the Creative Commons Attribution 4.0 License.
the Creative Commons Attribution 4.0 License.
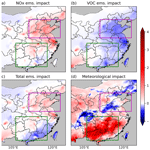
Aggravated surface O3 pollution primarily driven by meteorological variations in China during the 2020 COVID-19 pandemic lockdown period
Yi Wang
Daven K. Henze
Due to the lockdown during the COVID-19 pandemic in China from late January to early April in 2020, a significant reduction in primary air pollutants, as compared to the same time period in 2019, has been identified by satellite and ground observations. However, this reduction is in contrast with the increase of surface ozone (O3) concentration in many parts of China during the same period from 2019 to 2020. The reasons for this contrast are studied here from two perspectives: emission changes and inter-annual meteorological variations. Based on top-down constraints of nitrogen oxide (NOx) emissions from TROPOMI measurements and GEOS-Chem model simulations, our analysis reveals that NOx and volatile organic compound (VOC) emission reductions as well as meteorological variations lead to 8 %, −3 % and 1 % changes in O3 over North China, respectively. In South China, however, we find that meteorological variations cause ∼ 30 % increases in O3, which is much larger than −1 % and 2 % changes due to VOC and NOx emission reductions, respectively, and the overall O3 increase in the simulations is consistent with the surface observations. The higher temperature associated with the increase in solar radiation and the decreased relative humidity are the main reasons that led to the surface O3 increase in South China. Collectively, inter-annual meteorological variations had a larger impact than emission reductions on the aggravated surface O3 pollution in China during the lockdown period of the COVID-19 pandemic.
- Article
(14812 KB) - Full-text XML
- BibTeX
- EndNote
Surface ozone (O3), an important air pollutant that is harmful to human health (Jerrett et al., 2009) and stomatal conductance of green vegetation (Gong et al., 2020), is produced by photochemical reactions of nitrogen oxides (NOx) and volatile organic compounds (VOCs; Liu et al., 1987; Sillman et al., 1990). In addition to emissions, meteorological conditions, such as temperature, solar radiation and relative humidity, also have large impacts on surface O3 formation (Lu et al., 2019).
Ground observations show that surface O3 increased dramatically during the COVID-19 lockdown period in China by around 40 % on average (Tong et al., 2023) and even more than 100 % (Shi and Brasseur, 2020; Liu et al., 2021) depending on the time period and region. The reduction in economic activities during the lockdown period led to a significant decrease in several primary air pollutant emissions. The NO2 vertical column density (VCD) from satellite measurements and the surface NO2 concentrations from ground measurements were reduced by 40 %–60 % in China during the lockdown period (Bauwens et al., 2020; Shi and Brasseur, 2020; F. Liu et al., 2020; Zhang et al., 2020). A lower but discernible reduction in sulfur dioxide (SO2), carbon monoxide (CO) and formaldehyde (HCHO) has also been identified by satellite or ground-based observations in China (Shi and Brasseur, 2020; Levelt et al., 2022; Ghahremanloo et al., 2021). However, during this period, surface O3 concentrations increased, and the respective roles of meteorological factors and emission reduction for the aggravated surface O3 pollution during the lockdown in China need to be further quantified.
This study provides a quantitative analysis of the causes for the unexpectedly aggravated surface O3 pollution in China during the lockdown period of the pandemic from two perspectives using the GEOS-Chem model. One perspective involves anthropogenic emission reduction of NOx and VOC in response to the lockdown, possibly under a VOC-limiting chemical regime of surface O3 production (Guo et al., 2023). The other perspective involves the impact of natural variability in meteorological conditions. Previous studies have reported the enhanced surface O3 due to NOx emission decline during the lockdown period in North China using chemical transport model (CTM) simulations without controlling for the impacts of meteorological variability (Zhang et al., 2021; Huang et al., 2020; Miyazaki et al., 2020). Other studies quantified or excluded the meteorological impacts on surface O3 using statistical analysis instead of CTMs that account for the physical and chemical processes (Venter et al., 2020; Bi et al., 2022; Tong et al., 2023). Although a few studies have investigated the contributions from both emission reduction and meteorological variability to surface O3 increase using CTMs, most of their results have uncertainties due to the limitations of their analysis. For example, some of them keep the emissions unchanged (Zhao et al., 2020) or assume an arbitrarily uniform emission reduction instead of constraining the emission based on observations (Le et al., 2020; Liu et al., 2021). In cases where the emissions were constrained by the observations, the focus was limited to several cities in China (T. Liu et al., 2020). Furthermore, in these past studies, the surface O3 increase during the lockdown period of 2–4 weeks is quantified in reference to the time period right before the lockdown, instead of the same period in previous years; such comparisons, by design, cannot exclude the possibility that the seasonal variation in meteorology from early January to early April may have dominated the cause for the surface O3 increase. A comprehensive analysis of the contributions from emission reductions and meteorological variations to the surface O3 increase during the first round of the lockdown period, with respect to the same time period in previous years in China, is therefore overdue.
Here, we apply a top-down method to update NOx and VOC emissions in February and March in 2020 based on the TROPOMI NO2 and formaldehyde (HCHO) product. GEOS-Chem model simulations, with NOx and VOC emissions and meteorological fields in different time periods, are then conducted. Based on the difference in surface O3 concentration in different modeling sensitivity experiments, we quantitatively assess the respective roles of emission and meteorology in regulating surface O3 concentration in continental China. The ground observations of surface O3 and NO2 concentrations are compared with the model simulations to verify our analysis. Section 2 introduces the satellite and ground-based measurements, NOx emission update scheme, and the configurations of GEOS-Chem simulation experiments. Section 3 provides an evaluation of the constrained NOx emission and surface O3 simulations, as well as the analysis of the mechanism of the aggravated surface O3 pollution. The summary and conclusions are presented in Sect. 4.
2.1 TROPOMI NO2 and HCHO product
We used tropospheric NO2 and the HCHO level 2 VCD product provided by the TROPOspheric Monitoring Instrument (TROPOMI) on board the Sentinel-5 Precursor (S5P) satellite (Veefkind et al., 2012). S5P is a sun-synchronous polar orbit satellite launched on 13 October 2017, which covers the near-global domain in a single day. TROPOMI provides NO2 and HCHO retrievals at an approximately 7 km × 3.5 km spatial resolution (5.5 km × 3.5 km since 6 August 2019) from the ascending orbit, with an equatorial crossing time of ∼ 13:30 LT (local time; Van Geffen et al., 2020; De Smedt et al., 2018). The datasets were obtained from the NASA Goddard Earth Sciences Data and Information Services Center (https://daac.gsfc.nasa.gov, last access: 16 August 2023). A quality control procedure similar to Bauwens et al. (2020) but with slightly stricter criteria is adopted for TROPOMI NO2 and HCHO data. The TROPOMI retrievals, under one or more than one of the following conditions, are screened out for data quality control: (1) the quality assurance value is no larger than 0.5, (2) the cloud radiance fraction within the NO2 or HCHO retrieval window is larger than 0.3, (3) the solar zenith angle is larger than 70°, and (4) the viewing zenith angle is larger than 70°.
2.2 Ground O3 and NO2 measurements
Surface measurements of O3 and NO2 were collected from ∼ 1600 operational air quality monitoring stations, over mainland China managed by the China National Environmental Monitoring Centre (http://www.cnemc.cn/en/, last access: 5 September 2023). We calculated daily maximum 8 h average (MDA8) O3 concentration from hourly in situ measurements. Surface O3 is measured by the ultraviolet photometric method and indigo disulfonate spectrophotometry, following the national environmental standards of HJ 590-2010 and HJ 504-2009. Surface NO2 concentrations are measured by the chemiluminescence method (Zhang and Cao, 2015) that quantifies the NO2 concentrations by measuring the NO decomposed from NO2, which can cause a positive bias in the NO2 measurements (Steinbacher et al., 2007) because NOz (compounds produced from the atmospheric oxidation of NOx) can also be decomposed to NO. The true NO2 concentrations only account for 43 %–76 % and 70 %–83 % of measured values for rural and urban sites (Steinbacher et al., 2007). Following Wang et al. (2020b), we also applied a correction factor but with a lower value of 0.75 to the measured NO2, considering that we included both rural and urban sites. The sampling ports are placed at 3 to 15 m above ground level (a.g.l.) following the national environmental monitoring method standard HJ 664-2013. The measured data are reported in the units of µg m−3 under standard temperature (273.15 K) and pressure (101.325 kPa) according to the national environmental standard GB 3095-2012.
2.3 The GEOS-Chem model and its adjoint
The global 3D chemical transport model GEOS-Chem (Bey et al., 2001) version 12.7.2 is used here. We apply the nested-grid version of GEOS-Chem (Chen et al., 2009; Wang et al., 2004) with the horizontal resolution of 0.25° × 0.3125° and 47 vertical hybrid-sigma levels over East Asia (15–55° N, 70–140° E). The boundary conditions are obtained from the 2° × 2.5° global simulation. The model is driven by the GEOS-FP meteorological field provided by the NASA Global Modeling and Assimilation Office (GMAO). A detailed O3–NOx–hydrocarbon chemistry (Mao et al., 2010, 2013; Travis et al., 2016) is included in the GEOS-Chem model. The altitude of the surface O3 output from GEOS-Chem is specified at 9 to match the in situ measurements (Travis et al., 2017; Zhang et al., 2012). Through our sensitivity test using GEOS-Chem, the variation in surface O3 from 3 to 9 m a.g.l. is generally less than 0.723 ppb (75th percentile), and the median bias is 0.283 ppb. Travis et al. (2017) reported that from 60 to 10 , the MDA8 O3 could decrease by ∼ 3 ppb. Therefore, when comparing GEOS-Chem surface O3 with in situ measurements, the differences caused by inconsistent reported altitudes (9 m versus 3–15 m) can be ignored.
The global anthropogenic emission used in the GEOS-Chem model is the Community Emissions Data System (CEDS) inventory (Hoesly et al., 2018), which is replaced by the MIX inventory (Li et al., 2017) over the Asian region. Biogenic emissions for VOCs follow the Model of Emissions of Gases and Aerosols from Nature (MEGAN) inventory (Guenther et al., 2012). Natural NOx emissions include biomass burning from the GFED4 inventory (Van Der Werf et al., 2017), soil NOx emissions (Hudman et al., 2012) and lightning sources (Murray et al., 2012; Ott et al., 2010).
