the Creative Commons Attribution 4.0 License.
the Creative Commons Attribution 4.0 License.
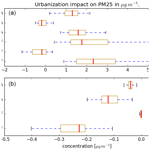
Impact of urbanization on fine particulate matter concentrations over central Europe
Alvaro Patricio Prieto Perez
Lukáš Bartík
Jan Karlický
Anahi Villalba-Pradas
Rural-to-urban transformation (RUT) is the process of turning a rural or natural land surface into an urban one, which brings about important modifications in the surface, causing well-known effects like the urban heat island (UHI), reduced wind speeds, and increased boundary layer heights. Moreover, with concentrated human activities, RUT introduces new emission sources which greatly perturb local and regional air pollution. Particulate matter (PM) is one of the key pollutants responsible for the deterioration of urban air quality and is still a major issue in European cities, with frequent exceedances of limit values. Here we introduce a regional chemistry–climate model (regional climate model RegCM coupled offline to chemistry transport model CAMx) study which quantifies how the process of RUT modified the PM concentrations over central Europe including the underlying controlling mechanisms that contribute to the final PM pollution. Apart from the two most studied ones, (i) urban emissions and (ii) urban canopy meteorological forcing (UCMF; i.e. the impact of modified meteorological conditions on air quality), we also analyse two less studied contributors to RUT's impact on air quality: (iii) the impact of modified dry-deposition velocities (DVs) due to urbanized land use and (iv) the impact of modified biogenic emissions due to urbanization-induced vegetation modifications and changes in meteorological conditions which affect these emissions. To calculate the magnitude of each of these RUT contributors, we perform a cascade of simulations, whereby each contributor is added one by one to the reference state, while focus is given on PM2.5 (particulate matter with diameter less then 2.5 µm). Its primary and secondary components, namely primary elemental carbon (PEC), sulfates (PSO4), nitrates (PNO3), ammonium (PNH4), and secondary organic aerosol (SOA), are analysed too.
The validation using surface measurements showed a systematic negative bias for the total PM2.5, which is probably caused by underestimated organic aerosol and partly by the negative bias in sulfates and elemental carbon. For ammonium and nitrates, the underestimation is limited to the warm season, while for winter, the model tends to overestimate their concentrations. However, in each case, the annual cycle is reasonably captured.
We evaluated the RUT impact on PM2.5 over a sample of 19 central European cities and found that the total impact of urbanization is about 2–3 and 1–1.5 µg m−3 in winter and summer, respectively. This is mainly driven by the impact of emissions alone causing a slightly higher impact (1.5–3.5 and 1.2–2 µg m−3 in winter and summer), while the effect of UCMF was a decrease at about 0.2–0.5 µg m−3 (in both seasons), which was mainly controlled by enhanced vertical eddy diffusion, while increases were modelled over rural areas. The transformation of rural land use into an urban one caused an increase in dry-deposition velocities by around 30 %–50 %, which alone resulted in a decrease in PM2.5 by 0.1–0.25 µg m−3 in both seasons. Finally, the impact of biogenic emission modifications due to modified land use and meteorological conditions caused a decrease in summer PM2.5 of about 0.1 µg m−3, while the winter effects were negligible. The total impact of urbanization on aerosol components is modelled to be (values indicate winter and summer averages) 0.4 and 0.3 µg m−3 for PEC, 0.05 and 0.02 µg m−3 for PSO4, 0.1 and 0.08 µg m−3 for PNO3, 0.04 and 0.03 µg m−3 for PNH4, and 0 and 0.05 µg m−3 for SOA. The main contributor of each of these components was the impact of emissions, which was usually larger than the total impact due to the fact that UCMF was counteracted with a decrease. For each aerosol component, the impact of modified DV was a clear decrease in concentration, and finally, the modifications of biogenic emissions impacted SOA predominantly, causing a summer decrease, while a very small secondary effect of secondary inorganic aerosol was modelled too (they increased).
In summary, we showed that when analysing the impact of urbanization on PM pollution, apart from the impact of emissions and the urban canopy meteorological forcing, one also has to consider the effect of modified land use and its impact on dry deposition. These were shown to be important in both seasons. For the effect of modified biogenic emissions, our calculations showed that they act on PM2.5 predominantly through SOA modifications, which only turned out to be important during summer.
- Article
(10514 KB) - Full-text XML
- BibTeX
- EndNote
In the upcoming years, more the 60 % of Earth's population will live in cities (United Nations, 2018), while urban areas in general represent only a tiny fraction of the habitable land. Moreover, the process of urbanization is predicted to continue by the end-of-the 21st century under all SSPs (Shared Socioeconomic Pathways) (Gao et al., 2020). It is thus a great desire to quantify the environmental footprints' urbanization or, more precisely, the causes of rural-to-urban transformation (RUT).
RUT acts via two primary pathways: (a) with the human activities concentrated in urban areas, a great amount of emissions are introduced (both green-house-gas and short-lived pollutants), which not only affect local but also regional and global air pollution (Im and Kanakidou, 2012; Markakis et al., 2015; Timothy and Lawrence, 2009; Butler and Lawrence, 2009; Stock et al., 2013; Huszar et al., 2021), and (b) urban land surfaces differ greatly from rural ones through the introduction of artificial objects and surfaces in specific geometries (e.g. street canyons and buildings), which affect the surface-air fluxes of energy, momentum, and material, with strong consequences on meteorological conditions (Oke, 1982; Oke et al., 2017; Karlický et al., 2020), and at the same time, in the long term, they modify the regional climate (Huszar et al., 2014; Karlický et al., 2018). In other words, cities have a strong impact on the whole atmospheric environment (Folberth et al., 2015).
Regarding the first pathway, it is clear that urban emissions alone substantially deteriorate the local air pollution (Thunis et al., 2021). They are composed of a mixture of different gases like oxides of nitrogen (NOx) originating mainly from road transportation (Huszar et al., 2016a, 2021), along with volatile organic compounds (VOCs), carbon monoxide (CO), and sulfur dioxide (SO2) or ammonia (NH3). Further, urban emissions also contain primary aerosol in the form of elemental carbon (PEC), primary organic aerosol (POA), and other primary material like metals (Freney et al., 2014; Allan et al., 2010; Rivellini et al., 2020; Yang et al., 2023). By introducing urban emissions, large quantities of these primary pollutants are added to their background levels. Moreover, they are potentially responsible – as precursors – for the formation of secondary pollutants too. NOx, together with VOCs (partly supported by CO), leads to the formation of ozone (O3), while the NOx-to-VOC ratio determines the amount of O3 formed or destroyed (Beekmann and Vautard, 2010; Xue et al., 2014). Emissions of gaseous pollutants further lead to formation of secondary aerosol. NOx, SO2, and NH3 are absorbed by water droplets, leading to the formation of secondary inorganic aerosol (SIA). This includes sulfates (PSO4), nitrates (PNO3), and ammonium (PNH4). The main precursor for PSO4 is SO2, and despite it exhibiting decreasing global emissions (Zhong et al., 2020), many urban areas are still marked with a significant perturbation of aerosol burden due to this pollutant (Guttikunda et al., 2003; Yang et al., 2011). It has to be further noted that sulfates can be emitted directly too and thus contribute to total particulate matter (PM) pollution (Li et al., 2018). Nitrogen oxides are the main precursors for nitrate aerosol, via forming nitric acid (HNO3), which is easily absorbed by water (Seinfeld and Pandis, 1998), and it is well known that urban NOx can significantly contribute to total PM pollution via the formation of PNO3 (e.g. Lin et al., 2010). Ammonia (NH3), while it constitutes a relatively small fraction of urban emissions (although there is an indication that transport emits much more ammonia than previously thought (e.g. Walters et al., 2022)), efficiently helps the formation of sulfate and nitrate aerosol by reacting to ammonium sulfates and ammonium nitrates and is found to be very important in connection with urban emissions (e.g. Behera and Sharma, 2010, and references therein). The thermodynamical equilibrium of the ammonium–sulfate–nitrate–water solution is, in general, rather complicated and highly dependent on the ratio of emissions of SO2–NOx–NH3 as well as on the prevailing meteorological conditions (Martin et al., 2004). This presents the potential of high variability of the contribution of different cities to total aerosol burden.
There is a large number of studies that investigate the perturbation of the atmospheric composition due to the urban emissions: Lawrence et al. (2007), Butler and Lawrence (2009), and Stock et al. (2013) investigated the global impact of emissions from large urban agglomerations. On a regional scale, Im et al. (2011a, b), Im and Kanakidou (2012), Finardi et al. (2014), Skyllakou et al. (2014), Markakis et al. (2015), Hodneborg et al. (2011), Huszar et al. (2016a), and Hood et al. (2018) looked at European cities (e.g. Paris, London, Istanbul, Athens), but the regional fingerprint of Asian megacity emissions has also been of great interest (Guttikunda et al., 2003, 2005; Tie et al., 2013). They all showed that the concentrations of primary pollutants (both gaseous and primary aerosol) are substantially increased locally but also on regional scales. On the other hand, secondary pollutants like ozone can respond differently: for example, for these cities, decreases in urban cores are often modelled due to emissions caused by high NOx-to-VOC ratios (e.g. Huszar et al., 2016a, 2021). Further, it was found that air pollution in cities is mainly determined by local sources; however a considerable part of the total concentration is associated with rural ones (Panagi et al., 2020; Thunis et al., 2021; Huszar et al., 2021).
Besides the direct impact of urban emissions, urbanization also influences air chemistry via so-called “urban canopy meteorological forcing” (UCMF), as introduced by Huszar et al. (2020a). The urban land surface brings about higher temperatures (urban heat island or UHI; Oke, 1982; Karlický et al., 2020; Sokhi et al., 2022), drag-induced wind-speed reductions (Huszar et al., 2018b; Zha et al., 2019), and enhanced vertical turbulent diffusion, along with elevated planetary boundary layer height (Ren et al., 2019; Wang et al., 2021). Further, it has a clear impact on the hydrological cycle by removing the precipitated water via drainage and thus decreasing the humidity over cities (Richard, 2004; Huszar et al., 2018b). UCMF then propagates to modifications in transport, deposition, and chemical transformation of the emitted pollutants, leading to modifications of their concentrations and linking the urban meteorological conditions to urban pollution very tightly (Ulpiani, 2021). The impact of UCMF on air quality in and around cities (or also rural areas) was a focus of many modelling studies that found that the most important components of UCMF are temperature, wind speed, and turbulence (Struzewska and Kaminski, 2012; Liao et al., 2014; Kim et al., 2015; Jacobson et al., 2015; Zhu et al., 2017; Zhong et al., 2018; Y. Li et al., 2019; Huszar et al., 2018a, 2020a, b; Wei et al., 2018). Due to UCMF, primary gas-phase pollutants and PM are decreased over cities (driven mainly by urban-land-surface-induced vertical eddy diffusion increase). In the case of secondary pollutants, the situation is more difficult as the total impact of UCMF is a combination of the direct impact on the secondary pollutant and the impact on its precursors; for example, for ozone the resulting effect is an increase over the surface (Janssen et al., 2017; Yim et al., 2019; Y. Li et al., 2019; Kim et al., 2021; Kang et al., 2022; Huszar et al., 2022).
Apart from the impact of urban emissions and the impact of UCMF, RUT influences the final air pollution via two other less studied pathways too. The first is the impact of urbanization-induced land-surface change on the dry deposition of pollutants, and the second is the modification of biogenic emissions due to change (decrease) of vegetation distribution due to urbanization. The land-surface type determines the resistances of that surface (and the canopy layer), which in turn determines the dry-deposition velocities (DVs) (Zhang et al., 2003; Cherin et al., 2015; Hardacre et al., 2021). It has been shown by many that by urbanization and the consequent reduction of vegetation in urban areas, the deposition velocities are greatly reduced for some gaseous pollutants (e.g. NO2, O3), leading to their increased concentrations (Nowak and Dwyer, 2007; Mcdonald-Buller et al., 2001; Song et al., 2008; Tao et al., 2015); for others due to higher reactivity on solid surfaces (compared to vegetated ones), the DVs are increased, leading to concentration increase (Zhang et al., 2003). For aerosol, DVs are mainly determined by the sedimentation of the particles and by aerodynamic and boundary resistances (Zhang et al., 2001). While sedimentation is determined by particle size and shape, surface resistances are a function of the roughness length and friction velocities (Wesely, 1989), which are enhanced over urbanized land surfaces compared to rural ones. This alone would lead to a decrease in PM concentration, bearing larger DVs. However, this is also modulated by the modifications of precursor concentrations as a result of the land-surface changes associated with RUT. For example, Huszar et al. (2022) modelled decreases in NO2 and SO2 concentration due to land-surface change (and hence dry-deposition modifications) alone, which would imply an amplification of the land-surface-induced decrease in nitrates and sulfates. Further, it can be assumed that ammonia (NH3) concentrations are also modified by modified dry-deposition velocities, which in turn has impacts on the amount of ammonium salts formed.
Regarding the influence of the modified biogenic emissions (BVOCs, biogenic volatile organic compounds) as a result of urbanization, one has to realize that (i) the urbanization reduces the amount of vegetation (e.g. turning cropland into urban built-up areas), which alone reduces the emission of biogenic substances (Song et al., 2008), and (ii) it was detailed above that urban areas exhibit higher temperatures, and moreover it seems that cloudiness is somewhat reduced above cities too (with respect to rural regions), meaning higher solar incident radiation at the surface (Karlický et al., 2020) – both promoting the vegetation metabolism, resulting in higher fluxes of BVOCs (Guenther et al., 2006). These two effects (i and ii) counteract each other, but the dominant one is probably the vegetation effect (Y. Li et al., 2019; Huszar et al., 2022); i.e. due to urbanization, BVOC emissions are reduced. As for the effect of such reduction, it is expected that near-surface ozone concentration will be decreased as urban areas are usually VOC-limited (Song et al., 2008). In the case of PM, this will act via modification of the formation of secondary organic aerosol (SOA). It has been shown by many that BVOCs are important precursors of SOA and responsible for the formation of biogenic secondary organic aerosol (BSOA; Gao et al., 2022). Couvidat et al. (2013) showed that almost one-third of the organic material in the Paris region originates from biogenic VOCs. The great importance of BVOCs in urban SOA formation was also confirmed by Sartelet et al. (2012), Hu et al. (2017), Nagori et al. (2019), and Ma et al. (2023), but of course, anthropogenic precursors also remain very important (Zhang et al., 2015; Guo et a., 2022). While it is clear that most of the BVOC emissions originate from rural and natural land surfaces (i.e. “non-urban” areas Lin et al., 2016), it is expected that any change in urban BVOC emissions as a result of urban expansion will have an immediate affect on SOA concentrations too and, hence, the total PM.
To summarize, urbanization (RUT) greatly modifies the air composition over both the cities themselves but also over surrounding rural areas, while this modification is the result of four impacts or contributors that add up to the background air pollution level (i.e. that without urbanization): namely, (i) the effect of urban emissions (“DEMIS”); (ii) the effect of urban canopy meteorological forcing (UCMF) on transport and chemical transformations (“DMET”); (iii) the effect of modified dry-deposition; velocities as a result of modified (urbanized) land surface (“DLU_D”) and, finally, (iv) the effect of modified emissions of BVOCs due to modified vegetation cover and meteorology (“DBVOC”). Together, they constitute the total RUT impact (“DTOT”).
These four impacts were also formulated in our previous paper (Huszar et al., 2022) in which we looked, using regional chemistry-transport models coupled to regional climate models, at their effect on gas-phase chemistry; however most of the other studies mentioned above focused either on the total impact of the urbanization or on some of the individual impacts without a detailed analysis of the contribution of each of them. The above-mentioned Huszar et al. (2022) study indeed aimed at the quantification of each individual impact as well as the total impact as one of the first studies of its kind analysing the central European domain at a moderate horizontal resolution. Here, as a follow-up study, we extend our analysis conducted there to particulate matter and will investigate how the total PM (as PM2.5, particles of diameter less than 2.5 µm) as well as its primary and secondary components, responds to these impacts. To fulfil this goal, a background or reference state has to be defined to which these impacts will be gradually added: for our purpose, that reference state will be the non-urbanized land surface without any urban emissions (only rural ones) and without the effect of UCMF. The analysis will focus on present-day conditions, which includes present day driving climate, emissions and land use. The four listed impacts will then be gradually added to this reference (i.e. non-urbanized) state in a cascading manner. To consider the uncertainty arising from a different background climate, size, and emissions from different cities, we conduct our analysis on a larger selection of 19 cities from central Europe.
As mentioned, our analysis will focus on PM2.5 and its components. Despite notable improvements in European PM pollution, EEA (2022) reports that in 2020, 96 % of the urban population in the European Union was exposed to high levels of PM2.5. This makes the investigation of the components and contributors to urban PM pollution very important. It also has to be noted that urban air quality is influenced not only by the local effects. Im and Kanakidou (2012) and Huszar et al. (2016a), for example, showed that emissions from other areas (rural or other, even distant cities) represent a major fraction of urban pollution burdens. Also the urban canopy meteorological forcing can act not only locally over cities but over regional scales too, and UCMF triggered by one city can have impact on other ones too, as shown by Huszar et al. (2014). Here we however will be interested in the local effects only, without looking at the inter-urban influences (i.e. the effect different cities pose on each other mutually).
