the Creative Commons Attribution 4.0 License.
the Creative Commons Attribution 4.0 License.
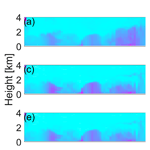
Lidar estimates of birch pollen number, mass, and CCN-related concentrations
Petri Tiitta
Xiaoxia Shang
Ari Leskinen
Pasi Ahola
Sanna Pätsi
Annika Saarto
Ville Vakkari
Uula Isopahkala
Mika Komppula
The accurate representation of microphysical properties of atmospheric aerosol particles – such as the number, mass, and cloud condensation nuclei (CCN) concentration – is key to constraining climate forcing estimations and improving weather and air quality forecasts. Lidars capable of vertically resolving aerosol optical properties have been increasingly utilized to study aerosol–cloud interactions, allowing for estimations of cloud-relevant microphysical properties. Recently, lidars have been employed to identify and monitor pollen particles in the atmosphere, an understudied aerosol particle with health and possibly climate implications. Lidar remote sensing of pollen is an emerging research field, and in this study, we present for the first time retrievals of particle number, mass, CCN, giant CCN (GCCN), and ultragiant CCN (UGCCN) concentration estimations of birch pollen derived from polarization lidar observations and specifically from a PollyXT lidar and a Vaisala CL61 ceilometer at 532 and 910 nm, respectively.
A pivotal role in these estimations is played by the conversion factors necessary to convert the optical measurements into microphysical properties. This set of conversion parameters for birch pollen is derived from in situ observations of major birch pollen events at Vehmasmäki station in eastern Finland. The results show that under well-mixed conditions, surface measurements from in situ instrumentation can be correlated with lidar observations at higher altitudes to estimate the conversion factors. Better linear agreement to the in situ observations was found at the longer wavelength of 910 nm, which is attributed to a combination of lower overlap and higher sensitivity to bigger particles compared to observations at 532 nm. Then, the conversion factors are applied to ground-based lidar observations and compared against in situ measurements of aerosol and pollen particles. In turn, this demonstrates the potential of ground-based lidars such as a ceilometer network with the polarization capacity to document large-scale birch pollen outbursts in detail and thus to provide valuable information for climate, cloud, and air quality modeling efforts, elucidating the role of pollen within the atmospheric system.
- Article
(5987 KB) - Full-text XML
- BibTeX
- EndNote
Pollen from genus Betula, commonly known as birch pollen, represents the most allergenic tree pollen type in northern, central, and eastern Europe (D'Amato et al., 2007). Currently, 8 %–16 % of the general population is sensitive to birch pollen, for half of whom this sensitivity manifests as respiratory and other allergy-related symptoms (Biedermann et al., 2019). The cross-reactivity of birch allergens with certain food can also trigger the pollen food allergy syndrome (Geroldinger-Simic et al., 2011; Wang et al., 2023), further impacting the quality of life of the people sensitive to this pollen type. The allergic symptoms are concentration dependent (Pfaar et al., 2017); thus, accurate information about the pollen load in the atmosphere is essential. Predictability of the pollination time and concentration in the air is important not only due to the adverse health effects but also for agricultural applications for certain crop species (Galveias et al., 2024) and its relevance to weather and climate. In this vein, climate change is projected to intensify the sensitization of the population to pollen even further (Lake et al., 2018; Zhang and Steiner, 2022) and may also make this type of bioaerosol relevant in aerosol–cloud interactions. Recent laboratory and model studies show that intact pollen grains and sub-pollen particles (SPPs) are likely to contribute to cloud processes and suppress precipitation (Wozniak et al., 2018), acting as cloud condensation nuclei (CCN), giant CCN (GCCN), ultragiant CCN (UGCCN) (Pope, 2010; Steiner et al., 2015; Griffiths et al., 2012; Mikhailov et al., 2019; Prisle et al., 2019), and ice nuclei (IN) (Diehl et al., 2001, 2002; Pummer et al., 2012; Hader et al., 2014; Dreischmeier et al., 2017; Gute et al., 2020; Burkart et al., 2021).
To date, more than 1000 stations worldwide (> 600 located in Europe, https://oteros.shinyapps.io/pollen_map/, last access: 15 September 2024) are monitoring pollen during the pollen season close to the ground level (Buters et al., 2018), with the majority of them using the Hirst-type sampler developed in the middle of the 20th century (Hirst, 1952). Although this way of sampling pollen has been standardized and it currently serves as the only reference method for pollen monitoring, it suffers from several drawbacks. Most frequently, data are available retrospectively within a week or more from the time of collection, limiting the breadth of application, and the temporal resolution varies from a couple of hours to a day. Furthermore, the analysis of the pollen samples is labor-intensive. Because of this, dispersion models have been exploiting pollen-specific phenological and meteorological relationships to forecast the concentration and distribution of pollen in the atmosphere (Sofiev et al., 2013). Huffman et al. (2020) and Buters et al. (2024) present an overview of recently developed instruments promising the autonomous and continuous identification of pollen in real time or near real time. These instruments utilize a broad range of measuring principles, from digital microscopy and holographic images to elastic scattering and fluorescence spectra. The advantage of using elastic scattering and fluorescence techniques (Veselovskii et al., 2021) as part of a remote sensor is that they can further provide systematic information on the vertical distribution of pollen in the atmosphere, information that is currently missing and that is essential for model verification and assimilation procedures.
Polarization lidars, which are active remote sensors, have been increasingly utilized to study pollen, as the non-spherical structure of some of the pollen types induce moderate to strong laser depolarization (Sassen, 2008; Noh et al., 2013; Sicard et al., 2016; Bohlmann et al., 2019). Past efforts have focused on the optical properties of different pollen types as well as their vertical distribution in the atmosphere (Bohlmann et al., 2019; Shang et al., 2020; Bohlmann et al., 2021; Shang et al., 2022). Although the linear particle depolarization ratio (PDR) of pollen has been the focus of laboratory-based studies (Cao et al., 2010; Cholleton et al., 2022a, b), the characteristic PDR of some pollen species in atmospheric conditions has been only determined recently (Filioglou et al., 2023). Knowledge about the PDR for different aerosol types is of paramount importance in lidar-based aerosol classification algorithms (Nicolae et al., 2018) and methodologies estimating the aerosol microphysical properties from lidar observations. In particular, in the polarization lidar photometer networking (POLIPHON) method (Ansmann et al., 2012; Mamouri and Ansmann, 2016, 2017) the contribution of spherical and non-spherical particles to the observed optical effect is determined utilizing aerosol type-dependent PDRs (Tesche et al., 2009). Then, estimations of the number, mass, CCN, and IN concentrations for an aerosol type are possible if specific conversion factors are known. These conversion factors are usually determined from Aerosol Robotic Network (AERONET) climatologies of optical and microphysical properties (Shinozuka et al., 2015; Mamouri and Ansmann, 2015, 2016; Ansmann et al., 2019; He et al., 2023). To this end, conversion factors for pollen particles have not been estimated. In addition, the 30 µm upper size limitation in the aerosol particle diameter of the AERONET inversion products may not be representative of the larger pollen species.
In this article, we extend the applicability of lidars to estimate the number, mass, and CCN-related concentration of birch pollen. The microphysical properties of birch pollen were estimated using a synergy of lidar observations and in situ aerosol instrumentation. Specifically, the conversion factors needed and estimates of the aforementioned microphysical properties for birch pollen were determined at 532 and 910 nm wavelengths utilizing observations from a PollyXT lidar and a Vaisala CL61 ceilometer. The lidar-derived microphysical estimates of birch pollen were compared against in situ pollen and aerosol observations and further aided utilizing the mixing-layer heights retrieved from a HALO Photonics StreamLine Pro Doppler lidar.
The paper is organized as follows. A summary of the site location, instrumentation, and methods are given in Sect. 2, with focus on the determination of pollen conversion factors from in situ observations. The results are presented in Sect. 3. Section 3 also includes a case study showcasing the eligibility of the lidar-derived pollen microphysical estimates. A discussion and concluding remarks are given in Sects. 4 and 5, respectively.
2.1 Site description
Between 2016 and 2023, six measurement campaigns were carried out at Vehmasmäki station in eastern Finland (62°44′ N, 27°33′ E; 190 m above sea level), focusing on pollen (Fig. 1). The rural station is surrounded by broad-leaved and coniferous trees, and it is located 18 km away from the city center of Kuopio. The site is equipped with a multi-wavelength PollyXT lidar (Engelmann et al., 2016), a Vaisala CL61 ceilometer, a HALO Photonics StreamLine Pro Doppler lidar, and various in situ instruments for aerosol characterization up to 10 µm aerosol particles as well as meteorological quantities from the station and a 318 m tall mast. In addition, a holographic imaging instrument (ICEMET, icing condition evaluation method) was installed on site in 2021, allowing for determining the shape and size distribution of 5–200 µm aerosol particles. The station has been operated by the Finnish Meteorological Institute since autumn 2012 (Hirsikko et al., 2014), and it is part of EARLINET (Shang et al., 2022) and PollyNET (Baars et al., 2016). During the measurement campaigns which lasted from March to August each year, the pollen type and concentration were determined from the samples collected with a Hirst-type volumetric air sampler (hereafter Burkard sampler). The dominant pollen types over the site are alder (Alnus), birch (Betula), pine (Pinus), and spruce (Picea) in spring and early summer, with herbaceous species such as Poaceae and Urticaceae later in the summer (Fig. 2). Typically, the aerosol load is low over the measurement location, and aerosol particles are located mostly within the first 2 km with occasional intrusions of smoke and dust particles in the free troposphere (Baars et al., 2016; Bohlmann et al., 2019; Shang et al., 2020). Therefore, the site presents favorable conditions for characterizing pollen particles and investigating their role in various atmospheric processes.
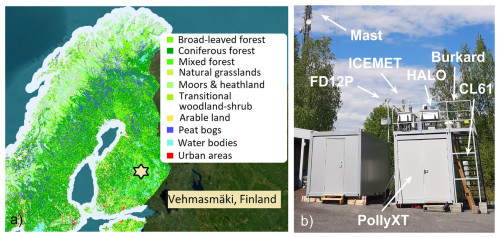
Figure 1(a) Measurement site location and land cover from the Copernicus CORINE (Coordination of Information on the Environment) Land Cover inventory in 2018 (https://www.eea.europa.eu/en/datahub/datahubitem-view/a5144888-ee2a-4e5d-a7b0-2bbf21656348, last access: 10 November 2024). (b) In situ and remote sensing instruments available on site. The Burkard sampler, ICEMET, Vaisala FD12P sensor, and HALO Photonics Doppler lidar are located on the roof of the main container at 4 m a.g.l. The Vaisala CL61 ceilometer is located at the back of the main container. The PollyXT, NanoScan, OPS (optical particle sizer), and AE (Aethalometer) instruments are located inside the container. In close proximity a 318 m tall mast equipped with weather sensors at different height levels provides profiles of various meteorological quantities.