The adjoint of the GEOS-Chem model (Henze et al., 2007; Henze et al., 2009) is a component of the four-dimensional variational (4D-Var) inversion method that can efficiently optimize spatially disaggregated aerosol and gas emissions. This is done simultaneously through iterative minimization of a cost function using the model adjoint to calculate the gradient of the cost function with respect to a large number of model parameters (such as anthropogenic NOx emissions in each grid box). The cost function is the sum of the error-weighted difference between forward model outputs and observations and the divergence of posterior model parameters from the prior estimate (Sect. 2.4). We developed and validated the observation operator for TROPOMI NO2 in the GEOS-Chem adjoint model version 35n similarly to Wang et al. (2020a) and used it to optimize the anthropogenic NOx emission during the lockdown period in China. The monthly NOx emission optimization is implemented using the 4D-Var method with the GEOS-Chem adjoint at a nested grid resolution of 0.25° × 0.3125° by assimilating the daily TROPOMI NO2 measurements. The prior anthropogenic NOx emission used in the GEOS-Chem adjoint is HTAP version 2 (Janssens-Maenhout et al., 2015), which is equivalent to the MIX inventory in East Asia (Li et al., 2017).
2.4 NOx and VOC emission updates
Two approaches are used to update the emissions during the lockdown period in 2020. The first is a simple mass balance approach (Leue et al., 2001; Martin et al., 2003; Vinken et al., 2014) for updating the NOx emission by assuming a constant NOx lifetime and ratio. In the period from 2010 to 2019, the anthropogenic NOx emissions declined significantly as a result of the clean air actions of the Chinese government (Zheng et al., 2018). We scale the anthropogenic NOx emission from year 2010 to 2019 using the spatially gridded ratio of mean TROPOMI tropospheric NO2 VCD in February–March 2019 to the GEOS-Chem-simulated NO2 column, with a default MIX 2010 emission (Appendix A) to obtain the baseline anthropogenic NOx emission in 2019, which is denoted as MIX 2019. To derive anthropogenic NOx emissions in 2020 in China during the COVID-19 lockdown (MIX 2020), the spatially gridded ratio of mean TROPOMI tropospheric NO2 VCD in February–March 2020 to that in February–March 2019 is taken as a scaling factor for the updated baseline anthropogenic NOx emission in 2019 (MIX 2019). The 2-month means of TROPOMI NO2 VCD in 2019 and 2020 are calculated with the physical oversampling procedure (Sun et al., 2018). Scaling factors in regions where the mean TROPOMI tropospheric NO2 VCD in February–March 2019 is less than 0.1 Dobson units (DU) are set to 1 for emission updates in both 2020 and 2019, assuming that the lockdown only affects the populated areas (that have high NO2 in 2019).
The second method for updating NOx emission is 4D-Var via the GEOS-Chem adjoint model. The anthropogenic NOx emissions in the 2020 lockdown period derived from the GEOS-Chem adjoint are hereafter denoted as 2020 Adjoint. Following Wang et al. (2020a), the cost function J for optimizing the NOx emission is defined as
where s is the tropospheric slant column density of TROPOMI NO2, which is the product of TROPOMI NO2 VCD and air mass factor. H is the TROPOMI NO2 observation operator that maps the modeled NO2 concentrations c to the observations in time and space and calculates the corresponding slant column density to make an apple-to-apple comparison of the model to TROPOMI. Ω is the spatial and temporal domain where both model simulations and observations are available. σ is the scaling factor of anthropogenic NOx emissions to be optimized, and σa is the prior emission scaling factor, which equals 1. Sobs and Sa are observational and prior error covariance matrices, respectively. γ is the regularization factor that balances the weights of the observational term and prior term. We assumed Sobs to be diagonal, following Wang et al. (2020a), with the diagonal values calculated as the square of the standard error in tropospheric NO2 slant column density from the TROPOMI product. The prior error in the NOx emissions is assumed to be 100 %. The spatial correlation of NOx emissions is considered in this study, and the off-diagonal elements of Sa are computed by assuming an exponentially decaying error correlation with a fixed decaying distance of 150 km following Qu et al. (2017). The γ value was determined as 500 via the total error minimization and L-curve test (Henze et al., 2009; Qu et al., 2017).
We developed the observation operator for the TROPOMI NO2 product in the GEOS-Chem adjoint model, with the GEOS-Chem NO2 vertical profiles and TROPOMI NO2 averaging kernel applied to minimize the discrepancies between the assumptions in the TROPOMI NO2 retrieval and GEOS-Chem model simulation. See Appendix B for additional details. The observation operator has been validated using the finite difference method (Appendix C).
For an anthropogenic VOC emissions update, we only applied the mass balance method based on the TROPOMI HCHO data. The default anthropogenic VOC emissions used in the GEOS-Chem are also part of the MIX 2010 inventory (Li et al., 2017). We ignore the change in anthropogenic VOC emissions from 2010 to 2019 (Appendix D). The baseline VOC emission in 2019 (MIX 2019) is identical to that of MIX 2010. The updated anthropogenic VOC emissions during the lockdown period are denoted as MIX 2020. HCHO is one species of VOC and may not be able to represent other VOC species. Unlike NOx, biogenic sources, meteorological impacts and the large retrieval uncertainties of HCHO (due to its low optical depth) prevent accurately quantifying the emission decline due to lockdown from satellite retrievals (Levelt et al., 2022). Vigouroux et al. (2020) reported that TROPOMI HCHO tends to be overestimated by ∼ 26 % for the HCHO column lower than 2.5 × 1015 and underestimated by ∼ 31 % for the HCHO column higher than 8.0 × 1015 . To optimize the signal, we spatially aggregate the ratio of TROPOMI HCHO in February–March 2020 to that in February–March 2019 to the resolution of 0.5°, which is used as the scaling factor for updating the anthropogenic VOC emissions during the lockdown period. The aggregation is based on the oversampling of TROPOMI HCHO at a 0.01° resolution, and the ratio is computed as the mean of the lowest 25th percentile of all ratios at a 0.01° resolution in each 0.5° × 0.5° grid box, which ensures that only statistically significant changes are considered. We assumed the change in anthropogenic VOC emissions over sparsely populated areas (TROPOMI NO2 in February–March 2019 less than 0.1 DU) is insignificant and assigned the ratio values as 1. To further evaluate the uncertainties associated with this approach, we also conducted a sensitivity study using a different threshold in the aggregation.
We assess the results from model experiments (as described in Sect. 2.5), adopting the updated NOx emission by comparing mean tropospheric NO2 VCD from GEOS-Chem and TROPOMI observations in February–March of 2019 and 2020. The averaging kernel of TROPOMI NO2 is applied to the modeled NO2 column for this comparison, following Sha et al. (2021). A further quantitative evaluation of the model results also used the TROPOMI measurements of HCHO and surface observations of O3 and NO2.
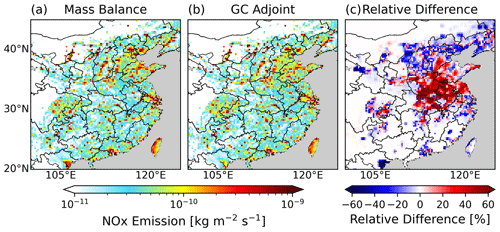
Figure 1Updated anthropogenic NOx emission during February–March 2020 from (a) the mass balance method and (b) the 4D-Var method as well as (c) their relative difference. The relative difference is calculated by subtracting the value in panel (a) from panel (b) and then dividing the difference by panel (a).
2.5 GEOS-Chem model experiments
A series of sensitivity experiments are conducted over China with different NOx and VOC emissions and GEOS-FP meteorological fields in different years using the GEOS-Chem (version 12.7.2) model. All simulations are conducted from 15 January to 31 March. The 17 d before 1 February is used for spin-up, and the model outputs for February and March are used for the analysis. The configurations of different simulations are listed in Table 1.
We use the following equations to quantify the contributions from NOx and VOC emission reductions due to COVID-19 and meteorological variations to the increase in surface O3 as follows:
where , and are the relative differences in surface O3 concentration caused by emission decline in NOx, VOC, and both NOx and VOC resulting from COVID-19. represents the relative contribution to the surface O3 change from the meteorological variation between 2 years. , , , , and are mean MDA8 surface O3 concentrations simulated by the modeling experiments Baseline (2019), 2020 Default, 2020 NOx, 2020 VOC, 2020 Lockdown and 2020 Adjoint, respectively (Table 1).
The difference in simulated surface O3 between 2020 and 2019 is the result of both emission reductions and meteorological variations and is denoted as . It is calculated and evaluated against the observed relative difference in mean MDA8 O3 in February to March between 2019 and 2020 at all ground sites as follows:
3.1 Changes in NOx and VOC emissions during COVID-19
We updated the anthropogenic NOx emissions during the COVID-19 lockdown using both the 4D-Var method and mass balance method (Figs. 1 and 2). The NOx emissions from the 4D-Var inversion share a similar spatial pattern and magnitude with those found using the mass balance method (Fig. 1). However, the NOx emissions from the 4D-Var inversion are lower overall than those from the mass balance method over North China by ∼ 10 % and larger over central China by ∼ 40 %. Figure 2a and b show that the 4D-Var NOx emission reduction is more severe over urban regions and displays a smoother spatial pattern than that from the mass balance approach, which is caused by the arbitrary cutoff with 0.1 DU of NO2 VCD in the latter. Furthermore, the 4D-Var inversion captured the NOx emission decline in northeast China where the mass balance approach did not because of the low NO2 VCD. During February–March 2020, the anthropogenic NOx emissions in East China decreased by ∼ 30 % compared to those in the same period in 2019.
We also scale the anthropogenic VOC emissions based on the TROPOMI HCHO data (Fig. 2c). The VOC emissions decrease by ∼ 20 %–30 % in East Asia and South Asia. The anthropogenic VOC emission changes in sparsely populated areas over northwest China are neglected. TROPOMI HCHO data cannot distinguish the anthropogenic emissions from biogenic and biomass burning sources for the Indochinese Peninsula in Southeast Asia because of the dense vegetation in this region. However, this study investigated the O3 pollution in China; Southeast Asia, with its dense vegetation, is outside our study domain. The impact of a VOC emission bias in Southeast Asia on surface O3 pollution in China is negligible, considering the generally short lifetime of biogenic VOC (Atkinson, 2000). For the populated urban regions in China, where the surface O3 pollution exerts more significant health impacts, the anthropogenic source dominates the VOC emissions (Williams and Koppmann, 2007).
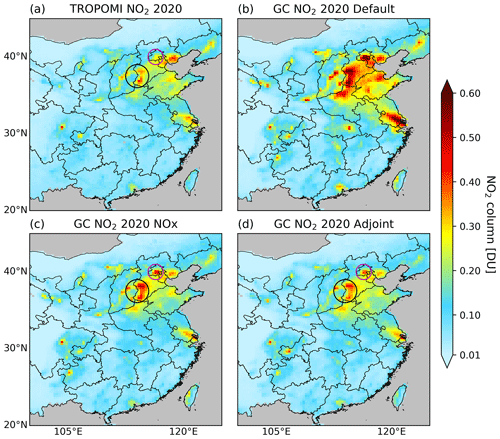
Figure 3Comparison of tropospheric NO2 VCD from (a) the TROPOMI product in February–March 2020 with that from the GEOS-Chem (b) 2020 Default simulation, (c) 2020 NOx simulation and (d) 2020 Adjoint simulation. The pink and black circles mark the areas where NOx emissions from 4D-Var mitigated the NO2 overestimation by the mass balance method. The emissions and meteorology configurations are listed in Table 1 for the GEOS-Chem 2020 Default simulation, 2020 NOx simulation and 2020 Adjoint simulation.
