the Creative Commons Attribution 4.0 License.
the Creative Commons Attribution 4.0 License.
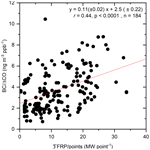
Long-term observations of black carbon and carbon monoxide in the Poker Flat Research Range, central Alaska, with a focus on forest wildfire emissions
Fumikazu Taketani
Masayuki Takigawa
Chunmao Zhu
Yongwon Kim
Petr Mordovskoi
Yugo Kanaya
Forest wildfires in interior Alaska represent an important black carbon (BC) source for the Arctic and sub-Arctic. However, BC observations in interior Alaska have not been sufficient to constrain the range of existing emissions. Here, we show our observations of BC mass concentrations and carbon monoxide (CO) mixing ratios in the Poker Flat Research Range (65.12° N, 147.43° W), located in central Alaska, from April 2016 to December 2020. The medians, 10th percentile ranges, and 90th percentile ranges of the hourly BC mass concentration and CO mixing ratio throughout the observation period were 13, 2.9, and 56 ng m−3 and 124.7, 98.7, and 148.3 ppb, respectively. Sporadically large peaks in the BC mass concentration and CO mixing ratio were observed at the same time, indicating influences from common sources. These BC peaks coincided with peaks at other comparative sites in Alaska, indicating large BC emissions in interior Alaska. Source estimation by FLEXPART-WRF (Flexible Particle Dispersion–Weather Research and Forecast) confirmed a contribution of boreal forest wildfires in Alaska and western Canada when high BC mass concentrations were observed. For these cases, we found a positive correlation (r=0.44) between the observed BC/ΔCO ratio and fire radiative power (FRP) observed in Alaska and Canada. This finding implies that the variability of the BC and CO emission ratio is associated with the intensity and time progress of forest wildfires and suggests that the BC emission factor and/or inventory could be potentially improved by FRP. We recommend that FRP be integrated into future bottom-up emission inventories to achieve a better understanding of the dynamics of pollutants from frequently occurring forest wildfires under the rapidly changing climate in the Arctic.
- Article
(3965 KB) - Full-text XML
-
Supplement
(1664 KB) - BibTeX
- EndNote
Climate change in the Arctic region has been strongly accelerated compared to the global average (Box et al., 2019; Bonfils et al., 2020). The near-surface air temperature increased between 1.8 and 3.1 °C in the period between 1971 and 2017 (Box et al., 2019). This rapid temperature increase in the Arctic region caused decreases in the extent of sea ice (Aizawa et al., 2021), resulting in the acceleration of Arctic warming (Cohen et al., 2014; Thackeray and Hall, 2019). Even if net CO2 emission is controlled to zero until the end of the 21st century (SSP1-2.6 scenario), modelling studies predicted a more than 3.5 °C temperature increase (Cai et al., 2021; Xie et al., 2022). However, there are still some difficulties associated with climate predictions based on global climate models because of the widespread use of different model hindcasts and forecasts (Overland et al., 2014). Specifically, it is known that the Arctic amplification process causes an acceleration in Arctic warming, but the process is highly complicated and is not sufficiently understood. This includes processes involved in aerosol concentration changes and the deposition of black carbon (BC) on snow and ice surfaces (Cohen et al., 2014). Thus, more research is required to understand Arctic climatic processes.
BC aerosols, which are formed by various incomplete combustion processes, such as fossil fuel and biomass burning (Bond et al., 2013), strongly contribute to warming by absorbing solar radiation (Bond et al., 2013; IPCC, 2021). In addition, BC deposited on snow and ice surfaces decreases surface albedo and contributes to snow melting and warming (Aoki et al., 2011; Bond et al., 2013; Oshima et al., 2020; IPCC, 2021). BC can be transported over long distances (estimated lifetimes are 3–6 d globally; Wang et al., 2014; Lund et al., 2018) and affects the climate and environment of remote regions, such as the Arctic (Wang et al., 2011; Matsui et al., 2022). However, large discrepancies among model estimations for BC climate effects on the Arctic remain (Gliß et al., 2021) because of a lack of observation data (IPCC, 2021) to constrain the models in terms of dependence on emission inventories (Pan et al., 2020; Matsui et al., 2022) and/or removal rates (Ikeda et al., 2017; Lund et al., 2018). For long-range transport from Asia to the Arctic, constraints on the major BC emissions from East Asia (Choi et al., 2020; Kanaya et al., 2020), ship-based observations for BC transport to the Arctic (Taketani et al., 2016, 2022), evaluation of the multimodel bias using these datasets (Whaley et al., 2022), and an improved understanding of transport mechanisms and source attributions (Ikeda et al., 2017; Zhu et al., 2020) have been achieved. However, more observational constraints are required for the characterization of BC emissions from boreal forest wildfires (Pan et al., 2020; AMAP, 2021).
Forest wildfires in the North American region, especially those that occur in Alaska every summer (Picotte et al., 2020), are one of the important BC emission sources in the Arctic and sub-Arctic troposphere, and they result in depositional fluxes on snow and ice over the Arctic and surrounding regions (Xu et al., 2017; AMAP, 2021; Matsui et al., 2022). The occurrences of these forest wildfires in interior Alaska have increased since the 1980s (Sierra-Hernández et al., 2022), and this increasing trend is predicted to continue (Hu et al., 2015; Box et al., 2019; AMAP, 2021). The emission of aerosols, including BC from forest wildfires, is projected to severely affect the environment (Halofsky et al., 2020) and climate (Schmale et al., 2021) in the future.
BC mass concentrations have long been observed in the atmosphere and snow at Utqiaġvik (formerly Barrow) (Eck et al., 2009; Garrett et al., 2011; Mori et al., 2020), which is a high Arctic coastal tundra site. Campaign studies on atmospheric BC mass concentrations were also conducted in interior Alaska using aircraft (Kondo et al., 2011b; Bian et al., 2013; Creamean et al., 2018). These campaign observations have provided an in-depth understanding of aerosol parameters related to wildfires. However, separate long-term observations of BC mass concentrations are required to characterize annual trends and seasonality. Fewer studies have reported atmospheric BC mass concentrations in interior and coastal Alaska (Polissar et al., 1996, 1998; Eck et al., 2009; Mouteva et al., 2015) and the high Arctic coastal site (Alert, Canada) (Garrett et al., 2011). To understand the long-term variations in BC mass concentration and their impacts on the climate and environment, more BC observation data from interior Alaska are needed (AMAP, 2011). In this study, we aimed to investigate detailed variations in BC mass concentration and its sources, with a focus on forest wildfires in interior Alaska, based on our monitoring of BC and carbon monoxide (CO) at the Poker Flat Research Range (PFRR), which is a University of Alaska Fairbanks (UAF) observational site in interior Alaska.
2.1 Observation site
We conducted BC and CO monitoring at the PFRR (65.12° N, 147.43° W, 500 m a.s.l.) starting in April 2016. The PFRR is located in the centre of interior Alaska (Fig. 1), approximately 35 km northeast of Fairbanks. The PFRR is surrounded by a predominant evergreen needled-leaved (black spruce, Picea mariana) forest with shrubland and herbaceous vegetation (Buchhorn et al., 2020). Note that the effects of deposition by trees and canopies can be ignored because the laboratory is located on a mountain hill with non-tall (∼ 2 m) sparse black spruce forest. In this study, BC and CO monitoring results were analysed between April 2016 and December 2020.
2.2 Measurements
BC was measured by a Continuous Soot Monitoring System (BCM3130, Kanomax, Japan) with a flow rate of 0.78 L min−1 at standard temperature and pressure (STP, i.e. 273 K and 1013 hPa). Sample air was introduced using an approximately 10 m conductive silicone tube (0.5 in. i.d.) from a height of 5.5 m above the ground. The measurement technique of BCM3130 is based on filter-based optical absorption, and thus other light-absorbing particles and scattering particles can be a source of interference on BC measurement (Bond et al., 1999; Kondo et al., 2009). To minimize interferences from these particles, coarse-mode particles (approximately > 1.0 µm), such as mineral dust, were removed by a PM1.0 cyclone (URG-2000-30ED, URG, USA) operated with a small flow regulation pump (∼ 4.5 L min−1 at STP). Note that as most BC particles are smaller than 1 µm (Bond et al., 2013), BC loss through the PM1.0 cyclone can be ignored. In addition, to remove nonrefractory particles, such as sulfate and organics, the sample air was heated to approximately 300 °C using a heated inlet before it was introduced into the instrument. More details of the instrument are described elsewhere (Miyazaki et al., 2008; Kondo et al., 2009, 2011a). The 1 min observation data were averaged to hourly data as the primary data. The limit-of-detection value (LOD) for hourly BC mass concentration was estimated to be 2 ng m−3, which is the sum of average hourly data and 3-σ values using 18 h of particle-free air measurements.
The CO mixing ratio was measured by an infrared absorption photometer (48iTLE, Thermo Fisher Scientific, USA) with a flow rate of 0.5 L min−1. Sample air was introduced using an approximately 10 m PFA tube from a height of 5.5 m above the ground. Internal zero measurements were carried out for 20 min every hour, and the CO mixing ratio was estimated from the difference in absorption between the sample and the zero measurements. Span gas (0.99 ppm , Taiyo-Nissan, Tokyo, Japan) calibration was performed in April 2016. We calculated ΔCO as the enhancement in CO from background levels (14 d moving 5th-percentile values of observation results). Cases with hourly ΔCO larger than 3-σ (13.9 ppb in median, 1-σ was derived from zero-mode measurements before and after the hourly ambient air observations) were only used for analysis. To validate our CO observations, we compared our observed CO mixing ratio with aircraft observations (less than 500 m a.g.l. above the PFRR) provided by the NOAA Global Monitoring Laboratory (https://doi.org/10.15138/39HR-9N34) (Fig. S1 in the Supplement), confirming a good agreement between these two observation results.
