the Creative Commons Attribution 4.0 License.
the Creative Commons Attribution 4.0 License.
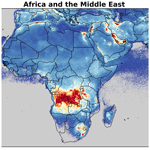
Feasibility of robust estimates of ozone production rates using a synergy of satellite observations, ground-based remote sensing, and models
Amir H. Souri
Gonzalo González Abad
Glenn M. Wolfe
Tijl Verhoelst
Corinne Vigouroux
Gaia Pinardi
Steven Compernolle
Bavo Langerock
Bryan N. Duncan
Matthew S. Johnson
Ozone pollution is secondarily produced through a complex, non-linear chemical process. Our understanding of the spatiotemporal variations in photochemically produced ozone (i.e., PO3) is limited to sparse aircraft campaigns and chemical transport models, which often carry significant biases. Hence, we present a novel satellite-derived PO3 product informed by bias-corrected TROPOspheric Monitoring Instrument (TROPOMI) HCHO, NO2, surface albedo data, and various models. These data are integrated into a parameterization that relies on HCHO, NO2, HCHO NO2, jNO2, and jO1D. Despite its simplicity, it can reproduce ∼ 90 % of the variance in observationally constrained PO3, with minimal biases in moderately to highly polluted regions. We map PO3 across various regions with respect to July 2019 at a 0.1° × 0.1° spatial resolution, revealing accelerated values (> 8 ppbv h−1) for numerous cities throughout Asia and the Middle East, resulting from elevated ozone precursors and enhanced photochemistry. In Europe and the United States, such high levels are only detected over Benelux, Los Angeles, and New York City. PO3 maxima are observed in various seasons and are attributed to changes in photolysis rates, non-linear ozone chemistry, and fluctuations in HCHO and NO2. Satellite errors result in moderate errors (10 %–20 %) in PO3 estimates over cities on a monthly average basis, while these errors exceed 50 % in clean areas and under low light conditions. Using the current algorithm, we demonstrate that satellite data can provide valuable information for robust PO3 estimation. This capability expands future research through the application of data to address significant scientific questions about locally produced ozone hotspots, seasonality, and long-term trends.
- Article
(21761 KB) - Full-text XML
-
Supplement
(667 KB) - BibTeX
- EndNote
Tropospheric ozone (O3) is a secondary pollutant formed through complex photochemical reactions involving various precursors, including nitrogen oxides (NOx = NO + NO2), volatile organic compounds (VOCs), aerosols, and halogens (Kleinman et al., 2002; Simpson et al., 2015; Li et al., 2019). Ozone not only poses significant risks to human health (Fleming et al., 2018) and agricultural productivity (Mills et al., 2018) but also influences the radiation budget, thereby affecting the climate (Gaudel et al., 2018). To mitigate the problem of elevated locally produced ozone, it is crucial to understand the spatiotemporal variability in the ozone production rate (PO3), defined as the number of ozone molecules generated through secondary chemical pathways in the atmosphere. Comprehensive studies of ozone chemistry, informed by observations, are typically confined to observationally rich air quality campaigns (e.g., Cazorla et al., 2012; Ren et al., 2013; Mazzuca et al., 2016; Souri et al., 2020a; Schroeder et al., 2020; Brune et al., 2022; Wolfe et al., 2022; Souri et al., 2023), which are sparse in time and space.
Significant advancements have been made in using various measurable ozone indicators to simplify the non-linear relationship between PO3, NOx, and VOCs into linear forms (Sillman and He, 2002). These forms include NOx-sensitive regimes (where PO3 is sensitive to NOx), VOC-sensitive regimes (where PO3 is sensitive to VOCs), and transitional regimes (where PO3 is sensitive to both NOx and VOCs). Among the numerous proposed indicators, the ratio of formaldehyde (HCHO) to nitrogen dioxide (NO2) (known as the FNR) has gained popularity (Tonnesen and Dennis, 2000a, b), despite its less effective performance compared to that of the H2O2 HNO3 ratio in fully explaining the HOx–ROx cycle (Silman and He, 2002; Souri et al., 2023). The preference for the FNR stems from the fact that both HCHO and NO2 can be informed by UV–Vis radiance data, such as those provided by the Ozone Monitoring Instrument (OMI) and the TROPOspheric Monitoring Instrument (TROPOMI) (Martin et al., 2004; Duncan et al., 2010; Choi et al., 2012; Choi and Souri, 2015a, b; Jin and Holloway, 2015; Jin et al., 2017; Schroeder et al., 2017; Souri et al., 2017; Jeon et al., 2018; Tao et al., 2022). Several limitations associated with the application of satellite-based FNRs have been identified, such as (i) the inherent limitations in understanding the radical termination in the HOx–ROx cycle (Souri et al., 2020a, 2023), (ii) the challenges associated with converting the column vertical density to near-surface concentrations (Jin et al., 2017; Schroeder et al., 2017; Souri et al., 2023), (iii) the spatial representativity associated with large satellite pixels (Souri et al., 2020a, 2023; Johnson et al., 2023), and (iv) retrieval errors (Souri et al., 2023; Johnson et al., 2023). Souri et al. (2023) concluded that retrieval errors make up the largest portion of the total errors associated with FNRs. These errors are becoming smaller with improved sensor designs, retrieval algorithms, and calibration over time.
While the characterization of ozone regimes offers valuable insights for regulators to prioritize effective emission control strategies, it does not provide information about the magnitude of PO3 or the absolute quantities of PO3 derivatives relative to its precursors. Consequently, chemical transport models under various emission scenarios are typically employed (e.g., Pan et al., 2019). These models allow for the execution of process-based scenarios to elucidate the response of PO3 to different emissions and can simulate four-dimensional PO3 data. However, the results of these simulations are based on various assumptions and inputs, which carry significant uncertainties. Therefore, it is essential to optimize some of the models' prognostic inputs using observations through inverse modeling/data assimilation. The primary advantage of inverse modeling/data assimilation using satellite observations is its ability to account for satellite errors and eliminate the influence of the a priori profile, thereby carrying only radiance information into the emission estimation. Numerous studies have utilized satellite observations to constrain NOx and VOC emissions for various applications (e.g., Stavrakou et al., 2016; Souri et al., 2016a; Miyazaki et al., 2017; Souri et al., 2017, 2020b, 2021; Choi et al., 2022; DiMaria et al., 2023). Souri et al. (2020b) made an early attempt to simultaneously optimize both NOx and VOC emissions over eastern Asia for a more accurate representation of PO3. Their joint inversion was able to account for the intertwined relationships between HCHO–NOx and NO2–VOC. However, the execution of chemical transport models optimized by multiple satellite observations remains prohibitively expensive, particularly with respect to high-resolution domains, such as those demanded by regulatory agencies.
Data-driven methods for estimating PO3 can serve as a more cost-effective alternative to physics-based methods. While using constrained chemical transport models provides a relatively robust framework grounded in certain explicit governing equations, these models require extensive computation resources and expertise. Conversely, data-driven algorithms make use of large datasets to identify patterns and make predictions with significantly reduced computational expenses. However, it is important to recognize that data-driven algorithms lack the ability to provide solid physical interpretability and generalizability. Despite this fundamental limitation, they are sensible tools for applications where rapid analysis over a wide spatial coverage is prioritized. Data-driven parameterizations for several components of atmospheric chemistry, such as OH (Anderson et al., 2022) and dry deposition (Silva et al., 2019), have been crafted for this reason. However, to our best knowledge, Chatfield et al. (2010) and Souri et al. (2023) are the only studies that have attempted to empirically parameterize PO3 using information from HCHO and NO2 mixing ratios.
Inspired by these works, we developed a novel product using TROPOMI observations, in conjunction with ground-based remote sensing and atmospheric models, to estimate PO3 and associated errors within the planetary boundary layer (PBL) across the globe. This enabled us to map PO3 across various regions at fine scales (i.e., 0.1° × 0.1°) for the first time.
2.1 Aircraft
To study PO3, we use various aircraft observations from several National Aeronautics and Space Administration (NASA) and National Oceanic and Atmospheric Administration (NOAA) atmospheric composition campaigns. We selected three sets of aircraft campaigns for the purpose of PO3 estimation, targeting (i) urban and suburban air quality using the Deriving Information on Surface Conditions from Column and Vertically Resolved Observations Relevant to Air Quality (DISCOVER-AQ) Baltimore–Washington (2011), DISCOVER-AQ Texas (2013), DISCOVER-AQ Colorado (2014), and Korea–United States Air Quality (KORUS-AQ; 2016) campaigns (Crawford et al., 2021); (ii) remote areas using the Atmospheric Tomography Mission (ATom) (Thompson et al., 2022) and the Intercontinental Chemical Transport Experiment – Phase B (INTEX-B) (Singh et al., 2009); and (iii) a mixture of isoprene-rich environments and large emitters using SENEX (Southeast Nexus) (Warneke et al., 2016). Figure 1 shows the locations of these campaigns. Inspired by a study by Miller and Brune (2022), we list the “when”, “where”, and “why” characteristics of these campaigns in Table S1 in the Supplement.
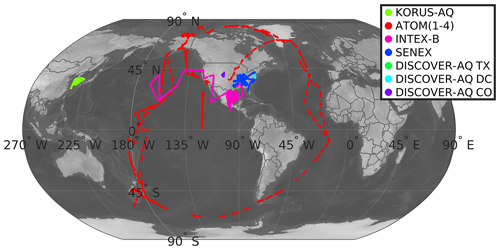
Figure 1The locations of the seven different atmospheric composition aircraft campaigns used in this study. KORUS-AQ: Korea–United States Air Quality. ATom: Atmospheric Tomography Mission. INTEX-B: Intercontinental Chemical Transport Experiment – Phase B. SENEX: Southeast Nexus. DISCOVER-AQ refers to the Deriving Information on Surface Conditions from Column and Vertically Resolved Observations Relevant to Air Quality campaigns in Texas (TX), Washington DC (DC), and Colorado (CO).
For aircraft campaigns targeting polluted areas, including DISCOVER-AQ, KORUS-AQ, and SENEX, we use 10 s merged data, whereas for measurement campaigns conducted in relatively remote areas, such as INTEX-B and ATom, we use 30 s merged data. A more detailed description of the measurements is provided in Sect. 3.2. We exclude times with no measurements of NO, NO2, or HCHO. The concentrations of OH and HO2 were only measured during the INTEX-B, ATom, and KORUS-AQ campaigns. Likewise, we void any data points lacking either HO2 or OH measurements for these campaigns. There are frequent gaps in some measurements, especially for VOCs, due to instrument issues or measurement techniques. Following Souri et al. (2020a), Miller and Brune (2022), Souri et al. (2023), and Bottorff et al. (2023), we fill the gaps in the measurements using a linear interpolation method, with no extrapolation allowed beyond 15 min. We drop any remaining gaps from the analysis. To better capture the rapid fluctuation in VOCs, we select the proton-transfer-reaction time-of-flight mass spectrometer (PTR-ToF-MS) instrument with high temporal resolution over the whole air sampler (WAS) when both instruments have measured the same quantity. Regarding the INTEX-B campaign, we drop the isoprene observations due to infrequent samples that downgrade the performance of our box model.
2.2 TROPOMI NO2 and HCHO
We use recently reprocessed daily Level-2 (L2) TROPOMI tropospheric NO2 and total HCHO columns (v2.4), derived from UV–Vis radiances captured by the European Space Agency (ESA) Sentinel-5 Precursor (S5P) spacecraft (∼ 328–496 nm) (Veefkind et al., 2012; De Smedt et al., 2021; van Geffen et al., 2022). This sensor has been operational since May 2018, providing global coverage of NO2 and HCHO at ∼ 01:30 local standard time at the Equator. Since NO2 and HCHO are optically thin absorbers in the UV–Vis range, meaning their concentrations do not substantially affect the sensitivity of the radiance to the optical thickness of the absorber, the retrieval follows a conventional two-step algorithm involving spectral fitting for slant column density (SCD) retrieval and air mass factor (AMF) calculations for conversion from SCDs to vertical column densities (VCDs). The product has a spatial resolution of 7.2 km (5.6 km as of August 2019) by 3.6 km at nadir. To remove unfit measurements, we use the provided quality flag (q_value) and choose only measurements above 0.75 for NO2 and 0.5 for HCHO. As the L2 product is not provided on a regular grid, we use a mass-conserved regridding technique based on barycentric linear interpolation to map the data onto a 0.1° × 0.1° regular grid.
Van Geffen et al. (2022) demonstrated that the reprocessed TROPOMI tropospheric NO2 columns exhibit a good level of correspondence with those obtained from ground-based MAX-DOAS (Multi-Axis Differential Optical Absorption Spectroscopy) sky spectrometers, with a correlation of 0.88 and a median bias of −23 %, improving on the older product versions, which were biased low by about 30 % with respect to ground-based measurements at polluted sites (Verhoelst et al., 2021). More information about the new modifications and their impacts on the retrieval can be found in van Geffen et al. (2022).
Studies by Vigouroux et al. (2020) and De Smedt et al. (2021) validated the reprocessed monthly-mean TROPOMI HCHO columns against Fourier-transform infrared (FTIR) and MAX-DOAS observations and found a good correlation above 0.8, with a negative bias of 20 %–30 % for polluted sites. The bias tends to be slightly positive or neutral over clean sites.
Error characterization of TROPOMI NO2 and HCHO using ground-based retrievals
To propagate TROPOMI retrieval errors to the PO3 product and to remove potential biases, we assume three origins for the errors: (i) random errors resulting from instrument noise, (ii) a fixed additive component that is magnitude-independent (i.e., a uniform offset persisting over all pixels), and (iii) unresolved systematic biases that are multiplicative and irreducible by oversampling. The first component is derived from the column precision variable provided along with the L2 product. In the spatial domain, we interpolate the squares of these errors in the same way we map the irregular L2 pixels onto the 0.1° × 0.1° regular grid. Moreover, we average the random errors over a month to reduce random noise by the square root of the number of pixels available at the same location (Eq. 3). Two other errors are determined by comparing FTIR observations for HCHO and MAX-DOAS observations for tropospheric NO2 with TROPOMI data (Sect. 4.3.3). A detailed explanation of how these datasets are paired can be found in Vigouroux et al. (2020) and Verhoelst et al. (2021). Both datasets cover the period from 2018–2023.
To achieve an optimal linear fit () between the paired observations – where a and b are the slope and offset to be determined, respectively – we follow a Monte Carlo chi-squared minimization such that is minimized. In this equation, and are the variances of y (TROPOMI) and x (the benchmark, i.e., FTIR or MAX-DOAS), respectively; the subscript i refers to the ith observation point; and f is the proposed linear fit subject to optimization. In terms of TROPOMI NO2 and HCHO, the errors are populated based on the L2 information. According to Verhoelst et al. (2021), a fixed error of 30 % is assumed for MAX-DOAS NO2 observations whose values are above 1.4 × 1015 molec. cm−2. Because of the detection limit of MAX-DOAS NO2, we set errors for values below that threshold to 1.4 × 1015 molec. cm−2. The FTIR retrieval errors described in Vigouroux et al. (2020) were used to populate the errors associated with this benchmark. The minimization is performed 10 000 times, each time with a set of random perturbations of x and y within their respective prescribed errors. This approach allows us to assess the robustness of the estimates across the range of errors associated with each data point.
The offset (a uniform additive term) and slope (a multiplicative error) drawn from the ground validation are used to correct the biases associated with TROPOMI via
Since there are errors associated with this adjustment resulting from instrument and representation errors, we augment the error in the slope and offset with respect to the total error and label it as the constant error (econst) using
where and are the squares of the errors in the offset and slope, calculated from the linear regression (Eq. 1). Ultimately, the sum of all three errors constitutes the total error, as shown in the following:
where m is the number of samples for a given grid and time frame and represents the square of the random errors.