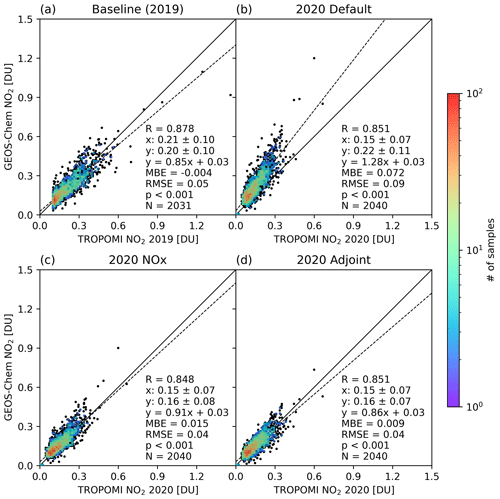
Figure 4Scatterplot of TROPOMI NO2 VCD versus the GEOS-Chem simulations for (a) Baseline (2019), (b) 2020 Default, (c) 2020 NOx and (d) 2020 Adjoint. TROPOMI data in February–March of 2019 were used in panel (a), and those of 2020 were used in panels (b)–(d). The emissions and meteorology configurations for GEOS-Chem simulations are listed in Table 1. Only pixels larger than 0.1 DU, with TROPOMI NO2 VCD in February–March 2019, are included in all comparisons.
3.2 Validation of NO2 simulations
We further assess our updated anthropogenic NOx emissions by comparing the NO2 VCD from TROPOMI with that from GEOS-Chem with the anthropogenic NOx emissions before and after the scaling (Figs. 3 and 4). Before updating the NOx emissions, the 2020 Default (Fig. 3b) simulation significantly overestimates the NO2 VCD compared to the TROPOMI NO2 observations (Fig. 3a). With the NOx emissions updated, the 2020 NOx (Fig. 3c) and 2020 Adjoint (Fig. 3d) simulations exhibit a much better agreement with the TROPOMI NO2 observation than the 2020 Default observation. However, Fig. 3c shows that the GEOS-Chem simulation with the NOx emissions from the mass balance approach overestimated the NO2 VCD over Beijing and the southwest Hebei Province (pink and black circles in Fig. 3) compared with TROPOMI data. The reason is that scaling factors are applied only to anthropogenic NOx emissions, not total NOx emissions, so it is expected that the model may still overestimate the NO2 column after scaling part of the total NOx emission. With the anthropogenic NOx emissions optimized by the 4D-Var method, the overestimation of NO2 VCD over Beijing and the southwest Hebei Province (pink and black circles in Fig. 3) is mitigated compared with the NOx emissions from the mass balance approach.
Figure 4 further displays the statistics for the comparison between the TROPOMI NO2 and GEOS-Chem simulations via the scatterplot. The Baseline (2019) simulation captures the magnitude of NO2 VCD observations in 2019 well (Fig. 4a). The root mean square error (RMSE) and mean bias error (MBE) for the simulation with 2020 NOx emissions derived from the mass balance method (Fig. 4b) decreased by 0.050 and 0.057 DU as compared to the 2020 Default (Fig. 4c) simulation. Compared with the result from the GEOS-Chem simulation 2020 NOx, emissions from the 2020 Adjoint simulation (Fig. 4d) further led to the reduction in the MBE of the NO2 VCD by 0.006 DU and improved the correlation coefficient by 0.003. The significant overestimation of several pixels, with the TROPOMI NO2 VCD larger than 0.4 DU by the simulation 2020 NOx, is also mitigated by the 2020 Adjoint simulation. The MBEs between GEOS-Chem and TROPOMI for the Baseline (2019), 2020 NOx and 2020 Adjoint simulations are −0.004, 0.015 and 0.009 DU, respectively. The corresponding relative biases are 1.9 %, 10 % and 6.0 %, which are all less than the relative uncertainty of ∼ 30 % for the TROPOMI tropospheric NO2 VCD over East China (Van Geffen et al., 2022). The improved agreement between the simulation with updated NOx emission and TROPOMI NO2 provides a basis for further analyzing the mechanism of aggravated surface O3 pollution.
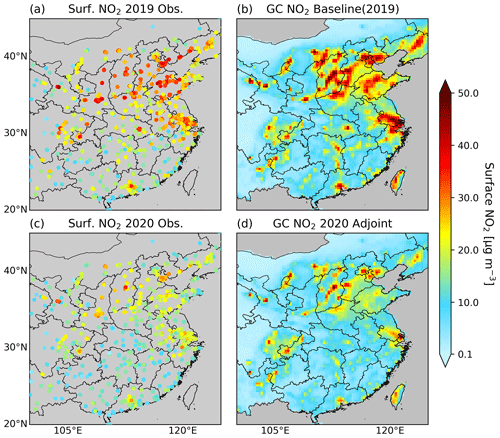
Figure 5Comparison of surface NO2 concentrations from ground measurements for (a) February–March 2019 and (c) February–March 2020 versus those from the GEOS-Chem (b) Baseline (2019) and (d) 2020 Adjoint simulations. Grey color means no data are presented.
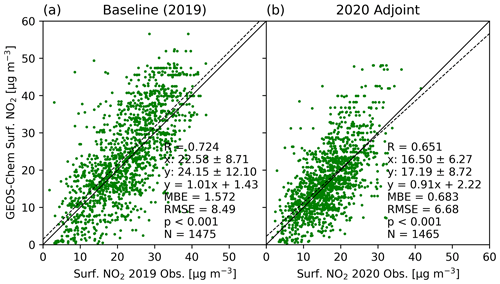
Figure 6Scatterplots for comparing the surface NO2 concentrations from the GEOS-Chem simulations and ground measurements. (a) The GEOS-Chem Baseline (2019) simulation versus ground measurements in February–March 2019. (b) The GEOS-Chem 2020 Adjoint simulation versus ground measurements in February–March 2020. Note that the number of ground sites differs in these 2 years.
Table 2Evaluation statistics for modeled surface NO2 compared with the in situ measurements.∗
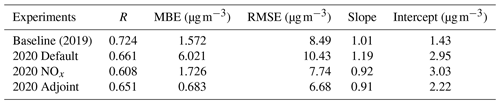
∗ The Baseline (2019) simulation experiment is compared with the ground measurements in February–March 2019. The other three experiments are compared with the ground measurements in February–March 2020.
Figures 5 and 6 show the comparison of surface NO2 between ground measurements and GEOS-Chem simulations. The GEOS-Chem Baseline (2019; Fig. 5b) and 2020 Adjoint (Fig. 5d) simulations capture both the spatial pattern and magnitude of surface NO2 measurements in February–March of 2019 (Fig. 5a) and 2020 (Fig. 5c) well, respectively. Figure 6 further displays the good agreements of surface NO2 from Baseline (2019) (Fig. 6a) and 2020 Adjoint (Fig. 6b) with the in situ measurements via scatterplots. Table 2 displays the evaluation statistics, including the correlation coefficient (R), MBE, RMSE, and the slope and intercept of the linear regression, for the simulated surface NO2 from various simulation experiments compared with the in situ measurements. The correlation coefficient, MBE and RMSE between the Baseline (2019) simulation and the ground measurements in February–March 2019 are 0.724, 1.572 and 8.49 µg m−3, respectively. Without updating the NOx emissions in 2020, the 2020 Default simulation overestimates the ground measurements of surface NO2 in February–March 2020 (Table 2). The slope for the linear regression is 1.19, and the MBE and RMSE are 6.021 and 10.43 µg m−3, respectively (Table 2). After updating the NOx emissions, the GEOS-Chem 2020 NOx and 2020 Adjoint simulations have good agreements with the in situ measurements in February–March 2020. The correlation coefficient between the simulation 2020 Adjoint and the in situ measurements is 0.651, which is higher than that of 0.608 for the simulation 2020 NOx versus the ground measurements (Table 2). The MBE and RMSE of 2020 Adjoint (0.683 and 6.68 µg m−3) are lower than those of 2020 NOx (1.726 and 7.74 µg m−3; Table 2). This result further indicates the superiority of 4D-Var for optimizing NOx emissions compared with the mass balance method (Cooper et al., 2017; Streets et al., 2013).
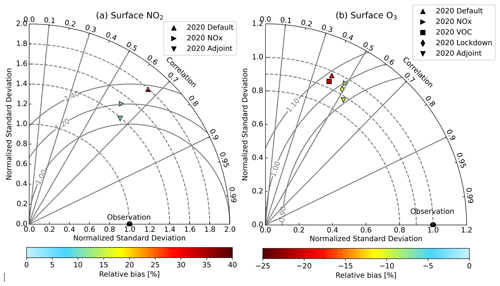
Figure 7Taylor diagram for evaluating the GEOS-Chem simulations of (a) surface NO2 and (b) surface O3 during the lockdown period (February–March 2020) using ground observations for different simulation experiments listed in Table 1. The evaluation of surface O3 only includes the areas where the NOx emissions, optimized by 4D-Var, reduced by more than 10 %.
Figure 7a is the Taylor diagram for evaluating the GEOS-Chem simulations of surface NO2 concentrations from the 2020 Default, 2020 NOx and 2020 Adjoint simulations using the in situ measurements. The 2020 Adjoint simulation (inverted triangle in Fig. 7a) has the best performance among these three simulations with the lowest relative bias and lowest normalized centered RMSE. Without updating the NOx emission, the 2020 Default simulation features a relative bias of ∼ 37 %. After updating the NOx emissions, the 2020 NOx simulation reduces the relative bias, the normalized centered RMSE and the normalized standard deviation from around 37 %, 1.38 and 1.80 to around 10 %, 1.20 and 1.51 compared with the 2020 Default simulation, but the correlation coefficient also decreases. Using the 4D-Var method, the 2020 Adjoint simulation further reduces the relative bias, normalized centered RMSE and normalized standard deviation and increases the correlation coefficient compared with the 2020 NOx simulation. We also validated the VOC emissions by comparing the simulated HCHO VCD with TROPOMI measurements (Appendix D).
