the Creative Commons Attribution 4.0 License.
the Creative Commons Attribution 4.0 License.
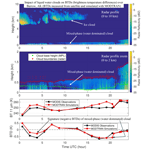
Remote-sensing detectability of airborne Arctic dust
Norman T. O'Neill
Keyvan Ranjbar
Liviu Ivănescu
Yann Blanchard
Seyed Ali Sayedain
Yasmin AboEl-Fetouh
Remote-sensing (RS)-based estimates of Arctic dust are oftentimes overestimated due to a failure in separating out the dust contribution from that of spatially homogeneous clouds or low-altitude cloud-like plumes. A variety of illustrations are given with a particular emphasis on questionable claims of using brightness temperature differences (BTDs) as a signature indicator of Arctic dust transported from mid-latitude deserts or generated by local Arctic sources. While there is little dispute about the presence of both Asian and local dust across the Arctic, the direct RS detectability of airborne dust, as ascribed to satellite (MODIS and AVHRR) measurements of significantly negative brightness temperature differences at 11 and 12 µm (BTD11–12), has been misrepresented in certain cases. While it is difficult to account for all examples of strongly negative BTD11–12 values in the Arctic, it is unlikely that airborne dust plays a significant role. One much more likely contributor would be water clouds in the Arctic inversion layer.
The RS detectability of the impact of Arctic dust (notably due to Arctic dust from local sources) can, however, be of significance. Sustained dust deposition can substantially decrease (visible to shortwave IR) snow and ice reflectance albedo (pan-chromatic reflectance) and the signal measured by satellite sensors. Significantly negative BTD11–12 values would, however, only represent a limited area near the drainage basin sources according to our event-level case studies. The enhanced ice-nucleating particle (INP) role of local Arctic dust can, for example, induce significant changes in the properties of low-level mixed-phase clouds (cloud optical depth changes <∼ 1) that can readily be detected by active and passive RS instruments. It is critical that the distinction between the RS detectability of airborne Arctic dust versus the RS detectability of the impacts of that dust be understood if we are to appropriately parameterize, for example, the radiative forcing influence of dust in this climate-sensitive region.
- Article
(5121 KB) - Full-text XML
-
Supplement
(26108 KB) - BibTeX
- EndNote
Vincent (2018) (VCT) reported on the use of MODIS and AVHRR thermal infrared (TIR) brightness temperature differences (BTDs) in the western Canadian Arctic (Beaufort Sea and Amundsen Gulf region) to detect the presence of “persistent low-level dust clouds” and dust deposited on ice, snow, and water. A later publication (Bowen and Vincent, 2021) (B&V) argued that negative BTD11–12 (BT11 µm – BT12 µm) values were a unique signature of dust (without explicitly distinguishing between airborne and surface deposited dust) and that this measure could directly be used to estimate the relative spatial extent of dust in the Arctic. Those two water bodies (along with other place names and geographic features that are discussed below) appear in the Fig. 1 map of the gray-shaded Canadian Arctic Archipelago (CAA) and associated Arctic and sub-Arctic regions.
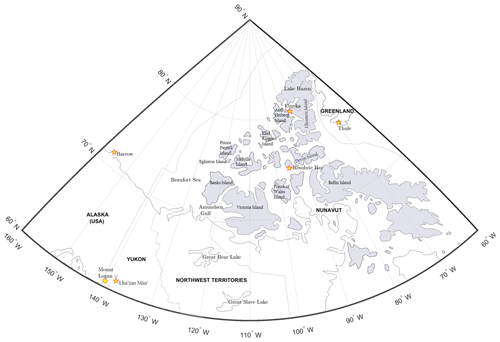
Figure 1Map of the (gray-shaded) islands of the Canadian Arctic Archipelago (CAA). The map also includes Arctic and subarctic research sites in Alaska, northern Canada, and Greenland (indicated with golden stars) and geographic features that are discussed in the main text.
We acknowledge the robustness of negative BTD11–12 values as a potential indicator of optically thick airborne dust embedded in a normal-lapse-rate atmospheric layer or as a sign of accumulated dust deposition. We disagree with the affirmation that airborne dust clouds of Asian origin were commonly detected using passive, satellite-based remote sensing (RS). Springtime Asian dust, while representing a robust pan-Arctic seasonal event, yields, on average, very weak coarse mode (CM), roughly submicron, aerosol optical depths (AODs) at 550 nm.1. The multi-year, six-station, pan-Arctic, AERONET/AEROCAN monthly binned (geometric means) climatology of AboEl-Fetouh et al. (2020) (AeF) indicates (their Fig. 7) that the Resolute Bay CM AODs are largely of the greatest amplitude across the six stations: using that site as a reference, their CM AODs were <∼ the Resolute Bay value ( during the Asian-dust-dominated April–May springtime and <∼ during the June–August (likely local dust) summertime). DODs (dust optical depths) will be ∼ CM AODs in the absence of any other significant CM source such as sea salt or volcanic ash (or CM cloud in the absence of proper cloud screening).
Springtime Asian dust aerosols at such small CM AOD (DOD) values are difficult, if not impossible, to detect using passive satellite-based RS techniques at any wavelength. The BTD11–12 variation per unit change in DOD is ∼ −0.3 K (see the discussion of Fig. B1 for details). The corresponding change in BTD11–12 for a springtime Asian dust DOD of 0.016 (the Resolute Bay maximum) would be an undetectable −0.005 K (an amplitude that is significantly less than the nominal MODIS BTD11–12 noise figure of 0.07 K – again, see the discussion of Fig. B1).
While the monthly averaged springtime Asian dust DODs are <∼ 0.016, there are springtime (mid-tropospheric) Asian dust events that do lead to more substantive DODs over the Arctic. In general, these are limited to a few notable days in a given year, with individual DODs being <∼ 0.4: the roughly week-long dust intrusion over Mount Logan (Yukon territory of Canada) in April of 2001 (DODs < ∼ 0.3);2 the roughly week-long, mid-tropospheric dust intrusions of April 2002 over Barrow, Alaska (now Utqiagvik) (DODs <∼ 0.4) (Stone et al., 2007); moderate DODs (< ∼ 0.1) associated with single-day intrusions over Barrow in April of 2015 (Zhao et al., 2022); and evidence that the sub-0.4 DODs of the April 2001 event were arguably a broad west coast phenomenon stretching from (at least) low-Arctic Canada to the southern United States (Thulasiraman et al., 2002). DODs ∼ 0.4 could incite a marginally detectable BTD11–12 signal (−0.3 K × 0.4 ∼ −0.12 K) and would be more easily identified in true-color and AOD imagery (at least over water).
VCT noted that a second dust source could have been locally generated dust storms (although dust from southern latitudes was claimed to be the major source). Indeed, Meinander et al. (2022) recently reviewed the importance of high-latitude dust generated from local sources. However, even strong local dust plumes will likely not induce large DODs beyond the short temporal and spatial window associated with their detectable plume presence. Outside this window, the monthly averaged DOD upper limit reported by AeF make it very unlikely that DODs could be detected using passive, satellite-based RS in the thermal infrared (TIR).
Empirical support for this affirmation is provided, for example, in the detection of a high-Arctic dust plume near its drainage-basin source by Ranjbar et al., 2021: their MODIS BTD11–12 values (amplitude ∼ 1.5 K) are ∼ the amplitude of the most extreme negative values (∼ −1.0 K) reported by VCT. However, Ranjbar's visible (532 nm) DODs were ∼ 0.5 (a BTD11–12-to-DOD sensitivity of dBTD11–12 / dDOD ∼ K per unit DOD). The AeF Resolute Bay summertime3 CM AOD maximum ( would, assuming approximate proportionality, produce generally undetectable BTD11–12 changes (|BTD 3 K).
As another source of independent support for the general weakness of Arctic DODs, we note that AeF's summertime DOD statistics are ∼ DOD computations derived from the simulated local dust (“Arctic dust”) polar map (Fig. 1 of Kawai et al., 2023 (KA)). The KA multi-year (2010–2019) “annual mean vertically integrated mass concentrations” (“Arctic dust mass” with units of mg m−2) yield DODs that are ∼ AeF's summertime CM AOD (see Fig. A1 of Sect. A3.2 in the Appendix, where we compare the KA DOD simulations for the four AeF sites in or near the CAA). The summertime constraint on their comparison comes from the Asian dust domination of AeF's springtime CM AOD (Asian dust is a dust component that is not modeled by the KA simulations).
Having argued that Arctic DODs are, in general, at the margins of RS detectability, we must also take issue with B&V's affirmation that “while it is possible that a substance other than mineral dust is causing large-scale negative BTD11–12 signatures in the polar environment, there is nothing in the literature to support this conjecture”. We will present an alternative mechanism below involving inversion-layer liquid (droplet) clouds whose cloud optical depths (CODs) are sufficiently large to induce significant negative BTD11–12 signatures.
In general, there is often a tendency in the literature to significantly overestimate DOD magnitudes of Asian dust. It is no trivial matter to decouple such relatively small DODs from very large CM CODs of clouds that are often in the neighborhood of those dust plumes. Such clouds may indeed result from dust nucleation: see, for example, Hildner et al. (2010) and their discussion involving a high-altitude Asian dust plume that apparently nucleates into a highly depolarizing cloud (captured by the AHSRL lidar above our Polar Environment Atmospheric Research Lab (PEARL) observatory at Eureka, Nunavut). In more general terms, Eck et al. (2009) noted the shortcomings in applying the AERONET (temporally based) V2 cloud-screening algorithm in the presence of spatially homogeneous clouds at Barrow, AK: spatially homogeneous (insufficiently variable) clouds such as thin cirrus are erroneously classified as dust (false-positive “dust”). More recently, Stone et al. (2014) underscored the potential for the same false-positive problem in their Barrow-based climatology of Arctic aerosols. Ranjbar et al. (2022) argued that the authors of a case study involving the transport of Asian dust into the High Arctic likely confused DOD with nearby COD and thereby significantly overestimated the DOD of a thin Asian dust plume about 7 km above the PEARL observatory.