2.2 The PollyXT lidar
The PollyXT (Engelmann et al., 2016) is a 12-channel high-power lidar, allowing for the estimation of the particle backscatter coefficient (β) at 355, 532, and 1064 nm wavelengths and the volume depolarization ratio (VDR) and particle linear depolarization ratio (PDR) at 355 and 532 nm wavelengths. Additionally, extinction coefficients at 355 and 532 nm are available during nighttime, utilizing the Raman technique (Ansmann et al., 1992). Water vapor mixing ratio profiles can be retrieved during dark hours using the 407 nm Raman-shifted wavelength (Filioglou et al., 2017). This PollyXT version features a second near-field telescope retaining full overlap at about 120 m. For the near field, the elastic 355 nm and 532 nm and the equivalent Raman-shifted wavelengths are detected. Information above 400 m is considered at 532 nm since PDR observations are detected at the far field only. Observations from 355 nm were omitted as the combination of the high overlap region which is at 800 m and the low birch PDR introduced high uncertainty in the retrievals, limiting the availability of cases and robust conclusions. The vertical resolution amounts to 7.5 m, and the temporal resolution is 30 s. A detailed description of the operating principle as well as uncertainties expected for the optical properties can be found in Engelmann et al. (2016) and Baars et al. (2016). To retrieve the necessary β and PDR profiles utilized in this work, the backward Klett inversion was performed (Klett, 1981) on 2 h temporally averaged profiles. A constant lidar ratio (LR) of 60 sr was used for the inversion (Bohlmann et al., 2019; Shang et al., 2020, 2022), while information below 400 m a.g.l. (above ground level) was omitted.
2.3 The Vaisala CL61 ceilometer
The Vaisala CL61 ceilometer is a 910.55 nm single-channel pulsed laser diode elastic lidar transmitting linearly polarized light into the atmosphere. An alternating polarizing sheet filter enables the recording of the return light in the same channel at two different polarization states, termed co-polar and cross-polar. This setup allows for the determination of both the attenuated backscatter coefficient and VDR. Full overlap is reached at about 300 m a.g.l., and raw profiles are available at a temporal resolution of 5 s (for the attenuated backscatter coefficient) and 10 s (for the VDR). The range resolution is 4.8 m.
To retrieve the β and PDR profiles, the forward Klett inversion was performed using a constant LR of 60 sr (Wiegner and Gasteiger, 2015). The calibration factor required for the forward inversion was determined following the stratocumulus cloud method (O'Connor et al., 2004). A 5 %–10 % uncertainty is anticipated with this method to the particle backscatter coefficient (Hopkin et al., 2019; Filioglou et al., 2023). In order to increase the signal-to-noise ratio (SNR) and harmonize the lidar observations to the temporal resolution of the Burkard sampler (see Sect. 2.5), 2 h temporal averaging was considered when retrieving the aforementioned optical properties. Information below 200 m a.g.l. was omitted. We only considered observations during 2021 and 2022, since during the birch pollen period in May 2023, the instrument experienced condensation in the main window, making the calibration challenging, and therefore those data were omitted to ensure high-quality retrievals.
2.4 The HALO Photonics StreamLine Pro Doppler lidar
A HALO Photonics StreamLine Pro scanning Doppler lidar (Pearson et al., 2009) was located at Vehmasmäki station during the campaigns. This pulsed Doppler lidar operates at 1565 nm and is capable of scanning within a 20° cone from vertical, i.e., elevation angles of 70–90°. The minimum usable range of the instrument is 90 m, as the lower range gates are affected by the outgoing pulse and the maximum range is 9.6 km a.g.l. The range resolution of the lidar is 30 m. The Doppler lidar was configured to perform a velocity azimuth display (VAD) scan with 24 azimuthal angles at 75° elevation angle every 15 min. Between VAD scans the lidar operated in vertical stare mode, alternating between co- and cross-polar receiver mode.
Data from the Doppler lidar were post-processed according to Vakkari et al. (2019), and an SNR threshold of 0.001 was applied to the vertically pointing measurements. Horizontal wind profiles were retrieved from the VAD scans following Browning and Wexler (1968). Turbulent kinetic energy (TKE) dissipation rate profiles were calculated using the method by O'Connor et al. (2010) from the co-polar vertical stare measurements with the horizontal wind profiles from VAD scans. Instrumental noise contribution was estimated from SNR profiles according to Pearson et al. (2009) and subtracted from the vertical wind variance time series before the TKE dissipation rate calculation. The mixing-layer height (MLH) was estimated from the TKE dissipation rate profiles using a threshold of 10−4 m2 s−3, similar to previous studies (e.g., Vakkari et al., 2015).
2.5 Burkard sampler: Hirst-type volumetric air sampler
Airborne pollen was collected at 4 m a.g.l. utilizing a Hirst-type volumetric air sampler manufactured by Burkard Manufacturing Co. Ltd in the UK (Hirst, 1952). The sampling tapes were cut in sections representing full days and analyzed with light microscopy. In the analyses, the pollen grains were identified at the genus or family level by comparing their characteristic shape and individual features with known pollen. The sample tapes were counted on a bi-hourly basis, taking four randomized samples per each strip representing a 2 h time period (Mäkinen, 1981). An uncertainty of 30 % is anticipated in the pollen concentration with this type of pollen sampling (Buters et al., 2012; Tormo-Molina et al., 2013; Adamov et al., 2021; Triviño et al., 2023).
In addition, 35 randomly selected images were extracted between 08:00 and 17:00 local time (LT) on 12 May 2021 and between 10 May 2023 at 03:00 LT and 11 May 2023 at 03:00 LT for every hour to investigate the particle size distribution of birch pollen. The images were acquired from the Burkard sampler using an optical microscope equipped with a digital microscope camera. The microscope glide table was first placed on a random crosswise position and then repositioned lengthwise 1 h ahead to obtain images representing every hour of the samples. Then, the Olympus cellSens Entry imaging software was used to mechanically measure the geometrical diameters of the particles identified as birch pollen grains in each image.
2.6 Aerosol in situ observations
2.6.1 Aerosol size distribution
We measured the aerosol size distribution in the size range from 10 nm to 200 µm with three different instruments: a NanoScan scanning mobility particle sizer (hereafter NS; model 3910, TSI Incorporated, USA), an optical particle sizer (hereafter OPS; model 3330, TSI Incorporated, USA), and a digital in-line holographic imaging instrument (hereafter ICEMET; University of Oulu, Finland; Kaikkonen et al., 2020). Samples for the NS and OPS were taken 5 m a.g.l. and delivered via a stainless-steel line to the instruments inside an air-conditioned container at a combined flow rate of 1.8 L min−1 (0.8 L min−1 for the NS, 1.0 L min−1 for the OPS). The ICEMET, in turn, was located on the roof of the container, and it was at the same height as the inlets for the NS and OPS (5 m a.g.l.). The NS and OPS measured one size distribution every 1 min, whereas the ICEMET recorded one hologram per second.
The NS size distribution (mobility diameter of 10–420 nm in 13 size bins) and OPS size distribution (optical diameter of 0.3–10 µm in 16 size bins) were combined in a similar way as in our earlier works (Filioglou et al., 2023; Leskinen et al., 2020) again by neglecting the last two bins of the NS aerosol size distribution and the first bin of the OPS aerosol size distribution, by converting the OPS optical diameters to geometric-mean-volume-equivalent diameters (Alas et al., 2019), and by using the long-term average of 1.46−0.009i of the complex refractive index at Vehmasmäki (Filioglou et al., 2023).
The main components of the ICEMET are a 660 nm wavelength laser diode, which acts as a point light source, and an image sensor with a resolution of 2048 × 2048 pieces of 3.45 µm pixels. The light source and image sensor are inside opposite disk-like housings behind protective windows. The disks are 10 cm in diameter and at a distance of 3 cm from each other. The sensing region between the disks is a truncated pyramid volume. When the coherent light from the light source scatters from the objects in the sensing volume, which is about 0.4 cm−3, and interferes with the other parts of the light field, a hologram, a complex diffraction pattern, is formed and recorded on the image sensor and later processed digitally. In this work, the diffraction patterns were processed using the ICEMET Server software (Molkoselkä, 2020), releases 1.6.0–1.14.0, depending on the year, giving the size, shape, and location of each particle in the sensing region. The ICEMET used in this work had a theoretical effective particle detection size limit of 5.3 µm and was equipped with a tail wing that turned the instrument according to the prevailing wind direction so that there was an open path for the particles to enter the sensing region. It must be noted that the ICEMET was not available during the birch pollen season in May 2022; therefore ICEMET observations in this work comprise those from the years 2021 and 2023.
2.6.2 Black carbon observations
The black carbon (BC) concentration was measured with an Aethalometer (hereafter AE; model AE-31, Aerosol Magee Scientific, Slovenia). The instrument collects sample on a quartz fiber filter, illuminates the sample with light sources at seven wavelengths (370–950 nm), records the light attenuation, and outputs the BC concentrations at the seven wavelengths with a selected time resolution (5 min in this work). The BC concentration hereafter refers to the output at 880 nm, which was corrected for filter loading and multiple scattering in a way similar to that in Leskinen et al. (2020). In the correction, a long-term average multiple-scattering correction factor of 4.75 at 880 nm at Vehmasmäki was used (Filioglou et al., 2023). The BC observations were used to filter out the presence of smoke particles during the pollen measurement campaigns.
2.7 The Vaisala FD12P weather sensor
To aid the analysis, co-located observations from a Vaisala FD12P weather sensor were also considered. The instrument is capable of deriving the visibility, precipitation type, intensity, and duration of precipitation at the measurement location. In the present study, the FD12P visibility and precipitation information were used to exclude cloudy times. In particular, cases where the visibility was less than 2 km or the precipitation flag was not 0, which is indication of cloud development, precipitation, or fog, were omitted.
2.8 Particle mass concentration calculations
2.8.1 Particle mass concentration from in situ observations
Surface particle mass concentration estimations of birch pollen were calculated utilizing aerosol size distributions from ICEMET observations. The 2 h temporally averaged aerosol number concentrations (dN) were converted to volume concentrations (dV) using the mean diameter (d) of each size bin following . The volume concentration of aerosol particles in the range between 12 and 35 µm was further summed and multiplied by the mass density (ρ) of birch, which is assumed to be ρ = 0.8 g cm−3 (Gregory, 1961), yielding the coarse-mode birch mass concentration. This size range is indicative of birch pollen considering discrepancies within the birch pollen family (Stiebing et al., 2022; Raith and Swoboda, 2023; Theuerkauf et al., 2024).
Rather than employing a fixed birch pollen size to estimate mass concentration from the Burkard sampler, the mean volume diameter in the range between 12 and 35 µm from the ICEMET was utilized.
2.8.2 Particle mass and number concentration from lidar observations
Lidar-derived number and mass concentration methods have emerged over the past 15 years. In particular, in Ansmann et al. (2011) a synergy of lidar–photometer observations was developed to estimate the mass concentration of aerosol particles (m). The method requires that the mass density (ρ), the extinction-to-volume conversion factor (cv), and the particle extinction coefficient for a specific aerosol type at a certain wavelength to be known according to , where λ is the wavelength. The goal of this study is to provide the conversion factor that permits the estimation of birch number and mass concentration from lidar observations at 910 nm utilizing observations from a Vaisala CL61 ceilometer. To retrieve the number concentration, a similar procedure is followed according to , where n is the number concentration and cn denotes the extinction-to-number conversion factor.