3.3 Evaluation of surface O3 simulations
We evaluated the GEOS-Chem simulations of MDA8 surface O3 from different simulation experiments listed in Table 1 using ground measurements. Figure 7b is the Taylor diagram for comparing the surface O3 concentrations during February–March 2020 from the ground measurements and GEOS-Chem simulations. We focused on areas with significant NOx emissions reduction to better assess the role of updated NOx emissions in improving surface O3 simulations. The ground sites are excluded where the NOx emissions from 4D-Var decline by less than 10 %. The correlation coefficient between the Baseline (2019) simulation and ground observations is ∼ 0.53, and the relative bias is around −25 %. By applying 2020 meteorological fields and scaling the VOC emissions, the correlation coefficients decreased to ∼ 0.40 for model 2020 Default and 2020 VOC simulations, with little reduction in the relative bias. By updating the NOx emissions, the relative bias reduced to around −10 %, while the correlation coefficients remained at ∼ 0.50 for model simulations 2020 NOx, 2020 Lockdown and 2020 Adjoint. This indicates that the NOx emission updates significantly improve the surface O3 simulations. Comparing the simulations 2020 Default and 2020 VOC, or 2020 NOx and 2020 Lockdown, the results show that scaling VOC emissions does not improve the surface O3 simulations significantly over continental China; however, over South China, the VOC emissions update reduces the relative bias by 3 %. Among all simulations, 2020 Adjoint exhibits the best performance with the lowest normalized centered RMSE, the largest correlation coefficient and a low relative bias of ∼ 10 %. This result further confirms the superiority of the 4D-Var with respect to the mass balance method for optimizing NOx emissions. Therefore, we used the 2020 Adjoint to evaluate the impacts of NOx emission on surface O3 in the following analysis.
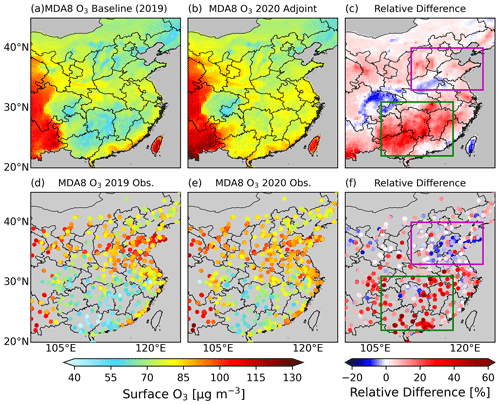
Figure 8Comparison of MDA8 surface O3 in 2019 and February–March 2020 and the relative difference between 2 years from the GEOS-Chem model simulations (a–c) versus ground observations (d–f). Also shown is the GEOS-Chem mean MDA8 O3 at 9 under standard temperature and pressure (STP; 273.15 K and 101.325 kPa) from the (a) Baseline (2019) and (b) 2020 Adjoint simulations (Table 1) together with (c) their relative difference. Ground observed mean MDA8 surface O3 under STP in (d) February–March 2019 and (e) February–March 2020 with (f) their relative difference. The pink and green boxes in panel (c) and panel (f) define the North China and South China domain.
Figure 8 compares the modeled surface O3 in February–March of 2019 (Fig. 8a) and 2020 (Fig. 8b) and the relative difference (Fig. 8c) computed from Eq. (6) with the in situ measurements (Fig. 8d–f). The ground observations show that the highest level of surface O3 pollution occurs in North China and in the southwest of China. The average MDA8 O3 in 2 months can reach up to ∼ 110 µg m−3 at STP (∼ 51.4 ppbv), which is higher than the China National Ambient Air Quality Standard daily maximum 8 h grade I standard of 100 µg m−3. The GEOS-Chem model underestimates the surface O3 over North China for both years compared with ground observations, which could be a result of the underestimation of biogenic VOC emissions (Appendix D). The underestimation of the simulated O3 over North China does not significantly affect our study purpose since this study focuses on revealing the impacts of emissions and meteorology change on the surface O3 change by each region. The bias is predominantly systematic and was substantially canceled when we computed the relative difference in the surface O3. The model captures well the magnitude and spatial distribution of surface O3 and the increasing trend in South China. In South China, the measured surface O3 in February–March 2020 increases by 30 %–50 %, while over North China, it increases generally by less than 20 % and even decreases in some regions. The relative differences in simulated surface O3 between 2 years is comparable to the ground observations over South China (green box in Fig. 8c and f). In the Sichuan Basin, the trend of the surface O3 change from the model is opposite to that of the measurements, which is probably caused by the inaccurate simulation of the meteorological effects (see Sect. 3.4) due to the complex terrain features in this region. The localized improvement of the model simulation is further needed for the regional study. Over North China (pink box in Fig. 8c and f), the average relative differences between 2 years from the model and observation are 4.27 % and −3.01 %, respectively, both of which are much smaller than their counterparts in South China. While the relative difference from model simulations has different signs as compared to that of observations on average, the change in O3 is indeed small and the model is able to capture the part of O3 decrease in the southwest part of the North China domain (Fig. 8c). We note that some previous studies showed a large increase in O3 in North China, but such an increase is in comparison with the O3 in the month right before the lockdown (not the same time in 2019; Shi and Brasseur, 2020; Liu et al., 2021).
3.4 Relative contribution from declining emissions and meteorological variations
Using Eqs. (2)–(5), we can analyze the mechanism of surface O3 increase in China during the COVID-19 pandemic (Fig. 9). NOx emission reduction as a result of the COVID-19 lockdown leads to a ∼ 8 % increase in the mean MDA8 surface O3 over North China (pink boxes in Fig. 9) between 2019 and February–March 2020 (Fig. 9a), while the VOC emission decline causes ∼ 3 % of O3 decrease (Fig. 9b). The average contribution of the meteorological variations to the surface O3 change is less than 1 % in North China (Fig. 9d). However, in South China, the inter-annual meteorological variations dominate the surface O3 increases, causing a ∼ 30 % increase (Fig. 9d), while the reduction in NOx and VOC emissions has little impact. The overall magnitude of emissions contribution to the surface O3 change over North China is ∼ 5 %, which is similar to that of the meteorological effects, but meteorological variations lead to both O3 increases and decreases in different regions. Over South China, the meteorological effect is much larger than the net effects of declining emissions. Overall, the impact of inter-annual meteorological variations between 2019 and 2020 is almost 30 times larger than the overall emissions impacts on the aggregated surface O3 pollution in China.
Our results are consistent with the conclusion from Zhao et al. (2020) that states that the meteorological variations have larger impacts than emissions reduction on surface O3 in the southern city of Guangzhou, but in Beijing, emission reduction has a larger impact during 23–29 January. Wang et al. (2022) also showed that ∼ 80 % of the O3 MDA8 increase during the COVID-19 lockdown period is caused by the meteorological factors and that ∼ 20 % is caused by the emission decline in East China. T. Liu et al. (2020) reported that the surface O3 increase in the major cites of the Yangtze River Delta region was driven by both emission reduction and meteorological variations to a similar degree from the pre-lockdown period (1–22 January 2020) to the lockdown period (23 January–29 February 2020). However, Zhao et al. (2020), Wang et al. (2022) and T. Liu et al. (2020) only focused on the lockdown period of 1 to 5 weeks in reference to the time period right before the lockdown instead of the same period in previous years, which did not provide a comprehensive analysis over the whole lockdown period, and Zhao et al. (2020) and T. Liu et al. (2020) did not separate the effects of the seasonal trend from the meteorological anomalies. Moreover, T. Liu et al. (2020) and Wang et al. (2022) only analyzed four representative cities of the East China region instead of showing the analysis at a national scale. Further, Zhao et al. (2020) did not update the anthropogenic emissions during the lockdown period, which brings significant uncertainties into their analysis. Previous studies found that the TROPOMI NO2 product has a negative bias of −7 % to −20 % (Verhoelst et al., 2021; Judd et al., 2020; Li et al., 2021). The sensitivity simulations indicate that this low bias does not significantly affect the model evaluation and our main conclusions (figures not shown).
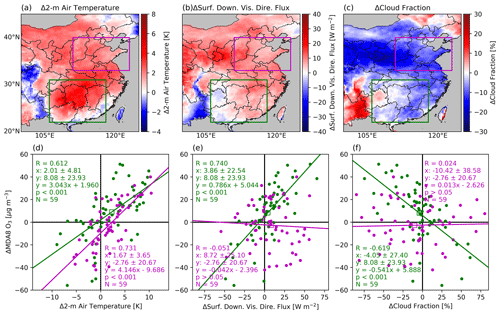
Figure 10The changes in (a) the 2 m air temperature, (b) the downward visible direct flux at the surface and (c) the cloud fraction from February–March 2019 to February–March 2020. Scatterplots between the daily difference in surface O3 measurements and (d) the 2 m air temperature, (e) the downward visible direct flux at the surface and (f) the cloud fraction in February–March between 2020 and 2019 over South China (green dots) and North China (pink dots). The green and pink open squares in (d–f) mark the predicted average change in surface MDA8 O3 in South China and North China, respectively, based on the linear regression against the change in meteorological variables.
3.5 Critical meteorological variables causing aggravated surface O3 pollution in South China
To identify the critical meteorological variables that led to the aggravated surface O3 pollution in South China, we investigated the correlations between the surface O3 concentrations and 2 m air temperature, downward visible direct flux at the surface, cloud fraction, relative humidity, and wind speed. The positive correlation between the surface O3 and temperature is widely observed and reported in the literature (Pusede et al., 2015). A higher temperature leads to higher concentrations of surface O3 because it improves the O3 production rate by affecting the organic reactivity, production of HOx radicals, and the formation and decomposition of peroxy nitrates and alkyl nitrates (Pusede et al., 2015). We calculated the daily difference in February–March between 2020 and 2019 (excluding 29 February 2020) for the daily mean of MDA8 O3 from ground measurements and 2 m air temperature and the downward visible direct flux at the surface and cloud fraction from GEOS-FP data used in our GEOS-Chem simulations for South China (22–31° N, 106–118° E; green box in Fig. 10a–c) and North China (33–40° N, 111–123° E; pink box in Fig. 10a–c). Figure 10 displays the difference in the 2-month mean 2 m air temperature (Fig. 10a), downward visible direct flux at the surface (Fig. 10b) and cloud fraction (Fig. 10c) in February–March between 2020 and 2019 as well as the scatterplot between the daily difference in measured surface O3 concentration and 2 m air temperature (Fig. 10d), downward visible direct flux at the surface (Fig. 10e) and cloud fraction (Fig. 10f) over both South China (green dots in Fig. 10d–f) and North China (pink dots in Fig. 10d–f). We found that the 2 m air temperature increased by ∼ 2.3 °C in South China and that the daily differences in surface O3 concentration and 2 m air temperature are well correlated, with a positive correlation coefficient of 0.612. Therefore, the temperature increase contributed to the significant aggravated surface O3 pollution in South China. The enhanced solar radiation at the surface could also promote the production of O3 via photochemical reactions. The correlation coefficient between the daily difference in surface O3 concentration and downward visible direct flux at the surface is as high as 0.740 in South China (Fig. 10e). The reason for the increase in temperature and solar radiation at the surface is the lower cloud fraction. By analyzing the GEOS-FP data, we found the cloud fraction decreased by ∼ 5 % (Fig. 10c), and the downward visible direct flux at the surface increased by 5 W m−2 (Fig. 10b) over South China. The lower cloud fraction increases the downward solar radiation at the surface during the lockdown period, leading to a higher surface air temperature. The change in cloud fraction is negatively correlated with the change in surface O3 in South China, with a correlation coefficient of −0.619 (Fig. 10f).
