burning in China was most intensive in spring as the relative contribution to total POA emissions was 21.4 %. Previous studies have also reported the significant contributions from biomass burning in China are mainly due to agricultural activities. For example, extreme crop residue burning events that occurred in northeast China and northwest China in 2003 greatly deteriorated air quality (Wang et al., 2020; Zhuang et al., 2018).
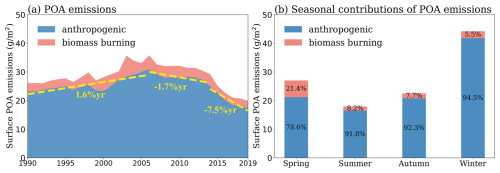
Figure 5(a) The 1990–2019 interannual variation of average surface anthropogenic (blue) and biomass burning (pink) primary organic aerosol (POA) emissions (unit: g m−2 per month). The dashed yellow line represents the linear regression fit for anthropogenic POA emissions over the periods 1990–2006, 2006–2014, and 2014–2019. (b) Seasonal average surface anthropogenic (blue) and biomass burning (pink) POA emissions (unit: g m−2 per month) and the relative contribution of different seasons (%).
As the largest source of POA, anthropogenic emissions of POA decreased by −7.8 % from 1990 to 2019; this is in good agreement with the change in POA concentration. As mentioned in Sect. 3.3, the change in POA concentration was non-monotonic, which can be explained by the varied changes in anthropogenic POA emissions, as shown in Fig. 5a. Anthropogenic POA emissions were primarily from residential usage of coal and biofuel (78 %), followed by a minor contribution from industry (18 %) (Zheng et al., 2018). It increased by 1.6 % per year over 1990–2006 along with the expansion of population and Gross Domestic Product (GDP) (Liu et al., 2022; Xing et al., 2022) and then started to gradually decrease by −1.7 % per year over 2006–2013 as a result of the emission control policies for industrial sources such as manufacturing boilers (Zheng et al., 2018). Since 2014, China has started to strictly implement more efficient and national emission control policies which greatly lower the anthropogenic emissions. Policies such as the “Action Plan for Air Pollution Prevention and Control” have been reported to play a pivotal role in emission reduction by imposing restrictions on coal use and implementing advanced emission control technologies (Cai et al., 2017; Cui et al., 2020; Feng et al., 2019; Maji et al., 2020). As a result, a rapid reduction in anthropogenic POA emissions started from 2014, which is attributed to broadly replace residential usage of coal and biofuel with electricity and gas. These policies significantly lowered the total POA emissions by −42.8 % over 2014–2019 (Zheng et al., 2018). Since residential POA emissions were mainly for cooking and heating purposes, we also analyzed the seasonality of anthropogenic POA emissions and found that winter indeed showed the greatest contribution (Fig. 5b). In summary, the changes in POA concentrations and POA emissions during the past 30 years in China could be well explained by the implementation of a series of air pollution management activities.
3.2.2 Attribution of SOA trend
SOA is produced from both anthropogenic and biogenic emissions of VOCs. ASOA contributed roughly 74.3 % to total SOA during the study period, with high concentrations found over urban areas such as the North China Plain, Yangtze River Delta, Sichuan Basin area, and Pearl River Delta. Anthropogenic VOCs mainly consist of aromatics (AVOCs), semi-volatile organic compounds (SVOCs), and intermediate-volatile organic compounds (IVOCs). In contrast to POA, which primarily comes from residential sector, emission sources of anthropogenic VOCs are quite complex and have shown different changes in China over the past decades. AVOC emissions are mainly from industry (21 %), solvent usage (25 %), and transportation (22 %) (Zheng et al., 2018). Both industry and solvent usage have gradually increased over the past decades and have subsequently led to enhanced AVOC emission, although transportation has slightly decreased. Anthropogenic AVOCs increased by 115.8 % from 1990 to 2019, with the most significant enhancement over urban clusters mentioned above. Although a prominent decrease in AVOCs was observed over 2014–2019, it remains the largest contributor of anthropogenic VOCs, as shown in Fig. 6b. IVOCs increased throughout the study period (Fig. 7b), and their importance in SOA formation grew over time (Fig. S10 in the Supplement). However, their relative contribution to total anthropogenic VOC emissions remained minor. SVOCs primarily originated from the same sources as POA (Chang et al., 2022). Although SVOCs dominated the formation of ASOA in 1990, accounting for 51.9 % of the total ASOA (Fig. S10c), their concentrations decreased after 2006 due to reduced emissions (Fig. 7b). Although SVOC emissions declined, the rise in AVOC emissions not only offset this reduction but also emerged as the primary driver of the sustained increase in ASOA.
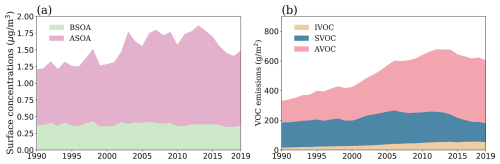
Figure 6(a) Interannual variations in modeled average surface concentrations of secondary organic aerosols from anthropogenic sources (ASOA) and biogenic sources (BSOA) (unit: µg m−3). (b) Interannual variations in emissions of aromatics (AVOCs), semi-volatile organic compounds (SVOCs), and intermediate-volatile organic compounds (IVOCs) (unit: g m−2 per month).
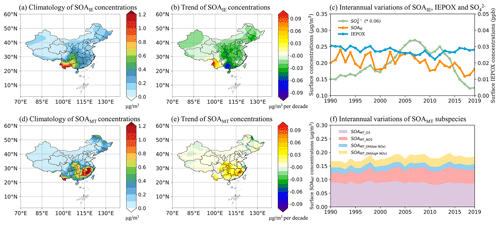
Figure 7(a) The 1990–2019 annual average of surface SOAIE (isoprene-epoxydiol-derived secondary organic aerosols; unit: µg m−3) concentrations. (b) The 1990–2019 annual average long-term trend of surface SOAIE (unit: µg m−3 per decade) concentrations. (c) Interannual variations of average surface SOAIE (left y axis), IEPOX (isoprene epoxydiol; right y axis), and (scaled by a factor of 0.06; left y axis) concentrations during 1990–2019. (d) The 1990–2019 annual average of surface SOAMT (monoterpene-derived secondary organic aerosols; unit: µg m−3) concentrations. (e) The 1990–2019 annual average long-term trend of surface SOAMT (unit: µg m−3 per decade) concentrations. (f) Interannual variations of average surface SOAMT subspecies concentrations during 1990–2019 (unit: µg m−3).