The study is structured in the following way: the Introduction is followed by the presentation of experimental tools (models), their configuration, and the data used. Next, the experiments performed are described and the results summarized in the Result section. Finally, these are discussed and conclusions are drawn.
2.1 Models used
The study uses the same models, model settings, and input data as Huszar et al. (2022). Here we will therefore only summarize the most relevant information about the model setup stressing the eventual differences.
The chemistry transport model (CTM) simulations were carried out by the CAMx version 7.10 model (Ramboll, 2020a) using the Carbon Bond 6 revision 5 (CB6r5) scheme (Cao et al., 2021). Aerosol physics and chemistry were treated with a static two-mode approach, and the ISORROPIA thermodynamic equilibrium model (Nenes and Pandis, 1998) was applied for the secondary inorganic aerosol formation. For secondary organic aerosol (SOA), the SOAP equilibrium scheme (Strader et al., 1999) was used. For wet- and dry-deposition treatment, the Seinfeld and Pandis (1998) and Zhang et al. (2001, 2003) methods were used.
CAMx was driven by the regional climate model (RCM) RegCM version 4.7 (Giorgi et al., 2012) using non-hydrostatic dynamics. Planetary boundary layer (PBL) physics, cloud and rain microphysics, convection, and radiation are treated following Holtslag et al. (1990), Hong et al. (2004), and Tiedtke et al. (1989). The atmosphere–biosphere–surface coupling was treated with the Community Land Model (CLM) version 4.5 (Oleson et al., 2013) land-surface scheme, and to account for the urban scale processes, the CLMU module within CLM4.5 was used (Oleson et al., 2008, 2010). CLMU adopts the classical canyon geometry approach; i.e. cities are represented as networks of street canyons with specified geometry and surface parameters (Oke et al., 2017), while anthropogenic heat (AH) from heating and waste heat from air conditioning are accounted for. AH from traffic is not calculated, which might result in some underestimation of temperature due to this missing heat source. RegCM was coupled offline to CAMx using the RegCM2CAMx interface developed by Huszar et al. (2012). The vertical eddy diffusion coefficients (Kv) are diagnosed using the CMAQ approach (Byun, 1999). Because of the offline character of the coupling, no feedback between RegCM's radiation scheme and chemistry is considered. Based on 10-year-long simulations, Huszar et al. (2016b) concluded that the radiative effects of urban pollutant emissions and secondarily formed pollutants are minor, which justified the offline coupling (which is not true for short-term effects that can be considerable; for example, Prieto Perez et al., 2022). On the other hand, all urban effects on meteorology, i.e. urban heat island or moisture island (and others, like increased vertical turbulence, lower over wind speeds over cities), are included in the meteorological data that are fed offline into CAMx, thus having a direct impact on chemistry in our simulations.
2.2 Model setup and data
Model simulations with RegCM and CAMx were conducted over a 9 km × 9 km resolution domain covering “larger” central Europe (from France to Ukraine and from northern Italy to Denmark) with 189 × 165 grid boxes, centred over the Czech capital, Prague (50.075∘ N, 14.44∘ E; Lambert conic conformal projection). In the vertical, the model grid spans 40 layers in RegCM up to 5 hPa, while CAMx uses the lowermost 18 layers up to about 12 km. The simulated years include December 2014 to December 2016, with the first month serving as the spin-up. Fenech et al. (2018) showed that the difference between the coarse- and fine-resolution PM2.5 concentrations is rather small, and our resolution is comparable to the resolution of the emissions (see below). The requirement of Tie et al. (2010) that the size of the city to resolution should be 6:1 means that preferably, a resolution of 6 km or finer should be used; however we rely on the fact that land use is represented as fractional land use, so even the smallest cities are resolved within the surface model in our RCM. Markakis et al. (2015) showed that the modelled PM2.5 concentrations for Paris are more sensitive to the emissions inventory's resolution than to the resolution at which the meteorology is resolved in the driving RCM.
RegCM simulations are forced with the ERA-Interim reanalysis (Simmons et al., 2010). Chemical boundary conditions are taken from the CAM-chem global climate-chemistry model (Buchholz et al., 2019; Emmons et al., 2020). Land use for RegCM and for the CAMx dry-deposition calculations is based on the 100 m resolution CORINE Land Cover (CLC 2012) data (https://land.copernicus.eu/pan-european/corine-land-cover, last access: 16 May 2023) except a small area over Belarus, where CORINE is not available, so the United States Geological Survey (USGS) data are used.
Anthropogenic emissions are taken from the European CAMS (Copernicus Atmosphere Monitoring Service) version CAMS-REG-APv1.1 inventory (Regional Atmospheric Pollutants; Granier et al., 2019) for the year 2015 combined with the Czech national emission data, the Register of Emissions and Air Pollution Sources (REZZO) dataset issued by the Czech Hydrometeorological Institute (https://www.chmi.cz/, last access: 16 May 2023) and the ATEM Traffic Emissions dataset provided by ATEM (Ateliér ekologických modelů – Studio of ecological models; https://www.atem.cz/, last access: 16 May 2023). These annual sector-based emission totals are decomposed to hourly speciated emissions fluxes using the Flexible Universal Processor for Modeling Emissions (FUME) emission model (http://fume-ep.org/, last access: 9 January 2024; Benešová et al., 2018) using the speciation and time-disaggregation factors of Passant (2002) and van der Gon et al. (2011). To account for the SOA formation from intermediate VOCs (IVOCs) which are normally not included in emission inventories, we proceeded following Ciarelli et al. (2017); Giani et al. (2019) to calculate IVOCs based on the known non-methane VOC and POA (primary organic aerosol) emissions from gasoline and diesel vehicles and emissions from biomass burning. This emission adjustment was not implemented in Huszar et al. (2022), but there we looked at gas-phase chemistry impacts only. It has been shown that major contributors to urban SOA precursors in the urban environment are asphalt-based vehicular emissions (Khare et al., 2020). These are strongly dependent on solar radiation and temperature, and the currently used anthropogenic emission model does not include them. Thus our estimates of the impact of urban emissions to SOA might be somehow underestimated, especially during hot sunny days (by not including these emissions, the winter error will, however, probably be much smaller). On the other hand, road dust re-suspension (Rienda and Alves, 2021) is partly included in our study, as the Czech ATEM traffic emissions consider these types of emissions. They are however not included in the European CAMS emissions; thus their effect is only included for Prague, implying further potential underestimation of the urbanization impact on total fine PM loads.
Biogenic emissions are computed offline using MEGANv2.1 (Model of Emissions of Gases and Aerosols from Nature version 2.1) with the algorithm described by Guenther et al. (2012) driven by RegCM meteorological fields (short-wave radiation, temperature, soil moisture, humidity). The necessary inputs for MEGAN were not part of the CORINE land-use information and were compiled based on Lawrence and Chase (2007) and Sindelarova et al. (2014, 2022). These include leaf area index (LAI) data (weekly data), plant functional types (PFT), and emission potentials of different plant types. Besides BVOCs, MEGAN also calculates the fluxes of soil-biogenic NO (nitrogen monoxide) emissions from bacterial activity (Yienger and Levy, 1995). As these emissions are a function of LAI and meteorological conditions, a fraction of the “DBVOC” impact will be composed of soil-NOx emissions modifications. Not presented here, in our experiments the soil-NOx emissions were about 2 orders of magnitude smaller compared to the BVOC emissions, and their effect is expected to be much smaller, including the effect of their urbanization-induced modulations. BVOC emission fluxes are strongly temperature dependent; i.e. higher temperatures result in enhanced emissions. In this regard, it is expected that urbanization-induced temperature increase will lead to higher BVOC fluxes. It has to be noted that PFT data are fractional, meaning that even the smallest fraction of a particular plant type is represented in the BVOC emissions, which allows as to account for the very small but not zero amount of urban vegetation. On the other hand, the PFT input is relatively old (year 2007), and some inaccuracies might be present in the vegetation fraction given the urban development that has taken place for the chosen cities since 2007.
For the purpose of the study, we had to isolate the emissions originating from selected urban areas from rural emissions. To achieve this, we used the masking capability of the emissions model used (FUME), while we used the administrative boundaries of the chosen cities based on the GADM public database (https://gadm.org, last access: 16 May 2023) which provide geographic shape files of these boundaries. Cities selected for the analysis are Berlin, Brussels, Budapest, Cluj-Napoca, Cologne, Frankfurt, Hamburg, Kraków, Lodz, Lyon, Milan, Munich, Prague, Turin, Vienna, Warsaw, Wroclaw, Zagreb, and Zurich. The choice considered the same criteria as in Huszar et al. (2021, 2022): the diameter of the city larger than 9 km (the grid cell size in our model); minimal orographic variability to reduce orographic effects (see, for example, Ganbat et al., 2015); sufficiently large distance between cities eliminating mutual influences; and, finally, no coastal cities to eliminate the effect of asymmetric land-use effects, like the sea-breeze effect (e.g. Ribeiro et al., 2018). Despite strict emission control policies, these cities are still often burdened with high air pollution due to PM (Khomenko et al., 2021; Sokhi et al., 2022; Balamurugan et al., 2022; Putaud et al., 2023).
The mutual interaction between the regional climate and chemistry transport model, as well as the emissions models (for biogenic and anthropogenic emissions), is depicted in Fig. 1. It shows as detailed above that meteorological conditions generated by the regional climate model (RegCM) drive both the biogenic emissions model (MEGAN) and the chemistry transport model (CAMx). The emissions fluxes calculated as the sum of anthropogenic and biogenic emissions are then fed into CAMx.
2.3 Model simulations
In a similar fashion to that in Huszar et al. (2022), we decomposed the total impact of urbanization (RUT) into the individual impacts or contributors (i.e. “DEMIS”, “DMET”, “DLU_D”, “DBOC”) listed in the Introduction. This required us to carry out a series of model simulations with each contributor added gradually one by one to the reference simulation to achieve the full urbanization case.
First, we carried out a pair of model simulations with RegCM, “Urban” and “Nourban”, with the former accounting for urban land surface treated with RegCM's urban canopy module and the latter accounting for land use being replaced by “crops” as the most common rural land-use type in the region analysed. We performed five simulations with CAMx that differ in the in-/exclusion of urbanized land surface, UCMF (acting on both atmospheric chemistry in general and on biogenic emissions), and urban emissions. These simulations are summarized in Table 1. The first simulation called “ENNNN” represents the hypothetical reference (background) state without urban emissions and with the urban land surface replaced by rural land surface in RegCM and CAMx, as well as in the BVOC calculations (with MEGAN). In the second experiment, “ENYNN”, the urban emissions are turned on. In the third experiment, “ENYUN”, the urban land use was “switched on” for the dry-deposition scheme in CAMx. In the fourth experiment, “ENYUU”, urban land use and UCMF (i.e. “Urban” meteorology) are accounted for in the biogenic emissions model, and finally, in the fifth experiment, “EUYUU”, all the urbanization-related effects are considered, representing the most realistic full case.
Table 1The list of CAMx simulations performed with the information of the effects considered. “Urban” and “Nourban” denote the urban land surface treated with RegCM's urban canopy module and the land use being replaced by “crops” as the most common rural land-use type, respectively.
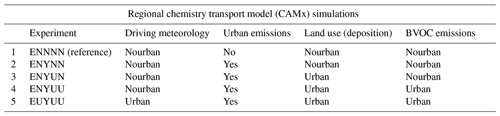
In the first experiment where urban emissions are disregarded, we only removed urban emissions for the 19 cities selected. For the effect of rural-to-urban land-use transformation on meteorological conditions, dry deposition, and biogenic emissions, we replaced the urban land by rural land over the entire domain (i.e. not only for the cities selected). It is clear that this has an effect on the background level of air pollutants and not only on local urban levels, but the effect is probably much smaller than local effects as (1) emissions from these areas were still considered, and (2) the urban meteorological effects from these (minor) urban areas have a rather small influence on air pollutants as UCMF over them is also small (see, for example, Huszar et al., 2014).
Similar to Huszar et al. (2022), we can mathematically express the concentrations ci of a pollutant i in a selected city with respect to RUT in the following way:
where ci,rural is the reference (background) concentration before RUT, and Δci,RUT is the total impact of urbanization.
In this study, we are concerned about the contributors to Δci,RUT (regardless of their sign), i.e.
where Δci,EMIS, Δci,MET, , and Δci,BVOC are the impacts of urban emissions, the impact of the urban canopy meteorological forcing, the impact of modified land use on dry deposition, and the impact of modifications of BVOC emissions, denoted above as “DEMIS”, “DMET”, “DLU_D”, and “DBVOC”.
These impacts will be calculated from the experiments listed in Table 1 as indicated below (the experiment number is shown in parentheses):
It has to be realized that, in fact, the contributors above act simultaneously, and feedbacks are present between them, so their impacts are not additive. The way that we calculated them however allows us to consider them to be additive, meaning that their sum is the total impact of urbanization. This is also a consequence of Eq. (3). Also, the impact of the order of application of the four contributors has to be discussed. The choice of starting with the “DEMIS” was motivated by “filling” the atmosphere with pollutants before any other contributor is “turned on”. We assumed with this choice that the other contributors act on a more realistic base state (see the “Discussion and conclusions” section for more details).
Our analysis will focus on near-surface PM2.5 concentrations as well as their secondary components, i.e. secondary inorganic aerosol (SIA), composed of sulfates (PSO4), nitrates (PNO3), and ammonium (PNH3), and secondary organic aerosol. Moreover, we will also focus on primary elemental carbon (PEC), which is an important fraction of urban emission loads. As the emissions of primary organic aerosol have very similar magnitude in our emission data compared to PEC, and it has the same deposition velocity (which is determined only by size), we will not explicitly analyse POA concentrations as we assume that the impacts of urbanization on POA will be very similar to the impact on PEC.
This section presents the results, and only a brief, rather general discussion is provided. A more detailed interpretation of the results is given in the “Discussion and conclusions” section, including a comparison with existing studies.
3.1 Validation
Here we compare the modelled concentrations of PM2.5 and their components (PSO4, PNO3, PNH4, SOA, and PEC) to observations. The measured data for PM2.5 are taken from the AirBase European air quality data (http://www.eea.europa.eu/data-and-maps/data/aqereporting-1, last access: 16 May 2023), while for PM components, data are taken from the EBAS database (https://ebas-data.nilu.no/, last access: 16 May 2023) from EMEP background sites. AirBase data are taken from all available rural and urban background stations in order to distinguish between model performances above both type of stations.
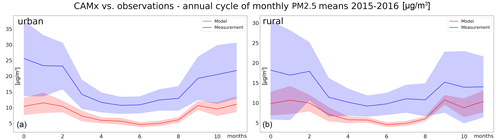
Figure 2Comparison of modelled (red) and observed (blue) PM2.5 monthly concentrations over urban (a) and rural (b) AirBase stations shown as a 2015–2016 mean. Shaded areas represent the standard deviation across all the stations. Units are µg m−3.
Figure 2 shows the average annual cycle of monthly means for urban and rural stations including the corresponding model values. Over urban stations, CAMx exhibits a strong underestimation around 5–10 µg m−3, and the underestimation is stronger in winter. CAMx performs slightly better over rural stations, with a smaller negative bias. In both cases, the annual cycle is reasonably captured. We also compared the analysed components (sulfates, nitrates, ammonium, and elemental carbon) with measurements. In this case the EMEP background station data acquired from EBAS (https://ebas.nilu.no/data-access/, last access: 5 May 2023) were used. Figure 3 shows that the model underestimates PSO4 by about 0.5–1 µg m−3, especially during summer when the model predicts minimum values, while in measurements, the values show a more or less uniform distribution during the year. PNO3 is overestimated during winter by about 2 µg m−3, and the model matches the summer values well. In the case of PNH4, an 0.5 µg m−3 overestimation of winter values and a similar underestimation of summer values are encountered. Thus, for both PNO3 and PNH4, the amplitude of the annual cycle is overestimated. For PEC, the match is very satisfactory, with a uniform model bias of −0.25 µg m−3 throughout the year, meaning that the annual cycle is very well captured by the model. The presented underestimation of fine PM is caused most probably by underestimation of the organic aerosol fraction, which is an important component of the urban PM. The strong underestimation of sulfates may be connected to overestimation of other inorganic aerosol components; for example, overestimated nitrates can consume the available ammonia and suppress the formation of PSO4. See the “Discussion and conclusions” section for more details.
3.2 The impact of individual contributors to RUT
Here we present the total impact of RUT as well as its individual contributors on PM2.5 concentrations as 2015–2016 DJF and JJA averages, averaged across the selected cities. Values from model grid boxes that cover the city centres are selected. The results are shown as box plots in Fig. 4; they show the first and third quartiles and the median values, along with the minimum and maximum values across all the cities.
