the Creative Commons Attribution 4.0 License.
the Creative Commons Attribution 4.0 License.
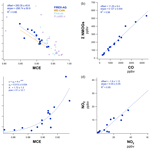
Parameterizations of US wildfire and prescribed fire emission ratios and emission factors based on FIREX-AQ aircraft measurements
Georgios I. Gkatzelis
Matthew M. Coggon
Chelsea E. Stockwell
Rebecca S. Hornbrook
Hannah Allen
Eric C. Apel
Megan M. Bela
Donald R. Blake
Ilann Bourgeois
Steven S. Brown
Pedro Campuzano-Jost
Jason M. St. Clair
James H. Crawford
John D. Crounse
Douglas A. Day
Joshua P. DiGangi
Glenn S. Diskin
Alan Fried
Jessica B. Gilman
Hongyu Guo
Johnathan W. Hair
Hannah S. Halliday
Thomas F. Hanisco
Reem Hannun
Alan Hills
L. Gregory Huey
Jose L. Jimenez
Joseph M. Katich
Aaron Lamplugh
Young Ro Lee
Jin Liao
Jakob Lindaas
Stuart A. McKeen
Tomas Mikoviny
Benjamin A. Nault
J. Andrew Neuman
John B. Nowak
Demetrios Pagonis
Jeff Peischl
Anne E. Perring
Felix Piel
Pamela S. Rickly
Michael A. Robinson
Andrew W. Rollins
Thomas B. Ryerson
Melinda K. Schueneman
Rebecca H. Schwantes
Joshua P. Schwarz
Kanako Sekimoto
Vanessa Selimovic
Taylor Shingler
David J. Tanner
Laura Tomsche
Krystal T. Vasquez
Patrick R. Veres
Rebecca Washenfelder
Petter Weibring
Paul O. Wennberg
Armin Wisthaler
Glenn M. Wolfe
Caroline C. Womack
Katherine Ball
Robert J. Yokelson
Carsten Warneke
Extensive airborne measurements of non-methane organic gases (NMOGs), methane, nitrogen oxides, reduced nitrogen species, and aerosol emissions from US wild and prescribed fires were conducted during the 2019 NOAA/NASA Fire Influence on Regional to Global Environments and Air Quality campaign (FIREX-AQ). Here, we report the atmospheric enhancement ratios (ERs) and inferred emission factors (EFs) for compounds measured on board the NASA DC-8 research aircraft for nine wildfires and one prescribed fire, which encompass a range of vegetation types.
We use photochemical proxies to identify young smoke and reduce the effects of chemical degradation on our emissions calculations. ERs and EFs calculated from FIREX-AQ observations agree within a factor of 2, with values reported from previous laboratory and field studies for more than 80 % of the carbon- and nitrogen-containing species. Wildfire emissions are parameterized based on correlations of the sum of NMOGs with reactive nitrogen oxides (NOy) to modified combustion efficiency (MCE) as well as other chemical signatures indicative of flaming/smoldering combustion, including carbon monoxide (CO), nitrogen dioxide (NO2), and black carbon aerosol. The sum of primary NMOG EFs correlates to MCE with an R2 of 0.68 and a slope of −296 ± 51 g kg−1, consistent with previous studies. The sum of the NMOG mixing ratios correlates well with CO with an R2 of 0.98 and a slope of 137 ± 4 ppbv of NMOGs per parts per million by volume (ppmv) of CO, demonstrating that primary NMOG emissions can be estimated from CO. Individual nitrogen-containing species correlate better with NO2, NOy, and black carbon than with CO. More than half of the NOy in fresh plumes is NO2 with an R2 of 0.95 and a ratio of NO2 to NOy of 0.55 ± 0.05 ppbv ppbv−1, highlighting that fast photochemistry had already occurred in the sampled fire plumes. The ratio of NOy to the sum of NMOGs follows trends observed in laboratory experiments and increases exponentially with MCE, due to increased emission of key nitrogen species and reduced emission of NMOGs at higher MCE during flaming combustion. These parameterizations will provide more accurate boundary conditions for modeling and satellite studies of fire plume chemistry and evolution to predict the downwind formation of secondary pollutants, including ozone and secondary organic aerosol.
- Article
(4905 KB) - Full-text XML
-
Supplement
(1152 KB) - BibTeX
- EndNote
Open biomass burning in the form of wildfires, prescribed forest management fires, and agricultural burns is one of the largest sources of trace gases and aerosols worldwide (Akagi et al., 2011; Crutzen and Andreae, 1990). It is the dominant global source of black carbon and primary organic aerosol (Bond et al., 2013) and accounts for more than 20 % of the global emissions of nitric oxide (NO) and carbon monoxide (CO) (Olivier et al., 2005; Yokelson et al., 2008; Wiedinmyer et al., 2011). It is the second-largest global source of non-methane organic gases (NMOGs) (Akagi et al., 2011) and a major source of greenhouse gases, including methane (CH4), carbon dioxide (CO2), and nitrous oxide (N2O) that impact the atmospheric carbon budget and climate (Sudo and Akimoto, 2007; Ward et al., 2012; Tian et al., 2016; Le Quéré et al., 2018).
During the last decade, the number of wildfires and prescribed fires in the United States has sometimes exceeded 74 000 and 450 000 yr−1, respectively (National Interagency Fire Center, 2015). Warming temperatures, drier climate, and a history of fire suppression are projected to increase the frequency and intensity of wildfires and lengthen fire seasons globally (Spracklen et al., 2009; Kloster et al., 2010; Pechony and Shindell, 2010; Moritz et al., 2012; Flannigan et al., 2013; Mann et al., 2016; Balch et al., 2017), which is already evident in the western United States, Canada, the eastern Mediterranean, Siberia, and Australia (Westerling et al., 2006; Keywood et al., 2013; Yue et al., 2015). Wildfires in the United States largely occur in the western conterminous states and Alaska and typically account for 12 000 to 40 000 km2 of the annual total area burned (National Interagency Fire Center, 2015). In the southeastern US, prescribed fires and agricultural burns are a common land management tool used to improve ecosystem health or facilitate planting crops (Wiedinmyer and Hurteau, 2010; Cochrane et al., 2012). Since prescribed fires in the southeast currently account for about 25 000 km2 yr−1 on average (National Interagency Fire Center, 2015), it is also important to characterize their emissions.
While wildfires and prescribed fires are favorable for many ecosystem functions, the atmospheric impacts of fire on climate, air quality, and health are a major concern. Particles directly emitted or formed via chemical processes have direct and indirect effects on climate by influencing the regional and global radiation balance and impacting cloud properties and precipitation (Braga et al., 2017; Cecchini et al., 2017; Hamilton et al., 2018; Thornhill et al., 2018; Kodros et al., 2020). Global mortality from outdoor pollution due to biomass burning smoke accounts for 600 000 premature deaths per year (Johnston et al., 2012), with particulate matter (PM) and O3 posing the greatest risk factors (Akagi et al., 2014; Dennekamp et al., 2015; Brey and Fischer, 2015; Knorr et al., 2017; Apte et al., 2018). In smoke plumes, O3 and secondary organic aerosols are photochemically produced from the interplay of NOx, NMOGs, and meteorology (Tsimpidi et al., 2017; Hodshire et al., 2019). An essential first step to elucidate the factors contributing to PM and O3 pollution downwind fires is to quantify primary gas- and particle-phase emissions.
Numerous studies have quantified emission factors (EFs; grams emitted per kg of dry fuel burned) for various fuel types and different fire characteristics using ground-based or airborne measurements in close proximity to wildland/prescribed fire plumes (e.g., Stockwell et al., 2016; Liu et al., 2017; Peng et al., 2020; Mouat et al., 2022; Lindaas et al., 2021; Permar et al., 2021) or controlled laboratory burns (e.g., Stockwell et al., 2014; Koss et al., 2018; Selimovic et al., 2018). Literature reviews to combine these results have been periodically conducted (Andreae and Merlet, 2001; Akagi et al., 2011; Andreae, 2019), with the most recent by Prichard et al. (2020). Nevertheless, uncertainties in the process-level understanding and model representation of fire emissions, plume rise, and chemistry still exist, which influence model performance in accurately capturing downwind O3 and secondary organic aerosol formation (Müller et al., 2016; Reddington et al., 2016; Shrivastava et al., 2017). These uncertainties can result from an insufficient understanding of the chemistry and total emissions of NOx and NMOGs across fuel types, ecosystems, and fire combustion conditions (Warneke et al., 2011; Yokelson et al., 2013; Hatch et al., 2017).
In this study, we calculate western US wildfire emission factors for a broad range of gas- and particle-phase species measured aboard the NASA DC-8 during the 2019 Fire Influence on Regional to Global Environments and Air Quality (FIREX-AQ) campaign, which included the most comprehensive payload to date for airborne sampling of biomass burning emissions. We compare our results to the most recent laboratory and airborne field studies, including the fire sciences laboratory component of FIREX-AQ (hereafter referred to as FireLab) (Koss et al., 2018); the fourth Fire Lab at Missoula Experiment, FLAME-4 (Stockwell et al., 2015); the Western Wildfire Experiment for Cloud Chemistry, Aerosol Absorption, and Nitrogen, WE-CAN (Permar et al., 2021); and the Studies of Emissions and Atmospheric Composition, Clouds and Climate Coupling by Regional Surveys, SEAC4RS (Liu et al., 2017; Wolfe et al., 2022), as well as results summarized in the review by Andreae (2019). We parameterize wildfire emissions based on correlations of carbon- and nitrogen-containing species to CO, NO2, black carbon, and modified combustion efficiency (MCE) to improve future modeling efforts to accurately capture the chemical evolution of wildfire smoke.
Platforms and instrumentation
The NASA DC-8 aircraft was deployed with an extensive suite of instruments to measure the gas- and particle-phase pollutants emitted and photochemically produced downwind of US wildfires. Figure 1 and Table 1 show the research flights analyzed here to capture freshly emitted wildfire smoke from 22 July to 3 September 2019. In total, 16 crosswind plume transects downwind from nine western wildfires and one eastern prescribed fire are analyzed, which represent a range of fuel types, including timber, grass, dead trees, logging debris, brush, and litter. The transects are selected based on aging proxies to examine emissions with minimal atmospheric processing. The physical age is determined based on transect proximity to the fire, an estimated plume rise time, and wind speed (Holmes et al., 2020) and ranged from 10–153 min (1–40 km) downwind for the plumes described here. The MCE, defined as , is commonly reported to quantify the fire conditions and describes the relative amount of flaming and smoldering combustion (Yokelson et al., 1996). Pure flaming fires have an MCE near 0.99, while smoldering fires vary over a wider range but are most often near 0.8 (Akagi et al., 2011). For the freshest plume crossings, the MCE was on average 0.90 ± 0.04 (range 0.94–0.85), suggesting a mix of flaming and smoldering emissions.
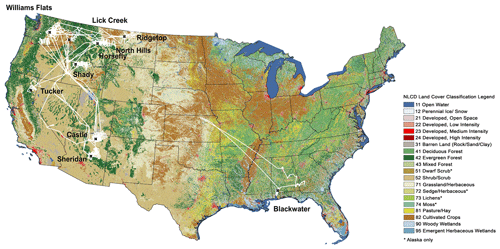
Figure 1Selected NASA DC-8 flight tracks for sampling the wildfire and prescribed fire plumes during the 2019 FIREX-AQ. Fires discussed in this study are denoted by black markers. The US map is colored by land cover classification.
Table 1Freshest plume crossings identified for analysis during FIREX-AQ 2019. Forest and shrubland fuel types were determined using the FCCS database, while cropland fires were classified with the Cropland Data Layer and DC-8 overflight videos (Warneke et al., 2022).
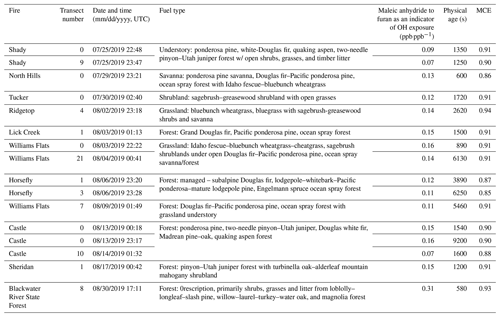
Multiple instruments performed measurements of gas- and particle-phase species summarized in Table 2. The University of Colorado aircraft aerosol mass spectrometer (CU HRAMS, AMS in the following) (Canagaratna et al., 2007; Guo et al., 2021) measured organic aerosol, particulate ammonium, and nitrate (pNOy) that consisted of inorganic nitrates (pNO3), organic nitrates (pRONO2), and nitroaromatics (pArNO2) (Day et al., 2022). Black carbon aerosol concentration was measured by a single-particle soot photometer (SP2) and scaled (∼ 10 %) to represent the total accumulation mode (Schwarz et al., 2008). NMOGs were measured by the NOAA proton transfer reaction time-of-flight mass spectrometer (PTR-ToF-MS) (Yuan et al., 2016); two whole-air samplers, namely the NOAA-integrated Whole-Air Sampler (NOAA iWAS; < 10 s sample time) (Lerner et al., 2017) and the University of California, Irvine Whole-Air Sampler (UCI WAS; < 40 s sample time) (Colman et al., 2001; Simpson et al., 2020); the NCAR Trace Organic Gas Analyzer (Apel et al., 2015), a fast online gas chromatograph outfitted with a time-of-flight mass spectrometer (TOGA-TOF; < 35 s sample time); the Caltech chemical ionization time-of-flight mass spectrometer (CIT-ToF-CIMS); and, for selected flights, the University of Innsbruck/University of Oslo (UIBK/UiO) PTR-ToF-MS (prototype PTR-TOF 4000X2; IONICON Analytik GmbH, Innsbruck, Austria). Three instruments were used in this study that measured formaldehyde: the In Situ Airborne Formaldehyde (ISAF) instrument (Liao et al., 2021), the Compact Atmospheric Multispecies Spectrometer (CAMS) (Weibring et al., 2007), and the UIBK/UiO PTR-ToF-MS. ISAF and CAMS correlated with an R2 coefficient of 0.99 and a slope of 1.27, as discussed by Liao et al. (2021), whereas the UIBK/UiO PTR-ToF-MS agreed better with the CAMS, with a slope of 1.02. In this study, we use the ISAF measurements which have the best time response compared to all other instruments and adjust the mixing ratios to match those reported by CAMS and the UIBK/UiO PTR-ToF-MS. The NOAA iodide chemical ionization mass spectrometer (NOAA CIMS) (Veres et al., 2020; Robinson et al., 2022) was used to measure formic acid (HCOOH), nitrous acid (HONO), and dinitrogen pentoxide (N2O5). CO and CH4 were measured via mid-IR wavelength modulation spectroscopy by the Differential Absorption Carbon Monoxide Measurement (DACOM) instrument (Sachse et al., 1991). CO2 was measured via nondispersive infrared absorption spectroscopy using a LICOR model 7000 analyzer (Vay et al., 2009). NO, NO2, and NOy were measured by the NOAA chemiluminescence instrument (Bourgeois et al., 2020). NOy measures the sum of reactive nitrogen compounds, including NO, NO2, HONO, peroxy nitrates, alkyl and multifunctional nitrates, and particulate nitrate. Additional measurements of HONO and NO2 were provided by the NOAA Airborne Cavity Enhanced Spectrometer (ACES) (Min et al., 2016) and NO by the NOAA laser-induced fluorescence instrument (NO-LIF) (Rollins et al., 2020). Glyoxal and methylglyoxal were measured by ACES, and ammonia (NH3) by the UIBK/UiO PTR-ToF-MS (Müller et al., 2016; Tomsche et al., 2023). The Georgia Tech CIMS (GT-CIMS) was used to measure peroxyacetyl nitrate (PAN) and other PAN-like compounds such as peroxylpropionyl nitrate, peroxyacryloyl nitrate, and peroxylbutyryl nitrate. Finally, the plume structure was obtained from aerosol backscatter measured with the NASA Langley Airborne Differential Absorption Lidar (DIAL). All measurements reported here are provided in the NASA FIREX-AQ data repository (Atmospheric Science Data Center, 2019).
