the Creative Commons Attribution 4.0 License.
the Creative Commons Attribution 4.0 License.
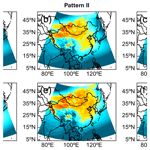
Impact of weather patterns and meteorological factors on PM2.5 and O3 responses to the COVID-19 lockdown in China
Michaela I. Hegglin
Yue Yuan
Haze events in the North China Plain (NCP) and a decline in ozone levels in Southern Coast China (SC) from 21 January to 9 February 2020 during the COVID-19 lockdown have attracted public curiosity and scholarly attention. Most previous studies focused on the impact of atmospheric chemistry processes associated with anomalous weather elements in these cases, but fewer studies quantified the impact of various weather elements within the context of a specific weather pattern. To identify the weather patterns responsible for inducing this unexpected situation and to further quantify the importance of different meteorological factors during the haze event, two approaches are employed. These approaches implemented the comparisons of observations in 2020 with climatology averaged over the years 2015–2019 with a novel structural SOM (self-organising map) model and with the prediction of the “business as usual” (hereafter referred to as BAU) emission strength by the GBM (gradient-boosting machine) model, respectively. The results reveal that the unexpected PM2.5 pollution and O3 decline from the climatology in NCP and SC could be effectively explained by the presence of a double-centre high-pressure system across China. Moreover, the GBM results provided a quantitative assessment of the importance of each meteorological factor in driving the predictions of PM2.5 and O3 under the specific weather system. These results indicate that temperature played the most crucial role in the haze event in NCP, as well as in the O3 change in SC. This valuable information will ultimately contribute to our ability to predict air pollution under future emission scenarios and changing weather patterns that may be influenced by climate change.
- Article
(8618 KB) - Full-text XML
-
Supplement
(12036 KB) - BibTeX
- EndNote
The coronavirus disease 2019 (COVID-19) pandemic has lasted for 4.5 years and has led to over 7 million deaths globally as of June 2023 (WHO, 2024). The Chinese government implemented strict lockdown measures nationwide during the first 2 months of 2020 to curb the spread of this pandemic (Le et al., 2020), which led to significant reductions in anthropogenic emissions, especially in the transportation sector (Xu et al., 2020; Wang et al., 2021; Liu et al., 2021). As a result, a decline not only in NO2 but also in PM2.5, PM10, SO2, and CO concentrations on a national scale was indicated by both satellite and ground-based measurements, although with the negative consequence of enhancements in O3 concentrations (Shen et al., 2022; Liu et al., 2021; He et al., 2020). Contrary to the situation in other regions from 21 January to 9 February 2020, Northern China (NC) and Southwestern China (SWC) experienced severe haze pollution and decreased O3 situations, respectively (Le et al., 2020; Huang et al., 2021; Wang et al., 2020). This exceptional situation during the haze event in China thus lends itself to a large-scale “experiment” to study the unusual phenomenon driven by atmospheric chemistry and meteorology.
PM2.5 and ground-level ozone (O3), especially in highly polluted regions, adversely affect human health (Lelieveld et al., 2015), agriculture (Feng et al., 2015; Wang et al., 2007), and the Earth's radiation budget (Liao et al., 2015; Dang and Liao, 2019), thereby leading to premature mortality, decreases in crop yields, and altering the climate. Anthropogenic PM2.5, in addition to being generated by fossil fuels and biomass burning, is also produced through the reactions of inorganics (e.g. NO, NO2, SO2, and NH3) and volatile organic compounds (VOCs) (Zheng et al., 2017). In contrast, O3 is not directly emitted but is formed through a series of photochemical reactions involving multiple precursors (e.g. carbon monoxide (CO), methane (CH4), VOCs, NO, and NO2) (Ge et al., 2013). Apart from intense local primary emissions and secondary chemical formation, stagnant meteorological conditions and regional transport are two additional contributors to severe haze and O3 pollution events (Shen et al., 2020). Recently, a series of air quality regulations (Clean Air Plans, CAPs) released by the Chinese government have resulted in a notable decrease in anthropogenic emissions, leading to a substantial improvement in air quality due to reductions in PM2.5 concentrations but a nationwide enhancement of O3 pollution in China (Shen et al., 2020; K. Li et al., 2019). It is known that the impacts of meteorological conditions and atmospheric chemical processes could result in non-linear responses of PM2.5 and O3 to the decreases in their precursor concentrations (H. Li et al., 2019; Li et al., 2020). However, the specific responses of air pollutants and atmospheric chemistry to emissions and meteorological conditions have not been clearly determined.
For the haze event in China introduced above, recent studies on the topic suggested that complex atmospheric chemistry processes triggered by emission reductions and meteorological conditions are responsible for the unexpected haze formation regionally during the COVID-19 lockdown (Le et al., 2020; Fu et al., 2021). In detail, the substantial decrease in NO2 emissions during the COVID-19 lockdown resulted in an increase in O3 levels and nighttime NO3 radical formation, enhancing the atmospheric oxidation capacity (AOC) and facilitating the formation of secondary aerosols. Additionally, the presence of anomalous relative humidity promoted heterogeneous chemistry processes (Le et al., 2020; Huang et al., 2021; Ma et al., 2022). After the formation, more generated secondary aerosols were transported toward the in situ measurement station in northern China (Lv et al., 2020). Meanwhile, some research pointed out that the high ambient humidity is also the key to the NC haze from the perspective of adjusting pH to control the formation efficiency of nitrate aerosol, which is one of the major species for NC haze (Chang et al., 2020; Sun et al., 2020). In addition to the influence of changes in chemical reactions, a physical mechanism known as the aerosol–planetary boundary layer (PBL) interaction is also considered to have had a significant impact on the haze formation (Su et al., 2020). For O3, the decline in climatology in SC was attributable to the weakened photochemistry reactions due to the emission reductions in and the dilution effect of the clean air masses on the mass loadings of NOx and VOC (Fu et al., 2021; Liu et al., 2021). Overall, meteorological conditions always played a critical role; high relative humidity is the trigger of aerosol heterogeneous chemistry by adjusting the particle pH or providing a reaction medium. Meanwhile, the transport of the secondary aerosol or clean air masses and shallow PBL height are primarily driven by wind and pressure, respectively. Importantly, the above weather elements are modulated synergistically by synoptic-scale weather patterns (SWPs) or large-scale atmospheric circulations.
Numerous studies have been conducted worldwide to explore the direct connections between SWPs and air quality fields (Dayan and Levy, 2002; Demuzere et al., 2009; Pope et al., 2015; Hegarty et al., 2007; Bei et al., 2016; Jiang et al., 2017), indicating that good air quality conditions are often observed under cyclonic weather systems with certain types and positions, while poor air quality is frequently associated with anticyclonic conditions. However, the relationship between air quality and SWPs can differ depending on location, time, and pollutants (Jiang et al., 2017; Liao et al., 2017). The classification methods for SWPs employed in these studies can generally be categorised into the following three groups: subjective (manual), mixed (hybrid), and objective (automated) (Huth et al., 2008). Objective classification methods for SWPs are known for their speed, objectivity, and high reproducibility, often achieving classification 100 % automatically. On the other hand, manual approaches for SWPs have the advantage of allowing the user to control the selection of representative weather types (Lewis and Keim, 2015). Hybrid classification combines the strengths of both manual and automated techniques, where the users define the classification types, but the classification process itself is performed automatically (Frakes and Yarnal, 1997; Lewis and Keim, 2015; Huth et al., 2008). At present, the subjective method was used to investigate the contribution of six SWPs to PM2.5 pollution in Northwest China (Bei et al., 2016). While subjective approaches are suitable for analysing short time series, they have significant limitations when applied to large datasets spanning extended periods of time (Chen et al., 2022). Hybrid classification for SWPs is more popular than the subjective one and was applied to explore the impact of SWPs on O3, PM2.5 and CO in the North China Plain (NCP), Yangtze River Delta (YRD), and Eastern China, respectively (Zhang et al., 2013, 2016; Han et al., 2018; Liao et al., 2017). As an objective classification and with its advantages, the self-organising map (SOM) algorithm has been used to identify the impact of different SWPs on O3 and PM2.5 in the YRD and Sichuan Basin (SCB), respectively (Shu et al., 2020; Zhan et al., 2019). In addition, the principle component analysis T mode, k-means clustering, and other clustering approaches (like the Lamb–Jenkinson method) also were adopted to quantify the impact of SWPs on O3 in NCP (Miao et al., 2017; Dong et al., 2020; Liu et al., 2019).