BSOA was found to play an important role especially during summer over south China, and recent studies also revealed that formation of BSOA is closely affected by anthropogenic pollutants such as sulfate and NOx (Liu et al., 2021b; Pye et al., 2013). To understand the contribution of BSOA to the long-term trend of total OA over China, we further analyzed the trend of SOAIE and SOAMT, which are the two main components of BSOA. During 1990–2019, SOAIE concentrations decreased by −0.01 µg m−3 per decade (−6.3 % per decade). The reduction in SOAIE appears to be primarily driven by the combined effect of IEPOX and availability, as the formation mechanism of SOAIE fundamentally relies on the heterogeneous uptake of IEPOX onto sulfate aerosols (Dong et al., 2022; Jo et al., 2019, 2021). On the one hand, anthropogenic emission of SO2 has been greatly lowered by the enforcement of the Energy Conservation and Emission Reduction (ECER), and a corresponding decrease in the concentrations of has been observed (Fig. S18d in the Supplement). This is responsible for the decrease in SOAIE since 2006. On the other hand, the precursor IEPOX showed an opposite decreasing trend (Fig. S18e and f) as compared to anthropogenic emission enhancement of NOx before 2011, since NOx would affect the oxidation pathway of isoprene. We find a nationwide reduction in SOAIE over the study period, with an exceptional increase in Yunnan province. The increase in SOAIE over Yunnan Province was due to the combined effect of enhancement in vegetation coverage resulting from ecosystem projects in China (Hua et al., 2018) and the increase in transported from peninsular southeast Asia (e.g., Vietnam, Thailand) resulted from the development of local industries (Dalsøren et al., 2009; Grandey et al., 2018). In summary, our results suggested that the change in SOAIE is affected by , which is consistent with previous studies using different models (Liu et al., 2021b; Qin et al., 2018).
Monoterpene-derived SOA was sensitive to both NOx level and biogenic emission, so we first analyzed the trend of SOAMT and its subspecies and then employed sensitivity simulations to distinguish the influences from chemistry and emission. Monoterpenes can be oxidized by different oxidants to form SOAMT, so the change in anthropogenic NOx emission may affect the trend of SOAMT by altering the oxidation pathways. In the long-term simulation we found that the net effect of different oxidation pathways led to an overall increasing trend of SOAMT, as shown in Fig. 7e and f. We defined four subspecies of SOAMT: SOAMT_O3, SOAMT_NO3, SOAMT_OH(low NOx), and SOAMT_OH(high NOx), which are produced from O3 oxidation, nitrate radical (NO3) oxidation, hydroxyl radical (OH) oxidation under low NOx conditions and OH oxidation under high NOx conditions, respectively. The main contributor to SOAMT concentration in China was SOAMT_O3 (34.6 %, Fig. S19a in the Supplement), followed by SOAMT_NO3 (31.2 %, Fig. S19c), SOAMT_OH(high NOx) (18.1 %, Fig. S19g), and SOAMT_OH(low NOx) (16.1 %, Fig. S19e). The different SOAMT components had regionally different or even opposite long-term trends. SOAMT_NO3 showed a regionally consistent and significant increasing trend (Fig. S19d) in line with SOAMT (4.1 % per decade) (Fig. 7e). A slight but solid increasing trend in SOAMT_OH(high NOx) is shown in Fig. S19h, which together with the enhancement of SOAMT_NO3 concentrations dominates the increasing trend in total SOAMT. SOAMT_O3 (Fig. S19b) and SOAMT_OH(low NOx) (Fig. S19f) had similar trends, but the change in SOAMT_OH(low NOx) was relatively smaller.
The decreasing trend in SOAMT_O3 and SOAMT_OH(low NOx) offset the enhancement in the other two SOAMT components. The different trends of SOAMT components were affected by the changes in oxidants, which were mainly determined by changes in anthropogenic NOx emissions (Fig. 8b). The surface concentrations of NOx and NO3 increased over 1990–2019, while O3 and OH increased relatively slowly, which led to differences in the variation of the SOAMT components during the study period. The total SOAMT has increased since 1990 primarily due to increased monoterpene emissions because of both a higher temperature (Fig. 8a) and also enhanced vegetation coverage (Guenther et al., 2012; Fu and Liao, 2014). Related studies suggested that vegetation coverage has increased over China in the past due to a series of ecological restoration and conservation policies (Guo et al., 2022), such as the Grain for Green Program (Yin et al., 2018) and Urban Ecological Civilization Construction. These policies have effectively promoted vegetation recovery and expansion, while also driving the continuous increase in urban greening areas. These changes collectively contributed to the rising trend in BVOC emissions and subsequent changes in SOA concentrations.
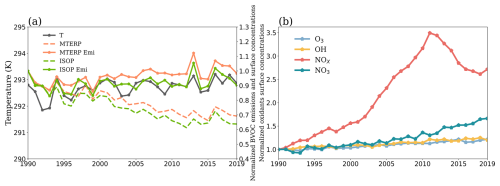
Figure 8(a) The 1990–2019 JJA time series of surface temperature (solid dark-gray line; left y axis; unit: K), relative changing ratio of surface concentrations for monoterpenes (dashed orange line; right y axis; MTERP), isoprene (dashed green line; right y axis; ISOP), monoterpene emissions (solid orange line; right y axis; MTERP Emi), and isoprene emissions (solid green line; right y axis; ISOP Emi). (b) The 1990–2019 JJA time series of relative changing ratio of surface concentrations for nitrogen oxides (NOx; red), ozone (O3; pale blue), the hydroxyl radical (OH; yellow), and the nitrate radical (NO3; cyan). All relative changing ratios are calculated as the concentration in each year divided by the concentration in 1990.
Since anthropogenic NOx emission change may have a nonlinear effect on SOAMT, we applied sensitivity simulations by perturbing NOx emissions and biogenic emissions respectively to quantify their contributions. We found that the response of SOAMT to NOx emission change was almost negligible when NOx emissions rose to twice the 100nudging or fell to half the 100nudging in July 2013 (Fig. 9b), although major atmospheric oxidizers such as O3, OH, NO3, and NOx showed significant changes (Fig. S22 in the Supplement). For example, the increase in NOx emissions drove SOAMT_OH(high NOx) and SOAMT_NO3 to increase, but meanwhile, the effect was offset by decreases in SOAMT_OH(low NOx) and SOAMT_O3. Similarly, under conditions of NOx emission reduction, the SOAMT response was small due to the offsetting relative changes in the SOAMT components. Our simulation results suggested that anthropogenic emission change has a very limited net effect on SOAMT over the study period.