In this study, we focus on quantifying total and speciated NMOG emissions, which were predominantly measured by PTR-ToF-MS, the two whole-air samplers, and the Trace Organic Gas Analyzer with Time-of-Flight mass spectrometer (TOGA-TOF). The same NOAA PTR-ToF-MS and the iWAS systems were used at the US Forest Service's Missoula Fire Sciences Laboratory (FireLab) in 2016 as a precursor to FIREX-AQ and described by Koss et al. (2018). Koss et al. (2018) speciated isomers measured by PTR-ToF-MS using gas chromatography pre-separation and reported isomer distributions for over 150 individual masses. Here, we compare these isomer distributions to the speciation derived based on the comparison of the GC-MS and PTR-ToF-MS measurements conducted aboard the NASA DC-8 (Table S5 in the Supplement). Two calibration methods were used to determine NMOG sensitivities for the PTR-ToF-MS. For commercially available compounds, sensitivities were determined by gravimetrically prepared standards or by liquid calibration, as described by Coggon et al. (2019). Sensitivities for other species were estimated based on calculated proton transfer rate coefficients, as described by Sekimoto et al. (2017). For the WAS system(s), NMOGs were calibrated using gravimetrically prepared standards, as described by Lerner et al. (2017). A detailed description of the PTR-ToF-MS and WAS setups as well as NMOG uncertainty is included in the Supplement.
3.1 Plumes with minimal photochemical aging
Emissions from wildfire plumes chemically transform once injected into the atmosphere (e.g., Akagi et al., 2012; Robinson et al., 2021; Decker et al., 2021; Xu et al., 2021). However, safety and operational constraints limit the proximity of airborne sampling to the fire. An essential first step to quantifying wildfire primary emissions is to identify plume samples that have undergone minimal chemical processing. Commonly, the freshest plumes are identified using the plume age calculated from the distance downwind of the wildfire using the average wind speed measured on board (e.g., Permar et al., 2021) but neglecting plume rise. The physical age does not necessarily identify plume crossings with the least chemical processing since the sampled smoke can be impacted by meteorology, solar radiation, radical concentrations, and sampling artifacts related to the aircraft's position relative to the center of the plume (Robinson et al., 2021; Decker et al., 2021; Wang et al., 2021).
Here, we account for oxidation by hydroxyl radical (OH) using the ratio of primary and secondary NMOG wildfire tracers, specifically furan (a primary species; Koss et al., 2018) and maleic anhydride (a slow-reacting, secondary species observed downwind of fires) (Zhao and Wang, 2017). Coggon et al. (2019) show that maleic anhydride quickly forms downwind of fires from the OH oxidation of furans, and Wang et al. (2021) show that the distribution of maleic anhydride in plumes closely mirrors the distribution of OH exposure. Since furan is a direct wildfire emission, and maleic anhydride is a chemical product of furan chemistry that is not significantly emitted from fires (Coggon et al., 2019; Wang et al., 2021), the ratio of maleic anhydride to furan (MA F) is expected to increase downwind of a fire and exhibit a minimum in the least-processed plumes. This ratio is used as a photochemical proxy to identify the freshest sampled plumes by extracting the lowest MA F transect per wildfire plume and reduce the effects of chemical degradation on our primary NMOG emission calculations. We note that this technique may not account for the faster photolysis of light-absorbing species (such as HONO) or fast interconversion between NO and NO2, though the sum of reactive nitrogen species (NOy) is expected to be conserved downwind of fires (Lindaas et al., 2021). We note that a quantitative relationship between MA F and OH exposure is not presented here as the yield of maleic anhydride from furan oxidation requires further laboratory quantification. Other furans also produce maleic anhydride (Coggon et al., 2019), and thus the MA F ratios used here are simply a proxy for screening out significantly processed emissions.
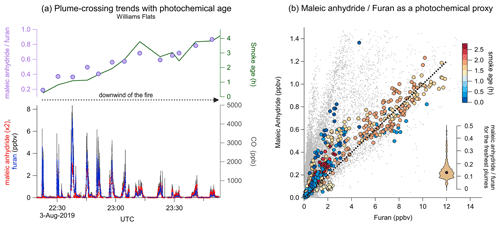
Figure 2(a) Mixing ratios of maleic anhydride, furan, and CO (bottom) and ratios of maleic anhydride to furan (top) in 12 crosswind plume transects of smoke from the Williams Flats fire on 3 August 2019. The maleic anhydride furan ratio increases as the plume ages during transport away from the Williams Flats. (b) Comparison of the maleic anhydride and furan mixing ratios used as a photochemical proxy to identify the freshest plume crossings during FIREX-AQ. Grey points are all 1 s resolution measurements during FIREX-AQ, and circles are the chosen freshest plume crossings colored by the physical smoke age. The violin plot shows the variability of the ratio of maleic anhydride to furan for the freshest wildfire transects.
Figure 2a shows the maleic anhydride, furan, and CO concentration downwind of the Williams Flats wildfire on 3 August 2019, as a characteristic example. Figure 2b shows the relationship between maleic anhydride and furan for all of the plume crossings sampled during FIREX-AQ. The freshest crossings for each fire are highlighted as circles colored by the estimated smoke age. Also shown are the MA F and the median physical smoke age calculated for each plume crossing. Here we use the high time resolution of PTR-ToF-MS for MA and furan concentrations, but furan is additionally scaled by 0.46 to match the TOGA GC-MS concentrations as discussed in Sect. 3.2. Volatile organic compound (VOC) and CO concentrations were highest closer to the wildfire and decreased downwind, primarily due to dilution. During the Williams Flats fire (Fig. 2a), the MA F increased from 0.20 to 0.86 downwind of the fire, indicating active chemical conversion of furan to maleic anhydride. The physical smoke age followed the same increase from 0.5 to 4 h. Figure 2b shows that the MA F for all of the freshest plume crossings had a median of 0.13 (0.10–0.16, 25th–75th), and their corresponding physical age was less than 1.46 h (0.6–1.74) (see Table 1). It is notable that certain fires with similar MA F ratios ranged in physical age from 15 min to as high as 3–4 h. These differences show how chemical processing in some plumes may be slow over long distances, while other plumes may undergo immediate oxidation. Despite these differences, the majority of chemically fresh plumes sampled during FIREX-AQ exhibited very similar MA F ratios (Fig. 2b).
Figure S1 in the Supplement further highlights differences in the physical and chemical age of a fire by focusing on the Williams Flats wildfire and the Blackwater prescribed fire. The DIAL image shows the shape and evolution of the wildfire smoke from overpass flights. For the Williams Flats fire, the DC-8 sampled emissions by performing raster patterns perpendicular to the smoke, whereas for the Blackwater fire, the DC-8 also flew along the smoke plume at various altitudes. For the Blackwater fire, the MA F increased rapidly up to 1.4 ppbv ppbv−1 30 km downwind of the wildfire, while for the Williams Flats fire, the ratio reached a maximum of 1 ppbv ppbv−1 120 km downwind of the fire. These differences further highlight the importance of accounting for the chemical rather than the physical age of a fire to determine the freshest transects.
The MA F for fresh, unaged smoke during the FireLab study was ∼ 0.04 ppbv ppbv−1 (Wang et al., 2021), showing that even the freshest plume transects sampled during FIREX-AQ were photochemically processed to some extent. For the remainder of this analysis, fire plumes sampled closest to the emission source that exhibited a MA F > 0.20 are excluded from the calculation of emission ratios and enhancements. This cutoff is based on the median MA F ratio observed for the freshest plume plumes sampled during FIREX-AQ (0.13 ppbv ppbv−1, Fig. 2b). The exception is the Blackwater prescribed fire that was the only fire representative of southeastern US fuel types included in our analysis, even though the freshest plume crossing had a MA F of 0.3. Further evaluation of biases during FIREX-AQ for fast-reacting species is discussed in Sect. 3.3.
3.2 Instrument comparisons
NMOG measurements obtained from the NOAA PTR-ToF-MS were compared to other instruments on board the DC-8, including TOGA-TOF, two WAS systems, CIT-CIMS, UIBK/UiO PTR-ToF-MS, and NOAA CIMS. Table S5 provides correlations of the PTR-ToF-MS measurements to other instruments. For calibrated compounds, the NOAA PTR-ToF-MS and the UIBK/UiO PTR-ToF-MS agreed within 10 %–35 % for methanol, acetonitrile, acetone, methyl ethyl ketone (MEK), benzene, toluene, C8 and C9 aromatics, and monoterpenes. The NOAA PTR-ToF-MS and NOAA CIMS agreed within uncertainty for hydrogen cyanide (HCN), isocyanic acid (HNCO), and formic acid, respectively. CIT-CIMS agreed with the NOAA PTR-ToF-MS for HCN, whereas for phenol it was lower by a factor 2. Both instruments were calibrated for phenol, suggesting that differences could be due to PTR-ToF-MS fragmentation of higher-molecular-weight gases that produce signals at the phenol ion mass or differences in the detection of other isomers from the two instruments.
Although the PTR-ToF-MS provides high time resolution measurements, it cannot speciate NMOG isomers detected at the same exact mass. In the following, we compare mixing ratios derived for the PTR-ToF-MS chemical formula to the combined isomer signals derived from GC-MS, given in parentheses. When compared to the iWAS, WAS, and TOGA-TOF measurements, the NOAA PTR-ToF-MS was within ±25 %–35 % for CH4O (methanol), C2H3N (acetonitrile), C2H4O (acetaldehyde), C2H6O (ethanol), C6H6 (benzene), C7H8 (toluene), C3H3N (acrylonitrile), C3H4O (acrolein), C3H6O (acetone + propanal), C8H10 (ethylbenzene + m-xylene, p-xylene, and o-xylene), and C4H6O (methyl vinyl ketone + methacrolein + 2-butenal). However, the NOAA PTR-ToF-MS was higher by a factor of 2 or more for C2H6S (dimethyl sulfide), C4H5N (pyrrole + butene nitrile isomers), C4H4O (furan), C3H6O2 (methyl acetate + ethyl formate + hydroxyacetone), C5H6O (2-methyl-furan + 3-methyl-furan), C5H4O2 (furfural + 3-furaldehyde), and C10H16 (monoterpenes), whereas CH3NO2 (nitromethane) agreed with the WAS but was lower than TOGA-TOF.
The discrepancies between the GC-MS techniques and PTR-ToF-MS for a number of key species, such as furans, generally show that the PTR-ToF-MS measures more signal than what can be accounted for by GC-MS. This observation likely results from a combination of (a) PTR-ToF-MS fragmentation of higher-molecular-weight gases that produce signals at parent ion masses, (b) the detection of isomers that cannot elute through a GC column, and (c) the detection of molecules that are lost to canister sampling. To investigate the causes of these discrepancies, Table S5 shows isomer distributions for masses detected by the PTR-ToF-MS that are known to represent the sum of two or more overlapping isomers. These isomer distributions are calculated from the ratio of GC-MS measurements to the corresponding PTR-ToF-MS mass. Each ratio represents the fraction of the total signal measured by PTR-ToF-MS that is associated with a given isomer. For example, GC-MS measurements identify 2-methylfuran and 3-methylfuran as the key isomers with the molecular formula C5H6O. The slope of isomers to PTR-ToF-MS measurements of C5H6O represents the isomer fraction detected by PTR-ToF-MS.
The isomer distributions shown in Table S5 are compared to those reported for laboratory smoke by Koss et al. (2018). Koss et al. (2018) assigned PTR-ToF-MS masses based on literature searches, intercomparisons of PTR-ToF-MS measurements to other in situ instrumentation, and offline analysis by coupling GC effluent of sampled smoke to the inlet of the PTR-ToF-MS (combined instrumental setup termed GC-PTR-ToF-MS). For low molecular weight gases known to elute through a GC column, Koss et al. (2018) assigned isomer distributions based on the total signal detected by GC-PTR-ToF-MS, which includes signals from parent ions produced from proton transfer as well as fragments from higher-molecular-weight gases that elute through a GC. For example, at C5H6O–H+ ( 83.0491), 51 % of the signal resulted from the elution of 2-methylfuran, 9 % resulted from 3-methylfuran, and 37 % was associated with other peaks in the chromatogram that produced signals at C5H6O–H+ (unidentified isomers + fragments of higher masses). We note that the PTR-ToF-MS instrument employed in this study is the same as that used by Koss et al. (2018) and is operated with the same drift field ( = 120 Td).
For species measured during FIREX-AQ where the PTR-ToF-MS reported significantly more mass than the GC instruments, we find that the isomer distributions derived in this study significantly differ from those derived by Koss et al. (2018) (Table S5). This is most pronounced for the monoterpenes but also the furanoic species, such as furan (C4H4O), methylfurans (C5H6O), and furfurals (C5H4O2). Hatch et al. (2017) showed that more than 30 different isomers can contribute to the monoterpenes signal based on two dimensional GC. However, the conventional GC instruments used during FIREX-AQ could only detect a fraction of these isomers. Furthermore, differences in sensitivity for the different isomers would further increase the quantification uncertainties for both GC and PTR-ToF-MS. For the furanoic masses, the PTR-ToF-MS measures a higher fraction of unknown isomers and fragments than what is reported by Koss et al. (2018). This result holds whether comparing against isomer distributions derived using TOGA (an online GC method) or WAS methods (a canister sampling method), suggesting that uncertainties due to differences in calibration are small. These results suggest that the total signal of furans measured by PTR-ToF-MS during FIREX-AQ is likely influenced by gases that cannot pass through a GC column, which includes the possibility of unidentified isomers and fragments from higher-molecular-weight species. We note that this result is not specific to the PTR-ToF-MS used in this study, as the agreement between the NOAA PTR-ToF-MS and UIBK/UiO PTR-ToF-MS for these masses is within 3 % (Table S5).
Furans are an important contributor to VOC reactivity and significantly contribute to the formation of ozone and other secondary gases (Gilman et al., 2015; Hatch et al., 2017; Coggon et al., 2019). For models employing emission factors of furans, we recommend using emission factors derived using GC-based methods given that multiple isomers can be detected with PTR-TOF-MS at the furan mass. This also applies to other specific compound classes. In Table S1, we include the methods used in this study to derive emission factors. For applications where the fast time resolution from PTR-ToF-MS is needed (e.g., in deriving cross-plume trends in gases) (Decker et al., 2021; Xu et al., 2021), the interpretation of trends in furans should include the possibility of unknown isomers and fragments.
3.3 Emission ratios and emission factors of US wildfire smoke
The freshest plume transects are used to estimate the primary emissions for individual fires. Table 3 shows the average compound-specific enhancement ratios to CO which we interpret as emission ratios (ERs) for most species and the inferred emission factors (EFs) calculated for more than 100 species and groups of species from the freshest wildfire plume transects sampled during FIREX-AQ. ERs and EFs for each fire are also calculated and provided in Tables S2 and S3. Given that fast chemistry already occurred in some fire transects, the ER and EF estimates of highly reactive species like HONO are lower bounds. ERs are the slope of a linear fit of each species with CO mixing ratios (see Sect. S1). EFs were calculated following Eq. (1):
where EFi is the emission factor of compound i calculated similarly to Akagi et al. (2011), FC is the carbon fraction of the fuel assumed to be 0.5 g g−1, MMi is the molar mass of i, AWC is the atomic mass of carbon (12 g mol−1), CO is the emission ratio of a compound relative to CO, NCx is the number of carbon atoms in C-containing species x, and CO is the emission ratio of species x to CO. This method assumes that all the carbon lost from the fuel as it burns is emitted and measured, which is a reasonable approximation as CO, CO2, and CH4 account for most of the emitted carbon (Akagi et al., 2011). The denominator of the last term estimates total carbon relative to CO. Species Cx includes all species shown in Table 3. The carbon not quantified by the suite of instrumentation available during FIREX-AQ likely results in emission factor overestimates of no more than 1 %–2 % (Yokelson et al., 2013; Stockwell et al., 2015).