Based on the studies mentioned above, previous research on the drivers for unusual haze and O3 decline events has concentrated on the influence of atmospheric chemistry processes accompanied by the anomaly of one or two weather elements but has not yet focused on the impact of weather elements in a comprehensive and synergistic way. Therefore, we here investigate the effect of anomalies in weather conditions with respect to climatology on PM2.5 and O3 concentrations during the haze event in the COVID-19 lockdown, specifically. To this end, we apply a novel SOM algorithm called structural SOM (S-SOM) to identify the most meaningful clustering number of weather patterns and compare it to other traditional SOM methods including ED-SOM and the SOM algorithm based on the Pearson correlation coefficient (hereafter named COR-SOM). Furthermore, after determining the weather patterns, we evaluate the contribution of SWPs to PM2.5 and O3 changes during the COVID-19 lockdown in China. Last, to better understand what role each meteorological factor played in the PM2.5 and O3 pollution during this period, the SHapley Additive exPlanation (SHAP) approach is used to evaluate their relative importance for the predictions of the machine learning (ML) model. The knowledge gained will ultimately help to predict air pollution under future emission scenarios and weather patterns potentially altered by climate change.
2.1 Observational and model dataset sources
The hourly observation dataset during the first 2 months (January and February) from 2015 to 2020, including two air pollutants (PM2.5 and O3) and six meteorological factors (pressure is P, precipitation is Precip, temperature is Temp, relative humidity is RH, wind speed is WS, wind direction is WD), was divided into two parts, namely the training dataset and test dataset, which were used to build a prediction model based on machine learning. Air pollutant and meteorological station datasets were downloaded from the National Environmental Monitoring Centre (http://www.cnemc.cn, last access: 28 May 2024) and the National Meteorological Science Data Center repository (https://data.cma.cn, last access: 28 May 2024). To better understand the climatological behaviour of air pollutants, 367 surface measurement stations across China are divided into eight different regions (including NCP for North China Plain, IM for Inner Mongolia, NEC for North Eastern China, YRD for Yangtze River Delta, CS for Central South, SC for Southern Coast, TP for Tibet Plateau, and NWC for North Western China) based on different typical climate characteristics (see the climate classification scheme at https://www.resdc.cn/data.aspx?DATAID=243, last access: 28 May 2024; Fig. 1). In addition, hourly surface ERA5 data with 0.25 × 0.25 spatial resolution, including mean sea level pressure (MSLP) (at 14:00 local time per day) and total solar radiation (SR), were retrieved from the European Centre for Medium-Range Weather Forecasts (ECMWF).
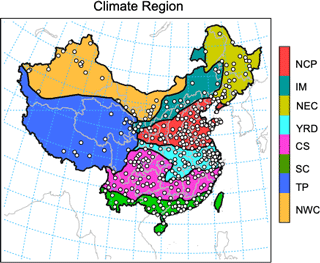
Figure 1The spatial distribution of air quality measurement stations in different climate regions (circles represent surface measurement stations; colours indicate different climate zones). The abbreviations used in the figure are as follows: NCP – North China Plain; IM – Inner Mongolia; NEC – North Eastern China; YRD – Yangtze River Delta; CS – Central South; SC – Southern Coast; TP – Tibet Plateau; and NWC – North Western China.
2.2 Structural SOM algorithm (S-SOM)
The SOM algorithm involves an iterative learning processes that progressively update the nodes in the output map until they converge to a stable solution. During each learning step, the SOM algorithm selects an input vector in a random way and then searches for a node that best matches that particular vector. Traditionally, the Euclidean distance (ED) in the SOM algorithm is often used as a criterion to search for the winning node that is closest to an input vector. ED is very popular in the SOM algorithm but with significant shortcomings when applied to compare structured inputs with temporal or spatial orders. As a result, the limitations of ED become particularly significant in climatology research, where the data are often given with a spatial and temporal structure, which might result in the degradation of the spatial correlations between air pressure patterns in weather maps (Doan et al., 2021).
The S-SOM algorithm is executed following the procedure proposed by Kohonen (1982) and is widely used in many studies. To begin, an S-SOM is initialised by configuring the SOM node and determining the number of training iterations. The training process involves three key steps:
-
selecting an input vector,
-
identifying the best-matching unit in the SOM for the input vector, and
-
updating the weight vectors of the SOM nodes using specific parameters.
The only difference between the traditional SOM and S-SOM is that the similarity index (S-SIM) rather than ED is used to compare the similarity between vectors. S-SOM was first proposed by Wang et al. (2004) and can be expressed in the following equation:
Here, x and y are two vectors, and l, c, and s are three comparison measurements representing luminance, contrast, and structure, respectively. The three comparison functions are as follows:
Here, the average and standard deviation values are represented by μ and σ, respectively. The parameters c1, c2, and c3 are used to stabilise the division operations involving a weak denominator. The luminance, contrast, and structure in the S-SOM formula are three elements of human perception. Luminance assesses the similarity in brightness values between images. Contrast quantifies the similarity in illumination variability among images. Last, the structure measures the correlation in spatial interdependencies between images, reflecting how the spatial elements of the images are related to each other (Wang and Bovik, 2009). Here, we can set the values of c1, c2, and c3 to 0 and the weights α, β, and γ to 1 to simplify the model (Doan et al., 2021). The final expression shows
As the function shows, S-SOM ranges from −1 to 1. A value of 1 indicates complete similarity, while a value of −1 indicates complete dissimilarity. S-SOM offers robust, user-friendly, and comprehensible alternatives to the conventional ED approach, particularly when dealing with datasets with a spatial and temporal order (Wang and Bovik, 2009).