Through sensitivity simulations by perturbing monoterpene emission, however, we found that the responses of SOAMT and its components were almost linear. When the monoterpene emissions increased to twice those of the 100nudging in July 2013, the concentrations of SOAMT and its components on the surface of China also increased by 2 times. Meanwhile, when the monoterpene emission decreased to half of the 100nudging, the concentrations of SOAMT and its components decreased by half too (Fig. 9a). Changes in monoterpene emissions showed a minor impact on atmospheric oxidants (Fig. S25 in the Supplement). In summary, we found that anthropogenic NOx emission change has a very limited impact on SOAMT, while enhanced monoterpene emissions due to a warmer surface temperature dominate the increasing trend over 1990–2019.
In this study we applied the CAM-chem model along with ground-based measurements and the CAQRA-aerosols dataset to investigate the long-term trend of OA in 1990–2019 in China. A slight increase in total OA by 1.8 % per decade was found to be a net effect of decrease in POA by −0.08 µg m−3 per decade (−2.7 % per decade) and enhancement of SOA by 0.16 µg m−3 per decade (10.8 % per decade). There are significant regional differences in the change trend of OA, which generally decreased in the east (e.g., Yangtze River Delta) and increased in the west (e.g., Sichuan Basin), and this trend was more significant in winter, indicating the dominant contribution from primary anthropogenic emission of POA.
Further analyzing the causes of POA and SOA trends, we found that the main factors affecting POA trends are biomass burning and anthropogenic emissions. Anthropogenic emissions accounted for 87.4 % and dominated the long-term trend of POA, but biomass burning dominated the IAV. We found that anthropogenic VOCs made a major contribution to total SOA by 74.3 %, and the spatiotemporal characteristics were well consistent with POA. For SOA produced from BVOCs, we found in the simulation that BSOA plays an important role over South China, especially in summer, with contribution up to 47.1 %. Total BSOA decreased by −4.1 % over the study period. Isoprene-derived SOAIE is greatly affected by heterogeneous reactions catalyzed by sulfate, which decreased rapidly from 2006 and resulted in a decline by −18.8 % over 1990–2019. On the other hand, monoterpene-derived SOAMT increased by 12.3 %. We found through sensitivity experiments that anthropogenic NOx emissions change had an almost negligible impact on total SOAMT, although the contributions from different oxidation pathways changed slightly. The trend in SOAMT was dominated by increased biological emissions due to a warmer climate.
Our study revealed the change in total OA in China during the past 30 years and the contribution from various driving factors. Anthropogenic emission, biomass burning emission, and biogenic emission all showed important and unique impacts on the long-term trend of OA, indicating that future air quality management would be recommended to take a comprehensive consideration of the abovementioned sources. In particular, we found that anthropogenic contributions to both POA and SOA substantially decreased from 2014, while biogenic contribution has the potential to increase under a warming climate. BSOA plays a minor role on a national scale but may have a significant contribution over densely vegetated southern areas of China during summer; most of it is hardly affected by anthropogenic emissions but is enhanced by a warming climate. This implies that future research may need to pay more attention to biogenic sources. In addition, it should be noticed that our current model may have deficiencies in terms of both emission inventory and chemical mechanism for simulating SOA. For example, the current model only considers the heterogeneous production of IEPOX-derived SOA, but recent studies show that isoprene may also produce SOA through intermediate oxidation gas-phase products hydroxymethylmethyl-α-lactone (HMML) and methacrylic acid epoxide (MAE) (He et al., 2018; Zhang et al., 2023), which are not considered in the current model. This may also be partially responsible for the underestimation of SOA mentioned earlier. Therefore, continuous development of the SOA chemical mechanisms is recommended to improve the simulation capability of the model. And, in addition, more detailed observations of OA components are needed to further investigate the interactions between biological and anthropogenic sources.
The long-term CAM6-Chem model OA and its subspecies data presented in this paper are available on Zenodo (https://doi.org/10.5281/zenodo.14742102, Zhang, 2025). Ground-based measurements for OA, POA, and SOA were obtained from the supplementary materials of published articles by Miao et al. (2021) and Chen et al. (2024). The publicly available high-resolution simulation dataset of PM2.5 composition over China (CAQRA-aerosols) (Kong, 2022) was obtained from the Data Integration Project of the National Natural Science Foundation's Air Pollution Complex Major Research Plan (project number: 92044303) via the China Air Pollution Data Center (CAPDC) (https://www.capdatabase.cn/resourceDownload/detail?id=207&type=2). The 24 h average PM2.5 and O3 data can be accessed from the China Environmental Monitoring Terminal (CEMT) National Urban Air Quality Real-Time Distribution Platform (NUAQRDP) (China Environmental Monitoring Terminal, 2022) (https://air.cnemc.cn:18007/). MODIS AOD data are available at https://doi.org/10.5067/MODIS/MOD08_M3.006 (Platnick et al., 2015). NO2 column concentration data can be accessed from the OMI (Ozone Monitoring Instrument) Level-3 dataset (Lamsal et al., 2022) on NASA's AURA satellite platform (https://doi.org/10.5067/MEASURES/MINDS/DATA304).
The supplement related to this article is available online at https://doi.org/10.5194/acp-25-3857-2025-supplement.
MW and XD designed the study. WZ performed the data analysis, produced the figures, and wrote the manuscript draft. YL and MY contributed to the model simulations. All the authors contributed to the discussion and editing of the manuscript.
At least one of the (co-)authors is a member of the editorial board of Atmospheric Chemistry and Physics. The peer-review process was guided by an independent editor, and the authors also have no other competing interests to declare.
Publisher's note: Copernicus Publications remains neutral with regard to jurisdictional claims made in the text, published maps, institutional affiliations, or any other geographical representation in this paper. While Copernicus Publications makes every effort to include appropriate place names, the final responsibility lies with the authors.
We greatly appreciate the High Performance Computing Center (HPCC) of Nanjing University for providing the computational resources used in this work. We also thank the Tsinghua University group for providing the MEIC data used in our simulations. The CESM project is supported primarily by the US National Science Foundation. We thank all the scientists, software engineers, and administrators who contributed to the development of CESM2. The authors are grateful for the outstanding efforts of two anonymous referees who provided challenging and insightful comments that improved this article.
This research has been supported by the National Natural Science Foundation of China (grant nos. 41925023 and 42361144711) and the Fundamental Research Funds for the Central Universities – CEMAC “GeoX” Interdisciplinary Program (grant no. 2024ZD05) of the Frontiers Science Center for Critical Earth Material Cycling, Nanjing University.
This paper was edited by Shaocheng Xie and reviewed by two anonymous referees.