2.3 Model calculation
The FLEXPART-WRF (Flexible Particle Dispersion–Weather Research and Forecast) model was used in backward mode to characterize the source areas and sectors for the sampled air masses at the PFRR. FLEXPART-WRF version 3.3 (Brioude et al., 2013) and WRF version 4.4 (Skamarock et al., 2019) were employed for this study. The FLEXPART-WRF model was driven by mass-weighted wind fields and perturbation within the planetary boundary layer (PBL) calculated by WRF, which covers the Northern Hemisphere with a 45 km horizontal resolution. The ERA5 global reanalysis (Hersbach et al., 2020) was used as the initial and lateral boundary conditions of WRF, and the meteorological field of WRF was also nudged to ERA5 with e-folding times of 3 and 12 h for wind fields and temperature, respectively. Wet deposition is the major removal process for BC, and the deposition process in FLEXPART version 10 (Grythe et al., 2017) was applied to the FLEXPART-WRF model and used in this study, with values of 10.0, 1.0, 0.9, and 0.1 employed as the collection efficiencies for wet deposition by rain and snow and the activation efficiencies of cloud condensation nuclei (CCN) and ice nuclei (IN) (Crain, Csnow, CCNeff, and INeff), respectively, which are estimated by Grythe et al. (2017) as the best parameters over several Arctic regions, i.e. Utqiaġvik, Alert, and Zeppelin. The FLEXPART-WRF calculation was conducted every 6 h from April 2016 to December 2020. For each simulation, 40 000 particles were released at 0.5×0.5 degrees (horizontally) and from 0 to 200 m a.g.l. (vertically) centred at the PFRR. The particles were tracked for 20 d at 6 h intervals, and most simulated particles reached PFRR within approximately 10 d (Fig. 4c). The primary output of the FLEXPART-WRF backward calculations was the potential emission sensitivity (PES), which expresses the residence time of particles at a given location and is used to characterize the transport pathways of the sampled air masses. The concentration of BC was estimated by multiplying PES and emissions based on a procedure reported by Sauvage et al. (2017). ECLIPSE (Evaluating the Climate and Air Quality Impacts of Short-Lived Pollutants) version 6b (Klimont et al., 2017) and GFED (Global Fire Emission Database) version 4.1 (Daily) (Randerson et al., 2017) were used as the anthropogenic and biomass burning emissions, respectively. Note that the Chinese BC emissions from ECLIPSE version 6b with the monthly profile of version 5 are certified with downwind atmospheric BC observations (Kanaya et al., 2020), while other bottom-up inventories might result in a factor of ∼ 2 overestimation. The PES fields were calculated with a horizontal resolution of 0.5×0.5 degrees. The contribution of particles within 100 m from the surface was considered for the calculation of PES for anthropogenic emissions. The plume height of the GFAS (Global Fire Assimilation System) (Di Giuseppe et al., 2016) was also used for the estimation of the injection height for biomass burning emissions. The fractional contribution of anthropogenic emissions was considered using eight sectors in the ECLIPSE emission, i.e. ship, gas flaring, waste incineration, transport, industry, energy, domestic, and agriculture, and the anthropogenic and biomass burning emissions were divided into eight regions, i.e. Europe, Central Asia, Russia, East Asia, Canada, Alaska, USA (excluding Alaska), and Others. The mean age of BC was also estimated by the mean lag time between release and observed time weighted by the amount of emission at each time period within the 20 d backward calculations.
2.4 Analysis of the effect of forest wildfire on the BC mass concentration at the PFRR
We characterized the observed ratios, which are known to be valuable indicators of emission sources and combustion conditions (Kondo et al., 2011b; Pan et al., 2017; Selimovic et al., 2019), in terms of fire radiative power (FRP), which accounts for forest wildfire intensity. To do this, we compared the ratio in cases of high BC mass concentrations (see Sect. 3.4) observed between June and September (406 h in total) and FRP observed by the Visible Infrared Imaging Radiometer Suite (VIIRS) on the Suomi NPP satellite. Air masses were traced for 4 d at the most using the Hybrid Single-Particle Lagrangian Integrated Trajectory model (HYSPLIT; (Stein et al., 2015)) with GDAS1 meteorological datasets (3 h archived 1° × 1° Global Data Assimilation System) from the National Centers for Environmental Prediction (http://ready.arl.noaa.gov/gdas1.php; last access: 2 November 2023). The calculation started from 500 m a.g.l. at the PFRR site, and fire spots were searched along with the trajectories.
The ratio is also affected by atmospheric processes (Kanaya et al., 2016; Choi et al., 2020), as only BC is lost via wet removal processes. To extract observation results that were not affected by wet removal processes, we used accumulated precipitation along the trajectory (APT) as an indicator of wet removal processes. Previous studies showed that the BC/ΔCO ratio can be changed when APT is larger than 1 mm (Choi et al., 2020; Kanaya et al., 2016; Kondo et al., 2011b). Therefore, the duration for the accumulation of fire spots was shortened when APT reached 1 mm or when the trajectory reached ground level. Rectangles were defined with ±0.5° in the longitudinal direction and ±0.25° in the latitudinal direction centring around hourly air mass positions. Following this, the FRP and the number of hot spots (points) were accumulated for individual rectangles over the duration of the trajectories. Finally, the total accumulated FRP (∑ FRP) was divided by the detected total points to yield an index describing the conditions of fires affecting the observed air masses. As for hot-spot datasets, archived VIIRS 375 m (VNP14IMGTML_NRT) datasets (countries were “United States” and “Canada”) from the Fire Information for Resource Management System (FIRMS) website (https://earthdata.nasa.gov/firms; last access: 2 November 2023) were used in this study. The selected confidence levels were “nominal” or “high”, and the selected type attributed to thermal anomalies was “presumed vegetation fire”. In addition, we used FRP values greater than 0.3 MW for each hot spot because hot spots smaller than 0.3 MW included outliers (Fig. S2). Only hot spots that were observed within the previous 24 h were considered. Through this procedure (hereafter, we simply use “back trajectory”), forest wildfires in Alaska and western Canada (∑ FRP > 0) were detected in 184 cases of hourly BC observation results. Note that we also confirmed that no back trajectories could suggest forest wildfires in other seasons.
3.1 Time series of observed BC and CO concentrations
The time series of BC mass concentration and CO mixing ratio are shown in Fig. 2, and those of annual median, 10th percentile, and 90th percentile values are summarized in Table 1. The median hourly BC mass concentration and 10th and 90th percentile values throughout the observation period were 13, 3, and 56 ng m−3, respectively. No clear increase in annual median BC mass concentration was observed (Table 1). Observed median BC mass concentrations were the same level as previous reports at Utqiaġvik (Barrow) (12 ng m−3), which showed BC mass concentration over the long term using the same instrument (BCM3130) employed in this study (Sinha et al., 2017; Mori et al., 2020). Abrupt peaks (up to 5540 ng m−3) were occasionally observed during summer at PFRR, but these peaks were not observed at Utqiagvik. On the other hand, increases in BC mass concentrations were reported in Utqiagvik between January and March but not in PFRR. These different variations may be attributed to the topological separation by the Brooks Range and the polar dome structure (Quinn et al., 2007; Sharma et al., 2013).
The median, 10th percentile, and 90th percentile of hourly CO mixing ratios throughout the observation period were 124.7, 99.0, and 148.2 ppb, respectively. Similar to BC, increases in the annual median CO mixing ratio were not observed, but contrary to the BC mass concentration, the CO mixing ratio showed clear seasonal variation that was high in spring (between February and April, 143.5 ppb in the median) and low in summer (July and August, 103.3 ppb in the median) (Fig. 2b). These observed CO mixing ratios and seasonal variations were consistent with previous studies that reported the CO mixing ratio at the PFRR (Kasai et al., 2005; Yurganov et al., 1998). In summer, CO peaks coincident with BC mass concentration were found, suggesting a common emission source for both BC and CO.
Table 1Annual summary of the observed hourly BC mass concentration and CO mixing ratio at the PFRR.
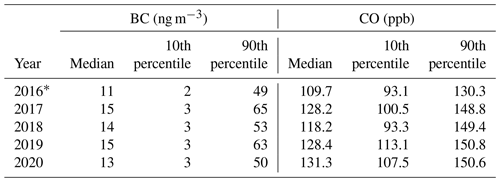
∗ Observations started on 28 April 2016.
3.2 Comparisons with other observation sites
We compared the BC observation results from the PFRR to those from other Alaskan sites (Table 2 and Fig. S3), i.e. Trapper Creek (TRCR), Denali (DENA), and Toolik Lake Field Station (TOOL), using datasets for 24 h filter samples collected every 3 d. The datasets were from the thermal/optical reflectance method at DENA, TRCR, and TOOL (http://views.cira.colostate.edu/fed/QueryWizard/; last access: 2 November 2023). A systematic bias might be present in terms of the methods used, but it is most likely within a factor of 2 from the actual conditions based on comparisons with recent data at various sites (Miyazaki et al., 2008; Kondo et al., 2009, 2011a; Kanaya et al., 2008; Ohata et al., 2021; Sinha et al., 2017). For the BC mass concentration observed at TRCR, DENA, and TOOL, datasets flagged V0 (valid value) were selected.