2.3 Gradient-boosting machine (GBM) model
The impact of meteorological factors on the variation in the air pollutant concentrations is typically determined via chemical transport models. However, these model predictions are associated with substantial uncertainty, since they rely on the correct quantification of changes in the emission inventory of each city under multi-faceted anthropogenic air pollution interventions (e.g. clean-air plans and COVID-19 lockdown measures). Besides, uncertainties can also be derived from the chemical mechanism (Knote et al., 2015; Weng et al., 2023). Here, a gradient-boosting machine (GBM) model was trained with observations of meteorological factors, with the GBM being able to capture the location-specific characteristics and thus being suitable for the prediction of air pollutant concentrations attributable to the impact of meteorology in different cities across China. Observations of meteorological factors, together with time variables from 2015 to 2019, are considered to be the training dataset to predict the concentrations of PM2.5 and O3 in China. The meteorological factors are listed as follows: P, Precip, Temp, RH, WS, and WD. The time variables include the Julian day (JD), day of week (DOW), holidays, and Chinese New Year (CNY) days in each year. For the GBM prediction model, cross-validation is mainly used to estimate how accurately a predictive model will perform in practice. To check the accuracy of the ML model used in our study, a time series split rolling cross-validation based on five splits was used, for which data used for the training task always preceded the data used for validation. In detail, the ML training model was used for 2015, 2015–2016, 2015–2017, 2015–2018, and 2015–2019, while the testing of the model then was implemented over the first 2 months of 2016, 2017, 2018, 2019, and 2020, respectively (Shen et al., 2022). The hyperparameters of the model that we selected are as follows: “number_leaves”, “objective”, “min_data_in_leaf”, “learning_rate”, “feature_fraction”, “bagging_fraction”, “bagging_freq”, and “metric” (detailed parameter information of the model can be accessed from https://lightgbm.readthedocs.io/en/latest/Parameters.html, last access: 28 May 2024). After selecting the best ML model under cross-validation, a ML experiment was designed to make a prediction of PM2.5 and O3 in the first 2 months of 2020.
2.4 SHapley Additive exPlanation (SHAP) method
Quantifying the importance of input features of the GBM model is as vital as the overall accuracy of the prediction itself. However, interpreting the higher accuracy achieved by ensemble or ML models on certain datasets can be a challenging task. To deal with this contradiction between higher accuracy and non-interpretability, SHAP, a game theory approach, is applied to calculate the importance value for each specific independent feature. In brief, the SHAP value of each feature is attributed to the difference in one prediction output with one feature versus the prediction output without this corresponding feature. SHAP's local explanations can vary in terms of being positive or negative, reflecting how predictors influence the predicted outcome. In contrast, other ML methods typically yield a single positive value, indicating overall importance. Specifically, in local interpretability analysis (Lundberg et al., 2020), SHAP indicates the contribution of each variable to the prediction of a specific sample. This contribution is assessed from the base value (the predicted mean value) to the final model output. Variables that push the prediction to higher values are displayed as positive, while those decreasing the prediction are shown to be negative. For each predicted model with n variables in one sample (xl) and the predicted output f(xl), the equation of the prediction function is described as follows:
where xl is the input with variable m in the prediction model f generating the SHAP value of Em(f,xl). E0(f,x) is the expected value for the prediction model over the whole dataset.
3.1 Spatial variations in the air pollutant and meteorology in climatology
The spatial distribution of the fractional differences in air pollutant concentrations during the haze event from 21 January to 9 February 2020, calculated between mean values during the event in 2020 and the values averaged over the same period from 2015 to 2019, for all six air pollutants is shown in Fig. 2. Half of the climate regions, including the YRD, CS, SC, and TP, showed different magnitudes in the decreases (increases) (Table 1) in the climatology for PM2.5, PM10, NO2, SO2, and CO (O3), which were primarily attributed to the significant anthropogenic emission reduction during the COVID-19 lockdown (Nie et al., 2021; Wang et al., 2022; Shen et al., 2022). However, contrary to expectations, PM2.5 concentrations did not drop as anticipated at the beginning of the lockdown in NCP, IM, NEC, and NWC. Instead, these regions experienced an unexpected increase of 8.6 %, 31.8 %, 22.3 %, and 2 % compared to climatology during the same period, respectively. Even though the decline was small, O3 showed an unexpected drop of −0.8 % in SC when compared to climatology during the same period. Our recent work also found a −0.9 % decline in O3 driven by the meteorological effect during the COVID-19 lockdown across China (Shen et al., 2022). As a fractional difference compared to climatology, the spatial distribution of the key meteorological variables RH, P, Precip, Temp, and WS are shown in Fig. 3. Generally, positive RH and negative WS anomalies are always accompanied by the strong regional elevation of PM2.5 in NCP, NEC, IM, and NWC. Positive P anomalies coupled with increased PM2.5 demonstrate the most prominent regional characteristics in NEC. In SC, the most noticeable features were observed as a combination of hotspot Precip anomalies and decreased O3 levels. Overall, the regional characteristics of PM2.5 and O3 all have a close relationship with different meteorological anomalies, which are usually controlled by the regionally prevailing SWPs.
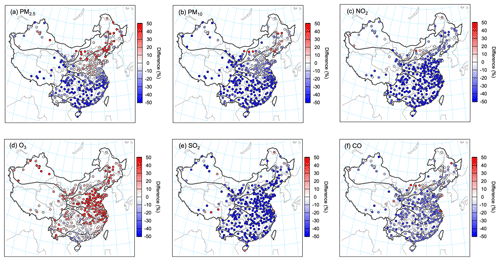
Figure 2The spatial distributions of fractional differences between mean values during the haze event in 2020 and the climatology over the same period during the years 2015–2019 for six air pollutants (including PM2.5, PM10, NO2, O3, SO2, and CO).
3.2 Identification of the SWPs during the unexpected haze event
To identify which SWPs can regionally induce an unexpected PM2.5 increase and O3 reduction compared to climatology averaged over the years 2015–2019, three different SOM methods were employed to identify different types of SWPs (from two to eight) using MSLP data in the first 2 months from 2015 to 2020 over China. Figure 4 shows MSLP patterns identified by S-SOM, COR-SOM, and ED-SOM running three nodes, respectively. Taking this three-node analysis as an example, we find that the three SWPs identified by S-SOM (Fig. 4a, b, and c) are clearly distinct from each other. On the other hand, ED-SOM (Fig. 4d and e) and COR-SOM (Fig. 4g and h) both classify two similar SWPs characterised by high-pressure systems over Siberia, thus resulting in a failure of clustering. This interpretation is supported by the result of clustering the number distributions for the three-node SWPs (Fig. 5d). It should be noted that cluster numbers do not necessarily correspond to the same pattern between S-, ED-, or COR-SOM. Here, it is found that S-SOM results are in a more “ordered” clustering of nodes, where a prominent node (62.9 %) is accompanied by two non-dominant nodes (7.5 % and 29.5 %). On the other hand, both ED-SOM and COR-SOM exhibit relatively similar cluster sizes with percentages of 27 %, 35.1 %, and 37.9 % for ED-SOM and 40.7 %, 34.6 %, and 24.7 % for COR-SOM, highlighting the prevalence of a more “flat” clustering pattern. It can be concluded that the better classification method for three-node SWPs is S-SOM with an ordered clustering number distribution accompanied by a prominent node (Doan et al., 2021). This consistent finding is also observed in other cases (e.g. node numbers smaller or greater than 3; Figs. S1–S12 in the Supplement). Then, we make a further comparison of the node number distribution of S-SOM (Fig. 5a), ED-SOM (Fig. 5b), and COR-SOM (Fig. 5c) in each year and find that S-SOM always has a prominent node with a value of more than 50 % (2015 with 50 %, 2016 with 85 %, 2017 with 64 %, 2018 with 81 %, 2019 with 63 %, and 2020 with 55 %), and the cluster sizes for ED-SOM and COR-SOM are close to each other as well, which is consistent with a recent study indicating a better performance of S-SOM (Doan et al., 2021). Therefore, in addition to the algorithmic advantages, the characteristics of ordered clustering nodes reinforce the superiority of the S-SOM approach.