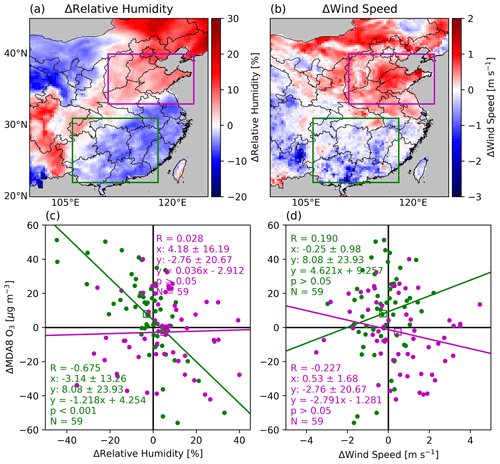
Figure 11Same as Fig. 10 but for (a, c) relative humidity and (b, d) wind speed.
In North China, the 2 m air temperature also increased by 1.8 ° C, but the measured surface MDA8 O3 decreased by 3 % (Fig. 8f). Figure 10d shows the daily difference in MDA8 O3, and the 2 m air temperature over North China also has a high correlation coefficient of 0.731. However, the intercept of the linear regression line is negative, indicating that surface O3 could decrease even though the temperature increases. This negative intercept is caused by the net effects of factors, other than temperature, including chemistry, emissions and other meteorological factors. It is a challenge to quantify the contributions of each individual factor because these factors are thermodynamically or dynamically related. The predicted average changes in surface MDA8 O3 in South China and North China are marked by the open green and pink squares, respectively, in Fig. 10d based on the linear regression. Because of the different intercepts, the predicted MDA8 O3 in South China increases by ∼ 9.0 µg m−3, while it decreases by 2.2 µg m−3 in North China, although the average temperature increased in both South and North China. Contrary to South China, the change in solar radiation at the surface and cloud fractions is poorly correlated with the change in surface O3 concentrations in North China (Fig. 10e and f), mainly due to a more important role of emission change in regulating the surface O3 in North China.
The impacts of relative humidity and wind speed on the surface O3 change are also investigated (Fig. 11). The relative humidity increased, on average, by ∼ 5.1 % in North China and decreased by ∼ 3.0 % in South China. The strong correlation (R = −0.675) between the change in relative humidity and surface O3 in South China indicates that the decrease in relative humidity also contributes to the increase in surface O3 pollution in South China, but the correlation between them in North China is very low. The wind speed also changed in opposite directions in South China and North China, but we cannot identify any significant impact of wind speed on the surface O3 pollution since the correlation coefficients are low in both South China and North China. In summary, the significant increase in surface O3 pollution during the lockdown period in South China could be primarily attributed to the higher temperature, enhanced solar radiation at the surface and decreased relative humidity.
A significant reduction in primary air pollutants has been identified by surface and satellite observations during the COVID-19 pandemic in China (Bauwens et al., 2020; Miyazaki et al., 2020), which is in contrast to the increase in surface O3. In this study, we analyzed the reasons for the enhanced surface O3 pollution from two perspectives: anthropogenic emissions reduction and inter-annual meteorological variations. We constrain the NOx emissions based on the TROPOMI NO2 product using both the mass balance and 4D-Var methods. The VOC emissions were also updated based on the TROPOMI HCHO product via the mass balance approach. We analyzed the contributions from emissions reduction and meteorological variations to surface O3 increases through a series of sensitivity simulations using the GEOS-Chem model.
The updated NOx emissions from the 4D-Var and mass balance approaches share a similar spatial pattern. However, the NOx emissions from 4D-Var are lower than those from the mass balance method over North China by ∼ 10 % but larger over central China by ∼ 40 %. The evaluation of the simulations with the updated emissions against the TROPOMI NO2, in situ measurements of surface NO2 and O3 indicates that the NOx emissions from the 4D-Var inversion leads to better model performance than that from the mass balance approach. The updated anthropogenic VOC emissions using the mass balance method are evaluated by comparing the GEOS-Chem HCHO and TROPOMI HCHO. However, the updated VOC emissions may still suffer from large uncertainties because of the low retrieval accuracy of TROPOMI HCHO (Vigouroux et al., 2020), large biogenic sources of VOC emissions and limited representativeness of HCHO for the whole VOC species.
The anthropogenic NOx emission decreased by ∼ 30 % over East China during February–March 2020 compared to the same period in 2019. Over North China, NOx emission reduction leads to a ∼ 8 % increase in the mean MDA8 surface O3, while the VOC emissions decline causes O3 to decrease by ∼ 3 %. The average contribution of meteorological variations to the surface O3 change is less than 1 % in North China. However, in South China, the inter-annual meteorological variation dominates the surface O3 increase, causing a ∼ 30 % increase, while the NOx and VOC emission reduction has nearly no impact on O3. Overall, the impact of inter-annual meteorological variations between 2019 and 2020 is almost 30 times larger than the impact of emissions on the enhanced surface O3 pollution in China.
The significant increase in surface O3 in South China could be attributed to the higher temperature, enhanced solar radiation at the surface and decreased relative humidity during the lockdown period. The lower cloud fraction increases the downward solar radiation at the surface during the lockdown period, leading to a higher surface air temperature. We cannot identify any significant impact of wind speed on the surface O3 pollution.
The default anthropogenic NOx emission over East Asia in GEOS-Chem is MIX 2010 (Li et al., 2017). To generate the anthropogenic NOx emission in 2019, we calculated the ratio of mean TROPOMI tropospheric NO2 VCD in February–March 2019 to GEOS-Chem simulated NO2 VCD with the default MIX 2010 emission as the scaling factor (Fig. A1). The scaling factors in regions where mean TROPOMI tropospheric NO2 VCD in February–March 2019 is less than 0.1 DU are set to 1. From 2010 to 2019, the anthropogenic NOx emission has declined significantly as a result of the clean air actions of the Chinese government (Zheng et al., 2018).
To optimize the NOx emissions and minimize the cost function (Eq. 1) with the 4D-Var method, the GEOS-Chem adjoint needs to compute the derivative of the cost function with respect to the model parameters to be optimized, which are the scaling factors of the anthropogenic NOx emissions in this study. An essential step is to calculate the adjoint forcing F, which is the derivative of the cost function with respect to the modeled NO2 concentration shown as Eq. (B1):
For each single TROPOMI NO2 observation, the adjoint forcing component f and cost function component j are computed as Eqs. (B2) and (B3):
Here, Mgc is the GEOS-Chem air mass factor applying the GEOS-Chem NO2 vertical profiles and TROPOMI NO2 averaging kernel. Mobs is the TROPOMI air mass factor. vgc and vobs are the tropospheric NO2 VCD from the GEOS-Chem model and TROPOMI observation, respectively. The product of the air mass factor and NO2 VCD is the NO2 slant column density. eobs is the standard error in the TROPOMI tropospheric NO2 VCD.
We calculated the GEOS-Chem air mass factor Mgc as Eq. (B4) following Qu et al. (2019).
Here, is the GEOS-Chem NO2 mixing ratio at the vertical layer i and is the pressure difference between the GEOS-Chem vertical layer i and i+1. is the scattering weight at the GEOS-Chem vertical layer i, which is calculated by the linear interpolation of the scattering weights at the vertical coordinate of the model TM5 used for the TROPOMI NO2 retrieval. The scattering weight at the TM5 vertical layer l () is computed as the product of the TROPOMI air mass factor and the TROPOMI averaging kernel at the TM5 vertical layer l () using Eqs. (B5) and (B6) (Eskes and Boersma, 2003; Palmer et al., 2001):
where Mgeo is the geometric air mass factor and θ0 and θ are the solar zenith angle and viewing zenith angle, respectively.
We validated the observation operator by comparing the sensitivity of the cost function with respect to the emission scaling factor from GEOS-Chem adjoint and a finite difference estimation as shown in Eq. (C1). We shut down the transport and excluded a priori the term from the cost function for the validation so that the gradient of the cost function component in each grid cell to the local emission scaling factor equals the gradient of total cost function to the emission scaling factor in the same grid cell.
Figure C1 compares the cost function sensitivities calculated from the GEOS-Chem adjoint and the finite difference method for the nested grids with the spatial resolution of 0.25° × 0.3125°. The spatial pattern and the magnitude of the cost function sensitivities from the two methods match with each other, with a correlation coefficient of 0.97. The statistics show that the agreement between the adjoint sensitivities and finite difference sensitivities in this study is comparable to that in Wang et al. (2020a), although we constrain the NOx emission at a much finer resolution of 0.25° × 0.3125° than in their study (2° × 2.5°).
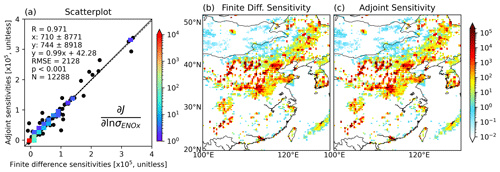
Figure C1Comparison of adjoint sensitivities and finite difference sensitivities. (a) Scatterplot of the adjoint sensitivity of the cost function with respect to the logarithm of the NOx emission scaling factor versus the finite difference sensitivities. The color scheme for panel (a) encodes the number of samples (the legend on the right of panel (a)). (b) Map of finite difference sensitivity. (c) Map of adjoint sensitivity.
It is not practical to validate the VOC emissions species by species in this study. We compare the GEOS-Chem simulation of HCHO with TROPOMI data for a rough validation of the VOC emissions. Overall, the GEOS-Chem Baseline (2019) and 2020 Adjoint simulations agree well with TROPOMI HCHO in February–March of 2019 and 2020, respectively (Fig. D1). The correlation coefficients for the two comparisons are 0.878 and 0.874, and the MBEs are −0.036 and −0.042 DU, respectively. This good agreement also supports our assumption of ignoring the change in VOC emissions from 2010 to 2019, since the anthropogenic VOC emission used for Baseline (2019) is equivalent to MIX 2010. However, Fig. D1 shows that GEOS-Chem tends to overestimate the HCHO VCD over urban regions and underestimate it over rural regions, which indicates the partition of total emissions may overestimate the anthropogenic emissions and underestimate the biogenic emissions, at least for HCHO. A more comprehensive evaluation for various species and emission sectors would be helpful for further improvement of the VOC emissions used in the model.
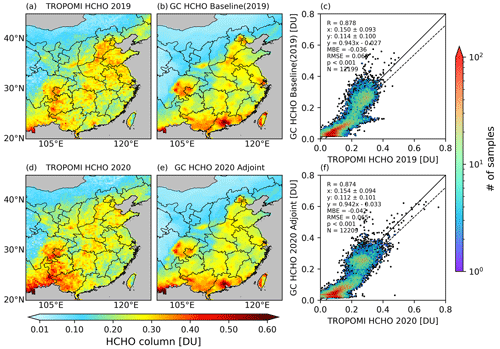
Figure D1Comparison of tropospheric HCHO VCD from TROPOMI and GEOS-Chem. (a) TROPOMI HCHO in February–March 2019. (b) GEOS-Chem HCHO from Baseline (2019). (c) Scatterplot between panel (a) and panel (b). (d) TROPOMI HCHO in February–March 2020. (e) GEOS-Chem HCHO from 2020 Adjoint. (f) Scatterplot between panel (d) and panel (e). The emissions and meteorology configurations for the GEOS-Chem Baseline (2019) and 2020 Adjoint simulations are listed in Table 1.