The BC mass concentration peaks were nearly coincided for the PFRR, DENA, TRCR, and TOOL (Fig. S3). The median and maximum daily BC mass concentrations observed at each site are summarized in Table 2. The median BC mass concentrations at DENA, TRCR, and TOOL were larger than those at the PFRR by 6–19 ng m−3 (Table 2), but the significance of the difference is unclear considering methodological differences and associated uncertainties (precision). Here, the uncertainties of the thermal/optical reflectance method varied between 12 and 14 ng m−3 in median values during the whole observation period. Note that our BC observation, which had a better LOD (2 ng m−3) and higher temporal resolution (1 h), could provide more reliable data in this low range. On the other hand, the maximum BC mass concentrations were higher at the PFRR within the period with common BC peaks than at TRCR and TOOL but similar at DENA (Table 2). This indicates that strong BC emissions in central Alaska were better captured at the PFRR than at other observation sites because PFRR is the only BC-measuring site located in the central interior of Alaska and is surrounded by regions where forest wildfires occur, while other BC observation sites are located on the edge or outside of interior Alaska. We will discuss source and emission ratio characterization in Sect. 3.4 and 3.5 by fully utilizing the superior temporal resolution and accuracy of our observations.
Table 2Summary of the locations of the observation sites and the BC mass concentrations in interior Alaska.
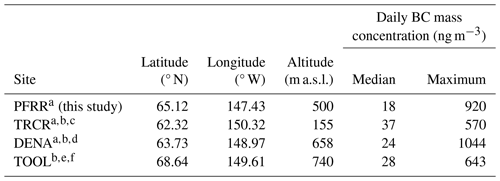
a Period of data used is 28 April 2016–2 December 2020. b Data utilized from the IMPROVE network. c TRCR: Trapper Creek. d DENA: Denali. e TOOL: Toolik Lake Field Station. f Period of data used is 13 November 2018–2 December 2020.
3.3 Comparison of observation and model simulations and possible BC sources
Figure 3a shows a time series of 6 h averages of the observation data and 6-hourly BC mass concentrations estimated by FLEXPART-WRF simulations. FLEXPART-WRF could capture the high BC mass concentration peaks (Fig. 3a) with a correlation coefficient of 0.7 (Fig. S4). The median of the simulated-to-observed ratio (observation data > LOD in this case) was 1.0 for the whole observation period, indicating good agreement between the model simulation and observations.
The source region and source sector contributions derived from the FLEXPART-WRF simulation are shown in Fig. 3b and c. The BC source sectors and regions varied clearly according to the season (Fig. 3b and c). In the warm season (between May and September), the possible BC source regions were Russia (3.6 %–74 % in the 10th–90th percentile), Alaska (12 %–85 % in the 10th–90th percentile), and sometimes Canada (1.0 %–21 % in the 10th–90th percentile) (Fig. 3b), and the possible source sector was estimated to be biomass burning (8.1 %–88 % in the 10th–90th percentile) (Fig. 3c), especially when BC mass concentration was high, suggesting that BC contributions from biomass burning that occurred in Russia, Alaska, and Canada are dominant for BC mass concentrations at the PFRR. As snow cover disappears from the ground and the atmospheric conditions become drier, forest wildfires caused by lightning increase in these warm seasons (Reap, 1991; Kaplan and Lau, 2021), resulting in increases in BC emissions from biomass burning (AMAP, 2021). We will focus on these cases of high BC mass concentrations from Alaska and discuss the relationship between forest wildfire intensity and the ratio in the following section.
On the other hand, in the cold seasons (between October and April), the domestic (24 %–48 % in the 10th–90th percentile) and transport sectors (25 %–48 % in the 10th–90th percentile) were estimated to be possible dominant BC source sectors (Fig. 3c). The dominant source region was Alaska (19 %–88 % in the 10th–90th percentile), and occasionally Russia (0.89 %–31 % in the 10th–90th percentile) and East Asia (1.2 %–41 % in the 10th–90th percentile) contributed to the BC mass concentration in PFRR (Fig. 3b).
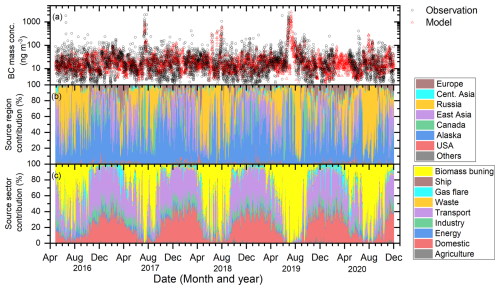
Figure 3Time series of (a) BC mass concentrations, (b) attribution of BC at the PFRR to source regions, and (c) to source sectors. Open black circles and open red triangles in (a) show the 6 h average observations and 6-hourly simulations, respectively. Individual colour bars in (b) and (c) depict the estimated contributions from the source regions and sectors, respectively. The FLEXPART-WRF model was used for all simulations.
3.4 Biomass burning contribution for cases of high BC mass concentrations
Hereafter, we focus on cases of high BC mass concentrations at the PFRR (647 h in total), which were selected with the 98th percentile value (171 ng m−3) as the threshold for the hourly BC mass concentration. The cumulative BC mass concentration observed in these cases of high BC mass concentrations accounted for 5.7 %–43 % of the annual BC mass concentration, although the duration of these periods was very short (17–187 h in a year). Most of these cases of high BC mass concentrations (approximately 90 %) were observed in warm seasons (between June and September) and were related to forest wildfires in Alaska. The median CO mixing ratio for the cases of high BC mass concentrations (174.7 ppb) was also higher than that in other periods (124.7 ppb), suggesting that both BC and CO were emitted from forest wildfires (see Sect. 3.3).
The normalized frequency distribution of the ratio for the cases of high BC mass concentrations is shown in Fig. 4a. The median, 10th percentile, and 90th percentile values of the ratio during these periods were 4.7, 1.8, and 18 ng m−3 ppb−1, respectively. These observed ratios in the cases of high BC mass concentrations were in the same range or sometimes higher than those in previous studies that reported the ratios from boreal forest wildfire emissions in Canada (Kondo et al., 2011b) and Siberia (Paris et al., 2009; Chi et al., 2013; Vasileva et al., 2017).
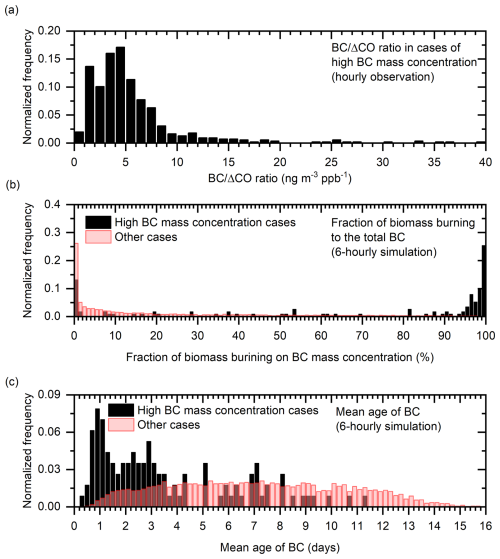
Figure 4(a) Histogram of the observed hourly ratio at the PFRR in cases of high BC mass concentrations (> 98th percentile). Histograms of the simulated 6-hourly (b) fractions of BC mass concentrations from biomass burning to the total BC and (c) mean age of BC estimated by the FLEXPART-WRF model. Black and red bars in (b) and (c) show the cases of high BC mass concentrations and the other cases (< 98th percentile), respectively.
The medians of the fractional contributions of biomass burning on total BC mass concentrations and the mean age of BC estimated by the FLEXPART-WRF simulation in these cases of high BC mass concentrations (> 154 ng m−3 in 6-hourly average) were higher and shorter (95.5 % and 2.6 d) than those in other periods (7.6 % and 6.9 d) (Fig. 4b and c), indicating a strong contribution of BC from neighbouring forest wildfires (Fig. S5). We also calculated the 6-hourly mass-weighted biomass burning contributions from individual source regions (six categories based on Fig. 3b, where Central Asia and Europe are included in Others) to the BC mass concentrations at the PFRR (Fig. 5). As a result, we found that large peaks, such as those observed between June and August in 2017, 2018, and 2019, coincided well with the peaks of BC contributions mostly from forest wildfires in Alaska (Fig. S5). BC from forest wildfires that occurred in western Canada also affected the BC concentration at PFRR (Fig. S6) but at a lesser frequency. Russia was also estimated as an effective BC source region (Fig. 3), but BC concentration did not exceed 0.1 µg m−3 in most cases (Fig. 5). These results confirmed that the observed cases of high BC mass concentrations were primarily affected by local forest wildfires in Alaska. These peaks were widely observed in Alaska (Sect. 3.2) and imply a large impact of local forest wildfires on BC mass concentration in this region. However, when these cases of high BC mass concentrations were selected, the median of the simulated-to-observed ratio was 0.30, indicating underestimation in the model simulation (possibly due to insufficient spatial resolution for neighbouring forest wildfires and difficulties in representing the vertical profiles of BC emissions) and/or in emission inventories in the cases of high BC mass concentrations. Several studies have indicated that differences in different inventories cause large uncertainties in model estimates of BC emissions, atmospheric concentrations, and radiative impacts, especially in boreal North America (Carter et al., 2020; Pan et al., 2020). The impact of different inventories on model estimates will be discussed in the future.