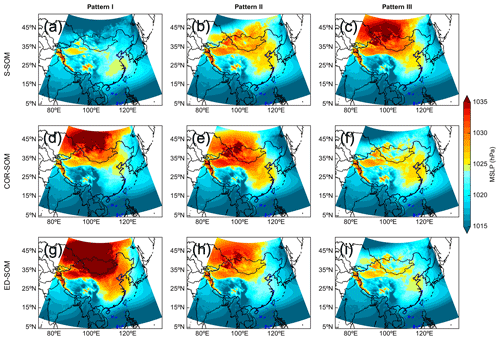
Figure 4Spatial distributions of three weather patterns for MSLP (mean sea level pressure) identified by S-SOM (a, b, c), COR-SOM (d, e, f), and ED-SOM (g, h, i) during the first 2 months from 2015 to 2020.
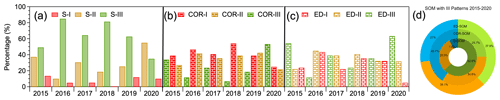
Figure 5Cluster size distributions identified by S-SOM (inner ring), COR-SOM (middle ring), and ED-SOM (outer ring) over the years 2015–2020 (d) and cluster days in each year. (a) S-SOM, (b) COR-SOM, and (c) ED-SOM.
In terms of structure characteristics of clustering number distribution for S-SOM, three-node SWPs (Fig. 4) and seven-node SWPs (Fig. S13) were regarded as being the optimal numbers of SWPs after checking the clustering number distribution for each run. From the top panel of Fig. 4, three types of SWPs identified by S-SOM demonstrate that NCP, YRD, NEC, and NWC are under the control or influence of different high-pressure systems. For seven-node SWPs identified by S-SOM, even though the high-pressure system varies in numbers and locations, some patterns (Fig. S13d and e) still have a relatively high similarity, which might be attributed to the over-splitting or a dataset that is too short to capture the full climatology. Overall, the result of the three-node SWPs of S-SOM is thus identified as the best solution to study the haze event in China in further detail.
3.3 Impact of weather elements on PM2.5 and O3 under the SWPs
To better understand the regional influence of different SWPs on PM2.5 and O3 concentration levels, NCP and NEC (SC), which have higher (lower) than expected concentrations for PM2.5 (O3) and have more measurement stations as well, were selected as the research domains. To investigate the cause of the unexpected PM2.5 and O3 variations with respect to climatology, a comparison of the identified three-node SWPs is made between the days of 2020 and 2015–2019. As shown in Fig. 6 and as detailed in Figs. 7–9, pattern I in 2020 (Fig. 6d) shows a north coastal high-pressure circulation system, located in the Yellow Sea, which is enhanced from that in 2015–2019 (Fig. 6a) and influences the NCP and NEC regions (see Figs. 7a, d–f and 8a, d–f) more strongly from the southeast direction with a generally warmer and, in the case of NEC, also faster airflow. The double-centre high-pressure system in pattern II is strengthened in 2020 (Fig. 6e) and located in the region of Mongolia and the Bohai Sea in China compared to 2015–2019 (Fig. 6b). This brings along a more stagnant, i.e. low speed and cold, but also extremely wet northern airflow controlling the NCP region (Fig. 7a, d–f) and a moderately wetter airflow dominating the NEC region (Fig. 8a, d–f). Pattern III, on the other hand, shows a much weakened Siberian high and a missing China north coastal high in 2020 (Fig. 6f), when compared to a pattern exhibiting two high-pressure centres during the 2015–2019 reference period (Fig. 6c). This leads to a generally warmer, slightly faster, and more humid airflow to the NCP (Fig. 7a, d–f) and NEC (Fig. 8a, d–f) regions. For SC, which is always located at the southernmost part of the observed high-pressure centres (Fig. 6), and for all three patterns, only small changes are seen in 2020 compared to the 2015–2019 time period with a more easterly component in the winds (Fig. 9a–c), leading to slightly warmer and (except for pattern III) moister airflow (Fig. 9d–f).
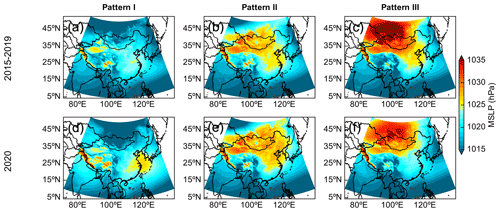
Figure 6Comparison of the three weather patterns between cluster days in 2020 (d, e, f) and 2015–2019 (a, b, c), respectively.
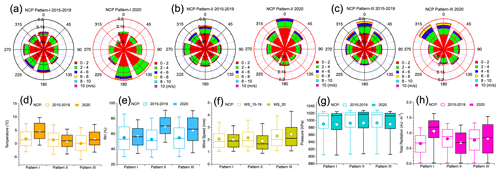
Figure 7Comparisons of different weather factors (including wind speed, wind direction, temperature, relative humidity, pressure, and total radiation) between cluster days in 2020 (red wind rose and solid box-and-whisker plots) and in 2015–2019 (black wind rose and hollow box-and-whisker plots) for the three weather patterns in NCP.
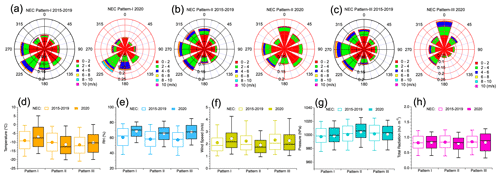
Figure 8Comparisons of different weather factors (including wind speed, wind direction, temperature, relative humidity, pressure, and total radiation) between cluster days in 2020 (red wind rose and solid box-and-whisker plots) and in 2015–2019 (black wind rose and hollow box-and-whisker plots) for the three weather patterns in NEC.
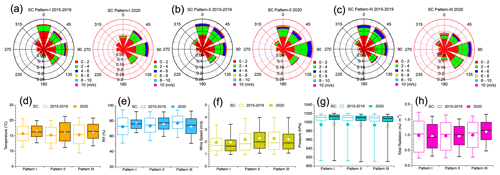
Figure 9Comparisons of different weather factors (including wind speed, wind direction, temperature, relative humidity, pressure, and total radiation) between cluster days in 2020 (red wind rose and solid box-and-whisker plots) and in 2015–2019 (black wind rose and hollow box-and-whisker plots) for the three weather patterns in SC.
We now turn to the discussion of the observed distributions of PM2.5 and O3 (Fig. 10) aggregated over the three SWPs and the regions of NCP, NEC, and SC for the 2020 and the 2015–2019 time periods, respectively. For PM2.5 in NCP (Fig. 10a), the mean values in patterns I, II, and III in 2015–2019 all remained at high pollution levels with values of 96.4, 92.6, and 87.7 µg m−3, respectively. In contrast, due to the anthropogenic emissions reductions during the lockdown period in 2020, the PM2.5 mean values for patterns I and III decreased to 68.8 and 59.8 µg m−3, even when coupled with a positive RH climatological anomaly (Fig. 7e: 2 % and 10 %), which could be conducive to generating additional PM2.5 generally. Unlike patterns I and III, the PM2.5 mean value in pattern II 2020 surprisingly keeps at an equivalent level (92.5 µg m−3) to pattern II in 2015–2019 (92.6 µg m−3) under a weather condition of a combination of the greatest RH anomaly (Fig. 7e; 17 %) and a negative WS anomaly (Fig. 7f; −0.3 m s−1), which offsets the contribution from the emissions reduction in NCP. For O3 in NCP (Fig. 10d), patterns I and III in 2020 exhibit greater temperature anomalies (Fig. 7d; 2.7 and 2.9 °C, consistent with higher total radiation levels; see Fig. 7h) and thus facilitate additional O3 generation (20 and 13 µg m−3). Pattern II in 2020 with a negative temperature anomaly (−0.1 °C, consistent with lower total radiation levels; see Fig. 7h) favours a more moderate O3 increase (3 µg m−3).