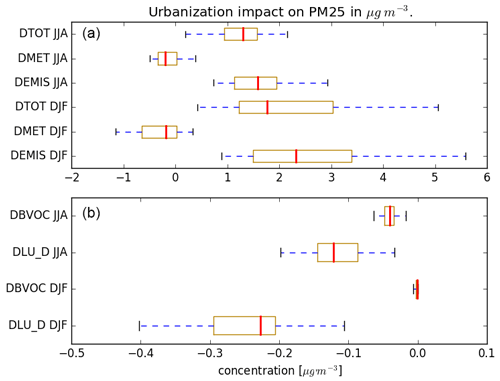
Figure 4The 2015–2016 DJF and JJA averaged total impact of urbanization as well as of each contributor to the urban concentrations of PM2.5 averaged over all chosen city. The box plots show the 25 % to 75 % quantiles including the minimum and maximum value across all cities. The red line shows the median value. Values are taken from model grid cell that covers the city centre. Panel (a) shows the two main contributors including the total impact (“DEMIS”, “DMET” and “DTOT”), and panel (b) shows the minor contributors (“DLU_D” and “DBVOC”). Units are µg m−3.
As expected, the highest impact is attributed to the effect of emission only causing an increase in urban concentrations by around 1.5 to 3.4 µg m−3 in DJF and by about 1.2 to 2 µg m−3 in JJA. The effect of UCMF on concentration is usually a decrease up to −1 and −0.4 µg m−3 in winter and summer, respectively. The impact of minor contributors associated with modified land use and deposition velocities and modified BVOC emissions is a decrease in “DLU_C” for both seasons by −0.2 to −0.3 µg m−3 in DJF and −0.08 to −0.15 µg m−3 in JJA, while the effect of BVOC modifications is very small, around −0.05 µg m−3 in summer and almost 0 in DJF. The total impact of urbanization on PM2.5 is a 1.2 to 3 µg m−3 increase in DJF and a smaller increase from 1 to 1.6 µg m−3 in JJA, while, of course, the impact of emissions dominates.
To examine the contribution of the most important aerosol components to these changes, we plotted a similar figure as above but individually for PSO4, PNO3, PNH4, PEC and SOA presented in Fig. 5. Sulfates respond to urban emissions alone by an increase of about 0.05–0.15 µg m−3 and up to 0.05 µg m−3 in DJF and JJA, respectively. The urban canopy meteorological forcing results in a decrease in sulfates in DJF by up to −0.05 µg m−3, while increases are modelled for JJA up to 0.025 µg m−3. The impact of dry-deposition change on PSO4 is a decrease: up to −0.025 µg m−3 in DJF and −0.01 µg m−3 in JJA. The BVOC effect on PSO4 is a slight increase in JJA and virtually 0 in DJF. The total impact of RUT on PSO4 is an increase up to 0.15 and 0.05 µg m−3 in DJF and JJA, respectively.
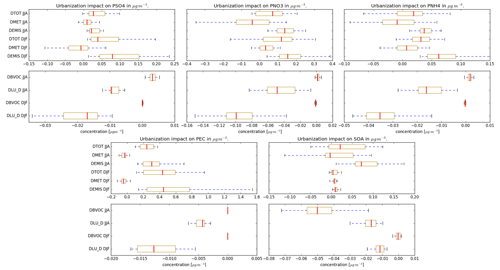
Figure 5Same as Fig. 4 but for PM2.5 components: PSO4, PNO3, and PNH4 (upper row) and PEC and SOA (lower row).
In the case of nitrates, the urban emissions alone increase urban concentrations by about 0.1 to 0.2 µg m−3 in both seasons (somewhat more in DJF). The effect of UCMF is an increase in DJF by about 0.05 µg m−3, while in JJA, decreases dominate up to −0.15 µg m−3. The impact of modified dry deposition is a decrease by about −0.08 to −0.11 µg m−3 in DJF and −0.03 to −0.06 µg m−3 in JJA. The impact of modified BVOC emissions is negligible, and the total impact of RUT on PNO3 is an increase up to about 0.16 and 0.12 µg m−3 in DJF and JJA, respectively.
For ammonium, urban emissions cause an increase by 0.04 to 0.09 µg m−3 and by 0.04 to 0.05 µg m−3 in DJF and JJA, respectively. The sign of the UCMF impact can be positive and negative in both seasons, with values between −0.01 and 0.025 µg m−3 in DJF and between −0.03 and 0.02 µg m−3 in JJA. The impact of dry-deposition modifications is negative in both seasons, with −0.03 to −0.04 µg m−3 and −0.01 to −0.02 µg m−3 decreases in winter and summer, respectively. The impact of modified BVOC emissions is again negligible. Finally, the total impact of RUT on PNH4 is an increase up to about 0.04 and 0.03 µg m−3 in DJF and JJA, respectively.
The impact on elemental carbon which is a primary component chemically inert in CAMx (with no chemical decay or reactions) is as follows: urban emissions cause an increase in PEC by around 0.2 to 0.5 µg m−3 in DJF and around 0.2 to 0.4 µg m−3 in JJA. The UCMF causes a slight decrease in PEC by around −0.05 to −0.1 µg m−3 in both seasons. The increased deposition velocities caused decreased concentrations of PEC by about −0.01 to −0.015 µg m−3 in DJF and around −0.005 µg m−3 in JJA. Being an inert PM component in CAMx, no impacts of BVOC modifications on PEC are modelled. The total impact of RUT on PEC is again dominated by urban emissions and reached 0.6 and 0.3 µg m−3 in DJF and JJA, respectively.
Finally, the impact secondary organic aerosol only has considerable values during JJA, when the oxidation of primary VOCs to semivolatile precursors of SOA dominantly takes place. The impact of urban emissions is an increase in SOA by up to 0.05 to 0.1 µg m−3, while urban meteorological changes cause SOA modifications usually between 0 and 0.05 µg m−3. Due to land-use modifications and associated deposition velocity increases, SOA responds with a decrease up to −0.02 µg m−3, while due to urbanization-induced BVOC modifications, SOA decreases by around −0.04 to −0.06 µg m−3. The total impact of RUT on SOA is an increase by 0.07 µg m−3 in JJA and a very tiny increase by up to 0.01 µg m−3 in DJF.
These results confirm that urban PM2.5 levels are a result of mainly the local input represented by urban emissions but get smaller if the urban meteorological characteristics are taken into account (in the form of UCMF). It is also seen that the urban land surface exhibits a stronger depositional sink to PM, causing the land-use impact to be negative, and finally, the urbanization-induced decrease in BVOC emissions leads, as expected, to suppressed SOA formation, negatively contributing to the overall RUT impact.
3.3 The spatial distribution of the impacts
To obtain a spatially resolved information about the impact of individual contributors to RUT, here we plot their 2-D distribution as DJF and JJA averages. We start with presenting the distribution of absolute modelled concentrations for comparison of the changes with absolute values in order to resolve the relative magnitude of these contributors.
Figure 6 shows the absolute modelled near-surface concentrations of DJF and JJA PM2.5 and its analysed components from the experiment with all urban effects considered (“EUYUU”). The PM2.5 concentrations reach 30–40 µg m−3 in winter, while rural areas are often over 8 µg m−3. In summer, concentrations are, as expected, smaller, reaching 10 µg m−3 being above 4 µg m−3 over rural areas. In DJF, the highest contribution is made by nitrates, reaching 20 µg m−3 over northern Italy and being about 4–6 µg m−3 over central Europe. The concentrations of sulfates are large, especially over Poland, reaching 2–3 µg m−3, while ammonium is largest over northern Italy, reaching 4–6 µg m−3, while other areas exhibit concentrations around 1–2 µg m−3. Elemental carbon contributes to total PM2.5 by values around 2–4 µg m−3 over northern Italy, while the contribution is clearly limited to urban areas over other regions within the domain (e.g. 1–2 µg m−3 over urban areas in eastern Europe). The SOA concentrations in JJA are usually between 0.2–1 µg m−3, reaching maxima again over Italy (2–3 µg m−3). In summer, the secondary inorganic aerosol concentrations are somewhat smaller, especially for ammonium (less than 0.6 µg m−3), while nitrates are largest over western Europe, reaching 2–3 µg m−3, and sulfates are largest over southern Europe, also reaching around 2–3 µg m−3. The PEC concentrations in JJA are small, usually around 0.1 to 0.4 µg m−3. SOA is larger in summer than in winter, reaching concentrations up to 2 µg m−3 and usually being around 0.4–1.5 µg m−3.
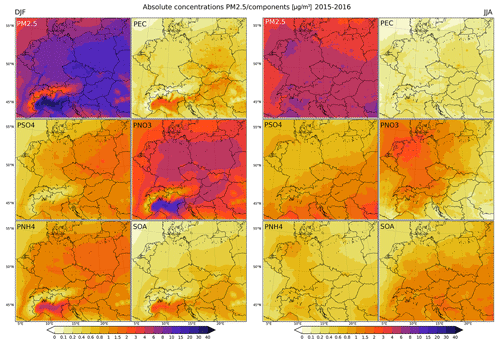
Figure 6The absolute DJF (left panel) and JJA (right panel) concentrations of modelled PM2.5 and its components (PEC, PSO4, PNO3, PNH4, and SOA) from the “EUYUU” experiment (all urban effects considered) averaged over 2015–2016 in µg m−3.
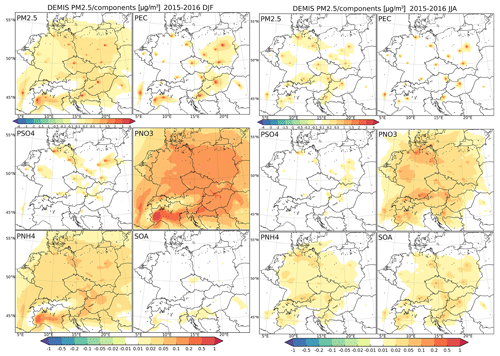
Figure 7The spatial distribution of the 2015–2016 DJF (left panel) and JJA (right panel) average urban emission impact “DEMIS” on PM2.5 and its components. Units are µg m−3. Note that PM2.5 has a separate colour bar.
3.3.1 The impact of urban emissions (DEMIS)
In Fig. 7 the DJF and JJA average spatial impact of urban emissions (“DEMIS”) on the near-surface concentrations of PM2.5 and its five analysed components is presented. Urban emission impact is in general higher in winter expect for SOA. In DJF, PM2.5 is increased over urban areas by up to 4 µg m−3, and the contribution to rural concentrations can also reach 0.5 µg m−3. In JJA, urban emissions contribute to total PM2.5 by about 1–3 µg m−3 over cities, while the rural contribution is small, reaching 0.02 µg m−3. The impact of urban emissions on PEC reaches 1 and 0.5 µg m−3 in city centres in DJF and JJA, respectively, while the impact over rural areas is less than 0.02 µg m−3. For sulfates, urban emissions impact urban concentrations up to 0.5 µg m−3 in winter, while the summer impact is smaller, reaching 0.1 µg m−3. The impact over rural areas is again less than 0.02 µg m−3 in both seasons. A much larger impact is modelled for nitrates, reaching 1 µg m−3 over Italy and exceeding 0.2 over most of central Europe in winter. In summer, the impact on PNO3 is smaller, reaching 0.5 µg m−3 over urban and 0.2 µg m−3 over rural areas. The impact of urban emissions on ammonium reaches 0.5 µg m−3 and is usually around 0.05 µg m−3 in DJF, while JJA concentrations are smaller, reaching 0.05 but usually less than 0.02 µg m−3. Finally, the impact on SOA is negligible in winter reaching 0.02 µg m−3 over city centres, while in summer, it can reach 0.2 µg m−3, and the contributions over rural areas can exceed 0.02 µg m−3. These result clearly show that emissions mainly act locally, but a large fraction of urban pollution is also caused by rural emissions or emissions from nearby cities. Further, it is clear that the impact on primary pollutants is more localized than the one on secondary components which are formed during ageing of the urban aerosol plume, impacting distant areas.
3.3.2 The impact of modified meteorological conditions (DMET)
In Fig. 8 the DJF and JJA average spatial impact of the urban canopy meteorological forcing (“DMET”) is presented. For PM2.5, it is characterized by decreases located above urban areas, reaching −2 µg m−3 in both seasons. Elsewhere, i.e. above rural areas, PM2.5 increases by up to 1–1.5 µg m−3. In the case of PEC, the decrease over urban areas is evident and reaches −0.2 µg m−3, especially during DJF, while some minor increases are modelled over rural land, reaching 0.05 µg m−3. The impact on secondary aerosol components is more complicated as apart from the direct impact, UCMF also impacts their precursors. Sulfates decrease above urban areas by about 0.2 to 0.5 µg m−3 in DJF and by 0.1 µg m−3 in JJA. Large rural regions show, on the other hand, increases of PSO4 by up to 0.1–0.2 µg m−3, mainly in winter. In the case of nitrates, some urban areas exhibit decreases in DJF (e.g. Berlin, the Ruhr area), but large increases are also modelled over rural areas and even urban ones, especially over northern Italy along the Po River, reaching 0.5 µg m−3. In summer, the decrease over urban areas is seen for most of the cities; however, over other areas, a strong increase inPNO3 is modelled reaching 0.5 µg m−3. The UCMF's impact on PNH4 is somewhat smaller and is, again, characterized by decreases above cities up to −0.2 µg m−3 in both seasons, while increases are modelled over rural areas and also some urban ones, reaching 0.3 µg m−3 in winter and 0.2 µg m−3 in summer. In the case of SOA, some urban areas over western and southern Europe exhibit decreases in JJA up to −0.05 µg m−3, but increases dominate, reaching 0.2 µg m−3. In DJF, the impact is very small, with a minor increase over rural areas up to 0.05 µg m−3. The simulated impact of UCMF on fine PM is most probably the result of enhanced vertical eddy diffusion caused by the urban canopy. This leads to decreases in PM concentrations by transporting material into higher levels. However, this can lead to elevated concentrations further from the sources (cities) when this material is deposited back to lower model levels.
3.3.3 The impact of dry-deposition modifications (DLUC_D)
Figure 9 depicts the DJF and JJA average spatial impact of the modified dry-deposition velocities due to urban land use. In both seasons, a clear decrease in concentrations is modelled, indicating that dry-deposition velocities increased, as was expected. The total PM2.5 concentrations decreased by up to −1.5 µg m−3 in winter over cities, while large rural areas exhibit a decrease up to −0.5 µg m−3. In summer, the decreases have a smaller magnitude, reaching −0.5 µg m−3 over urban areas, while over rural ones they reach −0.2 µg m−3. For PEC, decreases are limited mostly to urban areas, reaching −0.05 µg m−3 in DJF and −0.01 µg m−3 in JJA. Larger impacts are modelled for secondary aerosol, probably due to the fact that their precursors are also impacted. Sulfates decreased in winter by about 0.05 µg m−3 and by about 0.02 µg m−3 in summer, mainly over urban areas. Among SIA, the largest impacts are modelled for nitrates, exceeding −0.1 µg m−3 decrease in DJF over northern Italy but being large over rural areas too (about −0.05 µg m−3). In summer, PNO3 decreases by around 0.02 to 0.05 µg m−3, mainly over cities. In the case of PNH4 the decreases are, again, largest above cities, reaching −0.05 µg m−3 in both seasons (slightly stronger decrease in DJF). Over rural areas, the decrease is about −0.02 µg m−3 and −0.01 µg m−3 in DJF and JJA, respectively. SOA decreased due to modified dry-deposition velocities by around 0.02 µg m−3 above cities in both seasons, while over rural areas, it reaches about −0.01 µg m−3, slightly higher in JJA.
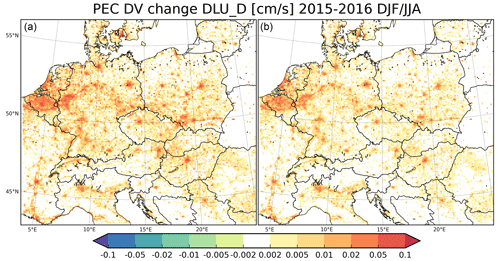
Figure 10The spatial distribution of the 2015–2016 DJF (a) and JJA (b) average impact of urban land use on dry-deposition velocities for PEC. Units are cm s−1.
The decreases above are the result of increased deposition velocities, which are depicted in Fig. 10 for PEC for both winter and summer. As the DVs in the model used (CAMx) are only a function of the size, all aerosols within the 0–2.5 μ size range (where all the secondary aerosols belong) have the same DV values, and here we only present the modification of DV for this component (for others, the figure would be the same). DVs increased clearly above urban areas, while the increase reaches 0.1 cm s−1 in DJF for some cities. For JJA, the increases are slightly smaller, usually between 0.02 and 0.1 cm s−1. Is has to be noted here that the deposition model used considered spatially uniform surface parameters relevant for deposition; however, cities are covered by a very high variety of different materials and the deposition velocities can vary from place to place with a great magnitude, so the results are rather a rough estimate.