2.8.3 The extinction-to-volume and extinction-to-number conversion factors (cv, cn)
To this end, cv is estimated using the relationship between the vertically integrated (column) particle volume concentration from photometric observations via the AERONET inversion and the layer mean particle extinction coefficient α from lidar observations (Ansmann et al., 2019). Related to the volume concentration, the AERONET algorithm considers particles with radii up to 15 µm (i.e., diameters up to 30 µm). This size limitation may introduce a significant bias in the volume size distribution of aerosols exhibiting that size, such as in fresh volcanic plumes, resulting in an underestimation of more than 100 % in the lidar-derived mass aerosol load (Ansmann et al., 2012). Since some pollen types are larger than 30 µm, the AERONET inversion method may not be representative of this aerosol type. To tackle the issue, the volume aerosol size distribution from the ICEMET was utilized considering the size range from 12 to 35 µm. The extinction-to-number conversion factor cn has a similar retrieval procedure, where the number aerosol size distribution from the ICEMET was utilized, instead of the volume one.
The second required parameter for the cv (cn) calculation is the α for the specific aerosol type. The birch extinction coefficient αbirch was derived by polarization lidar observations based on the backward (forward) Klett–Fernald inversion method for PollyXT (CL61) observations and the birch component separation method from Tesche et al. (2009). We assumed a simple, externally mixed two-component aerosol when using this separation technique. For the separation, the PDR of birch pollen from Filioglou et al. (2023) was utilized as the non-spherical aerosol component. A PDR of 0.03 was used as the spherical aerosol component (Shang et al., 2020; Bohlmann et al., 2019). Portin et al. (2014) have explored the chemical composition of the aerosol population in the area and found that sulfate, nitrate, ammonium, and organics are present at Kuopio, about 20 km from Vehmasmäki station, where the inorganic-to-total ratio was about 42 %. To account for the lidar overlap height limitation, the MLH from HALO Doppler observations was employed. Cases with an MLH top higher than 400 (200 m) were considered for PollyXT (CL61) observations, respectively, at any point during the 2 h temporal averaging, in which the share of birch pollen from the Burkard sampler was more than 90 % in the pollen mixture. Moreover, possible dust and smoke intrusions were excluded, utilizing BC observations from the AE instrument (BC < 0.1 µg m−3) and modeled dust optical depth (DOD; DOD < 0.03) provided by the World Meteorological Organization (WMO) Barcelona Dust Regional Center (https://dust.aemet.es/products/daily-dust-products, last access: 15 September 2024). From the surface up to the MLH top, the variation in βbirch may largely fluctuate depending on the amount, type, and distribution of birch pollen in the aerosol mixture. In order to reduce the uncertainty introduced by these factors and increase the comparability of the lidar observations to the surface observations, we used the mean βbirch between 400 and 450 m a.g.l. (200 and 250 m a.g.l.) for observations from the PollyXT (CL61), which was multiplied by an LR of 60 sr (Bohlmann et al., 2019; Shang et al., 2020, 2022) and converted to αbirch.
2.9 CCN-related concentrations
2.9.1 CCN, GCCN, and UGCCN estimation from in situ aerosol observations
For the estimation of the CCN (nCCN,birch), GCCN (nGCCN,birch), and UGCCN (nUGCCN,birch) number concentration, the number aerosol size distribution in the particle size range of 130 nm to 35 µm (n0.13–35 µm), 1 to 35 µm (n1–35 µm), and 10 to 35 µm (n10–35 µm) were considered, utilizing NS–OPS–ICEMET, OPS–ICEMET, and ICEMET observations, respectively. Note that all CCN-related estimations consider that birch pollen grains, submicron birch SPPs, and other biological material co-exist in the bioaerosol mixture without being able to be distinguished from each other with the current instrumental setup. The 130 nm size limit was chosen as most birch SPPs below this size remain inactive at 0.18 % supersaturation (ss) (Mikhailov et al., 2021). At this supersaturation, Mikhailov et al. (2021) found the hygroscopicity of birch pollen particles, the kappa value, to be k = 0.13 ± 0.02, and an estimation of the activated particles can be made according to
Since birch pollen is low in concentration in the atmosphere compared to other aerosol particles which may more actively contribute to nCCN and since with the current instrumental setup we cannot denote the existence of SPPs of birch pollen and other biological material (e.g., spores, fungi, algae) in the aerosol mixture, an extra step was necessary. To counterbalance the contribution of other particles, nCCN,birch was estimated by subtracting the average nCCN on site at times when there was no pollen indication in Burkard observations or dust/smoke intrusions. A mean nCCN of 27 cm−3 was estimated for Vehmasmäki station in 2021 and 2023. This number was then used during birch pollen times in order to determine nCCN,birch. For nGCCN,birch and nUGCCN,birch, a mean concentration of 0.13 and cm−3 was estimated, respectively. Furthermore at these large particle sizes, we consider that all particles are the reservoir of potential GCCN and UGCCN.
2.9.2 CCN, GCCN, and UGCCN estimation from lidar observations
A procedure similar to that of the number and mass concentration was followed for the CCN-related estimations from the lidar observations following the methodology of Mamouri and Ansmann (2016). Specifically, using the modified equations for birch pollen, the number concentrations of CCN, GCCN, and UGCCN can be estimated as follows:
with an enhancement factor fss,birch of 1.0 for an ss of 0.18 % and a number concentration of n0.13–35 µm,birch,dry (considering particles with a radius between 130 nm and 35 µm), n1–35 µm,birch,dry (considering particles with a radius between 1 and 35 µm), and n10–35 µm,birch,dry (considering particles with a radius between 10 and 35 µm). For the calculation of n0.13–35 µm,birch,dry, n1–35 µm,birch,dry, and n10–35 µm,birch,dry, the following equations were used:
For the conversion of αbirch into n0.13–35 µm,birch,dry, n1–35 µm,birch,dry, and n10–35 µm,birch,dry, the conversion parameters c0.13–35 µm,birch, c1–35 µm,birch, and c10–35 µm,birch and exponent x0.13–35 µm,birch needed to be determined. Equation (5) assumes a linear correlation of log n0.13–35 µm,birch with log αbirch. These parameters were determined for each wavelength using the NS–OPS–ICEMET, OPS–ICEMET, and ICEMET aerosol size distributions, respectively.
3.1 In situ birch pollen observations
3.1.1 Birch pollen size distribution
In order to get better insight into the comparability of the Burkard sampler and ICEMET observations with regard to the number and mass concentration, the birch pollen particle size distribution needs to be considered. Figure 3a–b show two light microscopy extracted images of the sampling tapes from Burkard sampler during high and low birch pollen concentration on 12 May 2021 around 09:00 LT and 10 May 2023 around 17:00 LT, respectively. These images represent a fraction of the birch size distribution at the given time and were randomly selected from the Burkard samples (see Sect. 2.5). Translating the images into birch pollen size distribution considering a 1 µm bin size, it is evident that birch pollen appears in a range of particle sizes (Fig. 3c–d). The analysis of 35 such samples determined a mean birch pollen size of 21.0 µm over the measurement site (Fig. 3e). The minimum and maximum birch pollen size concluded via this method was 15.6 and 25.7 µm, respectively, showcasing a difference of over 10 µm in the birch pollen size. Primarily, the size of birch pollen depends on the type of birch tree; therefore regional discrepancies may be anticipated within the birch family. Previously, birch pollen has been found in the range of 17.3–35 µm (Stiebing et al., 2022; Raith and Swoboda, 2023; Theuerkauf et al., 2024). Then, meteorological conditions such as the relative humidity and temperature may further affect the size and shape of atmospheric pollen.
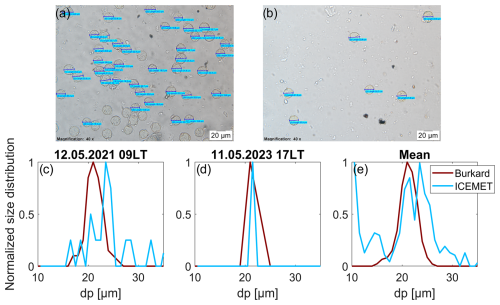
Figure 3Microscope-extracted images of birch pollen from the sampling tapes collected with the Burkard sampler on (a) 12 May 2021 at 09:00 LT and (b) 10 May 2023 at 17:00 LT. The Olympus cellSens Entry imaging software was used to mechanically measure the geometrical diameters of the particles (dp; blue lines) identified as birch pollen grains for up to 40 individual grains per sample. (c–d) Burkard-estimated normalized birch pollen size distributions during the two aforementioned cases considering a particle size bin of 1 µm (solid red lines) and the equivalent aerosol size distribution from the ICEMET between 12 and 35 µm (solid blue lines). (e) A 35-sample mean birch pollen size distribution from the Burkard sampler. The samples were randomly extracted between 12 May 2021 from 08:00 to 17:00 LT and from 10 May 2023 at 03:00 LT to 11 May 2023 at 03:00 LT every hour. Equivalent aerosol size distributions from the ICEMET instrument for the same cases are marked with solid blue lines.
Equivalent particle size distributions from the ICEMET are shown in Fig. 3c–e. For comparability reasons, the particle size distributions from the Burkard sampler and ICEMET are normalized. Both the Burkard sampler and ICEMET present similar particle size distributions, suggesting that the ICEMET instrument is able to observe aerosol particles in the size range of birch pollen. Please note that while Burkard samples present a snapshot of the birch size distribution at a given time, the ICEMET provides continuous monitoring of the aerosol particle size distribution, and in this case a ±15 min averaging around the Burkard samples was considered. Therefore marginal discrepancies are anticipated. Also, both instruments sense the geometrical particle diameter, and therefore their diameters are directly comparable. Primarily, the aerosol particle size distribution from ICEMET observations presents multiple aerosol modes in the 12–35 µm size range, which may possibly be a more realistic real-time representation of the birch pollen size distribution (Fig. 3e). Dust and volcanic aerosol presence is excluded using the modeled DOD and the Ozone Mapping and Profiler Suite (OMPS) sulfur dioxide (SO2) planetary boundary layer (PBL) available at https://worldview.earthdata.nasa.gov/ (last access: 31 January 2025).
3.1.2 Number and mass concentration comparison
Since the Burkard sampler and ICEMET present fundamentally different operating principles, it is essential to perform a comparison with regard to the number and mass concentrations as well. Figure 4a shows the progress of birch pollen season from Burkard (red line) and ICEMET observations (blue lines) from 11 May 2021 at 08:00 LT to 15 May 2021 at 08:00 LT. Cloud, fog, and precipitation cases were removed using observations from the FD12P sensor located on site. The ICEMET performed well over the intense birch pollen times, sufficiently mirroring the progress of the birch pollen season seen in Burkard observations. This is not the case for the absolute concentration of birch pollen. Further exploring the absolute concentration discrepancy in the 2 h data, the closest ICEMET concentration to the Burkard one within the 2 h time window is also presented (dashed blue line). Undoubtedly, the agreement is much better, but to this end, a solid conclusion on this discrepancy is not possible. It is not possible because a calibration standard for airborne pollen has not yet been developed, and the Burkard methodology is not foolproof. For example, the better agreement shown in Fig. 4a may result from methodological procedures in Burkard during the data analysis (e.g., the randomized four small areas as representative of the 2 h) and upscaling of these areas to reflect the 2 h pollen concentration assuming a constant multiplication factor (Mäkinen, 1981). This approach may bias the pollen concentration. Moreover, the discrepancy in absolute concentration may result from the different temporal resolution between the two techniques in conjunction to boundary layer changes and the inhomogeneous distribution of pollen within this 2 h time frame.