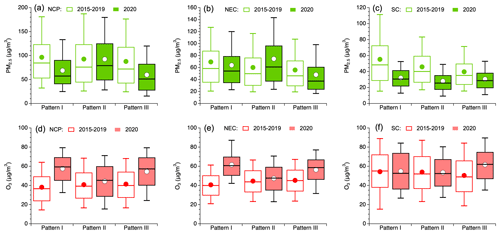
Figure 10Comparisons of PM2.5 (green colour) and O3 (red colour) between cluster days in 2020 (filled box-and-whisker plots) and in 2015–2019 (hollow box-and-whisker plots) for the three weather patterns in NCP (a, d), NEC (b, e), and SC (c, f).
In the NEC region, the maximum PM2.5 increase (15 µg m−3) occurred under the influence of pattern II in 2020 (Fig. 10b), with a negative wind speed anomaly (Fig. 8f; −0.3 m s−1) when compared to the same pattern in 2015–2019, indicating that the meteorological effect acts in the opposite way to the emission reductions during the COVID-19 lockdown period. Without an offsetting effect from the unfavourable meteorological conditions, mean values of PM2.5 for patterns I and III in 2020 decreased by 5 and 8 µg m−3, respectively. For O3 (Fig. 10e), unlike a negative temperature anomaly (Fig. 8d; −1.3 °C) in SWP II, both higher O3 increases in SWPs I (11 µg m−3) and III (11 µg m−3) compared to that in SWP II (2 µg m−3) are driven by positive temperature anomalies (Fig. 8d; 2 and 4.4 °C).
In the SC region, without an extreme weather element anomaly facilitating additional PM2.5 production, PM2.5 mean values for all three SWPs in 2020 are at a lower level than in 2015–2019 (Fig. 10c) and attributable to the emissions reductions during the COVID-19 lockdown. Higher precipitation levels in 2020 than during the 2015–2019 period also helped reduce PM2.5 levels (see Figs. 3c and S14). For O3 (Fig. 10f), a negative RH anomaly (Fig. 9e) for SWP III in 2020 led to the greatest O3 elevation for this region. On the other hand, the O3 in pattern I is found to remain at similar levels during both time periods since no significant differences in the weather patterns are found. Finally, a positive wind speed anomaly (Fig. 9f; 0.21 m s−1) is conducive to an unusual O3 decline (−0.5 µg m−3) in SWP II in 2020 when compared to 2015–2019, which is contrary to the O3 situation under the effect of all other SWPs discussed above.
Overall, we found that the unexpected PM2.5 pollution increase in NCP and NEC and an O3 decline in SC occur simultaneously but only during SWP II, which is equivalent to the situation found in the observations during the haze event. When we further investigate the calendar occurrences of the three different SWPs (Fig. S15), it is indeed found that 70 % of the haze days were associated with SWP II. This finding thus indicates that SWP II can be regarded as the representative weather pattern which best explains the cause of the unexpected haze and O3 decline events.
3.4 Predominant meteorological factors for PM2.5 and O3 pollution
After identifying which SWP could control the impact of each weather element on the PM2.5 and O3 levels, as observed during the haze event in 2020, we further use machine learning coupled with the SHAP approach to quantify the impact of each weather element on the PM2.5 and O3 under the business as usual (hereafter referred to as BAU) emission strength scenario during the haze event in 2020. This BAU scenario thereby is constructed by the gradient-boosting machine that trained the model using historical features to predict the future-dependent features without considering the huge emission reduction due to the COVID-19 lockdown. It is a counterfactual scenario assuming that the emission strength is the same as the BAU. In our previous study, the GBM model was applied to train daily data over 2015–2019 and predict six air pollutants including PM2.5 and O3 over the first 3 months of 2020 in 367 cities across China (Shen et al., 2022). The good performance of the GBM model was measured by achieving relatively high Pearson correlation coefficients (PCCs) and lower root mean squared errors (RMSEs) for the final predictions of PM2.5 and O3 (details can be found in the Supplement). Figures 11a, d, and g and S16 show the time series results in the first 2 months for PM2.5 and O3 between the observation and prediction in NCP, NEC, and SC, respectively. We find that the predictions generally agree well with the observations with reasonably high PCCs (NCP 0.7, NEC 0.6, and SC 0.8), indicating the good performance of the GBM model. Note that these predictions might be with high RMSEs due to the input being the BAU emissions instead of the lockdown emission reduction. Many studies have estimated PM2.5 and O3 using different prediction models, but they are limited to explain the final predictions (Xiao et al., 2018; Zhang et al., 2021; Jin et al., 2022), especially to provide details of specific input features (Weng et al., 2022). In our study, the SHAP module coupled to the GBM model was run to quantify the importance of the input variables during the haze event in 2020 (Fig. 11b, e, and h). On average, in the BAU scenario, the SHAP value of the time variables, including CNY, DOW, holidays, and JD, have no impact or negative impacts on PM2.5 and O3 (Fig. 11c, f, and i). For meteorological elements that enhanced the production of PM2.5, temperature ranked first among the six meteorological elements during the haze event, followed by RH, WS, and pressure in NCP versus WS, pressure, and RH in NEC, respectively. In terms of the positive SHAP values of temperature, pressure, RH, and WS in PM2.5 predictions, it reveals that those meteorological features push PM2.5 prediction to a higher value, suggesting that the final predictions were up to the baseline concentrations in NCP and NEC. In SC (Fig. 11i), positive mean SHAP values (2.2 µg m−3) for RH would be conducive to additional ozone generation due to the relatively lower values compared to before the haze event (Fig. 11g), thus pushing the predicted ozone higher. In contrast, a negative mean SHAP value (−5.5 µg m−3) for temperature during the haze event (Fig. 11i) would suppress ozone production attributable to smaller mean values, thus leading to a lower-ozone prediction. It should be noted that RH with a higher absolute SHAP value (9.8 µg m−3) exceeding temperature (−6.7 µg m−3) became the primary factor dominating the high-ozone level from 27 January to 2 February 2020. It is attributed to the SWP II in SC (Fig. 9d, f) with strong moist winds from the ocean, leading to the importance of RH surpassing temperature, which is consistent with the previous study (Weng et al., 2022). However, over the full period of the haze event, the negative effect of temperature dominated a higher-ozone level during the haze event based on the larger absolute SHAP value for temperature. The weaker than expected decrease in ozone as response to a lower temperature might be attributed to the emission reductions in the ozone precursors due to the COVID-19 lockdown measures. When we investigate the observed weather elements in 2020 against that averaged over 2015–2019, we can find that NCP and NEC were both under the control of SWP II, with lower temperatures and a higher RH, which facilitated the formation of PM2.5. Meanwhile, the SC region was influenced by the SWP II with higher temperatures, higher RH, and higher WS weather conditions, resulting in a decline in the O3 in climatology but a relative high-ozone level in prediction from the SHAP explanation. Overall, we can not only find the impact of weather elements on PM2.5 and O3 in the prediction scenario and in climatology, but we can also conclude that temperature plays a key role in such an impact.