2.3 TROPOMI surface albedo
To account for the effect of surface albedo on photolysis rates (Sect. 2.5), we use a newly developed algorithm based on the directionally dependent Lambertian-equivalent reflectivity (DLER) UV surface albedo climatology derived from TROPOMI radiance (Tilstra et al., 2024). This new database leverages 60 months of TROPOMI reprocessed radiance data and is produced at a grid resolution of 0.125° × 0.125°. This product outperformed traditional Lambertian-equivalent reflectivity (LER) products, such as OMI, when they were compared to MODIS surface bidirectional reflectance distribution function (BRDF) results (Tilstra et al., 2024).
2.4 Modern-Era Retrospective analysis for Research and Applications, Version 2 – Global Modeling Initiative (MERRA-2 GMI)
To convert TROPOMI vertical column densities of HCHO and NO2 to their volume mixing ratios in the PBL region, we use the MERRA-2 GMI (M2GMI) model (https://acd-ext.gsfc.nasa.gov/Projects/GEOSCCM/MERRA2GMI/, last access: 10 September 2023). This model serves as NASA's Goddard Earth Observing System (GEOS) chemistry–climate model (CCM), spanning the period of 1980–2019 and exploiting MERRA-2 (the second version of the Modern-Era Retrospective analysis for Research and Applications) to constrain meteorological fields (Orbe et al., 2017). The model uses the Global Modeling Initiative (GMI) chemical mechanism (Duncan et al., 2007), which involves over 120 species and 400 reactions. It has a resolution of approximately 0.5° latitude by 0.625° longitude, with 72 vertical layers stretching from the surface up to 0.1 hPa. Additional information about the configuration of this model can be found in Strode et al. (2019). To carry out the conversion, we apply the following conversion factor (γ) to the TROPOMI VCDs:
where is the average of the target trace gas mixing ratios within the planetary boundary layer height (PBLH), g is the acceleration of gravity (assumed to be 9.81 m s−2), NA is the Avogadro constant, Mair is the air molecular weight (assumed to be 28.96 g mol−1), q is the target trace gas mixing ratio at a given altitude, and dp is the thickness of each vertical grid box in the model (simulated in hectopascals). The denominator in Eq. (4) represents the modeled VCD. We integrate the modeled partial VCDs up to the top of the atmosphere for HCHO and up to the tropopause pressure layer for NO2.
2.5 The National Center for Atmospheric Research (NCAR) Tropospheric Ultraviolet and Visible (TUV) photolysis rate lookup table
To estimate the photolysis rates, JNO2 (NO2 + hν) and JO1D (O3 + hν), we use a comprehensive lookup table provided by the Framework for 0-D Atmospheric Modeling (F0AM) model (Sect. 3.2), created for clear-sky conditions. This lookup table is based on the calculation of more than 20 064 solar spectra over a wide range of solar zenith angles (SZAs) (a 0–90° range in steps of 5°), altitudes (a 0–15 km range in steps of 1 km), overhead total ozone column values (a 100–600 DU range in steps of 50 DU), and surface UV albedo values (a 0–1 range in steps of 0.2), using NCAR's Tropospheric Ultraviolet and Visible (TUV) radiation model (v5.2) and cross sections and quantum yields from the International Union of Pure and Applied Chemistry (IUPAC) and the Jet Propulsion Laboratory (JPL) (Wolfe et al., 2016). The L2 TROPOMI granule information populates the SZA, surface elevation, and surface UV albedo, while overhead total ozone columns are obtained from MERRA-2 GMI (Sect. 2.4), which is found to agree well with satellite observations (Souri et al., 2024). Any values between these tables are bilinearly interpolated for a smoother result.
In this section, we begin by discussing a robust regression model specifically developed for feature selection in the parameterization of PO3. We then describe the training dataset created for this purpose. Following that, we introduce a clustering technique, utilized to organize the training data, which enables us to identify the key drivers of PO3 variability. Finally, we provide a comprehensive overview of the PO3 estimation algorithm by integrating data from the TROPOMI retrievals, ground-based remote sensing, and various models.
3.1 Least absolute shrinkage and selection operator (LASSO)
Through the use of multilinear regression models, it is possible to establish a simple but robust relationship between multiple variables and a target. However, when dealing with a large number of variables, there is a chance of introducing overfitting issues. This can lead to predictions that are either overly optimistic or unrealistic for values outside of the training dataset. To avoid this, it is recommended to simplify the model by removing variables that are loosely connected with the target or highly correlated with other variables. This process is known as “model shrinkage” and can narrow down the number of possible solutions (i.e., variance) at the cost of increasing the biases between the observed target and predictions. Ideally, we want a model that minimizes the sum of the bias and the variance. To achieve this, we can use the least absolute shrinkage and selection operator (LASSO) (Tibshirani, 1996). This operator considers the following regression:
where is the response, X corresponds to n × p explanatory variables, represents the coefficients, α is the intercept, and ε=(ε1, …, εn)T represents the noise variables. Moreover, n represents the number of data points, and p represents the number of explanatory variables. We can label the regression model as sparse when many of the β values are zero, and we can label it as high-dimensional when p≫n. LASSO attempts to select variables such that the following cost function is minimized:
where and represent the optimized intercept and coefficients, respectively; λ is a non-negative regularization factor subject to tuning; i is the subscript of the ith explanatory variable; and ∥.∥2 is the L2-norm-based operator. The first term on the right side of Eq. (6) minimizes the squares of the residuals, whereas the second term reduces the sum of the absolute values of the coefficients, resulting in a simpler model with fewer parameters. Without the second term, the regression model becomes an ordinary least-squares estimation. The most critical element here is λ. A large λ value results in more aggressive regularization, leading to more model shrinkage, whereas a small value preserves a high-dimensional model. To optimize this value, we discretize λ into 100 values between 10−4 and 101, divide the training dataset into 10 folds (i.e., split the dataset into equally sized segments), determine the average of the cross-validated error prediction among all folds, and find the λ value that yields the smallest error. The final solution ensures a balanced model with respect to model parsimony and bias. All explanatory variables are standardized during the regularization procedure such that their mean becomes zero and their standard deviation becomes 1.
3.2 Photochemical box modeling
To produce training datasets for LASSO-based PO3 estimation, we use the Framework for 0-D Atmospheric Modeling (F0AM) box model (v4; Wolfe et al., 2016), constrained by a wide range of observations. These observations ensure that the model achieves a realistic range of values consistent with those found in the atmosphere. We follow past setups that apply the Carbon Bond 6 (CB06, r2) chemical mechanism within the F0AM model (Souri et al., 2020a, 2023). The model is constrained by aircraft data, including meteorology, photolysis rates, and trace gas concentrations. The model configuration and observations used are listed in Table S2.
Once the model is initialized and held constant with respect to a wide range of constraining quantities, it runs with a 30 min integration time cycle for 5 d to approach a steady-state environment. Several key compounds, including OH, HO2, HCHO, polyacrylonitrile (PAN), NO, and NO2, are initialized with aircraft observations, but they are left free to cycle with incoming solar-radiation variability. These compounds play a crucial role in validating the efficacy of model performance as well as the adequacy of the observations used as constraints. In particular, allowing HCHO to vary freely enables us to assess whether our mechanism for VOC treatment, the steady-state conditions, and the number of measured VOCs are sufficient for reasonably reproducing its concentrations. Although the individual concentrations of NO2 and NO are not constrained, we constrain the total NOx (NO + NO2). Not all aircraft campaigns measured all photolysis rates included in the chemical mechanism. We first initialize the photolysis rates included in CB06 using the lookup tables described in Sect. 2.5. If any photolysis reaction rates in CB06 were measured, we replace the initial guess with the observed values. For reactions with unmeasured photolysis rates, we apply a scaling factor made from the average ratio of the observed J values to the modeled J values. This approach is a sensible choice for accounting for large particles, such as clouds, as their extinction coefficient is somewhat non-selective in the UV–Vis range; however, applying a wavelength-independent scaling factor may introduce some biases into optically complex environments introduced by aerosols.
It is essential to acknowledge the inherent limitations of the box model in our research. The model does not consider the diverse physical loss pathways that trace gases may undergo, including deposition and transport. As a result, we simplify the physical loss by employing a first-order dilution rate equivalent to a lifetime of 24 h. This approach ensures that unconstrained trace gases, which take longer to break down, do not accumulate over time. Exact knowledge of dilution factors requires understanding molecular and turbulent diffusion, entrainment, detrainment, and deposition rates, all of which are unknown at the microscale level of aircraft observations. Nonetheless, studies by Brune et al. (2022) and Souri et al. (2023) showed that HO2, OH, NOx, and HCHO are relatively immune to the choice of dilution factor, whereas RO2 mixing ratios can vary, introducing some biases into PO3 estimates.
We determine the simulated PO3 using
where LO3 represents all possible chemical loss pathways of ozone (a negative stoichiometric multiplier matrix) and FO3 represents all possible chemical pathways producing ozone molecules (a positive stoichiometric multiplier matrix). This calculation is theoretically equivalent to the value obtained from a chemical solver quantifying the number of ozone molecules produced/lost at each model time step. The adoption of Eq. 7 facilitates the direct comparison of PO3 estimations with estimations derived from other models, including chemical-transport-model-based results (see Fig. 10 in Souri et al., 2021). Furthermore, it allows for seamless integration of these estimates into Lagrangian transport models for ozone forecasting purposes.
3.3 Clustering
The aim of using a classifier to categorize the large quantity and variety of aircraft data into groups with similar features is to enable us to study the primary contributors to PO3 under different chemical, solar, and meteorological conditions. Additionally, this approach helps us understand the range of atmospheric conditions included in the training dataset. To accomplish this, we employ a widely used technique known as k-means, which has been used in a variety of applications (e.g., Beddows et al., 2009; Souri et al., 2016b; Govender and Sivakumar, 2020). In this approach, centroids are distributed randomly throughout a multidimensional dataset, with each centroid representing a distinct class. The algorithm then assigns a label to each data point by identifying its shortest Euclidean distance to the centroids. Following the labeling of all data points, the algorithm updates the centroids based on the means of the newly labeled groups. This process continues iteratively until there is minimal change in the location of the centroids. It is worth noting that k-means does not guarantee an optimal solution, so we reinitialize the classification 1000 times with a new set of initial centroids. We select the result with the lowest value for the sum of the Euclidean distances among the data points and centroids to ensure the outcomes are not influenced by random seeding.
Redundant features in the input can significantly compromise the effectiveness of the classification, so we apply principal component analysis (PCA) to a matrix of datasets (Z) with n data points and p features to reduce the dimensions to those of a PCA-transformed matrix (Z′), i.e., n × q, where q < p. Despite this reduction in dimensions, Z′ preserves a significant variance in Z, helping us to overcome issues of dimensionality or overfitting.
We select 11 features simulated by the F0AM model, many of which are set to the observed values or have observationally constrained precursors. These features comprise the SZA, HCHO NO2, HCHO × NO2, HCHO, NO2, pressure, temperature, jNO2, jO1D, H2O, and NO2 NOy (NOy = NO + NO2 + PAN + HNO3 + alkyl nitrate + N2O5). There are indeed correlations among these features, such as between the SZA and jNO2 or between HCHO and HCHO × NO2; nonetheless, we use PCA to eliminate the possibility of these correlated factors causing overfitting issues.
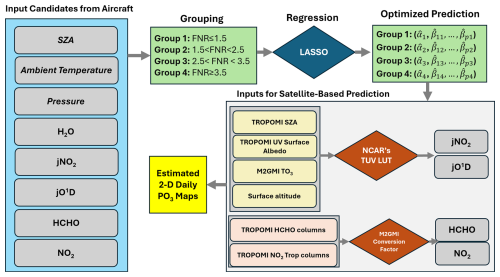
Figure 2Schematic illustration of the daily PO3 estimation process demonstrated in this study. This process consists of two major steps: (1) formulating PO3 as a function of various prognostic inputs derived from the box model results and (2) predicting PO3 based on optimized features/coefficients suggested by LASSO and using information obtained from TROPOMI, the TUV model, and M2GMI. LUT: lookup table. Trop columns: tropospheric columns.
3.4 The estimation of PO3
In order to predict PO3, we developed empirical equations using LASSO to link PO3 with various relevant prognostic candidates related to ozone chemistry. A schematic presentation of how this estimation can be carried out to provide daily PO3 maps corresponding to the TROPOMI revisit time across the globe is shown in Fig. 2. It is important to note that relying solely on linear regressions for a non-linear problem is not a viable approach. To address this, we divided the data points into four distinct groups based on FNR values, meaning we divided a non-linear realm into smaller linear segments (i.e., an empirical linearization). In a study by Souri et al. (2023), a wide range of aircraft observations and box model results were used to determine that FNR values of ∼ 1.7 were a universal threshold for separating NOx-sensitive from VOC-sensitive regimes. We found that by breaking down the data points into slightly weaker or stronger variations within the regimes, we can improve the accuracy of our results. As a result, we established four distinct groups: VOC-sensitive regimes (FNR < 1.5), transitional regimes (1.5 < FNR < 2.5 and 2.5 < FNR < 3.5), and NOx-sensitive regimes (FNR > 3.5). The coefficients and intercepts based on the LASSO regressions for each group were computed separately. From a long list of explanatory parameters, we selected the SZA, temperature, pressure, H2O, jNO2, jO1D, HCHO, and NO2 as the most sensible candidates. The reasoning behind this selection will be discussed in Sect. 4.2.
Once the LASSO parameters are determined, we apply the linear functions to the variables modeled/observed in the PBL region. We show that the LASSO method suggests dropping the SZA, temperature, water vapor, and pressure as they do not provide significant information on PO3 compared to the other parameters. As for jNO2 and jO1D, we use the NCAR's TUV lookup table, described in Sect. 2.5. HCHO and NO2 are derived by converting the bias-corrected TROPOMI VCDs into PBL mixing ratios using MERRA-2 GMI, as described in Sect. 2.4. To carry out this conversion, we multiply the satellite VCDs by the ratio of the averaged modeled mixing ratios of a target gas (i.e., NO2 or HCHO) in the PBL region, divided by the modeled VCDs (Sect. 2.4). The PBL field also comes from MERRA-2 GMI.
4.1 Box model validation
In order to assess the accuracy of the assumptions used in the box model's setup, which involves factors such as chemical mechanisms, dilution rates, and photolysis rate corrections, we will compare the simulated values of HCHO, NO2, NO, PAN, HO2, and OH with their actual measured values. This comparison will help us determine whether our model falls within an acceptable range of errors, as seen in other reputable studies of photochemical box modeling. This comparison is shown in Fig. 3, which displays scatterplots illustrating data collected from all seven aircraft campaigns. A discussion of each parameter follows:
-
HCHO. The box model is proficient in capturing over 77 % of the variance in observations with less than 15 % absolute bias. While many box modeling studies prefer to have this compound constrained to potentially enhance the representation of HOx, doing so comes with the trade-off of hindering our ability to validate the number and quality of observed HCHO precursors and/or the treatment of VOCs. Besides the study by Souri et al. (2023), the work of Marvin et al. (2017) serves as one of the few studies that did not constrain this compound, aiming to verify the efficacy of different pathways involved in HCHO formation and loss as simulated by various chemical mechanisms. Marvin et al. (2017) reproduced HCHO formation during the SENEX campaign using the CB06 mechanism, achieving an R2 value of 0.66 and a bias of 32 % with 1 min averaged samples. In comparison, we recreated a variance of 86 % in observed HCHO during the same campaign, with a bias of 23 % (Fig. S1), using 10 s averaged samples. The remaining unresolved variance can be attributed to an incomplete list of VOC measurements from several campaigns, including DISCOVER-AQ, and errors in VOC measurements. It is unlikely that the chemical mechanism is the reason for this, as Marvin et al. (2017) did not observe substantial differences in R2 values among various chemical mechanisms, including the near-explicit Master Chemical Mechanism (MCM). A mild underestimation of HCHO could likely be due to the steady-state assumption, a fixed arbitrary dilution factor, or uncertain isoprene chemistry (Archibald et al., 2010; Wolfe et al., 2016; Marvin et al., 2017).