3.3.4 The impact of biogenic emissions (DBVOC)
Figure 11 presents the impact of modified biogenic emissions due to RUT on PM concentrations. It is clear that BVOC emissions are mainly important during the warm season, and that is why the impacts during DJF are much smaller than during JJA. Moreover, during summer, BVOCs can more readily oxidize to semi-volatile hydrocarbons forming SOA, so the impact on PM2.5 acts predominantly via impacting secondary organics concentrations. However due to feedback on the overall gas-phase chemistry and thus SIA precursors, SIAs are also slightly modified. In winter the impact on PM2.5 is only considerable above northern Italy reaching −0.05 µg m−3, while SOA and nitrates mainly contribute to these PM modifications. In the case of PNO3, they decrease above the same region by around 0.01–0.02 µg m−3, while SOA decreased by a similar magnitude (and slightly increased over other areas). Sulfates responded to BVOC changes by a slight decrease up to −0.01 µg m−3. In JJA, the impacts are in general much larger (as expected) and are mainly determined by the decreased SOA but also modulated by increases in SIA. The PM2.5 JJA decrease reaches −0.1 µg m−3 (again mainly over northern Italy) but is between −0.02 to −0.05 µg m−3 over large areas all over the domain. Regarding SIAs, all of them increased: by up to 0.02 µg m−3 for PNO3 and up to 0.01 µg m−3 in the case of PSO4 and PNH4. For SOA there is a clear decrease during JJA up to −0.1 µg m−3 over northern Italy and between −0.02 and −0.05 µg m−3 over large regions across the domain. As PEC is not affected by either gas-phase or aerosol chemistry, no modifications due to biogenic emission changes are modelled.
3.4 The diurnal variation of the impacts
Human activities change during the day, causing a typical diurnal cycle of urban emissions. Moreover, the urban canopy meteorological forcing also has a distinct diurnal pattern; for example, the modification of temperature is strongest during the night, and the impacts on wind and turbulence are the strongest during daytime (Huszar et al., 2018a). It is thus expected that the individual contributors to the total impact of RUT analysed here will have also a distinct diurnal cycle.
In Figs. 12 and 13 we present the diurnal cycles for the four contributors' impact on PM2.5 and its components during winter and summer averaged over all urban centres (we took the model grid box covering the city centre, in a similar way to that shown in Fig 4). In the case of PM2.5 in winter, “DEMIS” causes a typical diurnal variation, resembling the diurnal cycle of urban emissions (varying between 1.5 and 4 µg m−3); this is also seen for PEC, when maxima occur during morning and evening rush hours. A similar diurnal pattern is also seen for sulfates varying between 0.1 and 0.2 µg m−3. For other secondary aerosol components the diurnal cycles are characterized by only one maximum: for nitrates, the maximum occurs around noon; reaching 0.25 µg m−3, while for ammonium, the maximum emission impact is reached during morning reaching 0.1 µg m−3. SOAs are increased due to emission at most during early afternoon by up to 0.015 µg m−3. In the case of the impact of UCMF (“DMET”), it is usually negative for PM2.5 being lowest during the afternoon when it reaches around −1 µg m−3. For PEC and PSO4, the maximum decrease is about −0.2 and −0.1 µg m−3, respectively. For PNO3 and PNH4 and SOA, it is again negative during afternoon hours reaching −0.2, −0.07 and −0.02 µg m−3, respectively. The impact of increased deposition velocities is negative in all cases and throughout the whole day in winter. However, the diurnal patterns indicate that the maximum decrease is modelled for early afternoon hours, reaching −0.3 µg m−3 for PM2.5. For PEC, PSO4, PNO3, and PNH4, it reaches −0.02, −0.02, −0.01, and −0.04 µg m−3, respectively. For SOA, the maximum decrease reaches −0.016 µg m−3. As expected, the impact of modified BVOC emissions is almost negligible with weak maximum decrease during afternoon and evening hours for SOA (around −0.002 µg m−3).
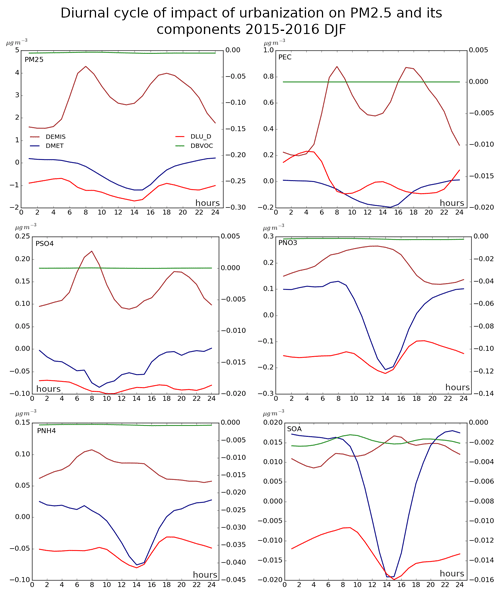
Figure 12Diurnal cycles of the impact of individual contributors to RUT averaged over 2015–2016 DJF for PM2.5, PEC, PSO4, PNO3, PNH4, and SOA. Colours are as follows: brown – DEMIS, blue – DMET, red – DLU_D, and green – DBVOC. The left y axis is for the two major contributors, DEMIS and DMET, while the right y axis belongs to the two smaller contributors, DLU_D and DBVOC. Units are µg m−3.
The JJA diurnal cycles of the impacts are similar to DJF in the case of “DEMIS”, with two maxima for PM2.5, PEC, and PSO4, while a single maximum due to emissions is modelled for the other components. For the “DMET”, again an early evening decrease is modelled, reaching −0.5 and −0.1 µg m−3 for PM2.5 and PEC. The impact on PSO4 is very small, reaching −0.01 µg m−3, while for PNO3, PNH4, and SOA, the maximum decrease is about −0.15, −0.04, and −0.05 µg m−3, respectively. The summer “DLU_D” impact on PM2.5 and its components has a distinct cycle compared to DJF, usually with a morning maximum decrease. This reaches −0.16 µg m−3 for PM2.5. It is also very small for PEC. For PSO4, PNO3, PNH4, and SOA, it reaches −0.02, −0.15, −0.03, and −0.025 µg m−3, respectively. In contrast to DJF, the impact of biogenic emissions changes due to urbanization shows a clear diurnal cycle for all PM components except PEC, which does not interact with gas-phase species. For PM2.5, concentrations decrease, and this decrease is at its maximum during evening hours, reaching −0.08 µg m−3. For PSO4, increases are modelled, reaching their maximum around noon (0.005 µg m−3), while for PNO3 and PNH4, an increase is modelled too, but during the afternoon there is a slight decrease in nitrate concentrations. In the case of SOA, a strong decrease is modelled during evening hours reaching −0.075 µg m−3, which clearly determines the overall cycle for total PM2.5. For other hours, the decrease in SOA in JJA is around −0.05 µg m−3.
The presented diurnal variations are in close relation to diurnal pattern of emissions, both anthropogenic (in the case of “DEMIS”) and biogenic (in the case of “DBVOC”), and also to the diurnal cycle of UCMF (in the case of “DMET”). While for emissions, these are more or less well known (maximum during daytime for BVOCs and two daytime maxima in the case of anthropogenic emissions), for UCMF the underlying causes are hidden in the diurnal pattern of individual components in UCMF (temperature, wind, planetary boundary layer height, turbulence, etc.), and these have been modelled and well described in our previous studies (Huszar et al., 2018a, b, 2020a). However, the diurnal pattern of deposition velocities for PM and their urbanization-induced modifications have not yet been evaluated. Therefore, here, to accompany the diurnal variation of concentrations, we plot the diurnal variation of the changes of DV due to the urban land surface as well as the absolute values (corresponding to a non-urbanized land surface). We chose PEC as a representative PM component (note that DVs are only a function of PM size, and we only consider fine aerosol in this study). The results are depicted in Fig. 14. The absolute DVs range between 0.8 and 1.2 mms−1 and 0.8 and 1.5 mms−1 in DJF and JJA, respectively. The maximum values are reached around noon. The changes due to the introduction of an urban land surface follow a very similar pattern, with the highest impact around noon, reaching 0.8 and 0.5 mm s−1 in DJF and JJA, respectively, and being about 0.5–0.6 and 0.3 mm s−1 during night-time for both seasons.
In this study, an analysis of the different contributors to the overall impact of urbanization (called rural-to-urban transformation, RUT) on fine particulate matter concentrations over central Europe was presented. It focused on the four most important contributors to RUT: the impact of urban emissions only (“DEMIS”), the impact of UCMF (urban canopy meteorological forcing) on PM transport and chemistry (“DMET”), the impact of modified dry-deposition velocities due to urbanized land cover (“DLU_D”), and the impact of modified biogenic emissions due to modified land cover (and associated vegetation change) and modified meteorological conditions (“DBVOC”). They were quantified by performing a set of model simulations whereby each of the contributors was added one by one, starting with the reference state, corresponding to non-urbanized land surface with no urban emissions.
The model biases identified for PM2.5 show that some of the PM2.5 components are strongly underestimated in CAMx (Fig. 2). Very similar underestimation was encountered previously by Huszar et al. (2021) for selected European cities, using the same resolution and same emission data (they used, however, an older version of the models). Ďoubalová et al. (2020), also using CAMx, reported a comparable negative bias. From the analysed aerosol components, sulfates were underestimated, but this, along with the model performance for nitrates and ammonia (Fig. 3), does not explain the strong negative bias for PM2.5. Most probably, the organic aerosol fraction was strongly underestimated in our model, which is a general problem in CTMs and has been encountered previously by many authors (Jiang et al., 2019; Ciarelli et al., 2017), while, at the same time, it is often considered the largest fraction of fine particulates in urban environments (Allan et al., 2010; Lanz et al., 2010). It is also probable that the bias is partly caused by the fact that the local wind-blown dust sources are not considered in our emissions model, while this dust source can significantly contribute to overall PM2.5 over central Europe, as shown recently by Liaskoni et al. (2023). The modelled negative bias for sulfates is similar to Bartik el al. (2021), who applied CAMx over a similar European domain using slightly newer emission data. Sulfates were moreover underestimated over Europe by the majority of the models used in the second phase of the Air Quality Model Evaluation International Initiative (AQMEII; Im et al., 2015) and can be connected to overestimation of other secondary inorganic components; i.e. ammonium preferably neutralizes nitrates instead of sulfates, leading to less ammonium sulfate formation (Im et al., 2015).
Due to the overall underestimated PM2.5 concentrations, it is expected that the impacts presented here for PM2.5 are underestimated too. This is true, especially for the impact of emissions, but as the effect of UCMF and deposition velocity change is also proportional to the concentrations they act on, the impacts presented below are underestimated most probably in these cases too.
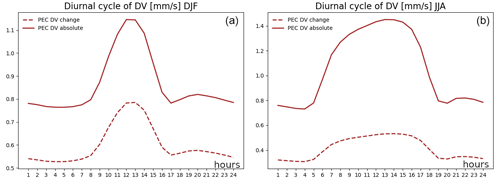
Figure 14Diurnal cycle of the DJF (a) and JJA (b) DV of PEC: solid lines denote absolute values, and dashed lines mean the change caused by the urban land surface. Units are mms−1.
The total impact of urbanization on PM2.5 over central European cities was calculated to be 1–1.5 µg m−3 on average in summer, while in winter, urbanization increased air pollution even more, by around 2–3 µg m−3 (Fig. 4). When comparing these results to other similar studies, one has to remember that this includes not only the impact of urban emissions but also the counteracting effect of UCMF and increased dry deposition and, in a minor way, also the impact of biogenic emissions changes. Indeed, urban emissions alone increased PM2.5 concentration by 1.2–2 µg m−3 and 1.5–3.5 µg m−3 in winter and summer. These numbers are close to what was modelled by Huszar et al. (2016a), who only looked at the effect of emissions. The reason we got slightly smaller numbers is that they used 2005 emissions, which were higher compared to our 2015 emissions used in this study. Our PM2.5 increases due to urban emissions only are also much lower than in Im and Kanakidou (2012), but they modelled Istanbul and Athens, which are large megacities, much larger than the average of our central European selection. Previously, Skyllakou et al. (2014) showed for Paris that the contribution of local sources to PM is smaller, around 1 µg m−3; however they used much coarser resolution and thus could not capture the city's core contributions. Indeed, the contributions of urban emissions to urban air pollution over the urban core are much larger if higher resolutions are applied, as seen in Huszar et al. (2021), as the largest contributions occur over city centres (Thunis et al., 2021). Finardi et al. (2014) made estimates on the impact of Po valley, a highly urbanized region in northern Italy, on PM2.5 concentration, and they found that local emissions contribute to local concentration by up to 10–20 µg m−3, which is a much larger contribution than our 4 µg m−3 simulated for Milan (a city belonging to this region); however, they simulated the contribution from a much larger, regional emission source and not only one city.
As already seen, the total urban impact is lower if other contributors besides the urban emissions are considered. From these, the impact of UCMF showed a clear decrease in PM2.5 concentrations by around 0.5 µg m−3, counteracting the increase due to urban emissions. Indeed, many showed that the most important component of UCMF is the enhanced vertical eddy transport, which removes pollutants from the surface layer (where they are emitted), causing decreased concentrations (Kim et al., 2015; Huszar et al., 2018b, 2020a; Zhu et al., 2017; Wei et al., 2018). Moreover, our spatial results showed that PM2.5 increases due to UCMF over rural areas, which was also seen in Huszar et al. (2018b) and is probably the result of the fact that the PM removed by increased turbulence is deposited to lower model levels over other regions further from the sources (cities). However, as seen in Huszar et al. (2018a), the strong reduction of wind speed over and around urban areas can be sometimes very strong, resulting in the turbulence decrease being counteracted. This in turn causes an increase in urban PM2.5 concentrations. This probably also contributed to the modelled increases in PM2.5 (e.g. over the Benelux states in JJA, similar to Huszar et al. (2018b)).
Regarding the impact of urbanized land surface on PM deposition, the results are in line with the expectations that increased DVs over cities (by 30 %–50 %) result in decreased concentrations of PM2.5 (by around −0.12 and −0.23 µg m−3 in summer vs. winter). Although this is a minor decrease, it is seen over the whole domain, with maxima, as expected, over cities. Moreover, the decrease in DV is the same across all the PM2.5 components as in the used CTM, DV is a function of aerosol size only. Here we have to note, however, that the dry-deposition parameterization used here (Zhang et al., 2001) considers urban areas to be flat surfaces with prescribed roughness length and other parameters relevant for the dry deposition. This certainly differs from reality, where the urban canopy is formed of individual objects with different surface materials and also a vegetation fraction, while in each of these cases the parameters controlling the dry deposition are different. For example, Cherin et al. (2015) showed that “dry-deposition velocities can vary by a factor of 24 between two surface types in urban areas”. Our results on the impact on urban land surface on PM2.5 dry deposition are therefore a very rough estimate.
Regarding the impact of modified biogenic emissions on PM2.5, these are of course acting predominantly via modifying SOA concentrations. As BVOCs are a main precursor of biogenic SOA, a decrease in biogenic emissions results in a decrease in SOA formation. This is more pronounced during summer, of course, when BVOC emissions are at their peak. We also modelled some secondary effects on PNO3 (and to a smaller extent on sulfates and ammonia), related to the influence on OH radicals, which in turn influences the oxidation of nitrates and also ammonium, causing them to decrease (Aksoyoglu et al., 2017).
We further discuss the modelled RUT-induced modifications of the analysed PM2.5 components. Looking at elemental carbon, it is an inert aerosol in CAMx without chemical decay, so it is influenced via direct pathways along with changes of emissions, meteorological conditions, and deposition. Indeed, urbanization increased PEC by about 0.2–0.6 µg m−3 in winter and by about 0.2–0.3 µg m−3 in summer (Fig. 5), which is mainly influenced by the urban emissions alone, causing slightly higher increases, and the increase is predominately limited to urban areas. Indeed, for example, Skyllakou et al. (2014) showed for Paris that almost 60 % of PEC originates from local sources, although they calculated somehow stronger contributions of urban emissions to the total PEC (around 0.3–0.4 µg m−3). However, Paris is at the high end of the size distribution of cities we selected, so its urban emissions will be also very large compared to the average in our selection. The total urbanization impact on PEC is again smaller than that of emission only, which is caused partly by the effect of UCMF on PEC. Similarly to the total PM2.5, PEC also responds to higher vertical eddy diffusion above cities by decreases. A similar decrease in PEC due to UCMF to that in our study was modelled by Huszar et al. (2018b), who showed that this decrease is a counteraction of a large decrease due to turbulence enhancement and a smaller increase due to reduced wind speeds. Our results further showed that the decrease is largest during afternoon hours, which is in line with Huszar et al. (2018b) and Huszar et al. (2020a). They showed that the impact of turbulence enhancement is largest during these hours of the day when the strongest mixing in and above urban canopy occurs. The impact of enhanced deposition velocities is again expected and exhibits a clear decrease in PEC concentrations above urban areas. The impact is larger in winter in line with the larger winter absolute PEC concentration compared to summer ones. Further we showed (and this was seen for PM2.5 too) that the strongest decrease in PEC due to increased DV occurs during daytime, which can be clearly explained by the peaking DV values during the day and thus the strongest influence of urban land surface (as shown in Fig. 14). This is a known behaviour of particle deposition velocities which are modelled to peak during early afternoon hours (e.g. Nho-Kim et al., 2004). Finally, as PEC is chemically inert, it does not respond to modification of biogenic emissions.