Table 3Emission ratios and emission factors of organic and nitrogen compounds from wildfire plumes. In italic are multiple isomers measured as a sum by the NOAA PTR-ToF-MS that were further speciated based on other GC-MS measurements from FIREX-AQ (column 1 in parentheses). Here, we show the ratio of each isomer measured by GC-MS to the total PTR-ToF-MS signal obtained in this mass.
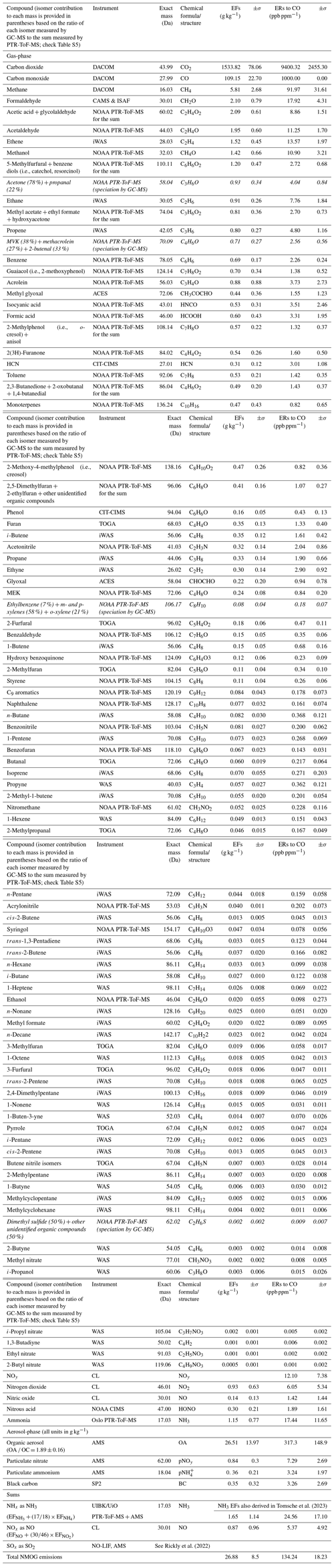
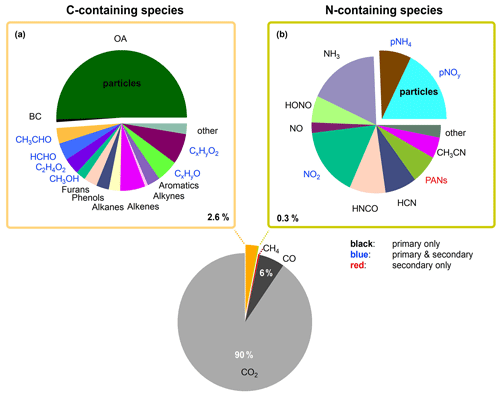
Figure 3Pie charts of carbon- and nitrogen-containing species average emission factors (g kg−1) for fresh wildfire smoke. The text labels indicate compounds with only direct emissions in black, compounds that are directly emitted and photochemically produced in blue, and PANs that are only photochemically produced in red, indicating some oxidation, even for the freshest plumes sampled. Although HCHO and CH3CHO are CxHyO species, and glycolaldehyde/acetic acid are CxHyO2 species, they are separately presented due to their high abundances.
Figure 3 shows the average chemical composition of freshly emitted wildfire smoke (in g kg−1; see Eq. 1). CO2, CO, and CH4 are 97 % of the total mass. The remaining 3 % consisted of gas- and particle-phase carbon-containing (C-containing, 2.6 %) and nitrogen-containing (N-containing, 0.3 %) species. A total of 50.4 % and 0.7 % of this remaining C-containing total mass results from organic aerosol and black carbon (BC), respectively. In the gas phase, 6.4 % of the remaining C-containing species mass, which includes all species in Fig. 3a, was phenolic compounds and furans, 4 % formaldehyde (HCHO), 4 % glycolaldehyde and acetic acid (C2H4O2), 3.7 % acetaldehyde (CH3CHO), 2.1 % methanol, 5.8 % remaining compounds with one oxygen atom (CxHyO), 6.9 % remaining compounds with two oxygen atoms (CxHyO2), 3.1 % aromatics, 6.3 % alkenes, 2.8 % alkanes, and 3.3 % other species. N-containing species mass, shown in Fig. 3b, consisted of organic and inorganic nitrate and other organic nitro compounds such as nitroaromatics (pNOy, 19 %) and ammonium (pNH, 8.5 %) in the particle-phase, whereas, the dominant gas-phase N-containing species mass was from ammonia (NH3, 18.5 %), followed by nitrogen dioxide (NO2, 17.5 %), isocyanic acid (HNCO, 8.5 %), hydrogen cyanide (HCN, 5 %), peroxyacyl nitrates (PANs, 7 %), nitrous acid (HONO, 4.8 %), nitric oxide (NO, 2.5 %), and others at 3 %. The high contribution of NO2 in comparison to NO and HONO and the existence of secondary pollutants, in particular PANs, also indicate that chemistry occurred from the time of emission to the time of detection. Given the fast conversion of NO and HONO to NO2 and nitrate and NH3 to particulate ammonium, we also include the conserved quantity of NOy in Table 3, as well as NOx as NO and NHx as NH3 + particulate ammonium. Emissions of SOx as SO2 that include the conversion of SO2 to particulate sulfate are discussed in Rickly et al. (2022).
3.4 FIREX-AQ field observations compared to laboratory and field studies
The sum of the NMOG EFs sampled during the FIREX-AQ campaign was 26.88 ± 8.5 g kg−1 (3σ), in agreement with the mean sum from western wildfires during the WE-CAN campaign of 26.1 ± 6.9 g kg−1 (Permar et al., 2021), temperate forest fires at 23.7 g kg−1 (Akagi et al., 2011) and 24.55 g kg−1 (Andreae, 2019), pine-forest understory prescribed fires at 27.6 g kg−1 (Yokelson et al., 2013), FLAME-4 laboratory coniferous canopy fires at 23.9 g kg−1 (Stockwell et al., 2015), and FireLab measurements of various different fuel types at 25 g kg−1 (Koss et al., 2018). The sum of FIREX-AQ NMOG ERs to CO on a molar basis was 134.2 ± 20 ppb ppm−1, in a similar range as WE-CAN at 148.3 ± 29.6 ppb ppm−1 and FireLab at 144.5 ppb ppm−1.
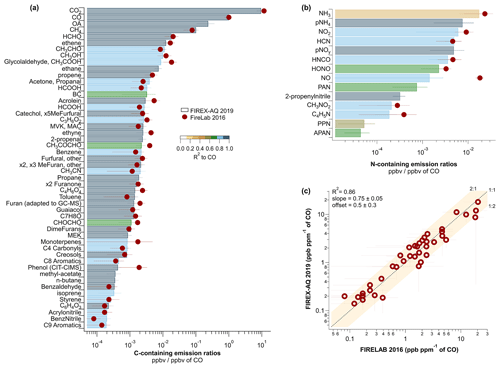
Figure 4Panels (a) and (b) show the emission ratios for FIREX-AQ (bars) and FireLab (circles) colored by the correlation coefficient and (c) the direct comparison of FIREX-AQ to FireLab emission ratios for gas-phase species. Error bars in all graphs indicate the 1σ standard deviation. The majority of the observations from FireLab 2016 were calculated using data from the NOAA PTR-ToF-MS; here we use measurements from the same instrument for FIREX-AQ for more direct comparisons.
Figure 4 compares the ERs of C-containing and N-containing compounds (ppb ppb−1 CO) with those measured at the FireLab (Koss et al., 2018; Selimovic et al., 2018). During FIREX-AQ, all NMOGs correlated well with CO with correlation coefficients R2 above 0.75, confirming that CO could be used as a proxy for estimating NMOG emissions close to the fire, as further discussed in Sect. 3.5. Variability in the correlations of individual species with CO was still evident – for example, species that are both emitted and photochemically produced exhibited lower correlation (e.g., acetic acid, acetone, and formic acid, R2 = 0.75–0.85) than compounds with only primary emissions from fires (e.g., aromatics, R2 > 0.95). N-containing species were weakly correlated with CO partly due to varying fuel N C (Roberts et al., 2020). In addition, lower correlation of NH3 could be due to variable amounts of ammonium formation in aging smoke or differences in instrument response times between a high-volatility compound, such as CO, compared to NH3, which may partition to the inlet and instrument walls before detection (Tomsche et al., 2023; Stockwell et al., 2014) and slow the instrument response time. Low correlations are also found for HONO, which is highly reactive and removed by photochemistry (Peng et al., 2020; Theys et al., 2020), as well as for glyoxal and methylglyoxal, which are photochemically formed and could partition differently to the particle phase depending on humidity (Mitsuishi et al., 2018; Ling et al., 2020). N-containing species were in good agreement except the higher contribution of NO and particulate ammonium in FireLab and FIREX-AQ, respectively. This difference reflects the depletion of NO and the secondary formation of particulate ammonium in field observations and promotes the finding that fast chemistry of reactive compounds occurred prior to the FIREX-AQ sampling. In summary, variability in post-emission processes, fuel nitrogen, and fast photochemistry are likely important factors that contribute to the differences in correlations between FIREX-AQ and FireLab measurements of NMOGs, NOy species, and CO.
While the PTR-ToF-MS is well-suited for detecting NMOGs, it is prone to fragmentation for a range of molecules, depending on their molecular structure (Pagonis et al., 2019). For such compounds, measurement uncertainties increase, and comparisons to previous studies that use different instrumentation become more challenging. As outlined in Sect. 2, the NOAA PTR-ToF-MS used in this study was the same instrument as used in the FireLab 3 years prior (Koss et al., 2018). This provided an important opportunity to compare field-derived emissions to laboratory studies. FireLab average ERs were calculated by comparing similar fuel types as measured during FIREX-AQ, including ponderosa pine, lodgepole pine, Douglas fir, subalpine fir, Engelmann spruce, loblolly pine, Jeffrey pine, juniper, manzanita, chamise, and bear grass laboratory burns. Overall, FIREX-AQ ERs agree with those from the FireLab within a factor of 2 for most compounds (see Fig. S3). Compounds with the largest differences were benzonitrile with a FIREX-AQ to FireLab ratio of 2.46, ethene (1.88), CH3CN (1.77), toluene (1.71), HCOOH (1.64), the sum of acetone and propanal (1.62), glycolaldehyde and acetic acid (0.50), monoterpenes (0.49), C4H5N species (0.47), syringol (0.32), and ethanol (0.28).
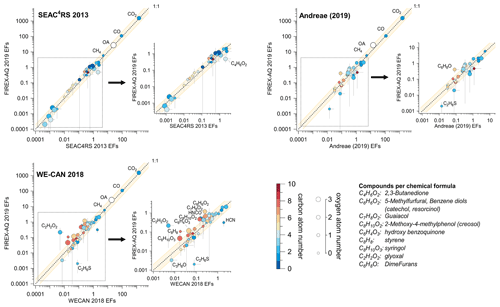
Figure 5Comparison of FIREX-AQ EFs to those from SEAC4RS 2013 (Liu et al., 2017), WE-CAN 2018 (Permar et al., 2021), and the review publication by Andreae (2019). Shaded areas show differences within a factor of 2.
Figure 5 and Table S6 compare FIREX-AQ observations against field-derived wildfire EFs from SEAC4RS (Liu et al., 2017), WE-CAN (Permar et al., 2021), and literature-average temperate forest EFs from Andreae (2019). For all studies, the measurements agree within a factor of 2 for 83 %, 87 %, and 78 % of the compounds reported during SEAC4RS, WE-CAN, and the Andreae (2019) temperate forest fire average (includes SEAC4RS), respectively. FIREX-AQ EFs were on average higher compared to previous studies. The average ratios (±1σ) of FIREX-AQ to WE-CAN, SEAC4RS, and temperate forest fires from Andreae (2019) were 1.42 ± 0.3, 1.26 ± 0.42, and 1.24 ± 0.36, respectively (see Table S6). Glyoxal and methylglyoxal were expected to have higher discrepancies due to their secondary production and relative-humidity-dependent particle-phase partitioning but also due to the higher quantification uncertainties in the previous studies. For example, during WE-CAN (Permar et al., 2021), a PTR-ToF-MS was used to detect these compounds, which are prone to fragmentation upon ionization in the PTR-ToF-MS. Furthermore, the calculated glyoxal sensitivity used by Permar et al. (2021) was high (Stönner et al., 2017) and could therefore lead to a significant underestimation. In this study, glyoxal and methylglyoxal were measured by cavity-enhanced spectroscopy, and the uncertainties were < 5 % (see Sect. 2). Furthermore, comparison of the FIREX-AQ to the FireLab EFs also measured by the same spectroscopic technique (see Fig. 4) (Zarzana et al., 2018) showed that glyoxal and methylglyoxal were in better agreement with FIREX-AQ compared to Permar et al. (2021) but still lower by 50 % and 75 %, respectively. Dimethyl sulfide (DMS) is a compound that originates predominantly from oceanic emissions, and its fire emissions were lower for this study compared to WE-CAN and the temperate forest fire emissions average but higher by 20 % compared to the SEAC4RS EFs. FIREX-AQ monoterpenes were higher than those in WE-CAN and FireLab by a factor of 2 and lower than the temperate forest fire emissions average (Andreae, 2019) by a factor of 2, which likely stems from the large variability of monoterpene emissions for different fuel types and the difficulties inherent with the large number of isomers (Hatch et al., 2017; Koss et al., 2018; Sekimoto et al., 2018). OA was 50 % higher compared to WE-CAN and temperate forest fire emissions but within 10 % when compared to the SEAC4RS OA emissions. Some of the OA estimates that went into the Andreae (2019) averaged OA EF value were calculated from OC with an assumed OA : OC value of 1.6, lower than the value of 1.89 used in this work (Table 3); while a correction of Andreae's data is not possible since it is not transparent which studies included in that compilation are affected, this will result in a small high bias in O : C. The variability of OA EFs highlights the importance of accounting for the partitioning and aging of OA when comparing OA EFs across biomass burning campaigns given that the fraction of the detected OA from wildfire plumes can be a mix of primary and secondary (Pagonis et al., 2020).
Focusing on the two large recent campaigns dedicated to wildfires, we note that differences can occur due to natural variability with 2018 being a more intense fire season (Jin et al., 2023) but also from the different fragmentation, inlet setups, and quantification uncertainties between the instruments used. Differences between the WE-CAN and FIREX-AQ EFs for oxygenated compounds could be due to the different quantification uncertainties between the two PTR-ToF-MS instruments. For both studies and instruments, assuming similar isomer sensitivities and no fragmentation interferences, sensitivities for calibrated compounds introduced a 15 % uncertainty, whereas sensitivities for uncalibrated species were estimated following theoretical methods described by Sekimoto et al. (2017), which have an uncertainty of 50 %. Several reactive oxygenated compounds that have implications for NOx loss processes such as the formation of nitrophenolic compounds (Finewax et al., 2018; Decker et al., 2021) were calibrated during FIREX-AQ but only calculated during WE-CAN, such as C7H8O (o-cresol, anisol), C7H8O2 (guaiacol), and C8H10O2 (creosol). One mass calibrated on both instruments was C6H6O2 (sum of 5-methyl-furfural, catechol, and resorcinol) but was still a factor of 5 higher during FIREX-AQ compared to WE-CAN. However, the FIREX-AQ ERs for C6H6O2 agreed within 45 % of the FireLab study, which used the same instrument, suggesting possible differences in fragmentation or isomer assignment between the FIREX-AQ and WE-CAN instruments. Styrene (C8H8) from FIREX-AQ (using PTR-MS) was a factor of 6 higher compared to the WE-CAN measurements (GC-MS) but agreed within 60 % with SEAC4RS (GC-MS) and FireLab EFs (PTR-MS). C6H8O (sum of 2,5-dimethylfuran, 2-ethylfuran, and other C2-substituted furan isomers), C8H10O3 (syringol), and C6H4O3 (hydroxy benzoquinone) were quantified using estimated calibration factors during both campaigns and therefore more uncertain and were higher by a factor of 2–5 during FIREX-AQ. Another influencing factor for the overall higher EFs for oxygenated compounds during FIREX-AQ could be due to the optimized inlet setups to limit wall losses prior to detection for the majority of the instruments (Table 2). Various oxygenated compounds are more analytically sticky and can therefore partition to the inlet line walls prior to their detection. For example, during FIREX-AQ the NOAA PTR-ToF-MS inlet line was 1 m long and heated at 60 ∘C to reduce condensation sinks resulting in less than 1 s residence times; in FireLab (Koss et al., 2018) a longer 16 m transfer line was used at 40 ∘C with a residence time comparable to FIREX-AQ, whereas in WE-CAN (Permar et al., 2021) the smoke to drift tube time was higher (∼ 2 s) at temperatures of 55–60 ∘C. This could therefore contribute to differences for larger or more oxygenated NMOGs between campaigns and partly explain the overall increased EFs during FIREX-AQ.