Analogous problems plague polar winter data. O'Neill et al. (2016) used lidar profiles and a spectral cloud-screening approach to estimate the large (star-photometer-derived) CM AOD errors that would be associated with the application of frequently inadequate (temporally based) cloud screening paradigms to polar winter optical depths acquired at the OPAL Eureka site. The authors concluded that “spatially homogeneous clouds and low-altitude ice clouds that remain after temporal cloud screening represent an inevitable systematic error in the estimation of AOD (more so for CM AOD): the (positive bias) AOD error was estimated to vary from 78 % to 210 % at Eureka and from 2 % to 157 % at Ny-Ålesund.” In a not unrelated finding, Zamora et al. (2022) pointed out that the CALIPSO (CALIOP) classification algorithm was likely misclassifying wintertime “diamond dust” as mineral dust in their pan-Arctic analysis.
In terms of satellite-based estimates of DOD, B&V claimed that average AOD was “a proxy for dust aerosol concentration” and employed the 1998 to 2010 SeaWiFS AOD climatology of Hsu et al. (2012) to report a slight increase in AOD over the global oceans (and, given their dust proxy assertion, a slight increase in DOD) in an apparent effort to support an increasing trend in their average “relative spatial extent of dust” (RSED) parameter over the Arctic and Antarctic. This is yet another instance of DOD overestimation in the literature: AOD is almost universally dominated by fine-mode (roughly submicron) particles and cannot be viewed as a proxy for “dust aerosol concentration” (while there is evidence that fine-mode dust exists, there is little evidence that it dominates other types of fine-mode aerosols). The proxy assumption is especially questionable when claiming to report a trend involving a minor AOD species (dust) coupled with a satellite AOD product that is less accurate than the AOD generated from ground-based AERONET data (for which a DOD trend analysis would be a challenge on its own merits): the bias error (amplitude >∼ 0.01) between the SeaWiFS AODs and AERONET AODs (Fig. 2 of Hsu et al., 2012), for example, is >∼ the spread of AeF's spring and summer geometric standard deviation envelope for Resolute Bay (.
A more realistic DOD satellite product over the Arctic is the ModIs Dust AeroSol (MIDAS) data set (Gkikas et al., 2021). The MIDAS reanalysis system is based on MODIS AODs coupled with mineral dust fraction (MDF; a semi-intensive parameter, DOD/AOD) derived from the Modern-Era Retrospective analysis for Research and Applications version 2 (MERRA-2), whose key components are the Goddard GOCART aerosol model and the Goddard Earth Observing System (GEOS). The MIDAS annual DOD (arithmetic) mean for the 2003–2017 period (for the more accurate retrievals over water around Resolute Bay) is ∼ 0.01±0.02 (where 0.02 is a computed estimate of retrieval uncertainty rather than a standard deviation). The AeF Resolute Bay value of 0.0051 is contained with the MIDAS uncertainty envelope.
Arctic aerosol events that are detectable using visible to near-IR passive, satellite-based RS techniques are, for the most part, either fine-mode (FM) smoke or, to a lesser extent, FM Arctic haze. Xian et al. (2022) present a comprehensive pan-Arctic investigation of FM and CM AODs using reanalysis simulations of three aerosol transport models tied to satellite-based retrievals and verified (at the FM and CM AOD level) using a network of Arctic AERONET stations. The monthly binned multi-reanalysis consensus (MRC) AODs of their three models are shown as a function of aerosol species for the period of 2003 to 2019 (their Fig. 2). The results for Resolute Bay show a year-round dominance of FM smoke and/or FM “ABF” aerosol (essentially anthropogenic sulfate-based Arctic haze or FM aerosols of biogenic origin), with CM dust aerosols having their greatest minority impact during the springtime Asian dust event (monthly arithmetic means of 〈DOD〉<∼0.03 compared to smoke and ABF monthly means of 〈FM AOD〉<∼0.1).
Returning to an event-level case presented by VCT, the claim of a “strong dust event” associated with VCT's Fig. 5 (and Fig. 3b) imagery was (if aerosols were to be ascribed any role) associated with an FM smoke event induced by fires in Alaska and the Canadian Northwest Territories (see Fig. S1a and its discussion). Figure S1b in the Supplement shows, if anything, that there is marginal correspondence at best between the position of the smoke plume over the Amundsen Gulf (as evidenced by the pattern of the smoke on the true-color image) and the patterns of negative blue-colored BTD11–12 values over the water regions south of Banks Island (at the southern extreme of the CAA). The principle optical effect in the massive region of blue-colored BTD11–12 values to the west of Banks Island is largely associated with the presence of “liquid water” clouds or “uncertain” phase clouds (see the WorldView classifications of Fig. S1b).
The spectral properties of water clouds, for CODs that are typically ≫ than the weak DODs described above, will likely dominate the BTD11–12 spectral signature of Asian dust or local dust that is not within the immediate range of its drainage basin source. We found numerous examples of the presence of low-level CM water clouds characterized by strong COD and weakly to strongly negative BTD11–12 values over the Beaufort Sea (illustrated by the COD >∼ 5 and BTD K case study of 29 May 2005 in Figs. S2 to S5). Given the arguments presented above on the general weakness of DODs and the likely absence of any strong local dust source in the middle of the frozen Beaufort Sea, it is very unlikely that the massive region of negative BTD11–12 values seen in cases such as that of Fig. S3 could be attributed to the direct thermal influence of dust aerosols.
We found (over a 2011–2018 sampling period) persistent if irregular winter-to-spring (October to April) and summertime events of moderately negative MODIS BTD11–12 values ( BTD11–12 >∼ −0.8 K) acquired near Barrow, where ground-based lidar and radar profiles indicated strong super-unity CODs associated with physically thin, near-surface water clouds (see the Supplement files “BTDBarrowSummer.xlsx” and “BTDBarrowWinter.xlsx” for details on our analysis of BTD11–12 results near Barrow). Such low-altitude mixed-phase (water mixed with ice) clouds have been reported in the literature: de Boer et al. (2009) and Shupe et al. (2015) provide lidar/radar-supported illustrations of mixed-phase events at Eureka and Barrow, respectively.4 The former paper reported a 4-year (2004–2007) frequency-of-occurrence (%) series (3-month-wide bins) of combined Barrow and Eureka results showing a general predominance of “SON” fall bins (∼ 10 %) at <∼ 1.5 km mean cloud-base height5 for single-layer, mixed-phase stratiform clouds. The latter paper provided a 2-year Barrow climatology (which is more relevant to the Barrow-region focus of the analysis that follows) showing that the monthly occurrence (%) was highest in October (∼ 40 %) while being only moderate and of lower altitude from March (∼ 10 %) to ∼ 25 % in April and May (with a strong preponderance of sub-1 km liquid occurrence). Yi et al. (2019) reported comprehensive satellite-based (MODIS and CALIOP) water cloud (“Arctic fog”) statistics for March to October in a large Arctic Ocean region roughly centered north of Barrow. Their water cloud limitation to fog (cloud base height = cloud height – cloud thickness being < 1000 feet (300 m)) was, however, rather restrictive with respect to the types of liquid cloud events that we investigate below (events requiring that the water cloud be imbedded in significant temperature slices of the Arctic inversion layer).
Nearly all our negative BTD11–12 Barrow examples shared one feature that is rarely mentioned in the typical BTD11–12 literature: the ubiquitous and strong Arctic temperature inversion up to altitudes ∼ 1 km that occurs during the polar winter and summer (see again the Supplement files “BTDBarrowSummer.xlsx” and “BTDBarrowWinter.xlsx” for details on our analysis near the Barrow site as well as Bradley et al., 1993, and Palo et al., 2017, for statistical summaries of the Arctic inversion layer). Inversion-layer cloud events are the most easily detectable instances of a fundamental principle: that a “warm cloud” in the Arctic inversion layer (in an atmosphere clear of higher-altitude clouds) transforms the more common negative-lapse-rate BTD11–12 signature from a generally positive to negative dependency with the degree of negativity being dependent (for a given surface emissivity, inversion-layer strength, and water vapor load) on the COD and effective particle radius (see, for example, Key, 2002, and Liu et al., 2004).
We generated MODTRAN-simulated BTD11–12 vs. BT11 graphics whose input parameters encompassed a wide variety of COD and particle size conditions about a specific 22 March 2015 event at Barrow (see the discussion of that event associated with Fig. 1 below). The resulting BTD11–12 vs. BT11 patterns are shown in Fig. B1, while Table 1 below presents a descriptive summary of those simulations6 (the optical details and boundary layer conditions associated with each BTD11–12 vs. BT11 pattern are given in Sect. B1). The “convex-downward” shape of a large-COD water cloud in an inversion layer will produce almost exclusively negative values that fundamentally depend on the COD = 0 and ∞ singularities on the BTD11–12 vs. BT11 patterns of Fig. B1 (while a high-altitude ice or liquid cloud will produce, as per the upper left graphic of Fig. B1, generally positive BTD11–12 values).
Table 1Empirical (E) and simulated (S) BTD11–12 vs. BT11 results for a variety of cloud or dust plumes embedded in positive-temperature-lapse-rate (inversion layer) or negative-lapse-rate regions. Blue, red, and brown text refers to water clouds, ice clouds, and dust plumes, respectively.