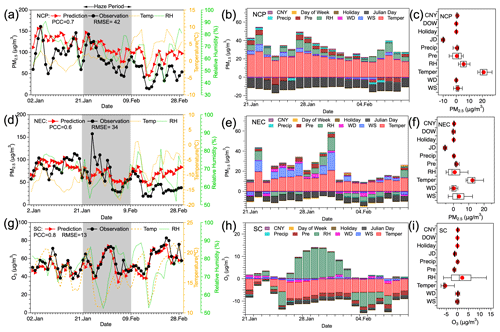
Figure 11Time series comparisons between observations (dotted black line) and predictions (triangled red line) combined with the SHAP values of the input variables (colourful bar) for the PM2.5 and O3 predictions in NCP (a, b), NEC (d, e), and SC (g, h), respectively (note that the box-and-whisker plots represent the mean SHAP value of the input variables during the prediction in NCP (c), NEC (f), and SC (i), respectively, and the shaded area (a, d, g) indicates the haze event period).
At the beginning of the COVID-19 pandemic, China suspended almost all non-essential human activities. However, serious haze pollution still occurred in North China during this period, triggering extensive investigations. On the other hand, while O3 concentrations were increasing across almost all of China due to the shift in the chemical regime, the SC region exhibited a decrease in O3. To further understand the role of meteorology in regulating air pollution during this period, we investigated in more detail the role of synoptic-scale weather patterns in driving the meteorology in these regions of China. To this end, we first determined the optimal approach for identifying synoptic-scale weather patterns out of three self-organising map methods. With the S-SOM method yielding the most optimal results, we then analysed the variation in the each meteorological factor under the control of the weather type that produces anomalous PM2.5 concentrations in the NCP and NEC and anomalous O3 concentrations in SC. Finally, we quantified the importance of each meteorological factor assuming a BAU scenario through a machine learning model coupled with a SHAP module.
The large-scale double-centre high-pressure system was identified by the optimal S-SOM method with a low-speed, cold, and extremely wet northern airflow controlling the NCP region; a low-speed, warm, and wet airflow from the Bohai Sea dominating the NEC region; and warmer air masses covering the SC region simultaneously. The above weather element anomalies controlled by the large-scale high-pressure system could well explain the unexpected PM2.5 pollution and O3 decline in climatology in NCP, NEC, and SC, respectively.
Moreover, the SHAP results indicate that, in the BAU scenario, the time series trend of PM2.5 and O3 have a high similarity with that of the observations, indicating a good performance of the prediction model (despite the differing emissions). The SHAP results stress the impact of meteorological conditions on PM2.5 and O3 and further quantify the importance of each weather element under the specific weather system, revealing the most important role that temperature played in PM2.5 pollution in NCP and NEC and in high O3 level (note that this has to be understood relative to a lower-ozone level compared to climatology) in SC, respectively.
Overall, this study provides a potential way to understand the synergistic effects of various meteorological factors in reducing pollution and to quantify the importance of each weather element as well. As a result, the provision of information on what role each weather element plays in unexpected air pollution cases can help policymakers to implement air pollution control strategies. However, our work will have to be expanded further and add more related meteorological factors to the GBM model to improve its performance. In fact, more studies should focus on the topic of understanding the impact of meteorology on different air pollutants in particular due to weather conditions in a changing climate.
Code and data are available upon reasonable request to the corresponding author (f.shen@fz-juelich.de).
The supplement related to this article is available online at: https://doi.org/10.5194/acp-24-6539-2024-supplement.
FS and MIH designed the experiments. FS and YY conducted the numerical experiment and wrote the article. MIH supervised the writing process and the experiment design. FS, MIH, and YY discussed the experimental design and analysis.
The contact author has declared that none of the authors has any competing interests.
Publisher’s note: Copernicus Publications remains neutral with regard to jurisdictional claims made in the text, published maps, institutional affiliations, or any other geographical representation in this paper. While Copernicus Publications makes every effort to include appropriate place names, the final responsibility lies with the authors.
This research has been supported by the Key Laboratory of Ecological Environment and Meteorology of Qinling and Loess Plateau, Shaanxi Province, China (grant no. 2023Y-22) and the Jining Meteorological Bureau (grant no. 2022JNZL09).
The article processing charges for this open-access publication were covered by the Forschungszentrum Jülich.
This paper was edited by Peer Nowack and reviewed by two anonymous referees.
Bei, N., Li, G., Huang, R.-J., Cao, J., Meng, N., Feng, T., Liu, S., Zhang, T., Zhang, Q., and Molina, L. T.: Typical synoptic situations and their impacts on the wintertime air pollution in the Guanzhong basin, China, Atmos. Chem. Phys., 16, 7373–7387, https://doi.org/10.5194/acp-16-7373-2016, 2016.
Chang, Y., Huang, R.-J., Ge, X., Huang, X., Hu, J., Duan, Y., Zou, Z., Liu, X., and Lehmann, M. F.: Puzzling Haze Events in China During the Coronavirus (COVID-19) Shutdown, Geophys. Res. Lett., 47, e2020GL088533, https://doi.org/10.1029/2020GL088533, 2020.
Chen, X., Wang, N., Wang, G., Wang, Z., Chen, H., Cheng, C., Li, M., Zheng, L., Wu, L., and Zhang, Q.: The influence of synoptic weather patterns on spatiotemporal characteristics of ozone pollution across Pearl River Delta of southern China, J. Geophys. Res.-Atmos., 127, e2022JD037121, https://doi.org/10.1029/2022JD037121, 2022.
Dang, R. and Liao, H.: Radiative forcing and health impact of aerosols and ozone in China as the consequence of clean air actions over 2012–2017, Geophys. Res. Lett., 46, 12511–12519, 2019.
Dayan, U. and Levy, I.: Relationship between synoptic-scale atmospheric circulation and ozone concentrations over Israel, J. Geophys. Res.-Atmos., 107, ACL 31-1–ACL 31-12, 2002.
Demuzere, M., Trigo, R. M., Vila-Guerau de Arellano, J., and van Lipzig, N. P. M.: The impact of weather and atmospheric circulation on O3 and PM10 levels at a rural mid-latitude site, Atmos. Chem. Phys., 9, 2695–2714, https://doi.org/10.5194/acp-9-2695-2009, 2009.
Doan, Q.-V., Kusaka, H., Sato, T., and Chen, F.: S-SOM v1.0: a structural self-organizing map algorithm for weather typing, Geosci. Model Dev., 14, 2097–2111, https://doi.org/10.5194/gmd-14-2097-2021, 2021.
Dong, Y., Li, J., Guo, J., Jiang, Z., Chu, Y., Chang, L., Yang, Y., and Liao, H.: The impact of synoptic patterns on summertime ozone pollution in the North China Plain, Sci. Total Environ., 735, 139559, https://doi.org/10.1016/j.scitotenv.2020.139559, 2020.
Feng, Z., Hu, E., Wang, X., Jiang, L., and Liu, X.: Ground-level O3 pollution and its impacts on food crops in China: a review, Environ. Pollut., 199, 42–48, 2015.
Frakes, B. and Yarnal, B.: A procedure for blending manual and correlation-based synoptic classifications, Int. J. Climatol., 17, 1381=-1396, https://doi.org/10.1002/(SICI)1097-0088(19971115)17:13<1381::AID-JOC204>3.0.CO;2-Q, 1997.
Fu, S., Guo, M., Fan, L., Deng, Q., Han, D., Wei, Y., Luo, J., Qin, G., and Cheng, J.: Ozone pollution mitigation in guangxi (south China) driven by meteorology and anthropogenic emissions during the COVID-19 lockdown, Environ. Pollut., 272, 115927, https://doi.org/10.1016/j.envpol.2020.115927, 2021.
Ge, B., Sun, Y., Liu, Y., Dong, H., Ji, D., Jiang, Q., Li, J., and Wang, Z.: Nitrogen dioxide measurement by cavity attenuated phase shift spectroscopy (CAPS) and implications in ozone production efficiency and nitrate formation in Beijing, China, J. Geophys. Res.-Atmos., 118, 9499–9509, 2013.