Further differences between FIREX-AQ and WE-CAN may also result from the methods used to identify and characterize young plumes. As described in Sect. 3.1, fresh plumes are identified during FIREX-AQ based on chemical aging proxies, whereas fresh plumes identified in WE-CAN are based on physical distance downwind. For highly reactive species, such as furans and oxygenated aromatics, strong fire-to-fire variability in OH exposure may alter emission factors, even in smoke with similar downwind age. Figure S2 compares the FIREX-AQ and WE-CAN field observations to the ERs obtained during the FireLab study for a variety of overlapping NMOGs with varying reactivities towards OH radicals. Given that FireLab experiments were performed under dark and warmer conditions in smoke aged just 5 s, it is expected that the more reactive compounds would show higher ERs when compared to field observations if the sampled smoke on board the aircraft was already aged. However, higher ERs were observed for various compounds measured during FIREX-AQ. In contrast, when comparing WE-CAN to FireLab ERs, the highly reactive compounds were lower, although the ERs of less reactive compounds were in good agreement. This indicates possible differences between FIREX-AQ and WE-CAN owing to variability in chemical oxidation, which has the largest impact on highly reactive species.
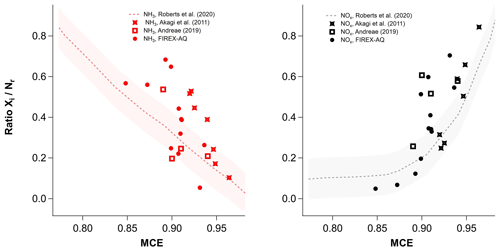
Figure 6Ratios of two N species to the total nitrogen, Nr, during FIREX-AQ compared to Roberts et al. (2020) based on a subalpine fir fire burned during FireLab and Andreae (2019) and Akagi et al. (2011) that include different land cover types.
The correlation to MCE for each species EFs was calculated for all wildfires as shown in Table S4 and compared to the WE-CAN observations. Correlation coefficients (R2) during FIREX-AQ were above 0.5 for 28 % of the species, 0.3–0.5 for 27 % of the species, and below 0.3 for the remaining species. The lowest correlations, below 0.1, were found for N-containing species, including particulate ammonium and pNOy, ammonia, acetonitrile, 2-butyl nitrate, methyl nitrate, pyrrole and butene nitrile isomers, and acrylonitrile. Nevertheless, agreement within a factor of 2 was found when compared to the slopes and R2 obtained from the WE-CAN campaign for most of the compounds. Figure 6 shows the dependence of two N-containing species on fire MCEs for the FIREX-AQ and FireLab (Roberts et al., 2020) studies as well as for a majority of fuel types by Akagi et al. (2011) and Andreae (2019). We report N-containing species as a ratio to the total reactive nitrogen Nr, defined as the sum of NO, NO2, HONO, HNCO, HCN, NH3, other N-containing VOCs, and particle-phase nitrate and ammonium. The dotted lines and shaded regions show FireLab parameterizations that describe how these ratios respond to changes in MCE (Roberts et al., 2020) for one subalpine fir fire burned during FireLab, whereas square and bent square markers indicate different land cover types from Andreae (2019) and Akagi et al. (2011), respectively. It should be noted that for Akagi et al. (2011) and Andreae (2019), Nr measurements are limited to the sum of NO, NO2, HONO, HCN, and NH3, and therefore the Nr could represent a lower limit. For both laboratory and field studies and independent of the fuel burnt, as MCE increases, NOx Nr increases, whereas NH3 Nr decreases. The FireLab MCE ranged from pure flaming (MCE = 0.99) to smoldering values (MCE < 0.8), but ambient observations during FIREX-AQ were limited to MCE values ranging from 0.85 to 0.95, which suggests both flaming and smoldering contributions to the sampled wildfire plumes.
3.5 Parameterization of organic- and nitrogen-containing emissions in wildfire plumes
The comparisons described above demonstrate that FIREX-AQ emissions agreed within a factor of 2 or better with previous laboratory and field studies for most C- and N-containing species for temperate ecosystem fuels. In the following, we relate primary wildfire emissions and emission factors to fire emissions measurable from space, e.g., CO (e.g., Schneising et al., 2020), NO2 (e.g., Martínez-Alonso et al., 2020), and BC (e.g., Konovalov et al., 2018), as well as MCE. Although current satellite retrievals for wildfire smoke can agree with airborne observations, e.g., for NOx and CO (Griffin et al., 2021; Stockwell et al., 2022), challenges in isolating the fire contribution from small or short-lived fires, as well as cloud coverage and aerosol interferences, add uncertainties to this quantification (e.g., Jung et al., 2019; Vasilkov et al., 2021). Here, we only focus on the parameterization of wildfire plumes and promote future efforts to quantify these compounds using satellite retrievals more accurately. Satellite-retrieved concentrations of CO and NO2 close to wildfires could then be used to estimate NMOG and NOy emissions and potentially better account for variability associated with fire emissions and improve modeling efforts to simplify and predict downwind formation of secondary pollutants, including ozone and secondary organic aerosol.
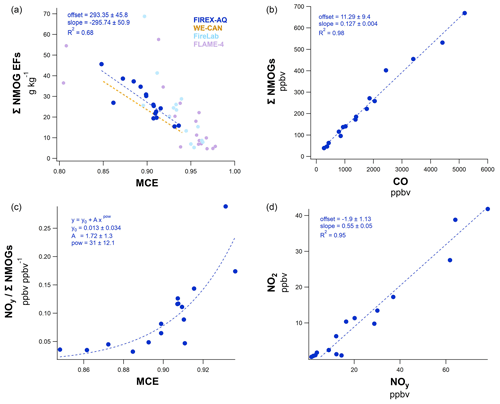
Figure 7Correlation trends observed for western US wildfire emissions for (a) the sum of median NMOG EFs compared to MCE for each wildfire. Each data point represents one fire from either FIREX-AQ, WE-CAN (Permar et al., 2021), FireLab (Koss et al., 2018), or FLAME-4 (Stockwell et al., 2015), with the name of each FIREX-AQ fire centered on the data points. (b) Sum of median NMOG mixing ratios plotted vs. CO, (c) ratio of median NOy species to the sum of NMOGs vs. MCE, and (d) median NOy mixing ratios vs. the median NO2 concentration. Dashed lines indicate linear fits for (a), (b), and (d) and a power function fit for (c).
Figure 7 shows correlations between the sum of the median mixing ratios of NMOGs and NOy with MCE, CO, and NO2, where CO and NO2 are two species available from satellite products that could be used as proxies for smoldering and flaming combustion (e.g., van der Velde et al., 2021; Urbanski et al., 2008), respectively. Figure 7a shows that the sum of FIREX-AQ NMOG EFs correlated with MCE with an R2 of 0.68, even though many of the individual compounds are poorly correlated with MCE (Table S4). The correlation of the FIREX-AQ MCE to the sum of NMOGs was in the same range as WE-CAN, FireLab, and FLAME-4 observations. WE-CAN was consistently lower, by around 10 %, which is partially due to differences in the assumed fraction of carbon employed in Eq. (1) (45.7 % for WE-CAN and 50 % for this study). FIREX-AQ sampled fires with lower MCEs on average than the lab experiments, with lab experiments showing highly variable EFs for MCE values below 0.9. Additional reasons for different FireLab and FLAME-4 EFs vs. MCE are discussed in detail by Permar et al. (2021) and include (1) rapid chemistry prior to sampling, which results in the degradation of short-lived species (Fig. S2) and/or less partitioning to particles at higher lab temperatures; (2) laboratory studies may more efficiently sample smoldering combustion emissions compared to aircraft observations where residual smoldering combustion emissions might not be lofted and therefore undersampled at the aircraft altitude; and (3) laboratory MCEs are often higher than in the field due to experimental conditions, including drier fuel and more efficient burning conditions (Yokelson et al., 2013; Holder et al., 2017; Selimovic et al., 2018), whereas field MCEs are calculated from single transects through smoke plumes that likely contain a different mix of flaming vs. smoldering (Wiggins et al., 2020). Nevertheless, the good agreement between two different aircraft studies during different years and the general agreement with FireLab and FLAME-4 study averages further highlight the consistency of total NMOG correlations with MCE in wildfire emissions despite the poorer correlations of individual compounds with MCE (Table S4).
Figure 7b relates the sum of the median NMOG mixing ratios to the median CO mixing ratios for all the freshest sampled wildfire plumes. CO results largely from smoldering combustion, which is the combustion process that also produces most NMOGs. NMOGs and CO are very well correlated, with a slope of 127 ± 4 (ppb ppm−1) and an R2 of 0.98, which demonstrates that total primary NMOG emissions are effectively represented by CO. Figure S4 shows that R2 values with CO for individual compounds were above 0.9 for the majority of primary NMOGs reported here, whereas, for secondary species, the correlations were below 0.3. CO columns are retrievable from space by, e.g., TROPOMI (Martínez-Alonso et al., 2020) and CRiS (NASA, 2015) and can be used to derive CO emissions that generally agree with in situ observations (Stockwell et al., 2022). The correlations from the FIREX-AQ measurements and others could be used to initialize total NMOG emissions from wildfire plumes in models.
Quantification of N-containing species is also essential for understanding and modeling the evolution and formation of secondary organic aerosol and ozone downwind of wildfires. Figure 7c shows the ratio of measured NOy by the chemiluminescence instrument (see Sect. 2) to the sum of NMOGs (in ppb ppb−1). A rapid increase in this ratio is observed as MCE increases, described by a power function fit. This increase follows the expectation that as fires transition from smoldering to flaming conditions, MCE increases, NMOGs EFs decrease, and fuel nitrogen leads to the formation of NOx through radical chemistry of N-containing compounds (Roberts et al., 2020). Figure 7d shows that NO2 represents a significant fraction of NOy, with a slope of 0.55 ± 0.03 (ppb ppb−1) and an R2 of 0.95. Furthermore, the correlation of individual N-containing species with NO2 is significantly higher than their correlation with CO mixing ratios (Fig. S4), promoting the finding that NO2 measurements could be used to initialize total NOy emissions and N species from wildfire plumes in models. Figure S5 shows additional correlations that could be used for modeling efforts, including the correlation of NOy to CO and NOy to BC.
These observations suggest that CO is a good proxy for species emitted from western wildfires primarily during smoldering conditions (i.e., NMOGs), whereas NO2 is a good proxy for species that are mostly emitted during flaming conditions (i.e., mostly NOy). Thus, in addition to coupling EFs with fuel consumption to derive emissions, we suggest future use of satellite retrievals close to the fire plume to quantify CO and NO2 concentrations in order to accurately determine EFs for all carbon and nitrogen-containing species for western US wildfire plumes as input to models. An important assumption, especially in determining emissions of N-containing species, is that NO2 should accurately represent NOy close to the fire. However, satellite retrievals that capture truly fresh emissions very close to the fire will be dominated by NO and HONO, whereas in highly oxidized plumes NO2 loss processes will lower its overall contribution to NOy. It is therefore important to provide a range of distances where this holds true. Bourgeois et al. (2022) find that for fires with highly reactive emissions, NO2 represents NOy within the first 15–30 min and a distance of 10–20 km downwind of the fire, assuming a wind speed of 10 m s−1. Current satellite retrievals for wildfire smoke have a spatial resolution of 3.5 km × 5.5 km (Griffin et al., 2021) which would be within the above range and high enough to represent plumes where NO2 is the dominant fraction of NOy.
We present ERs and EFs for NMOGs and nitrogen-containing compounds from nine western US wildfires and one southeastern US prescribed fire derived from data obtained aboard the NASA DC-8 during the 2019 FIREX-AQ mission. ERs and EFs were calculated for a total of 16 crosswind plume transects chosen to represent the freshest fire emissions. These transects were identified based on proxies (e.g., maleic anhydride furan ratio) for chemical aging, which can be rapid in fire plumes.
We performed detailed comparisons of FIREX-AQ emissions to previous laboratory and field studies with a focus on oxygenated organic compounds that were calibrated during this mission. FIREX-AQ ERs agree within a factor of 2 to the FireLab study for most compounds, with a correlation slope of 0.75 ± 0.05 and an R2 of 0.86. A comparison of the field-derived EFs from FIREX-AQ with those from SEAC4RS (Liu et al., 2017), WE-CAN (Permar et al., 2021), and temperate forest EFs from Andreae (2019) also agreed to within a factor of 2 for 87 %, 83 %, and 78 % of the compounds, respectively. However, FIREX-AQ EFs are on average higher compared to previous studies. For compounds that agree within a factor of 2, the average ratios of FIREX-AQ to WE-CAN, SEAC4RS, and the temperate forest fire literature average are 1.09 ± 0.3, 1.25 ± 0.33, and 1.18 ± 0.4, respectively, whereas for the remaining compounds, the ratios increase to 2.1 ± 1.64, 1.29 ± 1.01, and 1.32 ± 1.23. We suggest that these differences could be due to differences in the fuel and quantification methods applied for each study, as well as differences in photochemical loss of reactive species prior to detection. Additionally, differences in fire behavior and the lofting of smoke, including variations in the mixture of flaming and smoldering combustion, could also be contributing factors. We further compare the ratio of N-containing species to the total nitrogen (Ni Nr) vs. MCE and find that NOx Nr and NH3 Nr follow similar trends to those reported by Roberts et al. (2020).
We relate wildfire emissions of C- and N-containing species to CO, NO2, BC, and MCE based on correlations for use in chemical transport models. Results show that the sum of NMOG EFs correlates with MCE, with an R2 of 0.68 and a slope of −296 ± 51 g kg−1. A better correlation is observed between the sum of the median NMOG mixing ratios and median CO, with a slope of 0.127 ± 0.004 (ppb ppm−1) and an R2 of 0.98. Consistent correlation of individual NMOGs to CO is also evident for the majority of NMOGs, with R2 values greater than 0.9, suggesting significant potential for estimating wildfire NMOG emissions using space-based CO emissions.
For N-containing species, the sum of reactive nitrogen, NOy, correlates better with NO2 (R2 = 0.95, slope = 1.74 ± 0.1 ppbv ppbv−1) and BC (R2 = 0.88) than with CO (R2 = 0.7) close to wildfires. Furthermore, the ratio of NOy to the sum of NMOGs increases exponentially as MCE increases. This further highlights the important influence of fire behavior, e.g., flaming vs. smoldering fire conditions on the emissions of reactive nitrogen species. Future efforts to initialize models using the above emissions parameterization could improve the representation of fire emissions in models and their predictions on the downwind formation of secondary pollutants like ozone and secondary organic aerosol.
All measurements reported here can be accessed in the NASA FIREX-AQ data repository (Atmospheric Science Data Center, 2019) through the following link: https://www-air.larc.nasa.gov/cgi-bin/ArcView/firexaq (Langley Research Center, 2023).
The supplement related to this article is available online at: https://doi.org/10.5194/acp-24-929-2024-supplement.
