the Creative Commons Attribution 4.0 License.
the Creative Commons Attribution 4.0 License.
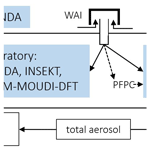
The Puy de Dôme ICe Nucleation Intercomparison Campaign (PICNIC): comparison between online and offline methods in ambient air
Michael P. Adams
Kevin Barry
Barbara Bertozzi
Heinz Bingemer
Cristian Boffo
Yannick Bras
Nicole Büttner
Dimitri Castarede
Daniel J. Cziczo
Paul J. DeMott
Romy Fösig
Megan Goodell
Kristina Höhler
Thomas C. J. Hill
Conrad Jentzsch
Luis A. Ladino
Ezra J. T. Levin
Stephan Mertes
Ottmar Möhler
Kathryn A. Moore
Benjamin J. Murray
Jens Nadolny
Tatjana Pfeuffer
David Picard
Carolina Ramírez-Romero
Mickael Ribeiro
Sarah Richter
Jann Schrod
Karine Sellegri
Frank Stratmann
Benjamin E. Swanson
Erik S. Thomson
Heike Wex
Martin J. Wolf
Evelyn Freney
Ice crystal formation in mixed-phase clouds is initiated by specific aerosol particles, termed ice-nucleating particles (INPs). Only a tiny fraction of all aerosol particles are INPs, providing a challenge for contemporary INP measurement techniques. Models have shown that the presence of INPs in clouds can impact their radiative properties and induce precipitation formation. However, for a qualified implementation of INPs in models, measurement techniques able to accurately detect the temperature-dependent INP concentration are needed. Here we present measurements of INP concentrations in ambient air under conditions relevant to mixed-phase clouds from a total of 10 INP methods over 2 weeks in October 2018 at the Puy de Dôme observatory in central France. A special focus in this intercomparison campaign was placed on having overlapping sampling periods. Although a variety of different measurement principles were used, the majority of the data show INP concentrations within a factor of 5 of one another, demonstrating the suitability of the instruments to derive model-relevant INP data.
Lower values of comparability are likely due to instrument-specific features such as aerosol lamina spreading in continuous-flow diffusion chambers, demonstrating the need to account for such phenomena when interpreting INP concentration data from online instruments. Moreover, consistently higher INP concentrations were observed from aerosol filters collected on the rooftop at the Puy de Dôme station without the use of an aerosol inlet.
- Article
(4579 KB) - Full-text XML
- BibTeX
- EndNote
The first formation of ice in mixed-phase clouds is triggered by specific aerosol particles, called ice-nucleating particles (INPs; Vali et al., 2015). The presence of INPs is important for the formation and further development of clouds, since they can determine cloud phase (e.g. by a rapid cloud glaciation and associated dissipation effect; Campbell and Shiobara, 2008; Murray et al., 2012; Paukert and Hoose, 2014; Kalesse et al., 2016; Desai et al., 2019; Murray and Liu, 2022; Carlsen and David, 2022; Creamean et al., 2022; Sze et al., 2023) and related radiative properties (e.g. Vergara-Temprado et al., 2018). In addition, INPs have an impact on precipitation formation (e.g. Mülmenstädt et al., 2015; Field and Heymsfield, 2015; Fan et al., 2017). However, the identification and quantification of ambient INPs remain challenging due to their rarity (Kanji et al., 2017) and limitations in measurement techniques (DeMott et al., 2017; Cziczo et al., 2017). For a better integration of INPs in models that is required to improve the representation of ice crystal formation and evolution in clouds (e.g. Coluzza et al., 2017; Burrows et al., 2022), a certain precision in INP measurement techniques is required, as studies have shown that a variability in the temperature-dependent INP number concentration impacts the representation of cloud properties (e.g. Phillips et al., 2003; Ervens et al., 2011; Tan et al., 2016; Vergara-Temprado et al., 2018; French et al., 2018).