An, Z., Huang, R.-J., Zhang, R., Tie, X., Li, G., Cao, J., Zhou, W., Shi, Z., Han, Y., Gu, Z., and Ji, Y.: Severe haze in northern China: A synergy of anthropogenic emissions and atmospheric processes, P. Natl. Acad. Sci. USA, 116, 8657–8666, https://doi.org/10.1073/pnas.1900125116, 2019.
Cai, S., Wang, Y., Zhao, B., Wang, S., Chang, X., and Hao, J.: The impact of the “Air Pollution Prevention and Control Action Plan” on PM2.5 concentrations in Jing-Jin-Ji region during 2012–2020, Sci. Total Environ., 580, 197–209, https://doi.org/10.1016/j.scitotenv.2016.11.188, 2017.
Chang, X., Zhao, B., Zheng, H., Wang, S., Cai, S., Guo, F., Gui, P., Huang, G., Wu, D., Han, L., Xing, J., Man, H., Hu, R., Liang, C., Xu, Q., Qiu, X., Ding, D., Liu, K., Han, R., Robinson, A. L., and Donahue, N. M.: Full-volatility emission framework corrects missing and underestimated secondary organic aerosol sources, One Earth, 5, 403–412, https://doi.org/10.1016/j.oneear.2022.03.015, 2022.
Chen, Q., Miao, R., Geng, G., Shrivastava, M., Dao, X., Xu, B., Sun, J., Zhang, X., Liu, M., Tang, G., Tang, Q., Hu, H., Huang, R.-J., Wang, H., Zheng, Y., Qin, Y., Guo, S., Hu, M., and Zhu, T.: Widespread 2013–2020 decreases and reduction challenges of organic aerosol in China, Nat. Commun., 15, 4465, https://doi.org/10.1038/s41467-024-48902-0, 2024.
China Environmental Monitoring Terminal: 24 h average PM2.5 and O3 data, China Environmental Monitoring Terminal (CEMT) National Urban Air Quality Real-Time Distribution Platform [data set], https://air.cnemc.cn:18007/ (last access: 20 April 2024), 2022.
Cui, L., Zhou, J., Peng, X., Ruan, S., and Zhang, Y.: Analyses of air pollution control measures and co-benefits in the heavily air-polluted Jinan city of China, 2013–2017, Sci. Rep.-UK, 10, 5423, https://doi.org/10.1038/s41598-020-62475-0, 2020.
Dalsøren, S. B., Isaksen, I. S. A., Li, L., and Richter, A.: Effect of emission changes in Southeast Asia on global hydroxyl and methane lifetime, Tellus B, 61, 588, https://doi.org/10.1111/j.1600-0889.2009.00429.x, 2009.
Ding, X., Zhang, Y., He, Q., Yu, Q., Shen, R., Zhang, Y., Zhang, Z., Lyu, S., Hu, Q., Wang, Y., Li, L., Song, W., and Wang, X.: Spatial and seasonal variations of secondary organic aerosol from terpenoids over China, J. Geophys. Res.-Atmos., 121, 14661–14678, https://doi.org/10.1002/2016JD025467, 2016.
Donahue, N. M., Robinson, A. L., Stanier, C. O., and Pandis, S. N.: Coupled Partitioning, Dilution, and Chemical Aging of Semivolatile Organics, Environ. Sci. Technol., 40, 2635–2643, https://doi.org/10.1021/es052297c, 2006.
Dong, X., Liu, Y., Li, X., Yue, M., Liu, Y., Ma, Z., Zheng, H., Huang, R., and Wang, M.: Modeling Analysis of Biogenic Secondary Organic Aerosol Dependence on Anthropogenic Emissions in China, Environ. Sci. Tech. Let., 9, 286–292, https://doi.org/10.1021/acs.estlett.2c00104, 2022.
Emmons, L. K., Schwantes, R. H., Orlando, J. J., Tyndall, G., Kinnison, D., Lamarque, J., Marsh, D., Mills, M. J., Tilmes, S., Bardeen, C., Buchholz, R. R., Conley, A., Gettelman, A., Garcia, R., Simpson, I., Blake, D. R., Meinardi, S., and Pétron, G.: The Chemistry Mechanism in the Community Earth System Model Version 2 (CESM2), J. Adv. Model. Earth Sy., 12, e2019MS001882, https://doi.org/10.1029/2019MS001882, 2020.
Fadel, M., Ledoux, F., Farhat, M., Kfoury, A., Courcot, D., and Afif, C.: PM2.5 characterization of primary and secondary organic aerosols in two urban-industrial areas in the East Mediterranean, J. Environ. Sci., 101, 98–116, https://doi.org/10.1016/j.jes.2020.07.030, 2021.
Fan, Y., Liu, C.-Q., Li, L., Ren, L., Ren, H., Zhang, Z., Li, Q., Wang, S., Hu, W., Deng, J., Wu, L., Zhong, S., Zhao, Y., Pavuluri, C. M., Li, X., Pan, X., Sun, Y., Wang, Z., Kawamura, K., Shi, Z., and Fu, P.: Large contributions of biogenic and anthropogenic sources to fine organic aerosols in Tianjin, North China, Atmos. Chem. Phys., 20, 117–137, https://doi.org/10.5194/acp-20-117-2020, 2020.
Feng, Y., Ning, M., Lei, Y., Sun, Y., Liu, W., and Wang, J.: Defending blue sky in China: Effectiveness of the “Air Pollution Prevention and Control Action Plan” on air quality improvements from 2013 to 2017, J. Environ. Manage., 252, 109603, https://doi.org/10.1016/j.jenvman.2019.109603, 2019.
Fu, Y. and Liao, H.: Impacts of land use and land cover changes on biogenic emissions of volatile organic compounds in China from the late 1980s to the mid-2000s: implications for tropospheric ozone and secondary organic aerosol, Tellus B, 66, 24987, https://doi.org/10.3402/tellusb.v66.24987, 2014.
Gelaro, R., McCarty, W., Suárez, M. J., Todling, R., Molod, A., Takacs, L., Randles, C. A., Darmenov, A., Bosilovich, M. G., Reichle, R., Wargan, K., Coy, L., Cullather, R., Draper, C., Akella, S., Buchard, V., Conaty, A., Da Silva, A. M., Gu, W., Kim, G.-K., Koster, R., Lucchesi, R., Merkova, D., Nielsen, J. E., Partyka, G., Pawson, S., Putman, W., Rienecker, M., Schubert, S. D., Sienkiewicz, M., and Zhao, B.: The Modern-Era Retrospective Analysis for Research and Applications, Version 2 (MERRA-2), J. Climate 30, 5419–5454, https://doi.org/10.1175/JCLI-D-16-0758.1, 2017.