CW, JPS, SSB, and JHC designed research; all authors performed measurements and advised research; and GIG and MMC wrote the paper with comments from all authors.
At least one of the (co-)authors is a member of the editorial board of Atmospheric Chemistry and Physics. The peer-review process was guided by an independent editor, and the authors also have no other competing interests to declare.
Publisher’s note: Copernicus Publications remains neutral with regard to jurisdictional claims made in the text, published maps, institutional affiliations, or any other geographical representation in this paper. While Copernicus Publications makes every effort to include appropriate place names, the final responsibility lies with the authors.
We would like to thank the NOAA/NASA FIREX-AQ science and aircraft operation teams.
Georgios I. Gkatzelis, Matthew M. Coggon, Chelsea E. Stockwell, Megan M. Bela, Ilann Bourgeois, Joseph M. Katich, Aaron Lamplugh, Stuart A. McKeen, J. Andrew Neuman, Jeff Peischl, Pamela S. Rickly, Michael A. Robinson, Rebecca H. Schwantes, Caroline C. Womack, and Carsten Warneke were supported by the NOAA cooperative agreement with CIRES (grant no. NA17OAR4320101). Robert J. Yokelson and Vanessa Selimovic were supported by NOAA (grant no. NA16OAR4310100) and NSF (grant no. 1748266). Jakob Lindaas, Glenn M. Wolfe, Reem Hannun, Jason M. St. Clair, and Thomas F. Hanisco were supported by the NASA Tropospheric Composition Program and NOAA Climate Program Office's Atmospheric Chemistry, Carbon Cycle and Climate (AC4) program (grant no. NA17OAR4310004). Demetrios Pagonis, Benjamin A. Nault, Hongyu Guo, Pedro Campuzano-Jost, Douglas A. Day, Melinda K. Schueneman, and Jose L. Jimenez were supported by NASA (grant nos. 80NSSC18K0630 and 80NSSC21K1451). Alan Fried was supported by NASA TCP (grant no. 80NSSC18K0628). The University of Innsbruck team was supported by the Austrian Federal Ministry for Transport, Innovation, and Technology (BMVIT, FFG, ASAP). Felix Piel received funding from the European Union's Horizon 2020 Research and Innovation program (IMPACT EU ITN (grant no. 674911)). Lu Xu, Krystal T. Vasquez, Hannah Allen, John D. Crounse, and Paul O. Wennberg were supported by NASA (grant nos. 80NSSC18K0660 and 80NSSC21K1704). This material is based upon work supported by the National Center for Atmospheric Research, sponsored by the National Science Foundation under cooperative agreement (grant no. 1852977).
The article processing charges for this open-access publication were covered by the Forschungszentrum Jülich.
This paper was edited by Manvendra Krishna Dubey and reviewed by two anonymous referees.
Akagi, S. K., Yokelson, R. J., Wiedinmyer, C., Alvarado, M. J., Reid, J. S., Karl, T., Crounse, J. D., and Wennberg, P. O.: Emission factors for open and domestic biomass burning for use in atmospheric models, Atmos. Chem. Phys., 11, 4039–4072, https://doi.org/10.5194/acp-11-4039-2011, 2011.
Akagi, S. K., Craven, J. S., Taylor, J. W., McMeeking, G. R., Yokelson, R. J., Burling, I. R., Urbanski, S. P., Wold, C. E., Seinfeld, J. H., Coe, H., Alvarado, M. J., and Weise, D. R.: Evolution of trace gases and particles emitted by a chaparral fire in California, Atmos. Chem. Phys., 12, 1397–1421, https://doi.org/10.5194/acp-12-1397-2012, 2012.
Akagi, S. K., Burling, I. R., Mendoza, A., Johnson, T. J., Cameron, M., Griffith, D. W. T., Paton-Walsh, C., Weise, D. R., Reardon, J., and Yokelson, R. J.: Field measurements of trace gases emitted by prescribed fires in southeastern US pine forests using an open-path FTIR system, Atmos. Chem. Phys., 14, 199–215, https://doi.org/10.5194/acp-14-199-2014, 2014.
Andreae, M. O.: Emission of trace gases and aerosols from biomass burning – an updated assessment, Atmos. Chem. Phys., 19, 8523–8546, https://doi.org/10.5194/acp-19-8523-2019, 2019.
Andreae, M. O. and Merlet, P.: Emission of trace gases and aerosols from biomass burning, Global Biogeochem. Cy., 15, 955–966, https://doi.org/10.1029/2000GB001382, 2001.
Apel, E. C., Emmons, L. K., Karl, T., Flocke, F., Hills, A. J., Madronich, S., Lee-Taylor, J., Fried, A., Weibring, P., Walega, J., Richter, D., Tie, X., Mauldin, L., Campos, T., Weinheimer, A., Knapp, D., Sive, B., Kleinman, L., Springston, S., Zaveri, R., Ortega, J., Voss, P., Blake, D., Baker, A., Warneke, C., Welsh-Bon, D., de Gouw, J., Zheng, J., Zhang, R., Rudolph, J., Junkermann, W., and Riemer, D. D.: Chemical evolution of volatile organic compounds in the outflow of the Mexico City Metropolitan area, Atmos. Chem. Phys., 10, 2353–2375, https://doi.org/10.5194/acp-10-2353-2010, 2010.
Apel, E. C., Hornbrook, R. S., Hills, A. J., Blake, N. J., Barth, M. C., Weinheimer, A., Cantrell, C., Rutledge, S. A., Basarab, B., Crawford, J., Diskin, G., Homeyer, C. R., Campos, T., Flocke, F., Fried, A., Blake, D. R., Brune, W., Pollack, I., Peischl, J., Ryerson, T., Wennberg, P. O., Crounse, J. D., Wisthaler, A., Mikoviny, T., Huey, G., Heikes, B., O'Sullivan, D., and Riemer, D. D.: Upper tropospheric ozone production from lightning NOx-impacted convection: Smoke ingestion case study from the DC3 campaign, J. Geophys. Res.-Atmos., 120, 2505–2523, https://doi.org/10.1002/2014JD022121, 2015.
Apte, J. S., Brauer, M., Cohen, A. J., Ezzati, M., and Pope, C. A.: Ambient PM2.5 Reduces Global and Regional Life Expectancy, Environ. Sci. Tech. Let., 5, 546–551, https://doi.org/10.1021/acs.estlett.8b00360, 2018.
Balch, J. K., Bradley, B. A., Abatzoglou, J. T., Nagy, R. C., Fusco, E. J., and Mahood, A. L.: Human-started wildfires expand the fire niche across the United States, P. Natl. Acad. Sci. USA, 114, 2946, https://doi.org/10.1073/pnas.1617394114, 2017.
Bond, T. C., Doherty, S. J., Fahey, D. W., Forster, P. M., Berntsen, T., DeAngelo, B. J., Flanner, M. G., Ghan, S., Kärcher, B., Koch, D., Kinne, S., Kondo, Y., Quinn, P. K., Sarofim, M. C., Schultz, M. G., Schulz, M., Venkataraman, C., Zhang, H., Zhang, S., Bellouin, N., Guttikunda, S. K., Hopke, P. K., Jacobson, M. Z., Kaiser, J. W., Klimont, Z., Lohmann, U., Schwarz, J. P., Shindell, D., Storelvmo, T., Warren, S. G., and Zender, C. S.: Bounding the role of black carbon in the climate system: A scientific assessment, J. Geophys. Res.-Atmos., 118, 5380–5552, https://doi.org/10.1002/jgrd.50171, 2013.
Bourgeois, I., Peischl, J., Thompson, C. R., Aikin, K. C., Campos, T., Clark, H., Commane, R., Daube, B., Diskin, G. W., Elkins, J. W., Gao, R.-S., Gaudel, A., Hintsa, E. J., Johnson, B. J., Kivi, R., McKain, K., Moore, F. L., Parrish, D. D., Querel, R., Ray, E., Sánchez, R., Sweeney, C., Tarasick, D. W., Thompson, A. M., Thouret, V., Witte, J. C., Wofsy, S. C., and Ryerson, T. B.: Global-scale distribution of ozone in the remote troposphere from the ATom and HIPPO airborne field missions, Atmos. Chem. Phys., 20, 10611–10635, https://doi.org/10.5194/acp-20-10611-2020, 2020.
Bourgeois, I., Peischl, J., Neuman, J. A., Brown, S. S., Allen, H. M., Campuzano-Jost, P., Coggon, M. M., DiGangi, J. P., Diskin, G. S., Gilman, J. B., Gkatzelis, G. I., Guo, H., Halliday, H. A., Hanisco, T. F., Holmes, C. D., Huey, L. G., Jimenez, J. L., Lamplugh, A. D., Lee, Y. R., Lindaas, J., Moore, R. H., Nault, B. A., Nowak, J. B., Pagonis, D., Rickly, P. S., Robinson, M. A., Rollins, A. W., Selimovic, V., St. Clair, J. M., Tanner, D., Vasquez, K. T., Veres, P. R., Warneke, C., Wennberg, P. O., Washenfelder, R. A., Wiggins, E. B., Womack, C. C., Xu, L., Zarzana, K. J., and Ryerson, T. B.: Comparison of airborne measurements of NO, NO2, HONO, NOy, and CO during FIREX-AQ, Atmos. Meas. Tech., 15, 4901–4930, https://doi.org/10.5194/amt-15-4901-2022, 2022.
Braga, R. C., Rosenfeld, D., Weigel, R., Jurkat, T., Andreae, M. O., Wendisch, M., Pöschl, U., Voigt, C., Mahnke, C., Borrmann, S., Albrecht, R. I., Molleker, S., Vila, D. A., Machado, L. A. T., and Grulich, L.: Further evidence for CCN aerosol concentrations determining the height of warm rain and ice initiation in convective clouds over the Amazon basin, Atmos. Chem. Phys., 17, 14433–14456, https://doi.org/10.5194/acp-17-14433-2017, 2017.
Brey, S. J. and Fischer, E. V.: Smoke in the City: How Often and Where Does Smoke Impact Summertime Ozone in the United States?, Environ. Sci. Technol., 50, 4144–4145, https://doi.org/10.1021/acs.est.5b05218, 2015.
Canagaratna, M. R., Jayne, J. T., Jimenez, J. L., Allan, J. D., Alfarra, M. R., Zhang, Q., Onasch, T. B., Drewnick, F., Coe, H., Middlebrook, A., Delia, A., Williams, L. R., Trimborn, A. M., Northway, M. J., DeCarlo, P. F., Kolb, C. E., Davidovits, P., and Worsnop, D. R.: Chemical and microphysical characterization of ambient aerosols with the aerodyne aerosol mass spectrometer, Mass Spectrom. Rev., 26, 185–222, https://doi.org/10.1002/mas.20115, 2007.
Cazorla, M., Wolfe, G. M., Bailey, S. A., Swanson, A. K., Arkinson, H. L., and Hanisco, T. F.: A new airborne laser-induced fluorescence instrument for in situ detection of formaldehyde throughout the troposphere and lower stratosphere, Atmos. Meas. Tech., 8, 541–552, https://doi.org/10.5194/amt-8-541-2015, 2015.
Cecchini, M. A., Machado, L. A. T., Andreae, M. O., Martin, S. T., Albrecht, R. I., Artaxo, P., Barbosa, H. M. J., Borrmann, S., Fütterer, D., Jurkat, T., Mahnke, C., Minikin, A., Molleker, S., Pöhlker, M. L., Pöschl, U., Rosenfeld, D., Voigt, C., Weinzierl, B., and Wendisch, M.: Sensitivities of Amazonian clouds to aerosols and updraft speed, Atmos. Chem. Phys., 17, 10037–10050, https://doi.org/10.5194/acp-17-10037-2017, 2017.
Cochrane, M. A., Moran, C. J., Wimberly, M. C., Baer, A. D., Finney, M. A., Beckendorf, K. L., Eidenshink, J., and Zhu, Z.: Estimation of wildfire size and risk changes due to fuels treatments, Int. J. Wildland Fire, 21, 357–367, https://doi.org/10.1071/WF11079, 2012.
Coggon, M. M., Lim, C. Y., Koss, A. R., Sekimoto, K., Yuan, B., Gilman, J. B., Hagan, D. H., Selimovic, V., Zarzana, K. J., Brown, S. S., Roberts, J. M., Müller, M., Yokelson, R., Wisthaler, A., Krechmer, J. E., Jimenez, J. L., Cappa, C., Kroll, J. H., de Gouw, J., and Warneke, C.: OH chemistry of non-methane organic gases (NMOGs) emitted from laboratory and ambient biomass burning smoke: evaluating the influence of furans and oxygenated aromatics on ozone and secondary NMOG formation, Atmos. Chem. Phys., 19, 14875–14899, https://doi.org/10.5194/acp-19-14875-2019, 2019.
Colman, J. J., Swanson, A. L., Meinardi, S., Sive, B. C., Blake, D. R., and Rowland, F. S.: Description of the Analysis of a Wide Range of Volatile Organic Compounds in Whole Air Samples Collected during PEM-Tropics A and B, Anal. Chem., 73, 3723–3731, https://doi.org/10.1021/ac010027g, 2001.
Crounse, J. D., McKinney, K. A., Kwan, A. J., and Wennberg, P. O.: Measurement of Gas-Phase Hydroperoxides by Chemical Ionization Mass Spectrometry, Anal. Chem., 78, 6726–6732, https://doi.org/10.1021/ac0604235, 2006.
Crutzen, P. J. and Andreae, M. O.: Biomass Burning in the Tropics: Impact on Atmospheric Chemistry and Biogeochemical Cycles, Science, 250, 1669, https://doi.org/10.1126/science.250.4988.1669, 1990.
Day, D. A., Campuzano-Jost, P., Nault, B. A., Palm, B. B., Hu, W., Guo, H., Wooldridge, P. J., Cohen, R. C., Docherty, K. S., Huffman, J. A., de Sá, S. S., Martin, S. T., and Jimenez, J. L.: A systematic re-evaluation of methods for quantification of bulk particle-phase organic nitrates using real-time aerosol mass spectrometry, Atmos. Meas. Tech., 15, 459–483, https://doi.org/10.5194/amt-15-459-2022, 2022.
Decker, Z. C. J., Robinson, M. A., Barsanti, K. C., Bourgeois, I., Coggon, M. M., DiGangi, J. P., Diskin, G. S., Flocke, F. M., Franchin, A., Fredrickson, C. D., Gkatzelis, G. I., Hall, S. R., Halliday, H., Holmes, C. D., Huey, L. G., Lee, Y. R., Lindaas, J., Middlebrook, A. M., Montzka, D. D., Moore, R., Neuman, J. A., Nowak, J. B., Palm, B. B., Peischl, J., Piel, F., Rickly, P. S., Rollins, A. W., Ryerson, T. B., Schwantes, R. H., Sekimoto, K., Thornhill, L., Thornton, J. A., Tyndall, G. S., Ullmann, K., Van Rooy, P., Veres, P. R., Warneke, C., Washenfelder, R. A., Weinheimer, A. J., Wiggins, E., Winstead, E., Wisthaler, A., Womack, C., and Brown, S. S.: Nighttime and daytime dark oxidation chemistry in wildfire plumes: an observation and model analysis of FIREX-AQ aircraft data, Atmos. Chem. Phys., 21, 16293–16317, https://doi.org/10.5194/acp-21-16293-2021, 2021.
Dennekamp, M., Straney, L. D., Erbas, B., Abramson, M. J., Keywood, M., Smith, K., Sim, M. R., Glass, D. C., Del Monaco, A., Haikerwal, A., and Tonkin, A. M.: Forest Fire Smoke Exposures and Out-of-Hospital Cardiac Arrests in Melbourne, Australia: A Case-Crossover Study, Environ. Health Persp., 123, 959–964, https://doi.org/10.1289/ehp.1408436, 2015.