The TROPOMI NO2 and HCHO product is available at the NASA Goddard Earth Sciences Data and Information Services Center (for NO2: https://daac.gsfc.nasa.gov/datasets/S5P_L2__NO2____HiR_1/summary, KNMI, 2019a and for HCHO: https://daac.gsfc.nasa.gov/datasets/S5P_L2__HCHO___HiR_1/summary, KNMI, 2019b). The ground O3 and NO2 measurements are available at the China National Environmental Monitoring Centre (http://www.cnemc.cn/en/, CNEMC, 2014).
ZL and JW designed the research, and ZL conducted the research. YW, DKH and XC contributed to the research design. ZL and JW wrote the manuscript, and XC and DKH contributed to the writing. YW and TS developed the codes for comparing the tropospheric NO2 VCD from the model and TROPOMI data. KS developed the codes for oversampling.
The contact author has declared that none of the authors has any competing interests.
Publisher's note: Copernicus Publications remains neutral with regard to jurisdictional claims made in the text, published maps, institutional affiliations, or any other geographical representation in this paper. While Copernicus Publications makes every effort to include appropriate place names, the final responsibility lies with the authors.
This research is supported by the NASA ACMAP (grant no. 80NSSC19K0950). We acknowledge the computational support from the High Performance Computing group at the University of Iowa.
This research has been supported by the National Aeronautics and Space Administration (grant no. 80NSSC19K0950).
This paper was edited by Hang Su and reviewed by two anonymous referees.
Atkinson, R.: Atmospheric chemistry of VOCs and NOx, Atmos. Environ., 34, 2063–2101, https://doi.org/10.1016/s1352-2310(99)00460-4, 2000.
Bauwens, M., Compernolle, S., Stavrakou, T., Muller, J. F., van Gent, J., Eskes, H., Levelt, P. F., van der A, R., Veefkind, J. P., Vlietinck, J., Yu, H., and Zehner, C.: Impact of Coronavirus Outbreak on NO2 Pollution Assessed Using TROPOMI and OMI Observations, Geophys. Res. Lett., 47, e2020GL087978, https://doi.org/10.1029/2020GL087978, 2020.
Bey, I., Jacob, D. J., Yantosca, R. M., Logan, J. A., Field, B. D., Fiore, A. M., Li, Q., Liu, H. Y., Mickley, L. J., and Schultz, M. G.: Global modeling of tropospheric chemistry with assimilated meteorology: Model description and evaluation, J. Geophys. Res.-Atmos., 106, 23073–23095, https://doi.org/10.1029/2001jd000807, 2001.
Bi, Z., Ye, Z., He, C., and Li, Y.: Analysis of the meteorological factors affecting the short-term increase in O3 concentrations in nine global cities during COVID-19, Atmos. Pollut. Res., 13, 101523, https://doi.org/10.1016/j.apr.2022.101523, 2022.
Chen, D., Wang, Y., McElroy, M. B., He, K., Yantosca, R. M., and Le Sager, P.: Regional CO pollution and export in China simulated by the high-resolution nested-grid GEOS-Chem model, Atmos. Chem. Phys., 9, 3825–3839, https://doi.org/10.5194/acp-9-3825-2009, 2009.
CNEMC – China National Environmental Monitoring Centre: In situ measurements of surface NO2 and O3 over China, https://www.cnemc.cn/en/ (last access: 5 September 2023), 2014.
Cooper, M., Martin, R. V., Padmanabhan, A., and Henze, D. K.: Comparing mass balance and adjoint methods for inverse modeling of nitrogen dioxide columns for global nitrogen oxide emissions, J. Geophys. Res.-Atmos., 122, 4718–4734, https://doi.org/10.1002/2016jd025985, 2017.
De Smedt, I., Theys, N., Yu, H., Danckaert, T., Lerot, C., Compernolle, S., Van Roozendael, M., Richter, A., Hilboll, A., Peters, E., Pedergnana, M., Loyola, D., Beirle, S., Wagner, T., Eskes, H., van Geffen, J., Boersma, K. F., and Veefkind, P.: Algorithm theoretical baseline for formaldehyde retrievals from S5P TROPOMI and from the QA4ECV project, Atmos. Meas. Tech., 11, 2395–2426, https://doi.org/10.5194/amt-11-2395-2018, 2018.
Eskes, H. J. and Boersma, K. F.: Averaging kernels for DOAS total-column satellite retrievals, Atmos. Chem. Phys., 3, 1285–1291, https://doi.org/10.5194/acp-3-1285-2003, 2003.
Ghahremanloo, M., Lops, Y., Choi, Y., and Mousavinezhad, S.: Impact of the COVID-19 outbreak on air pollution levels in East Asia, Sci. Total Environ., 754, 142226, https://doi.org/10.1016/j.scitotenv.2020.142226, 2021.
Gong, C., Lei, Y., Ma, Y., Yue, X., and Liao, H.: Ozone–vegetation feedback through dry deposition and isoprene emissions in a global chemistry–carbon–climate model, Atmos. Chem. Phys., 20, 3841–3857, https://doi.org/10.5194/acp-20-3841-2020, 2020.
Guenther, A. B., Jiang, X., Heald, C. L., Sakulyanontvittaya, T., Duhl, T., Emmons, L. K., and Wang, X.: The Model of Emissions of Gases and Aerosols from Nature version 2.1 (MEGAN2.1): an extended and updated framework for modeling biogenic emissions, Geosci. Model Dev., 5, 1471–1492, https://doi.org/10.5194/gmd-5-1471-2012, 2012.
Guo, J., Zhang, X. S., Gao, Y., Wang, Z. W., Zhang, M. G., Xue, W. B., Herrmann, H., Brasseur, G. P., Wang, T., and Wang, Z.: Evolution of Ozone Pollution in China: What Track Will It Follow?, Environ. Sci. Technol., 57, 109–117, https://doi.org/10.1021/acs.est.2c08205, 2023.
Henze, D. K., Hakami, A., and Seinfeld, J. H.: Development of the adjoint of GEOS-Chem, Atmos. Chem. Phys., 7, 2413–2433, https://doi.org/10.5194/acp-7-2413-2007, 2007.
Henze, D. K., Seinfeld, J. H., and Shindell, D. T.: Inverse modeling and mapping US air quality influences of inorganic PM2.5 precursor emissions using the adjoint of GEOS-Chem, Atmos. Chem. Phys., 9, 5877–5903, https://doi.org/10.5194/acp-9-5877-2009, 2009.
Hoesly, R. M., Smith, S. J., Feng, L., Klimont, Z., Janssens-Maenhout, G., Pitkanen, T., Seibert, J. J., Vu, L., Andres, R. J., Bolt, R. M., Bond, T. C., Dawidowski, L., Kholod, N., Kurokawa, J.-I., Li, M., Liu, L., Lu, Z., Moura, M. C. P., O'Rourke, P. R., and Zhang, Q.: Historical (1750–2014) anthropogenic emissions of reactive gases and aerosols from the Community Emissions Data System (CEDS), Geosci. Model Dev., 11, 369–408, https://doi.org/10.5194/gmd-11-369-2018, 2018.
Huang, X., Ding, A., Gao, J., Zheng, B., Zhou, D., Qi, X., Tang, R., Wang, J., Ren, C., Nie, W., Chi, X., Xu, Z., Chen, L., Li, Y., Che, F., Pang, N., Wang, H., Tong, D., Qin, W., Cheng, W., Liu, W., Fu, Q., Liu, B., Chai, F., Davis, S. J., Zhang, Q., and He, K.: Enhanced secondary pollution offset reduction of primary emissions during COVID-19 lockdown in China, Natl. Sci. Rev., 8, nwaa137, https://doi.org/10.1093/nsr/nwaa137, 2020.
Hudman, R. C., Moore, N. E., Mebust, A. K., Martin, R. V., Russell, A. R., Valin, L. C., and Cohen, R. C.: Steps towards a mechanistic model of global soil nitric oxide emissions: implementation and space based-constraints, Atmospheric Chemistry and Physics, 12, 7779–7795, https://doi.org/10.5194/acp-12-7779-2012, 2012.
Janssens-Maenhout, G., Crippa, M., Guizzardi, D., Dentener, F., Muntean, M., Pouliot, G., Keating, T., Zhang, Q., Kurokawa, J., Wankmüller, R., Denier van der Gon, H., Kuenen, J. J. P., Klimont, Z., Frost, G., Darras, S., Koffi, B., and Li, M.: HTAP_v2.2: a mosaic of regional and global emission grid maps for 2008 and 2010 to study hemispheric transport of air pollution, Atmos. Chem. Phys., 15, 11411–11432, https://doi.org/10.5194/acp-15-11411-2015, 2015.
Jerrett, M., Burnett, R. T., Pope, C. A., Ito, K., Thurston, G., Krewski, D., Shi, Y. L., Calle, E., and Thun, M.: Long-Term Ozone Exposure and Mortality, New Engl. J. Med., 360, 1085–1095, https://doi.org/10.1056/NEJMoa0803894, 2009.
Judd, L. M., Al-Saadi, J. A., Szykman, J. J., Valin, L. C., Janz, S. J., Kowalewski, M. G., Eskes, H. J., Veefkind, J. P., Cede, A., Mueller, M., Gebetsberger, M., Swap, R., Pierce, R. B., Nowlan, C. R., Abad, G. G., Nehrir, A., and Williams, D.: Evaluating Sentinel-5P TROPOMI tropospheric NO2 column densities with airborne and Pandora spectrometers near New York City and Long Island Sound, Atmos. Meas. Tech., 13, 6113–6140, https://doi.org/10.5194/amt-13-6113-2020, 2020.
KNMI – Koninklijk Nederlands Meteorologisch Instituut: Sentinel-5P TROPOMI Tropospheric NO2 1-Orbit L2 5.5 km × 3.5 km, GES DISC – Goddard Earth Sciences Data and Information Services Center [data set], https://daac.gsfc.nasa.gov/datasets/S5P_L2__NO2____HiR_1/summary (last access: 16 August 2023), 2019a.
KNMI – Koninklijk Nederlands Meteorologisch Instituut: Sentinel-5P TROPOMI Tropospheric Formaldehyde 1-Orbit L2 5.5 km × 3.5 km, GES DISC – Goddard Earth Sciences Data and In- formation Services Center [data set], https://daac.gsfc.nasa.gov/datasets/S5P_L2__HCHO___HiR_1/summary (last access: 16 August 2023), 2019b.