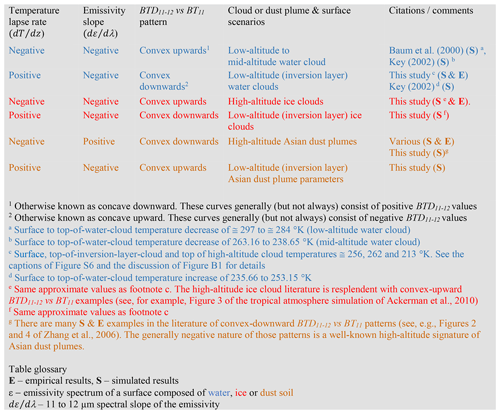
Figure 2a shows a radar profile for the specific inversion-layer (Fig. S6) Barrow illustration of the 22 March 2015 event (with Fig. 2b providing a zoom of the radar profile in the inversion layer between 0 and 2 km). Figure 2c shows both the MODIS-measured moderate-amplitude BTD11–12 values and the MODTRAN-simulated values (details in the figure caption). This demonstrates how (i) a warm, liquid-water, inversion-layer (negative BTD11–12) cloud (whose lower and upper boundaries are explicitly shown in Figs. S6 and 2b) coupled with the positive BTD11–12 presence of a cold (negative or normal-lapse-rate) ice cloud around 6 km altitude (during roughly the first half of the displayed time period) produces systematically varying BTD11–12 values that oscillate between the negative to positive extremes of the two phenomena and that (ii) MODTRAN radiative transfer simulations were largely successful in capturing the BTD11–12 oscillations.
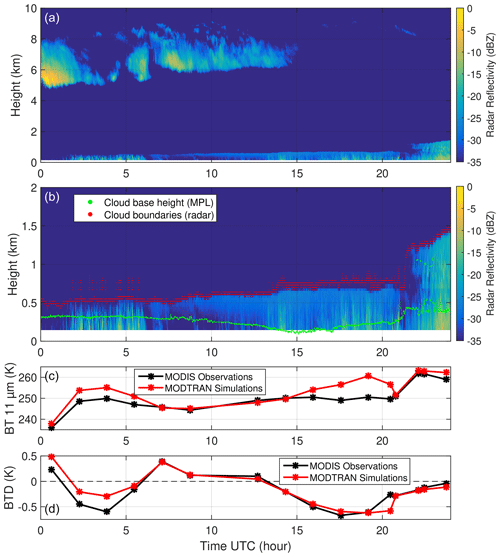
Figure 2Radar backscatter coefficient profiles acquired at the ARM Barrow site on 22 March 2015: (a) 0 to 10 km altitude and (b) a zoom from 0 to 2 km altitude. The green and red curves (as measured by the combination of lidar and radar backscatter profiles) indicate, respectively, the bottom and top of what we inferred to be a water-dominated, mixed-phase cloud that was partially contained in an inversion layer. The dynamical details of this event, including radiosonde temperature profiles, are given in Fig. S6 and its caption. The solid black curves of panel (c) show, for the MODIS pixel nearest to the Barrow site, the MODIS-measured BT11 time series (upper graph) and the MODIS-measured BTD11–12 time series (lower graph). The solid red curves show their MODTRAN-simulated analogues. These simulations employed input parameters representative of that day (see the captions of Figs. S6 and S7 for further details).
Specific details on the vertical extent of the mixed-phase backscatter coefficient profile, the water vs. ice COD contributions, and their relationship to the temperature (inversion layer) profile provided by the Barrow radiosonde profiles are presented in the discussion of Fig. S6. In those details, we argue that the radar profiles provide key information about the upper boundary of the water/mixed-phase cloud (beyond the upper bound defined by the extinction limit of the lidar) and its attendant extension to altitudes where there was actually a strong temperature inversion.
The same (Table 1) inversion-layer BTD11–12 convexity reversal should apply to warm, low-level ice clouds in an Arctic inversion layer: however, as shown in Fig. B1b, the amplitude of the convex-downward pattern can be insufficient to move the pattern into the negative BTD11–12 range. In any case, in our survey of significantly negative, near-Barrow BTD11–12 (MODIS) values, we did not find any obvious lidar/radar retrievals dominated by synchronous, low-level, optically thick ice clouds in the inversion layer. This is not unexpected, since the CODs of near-surface ice clouds are substantially smaller than those of water clouds (see Shonk et al., 2019, for a general statement and specific examples in the 29 December 2006 Eureka case study of de Boer et al. (2009) and Sects. 3d vs. 4c of Zuidema et al., 2005, for a 1–18 May 1998 case study at a floating ice camp ∼ 600 km northwest of Barrow). Morrison et al. (2012) also point out the dominance of water CODs over ice CODs across a 5 d (11–15 May 2011) Eureka event and underscore the persistence of Arctic mixed-phase clouds in general. This dominance of water COD over ice COD was also found in our Fig. 2 case study (see the caption of Fig. S6 for details).
We would note that a convex-down to convex-up pattern reversal would occur when comparing high-altitude dust clouds with warm dust clouds located in an Arctic inversion layer: the generally negative contours for the former would be transformed to positive contours (see Fig. B1d) that would actually confound the classic negative BTD11–12 signature of cold, high-altitude dust clouds (see, for example, Fig. 3 (MODIS BTD11–12) and Fig. 4 (simulations) of Zhang et al., 2006). Support for this affirmation for the case of local dust comes indirectly from Ranjbar et al. (2021): the lapse rate in the Lake Hazen case was, in all likelihood, an (inversion-layer-free) rate of decreasing temperature with increasing altitude. This results in the negative BTD11–12 plume values reported in that paper (the defining BTD11–12 vs. BTD11 pattern is more in the nature of the convex-down shapes of Fig. B1c).
The overarching message of this section is that negative BTD11–12 values in the Arctic are not a unique signature of the pervasiveness of dust across the Arctic. The BTD11–12 signature of airborne dust in the inversion layer would generally be too weak to detect and of the wrong sign with respect to the classical negative signature of desert dust plumes in a normal-lapse-rate environment. DODs in the Arctic are generally too small to induce significant BTD11–12 amplitudes. The BTD11–12 signature of deposited dust can be significantly negative but, as suggested by our case study on snow deposition of local dust (see the following section), tend to be spatially limited to dust-dominated regions of the drainage basin source.
An affirmation of the general marginality of airborne dust RS detectability in the Arctic is not to say that the impacts of Asian or local dust are necessarily marginal in terms of satellite-based RS. The cumulative deposition of local dust associated with weak DODs can (as also noted by VCT) be substantial over seasonal or longer timescales with significant changes in surface reflectance (and attendant impacts on early snowmelt coupled with a feedback effect of even greater reflectance changes). AVHRR remote-sensing imagery dating back as far as 1991 was employed by Woo et al. (1991) to argue that dust-covered areas on the Fosheim Peninsula (region of Eureka) were the first to experience snowmelt (Ranjbar et al., 2021 showed image browning regions on MODIS “Corrected Reflectance (True Color)” (RGB) images that corresponded to Woo's “dark spot” regions). Figure S8 shows what we argue are examples of dust deposition on snow or ice in the neighborhood of southern CAA drainage basins whose flow dynamics have induced local dust plumes. This illustrates how the accumulation of local dust deposition by dust plumes produces (i) true-color images of significantly modified snow reflectance in the visible spectral region (<∼ 60 % average reflectance change7) but only (ii) weakly positive BTD11–12 dust deposition signatures near their drainage basin sources (while also showing that significantly negative BTD11–12 signatures do occur in what are likely the very localized pure-dust regions of the drainage basins). This, as indicated in the legend of Fig. S8, is likely a BTD11–12 difference resulting from the greater dust surface emissivity of Band 12 relative to Band 11. These illustrations strongly suggest that significantly negative signatures of dust on snow or ice are likely to be very limited in their spatial extent.
The reflectance effects associated with the deposition of Asian dust on snow are less evident. Asian dust deposition was detected by ground teams at higher altitudes (where sources of local dust would be unlikely) in the Mount Logan (Yukon) region of the St. Elias range during the strong Asian dust event of April 2001 (Zdanowicz et al., 2006). The authors suggested that up to 45 % of the airborne dust mass abundance was deposited in the snow (over a 9 d period) and that the mechanism for deposition was scavenging by snowflakes. MODIS RGB images show no obvious impact: this effort to determine an impact is not helped by these agents of deposition arguably confounding/camouflaging the darkening impact of dust. Zhao et al. (2022) employed a variety of ground- and satellite-based passive and active RS data along with surface nephelometer measurements of the CM scattering coefficient to investigate the albedo (spectrally integrated reflectance) impact of dust deposition on snow during the 14 March 2013 and 20 April 2015 Asian dust events over Alert (Nunavut) and Barrow, Alaska, respectively.8 The CM scattering coefficients coupled with estimates of the dust plume (mid-tropospheric) altitude over each site suggested direct deposition links between the dust plumes and the surface dust (they did not attempt to elaborate on any explanation of the deposition dynamics). The authors employed a radiative transfer model to argue that daily dust deposition events could reduce snow surface (panchromatic) albedo by as much as 2.3 % at Barrow and 1.9 % at Alert. These albedo reductions would be quite substantial if dust depositions (in relatively unperturbed snow conditions) were allowed to accumulate over, for example, the 9 d period of the April 2001 event. However, the simulations of Groot Zwaaftink et al. (2016) on the substantially greater contribution of local Arctic dust (versus Asian or African dust) to dust deposition suggests that Asian and African dust would, in general, play a secondary reflectance perturbation role compared to local dust.