Gettelman, A. and Morrison, H.: Advanced Two-Moment Bulk Microphysics for Global Models. Part I: Off-Line Tests and Comparison with Other Schemes, J. Climate 28, 1268–1287, https://doi.org/10.1175/JCLI-D-14-00102.1, 2015.
Grandey, B. S., Yeo, L. K., Lee, H., and Wang, C.: The Equilibrium Climate Response to Sulfur Dioxide and Carbonaceous Aerosol Emissions From East and Southeast Asia, Geophys. Res. Lett., 45, 11318–11325, https://doi.org/10.1029/2018GL080127, 2018.
Guenther, A. B., Jiang, X., Heald, C. L., Sakulyanontvittaya, T., Duhl, T., Emmons, L. K., and Wang, X.: The Model of Emissions of Gases and Aerosols from Nature version 2.1 (MEGAN2.1): an extended and updated framework for modeling biogenic emissions, Geosci. Model Dev., 5, 1471–1492, https://doi.org/10.5194/gmd-5-1471-2012, 2012.
Guo, J., Gong, P., Dronova, I., and Zhu, Z.: Forest cover change in China from 2000 to 2016, Int. J. Remote Sens., 43, 593–606, https://doi.org/10.1080/01431161.2021.2022804, 2022.
He, Q., Ding, X., Fu, X., Zhang, Y., Wang, J., Liu, Y., Tang, M., Wang, X., and Rudich, Y.: Secondary Organic Aerosol Formation From Isoprene Epoxides in the Pearl River Delta, South China: IEPOX- and HMML-Derived Tracers, J. Geophys. Res.-Atmos., 123, 6999–7012, https://doi.org/10.1029/2017JD028242, 2018.
Hodzic, A., Kasibhatla, P. S., Jo, D. S., Cappa, C. D., Jimenez, J. L., Madronich, S., and Park, R. J.: Rethinking the global secondary organic aerosol (SOA) budget: stronger production, faster removal, shorter lifetime, Atmos. Chem. Phys., 16, 7917–7941, https://doi.org/10.5194/acp-16-7917-2016, 2016.
Hoyle, C. R., Boy, M., Donahue, N. M., Fry, J. L., Glasius, M., Guenther, A., Hallar, A. G., Huff Hartz, K., Petters, M. D., Petäjä, T., Rosenoern, T., and Sullivan, A. P.: A review of the anthropogenic influence on biogenic secondary organic aerosol, Atmos. Chem. Phys., 11, 321–343, https://doi.org/10.5194/acp-11-321-2011, 2011.
Hu, J., Wang, P., Ying, Q., Zhang, H., Chen, J., Ge, X., Li, X., Jiang, J., Wang, S., Zhang, J., Zhao, Y., and Zhang, Y.: Modeling biogenic and anthropogenic secondary organic aerosol in China, Atmos. Chem. Phys., 17, 77–92, https://doi.org/10.5194/acp-17-77-2017, 2017.
Hua, F., Wang, L., Fisher, B., Zheng, X., Wang, X., Yu, D. W., Tang, Y., Zhu, J., and Wilcove, D. S.: Tree plantations displacing native forests: The nature and drivers of apparent forest recovery on former croplands in Southwestern China from 2000 to 2015, Biol. Conserv., 222, 113–124, https://doi.org/10.1016/j.biocon.2018.03.034, 2018.
Huang, R.-J., Wang, Y., Cao, J., Lin, C., Duan, J., Chen, Q., Li, Y., Gu, Y., Yan, J., Xu, W., Fröhlich, R., Canonaco, F., Bozzetti, C., Ovadnevaite, J., Ceburnis, D., Canagaratna, M. R., Jayne, J., Worsnop, D. R., El-Haddad, I., Prévôt, A. S. H., and O'Dowd, C. D.: Primary emissions versus secondary formation of fine particulate matter in the most polluted city (Shijiazhuang) in North China, Atmos. Chem. Phys., 19, 2283–2298, https://doi.org/10.5194/acp-19-2283-2019, 2019.
Jo, D. S., Hodzic, A., Emmons, L. K., Marais, E. A., Peng, Z., Nault, B. A., Hu, W., Campuzano-Jost, P., and Jimenez, J. L.: A simplified parameterization of isoprene-epoxydiol-derived secondary organic aerosol (IEPOX-SOA) for global chemistry and climate models: a case study with GEOS-Chem v11-02-rc, Geosci. Model Dev., 12, 2983–3000, https://doi.org/10.5194/gmd-12-2983-2019, 2019.
Jo, D. S., Hodzic, A., Emmons, L. K., Tilmes, S., Schwantes, R. H., Mills, M. J., Campuzano-Jost, P., Hu, W., Zaveri, R. A., Easter, R. C., Singh, B., Lu, Z., Schulz, C., Schneider, J., Shilling, J. E., Wisthaler, A., and Jimenez, J. L.: Future changes in isoprene-epoxydiol-derived secondary organic aerosol (IEPOX SOA) under the Shared Socioeconomic Pathways: the importance of physicochemical dependency, Atmos. Chem. Phys., 21, 3395–3425, https://doi.org/10.5194/acp-21-3395-2021, 2021.
Kanellopoulos, P. G., Verouti, E., Chrysochou, E., Koukoulakis, K., and Bakeas, E.: Primary and secondary organic aerosol in an urban/industrial site: Sources, health implications and the role of plastic enriched waste burning, J. Environ. Sci., 99, 222–238, https://doi.org/10.1016/j.jes.2020.06.012, 2021.
Kong, L.: High-resolution simulation dataset of PM2.5 composition over China, China Air Pollution Data Center [data set], https://www.capdatabase.cn/resourceDownload/detail?id=207&type=2 (last access: 20 June 2024), 2022.
Kong, L., Tang, X., Zhu, J., Wang, Z., Li, J., Wu, H., Wu, Q., Chen, H., Zhu, L., Wang, W., Liu, B., Wang, Q., Chen, D., Pan, Y., Song, T., Li, F., Zheng, H., Jia, G., Lu, M., Wu, L., and Carmichael, G. R.: A 6-year-long (2013–2018) high-resolution air quality reanalysis dataset in China based on the assimilation of surface observations from CNEMC, Earth Syst. Sci. Data, 13, 529–570, https://doi.org/10.5194/essd-13-529-2021, 2021.