Le, T. H., Wang, Y., Liu, L., Yang, J. N., Yung, Y. L., Li, G. H., and Seinfeld, J. H.: Unexpected air pollution with marked emission reductions during the COVID-19 outbreak in China, Science, 369, 702–706, https://doi.org/10.1126/science.abb7431, 2020.
Leue, C., Wenig, M., Wagner, T., Klimm, O., Platt, U., and Jähne, B.: Quantitative analysis of NOx emissions from Global Ozone Monitoring Experiment satellite image sequences, J. Geophys. Res.-Atmos., 106, 5493–5505, https://doi.org/10.1029/2000jd900572, 2001.
Levelt, P. F., Stein Zweers, D. C., Aben, I., Bauwens, M., Borsdorff, T., De Smedt, I., Eskes, H. J., Lerot, C., Loyola, D. G., Romahn, F., Stavrakou, T., Theys, N., Van Roozendael, M., Veefkind, J. P., and Verhoelst, T.: Air quality impacts of COVID-19 lockdown measures detected from space using high spatial resolution observations of multiple trace gases from Sentinel-5P/TROPOMI, Atmos. Chem. Phys., 22, 10319–10351, https://doi.org/10.5194/acp-22-10319-2022, 2022.
Li, M., Zhang, Q., Kurokawa, J.-I., Woo, J.-H., He, K., Lu, Z., Ohara, T., Song, Y., Streets, D. G., Carmichael, G. R., Cheng, Y., Hong, C., Huo, H., Jiang, X., Kang, S., Liu, F., Su, H., and Zheng, B.: MIX: a mosaic Asian anthropogenic emission inventory under the international collaboration framework of the MICS-Asia and HTAP, Atmos. Chem. Phys., 17, 935–963, https://doi.org/10.5194/acp-17-935-2017, 2017.
Li, M., McDonald, B. C., McKeen, S. A., Eskes, H., Levelt, P., Francoeur, C., Harkins, C., He, J., Barth, M., Henze, D. K., Bela, M. M., Trainer, M., de Gouw, J. A., and Frost, G. J.: Assessment of Updated Fuel-Based Emissions Inventories Over the Contiguous United States Using TROPOMI NO2 Retrievals, J. Geophys. Res.-Atmos., 126, e2021JD035484, https://doi.org/10.1029/2021jd035484, 2021.
Liu, F., Page, A., Strode, S. A., Yoshida, Y., Choi, S., Zheng, B., Lamsal, L. N., Li, C., Krotkov, N. A., Eskes, H., van der A, R., Veefkind, P., Levelt, P. F., Hauser, O. P., and Joiner, J.: Abrupt decline in tropospheric nitrogen dioxide over China after the outbreak of COVID-19, Science Advances, 6, eabc2992, https://doi.org/10.1126/sciadv.abc2992, 2020.
Liu, S. C., Trainer, M., Fehsenfeld, F. C., Parrish, D. D., Williams, E. J., Fahey, D. W., Hubler, G., and Murphy, P. C.: Ozone production in the rural troposphere and the implications for regional and global ozone distributions, J. Geophys. Res.-Atmos., 92, 4191–4207, https://doi.org/10.1029/JD092iD04p04191, 1987.
Liu, T., Wang, X., Hu, J., Wang, Q., An, J., Gong, K., Sun, J., Li, L., Qin, M., Li, J., Tian, J., Huang, Y., Liao, H., Zhou, M., Hu, Q., Yan, R., Wang, H., and Huang, C.: Driving Forces of Changes in Air Quality during the COVID-19 Lockdown Period in the Yangtze River Delta Region, China, Environ. Sci. Tech. Let., 7, 779–786, https://doi.org/10.1021/acs.estlett.0c00511, 2020.
Liu, Y. M., Wang, T., Stavrakou, T., Elguindi, N., Doumbia, T., Granier, C., Bouarar, I., Gaubert, B., and Brasseur, G. P.: Diverse response of surface ozone to COVID-19 lockdown in China, Sci. Total Environ., 789, 147739, https://doi.org/10.1016/j.scitotenv.2021.147739, 2021.
Lu, X., Zhang, L., Chen, Y., Zhou, M., Zheng, B., Li, K., Liu, Y., Lin, J., Fu, T.-M., and Zhang, Q.: Exploring 2016–2017 surface ozone pollution over China: source contributions and meteorological influences, Atmos. Chem. Phys., 19, 8339–8361, https://doi.org/10.5194/acp-19-8339-2019, 2019.
Mao, J., Jacob, D. J., Evans, M. J., Olson, J. R., Ren, X., Brune, W. H., Clair, J. M. St., Crounse, J. D., Spencer, K. M., Beaver, M. R., Wennberg, P. O., Cubison, M. J., Jimenez, J. L., Fried, A., Weibring, P., Walega, J. G., Hall, S. R., Weinheimer, A. J., Cohen, R. C., Chen, G., Crawford, J. H., McNaughton, C., Clarke, A. D., Jaeglé, L., Fisher, J. A., Yantosca, R. M., Le Sager, P., and Carouge, C.: Chemistry of hydrogen oxide radicals (HOx) in the Arctic troposphere in spring, Atmos. Chem. Phys., 10, 5823–5838, https://doi.org/10.5194/acp-10-5823-2010, 2010.
Mao, J., Paulot, F., Jacob, D. J., Cohen, R. C., Crounse, J. D., Wennberg, P. O., Keller, C. A., Hudman, R. C., Barkley, M. P., and Horowitz, L. W.: Ozone and organic nitrates over the eastern United States: Sensitivity to isoprene chemistry, J. Geophys. Res.-Atmos., 118, 11256–11268, https://doi.org/10.1002/jgrd.50817, 2013.
Martin, R. V., Jacob, D. J., Chance, K., Kurosu, T. P., Palmer, P. I., and Evans, M. J.: Global inventory of nitrogen oxide emissions constrained by space-based observations of NO2 columns, J. Geophys. Res.-Atmos., 108, 4537, https://doi.org/10.1029/2003jd003453, 2003.
Miyazaki, K., Bowman, K., Sekiya, T., Jiang, Z., Chen, X., Eskes, H., Ru, M., Zhang, Y., and Shindell, D.: Air Quality Response in China Linked to the 2019 Novel Coronavirus (COVID-19) Lockdown, Geophys. Res. Lett., 47, e2020GL089252, https://doi.org/10.1029/2020gl089252, 2020.
Murray, L. T., Jacob, D. J., Logan, J. A., Hudman, R. C., and Koshak, W. J.: Optimized regional and interannual variability of lightning in a global chemical transport model constrained by LIS/OTD satellite data, J. Geophys. Res.-Atmos., 117, D20307, https://doi.org/10.1029/2012jd017934, 2012.
Ott, L. E., Pickering, K. E., Stenchikov, G. L., Allen, D. J., DeCaria, A. J., Ridley, B., Lin, R.-F., Lang, S., and Tao, W.-K.: Production of lightning NOx and its vertical distribution calculated from three-dimensional cloud-scale chemical transport model simulations, J. Geophys. Res., 115, D04301, https://doi.org/10.1029/2009jd011880, 2010.
Palmer, P. I., Jacob, D. J., Chance, K., Martin, R. V., Spurr, R. J. D., Kurosu, T. P., Bey, I., Yantosca, R., Fiore, A., and Li, Q. B.: Air mass factor formulation for spectroscopic measurements from satellites: Application to formaldehyde retrievals from the Global Ozone Monitoring Experiment, J. Geophys. Res.-Atmos., 106, 14539–14550, https://doi.org/10.1029/2000jd900772, 2001.
Pusede, S. E., Steiner, A. L., and Cohen, R. C.: Temperature and Recent Trends in the Chemistry of Continental Surface Ozone, Chem. Rev., 115, 3898–3918, https://doi.org/10.1021/cr5006815, 2015.
Qu, Z., Henze, D. K., Capps, S. L., Wang, Y., Xu, X., Wang, J., and Keller, M.: Monthly top-down NOx emissions for China (2005–2012): A hybrid inversion method and trend analysis, J. Geophys. Res.-Atmos., 122, 4600–4625, https://doi.org/10.1002/2016JD025852, 2017.
Qu, Z., Henze, D. K., Li, C., Theys, N., Wang, Y., Wang, J., Wang, W., Han, J., Shim, C., Dickerson, R. R., and Ren, X.: SO2 Emission Estimates Using OMI SO2 Retrievals for 2005–2017, J. Geophys. Res.-Atmos., 124, 8336–8359, https://doi.org/10.1029/2019JD030243, 2019.
Sha, T., Ma, X. Y., Zhang, H. X., Janechek, N., Wang, Y. Y., Wang, Y., Garcia, L. C., Jenerette, G. D., and Wang, J.: Impacts of Soil NOx Emission on O3 Air Quality in Rural California, Environ. Sci. Technol., 55, 7113–7122, https://doi.org/10.1021/acs.est.0c06834, 2021.
Shi, X. and Brasseur, G. P.: The Response in Air Quality to the Reduction of Chinese Economic Activities During the COVID-19 Outbreak, Geophys. Res. Lett., 47, e2020GL088070, https://doi.org/10.1029/2020gl088070, 2020.
Sillman, S., Logan, J. A., and Wofsy, S. C.: The sensitivity of ozone to nitrogen-oxides and hydrocarbons in regional ozone episodes, J. Geophys. Res.-Atmos., 95, 1837–1851, https://doi.org/10.1029/JD095iD02p01837, 1990.
Steinbacher, M., Zellweger, C., Schwarzenbach, B., Bugmann, S., Buchmann, B., Ordóñez, C., Prevot, A. S. H., and Hueglin, C.: Nitrogen oxide measurements at rural sites in Switzerland: Bias of conventional measurement techniques, J. Geophys. Res.-Atmos., 112, D11307, https://doi.org/10.1029/2006JD007971, 2007.
Streets, D. G., Canty, T., Carmichael, G. R., de Foy, B., Dickerson, R. R., Duncan, B. N., Edwards, D. P., Haynes, J. A., Henze, D. K., Houyoux, M. R., Jacob, D. J., Krotkov, N. A., Lamsal, L. N., Liu, Y., Lu, Z., Martin, R. V., Pfister, G. G., Pinder, R. W., Salawitch, R. J., and Wecht, K. J.: Emissions estimation from satellite retrievals: A review of current capability, Atmos. Environ., 77, 1011–1042, https://doi.org/10.1016/j.atmosenv.2013.05.051, 2013.
Sun, K., Zhu, L., Cady-Pereira, K., Chan Miller, C., Chance, K., Clarisse, L., Coheur, P.-F., González Abad, G., Huang, G., Liu, X., Van Damme, M., Yang, K., and Zondlo, M.: A physics-based approach to oversample multi-satellite, multispecies observations to a common grid, Atmos. Meas. Tech., 11, 6679–6701, https://doi.org/10.5194/amt-11-6679-2018, 2018.