Different methods to quantify ambient INP concentrations exist and are categorized into online instruments and offline freezing techniques. Online instruments measure real-time INP concentrations with a high temporal resolution (seconds to minutes). It has been shown that INP concentration can fluctuate considerably within short sampling times of minutes and hours (e.g. Prenni et al., 2009; Lacher et al., 2017; Welti et al., 2018; Paramonov et al., 2020). Therefore, online methods are required to catch such variability and relate it to, for example, changes in air mass and aerosol properties. On the other hand, currently available online instruments for ambient measurements typically sample only a few litres of air per minute. This limits the ability of these methods to detect low INP concentrations in ambient air. Offline methods are based on collecting aerosol particles on sampling substrates or into liquids, typically over longer periods of hours to days, and therefore can collect larger volumes of air (∼m3), increasing the likelihood of sampling the very rare INPs active at the highest temperatures. Results from offline INP measurements can also be obtained for shorter periods; however, this impacts the limit of detection and may lead to a lower or even zero number of very rare INPs. Due to the labour-intensive filter collection and analysis procedures, online methods are often preferred to measure INPs with a high time resolution. While offline INP analysis could impact the properties of the collected INPs due to the sampling and analysis procedure (e.g. physical or chemical alteration, particle breakup, and loss of coating material), they also allow for special treatments, for example, investigating the contribution of organic INPs by heat or peroxide treatments (e.g. Hill et al., 2016), to improve our understanding of the INP properties.
In order to accurately quantify INPs, existing methods need to be validated and compared with each other to address potential systematic biases. A set of different methods were compared in laboratory studies using well-known aerosol particles, e.g. by sharing samples of Snomax®, cellulose, or illite-rich samples amongst the community of the Ice Nuclei Research Unit (INUIT; Wex et al., 2015; Hiranuma et al., 2015, 2019), during the Leipzig Ice Nucleation chamber Comparison (LINC; Burkert-Kohn et al., 2017), and during the Fifth International Workshop on Ice Nucleation phase 2 (FIN-02; DeMott et al., 2018). Those experiments revealed a generally good agreement among a large set of freezing methods. Hiranuma et al. (2015) indicated that the aerosol particle generation method (dry versus wet suspension) can lead to changes in detected INP concentrations, which was also found by other laboratory studies (Emersic et al., 2016; Boose et al., 2016b). Moreover, in these studies, it was shown that the methods' comparability depended on the chosen aerosol particle type and nucleation temperature; below −10 °C, instruments showed good agreement using Snomax® and natural dust samples. Discrepancies occurred when using Snomax® above −10 °C, with illite NX (NX Nanopowder, Arginotec) above −25 °C, and with potassium feldspar between −20 and −25 °C.
Another aspect that is crucial for the intercomparison of ice nucleation techniques is the size range of aerosol particles that are INPs. Typically, online instruments, such as continuous flow diffusion chambers (CFDCs), limit the aerosol sampling to size to diameters below ∼3 µm (e.g. Rogers et al., 2001), as they commonly aim at measuring freshly formed ice crystals within the chamber using optical particle counters (OPCs), and a size overlap with unactivated large aerosol particles must be avoided because optical size alone is often the basis for distinguishing frozen and unfrozen particles. By contrast, offline techniques are able to sample those larger aerosol particles, e.g. using inline or open-faced filter holders. Many of these techniques collect aerosol particles on filters, which could lead to a reduced sampling of particles smaller than the pore size. However, theoretical calculations indicate that most particles smaller than the pore size will be sampled (Spurny and Lodge, 1972), and in a study by Ogura et al. (2016), it was found that ∼70 % of particles smaller than 100 nm are collected on the direct surface of 200 nm Nuclepore filters. Also, Soo et al. (2016) report that filters can have a high collection efficiency for particles much smaller than their nominal pore size, depending on the filter material and sampling flow. Moreover, not all particles may be released completely from