-
NO2 and NO. Comparisons for both species demonstrate a high degree of correspondence for values above 0.1 ppbv. Nonetheless, we note a substantial amount of fluctuation in the simulations in clean regions, particularly for NO. While we cannot rule out the possibility of chemical-mechanism uncertainty contributing to this deviation, the reported measurement errors for NO2 and NO are usually ±0.05 and ±0.1 ppbv, respectively. Consequently, it is likely that the measurement errors resulted in more spread in the comparisons. In particular, Shah et al. (2023) found that these measurements could be contaminated by various reactive nitrogen species in remote regions, precluding a robust validation of atmospheric models.
-
PAN. Our model reproduced 61 % of the variance observed in PAN with marginal absolute bias. According to Xu et al. (2021), the presence of oxygenated VOCs, particularly acetaldehyde, and the NO NO2 ratio are key factors controlling PAN levels. Although we constrained acetaldehyde, variations in the NO NO2 ratio in heavily polluted regions (where NOx levels exceed 1 ppbv) may have potentially led to biases in PAN simulations. Furthermore, our model's dilution factor was arbitrarily set, and it is possible that any bias caused by this factor was canceled out by other effects, leading to seemingly bias-free performance. However, Souri et al. (2023) showed that an incorrect dilution factor can significantly impact PAN performance, causing a sharp decline in R2, resulting in a value below 30 %. Therefore, the fact that our box model performed well with respect to PAN could be an indication that our choice of dilution factor was reasonable.
-
HO2 and OH. Based on our analysis of HO2 and OH simulations during the KORUS-AQ, INTEX-B, and ATom campaigns, we found a reasonable level of correspondence (R2 > 0.6) with the performance observed in previous studies conducted by Souri et al. (2020a), Brune et al. (2022), Miller and Brune (2022), and Souri et al. (2023), which focused on some of these campaigns. Although the box model OH simulations reported in Brune et al. (2020) regarding the ATom campaign seemed to be better than ours (an R2 value of ∼ 0.8 vs. an R2 value of ∼ 0.6), it is important to consider that their observations were averaged over 1 min intervals as opposed to 30 s intervals, as seen in our study. It should also be noted that there can be large errors in ATHOS (Airborne Tropospheric Hydrogen Oxides Sensor) HOx measurements, reaching up to ±40 % (Miller and Brune, 2022), so recreating the exact variance in the observations should not be the main objective. Nonetheless, the performance of our simulations in terms of HOx compared to observations suggests that the number of measured compounds and chemical mechanisms used in the model was effective. Our model's performance with respect to HOx is comparable to that of more sophisticated mechanisms that encompass a larger number of measured species (Brune et al., 2022; Miller and Brune, 2022).
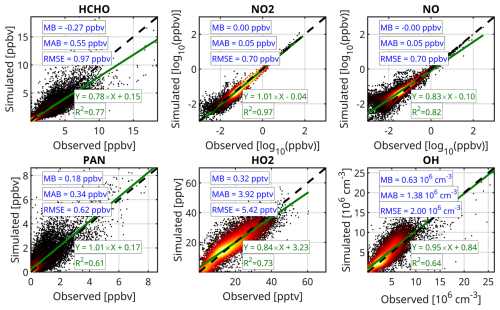
Figure 3A scatterplot comparison of simulations with observed concentrations for six unconstrained species. More than ∼ 133 000 observations were used for HCHO, NO2, NO, and PAN. HOx data points were limited to ∼ 55 000 observations. Heat maps show the density of the data. Linear fits were calculated using the ordinary least-squares method. MB: mean bias. MAB: mean absolute bias.
Overall, while there are inevitably some differences between the box model results and observations, they are consistent with what other studies have found in similar aircraft campaigns. Our extensive box model results, which consider a variety of meteorological, chemical, and photolysis rates, demonstrate satisfactory results for unconstrained compounds across a wide range of atmospheric conditions. This suggests that our training dataset, derived from the box model, is a reliable source for understanding local PO3.
It is important to note that even if a simulated data point does not match up perfectly with actual observations, it still plays a role in establishing PO3 and other explanatory variables. Hypothetically, one can generate synthetic training data points by running the box model with random numbers for the inputs; however, only a fraction of these can be truly observed in nature. Therefore, a mild outlier in our training dataset should be viewed as less likely to occur in nature (presuming that these campaigns represent all conditions occurring in nature) but still as a valuable data point derived from a physical model that can help bridge PO3 with explanatory variables.
4.2 Classification of aircraft data
Following the method described in Sect. 3.3, we cluster the cloud of aircraft data (∼ 133 000 points) into seven distinct classes. We describe these classes using three categories: pollution level, altitude, and SZA magnitude. Figure 4 illustrates violin plots of these classes for various chemical, solar, and meteorological conditions. Figure 5 presents corresponding violin plots of simulated PO3. A discussion of each class and its relationship to PO3 follows:
-
Class 1 (C1) – clean, high-altitude, high-SZA conditions. Characterized by high-altitude flights, cold ambient temperatures, and a negligible water vapor content, this class consists of observations typically taken at a relatively high SZA, with a median value of 50°. While high-altitude observations under clear-sky conditions often exhibit high photolysis rates due to reduced overhead ozone, the relatively high SZA of this class leads to low photolysis rates. FNRs tend to be large in this class due to a higher amount of HCHO relative to NO2, while FNP (HCHO × NO2) values and NO2 NOy ratios are low due to the pristine conditions. The lack of sufficient ozone precursors and reduced photochemistry make this class undergo the lowest PO3 rates, with a median of 0.11 ppbv h−1.
-
Class 2 (C2) – clean, high-altitude, low-SZA conditions. This category represents samples collected under low-SZA conditions, resulting in the highest photolysis rates among all classes. The mass of the ozone precursors and the ozone sensitivity conditions are similar to those in C1. However, C2 PO3 rates are approximately 60 % higher than those in C1 due to increased photochemistry.
-
Class 3 (C3) – moderately clean, medium-altitude, high-SZA conditions. This class is characterized by observations collected at medium altitudes and a high SZA. Airsheds in C3 experienced more polluted air compared to those in C1 and C2 due to their proximity to the surface. Photolysis rates in C3 are lower than those in C1, possibly due to higher levels of overhead ozone, although we cannot rule out the influence of varying surface albedo between the classes. Despite the lower photolysis rates, C3 PO3 levels (0.28 ppbv h−1) are higher than those of C2 and C1, indicating that pollution levels can have a more significant impact than favorable conditions for photochemistry.
-
Class 4 (C4) – moderately clean, medium-altitude, low-SZA conditions. This class differs from C3 in having a lower SZA (resulting in more photochemistry) and a slightly smaller number of ozone precursors. As a result of the lower ozone precursor concentration, C4 PO3 levels (0.19 ppbv h−1) are not only lower than those in C3 but also comparable to those in C2. This again implies that the concentration of ozone precursors is more important than photochemistry under these conditions.
-
Class 5 (C5) – extremely polluted, low-altitude, low-SZA conditions. This class features the highest concentration of ozone precursors among all classes (median FNP value of ∼ 58 ppbv2). Furthermore, it is characterized by low photolysis rates, due to its proximity to the surface, and high NO2 NOy ratios, indicative of a localized polluted airshed. Compared to the aforementioned classes, this class has the lowest FNR, indicating that it is mainly located in the VOC-sensitive regime. C5 PO3 values are much higher than those in the aforementioned classes, reaching 3.0 ppbv h−1.
-
Class 6 (C6) – polluted, low-altitude, low-SZA conditions. While this class shares similar features with C5 in terms of altitude, photolysis rates, and meteorology, it has a lower value (with a median value of 8 ppbv2). Despite the lower FNP value, C6 exhibits the highest levels of PO3 (5.2 ppbv h−1) among all classes. This is a result of reduced non-linearities as this class does not often fall into an extreme VOC-sensitive regime (with a median FNR of ∼ 1.0), where nitrogen oxides (NOx) can hamper ozone production. This tendency aligns with the findings of Souri et al. (2023), who also found that the highest amount of PO3, lying between the transitional regimes, gravitated toward the VOC-sensitive regime due to abundant ozone precursors and reduced negative chemical feedback from NOx.
-
Class 7 (C7) – moderately polluted, low-altitude, low-SZA conditions. C7 is characterized by aged air close to the surface and slightly higher photolysis rates compared to those in C5 and C6. C7 PO3 levels amount to 2.5 ppbv h−1, only slightly lower than the C5 value, despite having a much lower FNP value (with a median of 0.9 ppbv2). This could be due to the combined effect of higher photolysis rates and reduced non-linear ozone chemistry.
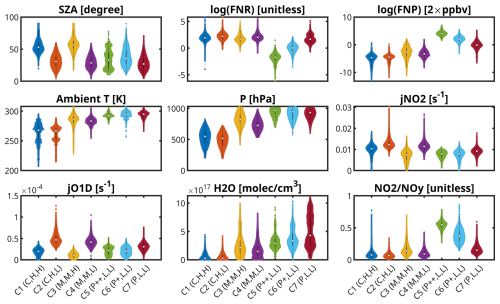
Figure 4Violin plots illustrating six different parameters derived from the box model, clustered into seven distinct categories. Each cluster is described using three types of labels: one for air pollution levels (C (clean), M (moderately clean), P (moderately polluted), P+ (polluted), and P++ (extremely polluted)), one for altitude (H (high), M (medium), and L (low)), and one for the SZA (H (high) and L (low)). Each white dot represents the median, and the bars indicate the 75th and 25th percentiles. Both the FNRs and FNPs are scaled using a logarithmic function to enable the simultaneous visualization of both low and high values within a single plot.
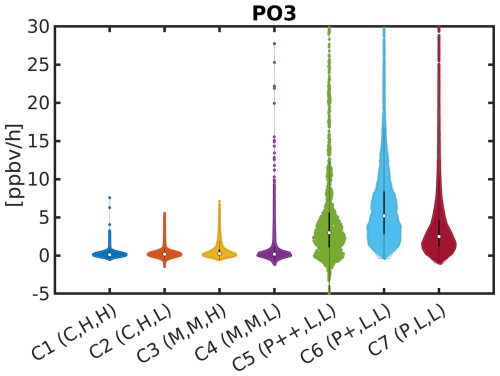
Figure 5Violin plots of simulated PO3 corresponding to the seven clusters described in Fig. 4. The lowest PO3 levels are seen in remote regions (air pollution levels: C–M), where ozone precursors are minimal. The highest PO3 levels do not occur in the most polluted (P++) regions due to the non-linear ozone chemistry.
An analysis of the aircraft data revealed that the levels of HCHO and NO2, as well as the rates of jNO2 and jO1D photolysis, play an important role in influencing PO3. Additionally, the FNR can offer insights into the sensitivity of PO3 to its main precursors. These findings align with numerous studies that have examined the factors driving PO3 (e.g., Duncan and Chameides, 1998; Thornton et al., 2002; Kleiman et al., 2002; Gerasopoulos et al., 2006; Chatfield et al., 2010; Baylon et al., 2018; Wang et al., 2020; Souri et al., 2023). Consequently, our PO3 estimates incorporate HCHO, NO2, jNO2, jO1D, and FNRs. While the cluster analysis did not definitively indicate whether meteorological conditions impact PO3, we also include ambient temperature, water vapor, pressure, and the SZA to determine whether they provide any additional insights into PO3 estimates.
4.3 Estimates of PO3
4.3.1 LASSO coefficients
Armed with a procedure that identifies important features in a linear model (Sect. 3.1), we now explore the use of LASSO for PO3 estimation. We make use of all data points generated by the observationally constrained box model across various atmospheric composition campaigns. Regarding the selected variables shown in Fig. 2, the LASSO algorithm assigns zero coefficients to the SZA, pressure, temperature, and water vapor, indicating that they offer less valuable information compared to the other variables. This decision was made by systematically adjusting the regularization factor within a 10-fold cross-validation framework to identify the optimal factor that balances solution variance and prediction bias. As a result, the LASSO algorithm suggests that HCHO, NO2, jNO2, and jO1D contain sufficient information to accurately predict PO3 for the most part.
Table 1Calibrated coefficients derived from the LASSO estimator using seven atmospheric composition aircraft campaigns.
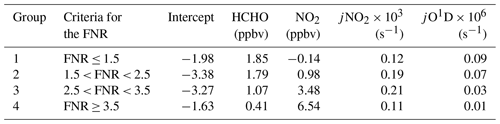
Table 1 provides the intercepts and corresponding coefficients for four different regions, classified by FNRs. While we do not expect a statistical model to fully single out the cause-and-effect relationship between explanatory variables and the target, we note that it exhibits a basic understanding of ozone chemistry; specifically, the HCHO coefficients increase as the FNR decreases (i.e., in more VOC-sensitive conditions). The same tendency is evident with respect to NO2 and higher FNRs (i.e., more NOx-sensitive conditions). The negative coefficient of NO2 in regions where FNR values ≤ 1.5 implies some level of non-linear feedback embedded in this parameterization. Both jNO2 and JO1D have positive coefficients across all chemical conditions, suggesting that higher photolysis rates accelerate PO3. JO1D has a smaller effect than jNO2 on PO3 over remote regions (with FNR values ≥ 3.5), perhaps due to redundant information compared to that concerning jNO2.
4.3.2 Validation of PO3 predictions
The validation of PO3 predictions against the box model results is performed in three progressively stringent stages: (i) using all data points included in the LASSO algorithm, (ii) randomly dropping data points, and (iii) dropping each air quality campaign from the LASSO estimation and using its data as a benchmark.
Figure 6a shows the scatterplot of predicted PO3 against the box model for all data points used to estimate the coefficients described in Sect. 4.3.1. Despite the algorithm's simplicity, we can recreate more than 88 % of the variance in PO3 with negligible absolute bias. Importantly, this suggests that our scientific problem is not overly complex. There is less than 30 % bias relative to the mean absolute bias of the prediction. The positive offset and slope smaller than 1 indicate a mild underestimation (overestimation) of PO3 in polluted (clean) regions. Figure 6b shows the same analysis for 20 000 randomly chosen data points (∼ 15 % of the total), which we purposefully dropped from the LASSO estimation to assess whether the predictor model can replicate values for points not used during the training. We find almost identical statistics for these points, suggesting that the prediction stays robust for points outside the training dataset. However, the most stringent method involves dropping each campaign dataset entirely to understand where the prediction model struggles most.
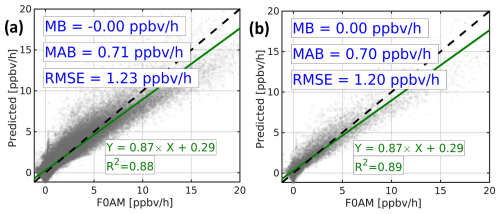
Figure 6Scatterplots comparing observationally constrained F0AM model PO3 with predictions based on proposed algorithms for either (a) all data points or (b) 20 000 data points randomly dropped as benchmarks. Despite the simplicity of the algorithm, we can reproduce a large variance in PO3 using only four explanatory variables.