A second substantial impact of Arctic dust particles is associated with their role as ice-nucleating particles (INPs) and their indirect effect on cloud dynamics. The core message of Kawai et al. (2023) was not a statement about the weak optical influence of local Arctic dust, but rather a simulation-based affirmation that local dust was the dominant INP source in the lower Arctic troposphere during summer and fall. A similar statement concerning the dominance of local dust over Asian dust as INPs was made by Xi et al. (2022) based on INP (droplet-freezing) measurements made near the source of local dust plumes at the sub-Arctic Lhù'ààn Mân' (Kluane Lake) site in the Canadian Yukon territory. Barr et al. (2023) reported on the greater INP activity of local dust (from glacial drainage basins on the southern Alaskan coast) relative to Arctic dust from low-latitude sources. Tobo et al. (2019) described the important role of local dust as an INP source in the Svalbard region and noted that the high ice-nucleating ability of the local dust was likely governed by the presence of organic matter. Shi et al. (2022) analyzed the radiative forcing impacts of local Arctic dust (what they called “HLD” for high-latitude dust whose source is in the Arctic or sub-Arctic). Their simulations (roughly supported by INP comparisons with measured INPs carried out at nine stations) show, for example, that HLD INPs likely instigated a maximum depletion in the liquid water path (LWP) of mixed-phase clouds in the fall (and lesser but still significant LWP changes during the summer and winter). Those LWP depletions (∼ 8 g m−2) amount to water COD reductions of <∼ 1.5 (at any wavelength for which the Mie extinction efficiency (Q) is ∼ the optically large-particle asymptotic value of ∼ 2). Such COD changes (along with their associated extinction coefficient profile change) would be readily detected using standard passive and active satellite-based sensors (from the visible to the thermal IR).
We presented a variety of examples showing how direct RS-based estimates of CM Arctic dust were oftentimes excessively large due to a failure in separating out the contribution of CM clouds (or cloud-like optical contributions). A particular emphasis was placed on a paper by Vincent (2018), who reported an optically strong airborne dust presence in the western Canadian Arctic that was ascribed to dust of Asian origin or dust from local sources. While we do not dispute the presence of both Asian and local dust in the Arctic, the direct RS detectability of airborne dust (attributed to satellite-measured values of significantly negative BTD11–12 values) was almost surely misrepresented. While it is difficult to account for all examples of strongly negative BTD11–12, it is very unlikely that airborne dust plays a major RS role in any case other than plumes of strong DOD (>∼ 0.5). One much more likely contributor would be water clouds (or, more generally stated, water-dominated mixed-phase clouds) in the Arctic inversion layer.
The RS detectability of the impact of Arctic dust and notably Arctic dust from local drainage basin sources can, however, be of significance. Sustained dust deposition can substantially decrease the (visible to shortwave IR) snow and ice reflectance and the attendant signal measured by satellite sensors (while significantly negative BTD11–12 values represent an extremely limited area according to our event-level case studies). The substantial ice-nucleating particle (INP) role of local Arctic dust can, for example, induce significant changes in the properties of low-level mixed-phase clouds (optical depth changes < ∼ unity) that can be readily detected by active and passive RS instruments. It is clearly critical that the distinction between the RS detectability of Arctic dust versus the RS detectability of the impacts of Arctic dust be understood if we are to properly account for and model the radiative forcing impacts of dust in the climate-sensitive Arctic region.
A1 Effective radius relationships for spherical particles
The effective radius for spherical particles is defined by Hansen and Travis (1974) (HT) as
where the very last relation amounts to a definition of Deff. Equation (A1) can then be recast in terms of total particle surface and particle-volume concentration:
From Eq. (A1), the effective diameter can be recast as
with a weighted mean of D, where the weight . Ginoux (2003) argues that the shape of dust particles is, in general, better represented by prolate ellipsoids (see the following section).
A2 Computation of Deff
Kawai et al. (2023) (KA) employed Kok's particle-volume size distribution (Kok, 2011) as the basis of their multi-year simulations (ultimately it was the starting point9 of their computations of seasonally averaged particle-mass columnar densities). Kok's particle-volume size distribution (his Eq. 6) is related to his particle-number size distribution (his Eq. 5) by . We can10 recast this as
where is the volume of a spherical particle of radius and . However, CKok (= 0.144 for Kok's CN and CV values of 0.9539 and 12.62 µm, respectively) is not close to unity as would be expected for small dust particles. Equation (A3) is apparently the correct inter-distribution relationship between Kok's (Fig. 6) “normalized” number and “normalized” volume size distributions.11
However, Eq. (A2a) applied to Kok's normalized distributions gives unrealistic estimates of the effective radius (0.78 µm).12 Those normalized distributions were not defined, so we tentatively have to conclude that the normalization precluded the application of Eq. (A2a).13 Dust particles are not sufficiently large to have substantial non-sphericity effects, so one expects the departure of CKok from unity to be relatively small. Ginoux (2003) cited Okada et al. (2001) to indicate that dust particles near their source (Chinese desert sites) displayed an ellipsoid aspect ratio (λ) of ∼ 1.5 and that moderately higher values of 2 showed no significant departure from sphericity (their Fig. 5, for example, shows that simulated particle-volume distributions for λ=2 were quite close to the spherical-particle AERONET inversions for six sites near or in the desert sources of dust). Accordingly, we can, in general, treat dust particles as being approximately spherical (, and the light-gray broken-line open circles in the Supplement (“Local_dust_PSDs.xlsx”) represent the appropriate distribution14 for the employment of Eq. (A2a). This yields a Deff value of 5.40 µm (reff= 2.70 µm).
A3 DOD computations for KA's local dust particles
A3.1 DOD mass efficiency (DODm)
If V is the columnar, particle-volume abundance ρ is the dust particle density, and A is the particle-number abundance, then the particle-mass abundance (Am) in the case of the KA local dust simulations15 (or any unimodal particle-volume or particle-mass distribution) is given by
where the concept of intensive parameters averaged over a unimodal particle size distribution is discussed, for example, in O'Neill et al. (2005). If the dust extinction cross-section is σ and Q is the dust extinction efficiency, then the dust optical depth (DOD) is
Employing the mean reff of 2.7 µm from the Kok distributions (Appendix A, Sect. A2) yields for λ = 0.5 µm. Q approaches an asymptote ∼ 2.3 for values of the product 10 (Fig. 16.3 of Hinds, 1999, with mr being the real part of the refractive index) and refractive indices representative of dust.16 Employing the MITR17 density of 2.6 g cm mg m−3 yields
One can define a “DOD mass efficiency” ×104 (DOD per unit columnar mass abundance) as
A3.2 DOD extracted from KA's particle-mass abundances
KA's multi-year Am averages derived from their Fig. 1 at the position of AeF's sites that are in or near the CAA were employed to compute the DOD estimates of Table A1 below. We note that “Eureka” is meant to represent the similar environments of two Eureka sites (the 5 m elevation OPAL site and the 615 m elevation PEARL (Ridge lab) site): the resolution of KA's Am values allows no such distinction to be made for the KA simulations.
Table A1Local dust DODs derived from the Am (mass abundances) of KA's Fig. 1 (at the position of the four AeF sites within or near the CAA).
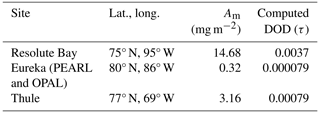
Figure A1 shows a comparison between AeF's CM AOD (geometric mean) summertime (JJA) climatology and the KA simulations. The KA simulations include no Asian dust component: their yearly means should, in principle, be ∼ the summertime AeF values (if the latter can be assumed to be dominated by local dust). The AeF CM AODs of the April and May springtime period are (as per AeF) largely dominated by Asian dust with values >∼ 0.06 (off scale in Fig. A1). We also note that PEARL and OPAL are separated by a distance of only 15 km. The simple subtraction labeled “OPAL – PEARL” in Fig. A1 is arguably a better measure of local dust DOD than their individual summertime means (assuming the local dust is largely limited to altitudes less than that of the PEARL (Ridge lab) site). The KA precision for the Resolute Bay, Thule, and “OPAL – PEARL” (Eureka) sites would then nominally be ∼ 0.001. This is a number that one hesitates to quote given the preponderance of uncertainties that plague both the simulations and the measurements (e.g., for the quality of local dust emissions about a given AeF site given the coarse KA spatial resolution of 1.9°×2.5° and the nominal AERONET AOD error ∼ 0.01–0.02 at an optical airmass of one; Eck et al., 2023). The most optimistic affirmation is arguably that the summertime dust estimates are the same order of magnitude.
A3.3 KA model “underestimation” of local DOD
Figure S4 of KA's Supplement suggests that their simulated 550 nm “annual mean zonally averaged dust AOD” significantly underestimates the local DOD relative to the CALIOP 532 nm estimate of local DOD.18 The third column of Table A2 (corresponding to the second column of Table A1) shows the DODs (τ) computed from KA's Fig. 1 at AeF's AERONET sites. The fourth column is a “correction” of KA's DODs (DOD – ΔDOD) to yield values that would account for the gap between the KA DODs and the CALIOP DODs. The result, relative to AeF's summertime estimate, is a better comparison for Eureka and Thule and a rather large overestimate for Resolute Bay. The limitation of such a “correction” is the credibility of CALIOP in classifying and estimating local DOD in the Arctic. There is no validation in the literature of CALIOP's capability in classifying local dust plumes. The conclusion of this exercise is simply that there is no reason to change the order of magnitude conclusion of the previous section.
A4 Estimation of Mount Logan DODs during the Asian dust event of April 2001
Table A3 shows visually extracted DODs from NAAPS simulations over the region of Mount Logan (Yukon) during the 11 to 19 April 2001 Asian dust event. The DOD values are the midpoints of the standard NAAPS color-scale bins. If there is no NAAPS DOD (no NAAPS dust at the position of Mount Logan), then the bin is assigned a value of 0.0.19 The arithmetic average of all the DOD values below is 〈DOD〉=0.13. This table supports the discussion above surrounding the well-documented Asian dust event of 2001 and the dust deposition consequences in the Mount Logan region.
Table A3Visually determined NAAPS DODs (https://www.nrlmry.navy.mil/aerosol-bin/aerosol/display_directory_all_t.cgi?DIR=/web/aerosol/public_html/globaer/ops_01/noramer/200104&TYPE=, last access: 10 August 2024) at Mount Logan, YK (60°34′ N, 140°24′ W), during the 11 to 19 April 2001 Asian dust event.
