the Creative Commons Attribution 4.0 License.
the Creative Commons Attribution 4.0 License.
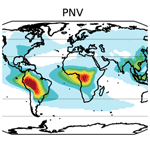
Influence of land cover change on atmospheric organic gases, aerosols, and radiative effects
Matthew Forrest
Andrea Pozzer
Alexandra P. Tsimpidi
Thomas Hickler
Jos Lelieveld
Holger Tost
Biogenic volatile organic compounds (BVOCs) are emitted in large quantities from the terrestrial biosphere and play a significant role in atmospheric gaseous and aerosol compositions. Secondary organic aerosols (SOAs) resulting from BVOC oxidation affect the radiation budget both directly, through the scattering and absorption of sunlight, and indirectly, by modifying cloud properties. Human activities have extensively altered natural vegetation cover, primarily by converting forests into agricultural land. In this work, a global atmospheric chemistry–climate model, coupled with a dynamic global vegetation model, was employed to study the impacts of perturbing the biosphere through human-induced land use change, thereby exploring changes in BVOC emissions and the atmospheric aerosol burden. A land use scheme was implemented to constrain tree plant functional type (PFT) cover based on land transformation fraction maps from the year 2015. Two scenarios were evaluated: (1) one comparing present-day land cover, which includes areas deforested for crops and grazing land, with potential natural vegetation (PNV) cover simulated by the model, and (2) an extreme reforestation scenario in which present-day grazing land is restored to natural vegetation levels. We find that, compared to the PNV scenario, present-day deforestation results in a 26 % reduction in BVOC emissions, which decreases the global biogenic SOA (bSOA) burden by 0.16 Tg (a decrease of 29 %), while the total organic aerosol (OA) burden decreases by 0.17 Tg (a reduction of 9 %). On the other hand, the extreme reforestation scenario, compared to present-day land cover, suggests an increase in BVOC emissions of 22 %, which increases the bSOA burden by 0.11 Tg and the total OA burden by 0.12 Tg – increases of 26 % and 6 %, respectively. For the present-day deforestation scenario, we estimate a positive total radiative effect (aerosol + cloud) of 60.4 mW m−2 (warming) relative to the natural vegetation scenario, while for the extreme reforestation scenario, we report a negative (cooling) effect of 38.2 mW m−2 relative to current vegetation cover.
- Article
(6140 KB) - Full-text XML
-
Supplement
(12601 KB) - BibTeX
- EndNote
Human activities have significantly modified natural vegetation cover, primarily through the conversion of forests into agricultural land. It is estimated that approximately half of the Earth's land surface has been affected by human activities (Hurtt et al., 2011). Land cover change (LCC) has a substantial impact on the Earth's system as the biosphere plays a central role in major biophysical and biogeochemical cycles, as well as in feedbacks with the atmosphere (Bonan, 2008). Forests store 45 % of the Earth's terrestrial carbon and can sequester large amounts of carbon (Field and Raupach, 2004). Furthermore, forests play a significant role in sustaining the hydrological cycle through evapotranspiration, which influences cloud formation; the onset of precipitation; and, consequently, surface temperatures (Betts et al., 2004; Vicente-Serrano et al., 2015). Land cover plays a crucial role in surface albedo, with dense forests being capable of absorbing up to 90 % of solar radiation (Forster et al., 2007). At high latitudes, forests can mask the high albedo of snow, leading to planetary warming through increased solar heating of the land (Bonan, 2008).
The terrestrial biosphere is also the primary source of emissions of biogenic volatile organic compounds (BVOCs), such as isoprene and various terpenes, accounting for around 90 % of the total volatile organic compounds (VOCs) emitted into the atmosphere (Guenther et al., 1995). Reported global emissions of isoprene, the primary BVOC, span a range of 412 to 682 Tg yr−1 (e.g. Sindelarova et al., 2014; Guenther et al., 2006; Vella et al., 2023a). BVOCs are highly reactive and short-lived, with lifetimes typically ranging from minutes to hours. Upon emission, they rapidly interact with tropospheric oxidant gases, thereby exerting a substantial influence on the oxidation capacity of the atmosphere (Lelieveld et al., 2008; Atkinson, 2000; Atkinson and Arey, 2003).
The short lifespan of BVOCs stems from their rapid oxidation upon release from the canopy. This oxidation primarily involves the OH radical, as well as other key oxidising agents, such as O3 and NO3 radicals (Shrivastava et al., 2017). These reactions produce various lower-volatility oxidation products that tend to partition into the aerosol phase, leading to the formation of biogenic secondary organic aerosols (bSOAs) (Kavouras et al., 1998; Spracklen et al., 2011).
Isoprene-derived oxidation products mainly contribute by condensing onto existing aerosols (aerosol growth), whereas oxidation products from monoterpenes, despite being less abundant than those from isoprene, play a crucial role in generating new particles (nucleation) (Jokinen et al., 2015). As a result, monoterpene precursors may have distinct climate impacts due to their influence on aerosol numbers. Through condensational growth, bSOAs participate in the absorption and scattering of solar shortwave radiation, contributing to aerosol–radiation interactions (ARIs). Furthermore, newly formed bSOA particles can grow to sufficient sizes to be activated as cloud droplets, thereby modifying cloud properties, such as albedo and lifetime, and effectively contributing to aerosol–cloud interactions (ACIs) (Forster et al., 2007) In this study, we do not include organic new-particle formation (NPF) and focus only on the role of organic precursors in supporting aerosol condensational growth.
BVOC emission rates are inherently linked to land cover, and LCC can ultimately affect the climate system by influencing short-lived climate forcers, e.g. aerosols (Scott et al., 2014). In this study, we investigate the changes in BVOC emissions resulting from cropland and grazing-land expansion on potential natural vegetation (PNV). PNV refers to the type of vegetation that would naturally occur in a specific area under certain climate, soil, and environmental conditions without human influence. We use the chemistry–climate model EMAC (ECHAM/MESSy (Modular Earth Submodel System) Atmospheric Chemistry), coupled with the dynamic global vegetation model (DGVM) LPJ-GUESS. The coupling is one-way, i.e. vegetation information in LPJ-GUESS is driven by climate states from EMAC, but feedbacks from vegetation to the atmosphere are suppressed, except with respect to changes in BVOC emission rates. Thus, we focus on quantifying the aerosol burden and radiative effects driven purely by BVOC emissions resulting from LCC, without accounting for changes in surface albedo, roughness length, or the hydrological cycle. Land cover change is simulated through a deforestation routine in LPJ-GUESS (which simulates PNV), systematically clearing cropland and grazing-land areas based on land cover data for 2015.
2.1 The EMAC modelling system
The EMAC (ECHAM/MESSy Atmospheric Chemistry) model is a numerical chemistry–climate modelling system that includes submodels that represent tropospheric and middle-atmospheric processes, as well as their interactions with oceans, land, and human activities. It originally combined ECHAM, an atmospheric general circulation model (GCM) (Roeckner et al., 2006), with the Modular Earth Submodel System (MESSy) (Jöckel et al., 2005) framework and philosophy, modularising physical processes and most of the infrastructure into submodels that can be further developed to improve existing process representations. New submodels can also be added to represent new or alternative process representations.
Aerosols are treated using the submodel GMXe (Pringle et al., 2010), in which aerosol microphysics are characterised by seven interactive log-normal modes that span the typical size range of aerosol species. These modes are further categorised into four hydrophilic aerosol modes (nucleation, Aitken, accumulation, and coarse) and three hydrophobic aerosol modes (Aitken, accumulation, and coarse). The representation of all aerosols assumes that particles are spherical. The properties of aerosols in each mode are fully determined by the total mass (the internal mixture of contributing species), density, number concentration, median radius, and width of the log-normal distribution. After each simulation step, aerosols may transfer between modes depending on size changes.
Organic aerosol species are additionally described by ORACLE, a submodel for the description of organic aerosol composition and evolution (Tsimpidi et al., 2014), taking into account the partitioning between aerosols and the gas phase based on the volatility basis set (VBS) framework (Donahue et al., 2006). ORACLE describes the following organic aerosols (OAs): secondary organic aerosols from the oxidation of anthropogenic VOCs (aSOAs) and biogenic VOCs (bSOAs); primary organic aerosols from emissions arising from fossil fuels (fPOAs) and biofuel combustion and biomass burning (bbPOAs); and secondary organic aerosols arising from the subsequent photochemical oxidation of fPOAs and bbPOAs (fSOAs and bbSOAs, respectively) (Tsimpidi et al., 2016, 2017). ORACLE treats mass yields of secondary organic aerosol (SOA) with respect to different lumped VOCs at varying levels of saturation concentration (C*), expressed in µg m−3, at 298 K, based on an assumed particle density of 1.5 g cm−3. Isoprene exhibits mass yields across the range of C*, with a peak of 0.03 at 10 µg m−3, before declining sharply to 0.015 at 100 µg m−3 and reaching zero at 1000 µg m−3. Monoterpenes show significantly higher yields, starting at 0.107 for a low value of C* (1 µg m−3) and peaking at 0.600 for a higher value of C* (1000 µg m−3), highlighting the much greater contribution of monoterpenes to SOA formation, especially under conditions of higher saturation concentration. More details on SOA mass yields in ORACLE can be found in Tsimpidi et al. (2014).
ORACLE employs a simple photochemical-ageing scheme that effectively models the combined impacts of the fragmentation and functionalisation of organic compounds. The module not only predicts the mass concentration of organic aerosol (OA) components but also predicts their oxidation state (expressed as O : C), enabling their categorisation into primary OAs (POAs – chemically unprocessed); freshly formed secondary OAs (SOAs), exhibiting a low oxygen content; and aged SOAs (highly oxygenated). By explicitly simulating the chemical conversion of OA from initial emissions to a highly oxygenated state during photochemical ageing, ORACLE facilitates the tracking of changes in OA hygroscopicity resulting from these reactions. This enables the computation of the OA particle's capability to serve as cloud condensation nuclei. The output from the ORACLE model, based on the described setup, has been compared with observational data from tropical regions (Hewitt et al., 2010; de Sá et al., 2019; Chen et al., 2009; Schmale et al., 2013; Tiitta et al., 2014; Zhang et al., 2010). We found that ORACLE provides surface OA concentrations within 60 % of the observed values recorded over these tropical forest regions. A thorough evaluation of ORACLE against aerosol mass spectrometer (AMS) measurements is provided in Tsimpidi et al. (2016). Details on the implementation of ORACLE (v2.0) in EMAC, along with a comprehensive model evaluation, can be found in Tsimpidi et al. (2018).
GMXe treats new-particle formation (NPF) based on temperature, relative humidity, and sulfuric acid (H2SO4) concentration (Vehkamäki et al., 2002). In this setup, organics do not contribute to NPF but rather participate in condensation through the VBS framework in ORACLE (as described above), where volatilities are governed by the oxidation of organic precursors.
Heterogeneous and gas-phase chemistry are treated through the MECCA submodel (Sander et al., 2019), employing version 1 of the Mainz Isoprene Mechanism (MIM1) as the chemical mechanism (Pöschl et al., 2000; Jöckel et al., 2006), which includes over 100 gas-phase species and more than 250 reactions. MIM1 includes the following BVOC oxidation pathways: isoprene + OH, isoprene + O3, and monoterpene oxidation (lumped species) with OH, O3, NO3, and O(1D). Dry-deposition, sedimentation, and wet-deposition processes are simulated using the submodels DDEP, SEDI (both Kerkweg et al., 2006), and SCAV (Tost et al., 2006a), respectively.
Convective cloud processes are taken into account based on the approach proposed by Tost et al. (2006b), utilising the convection schemes from Tiedtke (1989) and Nordeng (1994). Convective cloud microphysics is solely based on temperature and moisture profiles and does not account for the influence of aerosols on liquid droplet or ice formation processes. The vertical velocity distribution used for aerosol activation by grid-scale clouds in EMAC is calculated as the sum of the grid's mean vertical velocity and the turbulent contribution, as detailed by Brinkop and Roeckner (1995). Large-scale stratiform clouds are described by the CLOUD submodel, which, in the applied configuration, incorporates a two-moment cloud microphysics scheme for cloud droplets and ice crystals, as detailed by Lohmann et al. (1999, 2007), Lohmann and Ferrachat (2010), and Lohmann and Kärcher (2002). CLOUD solves prognostic equations for specific humidity, the liquid cloud mixing ratio, the ice cloud mixing ratio, cloud droplet number concentration (CDNC), and ice crystal number concentration (ICNC).