Han, H., Liu, J., Yuan, H., Jiang, F., Zhu, Y., Wu, Y., Wang, T., and Zhuang, B.: Impacts of synoptic weather patterns and their persistency on free tropospheric carbon monoxide concentrations and outflow in eastern China, J. Geophys. Res.-Atmos., 123, 7024–7046, 2018.
He, G., Pan, Y., and Tanaka, T.: The short-term impacts of COVID-19 lockdown on urban air pollution in China, Nature Sustainability, 3, 1005–1011, https://doi.org/10.1038/s41893-020-0581-y, 2020.
Hegarty, J., Mao, H., and Talbot, R.: Synoptic controls on summertime surface ozone in the northeastern United States, J. Geophys. Res.-Atmos., 112, D14306, https://doi.org/10.1029/2006JD008170, 2007.
Huang, X., Ding, A., Gao, J., Zheng, B., Zhou, D., Qi, X., Tang, R., Wang, J., Ren, C., Nie, W., Chi, X., Xu, Z., Chen, L., Li, Y., Che, F., Pang, N., Wang, H., Tong, D., Qin, W., Cheng, W., Liu, W., Fu, Q., Liu, B., Chai, F., Davis, S. J., Zhang, Q., and He, K.: Enhanced secondary pollution offset reduction of primary emissions during COVID-19 lockdown in China, Nat. Sci. Rev., 8, nwaa137, https://doi.org/10.1093/nsr/nwaa137, 2021.
Huth, R., Beck, C., Philipp, A., Demuzere, M., Ustrnul, Z., Cahynová, M., Kyselý, J., and Tveito, O. E.: Classifications of Atmospheric Circulation Patterns, Ann. N. Y. Acad. Sci., 1146, 105–152, https://doi.org/10.1196/annals.1446.019, 2008.
Jiang, N., Scorgie, Y., Hart, M., Riley, M. L., Crawford, J., Beggs, P. J., Edwards, G. C., Chang, L., Salter, D., and Virgilio, G. D.: Visualising the relationships between synoptic circulation type and air quality in Sydney, a subtropical coastal-basin environment, Int. J. Climatol., 37, 1211–1228, https://doi.org/10.1002/joc.4770, 2017.
Jin, H., Chen, X., Zhong, R., and Liu, M.: Influence and prediction of PM2.5 through multiple environmental variables in China, Sci. Total Environ., 849, 157910, https://doi.org/10.1016/j.scitotenv.2022.157910, 2022.
Knote, C., Tuccella, P., Curci, G., Emmons, L., Orlando, J. J., Madronich, S., Baró, R., Jiménez-Guerrero, P., Luecken, D., and Hogrefe, C.: Influence of the choice of gas-phase mechanism on predictions of key gaseous pollutants during the AQMEII phase-2 intercomparison, Atmos. Environ., 115, 553–568, 2015.
Kohonen, T.: A simple paradigm for the self-organized formation of structured feature maps, Competition and Cooperation in Neural Nets: Proceedings of the US-Japan Joint Seminar, Kyoto, Japan, 15–19 February 1982, Springer, Berlin, Heidelberg, 248–266, ISBN 978-3-540-11574-8, 1982.
Le, T., Wang, Y., Liu, L., Yang, J., Yung, Y. L., Li, G., and Seinfeld, J. H.: Unexpected air pollution with marked emission reductions during the COVID-19 outbreak in China, Science, 369, 702–706, 2020.
Lelieveld, J., Evans, J. S., Fnais, M., Giannadaki, D., and Pozzer, A.: The contribution of outdoor air pollution sources to premature mortality on a global scale, Nature, 525, 367–371, https://doi.org/10.1038/nature15371, 2015.
Lewis, A. B. and Keim, B. D.: A hybrid procedure for classifying synoptic weather types for Louisiana, USA, Int. J. Climatol., 35, 4247–4261, https://doi.org/10.1002/joc.4283, 2015.
Li, H., Cheng, J., Zhang, Q., Zheng, B., Zhang, Y., Zheng, G., and He, K.: Rapid transition in winter aerosol composition in Beijing from 2014 to 2017: response to clean air actions, Atmos. Chem. Phys., 19, 11485–11499, https://doi.org/10.5194/acp-19-11485-2019, 2019.
Li, K., Jacob, D. J., Liao, H., Zhu, J., Shah, V., Shen, L., Bates, K. H., Zhang, Q., and Zhai, S.: A two-pollutant strategy for improving ozone and particulate air quality in China, Nat. Geosci., 12, 906–910, https://doi.org/10.1038/s41561-019-0464-x, 2019.
Li, X., Zhao, B., Zhou, W., Shi, H., Yin, R., Cai, R., Yang, D., Dällenbach, K., Deng, C., Fu, Y., Qiao, X., Wang, L., Liu, Y., Yan, C., Kulmala, M., Zheng, J., Hao, J., Wang, S., and Jiang, J.: Responses of gaseous sulfuric acid and particulate sulfate to reduced SO2 concentration: A perspective from long-term measurements in Beijing, Sci. Total Environ., 721, 137700, https://doi.org/10.1016/j.scitotenv.2020.137700, 2020.
Liao, H., Chang, W., and Yang, Y.: Climatic effects of air pollutants over China: A review, Adv. Atmos. Sci., 32, 115–139, 2015.
Liao, Z., Gao, M., Sun, J., and Fan, S.: The impact of synoptic circulation on air quality and pollution-related human health in the Yangtze River Delta region, Sci. Total Environ., 607–608, 838–846, https://doi.org/10.1016/j.scitotenv.2017.07.031, 2017.
Liu, J., Wang, L., Li, M., Liao, Z., Sun, Y., Song, T., Gao, W., Wang, Y., Li, Y., Ji, D., Hu, B., Kerminen, V.-M., Wang, Y., and Kulmala, M.: Quantifying the impact of synoptic circulation patterns on ozone variability in northern China from April to October 2013–2017, Atmos. Chem. Phys., 19, 14477–14492, https://doi.org/10.5194/acp-19-14477-2019, 2019.
Liu, Y., Wang, T., Stavrakou, T., Elguindi, N., Doumbia, T., Granier, C., Bouarar, I., Gaubert, B., and Brasseur, G. P.: Diverse response of surface ozone to COVID-19 lockdown in China, Sci. Total Environ., 789, 147739, https://doi.org/10.1016/j.scitotenv.2021.147739, 2021.
Lundberg, S. M., Erion, G., Chen, H., DeGrave, A., Prutkin, J. M., Nair, B., Katz, R., Himmelfarb, J., Bansal, N., and Lee, S.-I.: From local explanations to global understanding with explainable AI for trees, Nature Machine Intelligence, 2, 56–67, 2020.
Lv, Z., Wang, X., Deng, F., Ying, Q., Archibald, A. T., Jones, R. L., Ding, Y., Cheng, Y., Fu, M., Liu, Y., Man, H., Xue, Z., He, K., Hao, J., and Liu, H.: Source–Receptor Relationship Revealed by the Halted Traffic and Aggravated Haze in Beijing during the COVID-19 Lockdown, Environ. Sci. Technol., 54, 15660–15670, https://doi.org/10.1021/acs.est.0c04941, 2020.
Ma, T., Duan, F., Ma, Y., Zhang, Q., Xu, Y., Li, W., Zhu, L., and He, K.: Unbalanced emission reductions and adverse meteorological conditions facilitate the formation of secondary pollutants during the COVID-19 lockdown in Beijing, Sci. Total Environ., 838, 155970, https://doi.org/10.1016/j.scitotenv.2022.155970, 2022.