As a result of urbanization, sulfates increased by about 0.05 µg m−3 in winter and 0.03–0.04 µg m−3 in summer, while urban emissions alone caused a slightly larger increase in winter (around 0.1 µg m−3) and a similar one in summer (Fig. 5). These are almost 10 times smaller values compared to PEC and in general show the low sulfur fraction of urban emissions in Europe. Indeed, sulfates are emitted mainly as a result of combustion; however strong reduction policies were implemented during the 80s and 90s which substantially reduced the sulfur content of combustion products (Vestreng et al., 2007). Sulfur emissions, and thus sulfate formation, are larger in eastern Europe (Fig. 7), especially in Poland, were coal combustion is still a significant energy producing method. This is also reflected in the emission data we used, and consequently, the largest urban contributions to regional sulfate levels are above eastern Europe where often coal combustion facilities are located even within the cities outskirts, or coal is used for domestic combustion. For this reason, winter sulfate increases due to urban emission are much larger than during summer. Previously, Skyllakou et al. (2014) showed a very small contribution of local sources to PSO4 concentrations (on the case of Paris), reaching less than 0.1 µg m−3 during summer, which is in line with the largest contributions in our case (e.g. over Hamburg due to shipping SO2 emissions or over Polish cities). Due to the urban canopy meteorological forcing, sulfates decreased over cities and increased over rural areas (Fig. 8). This is expected (similarly to PM2.5) as the main component acting within UCMF is the enhanced vertical eddy diffusion which removes material from the surface model layer and is deposited further from the sources, causing increase elsewhere. This behaviour was seen also by Huszar et al. (2018b), who have seen a decrease in sulfates of about up to −0.5 µg m−3, similar to our results. Moreover, sulfates decreased also due to decreases in their precursors, SO2 and NH3, driven by the same mechanism. Indeed, we showed in Huszar et al. (2022) too that SO2 usually decreases above cities by about 0.5 ppbv. NH3 also decreases, as seen in Fig. 15, by about up to 1 ppbv in both seasons, limiting the formation of ammonium sulfate. Also, Kang et al. (2022) showed that near the surface, sulfates decrease due to the enhanced urban mixing (an increase in the free troposphere above). It has to be noted, however, that the UCMF-induced modifications are a trade-off between the wind-speed decreases which increase the urban concentrations and the turbulence-induced decreases. In some studies, for example, Huszar et al. (2018b), the wind speed decrease is more important and can cause higher urban concentrations due to UCMF. As expected, sulfates decreased as a result of increased dry-deposition velocities (Fig. 9), and this is also amplified by the increased dry deposition of SO2 due to urbanization, as shown in Huszar et al. (2022). Finally, the impact of reduced urban BVOC emissions on PSO4 is negligible; only some very small increases during summer are modelled. This may be explained by the fewer OH radicals reacting to oxidize biogenic hydrocarbons, which thus can oxidize more SO2 (Aksoyoglu et al., 2017).
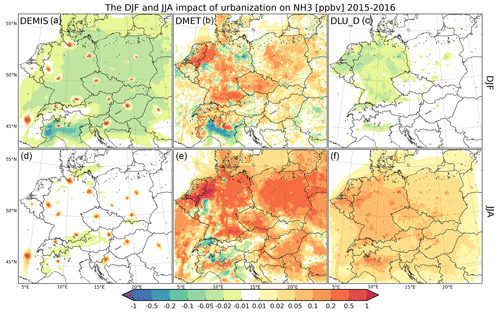
Figure 15The DJF (a, b, c) and JJA (d, e, f) impact of “DEMIS”, “DMET”, and “DL_U” on near-surface NH3 concentrations in parts per billion by volume (ppbv).
Urbanization increased nitrates and ammonium by about 0.1 and 0.25 µg m−3, respectively, with higher numbers in winter (Fig. 5), which is clear as the absolute concentrations of these secondary aerosols are also higher in winter. The impact of emissions alone is also clearly higher in winter, which is caused by stronger NOx emissions (mainly combustion and transport) and usually by higher ammonia emissions too during the colder parts of the year (although these can have a smaller late summer peak too; for example, Drugé et al., 2019) (see Fig. 7). Again, the emission impact is higher than the one from total urbanization, and this is caused (similarly to PM2.5, PEC or sulfates) by the effect of UCMF, which is dominated by increased vertical eddy diffusion. This reduces the near-surface concentrations of both aerosols and their precursors (NOx and NH3). Previously, Huszar et al. (2018b) showed decreases in PNO3 and PNH4 over central European cities due to UCMF by about 0.02–0.04 and 0.02 µg m−3 in summer (they did not look at winter), which is slightly smaller for nitrates than our numbers and is comparable to ammonium modification presented in this work. The decrease in PNO3 and PNH4 is also caused by the decreases of nitrogen oxides and ammonia, as seen in Fig. 15 or previously in Huszar et al. (2018b, 2022). Recently, Kang et al. (2022) also reported higher nitrate and ammonium values above a large Chinese agglomeration if the urban land surface and the associated UCMF were not considered. Similar to sulfates, PNO3 and PNH4 responded to urbanization-induced land-surface changes by increased dry-deposition velocities, resulting in their decrease. In winter, this was also partly caused by the reduced NH3 due to increased dry deposition; however for JJA, we modelled increased ammonia concentration due to dry-deposition changes due to urbanization (see Fig.15). Indeed, the dry-deposition model used (Zhang et al., 2003) predicts smaller dry-deposition velocities for urban areas compared to rural ones (e,g, crops) for NH3 in the case of dry canopies (i.e. summer conditions). Finally, as a result of decreased urban BVOC emissions, some increases in nitrates and ammonium are modelled, which can be connected to more OH available to oxidize NOx (Aksoyoglu et al., 2017), but also, less NOx is reacting with organic molecules to create organic nitrates (Fischer et al., 2014). For example, Jiang et al. (2019) showed that smaller biogenic emissions fluxes result in increased PNO3 and PNH4 concentrations over central Europe, while the impact on PNO3 is larger, which is in line with our results.
The impact of urbanization on secondary organic aerosol concentration is only notable during summer (Fig. 5), owing to the suppressed oxidation of VOCs in winter and small BVOC emissions responsible for biogenic SOA formation during the cold parts of the year (Gao et al., 2022; Zhai et al., 2023). The total summer impact of urbanization is about 0.03–0.05 µg m−3 (reaching 0.1 µg m−3), while the emission impact alone is again larger, often exceeding 0.1 µg m−3. A very similar summer contribution of urban emissions from Paris was modelled earlier by Skyllakou et al. (2014), who pointed out that the contribution of SOA to the total urban impact is about 5 %–10 %. Freney et al. (2014) arrived at similar results and argued that the contribution of SOA gets larger as the urban plume ages. Regarding the impact of UCMF on SOA in summer (Fig. 8), it is characterized by both increases and decreases, depending on the city, with an average slightly below 0 (around −0.01 µg m−3). The reason for this is probably the interplay between the reduction caused by the increased vertical eddy diffusion and the increase caused by the decreased urban wind speeds, while increased urban temperatures shift the partitioning between the gas phase and particulate phase towards the gas phase (Huszar et al., 2018b; Wang et al., 2009). This means the impact over a particular city depends on the relative magnitude of these three components of UCMF and how they act on SOA. The UCMF also caused a relatively large impact over rural areas, which can also be explained by the fact that SOA is formed more readily in an aged urban plume (Ortega et al., 2016), so, probably, the SOA precursors removed by the increased vertical eddy diffusion were transported over rural areas, while they were oxidized and condensed into SOA. Regarding the impact of increased PM deposition velocities due to urbanization, their impact follows that of secondary inorganic aerosol or PEC; i.e. the urban concentrations decreased (by around −0.02 µg m−3). Here, again, one must realize that the modified DVs also impacted the precursor species which oxidize to semi-volatile compounds. In CAMx in the Zhang deposition model for gases (Zhang et al., 2003), the deposition velocities for SOA precursors (and most of VOCs) are smaller for urban canopies, which means their concentrations are larger for urban areas. Hence, more SOA formation is favoured because of higher precursor abundances. This consequently means that the final impact on SOA is a counteraction between the decrease due to the direct impact of urbanization-induced land-use change on SOA deposition velocities and the increase due to higher precursor concentrations. In our simulation, the former was the dominating effect as SOA decreased above all urban areas. Finally, the urbanization-induced decrease in BVOC emissions resulted in a reduction of SOA concentrations (by about 0.04–0.06 µg m−3), and this decrease is modelled not only over urban areas but also over large rural regions, while the largest decrease is above Milan, which also has the warmest climate among the selected cities (Figs. 5 and 11). Indeed, SOA of biogenic origin is a an important contributor to urban SOA levels, so the modelled decreases were expected (Couvidat et al., 2013; Hu et al., 2017). Ghirardo et al. (2016) also calculated a strong influence of local BVOC emissions from urban trees in a Chinese megacity (although the anthropogenic influences were much larger). Again, the impact also affected rural areas, which can be explained by the repeated fact that in aged urban plumes, SOA forms more effectively.
In summary, we evaluated over a sample of 19 central European cities the impact of rural-to-urban transformation and its four contributors on PM urban (and rural) concentrations, including the impact on its primary and secondary components. We found that the two main controlling drivers are the impact of urban emissions themselves (increases the concentrations for PM2.5 and all of its analysed components) and the urban canopy meteorological forcing (usually decreases over urban areas, increases over rural ones). We showed however that two additional controlling mechanisms can play an important role within the process of urbanization although smaller by an order of magnitude than the effect of emissions and UCMF: the impact of dry-deposition velocity changes due to urbanization of land surface and the reduction of biogenic emissions by turning rural/natural land surfaces into urban built-up areas. The former results in decreases in concentrations due to increased deposition velocities, and the latter acts predominantly via modification of secondary organic aerosols and results in a decrease in PM2.5 concentrations (by reducing SOA). In summary, when the impact of urbanization on PM air pollution is analysed, all four contributors have to be accounted for.
It also has to be discussed what impact has the order of application of the contributors on their magnitude. The experiment design started with addition of the urban emission (DEMIS) to the reference (non-urbanized) state as this impact was assumed (and proven) to be the dominant. With this choice, the urban atmosphere was already filled with pollutants serving as the base state for the other impacts. The impact of the order of addition of the two further contributors, DLU and DBVOC, is probably small as their effect is also small. However, if they were applied before DEMIS, their magnitude would probably be even smaller: the deposition flux depends on the absolute concentrations (Zhang et al., 2003), while adding BVOCs to a high-NOx air can have mixed effects, leading to both increases and decreases in secondary organic aerosol (K. Li et al., 2019; Pullinen et al., 2020). Further, in our previous paper, Huszar et al. (2022), which had the same goal and modelling design as this paper but looked at the gas-phase chemistry, we analysed the effect of the order of the two sub-contributors of DBVOC in detail, which are the impact of reduced vegetation (DBVOC_L; see Huszar et al., 2022) and the impact of changed meteorological conditions that drive the MEGAN model, impacting BVOC fluxes (DBVOC_M). We found that (1) with regard to DBVOC, the changes of vegetation cover play a much more important role than the changed meteorological conditions, and (2) the partial impact of changed meteorological conditions is smaller if applied after DBVOC_L. As for the DMET impact, this is in general the second-strongest contributor, and one gets different magnitudes of the impacts if DMET is applied before DEMIS. Let us suppose that first the DMET contributor has been applied. This means that the meteorological conditions that drive the impact of emissions consider the urban canopy meteorological forcing (UCMF). Huszar et al. (2021) however showed that the impact of urban emissions is considerably (almost by 50 %) smaller if UCMF is accounted for. On the other hand, DMET applied as the first contributor would be very small as UCMF would act on much less polluted air (with missing urban emissions). So in conclusion, the DLU, DBVOC, and DMET impacts would be smaller if applied before DEMIS and somehow decoupled from reality ,motivating us to start the addition of the different components of urbanization by the emissions themselves. The choice of the order of the other three contributors rather has a much smaller effect.
We must also stress that the cities selected in this study are from a relatively small region, meaning that they do not exhibit a substantially different background climate. Moreover the typical “rural” vegetation was assumed to be crop, which might not be the case if cities in other parts of the world were considered (e.g. tropical areas), meaning that the impact of modified biogenic emissions could be much stronger. Further, some secondary effects of PM concentration changes can play a role too via direct and indirect radiative effects. For example, photolysis rates and temperatures are altered via the direct effect of aerosol, which in turn influences air chemistry (Han et al., 2020; Wang et al., 2022), or the vertical structure of urban boundary layer can be altered by the aerosol emitted that modifies the overall stability and convection (Miao et al., 2020; Slater et al., 2022; Fan et al., 2020; Yu et al., 2020; López-Romero et al., 2021), which in turn can modify the vertical mixing and precipitation with feedbacks on species concentration. Consequently, to obtain a more accurate quantification of the impact of rural-to-urban transformation on PM, these effects have to be included in modelling studies.
The RegCM4.7 model is freely available for public use at https://github.com/ICTP/RegCM (ICTP, 2021) (https://doi.org/10.5281/zenodo.7548172, Giorgi et al., 2023). CAMx version 7.10 is available at https://www.camx.com/download/ (Ramboll, 2020b, a). The RegCM2CAMx meteorological preprocessor used to convert RegCM outputs to CAMx inputs and the MEGAN v2.10 code as used by the authors are available upon request from the first author. The complete model configuration and all the simulated data (3-dimensional hourly data) used for the analysis are stored at the Dept. of Atmospheric Physics of the Charles University data storage facilities (about 5TB) and are available upon request from the first author.
PH created the concept and designed the experiments; PH and JK performed the model simulations; APPP, LB, and AVP contributed to input data preparation, model configuration, and analysis of the outputs; and all authors contributed to the manuscript text.
The authors declare that they have no conflict of interest.
Publisher’s note: Copernicus Publications remains neutral with regard to jurisdictional claims made in the text, published maps, institutional affiliations, or any other geographical representation in this paper. While Copernicus Publications makes every effort to include appropriate place names, the final responsibility lies with the authors.
We acknowledge the CAMS-REG-APv1.1 emissions dataset provided by the Copernicus Atmosphere Monitoring Service, the Air Pollution Sources Register (REZZO) dataset provided by the Czech Hydrometeorological Institute, and the ATEM Traffic Emissions dataset provided by ATEM (Studio of ecological models). We also acknowledge the providers of AirBase European Air Quality data (http://www.eea.europa.eu/data-and-maps/data/aqereporting-1, last access: 9 January 2024).
This research has been supported by the Technology Agency of the Czech Republic (grant no. SS02030031), the Grantová Agentura České Republiky (grant no. 19-10747Y), and the Univerzita Karlova v Praze (grant no. SVV 260709).
This paper was edited by Manish Shrivastava and reviewed by two anonymous referees.