CLOUD incorporates a prognostic cloud droplet nucleation process to represent aerosol–cloud interactions in large-scale clouds (excluding warm convective clouds) using aerosol information provided by GMXe. This prognostic nucleation scheme is based on an aerosol activation parameterisation called the “unified dust activation framework” (UAF). The UAF is based on the cloud activation parameterisation from Nenes and Seinfeld (2003) and includes dust–pollutant interactions during the activation process by taking into account the hydrophilicity of the dust using an adsorption theory, as well as the acquired hygroscopicity through Köhler theory. This routine provides values for the hygroscopicity parameter (κ) and the cloud condensation nuclei (CCN) number concentration at levels of 0.2 % and 0.4 % supersaturation for each aerosol size mode (Pringle et al., 2010).
For the evaluation of the radiative effects from clouds and aerosols, we employ methods from Ghan (2013). The calculation of the net radiative effect from aerosol–radiation interactions (REari) involves determining the difference between the net top-of-the-atmosphere shortwave radiative flux (F) and the radiative flux, excluding the scattering and absorption of solar radiation caused by the aerosols (Fclean). Fclean is computed in a separate radiation call within the radiation submodel RAD. Similarly, the radiative effect resulting from aerosol–cloud interactions (REaci) is derived by assessing the difference between Fclean and the flux that disregards the scattering and absorption caused by both clouds and aerosols (Fclear-sky, clean).
EMAC includes land surface and vegetation models, enabling comprehensive studies of vegetation–atmosphere interactions. The Lund–Potsdam–Jena General Ecosystem Simulator (LPJ-GUESS) was the first vegetation model integrated into EMAC (Forrest et al., 2020; Vella et al., 2023a), and more recently, the Jena Scheme for Biosphere–Atmosphere Coupling in Hamburg (JSBACH) has also been incorporated as a submodel (Martin et al., 2024), further expanding EMAC’s capacity to simulate land surface processes. In this work, we rely exclusively on LPJ-GUESS for vegetation calculations.
LPJ-GUESS
LPJ-GUESS (Smith et al., 2001, 2014) is a dynamic global vegetation model (DGVM) that employs an individual-based approach to modelling vegetation dynamics. These dynamics are simulated as the emergent outcome of plant growth and competition for light, space, and soil resources among woody-plant individuals and a herbaceous understorey in each of the replicate patches (50 in this study), representing random samples of each simulated locality or grid cell. The simulated plants are classified into 12 plant functional types (PFTs), distinguished by growth form; phenology; photosynthetic pathways (C3 or C4); bioclimatic limits for establishment and survival; and, for woody PFTs, allometry and life history strategy. The LPJ-GUESS version used in this study (v4.0) currently provides information on potential natural vegetation (PNV), and it does not incorporate LCC. In this work, however, a custom deforestation routine was integrated to constrain PNV using deforestation maps. The deforestation maps consist of values ranging from 0 to 1, where a value of 1 signifies complete deforestation within the respective grid cell. The routine eliminates the tree PFTs after every simulated year and prevents trees from establishing in the specified areas. This implementation allows us to constrain the vegetation cover and address the research questions presented in this work. However, the latest version of LPJ-GUESS (v4.1) features a more advanced land cover scheme, which will be incorporated into our current LPJ-GUESS version in future developments.
2.2 EMAC–LPJ-GUESS configuration
In this work, we use the standard coupled configuration EMAC–LPJ-GUESS, in which the vegetation in LPJ-GUESS is entirely determined by the EMAC atmospheric state, soil type, N deposition, and CO2 fluxes (Forrest et al., 2020); however, there is no feedback from the vegetation that influences the climate variables, except with respect to terrestrial BVOC emissions. The roughness length and albedo are kept as constant background values. The albedo is derived from satellite climatologies, while the roughness length is based on subgrid-scale orography and satellite-derived vegetation climatology. Vegetation changes do not feed back to the hydrological cycle. We use the native bucket model from ECHAM5, which employs fixed climatological vegetation (Hagemann and Dümenil Gates, 2002). In this setup, BVOCs are interactive tracers that can be oxidised to form secondary organic aerosols. This means that BVOCs can influence the oxidant chemistry of the atmosphere; however, we do not quantify such impacts in this work and instead focus solely on aerosol changes.
After each simulation day, EMAC computes average daily values for 2 m temperature, net downward shortwave radiation, and total precipitation and passes these state variables to LPJ-GUESS. Vegetation information (i.e. the leaf area index, foliar density, leaf area density distribution, and PFT fractional coverage) from LPJ-GUESS is then fed back into EMAC for the calculation of BVOC emission fluxes using EMAC's BVOC submodels. In this study, the BVOC fluxes in EMAC are calculated using version 2.04 of the Model of Emissions of Gases and Aerosols from Nature (MEGAN) (Guenther et al., 2006).
MEGAN is based on the work of Guenther et al. (1993, 1995), in which BVOC emission fluxes are calculated as a function of PFT-specific emission factors and non-dimensional activity factors. These activity factors consider sensitivities to the canopy environment, including parameters such as leaf area index (LAI), temperature, light, and leaf age. Notably, the current setup does not incorporate sensitivity to soil moisture. The “parameterised canopy environment emission activity” (PCEEA) algorithm is used, rather than an alternative detailed canopy environment model that calculates light and temperature values at each canopy depth. The PCEEA algorithm calculates the light sensitivity within the canopy as a function of the daily average above-canopy photosynthetic photon flux density (PPFD), the solar angle, and a non-dimensional factor describing PPFD transmission through the canopy.
This setup employs BVOC–aerosol–vegetation feedbacks, making vegetation and BVOC emissions sensitive to changes in temperature and above-canopy radiation (excluding diffused radiation) resulting from aerosol interactions. Nevertheless, these feedbacks are minimised by nudging meteorological fields towards observations. BVOC emissions from this model setup were evaluated and applied in other studies (e.g. Vella et al., 2023a, b).
2.3 Experimental design
The land cover scenarios were derived from version 3.2 of the History Database of the Global Environment (HYDE v3.2) (Klein Goldewijk et al., 2017). HYDE provides a wide range of land use products, encompassing both historical and projected data. To ensure an accurate representation, we relied on HYDE's cropland and grazing-land products from 2015, derived from high-resolution satellite data. These products were transformed into deforestation fraction maps (Fig. 1) to constrain the vegetation in the model. Three experiments were conducted to assess the impact of human-induced LCC on the natural land biosphere and atmospheric composition. The initial model run used simulated PNV without any deforestation. Additionally, two more model runs were conducted, incorporating deforestation. The first scenario aims to represent present-day land cover resulting from deforestation for cropland and grazing land. This is referred to as the scenario for deforested cropland and grazing land, i.e. the DCGL scenario (Fig. 1a). The DCGL scenario will sometimes be referred to as present-day deforestation or present-day land cover. The second scenario involves deforestation exclusively affecting cropland and is referred to as the deforested cropland (DCL) scenario (Fig. 1b). We use the DCL scenario to evaluate the potential impact of restoring all grazing land back to its natural state, essentially creating an extreme afforestation scenario while maintaining present-day croplands for agricultural food production.
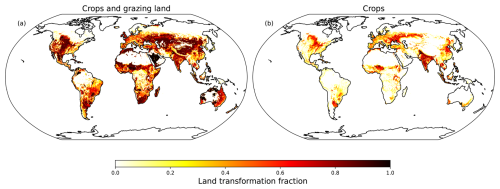
Figure 1Deforestation maps used for the deforestation tree PFTs in LPJ-GUESS. The products are derived from HYDE v3.2 based on data from the year 2015.
All simulations were conducted over 12 years (2000–2011), with the initial 2 years excluded from the analysis to ensure proper spin-up and equilibrium states in the analysed data. The initial vegetation states for all simulations were taken from a previous non-chemistry 50-year run under similar atmospheric states. For this study, the simulations were performed at a T63L31 resolution, i.e. at a resolution of approximately 1.9° × 1.9° (or approx. 180×180 km at the Equator) with 31 vertical levels. The meteorological fields in the troposphere were nudged towards ERA-Interim reanalysis data (Dee et al., 2011) for the years 2000–2012. Nudging the meteorology is important for preventing deviations in our simulations caused by feedbacks related to temperature and dynamics. By implementing nudging, these feedbacks are effectively suppressed, enabling us to evaluate changes in atmospheric states solely due to perturbed BVOC emissions resulting from land cover change.
The corresponding forcing at the sea surface (sea surface temperature (SST) and sea ice coverage (SIC)) is also inferred from the nudging data with continuous variation. Tracers were initialised using climatological data from previous simulations spanning the years from 2000 to 2020, while the CO2 concentrations in the radiation and vegetation schemes were kept fixed at 384 ppmv, representing the year 2015.
Greenhouse gas mixing ratios, including N2O, CH4, CO2, halons, and H2, were prescribed at the surface level using data from the Chemistry-Climate Model Initiative (CCMI) for the year 2015 (Eyring et al., 2013). Stratospheric H2SO4 mixing ratios were derived from a time series provided by the CCMI database. Biomass-burning emissions were simulated by the BIOBURN submodel, which imports dry-matter data from version 4.1 of the Global Fire Emissions Database (GFED4.1s) (Randerson et al., 2018) and employs emission factors from Andreae (2019), also based on the year 2015. Anthropogenic emissions of black carbon (BC), carbon monoxide (CO), nitrogen oxides (NOx), organic carbon (OC), sulfur dioxide (SO2), alcohols, and organic gases were based on the Copernicus Atmosphere Monitoring Service (CAMS-GLOB-ANT v4.2 and CAMS-GLOB-AIR v1.1) (Granier et al., 2019). Degassing volcanic-climatology data were obtained from the Aerosol Comparisons between Observations and Models (AeroCom) project (Dentener et al., 2006). Oceanic emissions and deposition were calculated online using the AIRSEA submodel (Pozzer et al., 2006; Lana et al., 2011; Fischer et al., 2012) for dimethyl sulfide (DMS), acetone (CH3COCH3), methanol (CH3OH) (with an under-saturation of 6 %), and isoprene (C5H8). Natural emissions of ammonia (NH3) were based on the Global Emissions Initiative (GEIA) database (Bouwman et al., 1997). Biogenic nitrogen oxide (NO), sea salt (Guelle et al., 2001), and dust emissions (Klingmüller et al., 2018) were calculated online with the aid of the ONEMIS submodel (Kerkweg et al., 2006), using corresponding climate states from EMAC. We note that all scenarios (PNV, DCGL, and DCL) use the same meteorology and emissions as described above. This means we do not account for past or future atmospheric forcing on vegetation and BVOC emissions (e.g. temperature changes). Instead, we focus only on the impact of perturbed BVOC emissions in a present climate state.
3.1 Present-day land cover
This section explores changes in the natural land biosphere caused by human deforestation with respect to crops and grazing land, based on HYDE land use data for 2015. We demonstrate how these alterations in the biosphere propagate to the atmosphere, affecting BVOC surface fluxes and impacting the aerosol burden and other atmospheric states.
3.1.1 Changes in vegetation states
Figure 2 shows the PNV scenario's spatial distribution of the vegetation fraction for tree (Fig. 2a) and grass (Fig. 2b) PFTs. The vegetation fraction refers to the area covered by vegetation per unit of ground area and is not to be confused with the leaf area index. Panels (c) and (d) show the changes in tree and grass PFTs, respectively, for the deforestation (DCGL−PNV) scenario. As illustrated in Fig. 2c, the deforestation routine implemented in LPJ-GUESS effectively prevents tree establishment in the transformed regions outlined in Fig. 1a, resulting in the dominance of grass PFTs in these areas (Fig. 2d). Present-day land cover decreases global tree coverage by 1026 Mha (million hectares), whereas it expands grass coverage by 953 Mha. We find that the global carbon biomass, defined as the amount of functional tissue in land vegetation (including roots), decreases from 567 PgC in the PNV scenario to 503 PgC in the DCGL scenario. This indicates that the present-day land biosphere has lost 64 PgC compared to natural vegetation levels (Fig. S1 in the Supplement).