the filter during the washing-off procedure before analysis, and particle collection efficiency can also be reduced by a possible bounce from the collection substrate when using stage impactors. Thus, the role of the dominant size of INPs is an important aspect to assess the suitability of an INP method to capture the picture of ambient conditions. Super-micrometre particles have been found to contribute to the majority of INPs in different studies in North America and Europe (Mason et al., 2016), the Arctic (Creamean et al., 2018), Cabo Verde islands (Gong et al., 2020), and the Yucatán Peninsula (Córdoba et al., 2021); however, this occurs with a varying fraction, potentially depending on the sampling location, the aerosol type, and the nucleation temperature. Contrastingly, the analysis of ice crystal residuals in the lower free troposphere revealed that the majority of particles were submicron in size (e.g. Mertes et al., 2007; Schmidt et al., 2017). Ice-active organic particles from marine sources were found to be submicron (Wilson et al., 2015) and super-micrometre (Mitts et al., 2021) in size, and it is unclear which size range is dominating the INP population in such remote marine environments. In laboratory-based intercomparison studies, it was suggested that generally good agreement between methods was achieved by controlling the aerosol particle size distributions used for the INP experiments (Wex et al., 2015; DeMott et al., 2018; Burkert-Kohn et al., 2017). At ambient conditions, however, aerosol particles and INPs can span a wide size range, which can be crucial for determining the real ambient INP concentration and for comparing INP measurement techniques that cover different size ranges (Knopf et al., 2018). This may be especially relevant for mineral dust, which is acknowledged to be a key INP in the troposphere at temperatures below −15 °C (e.g. Atkinson et al., 2013). The occurrence of super-micrometre dust particles close to emission sources is certainly higher compared to locations further away.
Ambient INP concentrations can be close to typical instrument detection limits (Boose et al., 2016a), and the way measurements close to detection limits are considered for averaging INP concentration over longer sampling intervals, which can be done for comparing different instruments, is another important aspect of making ambient measurements. Ambient INPs show a wide range of concentration across the relevant temperature range (e.g. Kanji et al., 2017), and it should be ensured that even low numbers of INPs, close to instruments' detection limits, are captured.
By conducting measurements on ambient aerosols, impacts from aerosol generation methods and domination by a single INP type are avoided, and the instruments are compared under realistic conditions such as the naturally low INP number concentration. DeMott et al. (2017) presented a field-based intercomparison campaign using four offline techniques and an online instrument (Colorado State University Continuous Flow Diffusion Chamber, CSU-CFDC) at different locations in the western USA, including agricultural areas, mountainous desert regions, and a coastal site. They generally found good agreement between instruments, especially when measurements were performed synchronously. However, a high bias for offline methods, sampling particles onto filters or into a bulk liquid, against an online method was observed below −20 °C. It is unclear if this might have been caused by a breakup of aggregates by partial solvation of aerosols that contain more than one INP or if larger INPs were not captured by the online method used in that study. In a recent study by Brasseur et al. (2022) in the Finnish boreal forest, three online instruments were compared over 4 d at a nucleation temperature below −29 °C and generally showed a good agreement. Such intercomparison efforts need to be expanded to cover the full range of mixed-phase cloud temperatures and conducted in environments in which mixed-phase clouds occur. INP intercomparison activities are especially relevant due to ongoing efforts for the establishment of INP monitoring networks. For example, at the European level, the ACTRIS (Aerosol, Clouds and Trace Gases Research Infrastructure) Topical Centre for Cloud In Situ Measurements (CIS) is currently in an implementation phase to include INP concentration as a parameter to be monitored at specific research stations. For such an effort, it is crucial to ensure that INP concentrations are accurately quantified using different online or offline instruments. This will contribute to developing harmonized data sets.