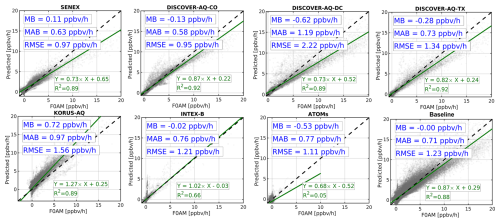
Figure 7As in Fig. 6b but with each campaign dropped from the LASSO estimation and subsequently used as an independent benchmark. The designed algorithm demonstrates a high degree of skill in predicting PO3 in polluted regions; however, it performs poorly in pristine areas.
Figure 7 shows several subplots pertaining to campaigns dropped from the analysis. It is immediately evident that our PO3 estimation demonstrates considerable skill in capturing PO3 in most polluted cases, including those from DISCOVER-AQ, KORUS-AQ, and SENEX, without using their individual datasets. This provides convincing evidence of a high degree of generalizability for the predictor. However, the model shows reduced performance with respect to INTEX-B for PO3 values < 1 ppbv h−1. Moreover, the model's predictive power is consistently poor for ATom, where a significant fraction of airsheds were sampled in pristine areas. We observe similarly poor performance for PO3 values < 1 ppbv h−1 in other campaigns, such as KORUS-AQ. Therefore, it is difficult to have confidence in the model's predictive power in remote regions, which may be due to the lack of inclusion of HOx, halogens, and H2O in the fit, as these can serve as important sinks for tropospheric ozone in those areas (Simpson et al., 2015). Nonetheless, while our predictive accuracy remains poor for this specific subset of data, the practical utility and significance of these specific regions (i.e., pristine areas) for air quality applications are notably limited. Given these results, we limit our predictions to PO3 values > 1 ppbv h−1 for the subsequent analyses.
4.3.3 TROPOMI NO2 and HCHO validation
To build confidence in our quantitative application of TROPOMI data for PO3 estimates, we validate the daily tropospheric NO2 and total HCHO columns against MAX-DOAS and FTIR observations based upon the validation framework outlined in Vigouroux et al. (2020) and Verhoelst et al. (2021). Both paired datasets were expanded to cover late 2023, providing a fuller picture of TROPOMI error characterization compared to previous studies. Figure 8 presents a comparison of daily TROPOMI data, the benchmarks, and the optimal fit associated with their errors for the period 2018–2023.
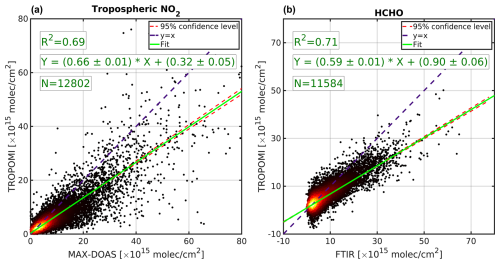
Figure 8Comparisons of TROPOMI tropospheric NO2 with MAX-DOAS observations (a) and TROPOMI HCHO with FTIR observations (b). The data points cover the period from 2018–2023. Errors in both ground-based remote sensing measurements and TROPOMI are considered in the fit. The data curation procedure is discussed in Verhoelst et al. (2021) and Vigouroux et al. (2020). A slope smaller than 1 suggests that both HCHO and NO2 retrievals are underestimated in polluted regions.
In the context of tropospheric NO2 comparison, we observe a slope smaller than 1 (∼ 0.66) with a positive offset (0.32 × 1015 molec. cm−2). This tendency has been repeatedly documented in various studies for different satellites or benchmarks (e.g., Griffin et al., 2019; Choi et al., 2020; Verhoelst et al., 2021; van Geffen et al., 2022). A slope smaller than 1, originating from unresolved systematic biases, implies that TROPOMI is biased low in polluted regions. A slightly positive offset suggests that TROPOMI NO2 is biased high in remote regions. The errors in the slope and offset are relatively small, providing evidence of the robustness of the optimal fit against the dataset variance. Nonetheless, we incorporate them into Eqs. (2) and (3) to take the adjustment error into consideration.
Despite the inherent difficulty in obtaining HCHO observations from UV–Vis imagery (Gonzalez Abad et al., 2019), the HCHO comparison exhibits good alignment with the benchmarks. As in the previous comparison, the slope is smaller than 1 (∼ 0.59), and the offset is positive (∼ 0.9 × 1015 molec. cm−2), agreeing within 10 % of studies by Vigouroux et al. (2020) and De Smedt et al. (2021). Consequently, we consider the fit errors and adjust all VCDs based on the slope and offset obtained from this comparison.
4.3.4 Maps of PO3 across various regions with qualitative descriptions
Taking advantage of the wealth of bias-corrected TROPOMI observations, we present the first-ever reported maps of PO3 at a 0.1 × 0.1° resolution within the PBL for July 2019 across various geographic regions. Moreover, due to the explicit nature of our algorithm, it is straightforward to break down the contributors of PO3 to gain insights into how each driver has shaped the distribution of PO3. Therefore, in addition to PO3 maps, we will show the magnitudes of various drivers of PO3, including NO2, HCHO, and FNR concentrations in the PBL region and the sum of scaled jO1D and jNO2 values, as well as their contributions to PO3. It is worth noting that these maps are only a snapshot of PO3 as its precursors can exhibit large interannual and interdecadal variability caused by meteorology, chemistry, and emissions. A discussion of each region follows.
Africa and the Middle East. Figure 9 illustrates accelerated rates of PO3 over this region, particularly concentrated over major cities, such as Tehran (Iran), Cairo (Egypt), Riyadh (Saudi Arabia), Baghdad (Iraq), Algiers (Algeria), and Johannesburg (South Africa). These urban areas consistently experience episodes of poor air quality (e.g., Chaichan et al., 2018; Belhout et al., 2018; Yousefian et al., 2020; Thompson et al., 2014; Boraiy et al., 2023; Choi and Souri, 2015a; Bililign et al., 2024). Biomass-burning activities in Africa (see Fig. 1 in Roberts et al., 2009) significantly contribute to the high rates of PO3. Moreover, we see accelerated PO3 rates over the Persian Gulf, a region housing oil and gas production facilities, leading to high PO3 rates in this region (Lelieveld et al., 2009; Choi and Souri, 2015a). Figure 10 shows that NO2 and HCHO concentrations are highly correlated in the Middle East (r = 0.82) due to co-emitted NOx and VOC emissions, predominantly from anthropogenic sources. Over the entire region, HCHO and NO2 concentrations are only moderately correlated (r = 0.61). This is due to the strong spatial heterogeneity of NOx and VOC emissions over Africa, which are not spatially correlated. One possible explanation for this could be the emissions' dependence on the type of fire combustion in Africa (van der Velde et al., 2021) and the location of biogenic isoprene emissions (Marais et al., 2014). For the most part, FNRs tend to fall within ranges of values above 3.5 (LASSO group 4 – highly NOx-sensitive). However, lower FNRs are prevalent in the core of cities due to elevated NOx emissions. The contributions of HCHO to PO3 occur predominantly over areas with low FNRs. These results suggest that NOx emissions dictate the locations of the maximum VOC contributions to PO3. The contribution of NO2 to PO3 behaves non-linearly, with negative values observed in the core of cities such as Johannesburg and Tehran (Fig. S2). Photolysis rates are high over areas with a low SZA and bright surface albedo (i.e., arid land). Accordingly, photolysis rates exhibit a latitudinal gradient in response to changes in the SZA. Greater contributions of photolysis rates to PO3 are observed in areas with low FNRs, as determined by the LASSO estimator (Table 1).
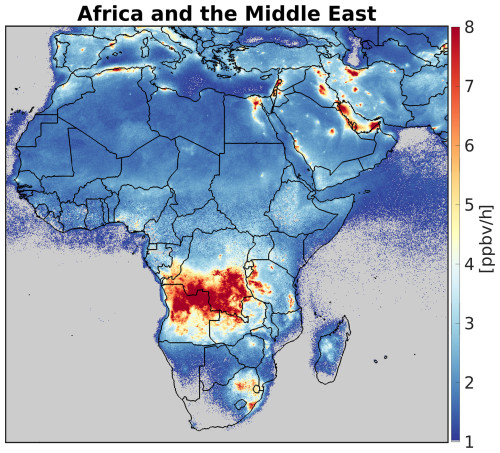
Figure 9Spatial distribution of PO3 within the PBL region, averaged over July 2019, for Africa and the Middle East. PO3 < 1 ppbv h−1 is masked due to algorithm deficiencies. Accelerated PO3 rates can be seen over major cities and regions of biomass-burning activity in Africa.
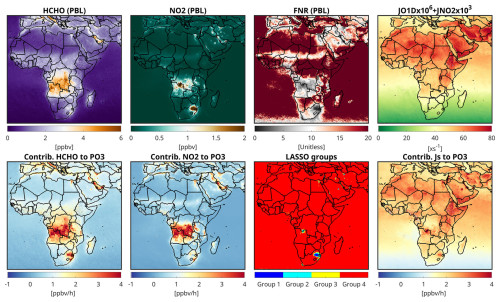
Figure 10The first row shows the PBL concentrations of HCHO, NO2, and FNRs, as well as the sum of scaled jO1D and jNO2, derived from TROPOMI and models with respect to July 2019. The second row shows the contributions of HCHO, NO2, and photolysis rates to PO3, along with the defined LASSO ozone production sensitivity regimes for PO3 estimates. Contrib: contribution. Js: photolysis rate.
Contiguous United States (CONUS). New York City, Los Angeles (LA), the San Francisco Bay Area, and Lake Michigan all experienced accelerated PO3 rates in July 2019, as shown in Fig. 11. All these regions fall into non-attainment areas (marginal to extreme) with respect to ozone standards and have been extensively studied (Wu et al., 2024; Kim et al., 2022; Stanier et al., 2021). A robust relationship between PO3 and ozone concentrations can only be established by factoring in physical processes such as horizontal and vertical transport, dry-deposition rates, and background values. In regions with high background ozone concentrations – for example, mountainous areas – even a moderate level of PO3 can elevate ozone concentrations to unhealthy levels. Conversely, if there is a strong correlation between PO3 and frequent ozone exceedances, such as those observed in the mentioned US cities, this indicates that locally produced ozone from chemical reactions is the primary factor contributing to such events. Except for LA, the vast majority of the CONUS exhibits large FNRs (> 3.5), meaning NO2 levels largely shape the spatial distribution of PO3 (Fig. 12). HCHO levels are found to be relatively large over LA, causing PO3 to increase due to its greater sensitivity to VOCs. In addition to high levels of HCHO and NO2 in several Californian regions, accelerated photochemistry caused by the bright surface albedo enhances PO3.
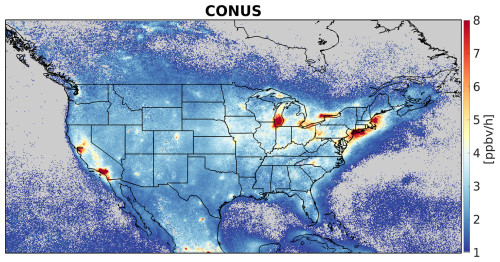
Figure 11As in Fig. 9 but for the CONUS. Elevated PO3 prevails over various areas, such as New York City, Los Angeles, the San Francisco Bay Area, and Lake Michigan.
Eastern and southeastern Asia. Figure 13 shows extremely accelerated PO3 rates over eastern Asia, particularly over the North China Plain, the Yangtze River Delta, the Pearl River Delta, and Seoul. These regions have experienced severely degraded air quality with respect to ozone (Souri et al., 2020a, b; Li et al., 2019; Colombi et al., 2023; Schroeder et al., 2020; Wang et al., 2017; Zhang et al., 2007). In southeastern Asia, Hanoi (Vietnam), Kuala Lumpur (Malaysia), and Jakarta (Indonesia) – which have also experienced heightened PO3 – have received less attention in the literature (Ahamad et al., 2020; Kusumaningtyas et al., 2024; Sakamoto et al., 2018). Figure 14 suggests that the chemical conditions of many regions in China and South Korea, falling within the transitional regimes (LASSO groups 2 and 3 – 1.5 < FNR < 3.5), have made them susceptible to high PO3 levels due to concurrently high concentrations of HCHO and NO2. Moreover, photochemistry appears to be active throughout the region.
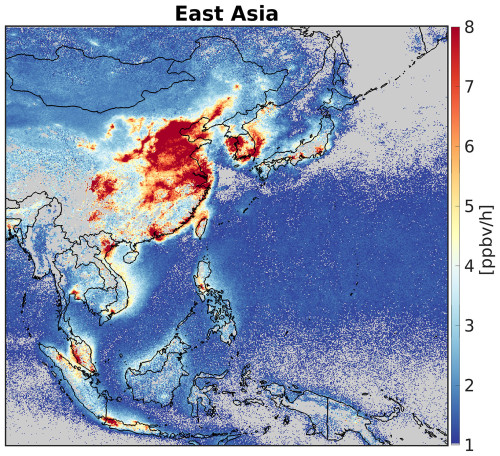
Figure 13As in Fig. 9 but for eastern and southeastern Asia. Due to heightened levels of photochemistry, NO2, and HCHO, we observe accelerated PO3 rates across the majority of the cities in eastern and southeastern Asia.
Europe. Figure 15 reveals high PO3 levels over Benelux (Belgium, the Netherlands, and Luxembourg); the Po Valley (Italy); and several major cities, such as Barcelona (Spain) and Rome (Italy). Benelux exhibits the largest hotspot of PO3 in the region (e.g., Zara et al., 2021). Benelux and a significant portion of England fall into the VOC-sensitive or transitional regimes (FNR < 2.5), as shown in Fig. 16. Due to diminished photochemistry in these high-latitude regions, we do not observe significant PBL concentrations of HCHO that would allow for PO3 levels to be as high as in the previous areas; moreover, non-linear NOx feedback has led to negative contributions of NO2 to PO3 in several cities, such as London. In general, low photolysis rates compared to the aforementioned regions have made most of Europe less prone to elevated PO3.
4.3.5 Seasonality of PO3 over the Middle East
It is valuable to study the seasonal variations in the contributors to PO3 over several major cities because the seasonality of PO3 drivers can vary from location to location. We decided to focus on several Middle Eastern regions that have experienced rapid growth and deteriorating air quality: Cairo (Egypt), Gaza (Palestine), Baghdad (Iraq), Riyadh (Saudi Arabia), Tehran (Iran), and the Persian Gulf. We illustrate the seasonality of the four major contributors to PO3 – NO2, HCHO, jNO2, and jO1D – with respect to 2019 in Fig. 17.
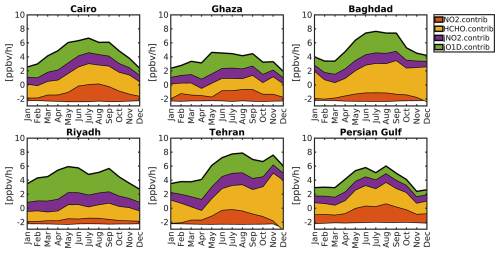
Figure 17The contributions of NO2, HCHO, jNO2, and jO1D to PBL PO3 for several major regions in the Middle East. These estimates are based on the proposed algorithm, which integrates TROPOMI, ground-based remote sensing, and atmospheric models to estimate PO3 using a statistical approach. PO3 tends to spike during summer due to increased HCHO levels, a higher sensitivity of PO3 to NOx, and enhanced photochemistry. However, Tehran shows a second peak in fall due to unusually high values of HCHO.