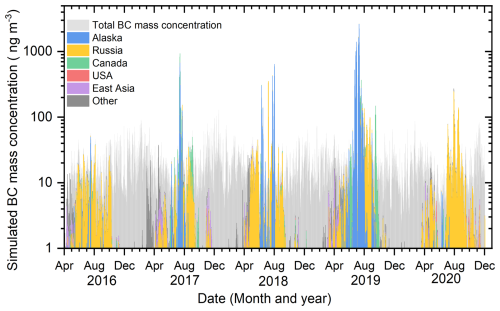
Figure 5A time series of the 6-hourly BC mass concentrations at the PFRR simulated by the FLEXPART-WRF model. Light grey bars show the total BC mass concentrations. Other individual colour bars (overlaid on the light grey bars) show the BC mass concentrations for biomass burning from each source region.
3.5 Relationship between the ratio and FRP
In the previous section, we showed that most cases of high BC mass concentrations were related to forest wildfires in Alaska. Increases in biomass-burning-derived ratios with combustion efficiency were suggested from an observational study on boreal forest wildfire (Kondo et al., 2011b) and from laboratory-scale burning experiments of crop residues (Pan et al., 2017); however, in-depth studies examining variabilities in ratios based on long-term, near-forest observations have not been conducted. To consider the possibility that combustion conditions (flaming and smouldering) primarily control the ratio, we are going to investigate the relationship between the ratio and forest wildfire intensity in this section.
We found a positive correlation (r=0.44, p<0.0001, n=184) between the ratio and ∑ values (Fig. 6). This positive correlation between the ratio and ∑ FRP/point values, represented for the first time to our knowledge, is qualitatively consistent with previous studies that showed that high combustion efficiency (larger than 0.9 in modified combustion efficiency value, MCE) increased ratios (Selimovic et al., 2019; Kondo et al., 2011b; Pan et al., 2017), which is related to the fact that the BC production process is mostly related to the flaming process (high MCE), while that of CO is related to the smouldering process (low MCE). For example, Pan et al. (2017) measured BC, CO, and CO2 from biomass burning in small-scale combustion experiments. In their experiment, dry and wet wheat straw samples and dry rapeseed plant samples were burned, and the time evolution of ratio and MCE were observed. They reported that BC is mostly produced during the flaming process, and the evolution of the ratio that depends on the combustion stage could be confirmed (13.9±10.1 ng m−3 ppbv−1 for MCE larger than 0.95 cases, and less than 7.1 ng m−3 ppbv−1 for MCE smaller than 0.96 cases). Although these ratios are larger than our observed ratio, differences in fuels might be a possible reason. Selimovic et al. (2018) also burned some types of fuels, including coniferous trees, in a large indoor combustion facility and measured BC, CO, and CO2 with various other chemical species. They reported a high ratio (13.8 ng m−3 ppbv−1 on average) and a low ratio (4.7 ng m−3 ppbv−1 on average) in flaming-dominated and smouldering-dominated conditions, respectively, in the same range as our observed values. Moreover, Chakrabarty et al. (2016) tested Alaskan peat and Siberian peat in the combustion chamber under smouldering conditions, and low BC/ΔCO ratios (1.2–2.6 ng m−3 ppbv−1) were reported. The positive relationship between the ratio and MCE is also observed in the field measurements (Kondo et al., 2011b; Selimovic et al., 2019). Although MCE and FRP are different parameters, both parameters indicate combustion conditions and have a strong correlation (Wiggins et al., 2020). Therefore, for the first time, we report a positive correlation between the ratio and FRP as a combustion condition indicator. The wide range of ratios reported from boreal forest wildfires, from 1.7–3.4 ng m−3 ppb−1 (Kondo et al., 2011b) to 6.1–6.3 ng m−3 ppb−1 (Vasileva et al., 2017), could be better explained when the index introduced here (∑ FRP/point) is considered. This relationship should be taken into account when constructing future emission inventories from boreal forest wildfires.
A positive correlation was found after optimizing the spatial window size (±0.5° in the longitudinal direction and ±0.25° in the latitudinal direction) in which hot spots were taken into account for each hour along the trajectory (from −96 to 0 h), and the associated time window was used to determine coincident fires that affected the observations (from −24 to 0 h). Based on the spatial resolution of GDAS1 (1° × 1°), we set our initial windows as ±0.5° for latitude and longitude. However, PFRR is at a high latitude, and the geometrical length of latitude is approximately 2 times longer than that of longitude. For this reason, we finally defined latitudinal width as ±0.25°. Although we tested finer window size cases, a similar positive trend was confirmed. The remaining scatter might have arisen from differences in the detection of hot spots in the presence of clouds (Li et al., 2018). To overcome shortcomings in hot-spot detection, improvements in the frequency of hot-spot scanning should be made, for example, via the use of MODerate Resolution Imaging Spectroradiometer (MODIS) combined with VIIRS observations. It should be noted, however, that there is a bias in FRP observations between MODIS and VIIRS, especially for boreal forests (Li et al., 2018). Improvements in the accuracy and consistency of FRP analysis between multiple satellite observations can facilitate a more in-depth understanding of the relationship between FRP and the ratio.
The simulated-to-observed ratios in cases of high BC mass concentrations were low (0.30, Sect. 3.3), contrary to the good agreement observed in overall cases (1.0, Sect. 3.3). The ratios in commonly used emission inventories are 4.9 ng m−3 ppb−1 for GFED4s (van der Werf et al., 2017) and 4.4 ng m−3 ppb−1 for Andreae (2019) and are in a range similar to that of our median ratio. However, our observed ratios in cases of high BC mass concentrations for forest wildfires had a broad range between 1.7 and 7.3 ng m−3 ppb−1 at the 10th and 90th percentiles (median was 4.2 ng m−3 ppb−1), respectively, related to the ∑ FRP/point values. This implies that the BC emission factors from biomass burning could vary depending on the FRP. Although several previous inventory studies used FRP for the estimation of activity data (Carter et al., 2020), namely fuel burned or burned area, no inventories included the evolution of the emission factors of BC and/or CO. Our findings suggest the potential for improving BC emission inventories and/or emission factors by using FRP. In addition, BC emission estimation using satellites would be improved by using our results. CO emissions estimated by satellite observations are sometimes used to estimate other pollutant emissions from forest fires using emission ratios derived from in situ measurements (Zheng et al., 2023). As its extension, BC emissions could be estimated, regarding our quantified ratios and their evolutions with FRP directly as the emission ratio of BC to CO. The frequency of boreal forest fires may increase in the future (Box et al., 2019; Hu et al., 2015); as a result, their impact on climate and air quality might become more severe in Alaska and the Arctic (Kim et al., 2005; Schmale et al., 2018; Stohl et al., 2006). Our long-term observations of BC and CO at an hourly temporal resolution in the interior of Alaska provide unique information to test model simulations and emission inventories relevant to the climate and air quality of the Arctic.
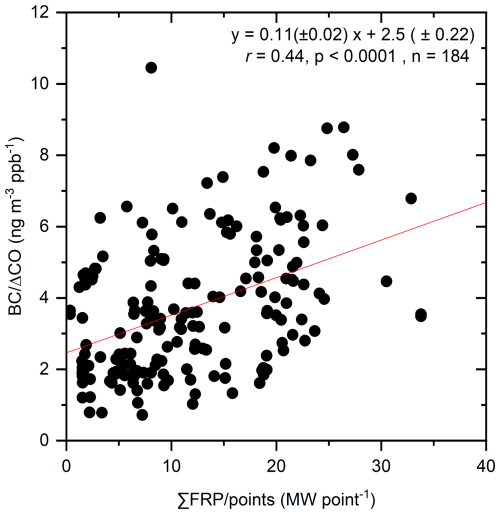
Figure 6A scatter plot (filled black circles) between the hourly ratio observed at the PFRR and the ∑ FRP/point values in cases of high BC concentrations (> 98th percentile). Data from June to September were analysed. The ∑ FRP/point values are the average FRP of the hot spots (points) present along the backward trajectories from the PFRR (see Sect. 2.4). A red line indicates the result of a linear regression fit.
We showed key features of the BC and CO concentrations observed at the PFRR in interior Alaska since 2016 in this paper. The annual medians of the BC mass concentration and CO mixing ratio were 11–15 ng m−3 and 109.7–131.3 ppb, respectively. Large and short-term increases in BC mass concentrations were sometimes observed between June and September. A clear seasonal variation was observed in the CO mixing ratio, which was high in spring (between February and April, 143.5 ppb in the median) and low in summer (July and August, 103.3 ppb in the median). The CO mixing ratio coincided with the high BC mass concentration peaks, suggesting a strong contribution from forest wildfires to BC and CO concentrations.
The BC mass concentrations observed at other sites in Alaska, i.e. DENA, TRCR, and TOOL, were compared with our results. The annual median BC mass concentrations at the PFRR were lower than those at TRCR, DENA, and TOOL, but coinciding BC mass concentration peaks were found at these observation sites. In these cases of high BC mass concentrations, BC mass concentrations at the PFRR were larger than those at TRCR and TOOL but similar at DENA, indicating that strong BC emissions from forest wildfires occurred in interior Alaska and affected broad areas in Alaska.
The dominance of forest wildfires in Alaska as a major cause of high BC mass concentration was also supported by the model simulations. We simulated BC mass concentration using the FLEXPART-WRF model and compared the simulations with the observation results. The model simulation could capture observational results (r=0.70) in which the median simulated-to-observed ratio was 1.0. The estimated BC source sectors and regions were biomass burning from Russia, Alaska, and sometimes Canada between May and September, while those for other periods were domestic sources and transport and were mainly from Alaska.