Finewax, Z., de Gouw, J. A., and Ziemann, P. J.: Identification and Quantification of 4-Nitrocatechol Formed from OH and NO3 Radical-Initiated Reactions of Catechol in Air in the Presence of NOx: Implications for Secondary Organic Aerosol Formation from Biomass Burning, Environ. Sci. Technol., 52, 1981–1989, https://doi.org/10.1021/acs.est.7b05864, 2018.
Flannigan, M., Cantin, A. S., de Groot, W. J., Wotton, M., Newbery, A., and Gowman, L. M.: Global wildland fire season severity in the 21st century, For. Ecol. Manag., 294, 54–61, https://doi.org/10.1016/j.foreco.2012.10.022, 2013.
Fried, A., Walega, J., Weibring, P., Richter, D., Simpson, I. J., Blake, D. R., Blake, N. J., Meinardi, S., Barletta, B. Hughes, S. C., Crawford, J. H., Diskin, G. Barrick, J., Hair, J., Fenn, M., Wisthaler, A., Mikoviny, T., Woo, J.-H. Park, M., Kim, J., Min, K.-E., Jeong, S., Wennberg, P. O., Kim, M. J., Crounse, J. D., Teng, A. P., Bennett, R., Yang-Martin, M., Shook, M. A., Huey, G., Tanner, D., Knote, C., Kim, J., Park, R., and Brune, W.: Airborne formaldehyde and volatile organic compound measurements over the Daesan petrochemical complex on Korea's northwest coast during the Korea-United States Air Quality study: Estimation of emission fluxes and effects on air quality, Elem. Sci. Anth., 8, 121, https://doi.org/10.1525/elementa.2020.121, 2020.
Gilman, J. B., Lerner, B. M., Kuster, W. C., Goldan, P. D., Warneke, C., Veres, P. R., Roberts, J. M., de Gouw, J. A., Burling, I. R., and Yokelson, R. J.: Biomass burning emissions and potential air quality impacts of volatile organic compounds and other trace gases from fuels common in the US, Atmos. Chem. Phys., 15, 13915–13938, https://doi.org/10.5194/acp-15-13915-2015, 2015.
Griffin, D., McLinden, C. A., Dammers, E., Adams, C., Stockwell, C. E., Warneke, C., Bourgeois, I., Peischl, J., Ryerson, T. B., Zarzana, K. J., Rowe, J. P., Volkamer, R., Knote, C., Kille, N., Koenig, T. K., Lee, C. F., Rollins, D., Rickly, P. S., Chen, J., Fehr, L., Bourassa, A., Degenstein, D., Hayden, K., Mihele, C., Wren, S. N., Liggio, J., Akingunola, A., and Makar, P.: Biomass burning nitrogen dioxide emissions derived from space with TROPOMI: methodology and validation, Atmos. Meas. Tech., 14, 7929–7957, https://doi.org/10.5194/amt-14-7929-2021, 2021.
Guo, H., Campuzano-Jost, P., Nault, B. A., Day, D. A., Schroder, J. C., Kim, D., Dibb, J. E., Dollner, M., Weinzierl, B., and Jimenez, J. L.: The importance of size ranges in aerosol instrument intercomparisons: a case study for the Atmospheric Tomography Mission, Atmos. Meas. Tech., 14, 3631–3655, https://doi.org/10.5194/amt-14-3631-2021, 2021.
Hamilton, D. S., Hantson, S., Scott, C. E., Kaplan, J. O., Pringle, K. J., Nieradzik, L. P., Rap, A., Folberth, G. A., Spracklen, D. V., and Carslaw, K. S.: Reassessment of pre-industrial fire emissions strongly affects anthropogenic aerosol forcing, Nat. Commun., 9, 3182, https://doi.org/10.1038/s41467-018-05592-9, 2018.
Hatch, L. E., Yokelson, R. J., Stockwell, C. E., Veres, P. R., Simpson, I. J., Blake, D. R., Orlando, J. J., and Barsanti, K. C.: Multi-instrument comparison and compilation of non-methane organic gas emissions from biomass burning and implications for smoke-derived secondary organic aerosol precursors, Atmos. Chem. Phys., 17, 1471–1489, https://doi.org/10.5194/acp-17-1471-2017, 2017.
Hodshire, A. L., Bian, Q., Ramnarine, E., Lonsdale, C. R., Alvarado, M. J., Kreidenweis, S. M., Jathar, S. H., and Pierce, J. R.: More Than Emissions and Chemistry: Fire Size, Dilution, and Background Aerosol Also Greatly Influence Near-Field Biomass Burning Aerosol Aging, J. Geophys. Res.-Atmos., 124, 5589–5611, https://doi.org/10.1029/2018JD029674, 2019.
Holder, A. L., Gullett, B. K., Urbanski, S. P., Elleman, R., O'Neill, S., Tabor, D., Mitchell, W., and Baker, K. R.: Emissions from prescribed burning of agricultural fields in the Pacific Northwest, Atmos. Environ., 166, 22–33, https://doi.org/10.1016/j.atmosenv.2017.06.043, 2017.
Holmes, C. D., Fite, C., Agastra, A., Schwarz, J. P., Yokelson, R. J., Bui, T. V., and Peterson, D. A.: Critical evaluation of smoke age inferred from different methods during FIREX-AQ, American Geophysical Union, https://ui.adsabs.harvard.edu/abs/2020AGUFMA225.0010H (last access: 14 December 2023), 2020.
Jin, L., Permar, W., Selimovic, V., Ketcherside, D., Yokelson, R. J., Hornbrook, R. S., Apel, E. C., Ku, I.-T., Collett Jr., J. L., Sullivan, A. P., Jaffe, D. A., Pierce, J. R., Fried, A., Coggon, M. M., Gkatzelis, G. I., Warneke, C., Fischer, E. V., and Hu, L.: Constraining emissions of volatile organic compounds from western US wildfires with WE-CAN and FIREX-AQ airborne observations, Atmos. Chem. Phys., 23, 5969–5991, https://doi.org/10.5194/acp-23-5969-2023, 2023.
Johnston, F. H., Henderson, S. B., Chen, Y., Randerson, J. T., Marlier, M., DeFries, R. S., Kinney, P., Bowman, D. M. J. S., and Brauer, M.: Estimated Global Mortality Attributable to Smoke from Landscape Fires, Environ. Health Persp., 120, 695–701, https://doi.org/10.1289/ehp.1104422, 2012.
Jung, Y., González Abad, G., Nowlan, C. R., Chance, K., Liu, X., Torres, O., and Ahn, C.: Explicit Aerosol Correction of OMI Formaldehyde Retrievals, Earth Space Sci., 6, 2087–2105, https://doi.org/10.1029/2019EA000702, 2019.
Keywood, M., Kanakidou, M., Stohl, A., Dentener, F., Grassi, G., Meyer, C. P., Torseth, K., Edwards, D., Thompson, A. M., Lohmann, U., and Burrows, J.: Fire in the Air: Biomass Burning Impacts in a Changing Climate, Crit. Rev. Environ. Sci. Technol., 43, 40–83, https://doi.org/10.1080/10643389.2011.604248, 2013.
Kloster, S., Mahowald, N. M., Randerson, J. T., Thornton, P. E., Hoffman, F. M., Levis, S., Lawrence, P. J., Feddema, J. J., Oleson, K. W., and Lawrence, D. M.: Fire dynamics during the 20th century simulated by the Community Land Model, Biogeosciences, 7, 1877–1902, https://doi.org/10.5194/bg-7-1877-2010, 2010.
Knorr, W., Dentener, F., Lamarque, J.-F., Jiang, L., and Arneth, A.: Wildfire air pollution hazard during the 21st century, Atmos. Chem. Phys., 17, 9223–9236, https://doi.org/10.5194/acp-17-9223-2017, 2017.
Kodros, J. K., Papanastasiou, D. K., Paglione, M., Masiol, M., Squizzato, S., Florou, K., Skyllakou, K., Kaltsonoudis, C., Nenes, A., and Pandis, S. N.: Rapid dark aging of biomass burning as an overlooked source of oxidized organic aerosol, P. Natl. Acad. Sci. USA, 117, 33028, https://doi.org/10.1073/pnas.2010365117, 2020.
Konovalov, I. B., Lvova, D. A., Beekmann, M., Jethva, H., Mikhailov, E. F., Paris, J.-D., Belan, B. D., Kozlov, V. S., Ciais, P., and Andreae, M. O.: Estimation of black carbon emissions from Siberian fires using satellite observations of absorption and extinction optical depths, Atmos. Chem. Phys., 18, 14889–14924, https://doi.org/10.5194/acp-18-14889-2018, 2018.
Koss, A. R., Sekimoto, K., Gilman, J. B., Selimovic, V., Coggon, M. M., Zarzana, K. J., Yuan, B., Lerner, B. M., Brown, S. S., Jimenez, J. L., Krechmer, J., Roberts, J. M., Warneke, C., Yokelson, R. J., and de Gouw, J.: Non-methane organic gas emissions from biomass burning: identification, quantification, and emission factors from PTR-ToF during the FIREX 2016 laboratory experiment, Atmos. Chem. Phys., 18, 3299–3319, https://doi.org/10.5194/acp-18-3299-2018, 2018.
Langley Research Center: FIREX-AQ 2019, National Aeronautics and Space Administration [data set], https://www-air.larc.nasa.gov/cgi-bin/ArcView/firexaq (last access: 14 December 2023), 2023.
Le Quéré, C., Andrew, R. M., Friedlingstein, P., Sitch, S., Hauck, J., Pongratz, J., Pickers, P. A., Korsbakken, J. I., Peters, G. P., Canadell, J. G., Arneth, A., Arora, V. K., Barbero, L., Bastos, A., Bopp, L., Chevallier, F., Chini, L. P., Ciais, P., Doney, S. C., Gkritzalis, T., Goll, D. S., Harris, I., Haverd, V., Hoffman, F. M., Hoppema, M., Houghton, R. A., Hurtt, G., Ilyina, T., Jain, A. K., Johannessen, T., Jones, C. D., Kato, E., Keeling, R. F., Goldewijk, K. K., Landschützer, P., Lefèvre, N., Lienert, S., Liu, Z., Lombardozzi, D., Metzl, N., Munro, D. R., Nabel, J. E. M. S., Nakaoka, S., Neill, C., Olsen, A., Ono, T., Patra, P., Peregon, A., Peters, W., Peylin, P., Pfeil, B., Pierrot, D., Poulter, B., Rehder, G., Resplandy, L., Robertson, E., Rocher, M., Rödenbeck, C., Schuster, U., Schwinger, J., Séférian, R., Skjelvan, I., Steinhoff, T., Sutton, A., Tans, P. P., Tian, H., Tilbrook, B., Tubiello, F. N., van der Laan-Luijkx, I. T., van der Werf, G. R., Viovy, N., Walker, A. P., Wiltshire, A. J., Wright, R., Zaehle, S., and Zheng, B.: Global Carbon Budget 2018, Earth Syst. Sci. Data, 10, 2141–2194, https://doi.org/10.5194/essd-10-2141-2018, 2018.
Lerner, B. M., Gilman, J. B., Aikin, K. C., Atlas, E. L., Goldan, P. D., Graus, M., Hendershot, R., Isaacman-VanWertz, G. A., Koss, A., Kuster, W. C., Lueb, R. A., McLaughlin, R. J., Peischl, J., Sueper, D., Ryerson, T. B., Tokarek, T. W., Warneke, C., Yuan, B., and de Gouw, J. A.: An improved, automated whole air sampler and gas chromatography mass spectrometry analysis system for volatile organic compounds in the atmosphere, Atmos. Meas. Tech., 10, 291–313, https://doi.org/10.5194/amt-10-291-2017, 2017.
Liao, J., Wolfe, G. M., Hannun, R. A., St. Clair, J. M., Hanisco, T. F., Gilman, J. B., Lamplugh, A., Selimovic, V., Diskin, G. S., Nowak, J. B., Halliday, H. S., DiGangi, J. P., Hall, S. R., Ullmann, K., Holmes, C. D., Fite, C. H., Agastra, A., Ryerson, T. B., Peischl, J., Bourgeois, I., Warneke, C., Coggon, M. M., Gkatzelis, G. I., Sekimoto, K., Fried, A., Richter, D., Weibring, P., Apel, E. C., Hornbrook, R. S., Brown, S. S., Womack, C. C., Robinson, M. A., Washenfelder, R. A., Veres, P. R., and Neuman, J. A.: Formaldehyde evolution in US wildfire plumes during the Fire Influence on Regional to Global Environments and Air Quality experiment (FIREX-AQ), Atmos. Chem. Phys., 21, 18319–18331, https://doi.org/10.5194/acp-21-18319-2021, 2021.
Lindaas, J., Pollack, I. B., Garofalo, L. A., Pothier, M. A., Farmer, D. K., Kreidenweis, S. M., Campos, T. L., Flocke, F., Weinheimer, A. J., Montzka, D. D., Tyndall, G. S., Palm, B. B., Peng, Q., Thornton, J. A., Permar, W., Wielgasz, C., Hu, L., Ottmar, R. D., Restaino, J. C., Hudak, A. T., Ku, I. T., Zhou, Y., Sive, B. C., Sullivan, A., Collett Jr, J. L., and Fischer, E. V.: Emissions of Reactive Nitrogen From Western U.S. Wildfires During Summer 2018, J. Geophys. Res.-Atmos., 126, e2020JD032657, https://doi.org/10.1029/2020JD032657, 2021.
Ling, Z., Xie, Q., Shao, M., Wang, Z., Wang, T., Guo, H., and Wang, X.: Formation and sink of glyoxal and methylglyoxal in a polluted subtropical environment: observation-based photochemical analysis and impact evaluation, Atmos. Chem. Phys., 20, 11451–11467, https://doi.org/10.5194/acp-20-11451-2020, 2020.
Liu, X., Huey, L. G., Yokelson, R. J., Selimovic, V., Simpson, I. J., Müller, M., Jimenez, J. L., Campuzano-Jost, P., Beyersdorf, A. J., Blake, D. R., Butterfield, Z., Choi, Y., Crounse, J. D., Day, D. A., Diskin, G. S., Dubey, M. K., Fortner, E., Hanisco, T. F., Hu, W., King, L. E., Kleinman, L., Meinardi, S., Mikoviny, T., Onasch, T. B., Palm, B. B., Peischl, J., Pollack, I. B., Ryerson, T. B., Sachse, G. W., Sedlacek, A. J., Shilling, J. E., Springston, S., St. Clair, J. M., Tanner, D. J., Teng, A. P., Wennberg, P. O., Wisthaler, A., and Wolfe, G. M.: Airborne measurements of western U.S. wildfire emissions: Comparison with prescribed burning and air quality implications, J. Geophys. Res.-Atmos., 122, 6108–6129, https://doi.org/10.1002/2016JD026315, 2017.
Mann, M. L., Batllori, E., Moritz, M. A., Waller, E. K., Berck, P., Flint, A. L., Flint, L. E., and Dolfi, E.: Incorporating Anthropogenic Influences into Fire Probability Models: Effects of Human Activity and Climate Change on Fire Activity in California, PLOS ONE, 11, e0153589, https://doi.org/10.1371/journal.pone.0153589, 2016.
Martínez-Alonso, S., Deeter, M., Worden, H., Borsdorff, T., Aben, I., Commane, R., Daube, B., Francis, G., George, M., Landgraf, J., Mao, D., McKain, K., and Wofsy, S.: 1.5 years of TROPOMI CO measurements: comparisons to MOPITT and ATom, Atmos. Meas. Tech., 13, 4841–4864, https://doi.org/10.5194/amt-13-4841-2020, 2020.
Min, K.-E., Washenfelder, R. A., Dubé, W. P., Langford, A. O., Edwards, P. M., Zarzana, K. J., Stutz, J., Lu, K., Rohrer, F., Zhang, Y., and Brown, S. S.: A broadband cavity enhanced absorption spectrometer for aircraft measurements of glyoxal, methylglyoxal, nitrous acid, nitrogen dioxide, and water vapor, Atmos. Meas. Tech., 9, 423–440, https://doi.org/10.5194/amt-9-423-2016, 2016.