HCHO levels (a proxy for VOCs) consistently have the greatest impact on PO3 throughout the year in these regions. Specifically, both Baghdad and Tehran experience high levels of HCHO, even during the colder months, which can be observed using TROPOMI. This suggests that regulations targeting the reduction of human-made VOC emissions should be prioritized in these regions. PO3 levels over Cairo, Gaza, Baghdad, and the Persian Gulf peak during summertime, while Tehran experiences a comparable peak in fall due to increased VOC emissions. Additionally, we notice a decrease in PO3 levels over the Persian Gulf and Riyadh in July, possibly due to a decline in HCHO contributions caused by meteorological factors. Even though NO2 concentrations decline in the summertime due to shorter lifetimes against OH, the higher amount of HCHO makes PO3 more sensitive to NO2 during this season. Gaza exhibits the least seasonal variation among these regions, likely due to consistently active photochemistry throughout the year.
4.3.6 The effect of satellite errors on PO3
Satellite retrieval errors have been identified as the primary obstacle to achieving a robust understanding of ozone chemistry using HCHO and NO2 data (Souri et al., 2023; Johnson et al., 2023); therefore, generating uncertainty maps is crucial for informing the scientific community about the credibility of our PO3 estimates. In this study, we utilize the equations outlined in Sect. 2.2 to propagate the errors in HCHO and NO2 retrievals to the final PO3 estimates. We achieve this by recalculating the PO3 value for a given pixel 10 000 times, with each recalculation based on a sample drawn from a normal distribution with a standard deviation equal to the satellite's total error. The standard deviation of these samples offers a good approximation of the impact of satellite errors on PO3 estimates.
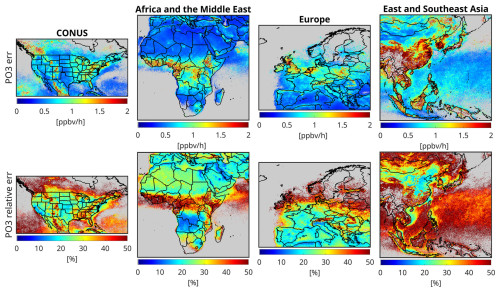
Figure 18The influence of satellite errors on PO3 estimates (both absolute and relative) over four major regions addressed in this work. The errors are based on monthly averaged TROPOMI errors. The errors tend to be mild over polluted regions (10 %–20 %), but they can exceed 50 % over pristine regions. Note that “err” stands for error.
Figure 18 illustrates the maps of absolute and relative PO3 errors over the targeted regions for the month of July. The errors in the PO3 estimates tend to be high (> 50 %) in remote regions, where the trace gas signals are small. However, the PO3 errors are within 10 %–20 % in polluted regions, where the signals are larger. Currently, the absence of absolute measurements of PO3 across this vast spatial coverage makes it challenging to assess the severity of these errors for PO3 applications. Nonetheless, any application based on this product should be recalculated within the reported errors using a Monte Carlo approach to gauge the significance of the outcome.
Providing data-driven and integrated maps of the ozone production rate (PO3) using a synergy of satellite retrievals, ground-based remote sensing, and atmospheric models enabled us to generate the first satellite-informed product of its kind, offering extensive spatial coverage and important applications for atmospheric chemistry. These data have indeed extended the use of the ratio of formaldehyde (HCHO) to nitrogen dioxide (NO2), known as the FNR, beyond its current role. Through this product, we can shed light on the effects of emission regulations, wildfires, widespread lockdowns, wars, and economic recessions on PO3 levels. Furthermore, using long-term records of satellite observations (e.g., those from OMI, starting in 2005, and TROPOMI, from 2018 onward), this product can inform emission regulators about locally produced ozone hotspots and, ultimately, enhance our understanding of the spatiotemporal variability in ozone formation over the past 2 decades.
In this study, we generated PO3 maps within the planetary boundary layer (PBL), constrained by bias-corrected TROPOspheric Monitoring Instrument (TROPOMI) observations, using a piecewise regularized regression model. This model was calibrated using a blend of data from a comprehensive suite of aircraft observations and a well-characterized box model. These maps, produced for various regions, allowed us to identify hotspots of locally produced ozone pollution with unprecedented resolution. Our findings indicate that numerous urban areas in the Middle East, eastern Asia, and southeastern Asia exhibit accelerated PO3 rates (> 8 ppbv h−1), attributed to high levels of anthropogenic nitrogen oxides (NOx = NO + NO2), volatile organic compounds (VOCs), and active photochemistry. In contrast, such elevated PO3 levels were less prevalent in the United States and Europe, with exceptions including Los Angeles, New York City, and the entire region of Benelux. Additionally, biomass-burning activities in Africa contributed to high PO3 rates across extensive areas. The seasonality of PO3 peaked around summer in several regions in the Middle East due to active photochemistry and concurrently high HCHO and NO2 levels; however, Tehran experienced elevated PO3 during fall due to large HCHO values, possibly produced from anthropogenic emissions.
The production of these maps relied heavily on a robust training dataset. To this end, we incorporated an extensive array of aircraft observations from multiple atmospheric composition campaigns, including DISCOVER-AQ, KORUS-AQ, INTEX-B, ATom, and SENEX, into the Framework for 0-D Atmospheric Modeling (F0AM) photochemical box model. This box model demonstrated a high level of correspondence (R2 > 0.6, with minimal biases) between several unconstrained compounds (e.g., HCHO, OH, HO2, PAN, NO, and NO2) and their observed counterparts, indicating its effectiveness in understanding local ozone chemistry. Utilizing a classification algorithm applied to the data obtained from the constrained box model, we identified HCHO; NO2; the ratio between HCHO and NO2 (known as the FNR); photolysis rates; and, to some extent, meteorological factors as good candidates for reproducing PO3 variability and magnitudes.
Subsequently, we employed a piecewise linear model known as LASSO, which performs feature selection by eliminating unimportant inputs, to parameterize PO3. A key component of this parameterization was the use of FNRs to empirically linearize the non-linear ozone chemistry. The LASSO algorithm indicated that more than 88 % of the variance in PO3 could be reproduced with low bias using only five parameters: the FNR, HCHO, NO2, jNO2 (the photolysis rate for NO2 + hν), and jO1D (the photolysis rate for O3 + hν). This parameterization demonstrated remarkable performance for the majority of air parcels collected in moderately to extremely polluted regions (PO3 > 1 ppbv h−1). However, it performed poorly in pristine regions due to the exclusion of certain ozone loss pathways, such as HOx (OH + HO2), which are more challenging to predict.
Fortunately, TROPOMI provides critical data for enhancing the representation of the FNR, HCHO, NO2, jNO2, and jO1D. We utilized TROPOMI's viewing geometry, UV surface albedo, and total ozone overhead from a model to predict jNO2 and jO1D, using lookup tables derived from NCAR's TUV model. To convert TROPOMI tropospheric NO2 and HCHO columns into their corresponding PBL mixing ratios, we employed the MERRA-2 GMI global transport model, which is extensively used in various studies. However, the coarse resolution of this model might have introduced underrepresentation issues, which could be mitigated in future research by using higher-spatial-resolution models.
To address the biases associated with the TROPOMI observations, we updated comparisons from Verhoelst et al. (2021) and Vigouroux et al. (2020) with a larger dataset of paired TROPOMI–FTIR and TROPOMI–MAX-DOAS measurements. TROPOMI retrievals significantly underestimated HCHO and NO2 magnitudes in polluted regions (exhibiting slope values of ∼ 0.6–0.7) and moderately overestimated them in pristine areas. These biases were corrected using regression lines, enabling a relatively unbiased application of the data.
To build confidence in our product, we propagated TROPOMI HCHO and NO2 errors to PO3 estimates using a Monte Carlo approach. The results indicated that PO3 estimates were uncertain (> 50 %) in clean regions due to a low trace gas signal in TROPOMI retrievals. However, in polluted regions, the errors were more moderate (10 %–20 %) due to the stronger signal.
Over the years, extensive efforts have been devoted to measuring various critical atmospheric compounds globally, developing robust atmospheric models, and enhancing satellite retrievals and their benchmarks. These advancements have enabled us to estimate PO3 maps within the PBL. Nonetheless, it is crucial to acknowledge some limitations of our work, many of which are the focus of ongoing research within our team:
- i.
Direct measurements of PO3 using specialized instruments (Cazorla and Brune, 2010; Sadanaga et al., 2017; Sklaveniti et al., 2018) are lacking in most atmospheric composition datasets, limiting our ability to fully understand the effects of assumptions made in the box model (such as the exclusion of heterogeneous chemistry) on PO3.
- ii.
There is potential for improvement in the parameterization process by employing more sophisticated algorithms, such as neural networks, which could increase the explained variance in the predicted PO3.
- iii.
The conversion of satellite column data to PBL mixing ratios requires error characterization and the use of finer-resolution models that are comparable in size to the PO3 grid boxes.
- iv.
Partially cloudy pixels and aerosols can affect photolysis rates, which should be considered in future parameterization efforts.
It is important to recognize that PO3 maps are just one piece of the puzzle when it comes to determining ozone concentrations. Several studies have indicated that accurately representing surface ozone is challenging due to difficulties in representing background ozone, transport, and dry-deposition rates (e.g., Zhang et al., 2023; Clifton et al., 2020). Therefore, we advise against directly linking high PO3 rates from our product to increased unhealthy ozone exposure. However, our product does provide indications as to whether heightened ozone concentrations are associated with chemistry contributions as opposed to other processes (e.g., meteorology or dry-deposition rates). Further investigation using additional tools/data is necessary to gather a full picture of these processes.
Despite these limitations, our novel product is a valuable asset to the atmospheric science community. It provides a more comprehensive understanding of the complexities associated with spatiotemporal variability in non-linear ozone chemistry over a large domain and enhances confidence in high-resolution maps of chemically produced ozone hotspots.
TROPOMI satellite data are derived from https://doi.org/10.5270/S5P-9bnp8q8 (Copernicus Sentinel-5P, 2021) and https://doi.org/10.5270/S5P-vg1i7t0 (Copernicus Sentinel-5P, 2020). The FTIR and MAX-DOAS observations were partly obtained from the Network for the Detection of Atmospheric Composition Change (NDACC) and are available through the NDACC website at https://ndacc.larc.nasa.gov (NDACC, 2025). The box model can be obtained from https://github.com/AirChem/F0AM (last access: 10 November 2024; https://doi.org/10.5281/zenodo.10069985, Wolfe and Haskins, 2023). The TROPOMI UV DLER database can be obtained from https://www.temis.nl/surface/albedo/tropomi_ler.php (Royal Netherlands Meteorological Institute, 2025).
The supplement related to this article is available online at https://doi.org/10.5194/acp-25-2061-2025-supplement.
AHS designed and implemented the research idea, analyzed the data, made all figures, and wrote the paper. TV, CV, GP, SC, and BL provided the paired TROPOMI and benchmark data. Other authors helped with the analysis, model setup, and interpretation.
At least one of the (co-)authors is a member of the editorial board of Atmospheric Chemistry and Physics. The peer-review process was guided by an independent editor, and the authors also have no other competing interests to declare.
Publisher's note: Copernicus Publications remains neutral with regard to jurisdictional claims made in the text, published maps, institutional affiliations, or any other geographical representation in this paper. While Copernicus Publications makes every effort to include appropriate place names, the final responsibility lies with the authors.
This article is part of the special issue “Tropospheric Ozone Assessment Report Phase II (TOAR-II) Community Special Issue (ACP/AMT/BG/GMD inter-journal SI)”. It is not associated with a conference.
We thank all of the principal investigators, pilots, and managers who collected the aircraft data used in our research and made them publicly available. We thank the FTIR HCHO measurement team, comprising Thomas Blumenstock, Martine De Mazière, Michel Grutter, James W. Hannigan, Nicholas Jones, Rigel Kivi, Erik Lutsch, Emmanuel Mahieu, Maria Makarova, Isamu Morino, Isao Murata, Tomoo Nagahama, Justus Notholt, Ivan Ortega, Mathias Palm, Amelie Röhling, Matthias Schneider, Dan Smale, Wolfgang Stremme, Kim String, Youwen Sun, Ralf Sussmann, Yao Té, and Pucai Wang. We thank the Meteorological Service Suriname and Cornelis Becker for their support. The MAX-DOAS data used in this publication were obtained from Alkis Bais, John Burrows, Ka Lok Chan, Michel Grutter, Cheng Liu, Hitoshi Irie, Vinod Kumar, Yugo Kanaya, Ankie Piters, Claudia Rivera-Cárdenas, Andreas Richter, Michel van Roozendael, Robert Ryan, Vinayak Sinha, and Thomas Wagner. Fast delivery of MAX-DOAS data tailored to the S5P validation was organized through the S5PVT AO project NIDFORVAL. We thank the IISER Mohali atmospheric chemistry facility for supporting the MAX-DOAS measurements obtained in Mohali, India. We also thank Julie M. Nicely for providing the merged ATom observations.
The measurements recorded in Paramaribo were supported by the BMBF (German Federal Ministry of Education and Research) as part of the TroStra subproject within the ROMIC II project (grant no. 01LG1904A). The NDACC FTIR stations in Bremen, Garmisch-Partenkirchen, Izaña, Ny-Ålesund, Paramaribo, and Karlsruhe are supported by the Bundesministerium für Wirtschaft und Klimaschutz (BMWK) via DLR under grants nos. 50EE1711A, 50EE1711B, and 50EE1711D. The measurements and data analysis for Bremen are supported by the Senate of Bremen. The NCAR FTS observation programs in Thule (Greenland), Boulder (Colorado, USA), and Mauna Loa (Hawaii) are supported through a contract with the National Aeronautics and Space Administration (NASA). The National Center for Atmospheric Research is sponsored by the National Science Foundation (NSF). The Thule effort is also supported by the NSF's Office of Polar Programs (OPP). Operations at the Rikubetsu and Tsukuba FTIR sites are supported in part by the GOSAT series project. The Paris TCCON site has received funding from Sorbonne Université, the French research center CNRS, and the French space agency CNES. The Jungfraujoch FTIR data are primarily available thanks to support provided by the F.R.S.–FNRS (Brussels), the GAW-CH program of MeteoSwiss (Zürich), and the HFSJG Foundation (Bern). Ground-based measurements from IUP Bremen are funded by DLR Bonn and provided through grant no. 50EE1709A. KNMI ground-based measurements for De Bilt and Cabauw are partly supported by the Ruisdael Observatory project of the Dutch Research Council (NWO; grant no. 184.034.015), by the Netherlands Space Office (NSO) for Sentinel-5P/TROPOMI validation, and by the ESA via the EU CAMS project.
This research has been supported by the National Aeronautics and Space Administration (grant no. 80NSSC23K1250). Funders facilitating the collection of data used in this article (rather than funders of this work specifically) are included in the Acknowledgements.
This paper was edited by Anne Perring and reviewed by two anonymous referees.
Ahamad, F., Griffiths, P. T., Latif, M. T., Juneng, L., and Xiang, C. J.: Ozone Trends from Two Decades of Ground Level Observation in Malaysia, Atmosphere, 11, 755, https://doi.org/10.3390/atmos11070755, 2020.
Anderson, D. C., Follette-Cook, M. B., Strode, S. A., Nicely, J. M., Liu, J., Ivatt, P. D., and Duncan, B. N.: A machine learning methodology for the generation of a parameterization of the hydroxyl radical, Geosci. Model Dev., 15, 6341–6358, https://doi.org/10.5194/gmd-15-6341-2022, 2022.
Archibald, A. T., Jenkin, M. E., and Shallcross, D. E.: An isoprene mechanism intercomparison, Atmos. Environ., 44, 5356–5364, https://doi.org/10.1016/j.atmosenv.2009.09.016, 2010.
Baylon, P., Jaffe, D. A., Hall, S. R., Ullmann, K., Alvarado, M. J., and Lefer, B. L.: Impact of Biomass Burning Plumes on Photolysis Rates and Ozone Formation at the Mount Bachelor Observatory, J. Geophys. Res.-Atmos., 123, 2272–2284, https://doi.org/10.1002/2017JD027341, 2018.