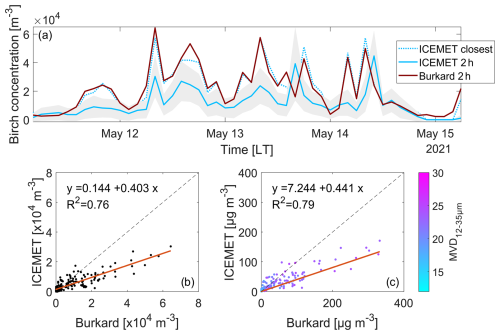
Figure 4(a) The progress of the birch pollen season between 11 May 2021 at 05:00 LT and 15 May 2021 at 05:00 LT at Vehmasmäki station in Finland. The 2 h temporal progress of the birch pollen season from Burkard is noted with the solid red line. The total ICEMET concentration in a size range between 12 and 35 µm at 2 h temporal resolution and the closest ICEMET concentration to that of Burkard within the 2 h time frame are marked with solid and dashed blue lines, respectively. The shaded area denotes the standard deviation within the 2 h period. (b) Scatter plots of the agreement between the Burkard and ICEMET number concentration considering birch cases during 2021 and 2023 with a 2 h temporal resolution. (c) Similar results for the mass concentration agreement between the two in situ instruments. To convert the number to mass concentration for Burkard observations, the mean volume diameter (MVD) of the size range between 12 and 35 µm from the ICEMET was considered. Both panels (b) and (c) contain additional information regarding the fit and correlation of the two datasets. The dashed black line represents the 1 : 1 reference line.
To put the time series into perspective, Fig. 4b–c present the overall agreement of birch concentration between the two instruments considering observations during 2021 and 2023. For the conversion of the Burkard birch pollen number concentration to mass, the mean volume diameter (MVD) from the ICEMET between 12 and 35 µm was considered. There is a systematic offset between Burkard- and ICEMET-estimated particle concentrations (assuming Burkard observations are correct). This similar feature was recently reported when Burkard observations were compared against newly developed automatic systems (Maya-Manzano et al., 2023).
3.2 Lidar birch pollen observations
3.2.1 Birch pollen conversion factors
Figure 5 summarizes the birch conversion factors for the number and mass concentration estimation utilizing the number and volume size distribution from ICEMET observations and equivalent mean birch extinction coefficients from the lidars. Despite the multiyear pollen observation availability on site, the conversion factors for the number and mass concentrations from the PollyXT are extracted from 2-year observations during 2021 and 2023, while equivalent factors from the CL61 ceilometer consider 2021 observations due to the instrument availability of the sensors involved. For the CCN-related conversion factors, the datasets used for CCN and GCCN are for 2021 for both lidars, while the UGCCN dataset for the PollyXT includes both 2021 and 2023. For the CL61, the UGCCN conversion factor is extracted from the 2021 dataset. Cases with an MLH lower than 400 m for the PollyXT and 200 m for the CL61, within the 2 h temporal averaging, and dust and smoke intrusions are excluded utilizing HALO Doppler lidar, AE observations, and modeled DOD. A total of 38 and 25 cases with a 2 h temporal averaging each are considered at 532 and 910 nm wavelengths, respectively, where the mean conversion factors are indicated by the regression lines and summarized in Table 1. The birch extinction lidar coefficient exhibits a linear relationship with the number and volume particle size distribution from ICEMET observations for the particle range 12–35 µm. The highest birch concentration on site is represented with the topmost point in all four panels. It was observed by the Burkard instrument on 12 May 2021 at 08:00 UTC (07:00–09:00 UTC). For the 532 nm wavelength this point deviates from the linearity, and it can be due to the transitioning of the boundary layer during the 2 h time frame along with the non-uniformity of the aerosol layer and the wavelength sensitivity to the aerosol particle size population. This is not valid at 910 nm due to the combination of the lower overlap for the CL61 and the higher sensitivity of this wavelength to bigger particles. For the derivation of the number and mass conversion factors, we consider birch pollen in the abovementioned particle size range. Nonetheless, we cannot exclude that smaller birch pollen, fragments of it, or other biological material particles are present in the aerosol mixture and therefore may contribute, due to their size in relation to the lidar wavelength, to the aerosol mixture and therefore to the estimated extinction coefficient. This mainly impacts cn, rather than cv, since the contribution of aerosol particles between 2.5–12 µm in the volume is insignificant (see Sect. 4).
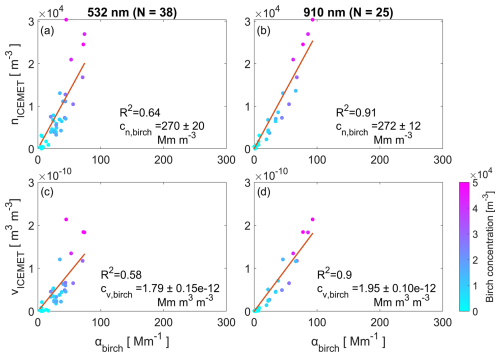
Figure 5Relationship between the birch extinction coefficient αbirch at 532 nm and (a) the particle number concentration nICEMET and (c) the volume concentration vICEMET considering particles between 12 and 35 µm. Correlations are shown utilizing the mean birch extinction coefficient between 400–450 m a.g.l. The slope indicates the conversion factors cn,birch and cv,birch and are also given as numbers in the panels along with the goodness of the fit expressed through R2 statistical value. Equivalent results at 910 nm are given in panels (b) and (d).
Table 1Birch conversion parameters essential to convert the particle extinction coefficient of birch αbirch at 532 and 910 nm into particle number and mass concentration. The mean values and standard error (SE) of the extinction-to-number and extinction-to-volume conversion factors (cn, cv) for birch pollen are derived from in situ ICEMET observations considering the particle size range of 12–35 µm. The necessary conversion factors for the CCN-related estimations c0.13–35 µm,birch, c1–35 µm,birch, c10–35 µm,birch, and x0.13–35 µm,birch (Eqs. 5–7) are obtained according to Sect. 2.9.2 from NS–OPS–ICEMET, OPS–ICEMET, and ICEMET observations, respectively.
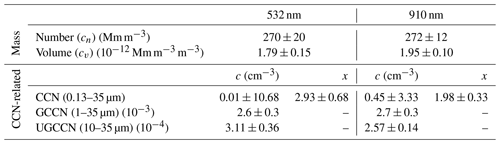
Regarding the c0.13–35 µm,birch, c1–35 µm,birch, and c10–35 µm,birch, as well as exponent x0.13–35 µm,birch, needed for the CCN, GCCN, and UGCCN concentration estimations, respectively, Fig. 6 presents the relationship between the birch extinction coefficient at 532 and 910 nm and the aerosol number concentration from 130 nm to 35 µm, from 1 to 35 µm and from 10 to 35 µm utilizing NS–OPS–ICEMET, OPS–ICEMET, and ICEMET observations, respectively. A total of 16 (19) cases were considered at 532 nm (910 nm), and the corresponding conversion factors are summarized in Table 1. Similar to the number and mass conversion factor estimation, cases where the MLH was lower than 400 m (200 m) for 532 nm (910 nm) are not considered. Also, cases with smoke or dust contribution are also excluded. In addition, for all the CCN-related conversion factors the number concentration was considered after subtracting the average number concentration of aerosols in the same size range over the measurement location (see Sect. 2.9). This was necessary since direct measurements of birch pollen fragments and other biological material and the distinction of them from the background aerosol population are not available.
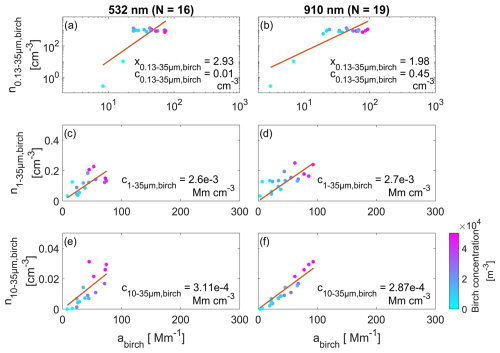
Figure 6Relationship between the birch extinction coefficient αbirch at 532 nm and the particle number concentration between (a) 0.13 and 35 µm, (c) 1 and 35 µm, and (e) 10 and 35 µm. Correlations are shown utilizing the mean birch extinction coefficient between 400–450 m a.g.l. Equivalent results at 910 nm between 200–250 m for the birch extinction coefficient are given in panels (b), (d), and (f). In panels (a) and (b) the regression analysis is applied to the log (n)−log (αbirch) data. The conversion factors c0.13–35 µm,birch, c1–35 µm,birch, c10–35 µm,birch, and x0.13–35 µm,birch indicate the intercept and slope of the regression, respectively, for each size range, and they are also summarized as numbers in each panel.
3.2.2 Lidar estimates of number, mass, and CCN-related profiles during a birch outbreak
The birch conversion factors in Table 1 were applied to 4 d lidar observations between 11 May 2021 at 08:00 LT and 15 May 2021 at 08:00 LT in which birch pollen peaked at Vehmasmäki station in Finland. Figure 7 summarizes the lidar-derived estimates of all microphysical properties and equivalent observations from in situ instrumentation (Fig. 7g–i).
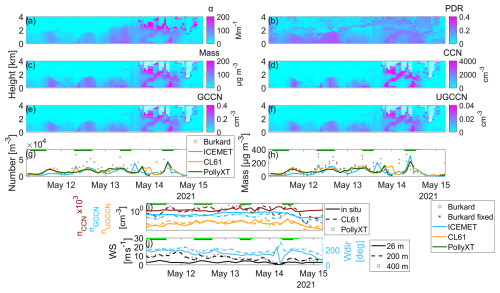
Figure 7Time series of optical and estimated microphysical aerosol properties between 11 May 2021 at 05:00 LT and 15 May 2021 at 05:00 LT at Vehmasmäki station in Finland. (a) Total particle extinction coefficient at 910 nm from CL61 ceilometer observations. (b) PDR at 910 nm. (c) Mass concentration estimated from 910 nm. (d) CCN concentration estimated from 910 nm. (e) GCCN concentration estimated from 910 nm. (f) UGCCN concentration estimated from 910 nm. (g) Comparison between in situ (Burkard and ICEMET) and lidar-estimated number concentrations. (h) Comparison between in situ (Burkard and ICEMET) mass concentrations at the surface and equivalent lidar-estimated mass concentrations. For the Burkard mass concentration estimation both the MVD from the ICEMET (circles) and a fixed birch pollen size of 22 µm (stars) are considered. For the CL61 ceilometer observations, a mean mass concentration between 200 and 250 m a.g.l. is considered. For the PollyXT lidar observations, a mean mass concentration between 400 and 450 m a.g.l. is considered. (i) CCN, GCCN, and UGCCN estimations from NS–OPS–ICEMET, OPS–ICEMET, and ICEMET at the surface and equivalent estimations from the PollyXT lidar (CL61) at 400–450 m a.g.l. (200–250 m a.g.l.) are shown, respectively. (j) Wind speed and direction at the surface (26 m) from the mast observations and at 200 and 400 m from the HALO Doppler lidar. The CL61 ceilometer data shown in panels (a)–(f) are retrieved with a 1 h temporal resolution, while the data in panels (g)–(j) have a 2 h temporal resolution. The times that the mixing-layer height was above the 400 m (200 m) height level are indicated by dark-green bars (light-green bars) in panels (g)–(j).