Mitsuishi, K., Iwasaki, M., Takeuchi, M., Okochi, H., Kato, S., Ohira, S.-I., and Toda, K.: Diurnal Variations in Partitioning of Atmospheric Glyoxal and Methylglyoxal between Gas and Particles at the Ground Level and in the Free Troposphere, ACS Earth Space Chem., 2, 915–924, https://doi.org/10.1021/acsearthspacechem.8b00037, 2018.
Moritz, M. A., Parisien, M.-A., Batllori, E., Krawchuk, M. A., Van Dorn, J., Ganz, D. J., and Hayhoe, K.: Climate change and disruptions to global fire activity, Ecosphere, 3, 49, https://doi.org/10.1890/ES11-00345.1, 2012.
Mouat, A. P., Paton-Walsh, C., Simmons, J. B., Ramirez-Gamboa, J., Griffith, D. W. T., and Kaiser, J.: Measurement report: Observations of long-lived volatile organic compounds from the 2019–2020 Australian wildfires during the COALA campaign, Atmos. Chem. Phys., 22, 11033–11047, https://doi.org/10.5194/acp-22-11033-2022, 2022.
Müller, M., Anderson, B. E., Beyersdorf, A. J., Crawford, J. H., Diskin, G. S., Eichler, P., Fried, A., Keutsch, F. N., Mikoviny, T., Thornhill, K. L., Walega, J. G., Weinheimer, A. J., Yang, M., Yokelson, R. J., and Wisthaler, A.: In situ measurements and modeling of reactive trace gases in a small biomass burning plume, Atmos. Chem. Phys., 16, 3813–3824, https://doi.org/10.5194/acp-16-3813-2016, 2016.
Atmospheric Science Data Center: Fire Influence on Regional to Global Environments and Air Quality [data set], NASA Langley Research Center, https://doi.org/10.5067/SUBORBITAL/FIREXAQ2019/DATA001, 2019.
National Interagency Fire Center: Total Wildland Fires and Acres (1960–2009), http://www.nifc.gov/fireInfo/fireInfo_stats_totalFires.html, last access: 24 October 2015.
Olivier, J. G. J., Van Aardenne, J. A., Dentener, F. J., Pagliari, V., Ganzeveld, L. N., and Peters, J. A. H. W.: Recent trends in global greenhouse gas emissions:regional trends 1970–2000 and spatial distribution of key sources in 2000, Environm. Sci., 2, 81–99, https://doi.org/10.1080/15693430500400345, 2005.
Pagonis, D., Sekimoto, K. and de Gouw, J. A.: A library of proton-transfer reactions of H3O+ ions used for trace gas detection, J. Am. Soc. Mass Spectrom., 30, 1330–1335, https://doi.org/10.1007/s13361-019-02209-3, 2019.
Pagonis, D., Selimovic, V., Campuzano-Jost, P., Guo, H., Day, D. A., Schueneman, M. K., Nault, B. A., Coggon, M. M., DiGangi, J. P., Diskin, G. S., Fortner, E. C., Gargulinski, E. M., Gkatzelis, G. I., Hair, J. W., Herndon, S. C., Holmes, C. D., Katich, J. M., Nowak, J. B., Perring, A. E., Saide, P., Shingler, T. J., Soja, A. J., Thapa, L. H., Warneke, C., Wiggins, E. B., Wisthaler, A., Yacovitch, T. I., Yokelson, R. J., and Jimenez, J. L.: Impact of Biomass Burning Organic Aerosol Volatility on Smoke Concentrations Downwind of Fires, Environ. Sci. Technol., 57, 17011–17021, https://doi.org/10.1021/acs.est.3c05017, 2023.
Pechony, O. and Shindell, D. T.: Driving forces of global wildfires over the past millennium and the forthcoming century, P. Natl. Acad. Sci. USA, 107, 19167, https://doi.org/10.1073/pnas.1003669107, 2010.
Peng, Q., Palm, B. B., Melander, K. E., Lee, B. H., Hall, S. R., Ullmann, K., Campos, T., Weinheimer, A. J., Apel, E. C., Hornbrook, R. S., Hills, A. J., Montzka, D. D., Flocke, F., Hu, L., Permar, W., Wielgasz, C., Lindaas, J., Pollack, I. B., Fischer, E. V., Bertram, T. H., and Thornton, J. A.: HONO Emissions from Western U.S. Wildfires Provide Dominant Radical Source in Fresh Wildfire Smoke, Environ. Sci. Technol., 54, 5954–5963, https://doi.org/10.1021/acs.est.0c00126, 2020.
Permar, W., Wang, Q., Selimovic, V., Wielgasz, C., Yokelson, R. J., Hornbrook, R. S., Hills, A. J., Apel, E. C., Ku, I. T., Zhou, Y., Sive, B. C., Sullivan, A. P., Collett Jr, J. L., Campos, T. L., Palm, B. B., Peng, Q., Thornton, J. A., Garofalo, L. A., Farmer, D. K., Kreidenweis, S. M., Levin, E. J. T., DeMott, P. J., Flocke, F., Fischer, E. V., and Hu, L.: Emissions of Trace Organic Gases From Western U.S. Wildfires Based on WE-CAN Aircraft Measurements, J. Geophys. Res.-Atmos., 126, e2020JD033838, https://doi.org/10.1029/2020JD033838, 2021.
Prichard, S. J., O'Neill, S. M., Eagle, P., Andreu, A. G., Drye, B., Dubowy, J., Urbanski, S., and Strand, T. M.: Wildland fire emission factors in North America: synthesis of existing data, measurement needs and management applications, Int. J. Wildland Fire, 29, 132–147, https://doi.org/10.1071/WF19066, 2020.
Reddington, C. L., Spracklen, D. V., Artaxo, P., Ridley, D. A., Rizzo, L. V., and Arana, A.: Analysis of particulate emissions from tropical biomass burning using a global aerosol model and long-term surface observations, Atmos. Chem. Phys., 16, 11083–11106, https://doi.org/10.5194/acp-16-11083-2016, 2016.
Richter, D., Weibring, P., Walega, J. G., Fried, A., Spuler, S. M., and Taubman, M. S.: Compact highly sensitive multi-species airborne mid-IR spectrometer, Appl. Phys. B., 119, 119–131, https://doi.org/10.1007/s00340-015-6038-8, 2015.
Rickly, P. S., Guo, H., Campuzano-Jost, P., Jimenez, J. L., Wolfe, G. M., Bennett, R., Bourgeois, I., Crounse, J. D., Dibb, J. E., DiGangi, J. P., Diskin, G. S., Dollner, M., Gargulinski, E. M., Hall, S. R., Halliday, H. S., Hanisco, T. F., Hannun, R. A., Liao, J., Moore, R., Nault, B. A., Nowak, J. B., Peischl, J., Robinson, C. E., Ryerson, T., Sanchez, K. J., Schöberl, M., Soja, A. J., St. Clair, J. M., Thornhill, K. L., Ullmann, K., Wennberg, P. O., Weinzierl, B., Wiggins, E. B., Winstead, E. L., and Rollins, A. W.: Emission factors and evolution of SO2 measured from biomass burning in wildfires and agricultural fires, Atmos. Chem. Phys., 22, 15603–15620, https://doi.org/10.5194/acp-22-15603-2022, 2022.
Roberts, J. M., Stockwell, C. E., Yokelson, R. J., de Gouw, J., Liu, Y., Selimovic, V., Koss, A. R., Sekimoto, K., Coggon, M. M., Yuan, B., Zarzana, K. J., Brown, S. S., Santin, C., Doerr, S. H., and Warneke, C.: The nitrogen budget of laboratory-simulated western US wildfires during the FIREX 2016 Fire Lab study, Atmos. Chem. Phys., 20, 8807–8826, https://doi.org/10.5194/acp-20-8807-2020, 2020.
Robinson, M. A., Decker, Z. C. J., Barsanti, K. C., Coggon, M. M., Flocke, F. M., Franchin, A., Fredrickson, C. D., Gilman, J. B., Gkatzelis, G. I., Holmes, C. D., Lamplugh, A., Lavi, A., Middlebrook, A. M., Montzka, D. M., Palm, B. B., Peischl, J., Pierce, B., Schwantes, R. H., Sekimoto, K., Selimovic, V., Tyndall, G. S., Thornton, J. A., Van Rooy, P., Warneke, C., Weinheimer, A. J., and Brown, S. S.: Variability and Time of Day Dependence of Ozone Photochemistry in Western Wildfire Plumes, Environ. Sci. Technol., 55, 10280–10290, https://doi.org/10.1021/acs.est.1c01963, 2021.
Robinson, M. A., Neuman, J. A., Huey, L. G., Roberts, J. M., Brown, S. S., and Veres, P. R.: Temperature-dependent sensitivity of iodide chemical ionization mass spectrometers, Atmos. Meas. Tech., 15, 4295–4305, https://doi.org/10.5194/amt-15-4295-2022, 2022.
Rollins, A. W., Rickly, P. S., Gao, R.-S., Ryerson, T. B., Brown, S. S., Peischl, J., and Bourgeois, I.: Single-photon laser-induced fluorescence detection of nitric oxide at sub-parts-per-trillion mixing ratios, Atmos. Meas. Tech., 13, 2425–2439, https://doi.org/10.5194/amt-13-2425-2020, 2020.
Ryerson, T. B., Williams, E. J., and Fehsenfeld, F. C.: An efficient photolysis system for fast-response NO2 measurements, J. Geophys. Res.-Atmos., 105, 26447–26461, https://doi.org/10.1029/2000JD900389, 2000.
Sachse, G. W., Collins, J., Hill, G. F., Wade, L. O., Burney, L. G., and Ritter, J. A.: Airborne tunable diode laser sensor for high-precision concentration and flux measurements of carbon monoxide and methane, Proc. SPIE, 1433, https://doi.org/10.1117/12.46162, 1991.
Schwarz, J. P., Gao, R. S., Spackman, J. R., Watts, L. A., Thomson, D. S., Fahey, D. W., Ryerson, T. B., Peischl, J., Holloway, J. S., Trainer, M., Frost, G. J., Baynard, T., Lack, D. A., de Gouw, J. A., Warneke, C., and Del Negro, L. A.: Measurement of the mixing state, mass, and optical size of individual black carbon particles in urban and biomass burning emissions, Geophys. Res. Lett., 35, L13810, https://doi.org/10.1029/2008gl033968, 2008.
Schneising, O., Buchwitz, M., Reuter, M., Bovensmann, H., and Burrows, J. P.: Severe Californian wildfires in November 2018 observed from space: the carbon monoxide perspective, Atmos. Chem. Phys., 20, 3317–3332, https://doi.org/10.5194/acp-20-3317-2020, 2020.
Schwarz, J. P., Spackman, J. R., Fahey, D. W., Gao, R. S., Lohmann, U., Stier, P., Watts, L. A., Thomson, D. S., Lack, D. A., Pfister, L., Mahoney, M. J., Baumgardner, D., Wilson, J. C., and Reeves, J. M.: Coatings and their enhancement of black carbon light absorption in the tropical atmosphere, J. Geophys. Res.-Atmos., 113, D03203, https://doi.org/10.1029/2007JD009042, 2008.
Sekimoto, K., Li, S.-M., Yuan, B., Koss, A., Coggon, M., Warneke, C., and de Gouw, J.: Calculation of the sensitivity of proton-transfer-reaction mass spectrometry (PTR-MS) for organic trace gases using molecular properties, Int. J. Mass Spectrom., 421, 71–94, https://doi.org/10.1016/j.ijms.2017.04.006, 2017.
Sekimoto, K., Koss, A. R., Gilman, J. B., Selimovic, V., Coggon, M. M., Zarzana, K. J., Yuan, B., Lerner, B. M., Brown, S. S., Warneke, C., Yokelson, R. J., Roberts, J. M., and de Gouw, J.: High- and low-temperature pyrolysis profiles describe volatile organic compound emissions from western US wildfire fuels, Atmos. Chem. Phys., 18, 9263–9281, https://doi.org/10.5194/acp-18-9263-2018, 2018.
Selimovic, V., Yokelson, R. J., Warneke, C., Roberts, J. M., de Gouw, J., Reardon, J., and Griffith, D. W. T.: Aerosol optical properties and trace gas emissions by PAX and OP-FTIR for laboratory-simulated western US wildfires during FIREX, Atmos. Chem. Phys., 18, 2929–2948, https://doi.org/10.5194/acp-18-2929-2018, 2018.
Shrivastava, M., Cappa, C. D., Fan, J., Goldstein, A. H., Guenther, A. B., Jimenez, J. L., Kuang, C., Laskin, A., Martin, S. T., Ng, N. L., Petaja, T., Pierce, J. R., Rasch, P. J., Roldin, P., Seinfeld, J. H., Shilling, J., Smith, J. N., Thornton, J. A., Volkamer, R., Wang, J., Worsnop, D. R., Zaveri, R. A., Zelenyuk, A., and Zhang, Q.: Recent advances in understanding secondary organic aerosol: Implications for global climate forcing, Rev. Geophys., 55, 509–559, https://doi.org/10.1002/2016RG000540, 2017.
Simpson, I. J., Colman, J. J., Swanson, A. L., Bandy, A. R., Thornton, D. C., Blake, D. R., and Rowland, F. S.: Aircraft Measurements of Dimethyl Sulfide (DMS) Using a Whole Air Sampling Technique, J. Atmos. Chem., 39, 191–213, https://doi.org/10.1023/A:1010608529779, 2001.
Simpson, I. J., Blake, D. R., Blake, N. J., Meinardi, S., Barletta, B., Hughes, S. C., Fleming, L. T., Crawford, J. H., Diskin, G. S., Emmons, L. K., Fried, A., Guo, H., Peterson, D. A., Wisthaler, A., Woo, J.-H., Barré, J., Gaubert, B., Kim, J., Kim, M. J., Kim, Y., Knote, C., Mikoviny, T., Pusede, S. E., Schroeder, J. R., Wang, Y., Wennberg, P. O., and Zeng, L.: Characterization, sources and reactivity of volatile organic compounds (VOCs) in Seoul and surrounding regions during KORUS-AQ, Elem. Sci. Anth., 8, 37, https://doi.org/10.1525/elementa.434, 2020.
Spracklen, D. V., Mickley, L. J., Logan, J. A., Hudman, R. C., Yevich, R., Flannigan, M. D., and Westerling, A. L.: Impacts of climate change from 2000 to 2050 on wildfire activity and carbonaceous aerosol concentrations in the western United States, J. Geophys. Res.-Atmos., 114, D20301, https://doi.org/10.1029/2008JD010966, 2009.
Stockwell, C. E., Yokelson, R. J., Kreidenweis, S. M., Robinson, A. L., DeMott, P. J., Sullivan, R. C., Reardon, J., Ryan, K. C., Griffith, D. W. T., and Stevens, L.: Trace gas emissions from combustion of peat, crop residue, domestic biofuels, grasses, and other fuels: configuration and Fourier transform infrared (FTIR) component of the fourth Fire Lab at Missoula Experiment (FLAME-4), Atmos. Chem. Phys., 14, 9727–9754, https://doi.org/10.5194/acp-14-9727-2014, 2014.
Stockwell, C. E., Veres, P. R., Williams, J., and Yokelson, R. J.: Characterization of biomass burning emissions from cooking fires, peat, crop residue, and other fuels with high-resolution proton-transfer-reaction time-of-flight mass spectrometry, Atmos. Chem. Phys., 15, 845–865, https://doi.org/10.5194/acp-15-845-2015, 2015.
Stockwell, C. E., Jayarathne, T., Cochrane, M. A., Ryan, K. C., Putra, E. I., Saharjo, B. H., Nurhayati, A. D., Albar, I., Blake, D. R., Simpson, I. J., Stone, E. A., and Yokelson, R. J.: Field measurements of trace gases and aerosols emitted by peat fires in Central Kalimantan, Indonesia, during the 2015 El Niño, Atmos. Chem. Phys., 16, 11711–11732, https://doi.org/10.5194/acp-16-11711-2016, 2016.