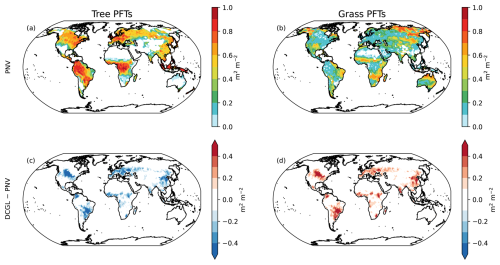
Figure 2Changes in tree and grass cover fractions (areas covered by vegetation per unit ground area). Panels (a) and (c) show the tree PFTs, while panels (b) and (d) show the grass PFTs. Panels (a) and (b) show the global distribution of the vegetation fractions in the PNV scenario. Panels (c) and (d) show the changes with respect to the deforestation (DCGL−PNV) scenario.
Here, we acknowledge some limitations in this assessment. LPJ-GUESS simulates only two grass PFTs, C1 and C2, which represent grasslands rather than agricultural crops. While the BVOC emission rates of crops differ from those of natural grasslands (Weber et al., 2023), we contend that this discrepancy is not substantial as most land use changes are related to grazing rather than crop cultivation. Additionally, in its current configuration, LPJ-GUESS does not simulate shrub PFTs, which means we may have also overlooked some biomass and BVOC emissions from grasslands.
3.1.2 BVOC surface emissions
The clearing of biomass due to deforestation practices impacts global BVOC emissions. Figure 3a–b show the surface isoprene and monoterpene emissions from the PNV scenario. The model simulates isoprene fluxes of up to 100 mg m−2 d−1 and monoterpene fluxes of up to 8 mg m−2 d−1 for the tropics. Figure 3c–d show the spatial changes in the BVOC fluxes resulting from deforestation.
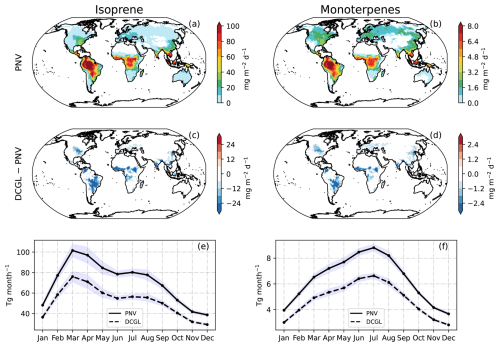
Figure 3Isoprene and monoterpene surface fluxes for the PNV scenario (a–b). Panels (c) and (d) show the spatial differences in isoprene and monoterpene emission fluxes (DCGL−PNV). Monthly emissions are based on the 10-year global averages for isoprene (e) and monoterpenes (f). The shading represents 1 standard deviation, derived from the monthly averages based on 10 simulated years. Fluxes in the Southern Hemisphere were shifted by 6 months to align with the seasonal cycle.
In Fig. 3e–f, temporal profiles of global monthly emission totals are depicted, with shading indicating 1σ variability based on 10 simulated years. To capture the true seasonal cycle, values from the Southern Hemisphere were shifted by 6 months before combining flux values from both hemispheres. In the PNV scenario, the global annual emission flux for isoprene totals 845.7 Tg, decreasing to 620.9 Tg in the DCGL scenario, marking a reduction of 224.8 Tg (a 27 % decrease relative to the PNV scenario). Similarly, for monoterpenes, the annual global emissions in the PNV scenario correspond to 76.0 and 57.3 Tg in the DCGL scenario, respectively, indicating a decrease of 18.7 Tg (a 25 % decrease relative to the PNV scenario). Consequently, the global annual source of BVOCs decreases by 243.5 Tg.
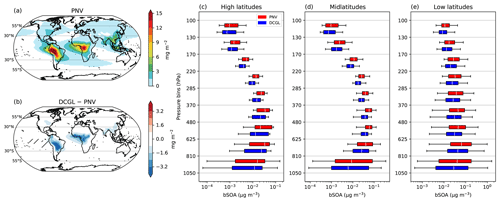
Figure 4Total column bSOA from the PNV scenario (a) and changes in bSOA from the deforestation (DCGL−PNV) scenario (b). Panels (c), (d), and (e) show the vertical profiles of bSOA, represented by box–whisker plots for different pressure bins. The white line marks the median, the box corresponds to the lower and upper quartiles, and the whiskers represent the 5th and 95th percentiles of the spatial mean over the 10 years simulated. The latitude ranges are defined as follows: high latitudes (90–55° S and 55–90° N), midlatitudes (55–30° S and 30–55° N), and low latitudes (30° S–30° N). A log scale is used for the x axis in panels (c), (d), and (e). Panel (b) includes diagonal crosshatching to indicate areas that are not statistically significant, based on a two-tailed Student's t test with a 90 % confidence level.
3.1.3 Aerosol burden, cloud interactions, and radiative effects
Figure 4 illustrates the bSOA burden, including the total column burden for the PNV scenario, as depicted in Fig. 4a, as well as changes in the column burden resulting from deforestation (Fig. 4b) and vertical profiles across three latitude bands (Fig. 4c–e). Figure 4b includes diagonal crosshatching to indicate areas that are not statistically significant, based on a two-tailed Student’s t test with a 90 % confidence level using annual global means. The model shows that most changes in the bSOA column burden are statistically significant, except with respect to certain regions of the tropical Pacific Ocean. The vertical profiles are represented using box–whisker plots, showcasing variations across different pressure bins. In the PNV scenario, the bSOA load exhibits its highest concentration over the Amazon forest and the Congo Basin, reaching a column mass of up to 15 mg m−2. For the PNV scenario, we estimate a total annual OA atmospheric burden of 1.87 Tg, of which 0.56 Tg corresponds to bSOA, while in the DCGL scenario, the total annual OA atmospheric burden decreases to 1.70 Tg, of which 0.40 Tg corresponds to bSOA. The bSOA burden decreases by 29 %, while the total OA decreases by 9 %.
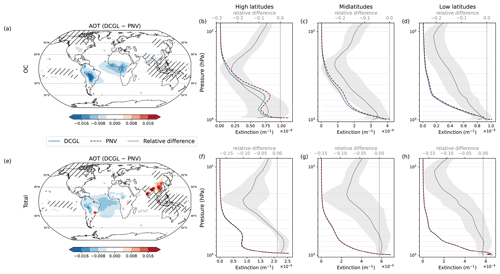
Figure 5Spatial maps of the total column aerosol optical thickness (AOT) at 550 nm for organic carbon (OC) (a) and total aerosol (e). Vertical profiles of aerosol extinction at 550 nm for the DCGL and PNV scenarios, along with the relative differences ((DCGL−PNV)/PNV) in different latitude bands. Panels (b), (c), and (d) correspond to organic carbon, and panels (f), (g), and (h) correspond to total aerosol. The latitude ranges are defined as follows: high latitudes (90–55° S and 55–90° N), midlatitudes (55–30° S and 30–55° N), and low latitudes (30° S–30° N). The grey area represents 1 standard deviation of the spatio-temporal mean (grey line). Please note the different scales for the relative differences. Panels (a) and (e) include diagonal crosshatching to indicate areas that are not statistically significant, based on a two-tailed Student's t test with a 90 % confidence level.
Figure 5 shows changes in the total column aerosol optical thickness (AOT) and extinction at 550 nm for OA (Fig. 5a–d) and for total aerosol (Fig. 5e–h). The aerosol optical thickness (AOT) is determined by integrating the aerosol extinction (m−1) over the full atmospheric column, making it a dimensionless quantity. Figure 5a illustrates the absolute changes in AOT resulting from organic carbon (OC) and indicates a decrease of up to 0.02 over the Amazon forest and the Congo Basin in Africa. Fig. 5b–d show a consistent decrease in OC extinction, with the highest relative differences peaking at around 250 hPa at high and middle latitudes and at around 100 hPa in the tropics. Our calculations indicate a global decrease in OC AOT of −7 %, with corresponding decreases of −10 %, −9 %, and −5 % at high, middle, and low latitudes. EMAC simulates the optical properties of six aerosol species: organic carbon (OC), black carbon (BC), water-soluble (WASO) inorganic aerosols, dust (DU), sea salt (SS), and aerosol-associated water (H2O). The aerosol extinction at 550 nm with respect to total aerosol suggests a decrease over the tropics (Fig. 5e); however, this trend varies significantly across latitudinal bands. Notably, there is a marginal increase of 0.1 % at high latitudes, with declines of −0.5 % and −0.6 % at middle and low latitudes, respectively. Diagonal crosshatching in Fig. 5a and e highlights regions where changes in OC and total aerosol extinction are not statistically significant. The model suggests that most changes in AOT are statistically significant, except in regions over the tropical Pacific Ocean and Southeast Asia.
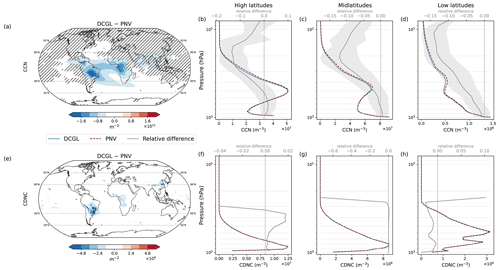
Figure 6Changes in cloud condensation nuclei (CCN) at 0.2 % supersaturation (a–d) and cloud droplet number concentration (CDNC) (e–h). Panels (a) and (e) show the spatial differences in the total column burden (the number of particles per square metre) for CCN and CDNC, respectively, emerging from the DCGL scenario as compared to natural vegetation (DCGL−PNV). Panels (b)–(d) and (f)–(h) show vertical profiles of total column data from the DCGL and PNV simulations, along with their relative differences. The figure details are the same as those in Fig. 5; however, the grey area representing 1 standard deviation of the spatio-temporal mean is depicted only in panels (b), (c), and (d). In panel (e), a dotted pattern is used to highlight areas with statistically significant differences.
Although this model setup excludes organic NPF, aerosol growth via the condensation of organics can still impact CCN and CDNC by increasing particle size. This growth pushes existing particles closer to the critical radius required for them to act as CCN and activate into cloud droplets, as described by Köhler theory in the activation parameterisation used by the CLOUD submodel in EMAC. Figure 6 illustrates changes in CCN at 0.2 % supersaturation and changes in CDNC. The reduced aerosol burden in the DCGL scenario leads to a decrease in CCN of up to 2×1011 particles m−2 over tropical regions. Our calculations suggest a global decrease in CCN of 4.8 %, with respective declines of 3 %, 5 %, and 5 % at high, middle, and low latitudes, respectively. Diagonal crosshatching in Fig. 6a indicates that CCN column burden changes are only statistically significant in tropical South America, tropical Africa, and much of the Southern Hemisphere. Fig. 6b–d show vertical profiles of CCN for both the DCGL and PNV scenarios. At high and middle latitudes, the difference in CCN concentration peaks around 200 hPa, showing a decrease of approximately 12 % in the DCGL scenario. In the tropics, the peak difference occurs higher up in the atmosphere (around 950 hPa), also showing a decrease of around 12 %. Our results indicate that the lower availability of CCN (Fig. 6a) induces a decrease in CDNC in South America, central Africa, and eastern China. Globally, deforestation leads to a decrease of 0.2 % in CDNC. Spatial averages over high and middle latitudes suggest general increases of 0.2 % and 0.3 %, respectively. Conversely, over the tropics, CDNC decreases by 0.6 %. Fig. 6e uses a dotted pattern to highlight statistically significant changes in the CDNC column burden. The model indicates that only small regions in South America show statistically significant changes. The smaller signal strength and significance in the CDNC column burden, compared to CCN changes, arise because most CCN do not coincide with the cloud layer and, therefore, do not interact with clouds. In a separate analysis, focusing on the pressure level at which the difference in CDNC between the two simulations is maximised, we find statistical significance across the board, occurring, on average, at around 700 hPa, or approximately 3.3 km. This means that, at this layer, global CCN influence cloud droplet numbers. Fig. 6f–h, which display vertical profiles of CDNC, show that the strongest influence on CDNC, characterised by a small but consistent reduction throughout the column, occurs in the tropics. This pattern suggests that shifts in aerosol loading directly affect cloud properties, particularly in regions influenced by perturbed organic aerosols from BVOC precursors. However, the magnitude of the effect appears to be small. Additionally, the standard deviation in the relative changes exceeds 1000 %, and, thus, it was excluded from Fig. 6f–h to prevent distortion of the scale. This high standard deviation highlights the spatial variability across latitudinal bands and suggests that the observed changes are spatially heterogeneous.