Beddows, D. C. S., Dall'Osto, M., and Harrison, R. M.: Cluster Analysis of Rural, Urban, and Curbside Atmospheric Particle Size Data, Environ. Sci. Technol., 43, 4694–4700, https://doi.org/10.1021/es803121t, 2009.
Belhout, D., Kerbachi, R., Relvas, H., and Miranda, A. I.: Air quality assessment in Algiers city, Air. Qual. Atmos. Health., 11, 897–906, https://doi.org/10.1007/s11869-018-0589-x, 2018.
Bililign, S., Brown, S. S., Westervelt, D. M., Kumar, R., Tang, W., Flocke, F., Vizuete, W., Ture, K., Pope, F. D., Demoz, B., Asa-Awuku, A., Levelt, P. F., Kalisa, E., Raheja, G., Ndyabakira, A., and Gatari, M. J.: East African Megacity Air Quality: Rationale and Framework for a Measurement and Modeling Program, B. Am. Meteorol. Soc., 105, E1584–E1602, https://doi.org/10.1175/BAMS-D-23-0098.1, 2024.
Boraiy, M., El-Metwally, M., Wheida, A., El-Nazer, M., Hassan, S. K., El-Sanabary, F. F., Alfaro, S. C., Abdelwahab, M., and Borbon, A.: Statistical analysis of the variability of reactive trace gases (SO2, NO2 and ozone) in Greater Cairo during dust storm events, J. Atmos. Chem., 80, 227–250, https://doi.org/10.1007/s10874-023-09449-4, 2023.
Bottorff, B., Lew, M. M., Woo, Y., Rickly, P., Rollings, M. D., Deming, B., Anderson, D. C., Wood, E., Alwe, H. D., Millet, D. B., Weinheimer, A., Tyndall, G., Ortega, J., Dusanter, S., Leonardis, T., Flynn, J., Erickson, M., Alvarez, S., Rivera-Rios, J. C., Shutter, J. D., Keutsch, F., Helmig, D., Wang, W., Allen, H. M., Slade, J. H., Shepson, P. B., Bertman, S., and Stevens, P. S.: OH, HO2, and RO2 radical chemistry in a rural forest environment: measurements, model comparisons, and evidence of a missing radical sink, Atmos. Chem. Phys., 23, 10287–10311, https://doi.org/10.5194/acp-23-10287-2023, 2023.
Brune, W. H., Miller, D. O., Thames, A. B., Allen, H. M., Apel, E. C., Blake, D. R., Bui, T. P., Commane, R., Crounse, J. D., Daube, B. C., Diskin, G. S., DiGangi, J. P., Elkins, J. W., Hall, S. R., Hanisco, T. F., Hannun, R. A., Hintsa, E. J., Hornbrook, R. S., Kim, M. J., McKain, K., Moore, F. L., Neuman, J. A., Nicely, J. M., Peischl, J., Ryerson, T. B., St. Clair, J. M., Sweeney, C., Teng, A. P., Thompson, C., Ullmann, K., Veres, P. R., Wennberg, P. O., and Wolfe, G. M.: Exploring Oxidation in the Remote Free Troposphere: Insights From Atmospheric Tomography (ATom), J. Geophys. Res.-Atmos., 125, e2019JD031685, https://doi.org/10.1029/2019JD031685, 2020.
Brune, W. H., Miller, D. O., Thames, A. B., Brosius, A. L., Barletta, B., Blake, D. R., Blake, N. J., Chen, G., Choi, Y., Crawford, J. H., Digangi, J. P., Diskin, G., Fried, A., Hall, S. R., Hanisco, T. F., Huey, G. L., Hughes, S. C., Kim, M., Meinardi, S., Montzka, D. D., Pusede, S. E., Schroeder, J. R., Teng, A., Tanner, D. J., Ullmann, K., Walega, J., Weinheimer, A., Wisthaler, A., and Wennberg, P. O.: Observations of atmospheric oxidation and ozone production in South Korea, Atmos. Environ., 269, 118854, https://doi.org/10.1016/j.atmosenv.2021.118854, 2022.
Cazorla, M. and Brune, W. H.: Measurement of Ozone Production Sensor, Atmos. Meas. Tech., 3, 545–555, https://doi.org/10.5194/amt-3-545-2010, 2010.
Cazorla, M., Brune, W. H., Ren, X., and Lefer, B.: Direct measurement of ozone production rates in Houston in 2009 and comparison with two estimation methods, Atmos. Chem. Phys., 12, 1203–1212, https://doi.org/10.5194/acp-12-1203-2012, 2012.
Clifton, O. E., Fiore, A. M., Massman, W. J., Baublitz, C. B., Coyle, M., Emberson, L., Fares, S., Farmer, D. K., Gentine, P., Gerosa, G., Guenther, A. B., Helmig, D., Lombardozzi, D. L., Munger, J. W., Patton, E. G., Pusede, S. E., Schwede, D. B., Silva, S. J., Sörgel, M., Steiner, A. L., and Tai, A. P. K.: Dry Deposition of Ozone Over Land: Processes, Measurement, and Modeling, Rev. Geophys., 58, e2019RG000670, https://doi.org/10.1029/2019RG000670, 2020.
Chaichan, M. T., Kazem, H. A., and Abed, T. A.: Traffic and outdoor air pollution levels near highways in Baghdad, Iraq, Environ. Dev. Sustain., 20, 589–603, https://doi.org/10.1007/s10668-016-9900-x, 2018.
Chatfield, R. B., Ren, X., Brune, W., and Schwab, J.: Controls on urban ozone production rate as indicated by formaldehyde oxidation rate and nitric oxide, Atmos. Environ., 44, 5395–5406, https://doi.org/10.1016/j.atmosenv.2010.08.056, 2010.
Choi, J., Henze, D. K., Cao, H., Nowlan, C. R., González Abad, G., Kwon, H.-A., Lee, H.-M., Oak, Y. J., Park, R. J., Bates, K. H., Maasakkers, J. D., Wisthaler, A., and Weinheimer, A. J.: An Inversion Framework for Optimizing Non-Methane VOC Emissions Using Remote Sensing and Airborne Observations in Northeast Asia During the KORUS-AQ Field Campaign, J. Geophys. Res.-Atmos., 127, e2021JD035844, https://doi.org/10.1029/2021JD035844, 2022.
Choi, S., Lamsal, L. N., Follette-Cook, M., Joiner, J., Krotkov, N. A., Swartz, W. H., Pickering, K. E., Loughner, C. P., Appel, W., Pfister, G., Saide, P. E., Cohen, R. C., Weinheimer, A. J., and Herman, J. R.: Assessment of NO2 observations during DISCOVER-AQ and KORUS-AQ field campaigns, Atmos. Meas. Tech., 13, 2523–2546, https://doi.org/10.5194/amt-13-2523-2020, 2020.
Choi, Y. and Souri, A. H.: Chemical condition and surface ozone in large cities of Texas during the last decade: Observational evidence from OMI, CAMS, and model analysis, Remote Sens. Environ., 168, 90–101, https://doi.org/10.1016/j.rse.2015.06.026, 2015a.
Choi, Y. and Souri, A. H.: Seasonal behavior and long-term trends of tropospheric ozone, its precursors and chemical conditions over Iran: A view from space, Atmos. Environ., 106, 232–240, https://doi.org/10.1016/j.atmosenv.2015.02.012, 2015b.
Choi, Y., Kim, H., Tong, D., and Lee, P.: Summertime weekly cycles of observed and modeled NOx and O3 concentrations as a function of satellite-derived ozone production sensitivity and land use types over the Continental United States, Atmos. Chem. Phys., 12, 6291–6307, https://doi.org/10.5194/acp-12-6291-2012, 2012.
Colombi, N. K., Jacob, D. J., Yang, L. H., Zhai, S., Shah, V., Grange, S. K., Yantosca, R. M., Kim, S., and Liao, H.: Why is ozone in South Korea and the Seoul metropolitan area so high and increasing?, Atmos. Chem. Phys., 23, 4031–4044, https://doi.org/10.5194/acp-23-4031-2023, 2023.
Copernicus Sentinel-5P: TROPOMI Level 2 Formaldehyde Total Column products, Version 02, European Space Agency, Copernicus Sentinel-5P (processed by ESA) [data set], https://doi.org/10.5270/S5P-vg1i7t0, 2020.
Copernicus Sentinel-5P: TROPOMI Level 2 Nitrogen Dioxide total column products, Version 02, European Space Agency, Copernicus Sentinel-5P (processed by ESA) [data set], https://doi.org/10.5270/S5P-9bnp8q8, 2021.
Crawford, J. H., Ahn, J.-Y., Al-Saadi, J., Chang, L., Emmons, L. K., Kim, J., Lee, G., Park, J.-H., Park, R. J., Woo, J. H., Song, C.-K., Hong, J.-H., Hong, Y.-D., Lefer, B. L., Lee, M., Lee, T., Kim, S., Min, K.-E., Yum, S. S., Shin, H. J., Kim, Y.-W., Choi, J.-S., Park, J.-S., Szykman, J. J., Long, R. W., Jordan, C. E., Simpson, I. J., Fried, A., Dibb, J. E., Cho, S., and Kim, Y. P.: The Korea–United States Air Quality (KORUS-AQ) field study, Elem. Sci. Anth., 9, 00163, https://doi.org/10.1525/elementa.2020.00163, 2021.
De Smedt, I., Pinardi, G., Vigouroux, C., Compernolle, S., Bais, A., Benavent, N., Boersma, F., Chan, K.-L., Donner, S., Eichmann, K.-U., Hedelt, P., Hendrick, F., Irie, H., Kumar, V., Lambert, J.-C., Langerock, B., Lerot, C., Liu, C., Loyola, D., Piters, A., Richter, A., Rivera Cárdenas, C., Romahn, F., Ryan, R. G., Sinha, V., Theys, N., Vlietinck, J., Wagner, T., Wang, T., Yu, H., and Van Roozendael, M.: Comparative assessment of TROPOMI and OMI formaldehyde observations and validation against MAX-DOAS network column measurements, Atmos. Chem. Phys., 21, 12561–12593, https://doi.org/10.5194/acp-21-12561-2021, 2021.
DiMaria, C. A., Jones, D. B. A., Worden, H., Bloom, A. A., Bowman, K., Stavrakou, T., Miyazaki, K., Worden, J., Guenther, A., Sarkar, C., Seco, R., Park, J.-H., Tota, J., Alves, E. G., and Ferracci, V.: Optimizing the Isoprene Emission Model MEGAN With Satellite and Ground-Based Observational Constraints, J. Geophys. Res.-Atmos., 128, e2022JD037822, https://doi.org/10.1029/2022JD037822, 2023.
Duncan, B. N. and Chameides, W. L.: Effects of urban emission control strategies on the export of ozone and ozone precursors from the urban atmosphere to the troposphere, J. Geophys. Res.-Atmos., 103, 28159–28179, https://doi.org/10.1029/98JD02145, 1998.
Duncan, B. N., Strahan, S. E., Yoshida, Y., Steenrod, S. D., and Livesey, N.: Model study of the cross-tropopause transport of biomass burning pollution, Atmos. Chem. Phys., 7, 3713–3736, https://doi.org/10.5194/acp-7-3713-2007, 2007.
Duncan, B. N., Yoshida, Y., Olson, J. R., Sillman, S., Martin, R. V., Lamsal, L., Hu, Y., Pickering, K. E., Retscher, C., Allen, D. J., and Crawford, J. H.: Application of OMI observations to a space-based indicator of NOx and VOC controls on surface ozone formation, Atmos. Environ., 44, 2213–2223, https://doi.org/10.1016/j.atmosenv.2010.03.010, 2010.
Fleming, Z. L., Doherty, R. M., von Schneidemesser, E., Malley, C. S., Cooper, O. R., Pinto, J. P., Colette, A., Xu, X., Simpson, D., Schultz, M. G., Lefohn, A. S., Hamad, S., Moolla, R., Solberg, S., and Feng, Z.: Tropospheric Ozone Assessment Report: Present-day ozone distribution and trends relevant to human health, Elem. Sci. Anth., 6, 12, https://doi.org/10.1525/elementa.273, 2018.
Gaudel, A., Cooper, O. R., Ancellet, G., Barret, B., Boynard, A., Burrows, J. P., Clerbaux, C., Coheur, P.-F., Cuesta, J., Cuevas, E., Doniki, S., Dufour, G., Ebojie, F., Foret, G., Garcia, O., Granados-Muñoz, M. J., Hannigan, J. W., Hase, F., Hassler, B., Huang, G., Hurtmans, D., Jaffe, D., Jones, N., Kalabokas, P., Kerridge, B., Kulawik, S., Latter, B., Leblanc, T., Le Flochmoën, E., Lin, W., Liu, J., Liu, X., Mahieu, E., McClure-Begley, A., Neu, J. L., Osman, M., Palm, M., Petetin, H., Petropavlovskikh, I., Querel, R., Rahpoe, N., Rozanov, A., Schultz, M. G., Schwab, J., Siddans, R., Smale, D., Steinbacher, M., Tanimoto, H., Tarasick, D. W., Thouret, V., Thompson, A. M., Trickl, T., Weatherhead, E., Wespes, C., Worden, H. M., Vigouroux, C., Xu, X., Zeng, G., and Ziemke, J.: Tropospheric Ozone Assessment Report: Present-day distribution and trends of tropospheric ozone relevant to climate and global atmospheric chemistry model evaluation, Elem. Sci. Anth., 6, 39, https://doi.org/10.1525/elementa.291, 2018.
Gerasopoulos, E., Kouvarakis, G., Vrekoussis, M., Donoussis, C., Mihalopoulos, N., and Kanakidou, M.: Photochemical ozone production in the Eastern Mediterranean, Atmos. Environ., 40, 3057–3069, https://doi.org/10.1016/j.atmosenv.2005.12.061, 2006.
Gonzalez Abad, G., Souri, A. H., Bak, J., Chance, K., Flynn, L. E., Krotkov, N. A., Lamsal, L., Li, C., Liu, X., Miller, C. C., Nowlan, C. R., Suleiman, R., and Wang, H.: Five decades observing Earth's atmospheric trace gases using ultraviolet and visible backscatter solar radiation from space, J. Quant. Spectrosc. Ra., 238, 106478, https://doi.org/10.1016/j.jqsrt.2019.04.030, 2019.
Govender, P. and Sivakumar, V.: Application of k-means and hierarchical clustering techniques for analysis of air pollution: A review (1980–2019), Atmos. Pollut. Res., 11, 40–56, https://doi.org/10.1016/j.apr.2019.09.009, 2020.
Griffin, D., Zhao, X., McLinden, C. A., Boersma, F., Bourassa, A., Dammers, E., Degenstein, D., Eskes, H., Fehr, L., Fioletov, V., Hayden, K., Kharol, S. K., Li, S.-M., Makar, P., Martin, R. V., Mihele, C., Mittermeier, R. L., Krotkov, N., Sneep, M., Lamsal, L. N., ter Linden, M., van Geffen, J., Veefkind, P., and Wolde, M.: High-Resolution Mapping of Nitrogen Dioxide With TROPOMI: First Results and Validation Over the Canadian Oil Sands, Geophys. Res. Lett., 46, 1049–1060, https://doi.org/10.1029/2018GL081095, 2019.
Jeon, W., Choi, Y., Souri, A. H., Roy, A., Diao, L., Pan, S., Lee, H. W., and Lee, S.-H.: Identification of chemical fingerprints in long-range transport of burning induced upper tropospheric ozone from Colorado to the North Atlantic Ocean, Sci. Total Environ., 613–614, 820–828, https://doi.org/10.1016/j.scitotenv.2017.09.177, 2018.