When we focused on cases of high BC mass concentrations (greater than 98 percentile values), we found that forest wildfires occurring in Alaska were the dominant source of BC in those cases from the model simulation results. The mean ages of BC and biomass burning contributions in these cases of high BC mass concentrations estimated by FLEXPART-WRF were 2.6 d and 95.5 %, respectively, relatively shorter and higher than those in other cases (6.9 d and 7.6 %, respectively). The peaks of the calculated biomass burning contributions from Alaska to BC mass concentrations at the PFRR coincided well with observed and simulated peaks in cases of high BC mass concentrations, suggesting that the forest wildfires that occurred around the PFRR are important.
The median observed ratio in cases of high BC mass concentrations related to forest wildfires was 4.2 ng m−3 ppb−1 and was in the same range as that in previous studies reporting the ratio of boreal forest wildfire emissions. Finally, we tracked air mass origin for 4 d using the HYSPLIT model with FRP satellite observations in these cases and investigated the relationship between the observed ratio and FRP, which was normalized by the number of hot spots (points) observed by VIIRS. A positive correlation was found between these parameters (r=0.44). For the first time, the properties of the ratio from boreal forest wildfires were systematically characterized in terms of FRP, suggesting the potential to improve emission inventories and/or emission factors by using FRP.
The BC and CO observation results are available online (https://ads.nipr.ac.jp/dataset/A20241101-003, Kinase et al., 2024). We used public data for BC observation results at Denali, Trapper Creek, and Toolik Lake Field Station (https://views.cira.colostate.edu/fed, Federal Land Manager Environmental Database, 2024). We used public BC emission inventory data from biomass burning (https://doi.org/10.3334/ORNLDAAC/1293, Randerson et al., 2018).
The supplement related to this article is available online at: https://doi.org/10.5194/acp-25-143-2025-supplement.
TK, FT, CZ, YKi, and YKa conducted and recorded observations for BC and CO at the PFRR site. MT conducted the FLEXPART-WRF model simulations. YKi assisted in the fieldwork at the PFRR site. TK, FT, MP, and YKa summarized the observation results, and TK wrote the first draft with MT. All authors contributed to the discussion and writing of the manuscript.
At least one of the (co-)authors is a member of the editorial board of Atmospheric Chemistry and Physics. The peer-review process was guided by an independent editor, and the authors also have no other competing interests to declare.
Publisher’s note: Copernicus Publications remains neutral with regard to jurisdictional claims made in the text, published maps, institutional affiliations, or any other geographical representation in this paper. While Copernicus Publications makes every effort to include appropriate place names, the final responsibility lies with the authors.
The authors acknowledge technical support from Takuma Miyakawa and help with fieldwork from Hideki Kobayashi, who are both researchers at JAMSTEC. The authors also thank all the supporting members at JAMSTEC. The authors thank NOAA ARL for providing the CO aircraft observation data, HYSPLIT model, and GDAS1 meteorological data. We also thank the IMPROVE network. IMPROVE is a collaborative association of state, tribal, and federal agencies and international partners. The U.S. Environmental Protection Agency is the primary funding source, with contracting and research support from the National Park Service. The Air Quality Group at the University of California, Davis, was the central analytical laboratory, and carbon analysis was carried out by the Desert Research Institute. We also thank the anonymous reviewers for their precise and valuable comments that greatly improved the paper.
This work was funded by the Arctic Challenge for Sustainability II (ArCS II) program grant no. JPMXD1420318865, the Arctic Challenge for Sustainability (ArCS) program grant no. JPMXD1300000000, and a National Research Foundation of Korea Grant from the South Korean Government (MSIT; the Ministry of Science and ICT, NRF-2021M1A5A1065425) (KOPRI-PN24011).
This paper was edited by Philip Stier and reviewed by two anonymous referees.
Aizawa, T., Ishii, M., Oshima, N., Yukimoto, S., and Hasumi, H.: Arctic warming and associated sea ice reduction in the early 20th century induced by natural forcings in MRI-ESM2.0 climate simulations and multimodel analyses, Geophys. Res. Lett., 48, 8, https://doi.org/10.1029/2020gl092336, 2021.
AMAP: The Impact of Black Carbon on Arctic Climate, Arctic Monitoring and Assessment Programme (AMAP), Oslo, Norway, 72 pp., https://www.amap.no/documents/download/977/inline (last access: 19 December, 2024), 2011.
AMAP: AMAP Assessment 2021: Impacts of Short-lived Climate Forcers on Arctic Climate, Air Quality, and Human Health, Arctic Monitoring and Assessment Programme (AMAP), Tromsø, Norway. x + 375 pp., https://www.amap.no/documents/doc/amap-assessment-2021-impacts-of-short-lived (last access: 19 December 2024), 2021.
Andreae, M. O.: Emission of trace gases and aerosols from biomass burning – an updated assessment, Atmos. Chem. Phys., 19, 8523–8546, https://doi.org/10.5194/acp-19-8523-2019, 2019.
Aoki, T., Kuchiki, K., Niwano, M., Kodama, Y., Hosaka, M., and Tanaka, T.: Physically based snow albedo model for calculating broadband albedos and the solar heating profile in snowpack for general circulation models, J. Geophys. Res., 116, D11, https://doi.org/10.1029/2010jd015507, 2011.
Bian, H., Colarco, P. R., Chin, M., Chen, G., Rodriguez, J. M., Liang, Q., Blake, D., Chu, D. A., da Silva, A., Darmenov, A. S., Diskin, G., Fuelberg, H. E., Huey, G., Kondo, Y., Nielsen, J. E., Pan, X., and Wisthaler, A.: Source attributions of pollution to the Western Arctic during the NASA ARCTAS field campaign, Atmos. Chem. Phys., 13, 4707–4721, https://doi.org/10.5194/acp-13-4707-2013, 2013.
Bond, T. C., Anderson, T. L., and Campbell, D.: Calibration and Intercomparison of Filter-Based Measurements of Visible Light Absorption by Aerosols, Aerosol Sci. Technol., 30, 582–600, https://doi.org/10.1080/027868299304435, 1999.
Bond, T. C., Doherty, S. J., Fahey, D. W., Forster, P. M., Berntsen, T., DeAngelo, B. J., Flanner, M. G., Ghan, S., Kärcher, B., Koch, D., Kinne, S., Kondo, Y., Quinn, P. K., Sarofim, M. C., Schultz, M. G., Schulz, M., Venkataraman, C., Zhang, H., Zhang, S., Bellouin, N., Guttikunda, S. K., Hopke, P. K., Jacobson, M. Z., Kaiser, J. W., Klimont, Z., Lohmann, U., Schwarz, J. P., Shindell, D., Storelvmo, T., Warren, S. G., and Zender, C. S.: Bounding the role of black carbon in the climate system: A scientific assessment, J. Geophys. Res., 118, 5380–5552, https://doi.org/10.1002/jgrd.50171, 2013.
Bonfils, C. J. W., Santer, B. D., Fyfe, J. C., Marvel, K., Phillips, T. J., and Zimmerman, S. R. H.: Human influence on joint changes in temperature, rainfall and continental aridity, Nat. Clim. Change, 10, 726–731, https://doi.org/10.1038/s41558-020-0821-1, 2020.
Box, J. E., Colgan, W. T., Christensen, T. R., Schmidt, N. M., Lund, M., Parmentier, F.-J. W., Brown, R., Bhatt, U. S., Euskirchen, E. S., Romanovsky, V. E., Walsh, J. E., Overland, J. E., Wang, M., Corell, R. W., Meier, W. N., Wouters, B., Mernild, S. H., Mård, J., Pawlak, J., and Olsen, M. S.: Key indicators of Arctic climate change: 1971–2017, Environ. Res. Lett., 14, 045010, https://doi.org/10.1088/1748-9326/aafc1b, 2019.
Brioude, J., Arnold, D., Stohl, A., Cassiani, M., Morton, D., Seibert, P., Angevine, W., Evan, S., Dingwell, A., Fast, J. D., Easter, R. C., Pisso, I., Burkhart, J., and Wotawa, G.: The Lagrangian particle dispersion model FLEXPART-WRF version 3.1, Geosci. Model Dev., 6, 1889–1904, https://doi.org/10.5194/gmd-6-1889-2013, 2013.
Buchhorn, M., Smets, B., Bertels, L., De Roo, B., Lesiv, M., Tsendbazar, N.-E., Herold, M., and Fritz, S.: Copernicus Global Land Service: Land Cover 100 m: collection 3: epoch 2019: Globe, https://doi.org/10.5281/zenodo.3939050, 2020.
Cai, Z., You, Q., Wu, F., Chen, H. W., Chen, D., and Cohen, J.: Arctic Warming Revealed by Multiple CMIP6 Models: Evaluation of Historical Simulations and Quantification of Future Projection Uncertainties, J. Climate, 34, 4871–4892, https://doi.org/10.1175/JCLI-D-20-0791.1, 2021.
Carter, T. S., Heald, C. L., Jimenez, J. L., Campuzano-Jost, P., Kondo, Y., Moteki, N., Schwarz, J. P., Wiedinmyer, C., Darmenov, A. S., da Silva, A. M., and Kaiser, J. W.: How emissions uncertainty influences the distribution and radiative impacts of smoke from fires in North America, Atmos. Chem. Phys., 20, 2073–2097, https://doi.org/10.5194/acp-20-2073-2020, 2020.
Chakrabarty, R. K., Gyawali, M., Yatavelli, R. L. N., Pandey, A., Watts, A. C., Knue, J., Chen, L.-W. A., Pattison, R. R., Tsibart, A., Samburova, V., and Moosmüller, H.: Brown carbon aerosols from burning of boreal peatlands: microphysical properties, emission factors, and implications for direct radiative forcing, Atmos. Chem. Phys., 16, 3033–3040, https://doi.org/10.5194/acp-16-3033-2016, 2016.