Miao, Y., Guo, J., Liu, S., Liu, H., Li, Z., Zhang, W., and Zhai, P.: Classification of summertime synoptic patterns in Beijing and their associations with boundary layer structure affecting aerosol pollution, Atmos. Chem. Phys., 17, 3097–3110, https://doi.org/10.5194/acp-17-3097-2017, 2017.
Nie, D., Shen, F., Wang, J., Ma, X., Li, Z., Ge, P., Ou, Y., Jiang, Y., Chen, M., and Chen, M.: Changes of air quality and its associated health and economic burden in 31 provincial capital cities in China during COVID-19 pandemic, Atmos. Res., 249, 105328, https://doi.org/10.1016/j.atmosres.2020.105328, 2021.
Pope, R. J., Savage, N. H., Chipperfield, M. P., Ordóñez, C., and Neal, L. S.: The influence of synoptic weather regimes on UK air quality: regional model studies of tropospheric column NO2, Atmos. Chem. Phys., 15, 11201–11215, https://doi.org/10.5194/acp-15-11201-2015, 2015.
Shen, F., Zhang, L., Jiang, L., Tang, M., Gai, X., Chen, M., and Ge, X.: Temporal variations of six ambient criteria air pollutants from 2015 to 2018, their spatial distributions, health risks and relationships with socioeconomic factors during 2018 in China, Environ. Int., 137, 105556, https://doi.org/10.1016/j.envint.2020.105556, 2020.
Shen, F., Hegglin, M. I., Luo, Y., Yuan, Y., Wang, B., Flemming, J., Wang, J., Zhang, Y., Chen, M., Yang, Q., and Ge, X.: Disentangling drivers of air pollutant and health risk changes during the COVID-19 lockdown in China, npj Clim. Atmos. Sci., 5, 54, https://doi.org/10.1038/s41612-022-00276-0, 2022.
Shu, L., Wang, T., Han, H., Xie, M., Chen, P., Li, M., and Wu, H.: Summertime ozone pollution in the Yangtze River Delta of eastern China during 2013–2017: Synoptic impacts and source apportionment, Environ. Pollut., 257, 113631, https://doi.org/10.1016/j.envpol.2019.113631, 2020.
Su, T., Li, Z., Zheng, Y., Luan, Q., and Guo, J.: Abnormally Shallow Boundary Layer Associated With Severe Air Pollution During the COVID-19 Lockdown in China, Geophys. Res. Lett., 47, e2020GL090041, https://doi.org/10.1029/2020GL090041, 2020.
Sun, Y., Lei, L., Zhou, W., Chen, C., He, Y., Sun, J., Li, Z., Xu, W., Wang, Q., Ji, D., Fu, P., Wang, Z., and Worsnop, D. R.: A chemical cocktail during the COVID-19 outbreak in Beijing, China: Insights from six-year aerosol particle composition measurements during the Chinese New Year holiday, Sci. Total Environ., 742, 140739, https://doi.org/10.1016/j.scitotenv.2020.140739, 2020.
Wang, H., Huang, C., Tao, W., Gao, Y., Wang, S., Jing, S., Wang, W., Yan, R., Wang, Q., and An, J.: Seasonality and reduced nitric oxide titration dominated ozone increase during COVID-19 lockdown in eastern China, npj Clim. Atmos. Sci., 5, 24, https://doi.org/10.1038/s41612-022-00249-3, 2022.
Wang, J., Lei, Y., Chen, Y., Wu, Y., Ge, X., Shen, F., Zhang, J., Ye, J., Nie, D., Zhao, X., and Chen, M.: Comparison of air pollutants and their health effects in two developed regions in China during the COVID-19 pandemic, J. Environ. Manage., 287, 112296, https://doi.org/10.1016/j.jenvman.2021.112296, 2021.
Wang, P., Chen, K., Zhu, S., Wang, P., and Zhang, H.: Severe air pollution events not avoided by reduced anthropogenic activities during COVID-19 outbreak, Resour. Conserv. Recy., 158, 104814, https://doi.org/10.1016/j.resconrec.2020.104814, 2020.
Wang, X., Manning, W., Feng, Z., and Zhu, Y.: Ground-level ozone in China: distribution and effects on crop yields, Environ. Pollut., 147, 394–400, 2007.
Wang, Z. and Bovik, A. C.: Mean squared error: Love it or leave it? A new look at signal fidelity measures, IEEE Signal Proc. Mag., 26, 98–117, 2009.
Wang, Z., Bovik, A. C., Sheikh, H. R., and Simoncelli, E. P.: Image quality assessment: from error visibility to structural similarity, IEEE T. Image Process., 13, 600–612, https://doi.org/10.1109/TIP.2003.819861, 2004.
Weng, X., Forster, G. L., and Nowack, P.: A machine learning approach to quantify meteorological drivers of ozone pollution in China from 2015 to 2019, Atmos. Chem. Phys., 22, 8385–8402, https://doi.org/10.5194/acp-22-8385-2022, 2022.
Weng, X., Li, J., Forster, G. L., and Nowack, P.: Large modeling uncertainty in projecting decadal surface ozone changes over city clusters of China, Geophys. Res. Lett., 50, e2023GL103241, https://doi.org/10.1029/2023GL103241, 2023.
WHO (World Health Organisation): COVID-19 deaths, WHO COVID-19 Dashboard, World Health Organisation, https://data.who.int/dashboards/covid19/deaths?n=o (last access: 28 May 2024), 2024.
Xiao, Q., Chang, H. H., Geng, G., and Liu, Y.: An ensemble machine-learning model to predict historical PM2.5 concentrations in China from satellite data, Environ. Sci. Technol., 52, 13260–13269, 2018.
Xu, J., Ge, X., Zhang, X., Zhao, W., Zhang, R., and Zhang, Y.: COVID-19 Impact on the Concentration and Composition of Submicron Particulate Matter in a Typical City of Northwest China, Geophys. Res. Lett., 47, e2020GL089035, https://doi.org/10.1029/2020GL089035, 2020.
Zhan, C.-C., Xie, M., Fang, D.-X., Wang, T.-J., Wu, Z., Lu, H., Li, M.-M., Chen, P.-L., Zhuang, B.-L., and Li, S.: Synoptic weather patterns and their impacts on regional particle pollution in the city cluster of the Sichuan Basin, China, Atmos. Environ., 208, 34–47, 2019.
Zhang, M., Wu, D., and Xue, R.: Hourly prediction of PM2.5 concentration in Beijing based on Bi-LSTM neural network, Multimed. Tools Appl., 80, 24455–24468, 2021.
Zhang, Y., Mao, H., Ding, A., Zhou, D., and Fu, C.: Impact of synoptic weather patterns on spatio-temporal variation in surface O3 levels in Hong Kong during 1999–2011, Atmos. Environ., 73, 41–50, 2013.
Zhang, Y., Ding, A., Mao, H., Nie, W., Zhou, D., Liu, L., Huang, X., and Fu, C.: Impact of synoptic weather patterns and inter-decadal climate variability on air quality in the North China Plain during 1980–2013, Atmos. Environ., 124, 119–128, 2016.
Zheng, M., Yan, C., Wang, S., He, K., and Zhang, Y.: Understanding PM2.5 sources in China: challenges and perspectives, Nat. Sci. Rev., 4, 801–803, https://doi.org/10.1093/nsr/nwx129, 2017.