Jin, X. and Holloway, T.: Spatial and temporal variability of ozone sensitivity over China observed from the Ozone Monitoring Instrument, J. Geophys. Res.-Atmos., 120, 7229–7246, https://doi.org/10.1002/2015JD023250, 2015.
Jin, X., Fiore, A. M., Murray, L. T., Valin, L. C., Lamsal, L. N., Duncan, B., Folkert Boersma, K., De Smedt, I., Abad, G. G., Chance, K., and Tonnesen, G. S.: Evaluating a Space-Based Indicator of Surface Ozone-NOx-VOC Sensitivity Over Midlatitude Source Regions and Application to Decadal Trends, J. Geophys. Res.-Atmos., 122, 10439–10461, https://doi.org/10.1002/2017JD026720, 2017.
Johnson, M. S., Souri, A. H., Philip, S., Kumar, R., Naeger, A., Geddes, J., Judd, L., Janz, S., Chong, H., and Sullivan, J.: Satellite remote-sensing capability to assess tropospheric-column ratios of formaldehyde and nitrogen dioxide: case study during the Long Island Sound Tropospheric Ozone Study 2018 (LISTOS 2018) field campaign, Atmos. Meas. Tech., 16, 2431–2454, https://doi.org/10.5194/amt-16-2431-2023, 2023.
Kim, S.-W., McDonald, B. C., Seo, S., Kim, K.-M., and Trainer, M.: Understanding the Paths of Surface Ozone Abatement in the Los Angeles Basin, J. Geophys. Res.-Atmos., 127, e2021JD035606, https://doi.org/10.1029/2021JD035606, 2022.
Kleinman, L. I., Daum, P. H., Imre, D., Lee, Y.-N., Nunnermacker, L. J., Springston, S. R., Weinstein-Lloyd, J., and Rudolph, J.: Ozone production rate and hydrocarbon reactivity in 5 urban areas: A cause of high ozone concentration in Houston, Geophys. Res. Lett., 29, 105-1–105-4, https://doi.org/10.1029/2001GL014569, 2002.
Kusumaningtyas, S. D. A., Tonokura, K., Muharsyah, R., Gunawan, D., Sopaheluwakan, A., Iriana, W., Lestari, P., Permadi, D. A., Rahmawati, R., and Samputra, N. A. R.: Comprehensive analysis of long-term trends, meteorological influences, and ozone formation sensitivity in the Jakarta Greater Area, Sci. Rep., 14, 9605, https://doi.org/10.1038/s41598-024-60374-2, 2024.
Lelieveld, J., Hoor, P., Jöckel, P., Pozzer, A., Hadjinicolaou, P., Cammas, J.-P., and Beirle, S.: Severe ozone air pollution in the Persian Gulf region, Atmos. Chem. Phys., 9, 1393–1406, https://doi.org/10.5194/acp-9-1393-2009, 2009.
Li, K., Jacob, D. J., Liao, H., Shen, L., Zhang, Q., and Bates, K. H.: Anthropogenic drivers of 2013–2017 trends in summer surface ozone in China, P. Natl. Acad. Sci. USA, 116, 422–427, https://doi.org/10.1073/pnas.1812168116, 2019.
Marais, E. A., Jacob, D. J., Guenther, A., Chance, K., Kurosu, T. P., Murphy, J. G., Reeves, C. E., and Pye, H. O. T.: Improved model of isoprene emissions in Africa using Ozone Monitoring Instrument (OMI) satellite observations of formaldehyde: implications for oxidants and particulate matter, Atmos. Chem. Phys., 14, 7693–7703, https://doi.org/10.5194/acp-14-7693-2014, 2014.
Martin, R. V., Fiore, A. M., and Van Donkelaar, A.: Space-based diagnosis of surface ozone sensitivity to anthropogenic emissions, Geophys. Res. Lett., 31, L06120, https://doi.org/10.1029/2004GL019416, 2004.
Marvin, M. R., Wolfe, G. M., Salawitch, R. J., Canty, T. P., Roberts, S. J., Travis, K. R., Aikin, K. C., de Gouw, J. A., Graus, M., Hanisco, T. F., Holloway, J. S., Hübler, G., Kaiser, J., Keutsch, F. N., Peischl, J., Pollack, I. B., Roberts, J. M., Ryerson, T. B., Veres, P. R., and Warneke, C.: Impact of evolving isoprene mechanisms on simulated formaldehyde: An inter-comparison supported by in situ observations from SENEX, Atmos. Environ., 164, 325–336, https://doi.org/10.1016/j.atmosenv.2017.05.049, 2017.
Mazzuca, G. M., Ren, X., Loughner, C. P., Estes, M., Crawford, J. H., Pickering, K. E., Weinheimer, A. J., and Dickerson, R. R.: Ozone production and its sensitivity to NOx and VOCs: results from the DISCOVER-AQ field experiment, Houston 2013, Atmos. Chem. Phys., 16, 14463–14474, https://doi.org/10.5194/acp-16-14463-2016, 2016.
Miller, D. O. and Brune, W. H.: Investigating the Understanding of Oxidation Chemistry Using 20 Years of Airborne OH and HO2 Observations, J. Geophys. Res.-Atmos., 127, e2021JD035368, https://doi.org/10.1029/2021JD035368, 2022.
Mills, G., Pleijel, H., Malley, C. S., Sinha, B., Cooper, O. R., Schultz, M. G., Neufeld, H. S., Simpson, D., Sharps, K., Feng, Z., Gerosa, G., Harmens, H., Kobayashi, K., Saxena, P., Paoletti, E., Sinha, V., and Xu, X.: Tropospheric Ozone Assessment Report: Present-day tropospheric ozone distribution and trends relevant to vegetation, Elem. Sci. Anth., 6, 47, https://doi.org/10.1525/elementa.302, 2018.
Miyazaki, K., Eskes, H., Sudo, K., Boersma, K. F., Bowman, K., and Kanaya, Y.: Decadal changes in global surface NOx emissions from multi-constituent satellite data assimilation, Atmos. Chem. Phys., 17, 807–837, https://doi.org/10.5194/acp-17-807-2017, 2017.
Network for the Detection of Atmospheric Composition Change (NDACC): Measurement Stations, Network for the Detection of Atmospheric Composition Change (NDACC) [data set], https://ndacc.larc.nasa.gov, last access: 2 February 2025.
Orbe, C., Oman, L. D., Strahan, S. E., Waugh, D. W., Pawson, S., Takacs, L. L., and Molod, A. M.: Large-Scale Atmospheric Transport in GEOS Replay Simulations, J. Adv. Model. Earth Syst., 9, 2545–2560, https://doi.org/10.1002/2017MS001053, 2017.
Pan, S., Roy, A., Choi, Y., Eslami, E., Thomas, S., Jiang, X., and Gao, H. O.: Potential impacts of electric vehicles on air quality and health endpoints in the Greater Houston Area in 2040, Atmos. Environ., 207, 38–51, https://doi.org/10.1016/j.atmosenv.2019.03.022, 2019.
Ren, X., van Duin, D., Cazorla, M., Chen, S., Mao, J., Zhang, L., Brune, W. H., Flynn, J. H., Grossberg, N., Lefer, B. L., Rappenglück, B., Wong, K. W., Tsai, C., Stutz, J., Dibb, J. E., Thomas Jobson, B., Luke, W. T., and Kelley, P.: Atmospheric oxidation chemistry and ozone production: Results from SHARP 2009 in Houston, Texas, J. Geophys. Res.-Atmos., 118, 5770–5780, https://doi.org/10.1002/jgrd.50342, 2013.
Roberts, G., Wooster, M. J., and Lagoudakis, E.: Annual and diurnal african biomass burning temporal dynamics, Biogeosciences, 6, 849–866, https://doi.org/10.5194/bg-6-849-2009, 2009.
Royal Netherlands Meteorological Institute (KNMI): TROPOMI surface LER & DLER database, Royal Netherlands Meteorological Institute (KNMI) [data set], https://www.temis.nl/surface/albedo/tropomi_ler.php, last access: 2 February 2025.
Sadanaga, Y., Kawasaki, S., Tanaka, Y., Kajii, Y., and Bandow, H.: New System for Measuring the Photochemical Ozone Production Rate in the Atmosphere, Environ. Sci. Technol., 51, 2871–2878, https://doi.org/10.1021/acs.est.6b04639, 2017.
Sakamoto, Y., Shoji, K., Bui, M. T., Ph?m, T. H., Vu, T. A., Ly, B. T., and Kajii, Y.: Air quality study in Hanoi, Vietnam in 2015–2016 based on a one-year observation of NOx, O3, CO and a one-week observation of VOCs, Atmos. Pollut. Res., 9, 544–551, https://doi.org/10.1016/j.apr.2017.12.001, 2018.
Schroeder, J. R., Crawford, J. H., Fried, A., Walega, J., Weinheimer, A., Wisthaler, A., Müller, M., Mikoviny, T., Chen, G., Shook, M., Blake, D. R., and Tonnesen, G. S.: New insights into the column CH2O NO2 ratio as an indicator of near-surface ozone sensitivity, J. Geophys. Res.-Atmos., 122, 8885–8907, https://doi.org/10.1002/2017JD026781, 2017.
Schroeder, J. R., Crawford, J. H., Ahn, J.-Y., Chang, L., Fried, A., Walega, J., Weinheimer, A., Montzka, D. D., Hall, S. R., Ullmann, K., Wisthaler, A., Mikoviny, T., Chen, G., Blake, D. R., Blake, N. J., Hughes, S. C., Meinardi, S., Diskin, G., Digangi, J. P., Choi, Y., Pusede, S. E., Huey, G. L., Tanner, D. J., Kim, M., and Wennberg, P.: Observation-based modeling of ozone chemistry in the Seoul metropolitan area during the Korea-United States Air Quality Study (KORUS-AQ), Elem. Sci. Anth., 8, 3, https://doi.org/10.1525/elementa.400, 2020.
Shah, V., Jacob, D. J., Dang, R., Lamsal, L. N., Strode, S. A., Steenrod, S. D., Boersma, K. F., Eastham, S. D., Fritz, T. M., Thompson, C., Peischl, J., Bourgeois, I., Pollack, I. B., Nault, B. A., Cohen, R. C., Campuzano-Jost, P., Jimenez, J. L., Andersen, S. T., Carpenter, L. J., Sherwen, T., and Evans, M. J.: Nitrogen oxides in the free troposphere: implications for tropospheric oxidants and the interpretation of satellite NO2 measurements, Atmos. Chem. Phys., 23, 1227–1257, https://doi.org/10.5194/acp-23-1227-2023, 2023.
Sillman, S. and He, D.: Some theoretical results concerning O3-NOx-VOC chemistry and NOx-VOC indicators, J. Geophys. Res.-Atmos., 107, ACH 26-1–ACH 26-15, https://doi.org/10.1029/2001JD001123, 2002.
Silva, S. J., Heald, C. L., Ravela, S., Mammarella, I., and Munger, J. W.: A Deep Learning Parameterization for Ozone Dry Deposition Velocities, Geophys. Res. Lett., 46, 983–989, https://doi.org/10.1029/2018GL081049, 2019.
Simpson, W. R., Brown, S. S., Saiz-Lopez, A., Thornton, J. A., and von Glasow, R.: Tropospheric Halogen Chemistry: Sources, Cycling, and Impacts, Chem. Rev., 115, 4035–4062, https://doi.org/10.1021/cr5006638, 2015.
Singh, H. B., Brune, W. H., Crawford, J. H., Flocke, F., and Jacob, D. J.: Chemistry and transport of pollution over the Gulf of Mexico and the Pacific: spring 2006 INTEX-B campaign overview and first results, Atmos. Chem. Phys., 9, 2301–2318, https://doi.org/10.5194/acp-9-2301-2009, 2009.
Sklaveniti, S., Locoge, N., Stevens, P. S., Wood, E., Kundu, S., and Dusanter, S.: Development of an instrument for direct ozone production rate measurements: measurement reliability and current limitations, Atmos. Meas. Tech., 11, 741–761, https://doi.org/10.5194/amt-11-741-2018, 2018.
Souri, A. H., Choi, Y., Jeon, W., Li, X., Pan, S., Diao, L., and Westenbarger, D. A.: Constraining NOx emissions using satellite NO2 measurements during 2013 DISCOVER-AQ Texas campaign, Atmos. Environ., 131, 371–381, https://doi.org/10.1016/j.atmosenv.2016.02.020, 2016a.
Souri, A. H., Choi, Y., Li, X., Kotsakis, A., and Jiang, X.: A 15-year climatology of wind pattern impacts on surface ozone in Houston, Texas, Atmos. Res., 174–175, 124–134, https://doi.org/10.1016/j.atmosres.2016.02.007, 2016b.
Souri, A. H., Choi, Y., Jeon, W., Woo, J.-H., Zhang, Q., and Kurokawa, J.: Remote sensing evidence of decadal changes in major tropospheric ozone precursors over East Asia, J. Geophys. Res.-Atmos., 122, 2474–2492, https://doi.org/10.1002/2016JD025663, 2017.
Souri, A. H., Nowlan, C. R., Wolfe, G. M., Lamsal, L. N., Chan Miller, C. E., Abad, G. G., Janz, S. J., Fried, A., Blake, D. R., Weinheimer, A. J., Diskin, G. S., Liu, X., and Chance, K.: Revisiting the effectiveness of HCHO NO2 ratios for inferring ozone sensitivity to its precursors using high resolution airborne remote sensing observations in a high ozone episode during the KORUS-AQ campaign, Atmos. Environ., 224, 117341, https://doi.org/10.1016/j.atmosenv.2020.117341, 2020a.
Souri, A. H., Nowlan, C. R., González Abad, G., Zhu, L., Blake, D. R., Fried, A., Weinheimer, A. J., Wisthaler, A., Woo, J.-H., Zhang, Q., Chan Miller, C. E., Liu, X., and Chance, K.: An inversion of NOx and non-methane volatile organic compound (NMVOC) emissions using satellite observations during the KORUS-AQ campaign and implications for surface ozone over East Asia, Atmos. Chem. Phys., 20, 9837–9854, https://doi.org/10.5194/acp-20-9837-2020, 2020b.
Souri, A. H., Chance, K., Bak, J., Nowlan, C. R., González Abad, G., Jung, Y., Wong, D. C., Mao, J., and Liu, X.: Unraveling pathways of elevated ozone induced by the 2020 lockdown in Europe by an observationally constrained regional model using TROPOMI, Atmos. Chem. Phys., 21, 18227–18245, https://doi.org/10.5194/acp-21-18227-2021, 2021.
Souri, A. H., Johnson, M. S., Wolfe, G. M., Crawford, J. H., Fried, A., Wisthaler, A., Brune, W. H., Blake, D. R., Weinheimer, A. J., Verhoelst, T., Compernolle, S., Pinardi, G., Vigouroux, C., Langerock, B., Choi, S., Lamsal, L., Zhu, L., Sun, S., Cohen, R. C., Min, K.-E., Cho, C., Philip, S., Liu, X., and Chance, K.: Characterization of errors in satellite-based HCHO NO2 tropospheric column ratios with respect to chemistry, column-to-PBL translation, spatial representation, and retrieval uncertainties, Atmos. Chem. Phys., 23, 1963–1986, https://doi.org/10.5194/acp-23-1963-2023, 2023.
Souri, A. H., Duncan, B. N., Strode, S. A., Anderson, D. C., Manyin, M. E., Liu, J., Oman, L. D., Zhang, Z., and Weir, B.: Enhancing long-term trend simulation of the global tropospheric hydroxyl (TOH) and its drivers from 2005 to 2019: a synergistic integration of model simulations and satellite observations, Atmos. Chem. Phys., 24, 8677–8701, https://doi.org/10.5194/acp-24-8677-2024, 2024.