Chi, X., Winderlich, J., Mayer, J.-C., Panov, A. V., Heimann, M., Birmili, W., Heintzenberg, J., Cheng, Y., and Andreae, M. O.: Long-term measurements of aerosol and carbon monoxide at the ZOTTO tall tower to characterize polluted and pristine air in the Siberian taiga, Atmos. Chem. Phys., 13, 12271–12298, https://doi.org/10.5194/acp-13-12271-2013, 2013.
Choi, Y., Kanaya, Y., Takigawa, M., Zhu, C., Park, S.-M., Matsuki, A., Sadanaga, Y., Kim, S.-W., Pan, X., and Pisso, I.: Investigation of the wet removal rate of black carbon in East Asia: validation of a below- and in-cloud wet removal scheme in FLEXible PARTicle (FLEXPART) model v10.4, Atmos. Chem. Phys., 20, 13655–13670, https://doi.org/10.5194/acp-20-13655-2020, 2020.
Cohen, J., Screen, J. A., Furtado, J. C., Barlow, M., Whittleston, D., Coumou, D., Francis, J., Dethloff, K., Entekhabi, D., Overland, J., and Jones, J.: Recent Arctic amplification and extreme mid-latitude weather, Nat. Geosci., 7, 627–637, https://doi.org/10.1038/ngeo2234, 2014.
Creamean, J. M., Maahn, M., de Boer, G., McComiskey, A., Sedlacek, A. J., and Feng, Y.: The influence of local oil exploration and regional wildfires on summer 2015 aerosol over the North Slope of Alaska, Atmos. Chem. Phys., 18, 555–570, https://doi.org/10.5194/acp-18-555-2018, 2018.
Di Giuseppe, F., Remy, S., Pappenberger, F., and Wetterhall, F.: Improving GFAS and CAMS biomass burning estimations by means of the Global ECMWF Fire Forecast system (GEFF), ECMWF Technical Memoranda, 790, 1–20, https://doi.org/10.21957/uygqqtyh7, 2016.
Eck, T. F., Holben, B. N., Reid, J. S., Sinyuk, A., Hyer, E. J., O'Neill, N. T., Shaw, G. E., Vande Castle, J. R., Chapin, F. S., Dubovik, O., Smirnov, A., Vermote, E., Schafer, J. S., Giles, D., Slutsker, I., Sorokine, M., and Newcomb, W. W.: Optical properties of boreal region biomass burning aerosols in central Alaska and seasonal variation of aerosol optical depth at an Arctic coastal site, J. Geophys. Res., 114, D11, https://doi.org/10.1029/2008jd010870, 2009.
Federal Land Manager Environmental Database (FED): Cooperative Institute for Research in the Atmosphere/Colorado State University, FED, https://views.cira.colostate.edu/fed (last access: 19 December 2024), 2024.
Garrett, T. J., Brattström, S., and Sharma, S.: The role of scavenging in the seasonal transport of black carbon and sulfate to the Arctic, Geophys. Res. Lett., 38, 16, https://doi.org/10.1029/2011GL048221, 2011.
Gliß, J., Mortier, A., Schulz, M., Andrews, E., Balkanski, Y., Bauer, S. E., Benedictow, A. M. K., Bian, H., Checa-Garcia, R., Chin, M., Ginoux, P., Griesfeller, J. J., Heckel, A., Kipling, Z., Kirkevåg, A., Kokkola, H., Laj, P., Le Sager, P., Lund, M. T., Lund Myhre, C., Matsui, H., Myhre, G., Neubauer, D., van Noije, T., North, P., Olivié, D. J. L., Rémy, S., Sogacheva, L., Takemura, T., Tsigaridis, K., and Tsyro, S. G.: AeroCom phase III multi-model evaluation of the aerosol life cycle and optical properties using ground- and space-based remote sensing as well as surface in situ observations, Atmos. Chem. Phys., 21, 87–128, https://doi.org/10.5194/acp-21-87-2021, 2021.
Grythe, H., Kristiansen, N. I., Groot Zwaaftink, C. D., Eckhardt, S., Ström, J., Tunved, P., Krejci, R., and Stohl, A.: A new aerosol wet removal scheme for the Lagrangian particle model FLEXPART v10, Geosci. Model Dev., 10, 1447–1466, https://doi.org/10.5194/gmd-10-1447-2017, 2017.
Halofsky, J. E., Peterson, D. L., and Harvey, B. J.: Changing wildfire, changing forests: the effects of climate change on fire regimes and vegetation in the Pacific Northwest, USA, Fire Ecol., 16, 4, https://doi.org/10.1186/s42408-019-0062-8, 2020.
Hersbach, H., Bell, B., Berrisford, P., Hirahara, S., Horányi, A., Muñoz-Sabater, J., Nicolas, J., Peubey, C., Radu, R., Schepers, D., Simmons, A., Soci, C., Abdalla, S., Abellan, X., Balsamo, G., Bechtold, P., Biavati, G., Bidlot, J., Bonavita, M., Chiara, G., Dahlgren, P., Dee, D., Diamantakis, M., Dragani, R., Flemming, J., Forbes, R., Fuentes, M., Geer, A., Haimberger, L., Healy, S., Hogan, R. J., Hólm, E., Janisková, M., Keeley, S., Laloyaux, P., Lopez, P., Lupu, C., Radnoti, G., Rosnay, P., Rozum, I., Vamborg, F., Villaume, S., and Thépaut, J.-N.: The ERA5 global reanalysis, Q. J. Roy. Meteor. Soc., 146, 1999–2049, https://doi.org/10.1002/qj.3803, 2020.
Hu, F. S., Higuera, P. E., Duffy, P., Chipman, M. L., Rocha, A. V., Young, A. M., Kelly, R., and Dietze, M. C.: Arctic tundra fires: natural variability and responses to climate change, Front. Ecol. Environ., 13, 369–377, https://doi.org/10.1890/150063, 2015.
Ikeda, K., Tanimoto, H., Sugita, T., Akiyoshi, H., Kanaya, Y., Zhu, C., and Taketani, F.: Tagged tracer simulations of black carbon in the Arctic: transport, source contributions, and budget, Atmos. Chem. Phys., 17, 10515–10533, https://doi.org/10.5194/acp-17-10515-2017, 2017.
IPCC: Climate change 2021: the physical science basis, edited by: Masson-Delmotte, V., Zhai, P., Pirani, A., Connors, S. L., Péan, C., Berger, S., Caud, N., Chen, Y., Goldfarb, L., Gomis, M. I., Huang, M., Leitzell, K., Lonnoy, E., Matthews, J. B. R., Maycock, T. K., Waterfield, T., Yelekçi, O., Yu, R., and Zhou, B., Cambridge University Press Cambridge, UK, https://doi.org/10.1017/9781009157896, 2021.
Kanaya, Y., Komazaki, Y., Pochanart, P., Liu, Y., Akimoto, H., Gao, J., Wang, T., and Wang, Z.: Mass concentrations of black carbon measured by four instruments in the middle of Central East China in June 2006, Atmos. Chem. Phys., 8, 7637–7649, https://doi.org/10.5194/acp-8-7637-2008, 2008.
Kanaya, Y., Pan, X., Miyakawa, T., Komazaki, Y., Taketani, F., Uno, I., and Kondo, Y.: Long-term observations of black carbon mass concentrations at Fukue Island, western Japan, during 2009–2015: constraining wet removal rates and emission strengths from East Asia, Atmos. Chem. Phys., 16, 10689–10705, https://doi.org/10.5194/acp-16-10689-2016, 2016.
Kanaya, Y., Yamaji, K., Miyakawa, T., Taketani, F., Zhu, C., Choi, Y., Komazaki, Y., Ikeda, K., Kondo, Y., and Klimont, Z.: Rapid reduction in black carbon emissions from China: evidence from 2009–2019 observations on Fukue Island, Japan, Atmos. Chem. Phys., 20, 6339–6356, https://doi.org/10.5194/acp-20-6339-2020, 2020.
Kaplan, J. O. and Lau, K. H.-K.: The WGLC global gridded lightning climatology and time series, Earth Syst. Sci. Data, 13, 3219–3237, https://doi.org/10.5194/essd-13-3219-2021, 2021.
Kasai, Y. J., Koshiro, T., Endo, M., Jones, N. B., and Murayama, Y.: Ground-based measurement of strato–mesospheric CO by a FTIR spectrometer over Poker Flat, Alaska, Adv. Space Res., 35, 2024–2030, https://doi.org/10.1016/j.asr.2005.04.099, 2005.
Kim, Y., Hatsushika, H., Muskett, R. R., and Yamazaki, K.: Possible effect of boreal wildfire soot on Arctic sea ice and Alaska glaciers, Atmos. Environ., 39, 3513–3520, https://doi.org/10.1016/j.atmosenv.2005.02.050, 2005.
Kinase, T., Kanaya, Y., Taketani, F., Takigawa, M., Kobayashi, H., Zhu, C., and Kim, Y.: Mass concentration of BC(black carbon) measurment by COSMOS and mixing ratio of CO (carbon monoxide) measured by 48iTLE at Poker Flat Research Range (PFRR), Alaska, USA, 1.00, Arctic Data archive System (ADS), Japan [data set], https://ads.nipr.ac.jp/dataset/A20241101-003 (last access: 19 December 2024), 2024.