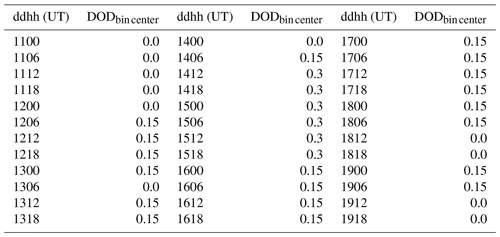
B1 MODTRAN simulations of BT11–12 vs. BT11 patterns for liquid water, ice, and dust
Figure B1 shows MODTRAN BTD11–12 vs. BT11 patterns over a “snow/ice” surface for water and ice clouds (left-hand graphs) and dust plumes (right-hand graphs) at high altitude (top graphs) and within a low-altitude inversion layer (bottom graphs). The general atmospheric conditions and cloud parameterization represent a range of values that include the specific conditions of Figs. 2a, 2b, S6, and S7 (conditions of Barrow and its surroundings on 22 March 2015). The temperatures employed in the MODTRAN simulations at the snow/ice surface, inversion-layer cloud-top, and high-altitude cloud-top were, respectively, 255.56, 262.05, and 212.66 K. These graphs provide support for all the simulation (S)-based BT11–12 vs. BT11 classifications (BTD11–12 vs. BT11 pattern characterization) of Table 1.
The optical properties of the liquid and dust particles were generated with a Mie Code (MiePlot4621, written by Philip Laven (http://www.philiplaven.com/mieplot.htm, last access: 10 August 2024)) using the refractive index of water (Hale and Querry, 1973) and dust20 for monodisperse particles. The optical properties of the ice crystals were extracted from Ping Yang's database (Yang et al., 2013) and correspond to a modified gamma distribution with effective variance of 0.1 (Petty and Huang, 2011) of severely roughened column aggregates (Yang et al., 2013). This is the same distribution that is assumed in the Collection 6 MODIS cloud product (Holz et al., 2016).
For weak DODs associated with high-altitude Asian dust, the BTD11–12 to DOD sensitivity (dBTD11–12/dDOD) would be best represented by a slope near DOD = 0 (τ= 0 on the graphs) for the case of the nearly 1.5 µm peak radius of springtime Asian dust (see, for example, the right panel of Fig. 16 in Burton et al., 2012, and Fig. 3 of AeF for the springtime Asian dust particle size distribution). This yields a value (from the detailed numerical results employed in generating these graphs) of −0.30 K per unit change in DOD.
The brightness temperatures correspond to the EOS-1 TERRA MODIS spectral response functions for bands 31 (max. at 11.0 µm) and 32 (max. at 11.9 µm), downloaded from the Satellite Application Facility for Numerical Weather Prediction (NWP SAF) website (https://nwp-saf.eumetsat.int/site/software/rttov/download/coefficients/spectral-response-functions/, last access: 10 August 2024).
A nominal noise figure for MODIS Band 31 (the 11 µm band) is 0.05 K (the cloud-discrimination ATBD of Ackerman et al., 2010). Given a roughly equivalent (incoherent) noise for Band 32 (the 12 µm band) yields a BTD11–12 noise value of 0.07 K.
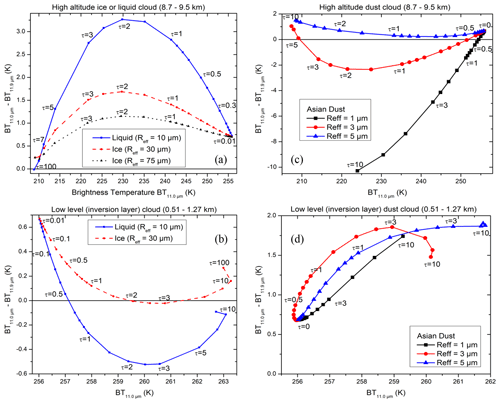
Figure B1BTD11–12 vs. BT11 simulations for different types of clouds over a “snow/ice” surface (surface of Feldman et al., 2014). Panels (a) and (b), respectively: ice and liquid cloud at a high altitude and within a low-altitude temperature inversion layer. Panels (c) and (d), respectively: Asian dust cloud at a high altitude and within a low-altitude inversion layer. The wavelength-dependent optical depth (τ) is reported at a wavelength of 550 nm in order to make a link with optical effects in the visible wavelength region. The significant curvature of the low-level red “Reff = 3 µm” curve in panel (d) suggests a balanced radiative transfer condition wherein there is little change in BTD11 with increasing cloud optical depth for values ∼0 to 0.5 (an approach to an idealistic singularity of a straight vertical line). We determined that this effect was largely due to non-linearities in the spectra of the extinction efficiency and the 550 nm referencing of the cloud optical depth (τ).
B2 Choice of refractive indices at 11 and 12 µm
The refractive indices of water droplets and ice crystals are, as per the previous section, relatively well constrained and well known. The observed dust refractive indices in the literature are principally dependent on dust composition (see, for example, Volz, 1972; Koepke et al., 1997; Rothman et al., 2009; and Sadrian et al., 2023): this dependence impacts the behavior of the BTD11–12 vs. BT11 patterns. A unique choice of refractive index based on dust composition is not possible given the diversity of dust types that characterize Asian and local dust over the Arctic (coupled with the often incomplete information on their composition).
Figure B2 illustrates the infrared refractive index and (simulated) surface emissivity (ε) spectrum of water and ice particles, as well as two distinctly different complex refractive indices of dust, for two frequently referenced citations (Koepke, 1997, with principal components of quartz and clay and Volz, 1973, with principal components of clay, illite, and kaolinite). Those different refractive indices result in significantly different emissivity spectral slopes between 11 and 12 µm.
We chose the Volz (1973) refractive indices essentially because the 11 to 12 µm spectral slope of the derived ε values was of the same sign as the ε slopes presented in VCT. This choice was underpinned by different levels of empirical and simulated evidence: the BTD11–12 vs. BT11 “convex-downward” pattern (generally indicating negative BTD11–12 values) for a normal () lapse rate (Fig. B1 and Table 1) is coherent with satellite-based Asian dust measurements (as per footnote g of Table 1), as is the negative lapse rate and attendant negative BTD11–12 across the optically thick local dust Lake Hazen plume that was discussed above. Another level of empirical evidence in the Arctic was the significantly negative BTD11–12 values in the dust emission regions of the drainage basins on Eglinton Island and Banks Island (see Fig. S8 and its caption). We also found moderately negative BTD11–12 values in areas around Lake Hazen that were clearly the result of dust deposition on snow and ice.
ABF | Essentially anthropogenic sulfate-based Arctic haze or FM aerosols of biogenic origin |
AERONET | Worldwide NASA network of combined sun photometer/sky-scanning radiometers manufactured by CIMEL Éléctronique. See AERONET website (https://aeronet.gsfc.nasa.gov/, last access: 10 August 2024) for documentation and data downloads. |
AHSRL | Arctic High Spectral Resolution Lidar |
AOD | The community uses “AOD” to represent anything from nominal aerosol optical depth, which has not been cloud-screened, to the conceptual (theoretical) interpretation of aerosol optical depth. In this paper, we use it in the latter sense and apply adjectives as required. |
Aqua | Polar-orbiting NASA satellite whose payload includes the MODIS-Aqua multi-band imager. Aqua passes south to north over the Equator in the afternoon (originally known as EOS PM-1) |
ARM | Atmospheric Radiation Measurement |
β | Backscatter cross-section (m−1 sr−1) |
BT, BTD | Brightness temperature, brightness temperature difference |
CAA | Canadian Arctic Archipelago |
CM | Coarse mode (generally referring to particles of super-micrometer radii) |
COD | Cloud optical depth |
DOD | Dust optical depth |
FM | Fine mode (generally referring to particles of sub-micrometer radii) |
HLD | High-latitude dust |
HYSPLIT | HYbrid Single-Particle Lagrangian Integrated Trajectory |
INP | Ice-nucleating particle |
KAZRGE | Ka-band ARM Zenith Radar (KAZR) (https://adc.arm.gov//metadata/html/nsakazrgeC1.b1.html, last access: 10 August 2024) general mode. Zenith-pointing Doppler radar operating at 35 GHz (8.6 mm). |
LWP | Liquid water path |
MISR | Multi-angle Imaging SpectroRadiometer |
MODIS | Moderate Resolution Imaging Spectroradiometer |
NSHSRL | North Slope High Spectral Resolution Lidar |
Terra | Terra passes from north to south across the Equator in the morning. |
TIR | Thermal infrared |
Final MATLAB codes employed in the generation of the figures are freely available (see https://doi.org/10.5281/zenodo.14201222, O'Neill et al., 2024a). (1) MODIS RGB (true color) and SWIR images were georeferenced using ENVI version 5 (Exelis Visual Information Solutions, Boulder, Colorado), and BT11 and BT11–12 images (Figs. S1 to S3) were calculated using the MATLAB code “modis_bright.m”. (2) For MISR satellite data processing (Figs. S4 and S5), we used the MISR INteractive eXplorer (MINX) software tool (https://github.com/nasa/MINX, NASA Langley Atmospheric Science Data Center, 2019). The 2D histogram in Fig. S5 was created using the MATLAB histogram plot function applied to the MISR products. (3) The MATLAB code “modis_bright.m” was also employed to create the near-Barrow BTD11–12 values in the Supplement files (BTDBarrowSummer.xlsx and BTDBarrowWinter.xlsx). This code is a revision of the code originally written by Liam Gumley and translated to MATLAB by Shaima Nasiri, both from the Space Science and Engineering Center (SSEC) at the University of Wisconsin – Madison. (4) The BT11 and BTD11–12 radiative transfer simulations in Figs. 2c and B1 were carried out usingMODTRAN 6.0 (MODerate resolution atmospheric TRANsmission; https://doi.org/10.1117/12.2050433, Berk et al., 2014). The BT11 and BTD11–12 “MODIS observations” were computed using the MATLAB code “modis_bright.m”. (5) The refractive indices of the particle types shown in Fig. B2 are available from EODG (2024), University of Oxford. The emissivity calculations in Fig. B2c were carried out using the MATLAB code “calcul_emissivity_article_Dust_Arctic.m”. (6) HSRL lidar, MPL lidar, and radar profile data for the North Slope of Alaska (NSA) site (Figs. 2a, b, and S6) are from the Atmospheric Radiation Measurement (ARM) website (ARM, 2014, https://doi.org/10.5439/1393438; ARM, 2011, https://doi.org/10.5439/1984765). (7) CALIPSO IIR data products downloaded from the ICARE Data and Service Center (https://doi.org/10.5067/CALIOP/CALIPSO/CAL_IIR_L2_Swath-Standard-V4-20, NASA/LARC/SD/ASDC, 2019), used in the generation of Fig. S7, are freely available (see https://doi.org/10.5281/zenodo.14507749, O'Neill et al., 2024b).