Lamsal, L. N., Krotkov, N. A., Marchenko, S. V., Joiner, J., Oman, L., Vasilkov, A., Fisher, B., Qin, W., Yang, E.-S., Fasnacht, Z., Choi, S., Leonard, P., and Haffner, D.: OMI/Aura NO2 Column Daily L3 Global Gridded 0.25 degree × 0.25 degree, Greenbelt, MD, USA, Goddard Earth Sciences Data and Information Services Center (GES DISC) [data set], https://doi.org/10.5067/MEASURES/MINDS/DATA304, 2022.
Lee, S. C., Chiu, M. Y., Ho, K. F., Zou, S. C., and Wang, X.: Volatile organic compounds (VOCs) in urban atmosphere of Hong Kong, Chemosphere, 48, 375–382, https://doi.org/10.1016/S0045-6535(02)00040-1, 2002.
Levy, R. C., Mattoo, S., Munchak, L. A., Remer, L. A., Sayer, A. M., Patadia, F., and Hsu, N. C.: The Collection 6 MODIS aerosol products over land and ocean, Atmos. Meas. Tech., 6, 2989–3034, https://doi.org/10.5194/amt-6-2989-2013, 2013.
Li, M., Liu, H., Geng, G., Hong, C., Liu, F., Song, Y., Tong, D., Zheng, B., Cui, H., Man, H., Zhang, Q., and He, K.: Anthropogenic emission inventories in China: a review, Natl. Sci. Rev., 4, 834–866, https://doi.org/10.1093/nsr/nwx150, 2017.
Lin, C. Q., Liu, G., Lau, A. K. H., Li, Y., Li, C. C., Fung, J. C. H., and Lao, X. Q.: High-resolution satellite remote sensing of provincial PM2.5 trends in China from 2001 to 2015, Atmos. Environ., 180, 110–116, https://doi.org/10.1016/j.atmosenv.2018.02.045, 2018.
Liu, G., Ma, X., Li, W., Chen, J., Ji, Y., and An, T.: Pollution characteristics, source appointment and environmental effect of oxygenated volatile organic compounds in Guangdong-Hong Kong-Macao Greater Bay Area: Implication for air quality management, Sci. Total Environ., 919, 170836, https://doi.org/10.1016/j.scitotenv.2024.170836, 2024.
Liu, P., Zhou, H., Chun, X., Wan, Z., Liu, T., Sun, B., Wang, J., and Zhang, W.: Characteristics of fine carbonaceous aerosols in Wuhai, a resource-based city in Northern China: Insights from energy efficiency and population density, Environ. Pollut., 292, 118368, https://doi.org/10.1016/j.envpol.2021.118368, 2022.
Liu, X., Ma, P.-L., Wang, H., Tilmes, S., Singh, B., Easter, R. C., Ghan, S. J., and Rasch, P. J.: Description and evaluation of a new four-mode version of the Modal Aerosol Module (MAM4) within version 5.3 of the Community Atmosphere Model, Geosci. Model Dev., 9, 505–522, https://doi.org/10.5194/gmd-9-505-2016, 2016.
Liu, Y., Dong, X., Wang, M., Emmons, L. K., Liu, Y., Liang, Y., Li, X., and Shrivastava, M.: Analysis of secondary organic aerosol simulation bias in the Community Earth System Model (CESM2.1), Atmos. Chem. Phys., 21, 8003–8021, https://doi.org/10.5194/acp-21-8003-2021, 2021a.
Liu, Y., Liu, Y., Wang, M., Dong, X., Zheng, Y., Shrivastava, M., Qian, Y., Bai, H., Li, X., and Yang, X.-Q.: Anthropogenic–biogenic interaction amplifies warming from emission reduction over the southeastern US, Environ. Res. Lett., 16, 124046, https://doi.org/10.1088/1748-9326/ac3285, 2021b.
Liu, Y., Dong, X., Emmons, L. K., Jo, D. S., Liu, Y., Shrivastava, M., Yue, M., Liang, Y., Song, Z., He, X., and Wang, M.: Exploring the Factors Controlling the Long-Term Trend (1988–2019) of Surface Organic Aerosols in the Continental United States by Simulations, J. Geophys. Res.-Atmos., 128, e2022JD037935, https://doi.org/10.1029/2022JD037935, 2023.
Lu, X., Zhang, S., Xing, J., Wang, Y., Chen, W., Ding, D., Wu, Y., Wang, S., Duan, L., and Hao, J.: Progress of Air Pollution Control in China and Its Challenges and Opportunities in the Ecological Civilization Era, Engineering, 6, 1423–1431, https://doi.org/10.1016/j.eng.2020.03.014, 2020.
Ma, Z., Hu, X., Sayer, A. M., Levy, R., Zhang, Q., Xue, Y., Tong, S., Bi, J., Huang, L., and Liu, Y.: Satellite-Based Spatiotemporal Trends in PM2.5 Concentrations: China, 2004–2013, Environ. Health Persp., 124, 184–192, https://doi.org/10.1289/ehp.1409481, 2016.
Maji, K. J., Li, V. Ok., and Lam, J. Ck.: Effects of China's current Air Pollution Prevention and Control Action Plan on air pollution patterns, health risks and mortalities in Beijing 2014–2018, Chemosphere, 260, 127572, https://doi.org/10.1016/j.chemosphere.2020.127572, 2020.
Malm, W. C. and Hand, J. L.: An examination of the physical and optical properties of aerosols collected in the IMPROVE program, Atmos. Environ., 41, 3407–3427, https://doi.org/10.1016/j.atmosenv.2006.12.012, 2007.
Miao, R., Chen, Q., Shrivastava, M., Chen, Y., Zhang, L., Hu, J., Zheng, Y., and Liao, K.: Process-based and observation-constrained SOA simulations in China: the role of semivolatile and intermediate-volatility organic compounds and OH levels, Atmos. Chem. Phys., 21, 16183–16201, https://doi.org/10.5194/acp-21-16183-2021, 2021.
Platnick, S., Meyer, K. G., King, M. D., Wind, G., Amarasinghe, N., Marchant, B., Arnold, G. T., Zhang, Z., Hubanks, P. A., Holz, R. E., Yang, P., Ridgway, W. L., and Riedi, J.: MODIS Atmosphere L3 Monthly Product, NASA MODIS Adaptive processing system, Goddard Space Flight Center, USA [data set], https://doi.org/10.5067/MODIS/MOD08_M3.006, 2015.
Pye, H. O. T., Pinder, R. W., Piletic, I. R., Xie, Y., Capps, S. L., Lin, Y.-H., Surratt, J. D., Zhang, Z., Gold, A., Luecken, D. J., Hutzell, W. T., Jaoui, M., Offenberg, J. H., Kleindienst, T. E., Lewandowski, M., and Edney, E. O.: Epoxide Pathways Improve Model Predictions of Isoprene Markers and Reveal Key Role of Acidity in Aerosol Formation, Environ. Sci. Technol., 47, 11056–11064, https://doi.org/10.1021/es402106h, 2013.