On 12 May 2021, the highest concentration of birch pollen was recorded by the Burkard sampler over Vehmasmäki station (64 380 particles m−3 at 10:00–12:00 LT) and the second highest concentration was recorded at the nearby Kuopio pollen monitoring site (62°8′ N, 27°63′ E; 98 m a.s.l.), which maintains a continuous 43-year-long airborne pollen monitoring dataset. As a reference, birch pollen concentrations of less than 6000 particles m−3 are observed on site 95 % of the time during pollen season. The lidar estimations of birch pollen concentration reveal that the number of pollen particles is greatest near the ground, decreasing as one moves upwards in a non-convective boundary layer. A mean (min–max) CCN concentration of 2500 (429–4741) cm−3 was estimated from the lidar observations at 910 nm during the day at 200 m a.g.l. with 0.20 (0.09–0.29) and 20.9 (9.2–30.9) × 10−3 cm−3 for the GCCN and UGCCN, respectively. During the nighttime of 13 May 2021 and midday of the next day, there is still a notable birch pollen load, but at the same time the BC concentration (0.13–0.22 µg m−3) and DOD (0.05 to 0.09) also rise, indicating a complex aerosol mixture. In turn, this complicates the decomposition of the lidar profiles since both dust and birch induce high PDR values. Nevertheless, Fig. 7g–i present the surface microphysical properties from in situ synergy and the Burkard sampler. Similar to Sect. 3.1, the lidars are capable of following the progress of the birch pollen season, even though the least amount of information is available at 200 m a.g.l. or higher. This is particularly valid for a convective boundary layer or when boundary layer processes during nighttime between the surface layer and the residual layer present minimal discrepancies. In fact, the smaller the wind speed difference between the surface and the elevated layer, the better the agreement between these two height levels. In turn this implies that, during unstable atmospheric conditions, higher discrepancies between the lidar-estimated and in situ estimated quantities are anticipated, due to the long temporal averaging of non-uniform aerosol layers together with the sensitivity of the specific wavelength to the aerosol particle size distribution.
So far, the most common method for sampling pollen particles has been point measurements at ground level using the Hirst-type collection technique. However, new in situ and remote sensing methods for pollen monitoring are beginning to emerge. The lack of a reliable calibration standard and the limitations of the Hirst-type collection method as a reference method make the assessment of the accuracy of other pollen monitoring instruments challenging. Consequently, aligning in situ and lidar observations with Burkard data is not an optimal approach and could lead to the propagation of errors. In this direction, Maya-Manzano et al. (2023) report an offset between all nine novel automatic pollen observational instruments with the classic Hirst-type observations. This was also found in this study when ICEMET observations were compared against the Hirst-type collector. Thus, a robust calibration standard needs to be developed to improve the reliability of airborne pollen monitoring.
The concentration of pollen is a critical parameter for aerosol models and health-related applications. Estimating pollen levels using lidar observations enhances the validation and assimilation efforts while providing timely information to the public about potential peaks of the pollen season. In this study, we have provided the means to estimate the number and mass concentration of birch pollen from lidar observations assuming that birch pollen particles reside in the 12–35 µm size range. For estimating the mass concentration, even if smaller coarse birch pollen particles or other biological material is present in the atmosphere (> 2.5 µm diameter), the uncertainty in cv at 910 nm is in the order of 5 % with a re-estimated cv of 1.90 ± 0.10, which is within the uncertainty range of the 12–35 µm size range. Assuming an AERONET-equivalent particle size range (1.2–30 µm diameter), a cv of 1.84 ± 0.08 is obtained, which presents a 6 % discrepancy from the 12–35 µm size range. In comparison, when using the AERONET method (Level 1.5), a cv of 1.24 ± 0.06 is estimated. This is not the case for the number concentration estimation in which the inclusion of aerosol particles above 2.5 µm leads to a cn factor that is an order of magnitude higher than the 12–35 µm size range, with the relationship between the volume concentration and extinction no longer being linear.
In this study, the particle extinction coefficient was estimated by multiplying the particle backscatter coefficient with an LR of 60 sr for both wavelengths. The value of the LR is a mean statistical value at 532 nm estimated from Raman observations for mixtures of birch and background aerosols with an unknown relative contribution and little to no wavelength dependence between 355 and 532 nm wavelengths (Bohlmann et al., 2019; Shang et al., 2020). To this end, no LR has been reported for the 910 nm wavelength. To account for both the LR uncertainty at 532 nm due to the birch share in the aerosol mixture in previous studies and a possible wavelength dependence between 532 and 910 nm wavelengths, a sensitivity study due to the selection of LRs to the conversion factors has been added (Tables A1 and A2). It is apparent that an inappropriate selection of the LR can significantly influence the conversion factors, consequently affecting the accuracy of derived microphysical properties.
This is the first time that lidar observations have been used to estimate the number concentration of potential CCN, GCCN, and UGCCN of birch pollen. The CCN parameterization was restricted to supersaturation below 0.2 %, but smaller particles can be activated at higher supersaturation, resulting in a higher number concentration of potential CCN (Mikhailov et al., 2021). We have also assumed that the elevated CCN concentrations during the birch pollen period are caused by the presence of SPPs and other biological material, but with the current instrumental setup we cannot confirm the presence of submicron birch pollen. Essentially, the capacity of aerosol particles to act as CCN depends on their size, chemical composition, hygroscopicity, morphology, and the supersaturation at the cloud layer, which in turn is influenced by updraft velocities. Previously, laboratory and model-based studies have confirmed the CCN and GCCN activity of birch pollen (Pope, 2010; Griffiths et al., 2012; Steiner et al., 2015). In Wozniak et al. (2018), given a high enough number concentration of pollen fragments, a 32 % suppression in precipitation in clean continental aerosol conditions was foreseen. Nevertheless, the presence of pollen fragments in the atmosphere is not monitored. Under high relative humidity, pollen particles rapture, but there are not yet atmospheric observations to enumerate the frequency, concentration, and size distribution of these fragments. Here, we presume the existence of SPPs by comparing two periods, one with birch pollen and another one without it, but other biological particles co-exist in the aerosol mixture. Nevertheless, the lidar-estimated and in situ estimated birch CCN-related concentrations during the peak birch pollen season indicate a potential source of CCN for atmospheric cloud processes which is not currently being considered.
Although there are no atmospheric studies of birch pollen CCN-related concentration, there is a plethora of studies for other aerosol particles. In this context, CCN concentrations in a central European city ranged from 160 to 3600 cm−3, with an average of 820 cm−3 (Burkart et al., 2011). Enhancement in CCN concentration was seen in coastal southeastern Florida when biomass burning aerosol particles were present in the atmosphere (1408 ± 976 cm−3 at ss = 0.2 %) (Edwards et al., 2021). In a boreal forest, measured concentrations in the order of 102–103 cm−3 were found at an ss of 0.2 % (Sihto et al., 2011), with elevated concentrations anticipated during a fire episode (Kommula et al., 2024).
Regarding GCCN and UGCCN, giant sea salt particles with a radius larger than 5 µm are reported in concentrations of 10−4–10−2 cm−3 in Feingold et al. (1999). Higher concentrations were reported when stronger winds prevail (Smith et al., 1989), while Gonzalez et al. (2022) reports a concentration of sea salt particles above 1 µm to be in the order of 10−1 cm−3. Similar to a marine boundary layer in which the 25 µm giant sea salt particles are well mixed in the surface layer (Lewis and Schwartz, 2004), birch pollen can be similarly well mixed and influenced by turbulence and convection, yet under stable boundary layer concentrations it may be diminished due to gravitational settling. Nevertheless, modeling and field studies have shown that pollen has the capacity to travel long distances and remain aloft for days. The birch GCCN and UGCCN concentration estimated at 200 m a.g.l. in this paper is 0.20 (0.09–0.29) and 20.9 (9.2–30.9) × 10−3 cm−3 on 12 May 2021, respectively. On this day BC, dust, and volcanic intrusions were marginal; thus birch pollen can also be cloud relevant (in the order of 10−3 cm−3) in atmospheric conditions and therefore be able to affect cloud precipitation efficiency (Cotton and Yuter, 2009). Although this case is exceptional, at other times cloud-relevant concentrations could be achieved by adding up other pollen species together.
We expanded the applicability of polarization lidars to assess the microphysical properties of birch pollen utilizing a synergy of aerosol size distributions from novel in situ instrumentation. In line with the POLIPHON method, it permits the profiling of birch number and mass concentrations as well as estimates of CCN, GCCN, and UGCCN concentrations from single-wavelength backscatter polarization lidar observations at 532 and 910 nm. The pivotal conversion factors required to convert the optical into microphysical properties in the POLIPHON method derived from a synergy of the NS, OPS, and ICEMET in situ observations which provided aerosol size distributions from 10 nm to 200 µm. Typically, conversion factors are obtained using AERONET climatologies. However, AERONET inversion products account for aerosol particle sizes up to 30 µm in diameter. To accurately account for pollen, it is essential to include larger aerosol particle sizes. The novel approach can be used as an alternative method to derive the conversion factors of other large aerosol particles, for example, volcanic ash particles and larger pollen types.
By selecting cases with a well-mixed boundary layer, surface measurements from in situ instrumentation were correlated against lidar observations at higher altitudes to determine the conversion factors. Although a linear relationship was observed across both wavelengths, the best agreement was seen at 910 nm. This was attributed to the lower overlap region and the higher sensitivity of this longer wavelength in detecting large aerosol particles. We should note that birch pollen grains, SPPs, and other biological material all co-exist in the bioaerosol mixture without being able to distinguish their individual optical effect with the current instrumental setup. Therefore, efforts should be made to characterize this effect, if any. Moreover, for the derivation of the conversion factors an LR is presumed. To this end, the actual LR wavelength dependence of birch pollen is not known, and in this study we have tackled the issue by estimating the conversion factors for a range of LRs. Also, the conversion parameters of other pollen particles having their optical properties characterized first remain to be investigated in detail.
Then, the microphysical properties of birch pollen were investigated using observations from a PollyXT lidar and a Vaisala CL61 ceilometer with polarization capability at Vehmasmäki, a rural site in eastern Finland. The novel pollen retrieval technique developed holds particular significance for ground-based lidar networks such as that of a ceilometer and spaceborne lidars featuring polarization capability permitting the characterization of pollen microphysical and optical properties. In this way, point measurements at ground level providing limited information to forecasting models, as well as health-related applications, can be broadened both in space and time utilizing the lidar technique.
Level 1 CL61 ceilometer and HALO Doppler data between 11 and 15 May 2021 are available via the Cloudnet portal (https://hdl.handle.net/21.12132/2.d51fdac9e1114762, Komppula and O'Connor, 2023). PollyXT Level 1 and Level 2 observations are visualized at https://polly.tropos.de/ (last access: 3 February 2025) and are available upon request. Level 3 data are available upon request.
MF conceptualized the original paper, analyzed the PollyXT and CL61 ceilometer and FD12P data, and performed the analysis considering all data sources. AL and PT were responsible for and provided the in situ ICEMET aerosol data. AL provided the AE, NS, and OPS data. VV provided the HALO Doppler data. AS, PA, and SP analyzed the pollen samples and provided the pollen data. MF, AL, PT, XS, UI, and MK were responsible for the lidars and ensured good in situ operation. They also prepared the pollen samples during the pollen campaigns. MF prepared the manuscript with contributions from all co-authors. All authors were involved in editing the paper, interpreting the results, and the discussion of the manuscript.