Klimont, Z., Kupiainen, K., Heyes, C., Purohit, P., Cofala, J., Rafaj, P., Borken-Kleefeld, J., and Schöpp, W.: Global anthropogenic emissions of particulate matter including black carbon, Atmos. Chem. Phys., 17, 8681–8723, https://doi.org/10.5194/acp-17-8681-2017, 2017.
Kondo, Y., Sahu, L., Kuwata, M., Miyazaki, Y., Takegawa, N., Moteki, N., Imaru, J., Han, S., Nakayama, T., Oanh, N. T. K., Hu, M., Kim, Y. J., and Kita, K.: Stabilization of the Mass Absorption Cross Section of Black Carbon for Filter-Based Absorption Photometry by the use of a Heated Inlet, Aerosol Sci. Technol., 43, 741–756, https://doi.org/10.1080/02786820902889879, 2009.
Kondo, Y., Sahu, L., Moteki, N., Khan, F., Takegawa, N., Liu, X., Koike, M., and Miyakawa, T.: Consistency and traceability of black carbon measurements made by laser-induced incandescence, thermal-optical transmittance, and filter-based photo-absorption techniques, Aerosol Sci. Technol., 45, 295–312, https://doi.org/10.1080/02786826.2010.533215, 2011a.
Kondo, Y., Matsui, H., Moteki, N., Sahu, L., Takegawa, N., Kajino, M., Zhao, Y., Cubison, M. J., Jimenez, J. L., Vay, S., Diskin, G. S., Anderson, B., Wisthaler, A., Mikoviny, T., Fuelberg, H. E., Blake, D. R., Huey, G., Weinheimer, A. J., Knapp, D. J., and Brune, W. H.: Emissions of black carbon, organic, and inorganic aerosols from biomass burning in North America and Asia in 2008, J. Geophys. Res., 116, D8, https://doi.org/10.1029/2010jd015152, 2011b.
Li, F., Zhang, X., Kondragunta, S., and Csiszar, I.: Comparison of fire radiative power estimates from VIIRS and MODIS observations, J. Geophys. Res., 123, 4545–4563, https://doi.org/10.1029/2017jd027823, 2018.
Lund, M. T., Samset, B. H., Skeie, R. B., Watson-Parris, D., Katich, J. M., Schwarz, J. P., and Weinzierl, B.: Short Black Carbon lifetime inferred from a global set of aircraft observations, npj Clim. Atmos. Sci., 1, 1–8, https://doi.org/10.1038/s41612-018-0040-x, 2018.
Matsui, H., Mori, T., Ohata, S., Moteki, N., Oshima, N., Goto-Azuma, K., Koike, M., and Kondo, Y.: Contrasting source contributions of Arctic black carbon to atmospheric concentrations, deposition flux, and atmospheric and snow radiative effects, Atmos. Chem. Phys., 22, 8989–9009, https://doi.org/10.5194/acp-22-8989-2022, 2022.
Miyazaki, Y., Kondo, Y., Sahu, L. K., Imaru, J., Fukushima, N., and Kano, M.: Performance of a newly designed continuous soot monitoring system (COSMOS), J. Environ. Monit., 10, 1195–1201, https://doi.org/10.1039/b806957c, 2008.
Mori, T., Kondo, Y., Ohata, S., Zhao, Y., Sinha, P. R., Oshima, N., Matsui, H., Moteki, N., and Koike, M.: Seasonal variation of wet deposition of black carbon in arctic Alaska, J. Geophys. Res., 125, 16, https://doi.org/10.1029/2019jd032240, 2020.
Mouteva, G. O., Czimczik, C. I., Fahrni, S. M., Wiggins, E. B., Rogers, B. M., Veraverbeke, S., Xu, X., Santos, G. M., Henderson, J., Miller, C. E., and Randerson, J. T.: Black carbon aerosol dynamics and isotopic composition in Alaska linked with boreal fire emissions and depth of burn in organic soils, Global Biogeochem. Cy., 29, 1977–2000, https://doi.org/10.1002/2015gb005247, 2015.
Ohata, S., Mori, T., Kondo, Y., Sharma, S., Hyvärinen, A., Andrews, E., Tunved, P., Asmi, E., Backman, J., Servomaa, H., Veber, D., Eleftheriadis, K., Vratolis, S., Krejci, R., Zieger, P., Koike, M., Kanaya, Y., Yoshida, A., Moteki, N., Zhao, Y., Tobo, Y., Matsushita, J., and Oshima, N.: Estimates of mass absorption cross sections of black carbon for filter-based absorption photometers in the Arctic, Atmos. Meas. Tech., 14, 6723–6748, https://doi.org/10.5194/amt-14-6723-2021, 2021.
Oshima, N., Yukimoto, S., Deushi, M., Koshiro, T., Kawai, H., Tanaka, T. Y., and Yoshida, K.: Global and Arctic effective radiative forcing of anthropogenic gases and aerosols in MRI-ESM2.0, Prog. Earth Planet. Sci., 7, 38, https://doi.org/10.1186/s40645-020-00348-w, 2020.
Overland, J. E., Wang, M., Walsh, J. E., and Stroeve, J. C.: Future Arctic climate changes: Adaptation and mitigation time scales, Earths Future, 2, 68–74, https://doi.org/10.1002/2013ef000162, 2014.
Pan, X., Kanaya, Y., Taketani, F., Miyakawa, T., Inomata, S., Komazaki, Y., Tanimoto, H., Wang, Z., Uno, I., and Wang, Z.: Emission characteristics of refractory black carbon aerosols from fresh biomass burning: a perspective from laboratory experiments, Atmos. Chem. Phys., 17, 13001–13016, https://doi.org/10.5194/acp-17-13001-2017, 2017.
Pan, X., Ichoku, C., Chin, M., Bian, H., Darmenov, A., Colarco, P., Ellison, L., Kucsera, T., da Silva, A., Wang, J., Oda, T., and Cui, G.: Six global biomass burning emission datasets: intercomparison and application in one global aerosol model, Atmos. Chem. Phys., 20, 969–994, https://doi.org/10.5194/acp-20-969-2020, 2020.
Paris, J.-D., Stohl, A., Nédélec, P., Arshinov, M. Yu., Panchenko, M. V., Shmargunov, V. P., Law, K. S., Belan, B. D., and Ciais, P.: Wildfire smoke in the Siberian Arctic in summer: source characterization and plume evolution from airborne measurements, Atmos. Chem. Phys., 9, 9315–9327, https://doi.org/10.5194/acp-9-9315-2009, 2009.
Picotte, J. J., Bhattarai, K., Howard, D., Lecker, J., Epting, J., Quayle, B., Benson, N., and Nelson, K.: Changes to the Monitoring Trends in Burn Severity program mapping production procedures and data products, Fire Ecol., 16, 16, https://doi.org/10.1186/s42408-020-00076-y, 2020.
Polissar, A. V., Hopke, P. K., Malm, W. C., and Sisler, J. F.: The ratio of aerosol optical absorption coefficients to sulfur concentrations, as an indicator of smoke from forest fires when sampling in polar regions, Atmos. Environ., 30, 1147–1157, https://doi.org/10.1016/1352-2310(95)00334-7, 1996.
Polissar, A. V., Hopke, P. K., and Malm, W. C.: Atmospheric aerosol over Alaska: 1. Spatial and seasonal variability, J. Geophys. Res., 103, D15, https://doi.org/10.1029/98JD01365, 1998.
Quinn, P. K., Shaw, G., Andrews, E., Dutton, E. G., Ruoho-Airola, T., and Gong, S. L.: Arctic haze: current trends and knowledge gaps, Tellus B Chem. Phys. Meteorol., 59, 99, https://doi.org/10.1111/j.1600-0889.2006.00236.x, 2007.
Randerson, J. T., van der Werf, G. R., Giglio, L., Collatz, G. J., and Kasibhatla, P. S.: Global Fire Emissions Database, Version 4.1 (GFEDv4), ORNL DAAC, USA [data set], https://doi.org/10.3334/ORNLDAAC/1293, 2017.
Reap, R. M.: Climatological Characteristics and Objective Prediction of Thunderstorms over Alaska, Weather Forecast., 6, 309–319, https://doi.org/10.1175/1520-0434(1991)006<0309:CCAOPO>2.0.CO;2, 1991.
Sauvage, B., Fontaine, A., Eckhardt, S., Auby, A., Boulanger, D., Petetin, H., Paugam, R., Athier, G., Cousin, J.-M., Darras, S., Nédélec, P., Stohl, A., Turquety, S., Cammas, J.-P., and Thouret, V.: Source attribution using FLEXPART and carbon monoxide emission inventories: SOFT-IO version 1.0, Atmos. Chem. Phys., 17, 15271–15292, https://doi.org/10.5194/acp-17-15271-2017, 2017.
Schmale, J., Arnold, S. R., Law, K. S., and Thorp, T.: Local Arctic air pollution: A neglected but serious problem, Earth's Future, https://doi.org/10.1029/2018EF000952, 2018.
Schmale, J., Zieger, P., and Ekman, A. M. L.: Aerosols in current and future Arctic climate, Nat. Clim. Chang., 11, 95–105, https://doi.org/10.1038/s41558-020-00969-5, 2021.
Selimovic, V., Yokelson, R. J., Warneke, C., Roberts, J. M., de Gouw, J., Reardon, J., and Griffith, D. W. T.: Aerosol optical properties and trace gas emissions by PAX and OP-FTIR for laboratory-simulated western US wildfires during FIREX, Atmos. Chem. Phys., 18, 2929–2948, https://doi.org/10.5194/acp-18-2929-2018, 2018.