Pye, H. O. T., D'Ambro, E. L., Lee, B. H., Schobesberger, S., Takeuchi, M., Zhao, Y., Lopez-Hilfiker, F., Liu, J., Shilling, J. E., Xing, J., Mathur, R., Middlebrook, A. M., Liao, J., Welti, A., Graus, M., Warneke, C., De Gouw, J. A., Holloway, J. S., Ryerson, T. B., Pollack, I. B., and Thornton, J. A.: Anthropogenic enhancements to production of highly oxygenated molecules from autoxidation, P. Natl. Acad. Sci. USA, 116, 6641–6646, https://doi.org/10.1073/pnas.1810774116, 2019.
Qin, M., Wang, X., Hu, Y., Ding, X., Song, Y., Li, M., Vasilakos, P., Nenes, A., and Russell, A. G.: Simulating Biogenic Secondary Organic Aerosol During Summertime in China, J. Geophys. Res.-Atmos., 123, 11100–11119, https://doi.org/10.1029/2018JD029185, 2018.
Schwantes, R. H., Emmons, L. K., Orlando, J. J., Barth, M. C., Tyndall, G. S., Hall, S. R., Ullmann, K., St. Clair, J. M., Blake, D. R., Wisthaler, A., and Bui, T. P. V.: Comprehensive isoprene and terpene gas-phase chemistry improves simulated surface ozone in the southeastern US, Atmos. Chem. Phys., 20, 3739–3776, https://doi.org/10.5194/acp-20-3739-2020, 2020.
Shilling, J. E., Zaveri, R. A., Fast, J. D., Kleinman, L., Alexander, M. L., Canagaratna, M. R., Fortner, E., Hubbe, J. M., Jayne, J. T., Sedlacek, A., Setyan, A., Springston, S., Worsnop, D. R., and Zhang, Q.: Enhanced SOA formation from mixed anthropogenic and biogenic emissions during the CARES campaign, Atmos. Chem. Phys., 13, 2091–2113, https://doi.org/10.5194/acp-13-2091-2013, 2013.
Shrivastava, M., Cappa, C. D., Fan, J., Goldstein, A. H., Guenther, A. B., Jimenez, J. L., Kuang, C., Laskin, A., Martin, S. T., Ng, N. L., Petaja, T., Pierce, J. R., Rasch, P. J., Roldin, P., Seinfeld, J. H., Shilling, J., Smith, J. N., Thornton, J. A., Volkamer, R., Wang, J., Worsnop, D. R., Zaveri, R. A., Zelenyuk, A., and Zhang, Q.: Recent advances in understanding secondary organic aerosol: Implications for global climate forcing, Rev. Geophys., 55, 509–559, https://doi.org/10.1002/2016RG000540, 2017.
Shrivastava, M., Andreae, M. O., Artaxo, P., Barbosa, H. M. J., Berg, L. K., Brito, J., Ching, J., Easter, R. C., Fan, J., Fast, J. D., Feng, Z., Fuentes, J. D., Glasius, M., Goldstein, A. H., Alves, E. G., Gomes, H., Gu, D., Guenther, A., Jathar, S. H., Kim, S., Liu, Y., Lou, S., Martin, S. T., McNeill, V. F., Medeiros, A., De Sá, S. S., Shilling, J. E., Springston, S. R., Souza, R. A. F., Thornton, J. A., Isaacman-VanWertz, G., Yee, L. D., Ynoue, R., Zaveri, R. A., Zelenyuk, A., and Zhao, C.: Urban pollution greatly enhances formation of natural aerosols over the Amazon rainforest, Nat. Commun., 10, 1046, https://doi.org/10.1038/s41467-019-08909-4, 2019.
Tilmes, S., Hodzic, A., Emmons, L. K., Mills, M. J., Gettelman, A., Kinnison, D. E., Park, M., Lamarque, J.-F., Vitt, F., Shrivastava, M., Campuzano-Jost, P., Jimenez, J. L., and Liu, X.: Climate Forcing and Trends of Organic Aerosols in the Community Earth System Model (CESM2), J. Adv. Model. Earth Sy., 11, 4323–4351, https://doi.org/10.1029/2019MS001827, 2019.
Tong, D., Cheng, J., Liu, Y., Yu, S., Yan, L., Hong, C., Qin, Y., Zhao, H., Zheng, Y., Geng, G., Li, M., Liu, F., Zhang, Y., Zheng, B., Clarke, L., and Zhang, Q.: Dynamic projection of anthropogenic emissions in China: methodology and 2015–2050 emission pathways under a range of socio-economic, climate policy, and pollution control scenarios, Atmos. Chem. Phys., 20, 5729–5757, https://doi.org/10.5194/acp-20-5729-2020, 2020.
Wang, L., Jin, X., Wang, Q., Mao, H., Liu, Q., Weng, G., and Wang, Y.: Spatial and temporal variability of open biomass burning in Northeast China from 2003 to 2017, Atmospheric Ocean. Sci. Lett., 13, 240–247, https://doi.org/10.1080/16742834.2020.1742574, 2020.
Xing, L., Fu, T.-M., Liu, T., Qin, Y., Zhou, L., Chan, C. K., Guo, H., Yao, D., and Duan, K.: Estimating organic aerosol emissions from cooking in winter over the Pearl River Delta region, China, Environ. Pollut., 292, 118266, https://doi.org/10.1016/j.envpol.2021.118266, 2022.
Xu, B., Wang, T., Ma, D., Song, R., Zhang, M., Gao, L., Li, S., Zhuang, B., Li, M., and Xie, M.: Impacts of regional emission reduction and global climate change on air quality and temperature to attain carbon neutrality in China, Atmos. Res., 279, 106384, https://doi.org/10.1016/j.atmosres.2022.106384, 2022.
Xu, Z. N., Nie, W., Liu, Y. L., Sun, P., Huang, D. D., Yan, C., Krechmer, J., Ye, P. L., Xu, Z., Qi, X. M., Zhu, C. J., Li, Y. Y., Wang, T. Y., Wang, L., Huang, X., Tang, R. Z., Guo, S., Xiu, G. L., Fu, Q. Y., Worsnop, D., Chi, X. G., and Ding, A. J.: Multifunctional Products of Isoprene Oxidation in Polluted Atmosphere and Their Contribution to SOA, Geophys. Res. Lett., 48, e2020GL089276, https://doi.org/10.1029/2020GL089276, 2021.