The contact author has declared that none of the authors has any competing interests.
Publisher's note: Copernicus Publications remains neutral with regard to jurisdictional claims made in the text, published maps, institutional affiliations, or any other geographical representation in this paper. While Copernicus Publications makes every effort to include appropriate place names, the final responsibility lies with the authors.
The authors gratefully acknowledge the support of the Finnish Research Impact Foundation through the Tandem Industry Academia (TIA) program. Dust data and/or images were provided by the WMO Barcelona Dust Regional Center and the partners of the Sand and Dust Storm Warning Advisory and Assessment System (SDS-WAS) for Northern Africa, the Middle East, and Europe. We acknowledge the Aerosol, Clouds and Trace Gases Research Infrastructure (ACTRIS) for providing the dataset used in this study; the dataset was produced by the Finnish Meteorological Institute and is available on https://cloudnet.fmi.fi/ (last access: 15 September 2024).
This research has been supported by the Finnish Research Impact Foundation through the Tandem Industry Academia (TIA) program.
This paper was edited by Matthias Tesche and reviewed by three anonymous referees.
Adamov, S., Lemonis, N., Clot, B., Crouzy, B., Gehrig, R., Graber, M., Sallin, C., and Tummon, F.: On the measurement uncertainty of Hirst-type volumetric pollen and spore samplers, Aerobiologia, 40, 77–91, https://doi.org/10.1007/s10453-021-09724-5, 2021. a
Alas, H. D. C., Weinhold, K., Costabile, F., Di Ianni, A., Müller, T., Pfeifer, S., Di Liberto, L., Turner, J. R., and Wiedensohler, A.: Methodology for high-quality mobile measurement with focus on black carbon and particle mass concentrations, Atmos. Meas. Tech., 12, 4697–4712, https://doi.org/10.5194/amt-12-4697-2019, 2019. a
Ansmann, A., Riebesell, M., Wandinger, U., Weitkamp, C., Voss, E., W., L., and Michaelis, W.: Combined raman elastic-backscatter LIDAR for vertical profiling of moisture, aerosol extinction, backscatter, and LIDAR ratio, Appl. Phys. B, 55, 18–20, https://doi.org/10.1007/BF00348608, 1992. a
Ansmann, A., Tesche, M., Seifert, P., Groß, S., Freudenthaler, V., Apituley, A., Wilson, K. M., Serikov, I., Linné, H., Heinold, B., Hiebsch, A., Schnell, F., Schmidt, J., Mattis, I., Wandinger, U., and Wiegner, M.: Ash and fine-mode particle mass profiles from EARLINET-AERONET observations over central Europe after the eruptions of the Eyjafjallajökull volcano in 2010, J. Geophys. Res.-Atmos., 116, D00U02, https://doi.org/10.1029/2010JD015567, 2011. a
Ansmann, A., Seifert, P., Tesche, M., and Wandinger, U.: Profiling of fine and coarse particle mass: case studies of Saharan dust and Eyjafjallajökull/Grimsvötn volcanic plumes, Atmos. Chem. Phys., 12, 9399–9415, https://doi.org/10.5194/acp-12-9399-2012, 2012. a, b
Ansmann, A., Mamouri, R.-E., Hofer, J., Baars, H., Althausen, D., and Abdullaev, S. F.: Dust mass, cloud condensation nuclei, and ice-nucleating particle profiling with polarization lidar: updated POLIPHON conversion factors from global AERONET analysis, Atmos. Meas. Tech., 12, 4849–4865, https://doi.org/10.5194/amt-12-4849-2019, 2019. a, b
Baars, H., Kanitz, T., Engelmann, R., Althausen, D., Heese, B., Komppula, M., Preißler, J., Tesche, M., Ansmann, A., Wandinger, U., Lim, J.-H., Ahn, J. Y., Stachlewska, I. S., Amiridis, V., Marinou, E., Seifert, P., Hofer, J., Skupin, A., Schneider, F., Bohlmann, S., Foth, A., Bley, S., Pfüller, A., Giannakaki, E., Lihavainen, H., Viisanen, Y., Hooda, R. K., Pereira, S. N., Bortoli, D., Wagner, F., Mattis, I., Janicka, L., Markowicz, K. M., Achtert, P., Artaxo, P., Pauliquevis, T., Souza, R. A. F., Sharma, V. P., van Zyl, P. G., Beukes, J. P., Sun, J., Rohwer, E. G., Deng, R., Mamouri, R.-E., and Zamorano, F.: An overview of the first decade of PollyNET: an emerging network of automated Raman-polarization lidars for continuous aerosol profiling, Atmos. Chem. Phys., 16, 5111–5137, https://doi.org/10.5194/acp-16-5111-2016, 2016. a, b, c
Biedermann, T., Winther, L., Till, S. J., Panzner, P., Knulst, A., and Valovirta, E.: Birch pollen allergy in Europe, Allergy, 74, 1237–1248, https://doi.org/10.1111/all.13758, 2019. a
Bohlmann, S., Shang, X., Giannakaki, E., Filioglou, M., Saarto, A., Romakkaniemi, S., and Komppula, M.: Detection and characterization of birch pollen in the atmosphere using a multiwavelength Raman polarization lidar and Hirst-type pollen sampler in Finland, Atmos. Chem. Phys., 19, 14559–14569, https://doi.org/10.5194/acp-19-14559-2019, 2019. a, b, c, d, e, f, g
Bohlmann, S., Shang, X., Vakkari, V., Giannakaki, E., Leskinen, A., Lehtinen, K. E. J., Pätsi, S., and Komppula, M.: Lidar depolarization ratio of atmospheric pollen at multiple wavelengths, Atmos. Chem. Phys., 21, 7083–7097, https://doi.org/10.5194/acp-21-7083-2021, 2021. a
Browning, K. A. and Wexler, R.: The Determination of Kinematic Properties of a Wind Field Using Doppler Radar, J. Appl. Meteorol. Clim., 7, 105–113, https://doi.org/10.1175/1520-0450(1968)007<0105:TDOKPO>2.0.CO;2, 1968. a
Burkart, J., Steiner, G., Reischl, G., and Hitzenberger, R.: Long-term study of cloud condensation nuclei (CCN) activation of the atmospheric aerosol in Vienna, Atmos. Environ., 45, 5751–5759, https://doi.org/10.1016/j.atmosenv.2011.07.022, 2011. a
Burkart, J., Gratzl, J., Seifried, T. M., Bieber, P., and Grothe, H.: Isolation of subpollen particles (SPPs) of birch: SPPs are potential carriers of ice nucleating macromolecules, Biogeosciences, 18, 5751–5765, https://doi.org/10.5194/bg-18-5751-2021, 2021. a
Buters, J., Clot, B., Galán, C., Gehrig, R., Gilge, S., Hentges, F., O'Connor, D., Sikoparija, B., Skjoth, C., Tummon, F., Adams-Groom, B., Antunes, C. M., Bruffaerts, N., Çelenk, S., Crouzy, B., Guillaud, G., Hajkova, L., Seliger, A. K., Oliver, G., Ribeiro, H., Rodinkova, V., Saarto, A., Sauliene, I., Sozinova, O., and Stjepanovic, B.: Automatic detection of airborne pollen: an overview, Aerobiologia, 40, 13–37, https://doi.org/10.1007/s10453-022-09750-x, 2024. a
Buters, J. T., Thibaudon, M., Smith, M., Kennedy, R., Rantio-Lehtimäki, A., Albertini, R., Reese, G., Weber, B., Galan, C., Brandao, R., Antunes, C. M., Jäger, S., Berger, U., Celenk, S., Grewling, L., Jackowiak, B., Sauliene, I., Weichenmeier, I., Pusch, G., Sarioglu, H., Ueffing, M., Behrendt, H., Prank, M., Sofiev, M., and Cecchi, L.: Release of Bet v 1 from birch pollen from 5 European countries. Results from the HIALINE study, Atmos. Environ., 55, 496–505, https://doi.org/10.1016/j.atmosenv.2012.01.054, 2012. a
Buters, J. T. M., Antunes, C., Galveias, A., Bergmann, K. C., Thibaudon, M., Galán, C., Schmidt-Weber, C., and Oteros, J.: Pollen and spore monitoring in the world, Clinical and Translational Allergy, 8, 9, https://doi.org/10.1186/s13601-018-0197-8, 2018. a
Cao, X., Roy, G. A., and Bernier, R.: Lidar polarization discrimination of bioaerosols, Opt. Eng., 49, 116201, https://doi.org/10.1117/1.3505877, 2010. a
Cholleton, D., Bialic, É., Dumas, A., Kaluzny, P., Rairoux, P., and Miffre, A.: Laboratory evaluation of the scattering matrix of ragweed, ash, birch and pine pollen towards pollen classification, Atmos. Meas. Tech., 15, 1021–1032, https://doi.org/10.5194/amt-15-1021-2022, 2022a. a
Cholleton, D., Rairoux, P., and Miffre, A.: Laboratory Evaluation of the (355, 532) nm Particle Depolarization Ratio of Pure Pollen at 180.0° Lidar Backscattering Angle, Remote Sens., 14, 3767, https://doi.org/10.3390/rs14153767, 2022b. a
Cotton, W. R. and Yuter, S.: Principles of Cloud and Precipitation Formation, Springer Netherlands, Dordrecht, ISBN 978-1-4020-8690-8, 13–43, https://doi.org/10.1007/978-1-4020-8690-8_2, 2009. a
D'Amato, G., Cecchi, L., Bonini, S., Nunes, C., Annesi-Maesano, I., Behrendt, H., Liccardi, G., Popov, T., and Van Cauwenberge, P.: Allergenic pollen and pollen allergy in Europe, Allergy, 62, 976–990, https://doi.org/10.1111/j.1398-9995.2007.01393.x, 2007. a
Diehl, K., Quick, C., Matthias-Maser, S., Mitra, S., and Jaenicke, R.: The ice nucleating ability of pollen: Part I: Laboratory studies in deposition and condensation freezing modes, Atmos. Res., 58, 75–87, https://doi.org/10.1016/S0169-8095(01)00091-6, 2001. a
Diehl, K., Matthias-Maser, S., Jaenicke, R., and Mitra, S.: The ice nucleating ability of pollen: Part II. Laboratory studies in immersion and contact freezing modes, Atmos. Res., 61, 125–133, https://doi.org/10.1016/S0169-8095(01)00132-6, 2002. a
Dreischmeier, K., Budke, C., Wiehemeier, L., Kottke, T., and Koop, T.: Boreal pollen contain ice-nucleating as well as ice-binding “antifreeze” polysaccharides, Sci. Rep., 7, 41890, https://doi.org/10.1038/srep41890, 2017. a
Edwards, E.-L., Corral, A. F., Dadashazar, H., Barkley, A. E., Gaston, C. J., Zuidema, P., and Sorooshian, A.: Impact of various air mass types on cloud condensation nuclei concentrations along coastal southeast Florida, Atmos. Environ., 254, 118371, https://doi.org/10.1016/j.atmosenv.2021.118371, 2021. a