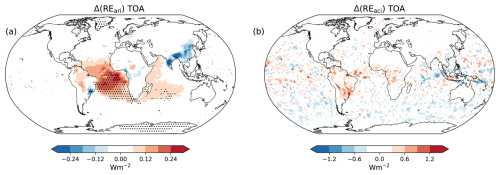
Figure 7Aerosol and cloud radiative effects mediated by aerosol changes from the deforestation (DCGL−PNV) scenario. Panels (a) and (b) show the top-of-the-atmosphere (TOA) direct radiative effect (ARI) and indirect radiative effect (ACI), respectively. A dotted pattern is used to highlight areas with statistically significant differences, based on a two-tailed Student's t test with a 90 % confidence level.
Figure 7 shows the changes in REari and REaci resulting from the conversion of natural vegetation to cropland and grazing land. We estimate a global increase in the aerosol radiative effect (REari) of 60.4 mW m−2, with the tropics showing a notably higher increase, averaging 91.2 mW m−2. Most of the REaci values are positive in tropical regions due to the reduced aerosols. In contrast, the increased aerosol extinction observed over Southeast Asia (Fig. 5e) corresponds to a negative, or cooling, effect in that area. The model indicates a less prominent signal in the cloud radiative effect (REaci), with a noticeable increase of +27.5 mW m−2 over the tropics but a minor global cooling effect of −8.7 mW m−2. The simulations indicate that the signal in REari is significant over the tropical Atlantic Ocean, parts of South America, and India. However, the model suggests that REaci changes are not statistically significant. Figure S6 includes maps showing relative changes in bSOA, AOT, CCN, and CDNC, as well as radiative effects resulting from present-day land use cover compared to natural vegetation cover.
3.2 Grazing-land restoration
In this section, we evaluate the changes resulting from the restoration of all grazing land to natural vegetation levels. In Sect. 3.1, the PNV scenario was used as the baseline to assess changes resulting from present-day land use cover (DCGL). In the following section, the DCGL scenario serves as the baseline, with the sensitivity run being the DCL scenario, including only deforested crop cultivation, as shown in Fig. 1b. These analyses therefore represent an extreme reforestation scenario.
3.2.1 Changes in vegetation and BVOC emissions
In this scenario, where it is assumed that all grazing land is restored to natural vegetation levels (DCGL−DCL), global tree cover increases by 600 Mha compared to present-day land use cover (Fig. S2). We also find that, in this scenario, global biomass increases to 546 PgC, which is an increase of 43 PgC compared to present-day land cover. Figure S1 shows the spatial distribution of biomass per unit land area and the shifts resulting from the land use scenarios considered here.
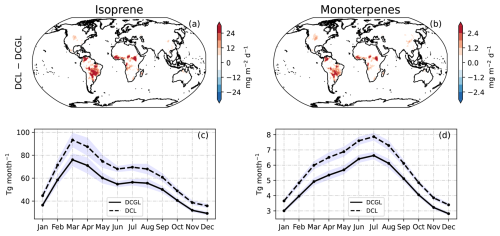
Figure 8Spatial differences in isoprene and monoterpene emission fluxes (DCL−DCGL) (a–b). Monthly emissions based on the 10-year averages for isoprene (c) and monoterpenes (d). The shading represents 1 standard deviation, derived from the monthly averages based on 10 simulated years. Fluxes in the Southern Hemisphere were shifted by 6 months to align with the seasonal cycle.
Figure 8 depicts the changes in surface isoprene and monoterpene emissions resulting from the grazing-land restoration run, compared to present-day land cover. These emission changes align with the alterations in vegetation cover (Fig. S2). Globally, annual isoprene emissions in the DCL scenario increase by 140.4 Tg (+23 %) relative to the DCGL scenario, while monoterpene emissions increase by 11.6 Tg (+20 %).
3.2.2 Changes in atmospheric states
Figure 9 summarises the changes in atmospheric states following the conversion of grazing land to reforestation (DCL−DCGL), including radiative effects from aerosol and cloud properties (Fig. 9e–f). The column mass of bSOA (Fig. 9a) experiences a notable increase of over 3 mg m−2 across tropical South America and central Africa (Fig. S3b). This rise increases the bSOA burden from 0.40 Tg, taken from the baseline scenario, to 0.50 Tg (+0.10 Tg (+25.7 %)), consequently perturbing the total OA burden, which increases from 1.70 to 1.80 Tg (+0.11 Tg (+6.3 %)). Figure S3 includes vertical bSOA profiles for the three latitude bands. The observed changes are statistically significant, except in regions over the tropical Pacific Ocean and Southeast Asia. The global mean OC AOT at 550 nm increases by 3.8 %, with corresponding increases of 5.5 %, 5 %, and 2.4 % at high, middle, and low latitudes, respectively. The global mean AOT at 550 nm (Fig. 9b) with respect to total aerosol increases by 0.3 %, with a corresponding decrease of 0.1 % at high latitudes and an increase of 0.4 % at both middle and low latitudes. Statistically significant changes in AOT at 550 nm occur only in certain regions of the tropical Atlantic Ocean and in parts of South America (see Fig. 9b). Spatial maps of the AOT and extinction vertical profiles for the different latitude bands are shown in Fig. S4.
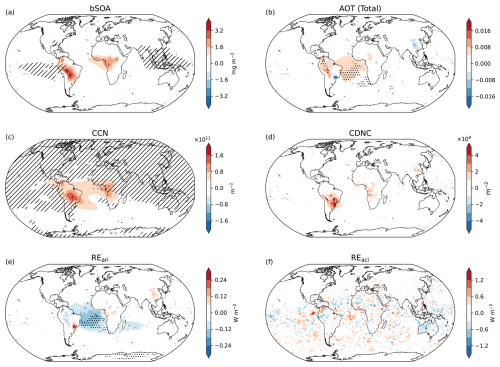
Figure 9Absolute changes in atmospheric states resulting from the restoration of present-day grazing land. Maps show variations in (a) bSOA column mass, (b) total aerosol optical thickness (AOT), (c) cloud condensation nuclei (CCN), (d) cloud droplet number concentration (CDNC), (e) aerosol radiative effects, and (f) cloud radiative effects. Diagonal crosshatching is applied in panels (a) and (c) to indicate areas that are not statistically significant, while a dotted pattern is used in panels (b), (d), (e), and (f) to highlight areas with statistically significant differences, based on a two-tailed Student's t test with a 90 % confidence level.
In this scenario, we estimate a global increase in CCN of 3.5 %, with increases of 2.5 %, 3.7 %, and 3.7 % at high, middle, and low latitudes, respectively. Statistically significant changes occur in tropical South America, the tropical Atlantic Ocean, and most of the Southern Hemisphere (Fig. 9c). CDNC increases globally by 0.2 %, with decreases of 0.1 % at high and middle latitudes and an increase of 0.5 % in the tropics. Statistically significant changes only occur in a small part of South America (see Fig. 9d). Spatial maps and vertical profiles for the different latitude bands for CCN and CDNC can be found in Fig. S5. The global ARI effect (REari) corresponds to −38.2 mW m−2, while the radiative effect resulting from changes in cloud properties (REaci) is found to correspond to +1.6 mW m−2. While REari is statistically significant over some parts of the tropical Atlantic Ocean, REaci changes are not statistically significant.
Table 1 outlines the changes in vegetation and atmospheric variables for the two land cover scenarios considered in this study: present-day land cover vs. natural vegetation (DCGL−PNV), representing deforestation, and the restoration of grazing land vs. present-day land cover (DCL−DCGL), representing reforestation. The results indicate that both the deforestation of natural vegetation relative to present-day land cover and the reforestation of present-day grazing lands significantly impact vegetation and atmospheric variables.
Table 1Changes in vegetation and atmospheric variables for the two land cover scenarios: present-day land cover vs. natural vegetation (DCGL−PNV) and the restoration of grazing land vs. present-day land cover (DCL−DCGL). Values for tree cover, vegetation biomass, isoprene, monoterpenes, bSOA, and OA are global yearly sums, whereas values for AOT, CCN, CDNC, REari, and REaci are global yearly means.
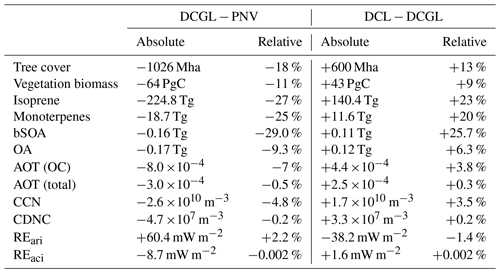
The present study offers insights into the ongoing impact of human-induced deforestation and potential reforestation on atmospheric aerosols, building upon the existing literature. In this study, a decline or increase in vegetation was assumed to drive changes in BVOC emissions and their impacts on atmospheric aerosols. This assumption can be validated by comparing the calculated decline in tree cover and vegetation biomass over the years from the vegetation model with values from the literature. For instance, Hu et al. (2021) estimate that between 1992 and 2018, 722 Mha of forests were converted into agricultural land, while Bhan et al. (2022) estimate the terrestrial biosphere's global carbon stock for 1950 to be 450 PgC. These estimates align with the values simulated by LPJ-GUESS, suggesting that the changes in the biosphere due to land use changes incorporated into this study are consistent with earlier findings.
4.1 BVOC emission changes
Studies agree that land use changes predominantly affect BVOC emissions through the expansion of croplands in tropical regions, such as the Amazon, central Africa, and Southeast Asia (Ganzeveld et al., 2010; Lathière et al., 2010; Hantson et al., 2017; Szogs et al., 2017). For example, Lathière et al. (2006) show a reduction of approximately 24 % in isoprene emissions between 1901 and 2002. Additionally, Unger (2014) reports that land cover changes from the 1850s to the 2000s resulted in a global decrease of approximately 35 % in BVOC emissions. In a comprehensive literature review exploring the influence of land use and land cover change (LULCC) on atmospheric composition and climate, it was estimated that from the preindustrial era to the present, LULCC has led to a decrease of 15 %–36 % in global isoprene emissions (Heald and Spracklen, 2015). Scott et al. (2018) report global decreases in isoprene and monoterpenes emissions of 87 % and 94 %, respectively, resulting from complete global deforestation. The 26 % decrease in BVOC emissions reported in this study is in line with findings reported in the literature, particularly because many studies analyse changes from preindustrial times, whereas our work focuses on changes resulting from human land use compared to natural vegetation. Although neither cropland nor grazing-land expansion was as significant as during or after the industrial era, it did occur on a smaller scale. Attention must also be given when comparing studies with and without temperature feedback, given the strong dependence of BVOC emissions on temperature. In this work, similar to the calculations in Scott et al. (2018), temperature feedbacks were suppressed.
The global yearly isoprene and monoterpene emission budgets from this study are relatively high compared to global estimates observed in the literature, as shown in Vella et al. (2023a). Some atmospheric chemistry studies using MEGAN in EMAC, e.g. Pozzer et al. (2022), employ a global scaling factor to dampen the global emissions to desired values. However, in this study, no scaling factors were applied, which means that the values reported here may be slightly overestimated.