Allan, J. D., Williams, P. I., Morgan, W. T., Martin, C. L., Flynn, M. J., Lee, J., Nemitz, E., Phillips, G. J., Gallagher, M. W., and Coe, H.: Contributions from transport, solid fuel burning and cooking to primary organic aerosols in two UK cities, Atmos. Chem. Phys., 10, 647–668, https://doi.org/10.5194/acp-10-647-2010, 2010. a, b
Aksoyoglu, S., Ciarelli, G., El-Haddad, I., Baltensperger, U., and Prévôt, A. S. H.: Secondary inorganic aerosols in Europe: sources and the significant influence of biogenic VOC emissions, especially on ammonium nitrate, Atmos. Chem. Phys., 17, 7757–7773, https://doi.org/10.5194/acp-17-7757-2017, 2017. a, b, c
Balamurugan, V., Chen, J., Qu, Z., Bi, X., and Keutsch, F. N.: Secondary PM2.5 decreases significantly less than NO2 emission reductions during COVID lockdown in Germany, Atmos. Chem. Phys., 22, 7105–7129, https://doi.org/10.5194/acp-22-7105-2022, 2022. a
Bartík, L., Huszár, P., Vlček, O., and Eben, K.: Sensitivity of Secondary Inorganic Aerosol Concentrations to Precursor Emissions and Inorganic Aerosol Modules in CAMx over Central Europe, in: WDS'21 Proceedings of Contributed Papers – Physics, edited by: Šafránková, J. and Pavlů, J., Prague, Matfyzpress, 77–84, https://www.mff.cuni.cz/veda/konference/wds/proc/pdf21/WDS21_10_f8_Bartik.pdf (last access: 5 May 2023), 2021. a
Beekmann, M. and Vautard, R.: A modelling study of photochemical regimes over Europe: robustness and variability, Atmos. Chem. Phys., 10, 10067–10084, https://doi.org/10.5194/acp-10-10067-2010, 2010. a
Behera, N. S. and Sharma, M.: Investigating the potential role of ammonia in ion chemistry of fine particulate matter formation for an urban environment, Sci. Total Environ., 408, 3569–3575, 2010. a
Benešová, N., Belda, M., Eben, K., Geletič, J., Huszár, P., Juruš, P., Krč, P., Resler, J., and Vlček, O.: New open source emission processor for air quality models, in: Proceedings of Abstracts 11th International Conference on Air Quality Science and Application, edited by: Sokhi, R., Tiwari, P. R., Gállego, M. J., Craviotto Arnau, J. M., Castells Guiu, C., and Singh, V., https://doi.org/10.18745/PB.19829, 27 pp., University of Hertfordshire. Paper presented at Air Quality 2018 conference, Barcelona, 12–16 March, 2018. a
Butler, T. M. and Lawrence, M. G.: The influence of megacities on global atmospheric chemistry: a modelling study, Environ. Chem., 6, 219–225, https://doi.org/10.1071/EN08110, 2009. a, b
Buchholz, R. R., Emmons, L. K., Tilmes, S., and The CESM2 Development Team: CESM2.1/CAM-chem Instantaneous Output for Boundary Conditions. UCAR/NCAR – Atmospheric Chemistry Observations and Modeling Laboratory. Subset used Lat: 10 to 80, Lon: −20 to 50, December 2014–January 2017, https://doi.org/10.5065/NMP7-EP60, 2019. a
Byun, D. W. and Ching, J. K. S.: Science Algorithms of the EPA Model-3 Community Multiscale Air Quality (CMAQ) Modeling System, Office of Research and Development, U.S. EPA, North Carolina, EPA/600/R-99/030, 1999. a
Cao, L., Li, S., and Sun, L.: Study of different Carbon Bond 6 (CB6) mechanisms by using a concentration sensitivity analysis, Atmos. Chem. Phys., 21, 12687–12714, https://doi.org/10.5194/acp-21-12687-2021, 2021. a
Cherin, N., Roustan, Y., Musson-Genon, L., and Seigneur, C.: Modelling atmospheric dry deposition in urban areas using an urban canopy approach, Geosci. Model Dev., 8, 893–910, https://doi.org/10.5194/gmd-8-893-2015, 2015. a, b
Ciarelli, G., Aksoyoglu, S., El Haddad, I., Bruns, E. A., Crippa, M., Poulain, L., Äijälä, M., Carbone, S., Freney, E., O'Dowd, C., Baltensperger, U., and Prévôt, A. S. H.: Modelling winter organic aerosol at the European scale with CAMx: evaluation and source apportionment with a VBS parameterization based on novel wood burning smog chamber experiments, Atmos. Chem. Phys., 17, 7653–7669, https://doi.org/10.5194/acp-17-7653-2017, 2017. a, b
Couvidat, F., Kim, Y., Sartelet, K., Seigneur, C., Marchand, N., and Sciare, J.: Modeling secondary organic aerosol in an urban area: application to Paris, France, Atmos. Chem. Phys., 13, 983–996, https://doi.org/10.5194/acp-13-983-2013, 2013. a, b
Ďoubalová, J., Huszár, P., Eben, K., Benešová, N., Belda, M., Vlček, O., Karlický, J., Geletič, J., and Halenka, T.: High Resolution Air Quality Forecasting Over Prague within the URBI PRAGENSI Project: Model Performance During the Winter Period and the Effect of Urban Parameterization on PM, Atmosphere, 11, 625, https://doi.org/10.3390/atmos11060625, 2020. a
Drugé, T., Nabat, P., Mallet, M., and Somot, S.: Model simulation of ammonium and nitrate aerosols distribution in the Euro-Mediterranean region and their radiative and climatic effects over 1979–2016, Atmos. Chem. Phys., 19, 3707–3731, https://doi.org/10.5194/acp-19-3707-2019, 2019. a
EEA: Air quality in Europe 2022, Report no. 05/2022, https://www.eea.europa.eu//publications/air-quality-in-europe-2022 (last access: 25 January 2023), 2022. a
Emmons, L. K., Schwantes, R. H., Orlando, J. J., Tyndall, G., Kinnison, D., Lamarque, J.-F., Marsh, D., Mills, M. J., Tilmes, S., Bardeen, C., Buchholtz, R. R., Conley, A., Gettelman, A., Garcia, R., Simpson, I., Blake, D. R., Meinardi, S., and Petron, G.: The Chemistry Mechanism in the Community Earth System Model version 2 (CESM2), J. Adv. Model. Earth Sys., 12, e2019MS001882. https://doi.org/10.1029/2019MS001882, 2020. a
Fan, J., Zhang, Y., Li, Z., Hu, J., and Rosenfeld, D.: Urbanization-induced land and aerosol impacts on sea-breeze circulation and convective precipitation, Atmos. Chem. Phys., 20, 14163–14182, https://doi.org/10.5194/acp-20-14163-2020, 2020. a
Fenech, S., Doherty, R. M., Heaviside, C., Vardoulakis, S., Macintyre, H. L., and O'Connor, F. M.: The influence of model spatial resolution on simulated ozone and fine particulate matter for Europe: implications for health impact assessments, Atmos. Chem. Phys., 18, 5765–5784, https://doi.org/10.5194/acp-18-5765-2018, 2018. a
Finardi, S., Silibello, C., D'Allura, A., and Radice, P.: Analysis of pollutants exchange between the Po Valley and the surrounding European region, Urban Clim., 10, 682–702, https://doi.org/10.1016/j.uclim.2014.02.002, 2014. a, b
Fischer, E. V., Jacob, D. J., Yantosca, R. M., Sulprizio, M. P., Millet, D. B., Mao, J., Paulot, F., Singh, H. B., Roiger, A., Ries, L., Talbot, R. W., Dzepina, K., and Pandey Deolal, S.: Atmospheric peroxyacetyl nitrate (PAN): a global budget and source attribution, Atmos. Chem. Phys., 14, 2679–2698, https://doi.org/10.5194/acp-14-2679-2014, 2014. a
Folberth, G. A., Butler, T. M., Collins, W. J., and Rumbold, S. T.: Megacities and climate change – A brief overview, Environ. Pollut., 203, 235–242, https://doi.org/10.1016/j.envpol.2014.09.004, 2015. a
Freney, E. J., Sellegri, K., Canonaco, F., Colomb, A., Borbon, A., Michoud, V., Doussin, J.-F., Crumeyrolle, S., Amarouche, N., Pichon, J.-M., Bourianne, T., Gomes, L., Prevot, A. S. H., Beekmann, M., and Schwarzenböeck, A.: Characterizing the impact of urban emissions on regional aerosol particles: airborne measurements during the MEGAPOLI experiment, Atmos. Chem. Phys., 14, 1397–1412, https://doi.org/10.5194/acp-14-1397-2014, 2014. a, b
Ganbat, G., Baik, J. J., and Ryu, Y. H.: A numerical study of the interactions of urban breeze circulation with mountain slope winds, Theor. App. Clim., 120, 123–135, 2015. a
Gao, J. and O'Neill, B. C.: Mapping global urban land for the 21st century with data-driven simulations and Shared Socioeconomic Pathways, Nat. Com., 11, 2302, https://doi.org/10.1038/s41467-020-15788-7, 2020. a
Gao, Y., Ma, M., Yan, F., Su, H., Wang, S., Liao, H., Zhao, B., Wang, X., Sun, Y., Hopkins, J. R., Chen, Q., Fu, P., Lewis, A. C., Qiu, Q., Yao, X., and Gao, H.: Impacts of biogenic emissions from urban landscapes on summer ozone and secondary organic aerosol formation in megacities, Sci. Total Environ., 814, 152654, https://doi.org/10.1016/j.scitotenv.2021.152654, 2022. a, b
Ghirardo, A., Xie, J., Zheng, X., Wang, Y., Grote, R., Block, K., Wildt, J., Mentel, T., Kiendler-Scharr, A., Hallquist, M., Butterbach-Bahl, K., and Schnitzler, J.-P.: Urban stress-induced biogenic VOC emissions and SOA-forming potentials in Beijing, Atmos. Chem. Phys., 16, 2901–2920, https://doi.org/10.5194/acp-16-2901-2016, 2016. a
Giani, P., Balzarini, A., Pirovano, G., Gilardoni, S., Paglione, M., Colombi, C., Gianelle, V. L., Belis, C. A., Poluzzi, V., and Lonati, G.: Influence of semi- and intermediate-volatile organic compounds (S/IVOC) parameterizations, volatility distributions and aging schemes on organic aerosol modelling in winter conditions, Atmos. Environ., 213, 11–24, https://doi.org/10.1016/j.atmosenv.2019.05.061, 2019. a
Giorgi, F., Coppola, E., Solmon, F., Mariotti, L., Sylla, M., Bi, X., Elguindi, N., Diro, G. T., Nair, V., Giuliani, G., Cozzini, S., Guettler, I., O'Brien, T. A., Tawfi, A. B., Shalaby, A., Zakey, A., Steiner, A., Stordal, F., Sloan, L., and Brankovic, C.: RegCM4: model description and preliminary tests over multiple CORDEX domains, Clim. Res., 52, 7–29, 2012. a
Giorgi, F., Coppola, E., Giuliani, G., Ciarlo, J., Pichelli, E., Nogherotto, R., Raffaele, F., Malguzzi, P., Davolio, S., Stocchi, P., and Drofa, O.: RegCM-NH V5 code (5.0.0), Zenodo [code], https://doi.org/10.5281/zenodo.7548172, 2023. a
ICTP: The Regional Climate Model version 4.7 source code (provided by Graziano Giuliani), GitHub [code], https://github.com/ICTP/RegCM (last access: 16 May 2023), 2021. a
Granier, C.S., Darras, H., Denier van der Gon, J., Doubalova, N., Elguindi, B., Galle, M., Gauss, M., Guevara, J.-P., Jalkanen, J., and Kuenen, C.: The Copernicus Atmosphere Monitoring Service Global and Regional Emissions; Report April 2019 version [Research Report], ECMWF, Reading, UK, https://doi.org/10.24380/d0bn-kx16, 2019. a
Guenther, A., Karl, T., Harley, P., Wiedinmyer, C., Palmer, P. I., and Geron, C.: Estimates of global terrestrial isoprene emissions using MEGAN (Model of Emissions of Gases and Aerosols from Nature), Atmos. Chem. Phys., 6, 3181–3210, https://doi.org/10.5194/acp-6-3181-2006, 2006. a
Guenther, A. B., Jiang, X., Heald, C. L., Sakulyanontvittaya, T., Duhl, T., Emmons, L. K., and Wang, X.: The Model of Emissions of Gases and Aerosols from Nature version 2.1 (MEGAN2.1): an extended and updated framework for modeling biogenic emissions, Geosci. Model Dev., 5, 1471–1492, https://doi.org/10.5194/gmd-5-1471-2012, 2012. a
Guo, Y., Yan, C., Liu, Y., Qiao, X., Zheng, F., Zhang, Y., Zhou, Y., Li, C., Fan, X., Lin, Z., Feng, Z., Zhang, Y., Zheng, P., Tian, L., Nie, W., Wang, Z., Huang, D., Daellenbach, K. R., Yao, L., Dada, L., Bianchi, F., Jiang, J., Liu, Y., Kerminen, V.-M., and Kulmala, M.: Seasonal variation in oxygenated organic molecules in urban Beijing and their contribution to secondary organic aerosol, Atmos. Chem. Phys., 22, 10077–10097, https://doi.org/10.5194/acp-22-10077-2022, 2022. a
Guttikunda, K. S., Carmichael, G. R., Calori, G., Eck, C., and Woo, J.-H.: The contribution of megacities to regional sulfur pollution in Asia, Atmos. Environ., 37, 11–22, https://doi.org/10.1016/S1352-2310(02)00821-X, 2003. a, b
Guttikunda, S. K., Tang, Y., Carmichael, G. R., Kurata, G., Pan, L., Streets, D. G., Woo, J.-H., Thongboonchoo, N., and Fried, A.: Impacts of Asian megacity emissions on regional air quality during spring 2001, J. Geophys. Res., 110, D20301, https://doi.org/10.1029/2004JD004921, 2005. a
Han, W., Li, Z., Wu, F., Zhang, Y., Guo, J., Su, T., Cribb, M., Fan, J., Chen, T., Wei, J., and Lee, S.-S.: The mechanisms and seasonal differences of the impact of aerosols on daytime surface urban heat island effect, Atmos. Chem. Phys., 20, 6479–6493, https://doi.org/10.5194/acp-20-6479-2020, 2020. a
Hardacre, C., Mulcahy, J. P., Pope, R. J., Jones, C. G., Rumbold, S. T., Li, C., Johnson, C., and Turnock, S. T.: Evaluation of SO2, SO and an updated SO2 dry deposition parameterization in the United Kingdom Earth System Model, Atmos. Chem. Phys., 21, 18465–18497, https://doi.org/10.5194/acp-21-18465-2021, 2021. a
Hodnebrog, Õ., Stordal, F., and Berntsen, T. K.: Does the resolution of megacity emissions impact large scale ozone?, Atmos. Environ., 45, 6852–6862, 2011. a
Holtslag, A. A. M., de Bruijn, E. I. F., and Pan, H.-L.: A high resolution air mass transformation model for shortrange weather forecasting, Mon. Weather Rev., 118, 1561–1575, 1990. a
Hong, S.-Y., Dudhia, J. and Chen, S.-H.: A Revised Approach to Ice Microphysical Processes for the Bulk Parameterization of Clouds and Precipitation, Month. Weather Rev., 132, 103–120, https://doi.org/10.1175/1520-0493(2004)132<0103:ARATIM>2.0.CO;2, 2004. a
Hood, C., MacKenzie, I., Stocker, J., Johnson, K., Carruthers, D., Vieno, M., and Doherty, R.: Air quality simulations for London using a coupled regional-to-local modelling system, Atmos. Chem. Phys., 18, 11221–11245, https://doi.org/10.5194/acp-18-11221-2018, 2018. a
Hu, J., Wang, P., Ying, Q., Zhang, H., Chen, J., Ge, X., Li, X., Jiang, J., Wang, S., Zhang, J., Zhao, Y., and Zhang, Y.: Modeling biogenic and anthropogenic secondary organic aerosol in China, Atmos. Chem. Phys., 17, 77–92, https://doi.org/10.5194/acp-17-77-2017, 2017. a, b
Huszar, P., Miksovsky, J., Pisoft, P., Belda, M., and Halenka, T.: Interactive coupling of a regional climate model and a chemistry transport model: evaluation and preliminary results on ozone and aerosol feedback, Clim. Res., 51, 59–88, https://doi.org/10.3354/cr01054, 2012. a
Huszar, P., Halenka, T., Belda, M., Zak, M., Sindelarova, K., and Miksovsky, J.: Regional climate model assessment of the urban land-surface forcing over central Europe, Atmos. Chem. Phys., 14, 12393–12413, https://doi.org/10.5194/acp-14-12393-2014, 2014. a, b, c
Huszar, P., Belda, M., and Halenka, T.: On the long-term impact of emissions from central European cities on regional air quality, Atmos. Chem. Phys., 16, 1331–1352, https://doi.org/10.5194/acp-16-1331-2016, 2016a. a, b, c, d, e
Huszár, P., Belda, M., Karlický, J., Pišoft, P., and Halenka, T.: The regional impact of urban emissions on climate over central Europe: present and future emission perspectives, Atmos. Chem. Phys., 16, 12993–13013, https://doi.org/10.5194/acp-16-12993-2016, 2016b. a