Engelmann, R., Kanitz, T., Baars, H., Heese, B., Althausen, D., Skupin, A., Wandinger, U., Komppula, M., Stachlewska, I. S., Amiridis, V., Marinou, E., Mattis, I., Linné, H., and Ansmann, A.: The automated multiwavelength Raman polarization and water-vapor lidar PollyXT: the neXT generation, Atmos. Meas. Tech., 9, 1767–1784, https://doi.org/10.5194/amt-9-1767-2016, 2016. a, b, c
Feingold, G., Cotton, W. R., Kreidenweis, S. M., and Davis, J. T.: The Impact of Giant Cloud Condensation Nuclei on Drizzle Formation in Stratocumulus: Implications for Cloud Radiative Properties, J. Atmos. Sci., 56, 4100–4117, https://doi.org/10.1175/1520-0469(1999)056<4100:TIOGCC>2.0.CO;2, 1999. a
Filioglou, M., Nikandrova, A., Niemelä, S., Baars, H., Mielonen, T., Leskinen, A., Brus, D., Romakkaniemi, S., Giannakaki, E., and Komppula, M.: Profiling water vapor mixing ratios in Finland by means of a Raman lidar, a satellite and a model, Atmos. Meas. Tech., 10, 4303–4316, https://doi.org/10.5194/amt-10-4303-2017, 2017. a
Filioglou, M., Leskinen, A., Vakkari, V., O'Connor, E., Tuononen, M., Tuominen, P., Laukkanen, S., Toiviainen, L., Saarto, A., Shang, X., Tiitta, P., and Komppula, M.: Spectral dependence of birch and pine pollen optical properties using a synergy of lidar instruments, Atmos. Chem. Phys., 23, 9009–9021, https://doi.org/10.5194/acp-23-9009-2023, 2023. a, b, c, d, e, f
Galveias, A., Antunes, C., Costa, A. R., and Fraga, H.: Pollen- and Weather-Based Machine Learning Models for Estimating Regional Olive Production, Horticulturae, 10, 584, https://doi.org/10.3390/horticulturae10060584, 2024. a
Geroldinger-Simic, M., Zelniker, T., Aberer, W., Ebner, C., Egger, C., Greiderer, A., Prem, N., Lidholm, J., Ballmer-Weber, B. K., Vieths, S., and Bohle, B.: Birch pollen–related food allergy: Clinical aspects and the role of allergen-specific IgE and IgG4 antibodies, J. Allergy Clin. Immun., 127, 616–622, https://doi.org/10.1016/j.jaci.2010.10.027, 2011. a
Gonzalez, M. E., Corral, A. F., Crosbie, E., Dadashazar, H., Diskin, G. S., Edwards, E.-L., Kirschler, S., Moore, R. H., Robinson, C. E., Schlosser, J. S., Shook, M., Stahl, C., Thornhill, K. L., Voigt, C., Winstead, E., Ziemba, L. D., and Sorooshian, A.: Relationships between supermicrometer particle concentrations and cloud water sea salt and dust concentrations: analysis of MONARC and ACTIVATE data, Environmental Science: Atmospheres, 2, 738–752, https://doi.org/10.1039/D2EA00049K, 2022. a
Gregory, P. H.: The microbiology of the atmosphere, Leonard Hill, London, https://www.biodiversitylibrary.org/item/31014 (last access: 10 November 2024), 1961. a
Griffiths, P. T., Borlace, J.-S., Gallimore, P. J., Kalberer, M., Herzog, M., and Pope, F. D.: Hygroscopic growth and cloud activation of pollen: a laboratory and modelling study, Atmos. Sci. Lett., 13, 289–295, https://doi.org/10.1002/asl.397, 2012. a, b
Gute, E., David, R. O., Kanji, Z. A., and Abbatt, J. P. D.: Ice Nucleation Ability of Tree Pollen Altered by Atmospheric Processing, ACS Earth and Space Chemistry, 4, 2312–2319, https://doi.org/10.1021/acsearthspacechem.0c00218, 2020. a
Hader, J. D., Wright, T. P., and Petters, M. D.: Contribution of pollen to atmospheric ice nuclei concentrations, Atmos. Chem. Phys., 14, 5433–5449, https://doi.org/10.5194/acp-14-5433-2014, 2014. a
He, Y., Yin, Z., Ansmann, A., Liu, F., Wang, L., Jing, D., and Shen, H.: POLIPHON conversion factors for retrieving dust-related cloud condensation nuclei and ice-nucleating particle concentration profiles at oceanic sites, Atmos. Meas. Tech., 16, 1951–1970, https://doi.org/10.5194/amt-16-1951-2023, 2023. a
Hirsikko, A., O'Connor, E. J., Komppula, M., Korhonen, K., Pfüller, A., Giannakaki, E., Wood, C. R., Bauer-Pfundstein, M., Poikonen, A., Karppinen, T., Lonka, H., Kurri, M., Heinonen, J., Moisseev, D., Asmi, E., Aaltonen, V., Nordbo, A., Rodriguez, E., Lihavainen, H., Laaksonen, A., Lehtinen, K. E. J., Laurila, T., Petäjä, T., Kulmala, M., and Viisanen, Y.: Observing wind, aerosol particles, cloud and precipitation: Finland's new ground-based remote-sensing network, Atmos. Meas. Tech., 7, 1351–1375, https://doi.org/10.5194/amt-7-1351-2014, 2014. a
Hirst, J. M.: An automatic volumetric spore trap, Ann. Appl. Biol., 39, 257–265, https://doi.org/10.1111/j.1744-7348.1952.tb00904.x, 1952. a, b
Hopkin, E., Illingworth, A. J., Charlton-Perez, C., Westbrook, C. D., and Ballard, S.: A robust automated technique for operational calibration of ceilometers using the integrated backscatter from totally attenuating liquid clouds, Atmos. Meas. Tech., 12, 4131–4147, https://doi.org/10.5194/amt-12-4131-2019, 2019. a
Huffman, J., E., A., Perring, N. J., Clot, S. B., Crouzy, B., Tummon, F., Shoshanim, O., Damit, B., Schneider, J., Sivaprakasam, V., Zawadowicz, M. A., Crawford, I., Gallagher, M., Topping, D., Doughty, D. C., Hill, S. C., and Pan, Y.: Real-time sensing of bioaerosols: Review and current perspectives, Aerosol Sci. Tech., 54, 465–495, https://doi.org/10.1080/02786826.2019.1664724, 2020. a
Kaikkonen, V., Molkoselkä, E., and Mäkynen, A.: A rotating holographic imager for stationary cloud droplet and ice crystal measurements, Opt. Rev., 27, 205–216, https://doi.org/10.1007/s10043-020-00583-y, 2020. a
Klett, J. D.: Stable analytical inversion solution for processing lidar returns, Appl. Optics, 20, 211–220, https://doi.org/10.1364/AO.20.000211, 1981. a
Kommula, S. M., Buchholz, A., Gramlich, Y., Mielonen, T., Hao, L., Pullinen, I., Vettikkat, L., Ylisirniö, A., Joutsensaari, J., Schobesberger, S., Tiitta, P., Leskinen, A., Rees, D. H., Haslett, S. L., Siegel, K., Lunder, C., Zieger, P., Krejci, R., Romakkaniemi, S., Mohr, C., and Virtanen, A.: Effect of Long-Range Transported Fire Aerosols on Cloud Condensation Nuclei Concentrations and Cloud Properties at High Latitudes, Geophys. Res. Lett., 51, e2023GL107134, https://doi.org/10.1029/2023GL107134, 2024. a
Komppula, M. and O'Connor, E.: Custom collection of Doppler lidar, and lidar data from Vehmasmäki between 1 Apr and 30 Jun 2022, ACTRIS Cloud remote sensing data centre unit (CLU) [data set], https://hdl.handle.net/21.12132/2.d51fdac9e1114762 (last access: 15 August 2024), 2023. a
Lake, I., Jones, N., Agnew, M., Goodess, C., Giorgi, F., Hamaoui-Laguel, L., Semenov, M., Solmon, F., Storkey, J., Vautard, R., and Epstein, M.: Climate Change and Future Pollen Allergy in Europe, Environ Health Persp., 1, 385–391, https://doi.org/10.1289/EHP173, 2018. a
Leskinen, A., Ruuskanen, A., Kolmonen, P., Zhao, Y., Fang, D., Wang, Q., Gu, C., Jokiniemi, J., Hirvonen, M.-R., Lehtinen, K., Romakkaniemi, S., and Komppula, M.: The contribution of black carbon and non-BC absorbers to the aerosol absorption coefficient in Nanjing, China, Aerosol Air Qual. Res., 20, 590–605, https://doi.org/10.4209/aaqr.2019.06.0326, 2020. a, b
Lewis, E. R. and Schwartz, S. E.: Sea Salt Aerosol Production Fluxes: Estimates and Critical Analysis, chap. 5, pp. 299–344, American Geophysical Union (AGU), ISBN 9781118666050, https://agupubs.onlinelibrary.wiley.com/doi/book/10.1029/GM152 (last access: 3 February 2025), 2004. a
Mäkinen, Y.: Random sampling in the study of microscopic slides, Repsitory Of Aerobiology Laboratory, University Of Turku, 5, 27–43, 1981. a, b
Mamouri, R. E. and Ansmann, A.: Estimated desert-dust ice nuclei profiles from polarization lidar: methodology and case studies, Atmos. Chem. Phys., 15, 3463–3477, https://doi.org/10.5194/acp-15-3463-2015, 2015. a
Mamouri, R.-E. and Ansmann, A.: Potential of polarization lidar to provide profiles of CCN- and INP-relevant aerosol parameters, Atmos. Chem. Phys., 16, 5905–5931, https://doi.org/10.5194/acp-16-5905-2016, 2016. a, b, c
Mamouri, R.-E. and Ansmann, A.: Potential of polarization/Raman lidar to separate fine dust, coarse dust, maritime, and anthropogenic aerosol profiles, Atmos. Meas. Tech., 10, 3403–3427, https://doi.org/10.5194/amt-10-3403-2017, 2017. a
Maya-Manzano, J. M., Tummon, F., Abt, R., Allan, N., Bunderson, L., Clot, B., Crouzy, B., Daunys, G., Erb, S., Gonzalez-Alonso, M., Graf, E., Grewling, L., Haus, J., Kadantsev, E., Kawashima, S., Martinez-Bracero, M., Matavulj, P., Mills, S., Niederberger, E., Lieberherr, G., Lucas, R. W., O'Connor, D. J., Oteros, J., Palamarchuk, J., Pope, F. D., Rojo, J., Šaulienė, I., Schäfer, S., Schmidt-Weber, C. B., Schnitzler, M., Šikoparija, B., Skjøth, C. A., Sofiev, M., Stemmler, T., Triviño, M., Zeder, Y., and Buters, J.: Towards European automatic bioaerosol monitoring: Comparison of 9 automatic pollen observational instruments with classic Hirst-type traps, Sci. Total Environ., 866, 161220, https://doi.org/10.1016/j.scitotenv.2022.161220, 2023. a, b
Mikhailov, E., Ivanova, O., Nebosko, E., Vlasenko, S., and Ryshkevich, T. I.: Subpollen Particles as Atmospheric Cloud Condensation Nuclei, Izv. Atmos. Ocean. Phy.+, 55, 357–364, https://doi.org/10.1134/S000143381904008X, 2019. a
Mikhailov, E. F., Pöhlker, M. L., Reinmuth-Selzle, K., Vlasenko, S. S., Krüger, O. O., Fröhlich-Nowoisky, J., Pöhlker, C., Ivanova, O. A., Kiselev, A. A., Kremper, L. A., and Pöschl, U.: Water uptake of subpollen aerosol particles: hygroscopic growth, cloud condensation nuclei activation, and liquid–liquid phase separation, Atmos. Chem. Phys., 21, 6999–7022, https://doi.org/10.5194/acp-21-6999-2021, 2021. a, b, c