The supplement related to this article is available online at: https://doi.org/10.5194/acp-25-27-2025-supplement.
NTO: writing (original draft preparation and review and editing), conceptualization, methodology, investigation, formal analysis, visualization, validation, project administration, data curation, funding acquisition, and resources. KR: writing (review and editing), conceptualization, investigation, software, formal analysis, visualization, and validation. LI: writing (review and editing), conceptualization, investigation, software, formal analysis, visualization, and validation. YB: writing (review and editing), conceptualization, investigation, software, formal analysis, visualization, and validation. SAS: writing (review and editing), conceptualization, and validation. YA: writing (review and editing) and conceptualization.
The contact author has declared that none of the authors has any competing interests.
Publisher's note: Copernicus Publications remains neutral with regard to jurisdictional claims made in the text, published maps, institutional affiliations, or any other geographical representation in this paper. While Copernicus Publications makes every effort to include appropriate place names, the final responsibility lies with the authors.
This work was supported by the ESS-DA program of the Canadian Space Agency (CSA). We also gratefully acknowledge the long-standing research support provided by the Natural Sciences and Engineering Research Council of Canada (NSERC). With respect to the Barrow (Utqiagvik) North Slope Alaska (NSA) analysis, data were obtained from the Atmospheric Radiation Measurement (ARM) user facility, a U.S. Department of Energy (DOE) office of science user facility managed by the Biological and Environment Research program. Valuable in-kind support was provided by the AEROCAN network of Environment and Climate Change Canada (ECCC) and NASA AERONET. We also acknowledge the efforts of Antonis Gkikas of the National Observatory of Athens (NOA) Institute for Astronomy, Astrophysics, Space Applications and Remote Sensing (IAASARS) in providing detailed MIDAS DOD retrievals and uncertainties over the Arctic.
This research has been supported by the Canadian Space Agency (grant nos. 16SUASACIA and 21SUASACIA) and the Natural Sciences and Engineering Research Council of Canada (grant nos. RGPIN-2017-05531 and RGPIN-2023-04943).
This paper was edited by Stelios Kazadzis and reviewed by two anonymous referees.
AboEl-Fetouh, Y., O'Neill, N. T., Ranjbar, K., Hesaraki, S., Abboud, I., and Sobolewski, P. S.: Climatological-Scale Analysis of Intensive and Semi-intensive Aerosol Parameters Derived From AERONET Retrievals Over the Arctic, J. Geophys. Res.-Atmos., 125, e2019JD031569, https://doi.org/10.1029/2019jd031569, 2020.
Ackerman, S., Richard, F., Kathleen, S., Yinghui, L., Chris, M., Liam, G., Bryan, B., and Paul, M.: Discriminating clear-sky from cloud with MODIS algorithm theoretical basis document (MOD35), MODIS Cloud Mask Team, University of Wisconsin – Madison, https://modis.gsfc.nasa.gov/data/atbd/atbd_mod06.pdf (last access: 11 August 2024), 2010.
ARM – Atmospheric Radiation Measurement user facility: High Spectral Resolution Lidar (HSRL). 2015-03-22 to 2015-03-22, North Slope Alaska (NSA) Central Facility, Barrow AK (C1), Compiled by R. Bambha, E. Eloranta, J. Garcia, and B. Ermold, ARM Data Center [data set], https://doi.org/10.5439/1984765, 2011.
ARM – Atmospheric Radiation Measurement user facility: Active Remote Sensing of CLouds (ARSCL) product using Ka-band ARM Zenith Radars (ARSCLKAZRBND1KOLLIAS). 2015-03-22 to 2015-03-22, North Slope Alaska (NSA) Central Facility, Barrow AK (C1), Compiled by K. Johnson, S. Giangrande, and T. Toto, ARM Data Center [data set], https://doi.org/10.5439/1393438, 2014.
Barr, S. L., Wyld, B., McQuaid, J. B., Neely, R. R., and Murray, B. J.: Southern Alaska as a source of atmospheric mineral dust and ice-nucleating particles, Science Advances, 9, 1–12, https://doi.org/10.1126/sciadv.adg3708, 2023.
Baum, B. A., Soulen, P. F., Strabala, K. I., King, M. D., Ackerman, S. A., Menzel, W. P., and Yang, P.: Remote sensing of cloud properties using MODIS airborne simulator imagery during SUCCESS: 2. Cloud thermodynamic phase, J. Geophys. Res., 105, 11781–11792, https://doi.org/10.1029/1999JD901090, 2000.
Berk, A., Conforti, P., Kennett, R., Perkins, T., Hawes, F., and van den Bosch, J.: MODTRAN6: a major upgrade of the MODTRAN radiative transfer code, Proc. SPIE 9088, Algorithms and Technologies for Multispectral, Hyperspectral, and Ultraspectral Imagery XX, 90880H, SPIE [code], https://doi.org/10.1117/12.2050433, 2014.
Bowen, M. and Vincent, R. F.: An assessment of the spatial extent of polar dust using satellite thermal data, Sci. Rep., 11, 1–9, https://doi.org/10.1038/s41598-020-79825-7, 2021.
Bradley, R. S., Keimig, F. T., and Diaz, H. F.: Recent changes in the North American Arctic boundary layer in winter, J. Geophys. Res., 98, 8851–8858, https://doi.org/10.1029/93JD00311, 1993.
Burton, S. P., Ferrare, R. A., Hostetler, C. A., Hair, J. W., Rogers, R. R., Obland, M. D., Butler, C. F., Cook, A. L., Harper, D. B., and Froyd, K. D.: Aerosol classification using airborne High Spectral Resolution Lidar measurements – methodology and examples, Atmos. Meas. Tech., 5, 73–98, https://doi.org/10.5194/amt-5-73-2012, 2012.
de Boer, G., Eloranta, E. W., and Shupe, M. D.: Arctic mixed-phase stratiform cloud properties from multiple years of surface-based measurements at two high-latitude locations, J. Atmos. Sci., 66, 2874–2887, https://doi.org/10.1175/2009JAS3029.1, 2009.
EODG – Earth Observation Data Group: Aerosol Refractive Index Archive (ARIA), University of Oxford, data set, https://eodg.atm.ox.ac.uk/ARIA/ (last access: 11 August 2024), 2024.
Eck, T. F., Holben, B. N., Reid, J. S., Sinyuk, A., Hyer, E. J., O'Neill, N. T., Shaw, G. E., Vande Castle, J. R., Chapin, F. S., Dubovik, O., Smirnov, A., Vermote, E., Schafer, J. S., Giles, D., Slutsker, I., Sorokine, M., and Newcomb, W. W.: Optical properties of boreal region biomass burning aerosols in central Alaska and seasonal variation of aerosol optical depth at an Arctic coastal site, J. Geophys. Res.-Atmos., 114, D11201, https://doi.org/10.1029/2008JD010870, 2009.
Eck, T. F., Holben, B. N., Reid, J. S., Sinyuk, A., Giles, D. M., Arola, A., Slutsker, I., Schafer, J. S., Sorokin, M. G., Smirnov, A., Larosa, A. D., Kraft, J., Reid, E. A., Neill, N. T. O., Welton, E. J., and Menendez, A. R.: The extreme forest fires in California/Oregon in 2020: Aerosol optical and physical properties and comparisons of aged versus fresh smoke, Atmos. Environ., 305, 119798, https://doi.org/10.1016/j.atmosenv.2023.119798, 2023.
Feldman, D. R., Collins, W. D., Pincus, R., Huang, X., and Chen, X.: Far-infrared surface emissivity and climate, P. Natl. Acad. Sci. USA, 111, 16297–16302, https://doi.org/10.1073/pnas.1413640111, 2014.
Ginoux, P.: Effects of nonsphericity on mineral dust modeling, J. Geophys. Res.-Atmos., 108, 4052, https://doi.org/10.1029/2002jd002516, 2003.
Gkikas, A., Proestakis, E., Amiridis, V., Kazadzis, S., Di Tomaso, E., Tsekeri, A., Marinou, E., Hatzianastassiou, N., and Pérez García-Pando, C.: ModIs Dust AeroSol (MIDAS): a global fine-resolution dust optical depth data set, Atmos. Meas. Tech., 14, 309–334, https://doi.org/10.5194/amt-14-309-2021, 2021.
Groot Zwaaftink, C. D., Grythe, H., Skov, H., and Stohl, A.: Substantial contribution of northern high-latitude sources to mineral dust in the Arctic, J. Geophys. Res., 121, 13678–13697, https://doi.org/10.1002/2016JD025482, 2016.
Hale, G. M. and Querry, M. R.: Optical Constants of Water in the 200-nm to 200-µm Wavelength Region, Appl. Opt., 12, 555, https://doi.org/10.1364/ao.12.000555, 1973.
Hansen, J. E. and Travis, L. D.: Light scattering in planetary atmospheres, Space Sci. Rev., 16, 527–610, https://doi.org/10.1007/BF00168069, 1974.