Huszar, P., Karlický, J., Belda, M., Halenka, T. and Pisoft, P.: The impact of urban canopy meteorological forcing on summer photochemistry, Atmos. Environ., 176, 209–228, https://doi.org/10.1016/j.atmosenv.2017.12.037, 2018a. a, b, c, d
Huszar, P., Belda, M., Karlický, J., Bardachova, T., Halenka, T., and Pisoft, P.: Impact of urban canopy meteorological forcing on aerosol concentrations, Atmos. Chem. Phys., 18, 14059–14078, https://doi.org/10.5194/acp-18-14059-2018, 2018b. a, b, c, d, e, f, g, h, i, j, k, l, m
Huszar, P., Karlický, J., Ďoubalová, J., Šindelářová, K., Nováková, T., Belda, M., Halenka, T., Žák, M., and Pišoft, P.: Urban canopy meteorological forcing and its impact on ozone and PM2.5: role of vertical turbulent transport, Atmos. Chem. Phys., 20, 1977–2016, https://doi.org/10.5194/acp-20-1977-2020, 2020a. a, b, c, d, e
Huszar, P., Karlický, J., Ďoubalová, J., Nováková, T., Šindelářová, K., Švábik, F., Belda, M., Halenka, T., and Žák, M.: The impact of urban land-surface on extreme air pollution over central Europe, Atmos. Chem. Phys., 20, 11655–11681, https://doi.org/10.5194/acp-20-11655-2020, 2020b. a
Huszar, P., Karlický, J., Marková, J., Nováková, T., Liaskoni, M., and Bartík, L.: The regional impact of urban emissions on air quality in Europe: the role of the urban canopy effects, Atmos. Chem. Phys., 21, 14309–14332, https://doi.org/10.5194/acp-21-14309-2021, 2021. a, b, c, d, e, f, g, h
Huszar, P., Karlický, J., Bartík, L., Liaskoni, M., Prieto Perez, A. P., and Šindelářová, K.: Impact of urbanization on gas-phase pollutant concentrations: a regional-scale, model-based analysis of the contributing factors, Atmos. Chem. Phys., 22, 12647–12674, https://doi.org/10.5194/acp-22-12647-2022, 2022. a, b, c, d, e, f, g, h, i, j, k, l, m, n, o
Im, U., Poupkou, A., Incecik, S., Markakis, K., Kindap, T., Unal, A., Melas, D., Yenigun, O., Topcu, O., Odman, M. T., Tayanc, M., and Guler, M.: The impact of anthropogenic and biogenic emissions on surface ozone concentrations in Istanbul, Sci. Total Environ., 409, 1255–1265, https://doi.org/10.1016/j.scitotenv.2010.12.026, 2011a. a
Im, U., Markakis, K., Poupkou, A., Melas, D., Unal, A., Gerasopoulos, E., Daskalakis, N., Kindap, T., and Kanakidou, M.: The impact of temperature changes on summer time ozone and its precursors in the Eastern Mediterranean, Atmos. Chem. Phys., 11, 3847–3864, https://doi.org/10.5194/acp-11-3847-2011, 2011b. a
Im, U. and Kanakidou, M.: Impacts of East Mediterranean megacity emissions on air quality, Atmos. Chem. Phys., 12, 6335–6355, https://doi.org/10.5194/acp-12-6335-2012, 2012. a, b, c, d
Im, U., Bianconi, R., Solazzo, E., Kioutsioukis, I., Badia, A.,Balzarini, A., Baro, R., Bellasio, R., Brunner, D., Chemel, C.,Curci, G., Denier van der Gon, H., Flemming, J., Forkel, R.,Giordano, L., Jimenez-Guerrero, P., Hirtl, M., Hodzic, A.,Honzak,L., Jorba, O., Knote, C., Makar, P. A., Manders-Groot, A., Neal, L., Perez, J. L., Pirovano, G., Pouliot, G., San Jose, R., Savage,N., Schroder,W., Sokhi, R. S., Syrakov, D., Torian, A., Tuccella,P., Wang, K., Werhahn, J., Wolke, R., Zabkar, R., Zhang,Y., Zhang, J., Hogrefe, C., and Galmarini, S.: Evaluation of operational online coupled regional air quality models over Europe and North America in the context of AQMEII phase 2, Part II: particulate matter, Atmos. Environ., 115, 421–441, 2015. a, b
Jacobson, M. Z., Nghiem, S. V., Sorichetta, A., and Whitney, N.: Ring of impact from the mega-urbanization of Beijing between 2000 and 2009, J. Geophys. Res., 120, 5740–5756, https://doi.org/10.1002/2014JD023008, 2015. a
Janssen, R. H. H., Tsimpidi, A. P., Karydis, V. A., Pozzer, A., Lelieveld, J., Crippa, M., Prévôt, A. S. H., Ait-Helal, W., Borbon, A., Sauvage, S. and Locoge, N.: Influence of local production and vertical transport on the organic aerosol budget over Paris, J. Geophys. Res., 122, 8276–8296, https://doi.org/10.1002/2016JD026402, 2017. a
Jiang, J., Aksoyoglu, S., El-Haddad, I., Ciarelli, G., Denier van der Gon, H. A. C., Canonaco, F., Gilardoni, S., Paglione, M., Minguillón, M. C., Favez, O., Zhang, Y., Marchand, N., Hao, L., Virtanen, A., Florou, K., O'Dowd, C., Ovadnevaite, J., Baltensperger, U., and Prévôt, A. S. H.: Sources of organic aerosols in Europe: a modeling study using CAMx with modified volatility basis set scheme, Atmos. Chem. Phys., 19, 15247–15270, https://doi.org/10.5194/acp-19-15247-2019, 2019. a, b
Kang, H., Zhu, B., de Leeuw, G., Yu, B., van der A, R. J., and Lu, W.: Impact of urban heat island on inorganic aerosol in the lower free troposphere: a case study in Hangzhou, China, Atmos. Chem. Phys., 22, 10623–10634, https://doi.org/10.5194/acp-22-10623-2022, 2022. a, b, c
Karlický, J., Huszár, P., Halenka, T., Belda, M., Žák, M., Pišoft, P., and Mikšovský, J.: Multi-model comparison of urban heat island modelling approaches, Atmos. Chem. Phys., 18, 10655–10674, https://doi.org/10.5194/acp-18-10655-2018, 2018. a
Karlický, J., Huszár, P., Nováková, T., Belda, M., Švábik, F., Ďoubalová, J., and Halenka, T.: The “urban meteorology island”: a multi-model ensemble analysis, Atmos. Chem. Phys., 20, 15061–15077, https://doi.org/10.5194/acp-20-15061-2020, 2020. a, b, c
Kim, Y, Sartelet, K., Raut, J.-Ch., and Chazette, P.: Influence of an urban canopy model and PBL schemes on vertical mixing for air quality modeling over Greater Paris, Atmos. Environ., 107, 289–306, https://doi.org/10.1016/j.atmosenv.2015.02.011, 2015. a, b
Kim, G., Lee, J., Lee, M.-I., and Kim, D.: Impacts of urbanization on atmospheric circulation and aerosol transport in a coastal environment simulated by the WRF-Chem coupled with urban canopy model, Atmos. Environ., 249, 118253, https://doi.org/10.1016/j.atmosenv.2021.118253, 2021. a
Khare, P., Machesky, J., Soto, R., He, M., Presto, A. A. and Gentner, D. R.: Asphalt-related emissions are a major missing nontraditional source of secondary organic aerosol precursors, Sci. Adv., 6, eabb9785, https://doi.org/10.1126/sciadv.abb9785, 2020. a
Khomenko, S., Cirach, M., Pereira-Barboza, E., Mueller, N., Barrera-Gómez, J., Rojas-Rueda, D., de Hoogh, K., Hoek, G., and Nieuwenhuijsen, M.: Premature mortality due to air pollution in European cities: a health impact assessment, Lancet Planet. Health, 3, S2542519620302722, https://doi.org/10.1016/S2542-5196(20)30272-2, 2021. a
Lanz, V. A., Prévôt, A. S. H., Alfarra, M. R., Weimer, S., Mohr, C., DeCarlo, P. F., Gianini, M. F. D., Hueglin, C., Schneider, J., Favez, O., D'Anna, B., George, C., and Baltensperger, U.: Characterization of aerosol chemical composition with aerosol mass spectrometry in Central Europe: an overview, Atmos. Chem. Phys., 10, 10453–10471, https://doi.org/10.5194/acp-10-10453-2010, 2010. a
Lawrence, P. J. and Chase, T. N.: Representing a new MODIS consistent land surface in the Community Land Model (CLM 3.0), J. Geophys. Res.-Biogeo., 112, G01023, https://doi.org/10.1029/2006JG000168, 2007. a
Lawrence, M. G., Butler, T. M., Steinkamp, J., Gurjar, B. R., and Lelieveld, J.: Regional pollution potentials of megacities and other major population centers, Atmos. Chem. Phys., 7, 3969–3987, https://doi.org/10.5194/acp-7-3969-2007, 2007. a
Li, K., Liggio*, J., Han, Ch., Liu, Q., Moussa, S. G., Lee, P. and Li, S.-M.: Understanding the Impact of High-NOx Conditions on the Formation of Secondary Organic Aerosol in the Photooxidation of Oil Sand-Related Precursors, Environ. Sci. Technol., 53, 14420–14429, https://doi.org/10.1021/acs.est.9b05404, 2019. a
Li, X., Wu, J., Elser, M., Feng, T., Cao, J., El-Haddad, I., Huang, R., Tie, X., Prévôt, A. S. H., and Li, G.: Contributions of residential coal combustion to the air quality in Beijing–Tianjin–Hebei (BTH), China: a case study, Atmos. Chem. Phys., 18, 10675–10691, https://doi.org/10.5194/acp-18-10675-2018, 2018. a
Li, Y., Zhang, J., Sailor, D. J., and Ban-Weiss, G. A.: Effects of urbanization on regional meteorology and air quality in Southern California, Atmos. Chem. Phys., 19, 4439–4457, https://doi.org/10.5194/acp-19-4439-2019, 2019. a, b, c
Liao, J., Wang, T., Wang, X., Xie, M., Jiang, Z., Huang, X. and Zhu, J.: Impacts of different urban canopy schemes in WRF/Chem on regional climate and air quality in Yangtze River Delta, China, Atmos. Res., 145–146, 226–243, https://doi.org/10.1016/j.atmosres.2014.04.005, 2014. a
Liaskoni, M., Huszar, P., Bartík, L., Prieto Perez, A. P., Karlický, J., and Vlček, O.: Modelling the European wind-blown dust emissions and their impact on particulate matter (PM) concentrations, Atmos. Chem. Phys., 23, 3629–3654, https://doi.org/10.5194/acp-23-3629-2023, 2023. a
Lin, J., Junling An, Yu Qu, Yong Chen, Ying Li, Yujia Tang, Feng Wang, and Weiling Xiang: Local and distant source contributions to secondary organic aerosol in the Beijing urban area in summer, Atmos. Environ., Volume, 124, Part B, 176–185, https://doi.org/10.1016/j.atmosenv.2015.08.098, 2016. a
Lin, Y.-C., Cheng, M.-T., Lin, W.-H., Lan, Y.-Y., and Tsuang, B.-J.: Causes of the elevated nitrate aerosol levels during episodic days in Taichung urban area, Taiwan, Atmos. Environ., 44, 1632–1640, https://doi.org/10.1016/j.atmosenv.2010.01.039, 2010. a
López-Romero, J. M., Montávez, J. P., Jerez, S., Lorente-Plazas, R., Palacios-Peña, L., and Jiménez-Guerrero, P.: Precipitation response to aerosol–radiation and aerosol–cloud interactions in regional climate simulations over Europe, Atmos. Chem. Phys., 21, 415–430, https://doi.org/10.5194/acp-21-415-2021, 2021. a
Ma, J., Zhu, S., Wang, S., Wang, P., Chen, J., and Zhang, H.: Impacts of land cover changes on biogenic emission and its contribution to ozone and secondary organic aerosol in China, Atmos. Chem. Phys., 23, 4311–4325, https://doi.org/10.5194/acp-23-4311-2023, 2023. a
Markakis, K., Valari, M., Perrussel, O., Sanchez, O., and Honore, C.: Climate-forced air-quality modeling at the urban scale: sensitivity to model resolution, emissions and meteorology, Atmos. Chem. Phys., 15, 7703–7723, https://doi.org/10.5194/acp-15-7703-2015, 2015. a, b, c
Martin, S. T., Hung, H.-M., Park, R. J., Jacob, D. J., Spurr, R. J. D., Chance, K. V., and Chin, M.: Effects of the physical state of tropospheric ammonium-sulfate-nitrate particles on global aerosol direct radiative forcing, Atmos. Chem. Phys., 4, 183–214, https://doi.org/10.5194/acp-4-183-2004, 2004. a
Mcdonald-Buller, E., Wiedinmyer, C., Kimura, Y. and Allen, D.: Effects of land use data on dry deposition in a regional photochemical model for eastern Texas, J. Air Waste Manage. Assoc., 51, 1211–1218, https://doi.org/10.1080/10473289.2001.10464340, 2001. a
Miao, Y., Che, H., Zhang, X., and Liu, S.: Integrated impacts of synoptic forcing and aerosol radiative effect on boundary layer and pollution in the Beijing–Tianjin–Hebei region, China, Atmos. Chem. Phys., 20, 5899–5909, https://doi.org/10.5194/acp-20-5899-2020, 2020. a
Nagori, J., Janssen, R. H. H., Fry, J. L., Krol, M., Jimenez, J. L., Hu, W., and Vilà-Guerau de Arellano, J.: Biogenic emissions and land–atmosphere interactions as drivers of the daytime evolution of secondary organic aerosol in the southeastern US, Atmos. Chem. Phys., 19, 701–729, https://doi.org/10.5194/acp-19-701-2019, 2019. a
Nenes, A., Pandis, S. N., and Pilinis, C.: ISORROPIA: a new thermodynamic equilibrium model for multiphase multicomponent inorganic aerosols, Aquat. Geochem., 4, 123–152, 1998. a
Nho-Kim, E.-Y., Michou, M., and Peuch, V.-H.: Parameterization of size-dependent particle dry deposition velocities for global modeling, Atmos. Environ., 38, 1933–1942, https://doi.org/10.1016/j.atmosenv.2004.01.002, 2004. a
Nowak, D. J. and Dwyer, J. F.: Understanding the Benefits and Costs of Urban Forest Ecosystems, In: Urban and Community Forestry in the Northeast, edited by: Kuser, J. E., Springer Netherlands, 25–46, https://doi.org/10.1007/978-1-4615-4191-2_2, 2007. a
Oke, T. R.: The energetic basis of the urban heat island, Q. J. Roy. Meteor. Soc., 108, 1–24, https://doi.org/10.1002/qj.49710845502, 1982. a, b
Oke, T., Mills, G., Christen, A., and Voogt, J.: Urban Climates, Cambridge University Press, https://doi.org/10.1017/9781139016476, 2017. a, b
Oleson, K. W., Bonan, G. B., Feddema, J., Vertenstein, M., and Grimmond, C. S. B.: An urban parameterization for a global climate model. 1. Formulation and evaluation for two cities. J. Appl. Meteor. Clim., 47, 1038–1060, 2008. a
Oleson, K. W., Bonan, G. B., Feddema, J., Vertenstein, M., and Kluzek, E.: Technical Description of an Urban Parameterization for the Community Land Model (CLMU), NCAR TECHNICAL NOTE NCAR/TN-480+STR, National Center for Atmospheric Research, Boulder, Co, USA, 61–88, https://doi.org/10.5065/D6K35RM9, 2010. a
Oleson, K., Lawrence, D. M., Bonan, G. B., Drewniak, B., Huang, M., Koven, C. D., Levis, S., Li, F., Riley, W. J., Subin, Z. M., Swenson, S. C., Thornton, P. E., Bozbiyik, A., Fisher, R., Heald, C. L., Kluzek, E., Lamarque, J.-F., Lawrence, P. J., Leung, L. R., Lipscomb, W., Muszala, S., Ricciuto, D. M., Sacks, W., Sun, Y., Tang, J., and Yang, Z.-L.: Technical Description of version 4.5 of the Community Land Model (CLM), NCAR Technical Note NCAR/TN-503+STR, Boulder, Colorado, 420 pp., https://doi.org/10.5065/D6RR1W7M, 2013. a
Ortega, A. M., Hayes, P. L., Peng, Z., Palm, B. B., Hu, W., Day, D. A., Li, R., Cubison, M. J., Brune, W. H., Graus, M., Warneke, C., Gilman, J. B., Kuster, W. C., de Gouw, J., Gutiérrez-Montes, C., and Jimenez, J. L.: Real-time measurements of secondary organic aerosol formation and aging from ambient air in an oxidation flow reactor in the Los Angeles area, Atmos. Chem. Phys., 16, 7411–7433, https://doi.org/10.5194/acp-16-7411-2016, 2016. a
Panagi, M., Fleming, Z. L., Monks, P. S., Ashfold, M. J., Wild, O., Hollaway, M., Zhang, Q., Squires, F. A., and Vande Hey, J. D.: Investigating the regional contributions to air pollution in Beijing: a dispersion modelling study using CO as a tracer, Atmos. Chem. Phys., 20, 2825–2838, https://doi.org/10.5194/acp-20-2825-2020, 2020. a
Passant, N.: Speciation of UK Emissions of Non-methane Volatile Organic Compounds, DEFRA, Oxon, UK, https://uk-air.defra.gov.uk/assets/documents/reports/empire/AEAT_ENV_0545_final_v2.pdf (last access: 4 January 2024), 2002. a