4.2 Aerosol burden
The rapid oxidation of BVOCs yields oxygenated intermediate species near the surface, which act as precursors for the formation of bSOA. This explains the high abundance of bSOA in the lower atmosphere (see the vertical profiles in Fig. 4). As bSOA is dispersed in the atmosphere, concentrations typically decrease due to mixing and dilution. However, the vertical profile of bSOA is also governed by the vapour pressure of the oxygenated intermediates, which decreases with lower temperatures as these intermediates ascend in the troposphere. As they are transported upwards, the reduced volatility at lower temperatures prompts the transition of oxygenated intermediates into the aerosol phase, contributing to the formation of bSOA. Consequently, this interplay between oxidation, transport, and vapour-pressure-driven processes imparts a distinctive D-shaped vertical profile of bSOA concentrations, with elevated concentrations occurring near the surface, followed by an increase in bSOA towards higher altitudes and, ultimately, a decline in the upper atmosphere. At low latitudes (the tropics), this D-shaped bSOA concentration profile is only faint (Fig. 4e). The prevalence of warm air and relatively high boundary layers, as well as the occurrence of deep convection transporting both aerosol particles and precursors into the upper troposphere, leads to maximum gas-to-particle partitioning occurring at higher altitudes, typically around 100–200 hPa, resulting in a secondary local upper-tropospheric enhancement.
Several studies estimate the global mean burden of bSOA to be ∼ 0.5–0.77 Tg (Henze et al., 2008; Pye et al., 2010; Hoyle et al., 2007; Tsigaridis and Kanakidou, 2007; Tilmes et al., 2019). This indicates that, in the present-day land cover scenario, ORACLE provides a lower estimate of the bSOA burden (0.40 Tg) compared to values observed in the literature. Pozzer et al. (2022) evaluated OA values from ORACLE in EMAC and found that surface concentrations are well represented; however, OA is strongly underestimated in the free troposphere. This work by Pozzer et al. (2022) employed the Mainz Organic Mechanism (MOM), which is a more complex chemical mechanism compared to the one used in this study (i.e. the Mainz Isoprene Mechanism – MIM). Nevertheless, this indicates that even with a more complex mechanism, EMAC calculates lower OA values globally. Heald and Geddes (2016) report a 13 % decrease in the global annual mean tropospheric burden of bSOA between 1850 and 2000 due to land use change, while Scott et al. (2018) estimate a 91 % decrease in SOA resulting from simulated global deforestation. Our model calculations suggest a reduction of approximately 30 % in bSOA as a result of cropland and grazing-land deforestation compared to natural vegetation.
Regionally, the derived total AOT exhibits opposing effects. In particular, pockets of increased total AOT are evident in confined regions, notably in Southeast (SE) Asia (Fig. 5e). Here, extinction due to H2O and WASO compounds is found to increase, effectively masking the expected reduction in OA extinction from the lower number of BVOC precursors in the DCGL scenario. This phenomenon is linked to the growth of aerosol particles into what is commonly referred to as the “Greenfield gap” – a range characterised by lower deposition velocities and scavenging values (Greenfield, 1957). The extended atmospheric lifetime of particles in these regions adds to the burden of WASO compounds and their associated water uptake, thereby amplifying aerosol extinction. Moreover, the absence of organics exacerbates this effect, leading to disproportionate condensation on accumulation-mode particles at the expense of Aitken-mode particles. Consequently, the increased presence of water-soluble compounds, increased aerosol water content, and shift in size distribution towards larger particles collectively contribute to the observed increase in extinction in these specific regions. While all of these factors contribute, enhanced scattering is the dominant influence on aerosol optical thickness. Nevertheless, plotting the relative difference in total AOT (Fig. S6b) shows that this effect is not very prominent, with an increase in AOT in these regions of less than 4 %. This phenomenon occurs only in the DCGL−PNV scenario. In the DCL−DCGL scenario, the increase in organic aerosols leads to condensation on Aitken-mode particles, limiting growth in the accumulation mode, which aligns with our explanation.
4.3 Cloud properties and radiative effects
Studies focusing on changes in cloud properties due to perturbed BVOC precursors and SOA loading resulting from land use change are somewhat limited. Scott et al. (2014) investigated the impact of bSOA on surface CCN and CDNC at cloud height (approximately 900 hPa) in the present-day atmosphere and found that bSOA increases the annual mean concentration of CCN by 3.6 %–21.1 % and the global annual mean concentration of CDNC by 1.9 %–5.2 %. In this work, we show that present-day deforestation, when compared to the PNV scenario, yields a decrease of 4.8 % in the total column CCN but only a small decrease of 0.2 % in the column CDNC burden, with a slightly more prominent reduction of 0.6 % over tropical regions. The most significant change in cloud droplet count occurs over the Amazon, with an approximate 8 % decrease in this region (Fig. S7d). The changes in CDNC simulated by EMAC, however, seem to be very localised, particularly over the Amazon rainforest, but the global impact on CDNC is small. Deforestation-induced changes in CDNC are less than 10 % over the Amazon and less than 1 % globally (Figs. S6 and S7).
It is worth pointing out that the effect on cloud droplet numbers reported here only stems from aerosol–cloud interactions. In reality, reduced tree cover may lead to less evapotranspiration, which modifies the Bowen ratio (the ratio of sensible heat to latent heat) and potentially influences the cloud effect; however, this feedback is suppressed in our simulations. This is in addition to roughness changes and albedo changes that can also influence cloud cover (Cerasoli et al., 2021). Moreover, in this model setup, only aerosol–cloud interactions with large-scale clouds are considered. Therefore, the impact of changes in the aerosol burden on convective clouds and the corresponding potential radiative effects are not captured. The lack of organic NPF descriptions in the model may also result in the underestimation of the real influence of bSOA on CDNC and REaci reported in this work.
Biogenic SOA is known to exert net cooling effects on the Earth's climate by scattering a portion of incoming solar radiation back into space (Zhu et al., 2019; Sporre et al., 2020; Tilmes et al., 2019). Therefore, changes in aerosol number and composition due to deforestation result in net warming from the lack of aerosol scattering, while reforestation leads to the opposite effect, with a net cooling effect (Figs. 7a and 9e). Scott et al. (2018) showed that full deforestation results in a radiative effect of 117 mW m−2 due to aerosols and a radiative effect of 200 mW m−2 due to clouds. In contrast, projections for deforestation under the Representative Concentration Pathway (RCP) 8.5 scenario for 2100 indicate that aerosols account for only 6 mW m−2 of radiative effects, with negligible aerosol–radiation interactions (ARIs) Scott et al. (2018). Furthermore, O'Donnell et al. (2011) estimated the total effect of secondary organic aerosol (SOA) aerosol–cloud interactions (ACIs) to be 310 mW m−2.
The comparatively small ARI effect reported here from the deforestation scenario (60.4 mW m−2), relative to the PNV scenario, may stem from differences in methodology compared to other studies, many of which focus on the effects of full deforestation or total SOA (not bSOA). However, our REari estimates might underestimate the actual impact due to comparatively lower bSOA yields in our model runs. The radiative forcing from BVOCs is strongly influenced by SOA yields, which can vary considerably with environmental conditions. SOA yields are sensitive to factors such as temperature and oxidant concentrations, which can alter the overall aerosol burden and the yields' radiative properties (Sporre et al., 2020). Furthermore, the role of oxidant chemistry is crucial in determining the oxidation pathways and the formation of SOA. Variations in oxidant levels, particularly with respect to ozone and hydroxyl radicals, can substantially affect SOA formation rates and chemical composition (Weber et al., 2022). A more comprehensive analysis of the chemical processes influencing SOA yields and their associated radiative forcing should be further explored using the EMAC model.
Regarding the afforestation scenario, we acknowledge that this is an idealistic sensitivity analysis that perturbs only land use cover while maintaining present-day values for greenhouse gas concentrations and anthropogenic emissions. In different future scenarios, these key variables would play a crucial role in determining aerosol radiative effects. This is also highlighted in this study, where we showed that over Southeast Asia, where anthropogenic aerosols are present in relatively high numbers, the reduction in global OA due to deforestation resulted in changes in the aerosol size distribution over this area, leading to an opposing effect in aerosol extinction.
This work includes an analysis of the statistical significance of changes in atmospheric states resulting from perturbed surface BVOC emissions due to land use change. In this analysis, we applied a two-tailed Student's t test with a 90 % confidence level (p<0.1). The t test was performed on annual means to minimise noise resulting from annual internal variability. We show that changes in surface BVOC emissions lead to a statistically significant bSOA column mass burden on a global scale, excluding a portion of the tropical Pacific Ocean. Changes in AOT attributed to OA and total aerosol exhibit statistically significant differences overall, except in Southeast Asia. CCN changes predominantly occur in the tropics (excluding Southeast Asia) and the Southern Ocean. Changes in the CDNC column burden are largely not statistically significant, but CDNC changes at approximately 700 hPa do exhibit statistically significant differences. While REari exhibits a statistically significant signal over the tropical Atlantic Ocean, where most of the changes occur, REaci does not demonstrate statistical significance with respect to deforestation.
While some studies, such as those employing the UK Earth System Model (UKESM) and version 2 of the Community Earth System Model (CESM2), indicate statistically significant ARI effects resulting from deforestation at a 95 % confidence level (Weber et al., 2024), other studies, such as the one by Unger (2014), employing the Yale-E2 global carbon–chemistry–climate model, suggest that the global-scale impacts of cropland expansion on bSOA are not statistically significant relative to interannual variability in climate. We argue that the land cover changes assessed in EMAC significantly impact global aerosol budgets. However, it appears that the influence of total cloud droplet numbers and REaci on a global scale is likely to be minor. Here, we emphasise that the statistical significance of a result is not a property of an effect (whether it is large or small) but rather a measure of whether it can be detected (i.e. how likely it is that the result occurred by chance). Our results suggest that the impact on clouds and the corresponding radiative forcing is not significant; however, this is highly dependent on the model setup. We are limited by a 10-year simulation output as this output may not be sufficient for a signal in clouds to emerge, given the natural noise and internal variability in the climate system.
Understanding how land use changes alter atmospheric composition is crucial for grasping the impact of human activities on the Earth system with respect to both the past and the future. This study presents a comprehensive evaluation of two land use scenarios, focusing on how changes in BVOC emissions influence the atmosphere and climate. We find that present-day deforestation results in a 73 % decrease in isoprene emissions and a 75 % decrease in monoterpene emissions. Consequently, the bSOA burden decreases from 0.56 to 0.40 Tg, and the total OA burden decreases from 1.80 to 1.70 Tg. As expected, this leads to a reduction in OC extinction, especially in the tropics near the source of bSOA and its precursors. Upon examining the impact on total aerosol extinction, the model indicates declines in AOT over South America and the tropical Atlantic Ocean. However, aerosol extinction increases over SE Asia in the present-day deforestation scenario compared to PNV conditions. Particularly in SE Asia, the reduction in organic material results in the growth of water-soluble (WASO) inorganic compounds. The absence of organics leads to more condensation on accumulation-mode particles and less condensation on Aitken-mode particles, resulting in increased aerosol water uptake and a shift in the aerosol size distribution towards larger particles. This effectively increases aerosol extinction.
The perturbations in aerosols resulting from deforestation lead to a positive radiative effect in the tropics (warming), stemming from the reduced aerosol burden. The increased presence of WASO compounds over SE Asia contributes to a negative radiative effect (cooling) in this region. However, our findings indicate a global positive radiative effect of 60.4 mW m−2 (aerosol + cloud). In the reforestation scenario, isoprene and monoterpene fluxes increase by 23 % and 20 %, respectively, leading to an increase in the bSOA burden of 0.23 Tg and a negative radiative effect of 38.2 mW m−2.
The Modular Earth Submodel System (MESSy; https://doi.org/10.5281/zenodo.13768443, The MESSy Consortium, 2024) is continuously being developed and applied by a consortium of institutions. Usage of MESSy and access to the source code are licensed to all affiliates of institutions which are members of the MESSy Consortium. Institutions can become members of the MESSy Consortium by signing the MESSy Memorandum of Understanding. More information can be found on the MESSy Consortium website (http://www.messy-interface.org, MESSY, 2025). The model outputs relevant to this study are permanently stored in a Zenodo repository and are accessible via https://doi.org/10.5281/zenodo.13906983 (Vella et al., 2024). Analysis scripts are available upon request from the corresponding author.