Hess, M., Koepke, P., and Schult, I.: Optical Properties of Aerosols and Clouds: The Software Package OPAC, B. Am. Meteorol. Soc., 79, 831–844, https://doi.org/10.1175/1520-0477(1998)079<0831:OPOAAC>2.0.CO;2, 1998.
Hildner, R., Tripoli, G., Eloranta, E., and de Boer, G.: Understanding Aerosol-Cloud Interactions In Ice Saturated Environments Using CALIOP And AHSRL. Aerosols, Clouds, and Climate Session at the 90th American Meteorological Society Annual Meeting (AMS), Atlanta, Georgia, Zenodo, https://doi.org/10.5281/zenodo.14171514, 2010.
Hinds, W. C.: Aerosol technology: properties, behavior, and measurement of airborne particles. John Wiley & Sons, 483 pp., ISBN 0471194107, 1999.
Holz, R. E., Platnick, S., Meyer, K., Vaughan, M., Heidinger, A., Yang, P., Wind, G., Dutcher, S., Ackerman, S., Amarasinghe, N., Nagle, F., and Wang, C.: Resolving ice cloud optical thickness biases between CALIOP and MODIS using infrared retrievals, Atmos. Chem. Phys., 16, 5075–5090, https://doi.org/10.5194/acp-16-5075-2016, 2016.
Hsu, N. C., Gautam, R., Sayer, A. M., Bettenhausen, C., Li, C., Jeong, M. J., Tsay, S.-C., and Holben, B. N.: Global and regional trends of aerosol optical depth over land and ocean using SeaWiFS measurements from 1997 to 2010, Atmos. Chem. Phys., 12, 8037–8053, https://doi.org/10.5194/acp-12-8037-2012, 2012.
Kawai, K., Matsui, H., and Tobo, Y.: Dominant Role of Arctic Dust With High Ice Nucleating Ability in the Arctic Lower Troposphere, Geophys. Res. Lett., 50, e2022GL102470, https://doi.org/10.1029/2022GL102470, 2023.
Key, J.: The Cloud and Surface Parameter Retrieval (CASPR) System for Polar AVHRR: User's Guide, Cooperative Institute for Meteorological Satellite Studies, University of Wisconsin, 1225, 33–69, https://pubs.ssec.wisc.edu/research_Resources/publications/pdfs/SSECPUBS/SSEC_Publication_No_02_01_K1.pdf (last access: 10 August 2024), 2002.
Koepke, P., Hess, M., Schult, I., Shettle, E.P.:Global Aerosol Data Set. Report No. 243, Max-Planck-Institut für Meteorologie, Hamburg, https://doi.org/10.17617/2.3365749, 1997.
Kok, J. F.: A scaling theory for the size distribution of emitted dust aerosols suggests climate models underestimate the size of the global dust cycle, P. Natl. Acad. Sci. USA, 108, 1016–1021, https://doi.org/10.1073/pnas.1014798108, 2011.
Liu, Y., Key, J. R., Frey, R. A., Ackerman, S. A., and Menzel, W. P.: Nighttime polar cloud detection with MODIS, Remote Sens. Environ., 92, 181–194, https://doi.org/10.1016/j.rse.2004.06.004, 2004.
Meinander, O., Dagsson-Waldhauserova, P., Amosov, P., Aseyeva, E., Atkins, C., Baklanov, A., Baldo, C., Barr, S. L., Barzycka, B., Benning, L. G., Cvetkovic, B., Enchilik, P., Frolov, D., Gassó, S., Kandler, K., Kasimov, N., Kavan, J., King, J., Koroleva, T., Krupskaya, V., Kulmala, M., Kusiak, M., Lappalainen, H. K., Laska, M., Lasne, J., Lewandowski, M., Luks, B., McQuaid, J. B., Moroni, B., Murray, B., Möhler, O., Nawrot, A., Nickovic, S., O’Neill, N. T., Pejanovic, G., Popovicheva, O., Ranjbar, K., Romanias, M., Samonova, O., Sanchez-Marroquin, A., Schepanski, K., Semenkov, I., Sharapova, A., Shevnina, E., Shi, Z., Sofiev, M., Thevenet, F., Thorsteinsson, T., Timofeev, M., Umo, N. S., Uppstu, A., Urupina, D., Varga, G., Werner, T., Arnalds, O., and Vukovic Vimic, A.: Newly identified climatically and environmentally significant high-latitude dust sources, Atmos. Chem. Phys., 22, 11889–11930, https://doi.org/10.5194/acp-22-11889-2022, 2022.
Masuda, K., Takashima, T., and Takayama, Y.: Emissivity of pure and sea waters for the model sea surface in the infrared window regions, Remote Sens. Environ., 24, 313–329, https://doi.org/10.1016/0034-4257(88)90032-6, 1998.
Morrison, H., De Boer, G., Feingold, G., Harrington, J., Shupe, M. D., and Sulia, K.: Resilience of persistent Arctic mixed-phase clouds, Nat. Geosci., 5, 11–17, https://doi.org/10.1038/ngeo1332, 2012.
NASA Langley Atmospheric Science Data Center: MISR Interactive eXplorer (MINX) v4.1, GitHub [code], https://github.com/nasa/MINX (last access: 11 August 2024), 2019.
NASA/LARC/SD/ASDC: CALIPSO Infrared Imaging Radiometer (IIR) Level 2 Swath, V4–20, NASA Langley Atmospheric Science Data Center DAAC [data set], https://doi.org/10.5067/CALIOP/CALIPSO/CAL_IIR_L2_Swath-Standard-V4-20, 2019.
Okada, K., Heintzenberg, J., Kai, K., and Qin, Y.: Shape of atmospheric mineral particles collected in three Chinese arid-regions, Geophys. Res. Lett., 28, 3123–3126, https://doi.org/10.1029/2000GL012798, 2001.
O'Neill, N. T., Thulasiraman, S., Eck, T. F., and Reid, J. S.: Robust optical features of fine mode size distributions: Application to the Québec smoke event of 2002, J. Geophys. Res.-Atmos., 110, D11207, https://doi.org/10.1029/2004JD005157, 2005.
O'Neill, N. T., Baibakov, K., Hesaraki, S., Ivanescu, L., Martin, R. V., Perro, C., Chaubey, J. P., Herber, A., and Duck, T. J.: Temporal and spectral cloud screening of polar winter aerosol optical depth (AOD): impact of homogeneous and inhomogeneous clouds and crystal layers on climatological-scale AODs, Atmos. Chem. Phys., 16, 12753–12765, https://doi.org/10.5194/acp-16-12753-2016, 2016.
O'Neill, N. T., Ranjbar, K., Ivanescu, L., Blanchard, Y., Sayedain, S. A., and AboEl-Fetouh, Y.: Remote-sensing detectability of airborne Arctic dust – code, Zenodo [code], https://doi.org/10.5281/zenodo.14201222, 2024a.
O'Neill, N. T., Ranjbar, K., Ivanescu, L., Blanchard, Y., Sayedain, S. A., and AboEl-Fetouh, Y.: Remote-sensing detectability of airborne Arctic dust – dataset, Zenodo [data set], https://doi.org/10.5281/zenodo.14507749, 2024b.
Painter, T. H., Barrett, A. P., Landry, C. C., Neff, J. C., Cassidy, M. P., Lawrence, C. R., McBride, K. E., and Farmer, G. L.: Impact of disturbed desert soils on duration of mountain snow cover, Geophys. Res. Lett., 34, L12502, https://doi.org/10.1029/2007GL030284, 2007.
Palo, T., Vihma, T., Jaagus, J., and Jakobson, E.: Observations of temperature inversions over central Arctic sea ice in summer, Q. J. Roy. Meteor. Soc., 143, 2741–2754, https://doi.org/10.1002/qj.3123, 2017.
Petty, G. W. and Huang, W.: The modified gamma size distribution applied to inhomogeneous and nonspherical particles: Key relationships and conversions, J. Atmos. Sci., 68, 1460–1473, https://doi.org/10.1175/2011JAS3645.1, 2011.
Ranjbar, K., O'Neill, N. T., Ivanescu, L., King, J., and Hayes, P. L.: Remote sensing of a high-Arctic, local dust event over Lake Hazen (Ellesmere Island, Nunavut, Canada), Atmos. Environ., 246, 118102, https://doi.org/10.1016/j.atmosenv.2020.118102, 2021.
Ranjbar, K., O'Neill, N. T., and Aboel-Fetouh, Y.: Comment on “Short-cut transport path for Asian dust directly to the Arctic: a case Study” by Huang et al. (2015) in Environ. Res. Lett., Atmos. Chem. Phys., 22, 1757–1760, https://doi.org/10.5194/acp-22-1757-2022, 2022.
Rothman, L. S., Gordon, I. E., Barbe, A., Benner, D. C., Bernath, P. F., Birk, M., Boudon, V., Brown, L. R., Campargue, A., Champion, J. P., Chance, K., Coudert, L. H., Dana, V., Devi, V. M., Fally, S., Flaud, J. M., Gamache, R. R., Goldman, A., Jacquemart, D., Kleiner, I., and Vander Auwera, J.: The HITRAN 2008 molecular spectroscopic database, J. Quant. Spectrosc. Ra., 110, 533–572, https://doi.org/10.1016/j.jqsrt.2009.02.013, 2009.
Sadrian, M. R., Calvin, W. M., Perrin, A. E., Engelbrecht, J. P., and Moosmüller, H.: Variations in Infrared Complex Refractive Index Spectra of Surface Soils from Global Dust Entrainment Regions, Atmosphere, 14, 675, https://doi.org/10.3390/atmos14040675, 2023.
Shi, Y., Liu, X., Wu, M., Zhao, X., Ke, Z., and Brown, H.: Relative importance of high-latitude local and long-range-transported dust for Arctic ice-nucleating particles and impacts on Arctic mixed-phase clouds, Atmos. Chem. Phys., 22, 2909–2935, https://doi.org/10.5194/acp-22-2909-2022, 2022.