Tong, L., Liu, Y., Meng, Y., Dai, X. R., Huang, L. J., Luo, W. X., Yang, M. R., Pan, Y., Zheng, J., and Xiao, H.: Surface ozone changes during the COVID-19 outbreak in China: An insight into the pollution characteristics and formation regimes of ozone in the cold season, J. Atmos. Chem., 80, 103–120, https://doi.org/10.1007/s10874-022-09443-2, 2023.
Travis, K. R., Jacob, D. J., Fisher, J. A., Kim, P. S., Marais, E. A., Zhu, L., Yu, K., Miller, C. C., Yantosca, R. M., Sulprizio, M. P., Thompson, A. M., Wennberg, P. O., Crounse, J. D., St. Clair, J. M., Cohen, R. C., Laughner, J. L., Dibb, J. E., Hall, S. R., Ullmann, K., Wolfe, G. M., Pollack, I. B., Peischl, J., Neuman, J. A., and Zhou, X.: Why do models overestimate surface ozone in the Southeast United States?, Atmos. Chem. Phys., 16, 13561–13577, https://doi.org/10.5194/acp-16-13561-2016, 2016.
Travis, K. R., Jacob, D. J., Keller, C. A., Kuang, S., Lin, J., Newchurch, M. J., and Thompson, A. M.: Resolving ozone vertical gradients in air quality models, Atmos. Chem. Phys. Discuss. [preprint], https://doi.org/10.5194/acp-2017-596, 2017.
van der Werf, G. R., Randerson, J. T., Giglio, L., van Leeuwen, T. T., Chen, Y., Rogers, B. M., Mu, M., van Marle, M. J. E., Morton, D. C., Collatz, G. J., Yokelson, R. J., and Kasibhatla, P. S.: Global fire emissions estimates during 1997–2016, Earth Syst. Sci. Data, 9, 697–720, https://doi.org/10.5194/essd-9-697-2017, 2017.
van Geffen, J., Boersma, K. F., Eskes, H., Sneep, M., ter Linden, M., Zara, M., and Veefkind, J. P.: S5P TROPOMI NO2 slant column retrieval: method, stability, uncertainties and comparisons with OMI, Atmos. Meas. Tech., 13, 1315–1335, https://doi.org/10.5194/amt-13-1315-2020, 2020.
van Geffen, J., Eskes, H., Boersma, K. F., and Veefkind, J. P.: TROPOMI ATBD of the total and tropospheric NO2 data products, https://sentinel.esa.int/documents/247904/2476257/sentinel-5p-tropomi-atbd-no2-data-products (last access: 29 September 2023), 2022.
Veefkind, J. P., Aben, I., McMullan, K., Förster, H., de Vries, J., Otter, G., Claas, J., Eskes, H. J., de Haan, J. F., Kleipool, Q., van Weele, M., Hasekamp, O., Hoogeveen, R., Landgraf, J., Snel, R., Tol, P., Ingmann, P., Voors, R., Kruizinga, B., Vink, R., Visser, H., and Levelt, P. F.: TROPOMI on the ESA Sentinel-5 Precursor: A GMES mission for global observations of the atmospheric composition for climate, air quality and ozone layer applications, Remote Sens. Environ., 120, 70–83, https://doi.org/10.1016/j.rse.2011.09.027, 2012.
Venter, Z. S., Aunan, K., Chowdhury, S., and Lelieveld, J.: COVID-19 lockdowns cause global air pollution declines, P. Natl. Acad. Sci. USA, 117, 18984–18990, https://doi.org/10.1073/pnas.2006853117, 2020.
Verhoelst, T., Compernolle, S., Pinardi, G., Lambert, J.-C., Eskes, H. J., Eichmann, K.-U., Fjæraa, A. M., Granville, J., Niemeijer, S., Cede, A., Tiefengraber, M., Hendrick, F., Pazmiño, A., Bais, A., Bazureau, A., Boersma, K. F., Bognar, K., Dehn, A., Donner, S., Elokhov, A., Gebetsberger, M., Goutail, F., Grutter de la Mora, M., Gruzdev, A., Gratsea, M., Hansen, G. H., Irie, H., Jepsen, N., Kanaya, Y., Karagkiozidis, D., Kivi, R., Kreher, K., Levelt, P. F., Liu, C., Müller, M., Navarro Comas, M., Piters, A. J. M., Pommereau, J.-P., Portafaix, T., Prados-Roman, C., Puentedura, O., Querel, R., Remmers, J., Richter, A., Rimmer, J., Rivera Cárdenas, C., Saavedra de Miguel, L., Sinyakov, V. P., Stremme, W., Strong, K., Van Roozendael, M., Veefkind, J. P., Wagner, T., Wittrock, F., Yela González, M., and Zehner, C.: Ground-based validation of the Copernicus Sentinel-5P TROPOMI NO2 measurements with the NDACC ZSL-DOAS, MAX-DOAS and Pandonia global networks, Atmos. Meas. Tech., 14, 481–510, https://doi.org/10.5194/amt-14-481-2021, 2021.
Vigouroux, C., Langerock, B., Bauer Aquino, C. A., Blumenstock, T., Cheng, Z., De Mazière, M., De Smedt, I., Grutter, M., Hannigan, J. W., Jones, N., Kivi, R., Loyola, D., Lutsch, E., Mahieu, E., Makarova, M., Metzger, J.-M., Morino, I., Murata, I., Nagahama, T., Notholt, J., Ortega, I., Palm, M., Pinardi, G., Röhling, A., Smale, D., Stremme, W., Strong, K., Sussmann, R., Té, Y., van Roozendael, M., Wang, P., and Winkler, H.: TROPOMI–Sentinel-5 Precursor formaldehyde validation using an extensive network of ground-based Fourier-transform infrared stations, Atmos. Meas. Tech., 13, 3751–3767, https://doi.org/10.5194/amt-13-3751-2020, 2020.
Vinken, G. C. M., Boersma, K. F., van Donkelaar, A., and Zhang, L.: Constraints on ship NOx emissions in Europe using GEOS-Chem and OMI satellite NO2 observations, Atmos. Chem. Phys., 14, 1353–1369, https://doi.org/10.5194/acp-14-1353-2014, 2014.
Wang, H. L., Huang, C., Tao, W., Gao, Y. Q., Wang, S. W., Jing, S. A., Wang, W. J., Yan, R. S., Wang, Q., An, J. Y., Tian, J. J., Hu, Q. Y., Lou, S. R., Pöschl, U., Cheng, Y. F., and Su, H.: Seasonality and reduced nitric oxide titration dominated ozone increase during COVID-19 lockdown in eastern China, npj climate and atmospheric science, 5, 24, https://doi.org/10.1038/s41612-022-00249-3, 2022.
Wang, Y., Wang, J., Xu, X., Henze, D. K., Qu, Z., and Yang, K.: Inverse modeling of SO2 and NOx emissions over China using multisensor satellite data – Part 1: Formulation and sensitivity analysis, Atmos. Chem. Phys., 20, 6631–6650, https://doi.org/10.5194/acp-20-6631-2020, 2020a.
Wang, Y., Wang, J., Zhou, M., Henze, D. K., Ge, C., and Wang, W.: Inverse modeling of SO2 and NOx emissions over China using multisensor satellite data – Part 2: Downscaling techniques for air quality analysis and forecasts, Atmos. Chem. Phys., 20, 6651–6670, https://doi.org/10.5194/acp-20-6651-2020, 2020b.
Wang, Y. X., McElroy, M. B., Jacob, D. J., and Yantosca, R. M.: A nested grid formulation for chemical transport over Asia: Applications to CO, J. Geophys. Res.-Atmos., 109, D22307, https://doi.org/10.1029/2004jd005237, 2004.
Williams, J. and Koppmann, R.: Volatile Organic Compounds in the Atmosphere: An Overview, in: Volatile Organic Compounds in the Atmosphere, Wiley, 1–32, https://doi.org/10.1002/9780470988657.ch1, 2007.
Zhang, L., Jacob, D. J., Knipping, E. M., Kumar, N., Munger, J. W., Carouge, C. C., van Donkelaar, A., Wang, Y. X., and Chen, D.: Nitrogen deposition to the United States: distribution, sources, and processes, Atmos. Chem. Phys., 12, 4539–4554, https://doi.org/10.5194/acp-12-4539-2012, 2012.
Zhang, Q., Pan, Y., He, Y., Walters, W. W., Ni, Q., Liu, X., Xu, G., Shao, J., and Jiang, C.: Substantial nitrogen oxides emission reduction from China due to COVID-19 and its impact on surface ozone and aerosol pollution, Sci. Total Environ., 753, https://doi.org/10.1016/j.scitotenv.2020.142238, 2021.
Zhang, R. X., Zhang, Y. Z., Lin, H. P., Feng, X., Fu, T. M., and Wang, Y. H.: NOx Emission Reduction and Recovery during COVID-19 in East China, Atmosphere, 11, 433, https://doi.org/10.3390/atmos11040433, 2020.
Zhang, Y.-L. and Cao, F.: Fine particulate matter (PM2.5) in China at a city level, Sci. Rep.-UK, 5, 14884, https://doi.org/10.1038/srep14884, 2015.
Zhao, Y., Zhang, K., Xu, X., Shen, H., Zhu, X., Zhang, Y., Hu, Y., and Shen, G.: Substantial Changes in Nitrogen Dioxide and Ozone after Excluding Meteorological Impacts during the COVID-19 Outbreak in Mainland China, Environ. Sci. Tech. Let., 7, 402–408, https://doi.org/10.1021/acs.estlett.0c00304, 2020.
Zheng, B., Tong, D., Li, M., Liu, F., Hong, C., Geng, G., Li, H., Li, X., Peng, L., Qi, J., Yan, L., Zhang, Y., Zhao, H., Zheng, Y., He, K., and Zhang, Q.: Trends in China's anthropogenic emissions since 2010 as the consequence of clean air actions, Atmos. Chem. Phys., 18, 14095–14111, https://doi.org/10.5194/acp-18-14095-2018, 2018.
- Abstract
- Introduction
- Datasets and methods
- Results
- Summary
- Appendix A: NOx emission reduction in China from 2010 to 2019
- Appendix B: Applying the TROPOMI NO2 averaging kernel in the observation operator
- Appendix C: Validation of the TROPOMI NO2 observation operator
- Appendix D: Validation of HCHO simulations
- Data availability
- Author contributions
- Competing interests
- Disclaimer
- Acknowledgements
- Financial support
- Review statement
- References
- Abstract
- Introduction
- Datasets and methods
- Results
- Summary
- Appendix A: NOx emission reduction in China from 2010 to 2019
- Appendix B: Applying the TROPOMI NO2 averaging kernel in the observation operator
- Appendix C: Validation of the TROPOMI NO2 observation operator
- Appendix D: Validation of HCHO simulations
- Data availability
- Author contributions
- Competing interests
- Disclaimer
- Acknowledgements
- Financial support
- Review statement
- References