Molkoselkä, E. O.: ICEMET Server: Digital hologram processing and cloud droplet analysis software for ICEMET project, GitHub, https://github.com/molkoback/icemet-server (last access: 20 August 2024), 2020. a
Nicolae, D., Vasilescu, J., Talianu, C., Binietoglou, I., Nicolae, V., Andrei, S., and Antonescu, B.: A neural network aerosol-typing algorithm based on lidar data, Atmos. Chem. Phys., 18, 14511–14537, https://doi.org/10.5194/acp-18-14511-2018, 2018. a
Noh, Y. M., Lee, H., Mueller, D., Lee, K., Shin, D., Shin, S., Choi, T. J., Choi, Y. J., and Kim, K. R.: Investigation of the diurnal pattern of the vertical distribution of pollen in the lower troposphere using LIDAR, Atmos. Chem. Phys., 13, 7619–7629, https://doi.org/10.5194/acp-13-7619-2013, 2013. a
O'Connor, E. J., Illingworth, A. J., and Hogan, R. J.: A Technique for Autocalibration of Cloud Lidar, J. Atmos. Ocean. Tech., 21, 777–786, https://doi.org/10.1175/1520-0426(2004)021<0777:ATFAOC>2.0.CO;2, 2004. a
O'Connor, E. J., Illingworth, A. J., Brooks, I. M., Westbrook, C. D., Hogan, R. J., Davies, F., and Brooks, B. J.: A Method for Estimating the Turbulent Kinetic Energy Dissipation Rate from a Vertically Pointing Doppler Lidar, and Independent Evaluation from Balloon-Borne In Situ Measurements, J. Atmos. Ocean. Tech., 27, 1652–1664, https://doi.org/10.1175/2010JTECHA1455.1, 2010. a
Pearson, G., Davies, F., and Collier, C.: An Analysis of the Performance of the UFAM Pulsed Doppler Lidar for Observing the Boundary Layer, J. Atmos. Ocean. Tech., 26, 240–250, https://doi.org/10.1175/2008JTECHA1128.1, 2009. a, b
Pfaar, O., Bastl, K., Berger, U., Buters, J., Calderon, M. A., Clot, B., Darsow, U., Demoly, P., Durham, S. R., Galán, C., Gehrig, R., Gerth van Wijk, R., Jacobsen, L., Klimek, L., Sofiev, M., Thibaudon, M., and Bergmann, K. C.: Defining pollen exposure times for clinical trials of allergen immunotherapy for pollen-induced rhinoconjunctivitis – an EAACI position paper, Allergy, 72, 713–722, https://doi.org/10.1111/all.13092, 2017. a
Pope, F.: Pollen grains are efficient cloud condensation nuclei, Environ. Res. Lett., 5, 044015, https://doi.org/10.1088/1748-9326/5/4/044015, 2010. a, b
Portin, H., Leskinen, A., Hao, L., Kortelainen, A., Miettinen, P., Jaatinen, A., Laaksonen, A., Lehtinen, K. E. J., Romakkaniemi, S., and Komppula, M.: The effect of local sources on particle size and chemical composition and their role in aerosol–cloud interactions at Puijo measurement station, Atmos. Chem. Phys., 14, 6021–6034, https://doi.org/10.5194/acp-14-6021-2014, 2014. a
Prisle, N. L., Lin, J. J., Purdue, S., Lin, H., Meredith, J. C., and Nenes, A.: Cloud condensation nuclei activity of six pollenkitts and the influence of their surface activity, Atmos. Chem. Phys., 19, 4741–4761, https://doi.org/10.5194/acp-19-4741-2019, 2019. a
Pummer, B. G., Bauer, H., Bernardi, J., Bleicher, S., and Grothe, H.: Suspendable macromolecules are responsible for ice nucleation activity of birch and conifer pollen, Atmos. Chem. Phys., 12, 2541–2550, https://doi.org/10.5194/acp-12-2541-2012, 2012. a
Raith, M. and Swoboda, I.: Birch pollen: The unpleasant herald of spring, Front. Allergy, 4, 181675, https://doi.org/10.3389/falgy.2023.1181675, 2023. a, b
Sassen, K.: Boreal tree pollen sensed by polarization lidar: Depolarizing biogenic chaff, Geophys. Res. Lett., 35, L18810, https://doi.org/10.1029/2008GL035085, 2008. a
Shang, X., Giannakaki, E., Bohlmann, S., Filioglou, M., Saarto, A., Ruuskanen, A., Leskinen, A., Romakkaniemi, S., and Komppula, M.: Optical characterization of pure pollen types using a multi-wavelength Raman polarization lidar, Atmos. Chem. Phys., 20, 15323–15339, https://doi.org/10.5194/acp-20-15323-2020, 2020. a, b, c, d, e, f
Shang, X., Baars, H., Stachlewska, I. S., Mattis, I., and Komppula, M.: Pollen observations at four EARLINET stations during the ACTRIS-COVID-19 campaign, Atmos. Chem. Phys., 22, 3931–3944, https://doi.org/10.5194/acp-22-3931-2022, 2022. a, b, c, d
Shinozuka, Y., Clarke, A. D., Nenes, A., Jefferson, A., Wood, R., McNaughton, C. S., Ström, J., Tunved, P., Redemann, J., Thornhill, K. L., Moore, R. H., Lathem, T. L., Lin, J. J., and Yoon, Y. J.: The relationship between cloud condensation nuclei (CCN) concentration and light extinction of dried particles: indications of underlying aerosol processes and implications for satellite-based CCN estimates, Atmos. Chem. Phys., 15, 7585–7604, https://doi.org/10.5194/acp-15-7585-2015, 2015. a
Sicard, M., Izquierdo, R., Alarcón, M., Belmonte, J., Comerón, A., and Baldasano, J. M.: Near-surface and columnar measurements with a micro pulse lidar of atmospheric pollen in Barcelona, Spain, Atmos. Chem. Phys., 16, 6805–6821, https://doi.org/10.5194/acp-16-6805-2016, 2016. a
Sihto, S.-L., Mikkilä, J., Vanhanen, J., Ehn, M., Liao, L., Lehtipalo, K., Aalto, P. P., Duplissy, J., Petäjä, T., Kerminen, V.-M., Boy, M., and Kulmala, M.: Seasonal variation of CCN concentrations and aerosol activation properties in boreal forest, Atmos. Chem. Phys., 11, 13269–13285, https://doi.org/10.5194/acp-11-13269-2011, 2011. a
Smith, M. H., Consterdine, I. E., and Park, P. M.: Atmospheric loadings of marine aerosol during a Hebridean cyclone, Q. J. Roy. Meteor. Soc., 115, 383–395, https://doi.org/10.1002/qj.49711548610, 1989. a
Sofiev, M., Siljamo, P., Ranta, H., Linkosalo, T., Jaeger, S., Rasmussen, A., Rantio-Lehtimaki, A., Severova, E., and Kukkonen, J.: A numerical model of birch pollen emission and dispersion in the atmosphere. Description of the emission module, Int. J. Biometeorol., 57, 45–58, https://doi.org/10.1007/s00484-012-0532-z, 2013. a
Steiner, A. L., Books, S. D., Deng, C., Thornton, D. C. O., Pendleton, M. W., and Bryant, V.: Pollen as atmospheric cloud condensation nuclei, Geophys. Res. Lett., 42, 3596–3602, https://doi.org/10.1002/2015GL064060, 2015. a, b
Stiebing, C., Post, N., Schindler, C., Göhrig, B., Lux, H., Popp, J., Heutelbeck, A., and Schie, I. W.: Revealing the Chemical Composition of Birch Pollen Grains by Raman Spectroscopic Imaging, Int. J. Mol. Sci., 23, 5112, https://doi.org/10.3390/ijms23095112, 2022. a, b
Tesche, M., Ansmann, A., Müller, D., Althausen, D., Engelmann, R., Freudenthaler, V., and Groß, S.: Vertically resolved separation of dust and smoke over Cape Verde using multiwavelength Raman and polarization lidars during Saharan Mineral Dust Experiment 2008, J. Geophys. Res.-Atmos., 114, D13202, https://doi.org/10.1029/2009JD011862, 2009. a, b
Theuerkauf, M., Nehring, E., Gillert, A., Bodien, P. M., Hein, M., and Urban, B.: First automatic size measurements for the separation of dwarf birch and tree birch pollen in MIS 6 to MIS 1 records from Northern Germany, Ecol. Evol., 14, e11510, https://doi.org/10.1002/ece3.11510, 2024. a, b
Tormo-Molina, R., Maya-Manzano, J. M., Fernández-Rodríguez, S., Gonzalo Garijo, A., and Silva-Palacios, I.: Influence of environmental factors on measurements with Hirst spore traps, Grana, 52, 59–70, https://doi.org/10.1080/00173134.2012.718359, 2013. a
Triviño, M. M., Maya-Manzano, J. M., Tummon, F., Clot, B., Grewling, L., C., S.-W., and Buters, J.: Variability between Hirst-type pollen traps is reduced by resistance-free flow adjustment, Aerobiologia, 39, 257–273, https://doi.org/10.1007/s10453-023-09790-x, 2023. a
Vakkari, V., O'Connor, E. J., Nisantzi, A., Mamouri, R. E., and Hadjimitsis, D. G.: Low-level mixing height detection in coastal locations with a scanning Doppler lidar, Atmos. Meas. Tech., 8, 1875–1885, https://doi.org/10.5194/amt-8-1875-2015, 2015. a
Vakkari, V., Manninen, A. J., O'Connor, E. J., Schween, J. H., van Zyl, P. G., and Marinou, E.: A novel post-processing algorithm for Halo Doppler lidars, Atmos. Meas. Tech., 12, 839–852, https://doi.org/10.5194/amt-12-839-2019, 2019. a
Veselovskii, I., Hu, Q., Goloub, P., Podvin, T., Choël, M., Visez, N., and Korenskiy, M.: Mie–Raman–fluorescence lidar observations of aerosols during pollen season in the north of France, Atmos. Meas. Tech., 14, 4773–4786, https://doi.org/10.5194/amt-14-4773-2021, 2021. a
Wang, X., Chen, L., Ding, J., Wang, H., and Wang, X.: Profiles of Birch Allergen Component Sensitization and Its Association with Pollen Food Allergy Syndrome in Northern China, Journal of Asthma and Allergy, 16, 1241–1250, https://doi.org/10.2147/JAA.S427764, 2023. a
Wiegner, M. and Gasteiger, J.: Correction of water vapor absorption for aerosol remote sensing with ceilometers, Atmos. Meas. Tech., 8, 3971–3984, https://doi.org/10.5194/amt-8-3971-2015, 2015. a
Wozniak, M. C., Solmon, F., and Steiner, A. L.: Pollen Rupture and Its Impact on Precipitation in Clean Continental Conditions, Geophys. Res. Lett., 45, 7156–7164, https://doi.org/10.1029/2018GL077692, 2018. a, b
Zhang, Y. and Steiner, A.: Projected climate-driven changes in pollen emission season length and magnitude over the continental United States, Nat. Commun., 13, 1234, https://doi.org/10.1038/s41467-022-28764-0, 2022. a