Shonk, J. K. P., Chiu, J.-Y. C., Marshak, A., Giles, D. M., Huang, C.-H., Mace, G. G., Benson, S., Slutsker, I., and Holben, B. N.: The impact of neglecting ice phase on cloud optical depth retrievals from AERONET cloud mode observations, Atmos. Meas. Tech., 12, 5087–5099, https://doi.org/10.5194/amt-12-5087-2019, 2019.
Shupe, M. D., Turner, D. D., Zwink, A., Thieman, M. M., Mlawer, E. J., and Shippert, T.: Deriving arctic cloud microphysics at Barrow, Alaska: Algorithms, results, and radiative closure, J. Appl. Meteorol. Clim., 54, 1675–1689, https://doi.org/10.1175/JAMC-D-15-0054.1, 2015.
Stone, R. S., Anderson, G. P., Andrews, E., Dutton, E. G., Shettle, E. P., and Berk, A.: Incursions and radiative impact of Asian dust in northern Alaska, Geophys. Res. Lett., 34, L14815, https://doi.org/10.1029/2007GL029878, 2007.
Stone, R. S., Sharma, S., Herber, A., Eleftheriadis, K., and Nelson, D. W.: A characterization of Arctic aerosols on the basis of aerosol optical depth and black carbon measurements, Elementa, 2, 1–22, https://doi.org/10.12952/journal.elementa.000027, 2014.
Tobo, Y., Adachi, K., DeMott, P. J., Hill, T. C. J., Hamilton, D. S., Mahowald, N. M., Nagatsuka, N., Ohata, S., Uetake, J., Kondo, Y., and Koike, M.: Glacially sourced dust as a potentially significant source of ice nucleating particles, Nat. Geosci., 12, 253–258, https://doi.org/10.1038/s41561-019-0314-x, 2019.
Thulasiraman, S., O'Neill, N. T., Royer, A., Holben, B. N., Westphal, D. L., and McArthur, L. J. B.: Sunphotometric observations of the 2001 Asian duststorm over Canada and the U.S., Geophys. Res. Lett., 29, 1255, https://doi.org/10.1029/2001GL014188, 2002.
Vincent, R. F.: The Effect of Arctic Dust on the Retrieval of Satellite Derived Sea and Ice Surface Temperatures, Sci. Rep., 8, 6–15, https://doi.org/10.1038/s41598-018-28024-6, 2018.
Volz, F. E.: Infrared Refractive Index of Atmospheric Aerosol Substances. Appl. Opt., 11, 755, https://doi.org/10.1364/ao.11.000755, 1972.
Volz, F. E.: Infrared Optical Constants of Ammonium Sulfate, Sahara Dust, Volcanic Pumice, and Flyash, Appl. Opt., 12, 564, https://doi.org/10.1364/ao.12.000564, 1973.
Woo, M. K., Edlund, S. A., and Young, K. L.: Occurrence of early snow-free zones on Fosheim peninsula, Ellesmere Island, northwest territories, Curr. Res. Part B, Geol. Surv. Canada Pap. 91, 9–14, https://publications.gc.ca/collections/collection_2017/rncan-nrcan/M44-91-1B.pdf (last access: 10 August 2024), 1991.
Xi, Y., Xu, C., Downey, A., Stevens, R., Bachelder, J. O., King, J., Hayes, P. L., and Bertram, A. K.: Ice nucleating properties of airborne dust from an actively retreating glacier in Yukon, Canada, Environ. Sci.-Atmos., 2, 714–726, https://doi.org/10.1039/d1ea00101a, 2022.
Xian, P., Zhang, J., O'Neill, N. T., Toth, T. D., Sorenson, B., Colarco, P. R., Kipling, Z., Hyer, E. J., Campbell, J. R., Reid, J. S., and Ranjbar, K.: Arctic spring and summertime aerosol optical depth baseline from long-term observations and model reanalyses – Part 1: Climatology and trend, Atmos. Chem. Phys., 22, 9915–9947, https://doi.org/10.5194/acp-22-9915-2022, 2022.
Yang, P., Bi, L., Baum, B. A., Liou, K. N., Kattawar, G. W., Mishchenko, M. I., and Cole, B.: Spectrally consistent scattering, absorption, and polarization properties of atmospheric ice crystals at wavelengths from 0.2 to 100 µm, J. Atmos. Sci., 70, 330–347, https://doi.org/10.1175/JAS-D-12-039.1, 2013.
Yi, L., Li, K. F., Chen, X., and Tung, K. K.: Arctic fog detection using infrared spectral measurements, J. Atmos. Ocean. Tech., 36, 1643–1656, https://doi.org/10.1175/JTECH-D-18-0100.1, 2019.
Zamora, L. M., Kahn, R. A., Evangeliou, N., Groot Zwaaftink, C. D., and Huebert, K. B.: Comparisons between the distributions of dust and combustion aerosols in MERRA-2, FLEXPART, and CALIPSO and implications for deposition freezing over wintertime Siberia, Atmos. Chem. Phys., 22, 12269–12285, https://doi.org/10.5194/acp-22-12269-2022, 2022.
Zdanowicz, C., Hall, G., Vaive, J., Amelin, Y., Percival, J., Girard, I., Biscaye, P., and Bory, A.: Asian dustfall in the St. Elias Mountains, Yukon, Canada, Geochim. Cosmochim. Ac., 70, 3493–3507, https://doi.org/10.1016/j.gca.2006.05.005, 2006.
Zhang, P., Lu, N. M., Hu, X. Q., and Dong, C. H.: Identification and physical retrieval of dust storm using three MODIS thermal IR channels, Global Planet. Change, 52, 197–206, https://doi.org/10.1016/j.gloplacha.2006.02.014, 2006.
Zhao, X., Huang, K., Fu, J. S., and Abdullaev, S. F.: Long-range transport of Asian dust to the Arctic: identification of transport pathways, evolution of aerosol optical properties, and impact assessment on surface albedo changes, Atmos. Chem. Phys., 22, 10389–10407, https://doi.org/10.5194/acp-22-10389-2022, 2022.
Zuidema, P., Baker, B., Han, Y., Intrieri, J., Key, J., Lawson, P., Matrosov, S., Shupe, M., Stone, R., and Uttal, T.: An Arctic springtime mixed-phase cloudy boundary layer observed during SHEBA, J. Atmos. Sci., 62, 160–176, https://doi.org/10.1175/JAS-3368.1, 2005.
Note that, unless otherwise stated, our AODs and DODs will always be referenced to a wavelength of 550 nm.
See Sect. A4 for details on this well-documented event.
More typical of average spring-to-fall local DODs if one excludes the springtime Asian-dust-dominated DODs (see Sect. A3.2).
The reader will note that, for the purposes of this paper, we do not distinguish between liquid clouds and mixed-phase clouds. Below, we argue that the ice COD in mixed-phase clouds is typically negligible compared to the liquid water COD.
With a plume thickness of < ∼ 600 m. These are the 3-year results for Eureka: the single Barrow year of 2004, with a SON occurrence of 26 %, was more coherent with the Shupe et al. (2015) results.
As well as simulations and empirical evidence from the literature.
Compare with the “Dusty Snow” reflectance changes in the Fig. 1a spectra of Painter et al. (2007).
Those 2 intensive-analysis days were supplemented by neighboring days for which CM nephelometer measurements suggested the dust event extended beyond those 2 core days.
The emission (source) particle-volume size distribution.
Dropping his “d” (dust) subscript, using lowercase letters for these point volume parameters and and for their “normalized” distributions.
As verified by the fact that the black solid-line open-circle curve is very close to Kok's gold solid-line full-circle curve in the Supplement (“Local_dust_PSDs.xlsx”).
Versus, for example, a volume-weighted geometric mean diameter (VMD) of 6.51 µm (the AERONET inversion type of calculation).
Meaning that Kok's normalized distributions were not equally proportional to their physical representations (the physical representations being symbolized by the hatless variables in his section).
Meaning that we treat the distributions as being spherical-particle distributions.
The parameter that they call “vertically integrated …mass concentrations”.
1.53–0.0078i for the MITR (“mineral transported”) class of dust (the “opdat” directory of the OPAC package). The MITR density of Hess et al. (1998) transforms as 2.6 g cm 2.6×103 mg (10−2 m)−3 mg m−3.
Optical and µphysical parameters are listed in Table 1c of Hess et al. (1998).
Underestimates by a bias which we label ΔDOD. There are, for example, biases of ΔDOD ∼ −0.005 at the 75° N latitude of Resolute Bay and ∼ −0.003 near the 80° N latitude of Eureka and Thule: these values were estimated from KA's Fig. S4 ((−1) × [CALIOP curve – red KA simulation curve]).
Or a value of 0.15 if the edge of the 0.15-valued (yellow) colored plume cannot be visually separated from the position of Mount Logan.
See Sect. B2 for a discussion of our choice of dust refractive index.
- Abstract
- Introduction
- Arctic aerosol events that are readily detected by remote sensing
- Negative BTDs associated with liquid-phase clouds in the inversion layer
- RS detectability of dust impacts
- Conclusions
- Appendix A: Intensive and extensive microphysical and optical parameters of local and Asian dust
- Appendix B: Computational details in support of Table 1
- Appendix C: Acronym and symbol glossary
- Code and data availability
- Author contributions
- Competing interests
- Disclaimer
- Acknowledgements
- Financial support
- Review statement
- References
- Supplement
- Abstract
- Introduction
- Arctic aerosol events that are readily detected by remote sensing
- Negative BTDs associated with liquid-phase clouds in the inversion layer
- RS detectability of dust impacts
- Conclusions
- Appendix A: Intensive and extensive microphysical and optical parameters of local and Asian dust
- Appendix B: Computational details in support of Table 1
- Appendix C: Acronym and symbol glossary
- Code and data availability
- Author contributions
- Competing interests
- Disclaimer
- Acknowledgements
- Financial support
- Review statement
- References
- Supplement