Stockwell, C. E., Bela, M. M., Coggon, M. M., et al.: Airborne Emission Rate Measurements Validate Remote Sensing Observations and Emission Inventories of Western U.S. Wildfires, Environ. Sci. Technol., 56, 7564–7577, https://doi.org/10.1021/acs.est.1c07121, 2022.
Stönner, C., Derstroff, B., Klüpfel, T., Crowley, J. N., and Williams, J.: Glyoxal measurement with a proton transfer reaction time of flight mass spectrometer (PTR-TOF-MS): characterization and calibration, J. Mass Spectrom., 52, 30–35, https://doi.org/10.1002/jms.3893, 2017.
Sudo, K. and Akimoto, H.: Global source attribution of tropospheric ozone: Long-range transport from various source regions, J. Geophys. Res.-Atmos., 112, D12302, https://doi.org/10.1029/2006JD007992, 2007.
Theys, N., Volkamer, R., Müller, J. F., Zarzana, K. J., Kille, N., Clarisse, L., De Smedt, I., Lerot, C., Finkenzeller, H., Hendrick, F., Koenig, T. K., Lee, C. F., Knote, C., Yu, H., and Van Roozendael, M.: Global nitrous acid emissions and levels of regional oxidants enhanced by wildfires, Nat. Geosci., 13, 681–686, https://doi.org/10.1038/s41561-020-0637-7, 2020.
Tomsche, L., Piel, F., Mikoviny, T., Nielsen, C. J., Guo, H., Campuzano-Jost, P., Nault, B. A., Schueneman, M. K., Jimenez, J. L., Halliday, H., Diskin, G., DiGangi, J. P., Nowak, J. B., Wiggins, E. B., Gargulinski, E., Soja, A. J., and Wisthaler, A.: Measurement report: Emission factors of NH3 and NHx for wildfires and agricultural fires in the United States, Atmos. Chem. Phys., 23, 2331–2343, https://doi.org/10.5194/acp-23-2331-2023, 2023.
Thornhill, G. D., Ryder, C. L., Highwood, E. J., Shaffrey, L. C., and Johnson, B. T.: The effect of South American biomass burning aerosol emissions on the regional climate, Atmos. Chem. Phys., 18, 5321–5342, https://doi.org/10.5194/acp-18-5321-2018, 2018.
Tian, H., Lu, C., Ciais, P., Michalak, A. M., Canadell, J. G., Saikawa, E., Huntzinger, D. N., Gurney, K. R., Sitch, S., Zhang, B., Yang, J., Bousquet, P., Bruhwiler, L., Chen, G., Dlugokencky, E., Friedlingstein, P., Melillo, J., Pan, S., Poulter, B., Prinn, R., Saunois, M., Schwalm, C. R., and Wofsy, S. C.: The terrestrial biosphere as a net source of greenhouse gases to the atmosphere, Nature, 531, 225–228, https://doi.org/10.1038/nature16946, 2016.
Tsimpidi, A. P., Karydis, V. A., Pandis, S. N., and Lelieveld, J.: Global-scale combustion sources of organic aerosols: sensitivity to formation and removal mechanisms, Atmos. Chem. Phys., 17, 7345–7364, https://doi.org/10.5194/acp-17-7345-2017, 2017.
Urbanski, S. P., Hao, W. M. and Baker, S.: Chapter 4 Chemical Composition of Wildland Fire Emissions, Dev. Environ. Sci., 8, 79–107, https://doi.org/10.1016/S1474-8177(08)00004-1, 2008.
van der Velde, I. R., van der Werf, G. R., Houweling, S., Eskes, H. J., Veefkind, J. P., Borsdorff, T., and Aben, I.: Biomass burning combustion efficiency observed from space using measurements of CO and NO2 by the TROPOspheric Monitoring Instrument (TROPOMI), Atmos. Chem. Phys., 21, 597–616, https://doi.org/10.5194/acp-21-597-2021, 2021.
Vasilkov, A., Krotkov, N., Yang, E.-S., Lamsal, L., Joiner, J., Castellanos, P., Fasnacht, Z., and Spurr, R.: Explicit and consistent aerosol correction for visible wavelength satellite cloud and nitrogen dioxide retrievals based on optical properties from a global aerosol analysis, Atmos. Meas. Tech., 14, 2857–2871, https://doi.org/10.5194/amt-14-2857-2021, 2021.
Vay, S. A., Tyler, S. C., Choi, Y., Blake, D. R., Blake, N. J., Sachse, G. W., Diskin, G. S., and Singh, H. B.: Sources and transport of Δ14C in CO2 within the Mexico City Basin and vicinity, Atmos. Chem. Phys., 9, 4973–4985, https://doi.org/10.5194/acp-9-4973-2009, 2009.
Veres, P. R., Neuman, J. A., Bertram, T. H., Assaf, E., Wolfe, G. M., Williamson, C. J., Weinzierl, B., Tilmes, S., Thompson, C. R., Thames, A. B., Schroder, J. C., Saiz-Lopez, A., Rollins, A. W., Roberts, J. M., Price, D., Peischl, J., Nault, B. A., Møller, K. H., Miller, D. O., Meinardi, S., Li, Q., Lamarque, J.-F., Kupc, A., Kjaergaard, H. G., Kinnison, D., Jimenez, J. L., Jernigan, C. M., Hornbrook, R. S., Hills, A., Dollner, M., Day, D. A., Cuevas, C. A., Campuzano-Jost, P., Burkholder, J., Bui, T. P., Brune, W. H., Brown, S. S., Brock, C. A., Bourgeois, I., Blake, D. R., Apel, E. C., and Ryerson, T. B.: Global airborne sampling reveals a previously unobserved dimethyl sulfide oxidation mechanism in the marine atmosphere, P. Natl. Acad. Sci. USA, 117, 4505, https://doi.org/10.1073/pnas.1919344117, 2020.
Wang, S., Coggon, M. M., Gkatzelis, G. I., Warneke, C., Bourgeois, I., Ryerson, T. B., Peischl, J., Veres, P. R., Neuman, J. A., Hair, J., Shingler, T., Fenn, M., Diskin, G., Huey, L. G., Lee, Y. R., Apel, E. C., Hornbrook, R. S., Hills, A. J., Hall, S. R., Ullmann, K., Bela, M. M., Trainer, M. K., Kumar, R., Orlando, J. J., Flocke, F. M., and Emmons, L. K.: Chemical Tomography in a Fresh Wildland Fire Plume: a Large Eddy Simulation (LES) Study, J. Geophys. Res.-Atmos., 126, e2021JD035203, https://doi.org/10.1029/2021JD035203, 2021.
Ward, D. S., Kloster, S., Mahowald, N. M., Rogers, B. M., Randerson, J. T., and Hess, P. G.: The changing radiative forcing of fires: global model estimates for past, present and future, Atmos. Chem. Phys., 12, 10857–10886, https://doi.org/10.5194/acp-12-10857-2012, 2012.
Warneke, C., Roberts, J. M., Veres, P., Gilman, J., Kuster, W. C., Burling, I., Yokelson, R., and de Gouw, J. A.: VOC identification and inter-comparison from laboratory biomass burning using PTR-MS and PIT-MS, Int. J. Mass Spectrom., 303, 6–14, https://doi.org/10.1016/j.ijms.2010.12.002, 2011.
Warneke, C., Schwarz, J. P., Dibb, J., Kalashnikova, O., Frost, G., Al-Saad, J., Brown, S. S., Brewer, W. A., Soja, A., Seidel, F. C., Washenfelder, R. A., Wiggins, E. B., Moore, R. H., Anderson, B. E., Jordan, C., Yacovitch, T. I., Herndon, S. C., Liu, S., Kuwayama, T., Jaffe, D., Johnston, N., Selimovic, V., Yokelson, R., Giles, D. M., Holben, B. N., Goloub, P., Popovici, I., Trainer, M., Kumar, A., Pierce, R. B., Fahey, D., Roberts, J., Gargulinski, E. M., Peterson, D. A., Ye, X., Thapa, L. H., Saide, P. E., Fite, C. H., Holmes, C. D., Wang, S., Coggon, M. M., Decker, Z. C. J., Stockwell, C. E., Xu, L., Gkatzelis, G., Aikin, K., Lefer, B., Kaspari, J., Griffin, D., Zeng, L., Weber, R., Hastings, M., Chai, J., Wolfe, G. M., Hanisco, T. F., Liao, J., Campuzano Jost, P., Guo, H., Jimenez, J. L., and Crawford, J.: Fire Influence on Regional to Global Environments and Air Quality (FIREX-AQ), J. Geophys. Res.-Atmos., 128, e2022JD037758, https://doi.org/10.1029/2022JD037758, 2023.
Weibring, P., Richter, D., Walega, J. G., and Fried, A.: First demonstration of a high performance difference frequency spectrometer on airborne platforms, Opt. Express, 15, 13476–13495, https://doi.org/10.1364/OE.15.013476, 2007.
Westerling, A. L., Hidalgo, H. G., Cayan, D. R., and Swetnam, T. W.: Warming and Earlier Spring Increase Western U.S. Forest Wildfire Activity, Science, 313, 940–943, https://doi.org/10.1126/science.1128834, 2006.
Wiedinmyer, C. and Hurteau, M. D.: Prescribed Fire As a Means of Reducing Forest Carbon Emissions in the Western United States, Environ. Sci. Technol., 44, 1926–1932, https://doi.org/10.1021/es902455e, 2010.
Wiedinmyer, C., Akagi, S. K., Yokelson, R. J., Emmons, L. K., Al-Saadi, J. A., Orlando, J. J., and Soja, A. J.: The Fire INventory from NCAR (FINN): a high resolution global model to estimate the emissions from open burning, Geosci. Model Dev., 4, 625–641, https://doi.org/10.5194/gmd-4-625-2011, 2011.
Wiggins, E. B., Soja, A. J., Gargulinski, E., Halliday, H. S., Pierce, R. B., Schmidt, C. C., Nowak, J. B., DiGangi, J. P., Diskin, G. S., Katich, J. M., Perring, A. E., Schwarz, J. P., Anderson, B. E., Chen, G., Crosbie, E. C., Jordan, C., Robinson, C. E., Sanchez, K. J., Shingler, T. J., Shook, M., Thornhill, K. L., Winstead, E. L., Ziemba, L. D., and Moore, R. H.: High Temporal Resolution Satellite Observations of Fire Radiative Power Reveal Link Between Fire Behavior and Aerosol and Gas Emissions, Geophys. Res. Lett., 47, e2020GL090707, https://doi.org/10.1029/2020GL090707, 2020.
Wolfe, G. M., Hanisco, T. F., Arkinson, H. L., Blake, D. R., Wisthaler, A., Mikoviny, T., Ryerson, T. B., Pollack, I., Peischl, J., Wennberg, P. O., Crounse, J. D., St. Clair, J. M., Teng, A., Huey, L. G., Liu, X., Fried, A., Weibring, P., Richter, D., Walega, J., Hall, S. R., Ullmann, K., Jimenez, J. L., Campuzano-Jost, P., Bui, T. P., Diskin, G., Podolske, J. R., Sachse, G., and Cohen, R. C.: Photochemical evolution of the 2013 California Rim Fire: synergistic impacts of reactive hydrocarbons and enhanced oxidants, Atmos. Chem. Phys., 22, 4253–4275, https://doi.org/10.5194/acp-22-4253-2022, 2022.
Xu, L., Crounse, J. D., Vasquez, K., T., Allen, H., Wennberg, P. O., Bourgeois, I., Brown, S. S., Campuzano-Jost, P., Coggon, M. M., Crawford, J. H., DiGangi J. P., Diskin, G. S., Fried, A., Gargulinski, E. M., Gilman, J. B., Gkatzelis, G. I., Guo, H., Hair, J. W., Hall, S. R., Halliday, H. A., Hanisco, T. F., Hannun, R. A., Holmes, C. D., Huey, L. G., Jimenez, J. L., Lamplugh, A., Lee, Y. R., Liao, J., Lindaas, J., Neuman, J. A., Nowak, J. B., Peischl, J., Peterson, D. A., Piel, F., Richter, D., Rickly, P. S., Robinson, M. A., Rollins, A. W., Ryerson, T. B., Sekimoto, K., Selimovic, V., Shingler, T., Soja, A. J., St. Clair, J. M., Tanner, D. J., Ullmann, K., Veres P. R., Walega, J., Warneke, C., Washenfelder, R. A., Weibring, P., Wisthaler, A., Wolfe, G. M., Womack, C. C., and Yokelson, R. J.: Ozone chemistry in western U.S. wildfire plumes, Sci. Adv., 7, eabl3648, https://doi.org/10.1126/sciadv.abl3648, 2021.
Yokelson, R. J., Griffith, D. W. T., and Ward, D. E.: Open-path Fourier transform infrared studies of large-scale laboratory biomass fires, J. Geophys. Res.-Atmos., 101, 21067–21080, https://doi.org/10.1029/96JD01800, 1996.
Yokelson, R. J., Christian, T. J., Karl, T. G., and Guenther, A.: The tropical forest and fire emissions experiment: laboratory fire measurements and synthesis of campaign data, Atmos. Chem. Phys., 8, 3509–3527, https://doi.org/10.5194/acp-8-3509-2008, 2008.
Yokelson, R. J., Burling, I. R., Gilman, J. B., Warneke, C., Stockwell, C. E., de Gouw, J., Akagi, S. K., Urbanski, S. P., Veres, P., Roberts, J. M., Kuster, W. C., Reardon, J., Griffith, D. W. T., Johnson, T. J., Hosseini, S., Miller, J. W., Cocker III, D. R., Jung, H., and Weise, D. R.: Coupling field and laboratory measurements to estimate the emission factors of identified and unidentified trace gases for prescribed fires, Atmos. Chem. Phys., 13, 89–116, https://doi.org/10.5194/acp-13-89-2013, 2013.
Yuan, B., Koss, A., Warneke, C., Gilman, J. B., Lerner, B. M., Stark, H., and de Gouw, J. A.: A high-resolution time-of-flight chemical ionization mass spectrometer utilizing hydronium ions (H3O+ ToF-CIMS) for measurements of volatile organic compounds in the atmosphere, Atmos. Meas. Tech., 9, 2735–2752, https://doi.org/10.5194/amt-9-2735-2016, 2016.
Yue, C., Ciais, P., Cadule, P., Thonicke, K., and van Leeuwen, T. T.: Modelling the role of fires in the terrestrial carbon balance by incorporating SPITFIRE into the global vegetation model ORCHIDEE – Part 2: Carbon emissions and the role of fires in the global carbon balance, Geosci. Model Dev., 8, 1321–1338, https://doi.org/10.5194/gmd-8-1321-2015, 2015.
Zarzana, K. J., Selimovic, V., Koss, A. R., Sekimoto, K., Coggon, M. M., Yuan, B., Dubé, W. P., Yokelson, R. J., Warneke, C., de Gouw, J. A., Roberts, J. M., and Brown, S. S.: Primary emissions of glyoxal and methylglyoxal from laboratory measurements of open biomass burning, Atmos. Chem. Phys., 18, 15451–15470, https://doi.org/10.5194/acp-18-15451-2018, 2018.
Zhao, X. and Wang, L.: Atmospheric Oxidation Mechanism of Furfural Initiated by Hydroxyl Radicals, J. Phys. Chem. A, 121, 3247–3253, https://doi.org/10.1021/acs.jpca.7b00506, 2017.
Zheng, W., Flocke, F. M., Tyndall, G. S., Swanson, A., Orlando, J. J., Roberts, J. M., Huey, L. G., and Tanner, D. J.: Characterization of a thermal decomposition chemical ionization mass spectrometer for the measurement of peroxy acyl nitrates (PANs) in the atmosphere, Atmos. Chem. Phys., 11, 6529–6547, https://doi.org/10.5194/acp-11-6529-2011, 2011.