The supplement related to this article is available online at: https://doi.org/10.5194/acp-25-243-2025-supplement.
RV, HT, and MF implemented and tested the deforestation routine. RV prepared the model setup and performed the simulations with input from HT, AT, and AP. AT provided technical assistance for ORACLE and provided scripts to handle its output. RV evaluated the simulations, analysed the model results, and wrote the article. The results were interpreted by all co-authors, with a special focus on vegetation analysis provided by MF and TH. JL and HT supervised the project. All authors discussed the results and contributed to the review and editing of the paper.
At least one of the (co-)authors is a member of the editorial board of Atmospheric Chemistry and Physics. The peer-review process was guided by an independent editor, and the authors also have no other competing interests to declare.
Publisher’s note: Copernicus Publications remains neutral with regard to jurisdictional claims made in the text, published maps, institutional affiliations, or any other geographical representation in this paper. While Copernicus Publications makes every effort to include appropriate place names, the final responsibility lies with the authors.
This article is part of the special issue “The Modular Earth Submodel System (MESSy) (ACP/GMD inter-journal SI)”. It is not associated with a conference.
The model simulations were performed at the German Climate Computing Centre (DKRZ) through support from the Max Planck Society and JGU Mainz. This work was supported by the Max Planck Graduate Center (MPGC) in collaboration with Johannes Gutenberg-Universität Mainz. Andrea Pozzer acknowledges the European Commission's Horizon Europe project FOCI (grant no. 101056783). Holger Tost acknowledges funding from the Deutsche Forschungsgemeinschaft (DFG – German Research Foundation) as part of project TRR 301 (project no. 428312742).
The article processing charges for this open-access publication were covered by the Max Planck Society.
This paper was edited by Kelley Barsanti and reviewed by two anonymous referees.
Andreae, M. O.: Emission of trace gases and aerosols from biomass burning – an updated assessment, Atmos. Chem. Phys., 19, 8523–8546, https://doi.org/10.5194/acp-19-8523-2019, 2019. a
Atkinson, R.: Atmospheric chemistry of VOCs and NOx, Atmos. Environ., 34, 2063–2101, 2000. a
Atkinson, R. and Arey, J.: Gas-phase tropospheric chemistry of biogenic volatile organic compounds: a review, Atmos. Environ., 37, 197–219, 2003. a
Betts, R., Cox, P., Collins, M., Harris, P., Huntingford, C., and Jones, C.: The role of ecosystem-atmosphere interactions in simulated Amazonian precipitation decrease and forest dieback under global climate warming, Theor. Appl. Climatol., 78, 157–175, 2004. a
Bhan, M., Meyfroidt, P., Matej, S., Erb, K.-H., and Gingrich, S.: A mid-20th century inventory-based estimate of global terrestrial vegetation carbon stocks, J. Land Use Sci., 17, 429–453, 2022. a
Bonan, G. B.: Forests and climate change: forcings, feedbacks, and the climate benefits of forests, Science, 320, 1444–1449, 2008. a, b
Bouwman, A., Lee, D., Asman, W. A., Dentener, F., Van Der Hoek, K., and Olivier, J.: A global high-resolution emission inventory for ammonia, Global Biogeochem. Cy., 11, 561–587, 1997. a
Brinkop, S. and Roeckner, E.: Sensitivity of a general circulation model to parameterizations of cloud–turbulence interactions in the atmospheric boundary layer, Tellus A, 47, 197–220, 1995. a
Cerasoli, S., Yin, J., and Porporato, A.: Cloud cooling effects of afforestation and reforestation at midlatitudes, P. Natl. Acad. Sci. USA, 118, e2026241118, https://doi.org/10.1073/pnas.2026241118, 2021. a
Chen, Q., Farmer, D., Schneider, J., Zorn, S., Heald, C., Karl, T., Guenther, A., Allan, J., Robinson, N., Coe, H., Kimmel, J., Pauliquevis, T., Borrmann, S., Pöschl, U., Andreae, M., Artaxo, P., Jimenez, J., and Martin, S.: Mass spectral characterization of submicron biogenic organic particles in the Amazon Basin, Geophys. Res. Lett., 36, L20806, https://doi.org/10.1029/2009GL039880, 2009. a
Dee, D. P., Uppala, S. M., Simmons, A. J., Berrisford, P., Poli, P., Kobayashi, S., Andrae, U., Balmaseda, M. A., Balsamo, G., Bauer, P., Bechtold, P., Beljaars, A. C. M., van de Berg, L., Bidlot, J., Bormann, N., Delsol, C., Dragani, R., Fuentes, M., Geer, A. J., Haimberger, L., Healy, S. B., Hersbach, H., Hólm, E. V., Isaksen, L., Kållberg, P., Köhler, M., Matricardi, M., McNally, A. P., Monge-Sanz, B. M., Morcrette, J.-J., Park, B.-K., Peubey, C., de Rosnay, P., Tavolato, C., Thépaut, J.-N., and Vitart, F.: The ERA-Interim reanalysis: Configuration and performance of the data assimilation system, Q. J. Roy. Meteorol. Soc., 137, 553–597, 2011. a
Dentener, F., Kinne, S., Bond, T., Boucher, O., Cofala, J., Generoso, S., Ginoux, P., Gong, S., Hoelzemann, J. J., Ito, A., Marelli, L., Penner, J. E., Putaud, J.-P., Textor, C., Schulz, M., van der Werf, G. R., and Wilson, J.: Emissions of primary aerosol and precursor gases in the years 2000 and 1750 prescribed data-sets for AeroCom, Atmos. Chem. Phys., 6, 4321–4344, https://doi.org/10.5194/acp-6-4321-2006, 2006. a
de Sá, S. S., Rizzo, L. V., Palm, B. B., Campuzano-Jost, P., Day, D. A., Yee, L. D., Wernis, R., Isaacman-VanWertz, G., Brito, J., Carbone, S., Liu, Y. J., Sedlacek, A., Springston, S., Goldstein, A. H., Barbosa, H. M. J., Alexander, M. L., Artaxo, P., Jimenez, J. L., and Martin, S. T.: Contributions of biomass-burning, urban, and biogenic emissions to the concentrations and light-absorbing properties of particulate matter in central Amazonia during the dry season, Atmos. Chem. Phys., 19, 7973–8001, https://doi.org/10.5194/acp-19-7973-2019, 2019. a
Donahue, N. M., Robinson, A., Stanier, C., and Pandis, S.: Coupled partitioning, dilution, and chemical aging of semivolatile organics, Environ. Sci. Technol., 40, 2635–2643, 2006. a
Eyring, V., Lamarque, J.-F., Hess, P., Arfeuille, F., Bowman, K., Chipperfield, M., Duncan, B., Fiore, A., Gettelman, A., Giorgetta, M., Granier, C., Hegglin, M., Kinnison, D., Kunze, M., Langematz, U., Luo, B., Martin, R., Matthes, K., Newman, P., Peter, T., Robock, A., Ryerson, A., Saiz-Lopez, A., Salawitch, R., Schultz, M., Shepherd, T., Shindell, D., Stähelin, J., Tegtmeier, S., Thomason, L., Tilmes, S., Vernier, J.-P., Waugh, D., and Young, P.: Overview of IGAC/SPARC Chemistry-Climate Model Initiative (CCMI) Community Simulations in Support of Upcoming Ozone and Climate Assessments, https://www.geo.fu-berlin.de/met/ag/strat/publikationen/docs/Eyring-et-al_SPARC-Newsletter40.pdf (last access: 23 March 2016), 2013. a
Field, C. B. and Raupach, M. R.: The global carbon cycle: integrating humans, climate, and the natural world, vol. 62, Island Press, ISBN 1559635266, 2004. a
Fischer, E., Jacob, D. J., Millet, D. B., Yantosca, R. M., and Mao, J.: The role of the ocean in the global atmospheric budget of acetone, Geophys. Res. Lett., 39, L01807, https://doi.org/10.1029/2011gl050086, 2012. a
Forrest, M., Tost, H., Lelieveld, J., and Hickler, T.: Including vegetation dynamics in an atmospheric chemistry-enabled general circulation model: linking LPJ-GUESS (v4.0) with the EMAC modelling system (v2.53), Geosci. Model Dev., 13, 1285–1309, https://doi.org/10.5194/gmd-13-1285-2020, 2020. a, b
Forster, P., Ramaswamy, V., Artaxo, P., Berntsen, T., Betts, R., Fahey, D. W., Haywood, J., Lean, J., Lowe, D. C., Myhre, G., Nganga, J., Prinn, R., Raga, G., Schulz, M., and Van Dorland, R.: Changes in Atmospheric Constituents and in Radiative Forcing, in: Climate Change 2007: The Physical Science Basis. Contribution of Working Group I to the Fourth Assessment Report of the Intergovernmental Panel on Climate Change, edited by: Solomon, S., Qin, D., Manning, M., Chen, Z., Marquis, M., Averyt, K. B., Tignor, M., and Miller, H. L., Cambridge University Press, Cambridge, United Kingdom and New York, NY, USA, https://www.ipcc.ch/report/ar4/wg1/ (last access: 6 January 2025), 2007. a, b
Ganzeveld, L., Bouwman, L., Stehfest, E., van Vuuren, D. P., Eickhout, B., and Lelieveld, J.: Impact of future land use and land cover changes on atmospheric chemistry-climate interactions, J. Geophys. Res.-Atmos., 115, D23301, https://doi.org/10.1029/2010JD014041, 2010. a
Ghan, S. J.: Technical Note: Estimating aerosol effects on cloud radiative forcing, Atmos. Chem. Phys., 13, 9971–9974, https://doi.org/10.5194/acp-13-9971-2013, 2013. a
Granier, C., Darras, S., Denier van der Gon, H., Doubalova, J., Elguindi, N., Galle, B., Gauss, M., Guevara, M., Jalkanen, J.-P., Kuenen, J., Liousse, C., Quack, B., Simpson, D., and Sindelarova, K.: The Copernicus Atmosphere Monitoring Service global and regional emissions (April 2019 version), Tech. rep., Copernicus Atmosphere Monitoring Service (CAMS), https://doi.org/10.24380/d0bn-kx16, 2019. a
Greenfield, S. M.: Rain scavenging of radioactive particulate matter from the atmosphere, J. Atmos. Sci., 14, 115–125, 1957. a
Guelle, W., Schulz, M., Balkanski, Y., and Dentener, F.: Influence of the source formulation on modeling the atmospheric global distribution of sea salt aerosol, J. Geophys. Res.-Atmos., 106, 27509–27524, 2001. a
Guenther, A., Hewitt, C. N., Erickson, D., Fall, R., Geron, C., Graedel, T., Harley, P., Klinger, L., Lerdau, M., McKay, W., Pierce, T., Scholes, B., Steinbrecher, R., Tallamraju, R., Taylor, J., and Zimmerman, P.: A global model of natural volatile organic compound emissions, J. Geophys Res.-Atmos., 100, 8873–8892, 1995. a, b
Guenther, A., Karl, T., Harley, P., Wiedinmyer, C., Palmer, P. I., and Geron, C.: Estimates of global terrestrial isoprene emissions using MEGAN (Model of Emissions of Gases and Aerosols from Nature), Atmos. Chem. Phys., 6, 3181–3210, https://doi.org/10.5194/acp-6-3181-2006, 2006. a, b