Selimovic, V., Yokelson, R. J., McMeeking, G. R., and Coefield, S.: In situ measurements of trace gases, PM, and aerosol optical properties during the 2017 NW US wildfire smoke event, Atmos. Chem. Phys., 19, 3905–3926, https://doi.org/10.5194/acp-19-3905-2019, 2019.
Sharma, S., Ishizawa, M., Chan, D., Lavoué, D., Andrews, E., Eleftheriadis, K., and Maksyutov, S.: 16-year simulation of Arctic black carbon: Transport, source contribution, and sensitivity analysis on deposition, J. Geophys. Res., 118, 943–964, https://doi.org/10.1029/2012jd017774, 2013.
Sierra-Hernández, M. R., Beaudon, E., Porter, S. E., Mosley-Thompson, E., and Thompson, L. G.: Increased fire activity in Alaska since the 1980s: Evidence from an ice core-derived black carbon record, J. Geophys. Res., 127, 2, https://doi.org/10.1029/2021jd035668, 2022.
Sinha, P. R., Kondo, Y., Koike, M., Ogren, J. A., Jefferson, A., Barrett, T. E., Sheesley, R. J., Ohata, S., Moteki, N., Coe, H., Liu, D., Irwin, M., Tunved, P., Quinn, P. K., and Zhao, Y.: Evaluation of ground-based black carbon measurements by filter-based photometers at two Arctic sites, J. Geophys. Res., 122, 3544–3572, https://doi.org/10.1002/2016jd025843, 2017.
Skamarock, W. C., Klemp, J. B., Dudhia, J., Gill, D. O., Liu, Z., Berner, J., Wang, W., Powers, J. G., Duda, M. G., Barker, D. M., and Huang, X. Y.: A description of the advanced research WRF model version 4, National Center for Atmospheric Research: Boulder, CO, USA, 145, https://app.paperpile.com/view/?id=570636aa-cb06-0251-a2af-4369411e08a5 (last access: 19 December 2024), 2019.
Stein, A. F., Draxler, R. R., Rolph, G. D., Stunder, B. J. B., Cohen, M. D., and Ngan, F.: NOAA's HYSPLIT Atmospheric Transport and Dispersion Modeling System, B. Am. Meteorol. Soc., 96, 2059–2077, https://doi.org/10.1175/BAMS-D-14-00110.1, 2015.
Stohl, A., Andrews, E., Burkhart, J. F., Forster, C., Herber, A., Hoch, S. W., Kowal, D., Lunder, C., Mefford, T., Ogren, J. A., Sharma, S., Spichtinger, N., Stebel, K., Stone, R., Ström, J., Tørseth, K., Wehrli, C., and Yttri, K. E.: Pan-Arctic enhancements of light absorbing aerosol concentrations due to North American boreal forest fires during summer 2004, J. Geophys. Res., 111, D22, https://doi.org/10.1029/2006jd007216, 2006.
Taketani, F., Miyakawa, T., Takashima, H., Komazaki, Y., Pan, X., Kanaya, Y., and Inoue, J.: Shipborne observations of atmospheric black carbon aerosol particles over the Arctic Ocean, Bering Sea, and North Pacific Ocean during September 2014, J. Geophys. Res., 121, 1914–1921, https://doi.org/10.1002/2015jd023648, 2016.
Taketani, F., Miyakawa, T., Takigawa, M., Yamaguchi, M., Komazaki, Y., Mordovskoi, P., Takashima, H., Zhu, C., Nishino, S., Tohjima, Y., and Kanaya, Y.: Characteristics of atmospheric black carbon and other aerosol particles over the Arctic Ocean in early autumn 2016: Influence from biomass burning as assessed with observed microphysical properties and model simulations, Sci. Total Environ., 848, 157671, https://doi.org/10.1016/j.scitotenv.2022.157671, 2022.
Thackeray, C. W. and Hall, A.: An emergent constraint on future Arctic sea-ice albedo feedback, Nat. Clim. Chang., 9, 972–978, https://doi.org/10.1038/s41558-019-0619-1, 2019.
Vasileva, A., Moiseenko, K., Skorokhod, A., Belikov, I., Kopeikin, V., and Lavrova, O.: Emission ratios of trace gases and particles for Siberian forest fires on the basis of mobile ground observations, Atmos. Chem. Phys., 17, 12303–12325, https://doi.org/10.5194/acp-17-12303-2017, 2017.
Wang, H., Rasch, P. J., Easter, R. C., Singh, B., Zhang, R., Ma, P.-L., Qian, Y., Ghan, S. J., and Beagley, N.: Using an explicit emission tagging method in global modeling of source-receptor relationships for black carbon in the Arctic: Variations, sources, and transport pathways, J. Geophys. Res., 119, 12888–12909, https://doi.org/10.1002/2014jd022297, 2014.
Wang, Q., Jacob, D. J., Fisher, J. A., Mao, J., Leibensperger, E. M., Carouge, C. C., Le Sager, P., Kondo, Y., Jimenez, J. L., Cubison, M. J., and Doherty, S. J.: Sources of carbonaceous aerosols and deposited black carbon in the Arctic in winter-spring: implications for radiative forcing, Atmos. Chem. Phys., 11, 12453–12473, https://doi.org/10.5194/acp-11-12453-2011, 2011.
van der Werf, G. R., Randerson, J. T., Giglio, L., van Leeuwen, T. T., Chen, Y., Rogers, B. M., Mu, M., van Marle, M. J. E., Morton, D. C., Collatz, G. J., Yokelson, R. J., and Kasibhatla, P. S.: Global fire emissions estimates during 1997–2016, Earth Syst. Sci. Data, 9, 697–720, https://doi.org/10.5194/essd-9-697-2017, 2017.
Whaley, C. H., Mahmood, R., von Salzen, K., Winter, B., Eckhardt, S., Arnold, S., Beagley, S., Becagli, S., Chien, R.-Y., Christensen, J., Damani, S. M., Dong, X., Eleftheriadis, K., Evangeliou, N., Faluvegi, G., Flanner, M., Fu, J. S., Gauss, M., Giardi, F., Gong, W., Hjorth, J. L., Huang, L., Im, U., Kanaya, Y., Krishnan, S., Klimont, Z., Kühn, T., Langner, J., Law, K. S., Marelle, L., Massling, A., Olivié, D., Onishi, T., Oshima, N., Peng, Y., Plummer, D. A., Popovicheva, O., Pozzoli, L., Raut, J.-C., Sand, M., Saunders, L. N., Schmale, J., Sharma, S., Skeie, R. B., Skov, H., Taketani, F., Thomas, M. A., Traversi, R., Tsigaridis, K., Tsyro, S., Turnock, S., Vitale, V., Walker, K. A., Wang, M., Watson-Parris, D., and Weiss-Gibbons, T.: Model evaluation of short-lived climate forcers for the Arctic Monitoring and Assessment Programme: a multi-species, multi-model study, Atmos. Chem. Phys., 22, 5775–5828, https://doi.org/10.5194/acp-22-5775-2022, 2022.
Wiggins, E. B., Soja, A. J., Gargulinski, E., Halliday, H. S., Pierce, R. B., Schmidt, C. C., Nowak, J. B., DiGangi, J. P., Diskin, G. S., Katich, J. M., Perring, A. E., Schwarz, J. P., Anderson, B. E., Chen, G., Crosbie, E. C., Jordan, C., Robinson, C. E., Sanchez, K. J., Shingler, T. J., Shook, M., Thornhill, K. L., Winstead, E. L., Ziemba, L. D., and Moore, R. H.: High temporal resolution satellite observations of fire radiative power reveal link between fire behavior and aerosol and gas emissions, Geophys. Res. Lett., 47, 23, https://doi.org/10.1029/2020gl090707, 2020.
Xie, A., Zhu, J., Kang, S., Qin, X., Xu, B., and Wang, Y.: Polar amplification comparison among Earth's three poles under different socioeconomic scenarios from CMIP6 surface air temperature, Sci. Rep., 12, 16548, https://doi.org/10.1038/s41598-022-21060-3, 2022.
Xu, J.-W., Martin, R. V., Morrow, A., Sharma, S., Huang, L., Leaitch, W. R., Burkart, J., Schulz, H., Zanatta, M., Willis, M. D., Henze, D. K., Lee, C. J., Herber, A. B., and Abbatt, J. P. D.: Source attribution of Arctic black carbon constrained by aircraft and surface measurements, Atmos. Chem. Phys., 17, 11971–11989, https://doi.org/10.5194/acp-17-11971-2017, 2017.
Yurganov, L. N., Jaffe, D. A., Pullman, E., and Novelli, P. C.: Total column and surface densities of atmospheric carbon monoxide in Alaska, 1995, J. Geophys. Res., 103, 19337–19345, https://doi.org/10.1029/97jd02299, 1998.
Zheng, B., Ciais, P., Chevallier, F., Yang, H., Canadell, J. G., Chen, Y., van der Velde, I. R., Aben, I., Chuvieco, E., Davis, S. J., Deeter, M., Hong, C., Kong, Y., Li, H., Li, H., Lin, X., He, K., and Zhang, Q.: Record-high CO2 emissions from boreal fires in 2021, Science, 379, 912–917, https://doi.org/10.1126/science.ade0805, 2023.
Zhu, C., Kanaya, Y., Takigawa, M., Ikeda, K., Tanimoto, H., Taketani, F., Miyakawa, T., Kobayashi, H., and Pisso, I.: FLEXPART v10.1 simulation of source contributions to Arctic black carbon, Atmos. Chem. Phys., 20, 1641–1656, https://doi.org/10.5194/acp-20-1641-2020, 2020.