Yin, H., Pflugmacher, D., Li, A., Li, Z., and Hostert, P.: Land use and land cover change in Inner Mongolia - understanding the effects of China's re-vegetation programs, Remote Sens. Environ., 204, 918–930, https://doi.org/10.1016/j.rse.2017.08.030, 2018.
Yue, M., Dong, X., Wang, M., Emmons, L. K., Liang, Y., Tong, D., Liu, Y., and Liu, Y.: Modeling the Air Pollution and Aerosol-PBL Interactions Over China Using a Variable-Resolution Global Model, J. Geophys. Res.-Atmos., 128, e2023JD039130, https://doi.org/10.1029/2023JD039130, 2023.
Zaveri, R. A., Easter, R. C., Fast, J. D., and Peters, L. K.: Model for Simulating Aerosol Interactions and Chemistry (MOSAIC), J. Geophys. Res.-Atmos., 113, 2007JD008782, https://doi.org/10.1029/2007JD008782, 2008.
Zaveri, R. A., Easter, R. C., Singh, B., Wang, H., Lu, Z., Tilmes, S., Emmons, L. K., Vitt, F., Zhang, R., Liu, X., Ghan, S. J., and Rasch, P. J.: Development and Evaluation of Chemistry-Aerosol-Climate Model CAM5-Chem-MAM7-MOSAIC: Global Atmospheric Distribution and Radiative Effects of Nitrate Aerosol, J. Adv. Model. Earth Sy., 13, e2020MS002346, https://doi.org/10.1029/2020MS002346, 2021.
Zhang, G. J. and McFarlane, N. A.: Sensitivity of climate simulations to the parameterization of cumulus convection in the Canadian climate centre general circulation model, Atmos. Ocean, 33, 407–446, https://doi.org/10.1080/07055900.1995.9649539, 1995.
Zhang, H., Yee, L. D., Lee, B. H., Curtis, M. P., Worton, D. R., Isaacman-VanWertz, G., Offenberg, J. H., Lewandowski, M., Kleindienst, T. E., Beaver, M. R., Holder, A. L., Lonneman, W. A., Docherty, K. S., Jaoui, M., Pye, H. O. T., Hu, W., Day, D. A., Campuzano-Jost, P., Jimenez, J. L., Guo, H., Weber, R. J., De Gouw, J., Koss, A. R., Edgerton, E. S., Brune, W., Mohr, C., Lopez-Hilfiker, F. D., Lutz, A., Kreisberg, N. M., Spielman, S. R., Hering, S. V., Wilson, K. R., Thornton, J. A., and Goldstein, A. H.: Monoterpenes are the largest source of summertime organic aerosol in the southeastern United States, P. Natl. Acad. Sci. USA, 115, 2038–2043, https://doi.org/10.1073/pnas.1717513115, 2018.
Zhang, J., Liu, J., Ding, X., He, X., Zhang, T., Zheng, M., Choi, M., Isaacman-VanWertz, G., Yee, L., Zhang, H., Misztal, P., Goldstein, A. H., Guenther, A. B., Budisulistiorini, S. H., Surratt, J. D., Stone, E. A., Shrivastava, M., Wu, D., Yu, J. Z., and Ying, Q.: New formation and fate of Isoprene SOA markers revealed by field data-constrained modeling, Npj Clim. Atmospheric Sci., 6, 69, https://doi.org/10.1038/s41612-023-00394-3, 2023.
Zhang, Q., Jimenez, J. L., Canagaratna, M. R., Allan, J. D., Coe, H., Ulbrich, I., Alfarra, M. R., Takami, A., Middlebrook, A. M., Sun, Y. L., Dzepina, K., Dunlea, E., Docherty, K., DeCarlo, P. F., Salcedo, D., Onasch, T., Jayne, J. T., Miyoshi, T., Shimono, A., Hatakeyama, S., Takegawa, N., Kondo, Y., Schneider, J., Drewnick, F., Borrmann, S., Weimer, S., Demerjian, K., Williams, P., Bower, K., Bahreini, R., Cottrell, L., Griffin, R. J., Rautiainen, J., Sun, J. Y., Zhang, Y. M., and Worsnop, D. R.: Ubiquity and dominance of oxygenated species in organic aerosols in anthropogenically-influenced Northern Hemisphere midlatitudes, Geophys. Res. Lett., 34, 2007GL029979, https://doi.org/10.1029/2007GL029979, 2007.
Zhang, W.: Modeled OA and its subspecies data for this paper, Zenodo [data set], https://doi.org/10.5281/zenodo.14742102, 2025.
Zheng, B., Tong, D., Li, M., Liu, F., Hong, C., Geng, G., Li, H., Li, X., Peng, L., Qi, J., Yan, L., Zhang, Y., Zhao, H., Zheng, Y., He, K., and Zhang, Q.: Trends in China's anthropogenic emissions since 2010 as the consequence of clean air actions, Atmos. Chem. Phys., 18, 14095–14111, https://doi.org/10.5194/acp-18-14095-2018, 2018.
Zheng, H., Chang, X., Wang, S., Li, S., Zhao, B., Dong, Z., Ding, D., Jiang, Y., Huang, G., Huang, C., An, J., Zhou, M., Qiao, L., and Xing, J.: Sources of Organic Aerosol in China from 2005 to 2019: A Modeling Analysis, Environ. Sci. Technol., 57, 5957–5966, https://doi.org/10.1021/acs.est.2c08315, 2023a.
Zheng, H., Chang, X., Wang, S., Li, S., Yin, D., Zhao, B., Huang, G., Huang, L., Jiang, Y., Dong, Z., He, Y., Huang, C., and Xing, J.: Trends of Full-Volatility Organic Emissions in China from 2005 to 2019 and Their Organic Aerosol Formation Potentials, Environ. Sci. Tech. Let., 10, 137–144, https://doi.org/10.1021/acs.estlett.2c00944, 2023b.
Zhong, Y., Chen, J., Zhao, Q., Zhang, N., Feng, J., and Fu, Q.: Temporal trends of the concentration and sources of secondary organic aerosols in PM2.5 in Shanghai during 2012 and 2018, Atmos. Environ., 261, 118596, https://doi.org/10.1016/j.atmosenv.2021.118596, 2021.
Zhuang, Y., Li, R., Yang, H., Chen, D., Chen, Z., Gao, B., and He, B.: Understanding Temporal and Spatial Distribution of Crop Residue Burning in China from 2003 to 2017 Using MODIS Data, Remote Sens.-Basel, 10, 390, https://doi.org/10.3390/rs10030390, 2018.