Guenther, A. B., Zimmerman, P. R., Harley, P. C., Monson, R. K., and Fall, R.: Isoprene and monoterpene emission rate variability: model evaluations and sensitivity analyses, J. Geophys. Res.-Atmos., 98, 12609–12617, 1993. a
Hagemann, S. and Dümenil Gates, L.: An Improved Land Surface Parameter Dataset for Global and Regional Climate Models, MPI Report, Max Planck Institute for Meteorology, Hamburg, Germany, 336, 1–23, 2002. a
Hantson, S., Knorr, W., Schurgers, G., Pugh, T. A., and Arneth, A.: Global isoprene and monoterpene emissions under changing climate, vegetation, CO2 and land use, Atmos. Environ., 155, 35–45, 2017. a
Heald, C. L. and Geddes, J. A.: The impact of historical land use change from 1850 to 2000 on secondary particulate matter and ozone, Atmos. Chem. Phys., 16, 14997–15010, https://doi.org/10.5194/acp-16-14997-2016, 2016. a
Heald, C. L. and Spracklen, D. V.: Land use change impacts on air quality and climate, Chem. Rev., 115, 4476–4496, 2015. a
Henze, D. K., Seinfeld, J. H., Ng, N. L., Kroll, J. H., Fu, T.-M., Jacob, D. J., and Heald, C. L.: Global modeling of secondary organic aerosol formation from aromatic hydrocarbons: high- vs. low-yield pathways, Atmos. Chem. Phys., 8, 2405–2420, https://doi.org/10.5194/acp-8-2405-2008, 2008. a
Collier, C., Commane, R., Davies, F., Davison, B., DiCarlo, P., Di Marco, C. F., Dorsey, J. R., Edwards, P. M., Evans, M. J., Fowler, D., Furneaux, K. L., Gallagher, M., Guenther, A., Heard, D. E., Helfter, C., Hopkins, J., Ingham, T., Irwin, M., Jones, C., Karunaharan, A., Langford, B., Lewis, A. C., Lim, S. F., MacDonald, S. M., Mahajan, A. S., Malpass, S., McFiggans, G., Mills, G., Misztal, P., Moller, S., Monks, P. S., Nemitz, E., Nicolas-Perea, V., Oetjen, H., Oram, D. E., Palmer, P. I., Phillips, G. J., Pike, R., Plane, J. M. C., Pugh, T., Pyle, J. A., Reeves, C. E., Robinson, N. H., Stewart, D., Stone, D., Whalley, L. K., and Yin, X.: Overview: oxidant and particle photochemical processes above a south-east Asian tropical rainforest (the OP3 project): introduction, rationale, location characteristics and tools, Atmos. Chem. Phys., 10, 169–199, https://doi.org/10.5194/acp-10-169-2010, 2010. a
Hoyle, C. R., Berntsen, T., Myhre, G., and Isaksen, I. S. A.: Secondary organic aerosol in the global aerosol – chemical transport model Oslo CTM2, Atmos. Chem. Phys., 7, 5675–5694, https://doi.org/10.5194/acp-7-5675-2007, 2007. a
Hu, X., Næss, J. S., Iordan, C. M., Huang, B., Zhao, W., and Cherubini, F.: Recent global land cover dynamics and implications for soil erosion and carbon losses from deforestation, Anthropocene, 34, 100291, https://doi.org/10.1016/j.ancene.2021.100291, 2021. a
Hurtt, G. C., Chini, L. P., Frolking, S., Betts, R. A., Feddema, J., Fischer, G., Fisk, J. P., Hibbard, K., Houghton, R. A., Janetos, A., Jones, C. D., Kindermann, G., Kinoshita, T., Klein Goldewijk, K., Riahi, K., Shevliakova, E., Smith, S., Stehfest, E., Thomson, A., Thornton, P., van Vuuren, D. P., and Wang, Y. P.: Harmonization of land-use scenarios for the period 1500–2100: 600 years of global gridded annual land-use transitions, wood harvest, and resulting secondary lands, Climatic Change, 109, 117–161, 2011. a
Jöckel, P., Sander, R., Kerkweg, A., Tost, H., and Lelieveld, J.: Technical Note: The Modular Earth Submodel System (MESSy) – a new approach towards Earth System Modeling, Atmos. Chem. Phys., 5, 433–444, https://doi.org/10.5194/acp-5-433-2005, 2005. a
Jöckel, P., Tost, H., Pozzer, A., Brühl, C., Buchholz, J., Ganzeveld, L., Hoor, P., Kerkweg, A., Lawrence, M. G., Sander, R., Steil, B., Stiller, G., Tanarhte, M., Taraborrelli, D., van Aardenne, J., and Lelieveld, J.: The atmospheric chemistry general circulation model ECHAM5/MESSy1: consistent simulation of ozone from the surface to the mesosphere, Atmos. Chem. Phys., 6, 5067–5104, https://doi.org/10.5194/acp-6-5067-2006, 2006. a
Jokinen, T., Berndt, T., Makkonen, R., Kerminen, V.-M., Junninen, H., Paasonen, P., Stratmann, F., Herrmann, H., Guenther, A. B., Worsnop, D. R., Kulmala, M., Ehn, M., and Sipilä, M.: Production of extremely low volatile organic compounds from biogenic emissions: Measured yields and atmospheric implications, P. Natl. Acad. Sci. USA, 112, 7123–7128, 2015. a
Kavouras, I. G., Mihalopoulos, N., and Stephanou, E. G.: Formation of atmospheric particles from organic acids produced by forests, Nature, 395, 683–686, 1998. a
Kerkweg, A., Sander, R., Tost, H., and Jöckel, P.: Technical note: Implementation of prescribed (OFFLEM), calculated (ONLEM), and pseudo-emissions (TNUDGE) of chemical species in the Modular Earth Submodel System (MESSy), Atmos. Chem. Phys., 6, 3603–3609, https://doi.org/10.5194/acp-6-3603-2006, 2006. a, b
Klein Goldewijk, K., Beusen, A., Doelman, J., and Stehfest, E.: Anthropogenic land use estimates for the Holocene – HYDE 3.2, Earth Syst. Sci. Data, 9, 927–953, https://doi.org/10.5194/essd-9-927-2017, 2017. a
lingmüller, K., Metzger, S., Abdelkader, M., Karydis, V. A., Stenchikov, G. L., Pozzer, A., and Lelieveld, J.: Revised mineral dust emissions in the atmospheric chemistry–climate model EMAC (MESSy 2.52 DU_Astitha1 KKDU2017 patch), Geosci. Model Dev., 11, 989–1008, https://doi.org/10.5194/gmd-11-989-2018, 2018. a
Lana, A., Bell, T. G., Simó, R., Vallina, S. M., Ballabrera-Poy, J., Kettle, A. J., Dachs, J., Bopp, L., Saltzman, E. S., Stefels, J., Johnson, J. E., and Liss, P. S.: An updated climatology of surface dimethylsulfide concentrations and emission fluxes in the global ocean, Global Biogeochem. Cy., 25, GB1004, https://doi.org/10.1029/2010GB003850, 2011. a
Lathière, J., Hauglustaine, D. A., Friend, A. D., De Noblet-Ducoudré, N., Viovy, N., and Folberth, G. A.: Impact of climate variability and land use changes on global biogenic volatile organic compound emissions, Atmos. Chem. Phys., 6, 2129–2146, https://doi.org/10.5194/acp-6-2129-2006, 2006. a
Lathière, J., Hewitt, C., and Beerling, D.: Sensitivity of isoprene emissions from the terrestrial biosphere to 20th century changes in atmospheric CO2 concentration, climate, and land use, Global Biogeochem. Cy., 24, GB1004, https://doi.org/10.1029/2009GB003548, 2010. a
Lelieveld, J., Butler, T., Crowley, J., Dillon, T., Fischer, H., Ganzeveld, L., Harder, H., Lawrence, M., Martinez, M., Taraborrelli, D., and Williams, J.: Atmospheric oxidation capacity sustained by a tropical forest, Nature, 452, 737–740, 2008. a
Lohmann, U. and Ferrachat, S.: Impact of parametric uncertainties on the present-day climate and on the anthropogenic aerosol effect, Atmos. Chem. Phys., 10, 11373–11383, https://doi.org/10.5194/acp-10-11373-2010, 2010. a
Lohmann, U. and Kärcher, B.: First interactive simulations of cirrus clouds formed by homogeneous freezing in the ECHAM general circulation model, J. Geophys. Res.-Atmos., 107, AAC 8-1–AAC 8-13, https://doi.org/10.1029/2001JD000767, 2002. a
Lohmann, U., Feichter, J., Chuang, C. C., and Penner, J. E.: Prediction of the number of cloud droplets in the ECHAM GCM, J. Geophys. Res.-Atmos., 104, 9169–9198, 1999. a
Lohmann, U., Stier, P., Hoose, C., Ferrachat, S., Kloster, S., Roeckner, E., and Zhang, J.: Cloud microphysics and aerosol indirect effects in the global climate model ECHAM5-HAM, Atmos. Chem. Phys., 7, 3425–3446, https://doi.org/10.5194/acp-7-3425-2007, 2007. a
Martin, A., Gayler, V., Steil, B., Klingmüller, K., Jöckel, P., Tost, H., Lelieveld, J., and Pozzer, A.: Evaluation of the coupling of EMACv2.55 to the land surface and vegetation model JSBACHv4, Geosci. Model Dev., 17, 5705–5732, https://doi.org/10.5194/gmd-17-5705-2024, 2024. a
Nenes, A. and Seinfeld, J. H.: Parameterization of cloud droplet formation in global climate models, J. Geophys. Res.-Atmos., 108, 4415, https://doi.org/10.1029/2002JD002911, 2003. a
Nordeng, E. T.: Extended versions of the convective parametrization scheme at ECMWF and their impact on the mean and transient activity of the model in the tropics, Research Department Technical Memorandum, 206, 1–41, 1994. a
MESSY – Modular Earth Submodel System, http://www.messy-interface.org, last access: 7 January 2025. a
O'Donnell, D., Tsigaridis, K., and Feichter, J.: Estimating the direct and indirect effects of secondary organic aerosols using ECHAM5-HAM, Atmos. Chem. Phys., 11, 8635–8659, https://doi.org/10.5194/acp-11-8635-2011, 2011. a
Pöschl, U., von Kuhlmann, R., Poisson, N., and Crutzen, P. J.: Development and intercomparison of condensed isoprene oxidation mechanisms for global atmospheric modeling, J. Atmos. Chem., 37, 29–52, 2000. a
Pozzer, A., Jöckel, P., Sander, R., Williams, J., Ganzeveld, L., and Lelieveld, J.: Technical Note: The MESSy-submodel AIRSEA calculating the air-sea exchange of chemical species, Atmos. Chem. Phys., 6, 5435–5444, https://doi.org/10.5194/acp-6-5435-2006, 2006. a
Pozzer, A., Reifenberg, S. F., Kumar, V., Franco, B., Kohl, M., Taraborrelli, D., Gromov, S., Ehrhart, S., Jöckel, P., Sander, R., Fall, V., Rosanka, S., Karydis, V., Akritidis, D., Emmerichs, T., Crippa, M., Guizzardi, D., Kaiser, J. W., Clarisse, L., Kiendler-Scharr, A., Tost, H., and Tsimpidi, A.: Simulation of organics in the atmosphere: evaluation of EMACv2.54 with the Mainz Organic Mechanism (MOM) coupled to the ORACLE (v1.0) submodel, Geosci. Model Dev., 15, 2673–2710, https://doi.org/10.5194/gmd-15-2673-2022, 2022. a, b, c
Pringle, K. J., Tost, H., Message, S., Steil, B., Giannadaki, D., Nenes, A., Fountoukis, C., Stier, P., Vignati, E., and Lelieveld, J.: Description and evaluation of GMXe: a new aerosol submodel for global simulations (v1), Geosci. Model Dev., 3, 391–412, https://doi.org/10.5194/gmd-3-391-2010, 2010. a, b
Pye, H. O. T., Chan, A. W. H., Barkley, M. P., and Seinfeld, J. H.: Global modeling of organic aerosol: the importance of reactive nitrogen (NOx and NO3), Atmos. Chem. Phys., 10, 11261–11276, https://doi.org/10.5194/acp-10-11261-2010, 2010. a