Prieto Pérez, A. P., Huszár, P. and Karlický, J.: Extreme PM and Ozone Pollution over Central Europe: Interactions of the Urban Canopy Meteorological Forcing and Radiative Effects of Urban Emissions in WDS'22 Proceedings of Contributed Papers — Physics, edited by: Šafránková, J. and Pavlů, J., Prague, Matfyzpress, 204–210 pp., ISBN 978-80-7378-477-5, 2022. a
Pullinen, I., Schmitt, S., Kang, S., Sarrafzadeh, M., Schlag, P., Andres, S., Kleist, E., Mentel, T. F., Rohrer, F., Springer, M., Tillmann, R., Wildt, J., Wu, C., Zhao, D., Wahner, A., and Kiendler-Scharr, A.: Impact of NOx on secondary organic aerosol (SOA) formation from α-pinene and β-pinene photooxidation: the role of highly oxygenated organic nitrates, Atmos. Chem. Phys., 20, 10125–10147, https://doi.org/10.5194/acp-20-10125-2020, 2020. a
Putaud, J.-P., Pisoni, E., Mangold, A., Hueglin, C., Sciare, J., Pikridas, M., Savvides, C., Ondracek, J., Mbengue, S., Wiedensohler, A., Weinhold, K., Merkel, M., Poulain, L., van Pinxteren, D., Herrmann, H., Massling, A., Nordstroem, C., Alastuey, A., Reche, C., Pérez, N., Castillo, S., Sorribas, M., Adame, J. A., Petaja, T., Lehtipalo, K., Niemi, J., Riffault, V., de Brito, J. F., Colette, A., Favez, O., Petit, J.-E., Gros, V., Gini, M. I., Vratolis, S., Eleftheriadis, K., Diapouli, E., Denier van der Gon, H., Yttri, K. E., and Aas, W.: Impact of 2020 COVID-19 lockdowns on particulate air pollution across Europe, EGUsphere [preprint], https://doi.org/10.5194/egusphere-2023-434, 2023. a
Ramboll: CAMx User's Guide, Comprehensive Air Quality model with Extentions, version 7.10, Novato, California, https://camx-wp.azurewebsites.net/Files/CAMxUsersGuide_v7.10.pdf (last access: 9 January 2024), 2020a. a, b
Ramboll: CAMx version 7.10, Ramboll [code], https://www.camx.com/download/ (last access: 16 May 2023), 2020b. a
Ren, Y., Zhang, H., Wei, W., Wu, B., Cai, X., and Song, Y.: Effects of turbulence structure and urbanization on the heavy haze pollution process, Atmos. Chem. Phys., 19, 1041–1057, https://doi.org/10.5194/acp-19-1041-2019, 2019. a
Ribeiro, F. N. D., de Oliveira, A. P., Soares, J., de Miranda, R. M., Barlage, M., and Chen, F.: Effect of sea breeze propagation on the urban boundary layer of the metropolitan region of Sao Paulo, Brazil, Atmos. Res., 214, 174–188, https://doi.org/10.1016/j.atmosres.2018.07.015, 2018. a
Richards, K.: Observation and simulation of dew in rural and urban environments, Prog. Phys. Geogr., 28, 76–94, 2004. a
Rienda, I. C. and Alves, C. A.: Road dust resuspension: A review, Atmos. Res., 261, 105740, https://doi.org/10.1016/j.atmosres.2021.105740, 2021. a
Rivellini, L.-H., Adam, M. G., Kasthuriarachchi, N., and Lee, A. K. Y.: Characterization of carbonaceous aerosols in Singapore: insight from black carbon fragments and trace metal ions detected by a soot particle aerosol mass spectrometer, Atmos. Chem. Phys., 20, 5977–5993, https://doi.org/10.5194/acp-20-5977-2020, 2020. a
Sartelet, K. N., Couvidat, F., Seigneur, C., and Roustan, Y.: Impact of biogenic emissions on air quality over Europe and North America, Atmos. Environ., 53, 131–141, https://doi.org/10.1016/j.atmosenv.2011.10.046, 2012. a
Seinfeld, J. H. and Pandis, S. N.: Atmospheric Chemistry and Physics: From Air Pollution to Climate Change, J. Wiley, New York, ISBN 978-1-118-94740-1, 1998. a, b
Simmons, A. J., Willett, K. M., Jones, P. D., Thorne, P. W., and Dee, D. P.: Low-frequency variations in surface atmospheric humidity, temperature and precipitation: inferences from reanalyses and monthly gridded observational datasets, J. Geophys. Res., 115, D01110, https://doi.org/10.1029/2009JD012442, 2010. a
Sindelarova, K., Granier, C., Bouarar, I., Guenther, A., Tilmes, S., Stavrakou, T., Müller, J.-F., Kuhn, U., Stefani, P., and Knorr, W.: Global data set of biogenic VOC emissions calculated by the MEGAN model over the last 30 years, Atmos. Chem. Phys., 14, 9317–9341, https://doi.org/10.5194/acp-14-9317-2014, 2014. a
Sindelarova, K., Markova, J., Simpson, D., Huszar, P., Karlicky, J., Darras, S., and Granier, C.: High-resolution biogenic global emission inventory for the time period 2000–2019 for air quality modelling, Earth Syst. Sci. Data, 14, 251–270, https://doi.org/10.5194/essd-14-251-2022, 2022. a
Skyllakou, K., Murphy, B. N., Megaritis, A. G., Fountoukis, C., and Pandis, S. N.: Contributions of local and regional sources to fine PM in the megacity of Paris, Atmos. Chem. Phys., 14, 2343–2352, https://doi.org/10.5194/acp-14-2343-2014, 2014. a, b, c, d, e
Slater, J., Coe, H., McFiggans, G., Tonttila, J., and Romakkaniemi, S.: The effect of BC on aerosol–boundary layer feedback: potential implications for urban pollution episodes, Atmos. Chem. Phys., 22, 2937–2953, https://doi.org/10.5194/acp-22-2937-2022, 2022. a
Sokhi, R. S., Moussiopoulos, N., Baklanov, A., Bartzis, J., Coll, I., Finardi, S., Friedrich, R., Geels, C., Grönholm, T., Halenka, T., Ketzel, M., Maragkidou, A., Matthias, V., Moldanova, J., Ntziachristos, L., Schäfer, K., Suppan, P., Tsegas, G., Carmichael, G., Franco, V., Hanna, S., Jalkanen, J.-P., Velders, G. J. M., and Kukkonen, J.: Advances in air quality research – current and emerging challenges, Atmos. Chem. Phys., 22, 4615–4703, https://doi.org/10.5194/acp-22-4615-2022, 2022. a, b
Song, J., Webb, A., Parmenter, B. Allen, D. T., and McDonald-Buller, E.: Environmental Sci. Tech., 42, 7294–7300, https://doi.org/10.1021/es800645j, 2008. a, b, c
Stock, Z. S., Russo, M. R., Butler, T. M., Archibald, A. T., Lawrence, M. G., Telford, P. J., Abraham, N. L., and Pyle, J. A.: Modelling the impact of megacities on local, regional and global tropospheric ozone and the deposition of nitrogen species, Atmos. Chem. Phys., 13, 12215–12231, https://doi.org/10.5194/acp-13-12215-2013, 2013. a, b
Strader, R. Lurmann, F. and Pandis, S. N.: Evaluation of secondary organic aerosol formation in winter, Atmos. Environ., 33., 4849–4863, 1999. a
Struzewska, J. and Kaminski, J. W.: Impact of urban parameterization on high resolution air quality forecast with the GEM – AQ model, Atmos. Chem. Phys., 12, 10387–10404, https://doi.org/10.5194/acp-12-10387-2012, 2012. a
Tao, W., Liu, J., Ban-Weiss, G. A., Hauglustaine, D. A., Zhang, L., Zhang, Q., Cheng, Y., Yu, Y., and Tao, S.: Effects of urban land expansion on the regional meteorology and air quality of eastern China, Atmos. Chem. Phys., 15, 8597–8614, https://doi.org/10.5194/acp-15-8597-2015, 2015. a
Thunis, P., Clappier, A., de Meij, A., Pisoni, E., Bessagnet, B., and Tarrason, L.: Why is the city's responsibility for its air pollution often underestimated? A focus on PM2.5, Atmos. Chem. Phys., 21, 18195–18212, https://doi.org/10.5194/acp-21-18195-2021, 2021. a, b, c
Tie, X., Brasseur, G., and Ying, Z.: Impact of model resolution on chemical ozone formation in Mexico City: application of the WRF-Chem model, Atmos. Chem. Phys., 10, 8983–8995, https://doi.org/10.5194/acp-10-8983-2010, 2010. a
Tie, X., Geng, F., Guenther, A., Cao, J., Greenberg, J., Zhang, R., Apel, E., Li, G., Weinheimer, A., Chen, J., and Cai, C.: Megacity impacts on regional ozone formation: observations and WRF-Chem modeling for the MIRAGE-Shanghai field campaign, Atmos. Chem. Phys., 13, 5655–5669, https://doi.org/10.5194/acp-13-5655-2013, 2013. a
Tiedtke, M.: A Comprehensive Mass Flux Scheme for Cumulus Parameterization in Large-Scale Models, Mon. Weather Rev., 117, 1779–1800, https://doi.org/10.1175/1520-0493(1989)117, 1989. a
Timothy, M. and Lawrence, M. G.: The influence of megacities on global atmospheric chemistry: a modeling study, Environ. Chem., 6, 219–225, https://doi.org/10.1071/EN08110, 2009. a
Ulpiani, G.:On the linkage between urban heat island and urban pollution island: Three-decade literature review towards a conceptual framework, Sci. Total Environ., 751, 141727, https://doi.org/10.1016/j.scitotenv.2020.141727, 2021. a
United Nations: The World's Cities in 2018 – Data Booklet (ST/ESA/SER.A/417), United Nations, Department of Economic and Social Affairs, Population Division, New York, USA, https://www.un.org/en/development/desa/population/publications/pdf/urbanization/the_worlds_cities_in_2018_data_booklet.pdf (last access: 10 January 2024), 2018. a
van der Gon, H. D., Hendriks, C., Kuenen, J., Segers, A., and Visschedijk, A.: Description of current temporal emission patterns and sensitivity of predicted AQ for temporal emission patterns. EU FP7 MACC deliverable report D_D-EMIS_1.3, https://atmosphere.copernicus.eu/sites/default/files/2019-07/MACC_TNO_del_1_3_v2.pdf (last access: 10 January 2024), 2011. a
Vestreng, V., Myhre, G., Fagerli, H., Reis, S., and Tarrasón, L.: Twenty-five years of continuous sulphur dioxide emission reduction in Europe, Atmos. Chem. Phys., 7, 3663–3681, https://doi.org/10.5194/acp-7-3663-2007, 2007. a
Walters, W. W., Karod, M., Willcocks, E., Baek, B. H., Blum, D. E., and Hastings, M. G.: Quantifying the importance of vehicle ammonia emissions in an urban area of northeastern USA utilizing nitrogen isotopes, Atmos. Chem. Phys., 22, 13431–13448, https://doi.org/10.5194/acp-22-13431-2022, 2022. a
Wang, X., Wu, Z., Liang, G.: WRF/CHEM modeling of impacts of weather conditions modified by urban expansion on secondary organic aerosol formation over Pearl River Delta, Particuology, 7, 384–391, https://doi.org/10.1016/j.partic.2009.04.007, 2009. a
Wang, M., Tang, G., Liu, Y., Ma, M., Yu, M., Hu, B., Zhang, Y., Wang, Y., and Wang, Y.: The difference in the boundary layer height between urban and suburban areas in Beijing and its implications for air pollution, Atmos. Environ., 260, 118552, https://doi.org/10.1016/j.atmosenv.2021.118552, 2021. a
Wang, J., Xing, J., Wang, S., Mathur, R., Wang, J., Zhang, Y., Liu, C., Pleim, J., Ding, D., Chang, X., Jiang, J., Zhao, P., Sahu, S. K., Jin, Y., Wong, D. C., and Hao, J.: The pathway of impacts of aerosol direct effects on secondary inorganic aerosol formation, Atmos. Chem. Phys., 22, 5147–5156, https://doi.org/10.5194/acp-22-5147-2022, 2022. a
Wesely, M. L.: Parameterization of Surface Resistances to Gaseous Dry Deposition in Regional-Scale Numerical Models. Atmos. Environ., 23, 1293–1304, 1989. a
Wei, W., Zhang, H., Wu, B., Huang, Y., Cai, X., Song, Y., and Li, J.: Intermittent turbulence contributes to vertical dispersion of PM2.5 in the North China Plain: cases from Tianjin, Atmos. Chem. Phys., 18, 12953–12967, https://doi.org/10.5194/acp-18-12953-2018, 2018. a, b
Xue, L. K., Wang, T., Gao, J., Ding, A. J., Zhou, X. H., Blake, D. R., Wang, X. F., Saunders, S. M., Fan, S. J., Zuo, H. C., Zhang, Q. Z., and Wang, W. X.: Ground-level ozone in four Chinese cities: precursors, regional transport and heterogeneous processes, Atmos. Chem. Phys., 14, 13175–13188, https://doi.org/10.5194/acp-14-13175-2014, 2014. a
Yang, F., Tan, J., Zhao, Q., Du, Z., He, K., Ma, Y., Duan, F., Chen, G., and Zhao, Q.: Characteristics of PM2.5 speciation in representative megacities and across China, Atmos. Chem. Phys., 11, 5207–5219, https://doi.org/10.5194/acp-11-5207-2011, 2011. a
Yang, J., Ma, L., He, X., Au, W. C., Miao, Y., Wang, W.-X., and Nah, T.: Measurement report: Abundance and fractional solubilities of aerosol metals in urban Hong Kong – insights into factors that control aerosol metal dissolution in an urban site in South China, Atmos. Chem. Phys., 23, 1403–1419, https://doi.org/10.5194/acp-23-1403-2023, 2023. a
Yienger, J. J. and Levy, H.: Empirical model of global soil-biogenic NOx emissions, J. Geophys. Res.-Atmos., 100, 11447–11464, https://doi.org/10.1029/95JD00370, 1995. a
Yim, S. H. L., Wang, M., Gu, Y., Yang, Y., Dong, G., and Li, Q.: Effect of urbanization on ozone and resultant health effects in the Pearl River Delta region of China, J. Geophys. Res.-Atmos., 124, 11568–11579, https://doi.org/10.1029/2019JD030562, 2019. a
Yu, M., Tang, G., Yang, Y., Li, Q., Wang, Y., Miao, S., Zhang, Y., and Wang, Y.: The interaction between urbanization and aerosols during a typical winter haze event in Beijing, Atmos. Chem. Phys., 20, 9855–9870, https://doi.org/10.5194/acp-20-9855-2020, 2020. a
Zha, J., Zhao, D., Wu, J. and Zhang, P.: Numerical simulation of the effects of land use and cover change on the near-surface wind speed over Eastern China, Clim. Dynam., 53, 1783–1803, https://doi.org/10.1007/s00382-019-04737-w, 2019. a
Zhai, M., Kuang, Y., Liu, L., He, Y., Luo, B., Xu, W., Tao, J., Zou, Y., Li, F., Yin, C., Li, C., Xu, H., and Deng, X.: Insights into characteristics and formation mechanisms of secondary organic aerosols in the Guangzhou urban area, Atmos. Chem. Phys., 23, 5119–5133, https://doi.org/10.5194/acp-23-5119-2023, 2023. a
Zhang, L., Gong, S., Padro, J. and Barrie. L.: A size-segregated particle dry deposition scheme for an atmospheric aerosol module, Atmos. Environ., 35, 549–560, 2001. a, b, c
Zhang, L., Brook, J. R., and Vet, R.: A revised parameterization for gaseous dry deposition in air-quality models, Atmos. Chem. Phys., 3, 2067–2082, https://doi.org/10.5194/acp-3-2067-2003, 2003. a, b, c, d, e, f
Zhang, Q. J., Beekmann, M., Freney, E., Sellegri, K., Pichon, J. M., Schwarzenboeck, A., Colomb, A., Bourrianne, T., Michoud, V., and Borbon, A.: Formation of secondary organic aerosol in the Paris pollution plume and its impact on surrounding regions, Atmos. Chem. Phys., 15, 13973–13992, https://doi.org/10.5194/acp-15-13973-2015, 2015. a
Zhong, S., Qian, Y., Sarangi, C., Zhao, C., Leung, R., Wang, H., et al.: Urbanization effect on winter haze in the Yangtze River Delta region of China, Geophys. Res.-Lett, 45, 6710–6718, https://doi.org/10.1029/2018GL077239, 2018. a
Zhong, Q., Shen, H., Yun, X., Chen, Y., Ren, Y., Xu, H., Shen, G., Du, W., Meng, J., Li, W., Ma, J., and Tao, S.: Global Sulfur Dioxide Emissions and the Driving Forces, Environ. Sci. Technol., 54, 6508–6517, https://doi.org/10.1021/acs.est.9b07696, 2020. a
Zhu, K., Xie, M., Wang, T., Cai, J., Li, S., and Feng, W.: A modeling study on the effect of urban land surface forcing to regional meteorology and air quality over South China, Atmos. Environ., 152, 389–404, https://doi.org/10.1016/j.atmosenv.2016.12.053, 2017. a, b