Stanier, C. O., Pierce, R. B., Abdi-Oskouei, M., Adelman, Z. E., Al-Saadi, J., Alwe, H. D., Bertram, T. H., Carmichael, G. R., Christiansen, M. B., Cleary, P. A., Czarnetzki, A. C., Dickens, A. F., Fuoco, M. A., Hughes, D. D., Hupy, J. P., Janz, S. J., Judd, L. M., Kenski, D., Kowalewski, M. G., Long, R. W., Millet, D. B., Novak, G., Roozitalab, B., Shaw, S. L., Stone, E. A., Szykman, J., Valin, L., Vermeuel, M., Wagner, T. J., Whitehill, A. R., and Williams, D. J.: Overview of the Lake Michigan Ozone Study 2017, B. Am. Meteorol. Soc., 102, E2207–E2225, https://doi.org/10.1175/BAMS-D-20-0061.1, 2021.
Stavrakou, T., Müller, J.-F., Bauwens, M., De Smedt, I., Lerot, C., Van Roozendael, M., Coheur, P.-F., Clerbaux, C., Boersma, K. F., van der A, R., and Song, Y.: Substantial Underestimation of Post-Harvest Burning Emissions in the North China Plain Revealed by Multi-Species Space Observations, Sci. Rep., 6, 32307, https://doi.org/10.1038/srep32307, 2016.
Strode, S. A., Ziemke, J. R., Oman, L. D., Lamsal, L. N., Olsen, M. A., and Liu, J.: Global changes in the diurnal cycle of surface ozone, Atmos. Environ., 199, 323–333, https://doi.org/10.1016/j.atmosenv.2018.11.028, 2019.
Tao, M., Fiore, A. M., Jin, X., Schiferl, L. D., Commane, R., Judd, L. M., Janz, S., Sullivan, J. T., Miller, P. J., Karambelas, A., Davis, S., Tzortziou, M., Valin, L., Whitehill, A., Civerolo, K., and Tian, Y.: Investigating Changes in Ozone Formation Chemistry during Summertime Pollution Events over the Northeastern United States, Environ. Sci. Technol., 56, 15312–15327, https://doi.org/10.1021/acs.est.2c02972, 2022.
Thompson, A. M., Balashov, N. V., Witte, J. C., Coetzee, J. G. R., Thouret, V., and Posny, F.: Tropospheric ozone increases over the southern Africa region: bellwether for rapid growth in Southern Hemisphere pollution?, Atmos. Chem. Phys., 14, 9855–9869, https://doi.org/10.5194/acp-14-9855-2014, 2014.
Thompson, C. R., Wofsy, S. C., Prather, M. J., Newman, P. A., Hanisco, T. F., Ryerson, T. B., Fahey, D. W., Apel, E. C., Brock, C. A., Brune, W. H., Froyd, K., Katich, J. M., Nicely, J. M., Peischl, J., Ray, E., Veres, P. R., Wang, S., Allen, H. M., Asher, E., Bian, H., Blake, D., Bourgeois, I., Budney, J., Bui, T. P., Butler, A., Campuzano-Jost, P., Chang, C., Chin, M., Commane, R., Correa, G., Crounse, J. D., Daube, B., Dibb, J. E., DiGangi, J. P., Diskin, G. S., Dollner, M., Elkins, J. W., Fiore, A. M., Flynn, C. M., Guo, H., Hall, S. R., Hannun, R. A., Hills, A., Hintsa, E. J., Hodzic, A., Hornbrook, R. S., Huey, L. G., Jimenez, J. L., Keeling, R. F., Kim, M. J., Kupc, A., Lacey, F., Lait, L. R., Lamarque, J.-F., Liu, J., McKain, K., Meinardi, S., Miller, D. O., Montzka, S. A., Moore, F. L., Morgan, E. J., Murphy, D. M., Murray, L. T., Nault, B. A., Neuman, J. A., Nguyen, L., González, Y., Rollins, A., Rosenlof, K., Sargent, M., Schill, G., Schwarz, J. P., Clair, J. M. S., Steenrod, S. D., Stephens, B. B., Strahan, S. E., Strode, S. A., Sweeney, C., Thames, A. B., Ullmann, K., Wagner, N., Weber, R., Weinzierl, B., Wennberg, P. O., Williamson, C. J., Wolfe, G. M., and Zeng, L.: The NASA Atmospheric Tomography (ATom) Mission: Imaging the Chemistry of the Global Atmosphere, B. Am. Meteorol. Soc., 103, E761–E790, https://doi.org/10.1175/BAMS-D-20-0315.1, 2022.
Thornton, J. A., Wooldridge, P. J., Cohen, R. C., Martinez, M., Harder, H., Brune, W. H., Williams, E. J., Roberts, J. M., Fehsenfeld, F. C., Hall, S. R., Shetter, R. E., Wert, B. P., and Fried, A.: Ozone production rates as a function of NOx abundances and HOx production rates in the Nashville urban plume, J. Geophys. Res.-Atmos., 107, ACH 7-10-ACH 7-17, https://doi.org/10.1029/2001JD000932, 2002.
Tibshirani, R.: Regression Shrinkage and Selection via the Lasso, J. R. Stat. B (Methodological), 58, 267–288, 1996.
Tilstra, L. G., de Graaf, M., Trees, V. J. H., Litvinov, P., Dubovik, O., and Stammes, P.: A directional surface reflectance climatology determined from TROPOMI observations, Atmos. Meas. Tech., 17, 2235–2256, https://doi.org/10.5194/amt-17-2235-2024, 2024.
Tonnesen, G. S. and Dennis, R. L.: Analysis of radical propagation efficiency to assess ozone sensitivity to hydrocarbons and NOx: 1. Local indicators of instantaneous odd oxygen production sensitivity, J. Geophys. Res., 105, 9213–9225, https://doi.org/10.1029/1999JD900371, 2000a.
Tonnesen, G. S. and Dennis, R. L.: Analysis of radical propagation efficiency to assess ozone sensitivity to hydrocarbons and NOx: 2. Long-lived species as indicators of ozone concentration sensitivity, J. Geophys. Res., 105, 9227–9241, https://doi.org/10.1029/1999JD900372, 2000b.
van der Velde, I. R., van der Werf, G. R., Houweling, S., Eskes, H. J., Veefkind, J. P., Borsdorff, T., and Aben, I.: Biomass burning combustion efficiency observed from space using measurements of CO and NO2 by the TROPOspheric Monitoring Instrument (TROPOMI), Atmos. Chem. Phys., 21, 597–616, https://doi.org/10.5194/acp-21-597-2021, 2021.
van Geffen, J., Eskes, H., Compernolle, S., Pinardi, G., Verhoelst, T., Lambert, J.-C., Sneep, M., ter Linden, M., Ludewig, A., Boersma, K. F., and Veefkind, J. P.: Sentinel-5P TROPOMI NO2 retrieval: impact of version v2.2 improvements and comparisons with OMI and ground-based data, Atmos. Meas. Tech., 15, 2037–2060, https://doi.org/10.5194/amt-15-2037-2022, 2022.
Veefkind, J. P., Aben, I., McMullan, K., Förster, H., de Vries, J., Otter, G., Claas, J., Eskes, H. J., de Haan, J. F., Kleipool, Q., van Weele, M., Hasekamp, O., Hoogeveen, R., Landgraf, J., Snel, R., Tol, P., Ingmann, P., Voors, R., Kruizinga, B., Vink, R., Visser, H., and Levelt, P. F.: TROPOMI on the ESA Sentinel-5 Precursor: A GMES mission for global observations of the atmospheric composition for climate, air quality and ozone layer applications, Remote Sens. Environ., 120, 70–83, https://doi.org/10.1016/j.rse.2011.09.027, 2012.
Verhoelst, T., Compernolle, S., Pinardi, G., Lambert, J.-C., Eskes, H. J., Eichmann, K.-U., Fjæraa, A. M., Granville, J., Niemeijer, S., Cede, A., Tiefengraber, M., Hendrick, F., Pazmiño, A., Bais, A., Bazureau, A., Boersma, K. F., Bognar, K., Dehn, A., Donner, S., Elokhov, A., Gebetsberger, M., Goutail, F., Grutter de la Mora, M., Gruzdev, A., Gratsea, M., Hansen, G. H., Irie, H., Jepsen, N., Kanaya, Y., Karagkiozidis, D., Kivi, R., Kreher, K., Levelt, P. F., Liu, C., Müller, M., Navarro Comas, M., Piters, A. J. M., Pommereau, J.-P., Portafaix, T., Prados-Roman, C., Puentedura, O., Querel, R., Remmers, J., Richter, A., Rimmer, J., Rivera Cárdenas, C., Saavedra de Miguel, L., Sinyakov, V. P., Stremme, W., Strong, K., Van Roozendael, M., Veefkind, J. P., Wagner, T., Wittrock, F., Yela González, M., and Zehner, C.: Ground-based validation of the Copernicus Sentinel-5P TROPOMI NO2 measurements with the NDACC ZSL-DOAS, MAX-DOAS and Pandonia global networks, Atmos. Meas. Tech., 14, 481–510, https://doi.org/10.5194/amt-14-481-2021, 2021.
Vigouroux, C., Langerock, B., Bauer Aquino, C. A., Blumenstock, T., Cheng, Z., De Mazière, M., De Smedt, I., Grutter, M., Hannigan, J. W., Jones, N., Kivi, R., Loyola, D., Lutsch, E., Mahieu, E., Makarova, M., Metzger, J.-M., Morino, I., Murata, I., Nagahama, T., Notholt, J., Ortega, I., Palm, M., Pinardi, G., Röhling, A., Smale, D., Stremme, W., Strong, K., Sussmann, R., Té, Y., van Roozendael, M., Wang, P., and Winkler, H.: TROPOMI–Sentinel-5 Precursor formaldehyde validation using an extensive network of ground-based Fourier-transform infrared stations, Atmos. Meas. Tech., 13, 3751–3767, https://doi.org/10.5194/amt-13-3751-2020, 2020.
Wang, T., Xue, L., Brimblecombe, P., Lam, Y. F., Li, L., and Zhang, L.: Ozone pollution in China: A review of concentrations, meteorological influences, chemical precursors, and effects, Sci. Total Environ., 575, 1582–1596, https://doi.org/10.1016/j.scitotenv.2016.10.081, 2017.
Wang, W., Parrish, D. D., Li, X., Shao, M., Liu, Y., Mo, Z., Lu, S., Hu, M., Fang, X., Wu, Y., Zeng, L., and Zhang, Y.: Exploring the drivers of the increased ozone production in Beijing in summertime during 2005–2016, Atmos. Chem. Phys., 20, 15617–15633, https://doi.org/10.5194/acp-20-15617-2020, 2020.
Warneke, C., Trainer, M., de Gouw, J. A., Parrish, D. D., Fahey, D. W., Ravishankara, A. R., Middlebrook, A. M., Brock, C. A., Roberts, J. M., Brown, S. S., Neuman, J. A., Lerner, B. M., Lack, D., Law, D., Hübler, G., Pollack, I., Sjostedt, S., Ryerson, T. B., Gilman, J. B., Liao, J., Holloway, J., Peischl, J., Nowak, J. B., Aikin, K. C., Min, K.-E., Washenfelder, R. A., Graus, M. G., Richardson, M., Markovic, M. Z., Wagner, N. L., Welti, A., Veres, P. R., Edwards, P., Schwarz, J. P., Gordon, T., Dube, W. P., McKeen, S. A., Brioude, J., Ahmadov, R., Bougiatioti, A., Lin, J. J., Nenes, A., Wolfe, G. M., Hanisco, T. F., Lee, B. H., Lopez-Hilfiker, F. D., Thornton, J. A., Keutsch, F. N., Kaiser, J., Mao, J., and Hatch, C. D.: Instrumentation and measurement strategy for the NOAA SENEX aircraft campaign as part of the Southeast Atmosphere Study 2013, Atmos. Meas. Tech., 9, 3063–3093, https://doi.org/10.5194/amt-9-3063-2016, 2016.
Wolfe, G. and Haskins, J.: AirChem/F0AM: v4.3.0.1 (v4.3.0.1), Zenodo [code], https://doi.org/10.5281/zenodo.10069985, 2023.
Wolfe, G. M., Marvin, M. R., Roberts, S. J., Travis, K. R., and Liao, J.: The Framework for 0-D Atmospheric Modeling (F0AM) v3.1, Geosci. Model Dev., 9, 3309–3319, https://doi.org/10.5194/gmd-9-3309-2016, 2016.
Wolfe, G. M., Hanisco, T. F., Arkinson, H. L., Blake, D. R., Wisthaler, A., Mikoviny, T., Ryerson, T. B., Pollack, I., Peischl, J., Wennberg, P. O., Crounse, J. D., St. Clair, J. M., Teng, A., Huey, L. G., Liu, X., Fried, A., Weibring, P., Richter, D., Walega, J., Hall, S. R., Ullmann, K., Jimenez, J. L., Campuzano-Jost, P., Bui, T. P., Diskin, G., Podolske, J. R., Sachse, G., and Cohen, R. C.: Photochemical evolution of the 2013 California Rim Fire: synergistic impacts of reactive hydrocarbons and enhanced oxidants, Atmos. Chem. Phys., 22, 4253–4275, https://doi.org/10.5194/acp-22-4253-2022, 2022.
Wu, Y., Zhao, K., Ren, X., Dickerson, R. R., Huang, J., Schwab, M. J., Stratton, P. R., Daley, H., Li, D., and Moshary, F.: Ozone pollution episodes and PBL height variation in the NYC urban and coastal areas during LISTOS 2019, Atmos. Environ., 320, 120317, https://doi.org/10.1016/j.atmosenv.2023.120317, 2024.
Xu, W., Zhang, G., Wang, Y., Tong, S., Zhang, W., Ma, Z., Lin, W., Kuang, Y., Yin, L., and Xu, X.: Aerosol Promotes Peroxyacetyl Nitrate Formation During Winter in the North China Plain, Environ. Sci. Technol., 55, 3568–3581, https://doi.org/10.1021/acs.est.0c08157, 2021.
Yousefian, F., Faridi, S., Azimi, F., Aghaei, M., Shamsipour, M., Yaghmaeian, K., and Hassanvand, M. S.: Temporal variations of ambient air pollutants and meteorological influences on their concentrations in Tehran during 2012–2017, Sci. Rep., 10, 292, https://doi.org/10.1038/s41598-019-56578-6, 2020.
Zara, M., Boersma, K. F., Eskes, H., Denier van der Gon, H., Vilà-Guerau de Arellano, J., Krol, M., van der Swaluw, E., Schuch, W., and Velders, G. J. M.: Reductions in nitrogen oxides over the Netherlands between 2005 and 2018 observed from space and on the ground: Decreasing emissions and increasing O3 indicate changing NOx chemistry, Atmos. Environ. X, 9, 100104, https://doi.org/10.1016/j.aeaoa.2021.100104, 2021.
Zhang, C., Jiang, Z., Liu, M., Dong, Y., and Li, J.: Relationship between summer time near-surface ozone concentration and planetary boundary layer height in Beijing, Atmos. Res., 293, 106892, https://doi.org/10.1016/j.atmosres.2023.106892, 2023.
Zhang, J., Wang, T., Chameides, W. L., Cardelino, C., Kwok, J., Blake, D. R., Ding, A., and So, K. L.: Ozone production and hydrocarbon reactivity in Hong Kong, Southern China, Atmos. Chem. Phys., 7, 557–573, https://doi.org/10.5194/acp-7-557-2007, 2007.