Randerson, J., Van Der Werf, G., Giglio, L., Collatz, G., and Kasibhatla, P.: Global Fire Emissions Database, Version 4.1 (GFEDv4), ORNL DAAC [data set], Oak Ridge, Tennessee, USA, https://doi.org/10.3334/ORNLDAAC/1293, 2018. a
Roeckner, E., Brokopf, R., Esch, M., Giorgetta, M., Hagemann, S., Kornblueh, L., Manzini, E., Schlese, U., and Schulzweida, U.: Sensitivity of simulated climate to horizontal and vertical resolution in the ECHAM5 atmosphere model, J. Climate, 19, 3771–3791, 2006. a
Sander, R., Baumgaertner, A., Cabrera-Perez, D., Frank, F., Gromov, S., Grooß, J.-U., Harder, H., Huijnen, V., Jöckel, P., Karydis, V. A., Niemeyer, K. E., Pozzer, A., Riede, H., Schultz, M. G., Taraborrelli, D., and Tauer, S.: The community atmospheric chemistry box model CAABA/MECCA-4.0, Geosci. Model Dev., 12, 1365–1385, https://doi.org/10.5194/gmd-12-1365-2019, 2019. a
Schmale, J., Schneider, J., Nemitz, E., Tang, Y. S., Dragosits, U., Blackall, T. D., Trathan, P. N., Phillips, G. J., Sutton, M., and Braban, C. F.: Sub-Antarctic marine aerosol: dominant contributions from biogenic sources, Atmos. Chem. Phys., 13, 8669–8694, https://doi.org/10.5194/acp-13-8669-2013, 2013. a
Scott, C. E., Rap, A., Spracklen, D. V., Forster, P. M., Carslaw, K. S., Mann, G. W., Pringle, K. J., Kivekäs, N., Kulmala, M., Lihavainen, H., and Tunved, P.: The direct and indirect radiative effects of biogenic secondary organic aerosol, Atmos. Chem. Phys., 14, 447–470, https://doi.org/10.5194/acp-14-447-2014, 2014. a, b
Scott, C. E., Monks, S. A., Spracklen, D., Arnold, S., Forster, P., Rap, A., Äijälä, M., Artaxo, P., Carslaw, K., Chipperfield, M., Ehn, M., Gilardoni, S., Heikkinen, L., Kulmala, M., Petäjä, T., Reddington, C., Rizzo, L., Swietlicki, E., Vignati, E., and Wilson, C.: Impact on short-lived climate forcers increases projected warming due to deforestation, Nat. Commun., 9, 157, https://doi.org/10.1038/s41467-017-02412-4, 2018. a, b, c, d, e
Shrivastava, M., Cappa, C. D., Fan, J., Goldstein, A. H., Guenther, A. B., Jimenez, J. L., Kuang, C., Laskin, A., Martin, S. T., Ng, N. L., Petaja, T., Pierce, J. R., Rasch, P. J., Roldin, P., Seinfeld, J. H., Shilling, J., Smith, J. N., Thornton, J. A., Volkamer, R., Wang, J., Worsnop, D. R., Zaveri, R. A., Zelenyuk, A., and Zhang, Q.: Recent advances in understanding secondary organic aerosol: Implications for global climate forcing, Rev. Geophys., 55, 509–559, 2017. a
Sindelarova, K., Granier, C., Bouarar, I., Guenther, A., Tilmes, S., Stavrakou, T., Müller, J.-F., Kuhn, U., Stefani, P., and Knorr, W.: Global data set of biogenic VOC emissions calculated by the MEGAN model over the last 30 years, Atmos. Chem. Phys., 14, 9317–9341, https://doi.org/10.5194/acp-14-9317-2014, 2014. a
Smith, B., Prentice, I. C., and Sykes, M. T.: Representation of vegetation dynamics in the modelling of terrestrial ecosystems: comparing two contrasting approaches within European climate space, Global Ecol. Biogeogr., 10, 621–637, 2001. a
Smith, B., Wårlind, D., Arneth, A., Hickler, T., Leadley, P., Siltberg, J., and Zaehle, S.: Implications of incorporating N cycling and N limitations on primary production in an individual-based dynamic vegetation model, Biogeosciences, 11, 2027–2054, https://doi.org/10.5194/bg-11-2027-2014, 2014. a
Sporre, M. K., Blichner, S. M., Schrödner, R., Karset, I. H. H., Berntsen, T. K., van Noije, T., Bergman, T., O'Donnell, D., and Makkonen, R.: Large difference in aerosol radiative effects from BVOC-SOA treatment in three Earth system models, Atmos. Chem. Phys., 20, 8953–8973, https://doi.org/10.5194/acp-20-8953-2020, 2020. a, b
Spracklen, D. V., Jimenez, J. L., Carslaw, K. S., Worsnop, D. R., Evans, M. J., Mann, G. W., Zhang, Q., Canagaratna, M. R., Allan, J., Coe, H., McFiggans, G., Rap, A., and Forster, P.: Aerosol mass spectrometer constraint on the global secondary organic aerosol budget, Atmos. Chem. Phys., 11, 12109–12136, https://doi.org/10.5194/acp-11-12109-2011, 2011. a
Szogs, S., Arneth, A., Anthoni, P., Doelman, J. C., Humpenöder, F., Popp, A., Pugh, T. A., and Stehfest, E.: Impact of LULCC on the emission of BVOCs during the 21st century, Atmos. Environ., 165, 73–87, 2017. a
The MESSy Consortium: The Modular Earth Submodel System, Zenodo [code], https://doi.org/10.5281/zenodo.13768443, 2024. a
Tiedtke, M.: A comprehensive mass flux scheme for cumulus parameterization in large-scale models, Mon. Weather Rev., 117, 1779–1800, 1989. a
Tiitta, P., Vakkari, V., Croteau, P., Beukes, J. P., van Zyl, P. G., Josipovic, M., Venter, A. D., Jaars, K., Pienaar, J. J., Ng, N. L., Canagaratna, M. R., Jayne, J. T., Kerminen, V.-M., Kokkola, H., Kulmala, M., Laaksonen, A., Worsnop, D. R., and Laakso, L.: Chemical composition, main sources and temporal variability of PM1 aerosols in southern African grassland, Atmos. Chem. Phys., 14, 1909–1927, https://doi.org/10.5194/acp-14-1909-2014, 2014. a
Tilmes, S., Hodzic, A., Emmons, L., Mills, M., Gettelman, A., Kinnison, D. E., Park, M., Lamarque, J.-F., Vitt, F., Shrivastava, M., Campuzano-Jost, P., Jimenez, J., and Liu, X.: Climate forcing and trends of organic aerosols in the Community Earth System Model (CESM2), J. Adv. Model. Earth Sy., 11, 4323–4351, 2019. a, b
Tost, H., Jöckel, P., Kerkweg, A., Sander, R., and Lelieveld, J.: Technical note: A new comprehensive SCAVenging submodel for global atmospheric chemistry modelling, Atmos. Chem. Phys., 6, 565–574, https://doi.org/10.5194/acp-6-565-2006, 2006a. a
Tost, H., Jöckel, P., and Lelieveld, J.: Influence of different convection parameterisations in a GCM, Atmos. Chem. Phys., 6, 5475–5493, https://doi.org/10.5194/acp-6-5475-2006, 2006b. a
Tsigaridis, K. and Kanakidou, M.: Secondary organic aerosol importance in the future atmosphere, Atmos. Environ., 41, 4682–4692, 2007. a
Tsimpidi, A. P., Karydis, V. A., Pozzer, A., Pandis, S. N., and Lelieveld, J.: ORACLE (v1.0): module to simulate the organic aerosol composition and evolution in the atmosphere, Geosci. Model Dev., 7, 3153–3172, https://doi.org/10.5194/gmd-7-3153-2014, 2014. a, b
Tsimpidi, A. P., Karydis, V. A., Pandis, S. N., and Lelieveld, J.: Global combustion sources of organic aerosols: model comparison with 84 AMS factor-analysis data sets, Atmos. Chem. Phys., 16, 8939–8962, https://doi.org/10.5194/acp-16-8939-2016, 2016. a, b
Tsimpidi, A. P., Karydis, V. A., Pandis, S. N., and Lelieveld, J.: Global-scale combustion sources of organic aerosols: sensitivity to formation and removal mechanisms, Atmos. Chem. Phys., 17, 7345–7364, https://doi.org/10.5194/acp-17-7345-2017, 2017. a
Tsimpidi, A. P., Karydis, V. A., Pozzer, A., Pandis, S. N., and Lelieveld, J.: ORACLE 2-D (v2.0): an efficient module to compute the volatility and oxygen content of organic aerosol with a global chemistry–climate model, Geosci. Model Dev., 11, 3369–3389, https://doi.org/10.5194/gmd-11-3369-2018, 2018. a
Unger, N.: Human land-use-driven reduction of forest volatiles cools global climate, Nat. Clim. Change, 4, 907–910, https://doi.org/10.1038/nclimate2347, 2014. a, b
Vehkamäki, H., Kulmala, M., Napari, I., Lehtinen, K. E., Timmreck, C., Noppel, M., and Laaksonen, A.: An improved parameterization for sulfuric acid–water nucleation rates for tropospheric and stratospheric conditions, J. Geophys. Res.-Atmos., 107, AAC 3-1-AAC 3-10, https://doi.org/10.1029/2002JD002184, 2002. a
Vella, R., Forrest, M., Lelieveld, J., and Tost, H.: Isoprene and monoterpene simulations using the chemistry–climate model EMAC (v2.55) with interactive vegetation from LPJ-GUESS (v4.0), Geosci. Model Dev., 16, 885–906, https://doi.org/10.5194/gmd-16-885-2023, 2023a. a, b, c, d
Vella, R., Pozzer, A., Forrest, M., Lelieveld, J., Hickler, T., and Tost, H.: Changes in biogenic volatile organic compound emissions in response to the El Niño–Southern Oscillation, Biogeosciences, 20, 4391–4412, https://doi.org/10.5194/bg-20-4391-2023, 2023b. a
Vella, R., Forrest, M., Pozzer, A., Tsimpidi, A. P., Hickler, T., Lelieveld, J., and Tost, H.: Data used in “Land use change influence on atmospheric organic gases, aerosols, and radiative effects” (Vella et al., 2024), Zenodo [data set], https://doi.org/10.5281/zenodo.13906983, 2024. a
Vicente-Serrano, S. M., Van der Schrier, G., Beguería, S., Azorin-Molina, C., and Lopez-Moreno, J.-I.: Contribution of precipitation and reference evapotranspiration to drought indices under different climates, J. Hydrol., 526, 42–54, 2015. a
Weber, J., Archer-Nicholls, S., Abraham, N. L., Shin, Y. M., Griffiths, P., Grosvenor, D. P., Scott, C. E., and Archibald, A. T.: Chemistry-driven changes strongly influence climate forcing from vegetation emissions, Nat. Commun., 13, 7202, https://doi.org/10.1038/s41467-022-34944-9, 2022. a
Weber, J., King, J. A., Sindelarova, K., and Val Martin, M.: Updated isoprene and terpene emission factors for the Interactive BVOC (iBVOC) emission scheme in the United Kingdom Earth System Model (UKESM1.0), Geosci. Model Dev., 16, 3083–3101, https://doi.org/10.5194/gmd-16-3083-2023, 2023. a
Weber, J., King, J. A., Abraham, N. L., Grosvenor, D. P., Smith, C. J., Shin, Y. M., Lawrence, P., Roe, S., Beerling, D. J., and Martin, M. V.: Chemistry-albedo feedbacks offset up to a third of forestation’s CO2 removal benefits, Science, 383, 860–864, 2024. a
Zhang, Q., Parworth, C., Lechner, M., and Jimenez, J.: Aerosol Mass Spectrometer Global Database [data set], https://sites.google.com/site/amsglobaldatabase (last access: 6 January 2025), 2010. a
Zhu, J., Penner, J. E., Yu, F., Sillman, S., Andreae, M. O., and Coe, H.: Decrease in radiative forcing by organic aerosol nucleation, climate, and land use change, Nat. commun., 10, 423, https://doi.org/10.1038/s41467-019-08407-7, 2019. a