Here we present results from the Puy de Dôme ICe Nucleation Intercomparison Campaign (PICNIC). The Puy de Dôme station is a mountaintop station situated in central France at an altitude of 1465 m above sea level (a.s.l.). Given its altitude, it is often affected by air masses transported over long distances and, hence, can contain aerosol particles emitted from source regions far away. It is also an environment in which clouds form and occur; thus, the aerosol population being present at the Puy de Dôme is relevant for aerosol–cloud interactions. During PICNIC, seven offline techniques and three online instruments were compared over 14 d in October 2018. The aim here was to test the measurement techniques against each other in their original operation configuration, as each of them are well-established methods and were used already in several campaigns, and we wanted to create a link between these activities without changing measurement protocols. A key aspect is that offline and online instruments were intercompared during the same filter sampling time (offline instruments) or within 10 min (online instruments). Only when intercomparing the online to the offline methods were the time intervals not perfectly overlapping. Moreover, two main sampling locations inside the laboratory, via a total aerosol inlet, and one location directly outside on the laboratory's rooftop were used, addressing potential sampling biases due to particle losses in the inlet and by the use of upstream impactors necessary for some online instruments. Advances over past studies come from the use of a larger suite of methods and coordination of longer and shared sampling times.
2.1 Measurement location and time
The PICNIC campaign took place from 7 to 20 October 2018 at the Puy de Dôme (1465 m a.s.l.), which is located in central France. An overview of the measurement campaign will be presented by Freney et al. (in preparation), and some details are given by Bras et al. (2022). The station is located on a mountain chain; thus, the site is suitable for sampling atmospheric layers originating in the boundary layer, as well as in the lower free troposphere (Asmi et al., 2012; Farah et al., 2018; Baray et al., 2020). The site is operated by the Observatoire du Physique du Globe de Clermont-Ferrand (OPGC) and run by the Laboratoire de Météorologie Physique (LaMP) and is an observational facility of the ACTRIS (https://www.actris.eu/, last access: 21 February 2024) and the Global Atmospheric Watch measurement programmes. Continuous measurements of meteorological conditions, as well as aerosol physical and chemical properties, are provided. The submicron aerosol particle size distribution was measured using a custom-made scanning mobility particle sizer (with a particle diameter range from 10–560 nm) operated with a condensation particle counter (CPC; model 3010, TSI) via a whole-air inlet (WAI), with a 50 % cut size diameter of 30 µm. Also, aerosol particle concentrations between 0.5 and 2.5 µm were sporadically derived from the optical particle counter (OPC) of the CSU-CFDC (see Sect. 2.2.1) and corrected for a growth factor based on an assumption of ammonium sulfate composition.
Moreover, the transmission efficiency of the WAI is dependent on wind speed. Calculations show that at values of 7 (10) m s−1, 93 % (84 %) of the particles with a diameter of 10 µm are entering the inlet (Hangal and Willeke, 1990; Baron and Willeke, 2002). INP concentration measurements were conducted inside the laboratory, via two identical WAIs, as well as on the rooftop (Fig. 1). Full details on the measurement set-up of all online and offline techniques are provided in the following section.
In this study, we consider an agreement of INP concentration measurements if observations are consistent within factors of 2 and 5. It was indicated that the representations of INPs in models need to be predictable within a factor of 10 to not change cloud microphysics (Phillips et al., 2003), and our chosen values of 2 and 5 are thus even more conservative and can be considered to represent a good (factor 2) and agreeable (factor 5) comparison.
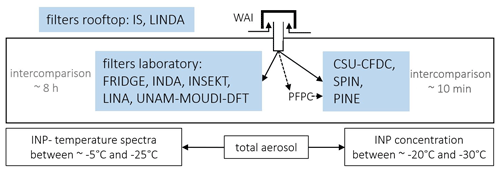
Figure 1Set-up of online instruments CSU-CFDC (Colorado State University Continuous Flow Diffusion Chamber), SPIN (Spectrometer for Ice Nuclei), and PINE (Portable Ice Nucleation Experiment), as well as filter collection for offline freezing analysis FRIDGE (FRankfurt Ice nucleation Deposition freezinG Experiment), INDA (Ice Nucleation Droplet Array), INSEKT (Ice Nucleation Spectrometer of the Karlsruhe Institute of Technology), IS (Ice Spectrometer), LINA (Leipzig Ice Nucleation Array), LINDA (LED-based Ice Nucleation Detection Apparatus), and the UNAM–MOUDI–DFT (Universidad Nacional Autónoma de México–Micro-Orifice Uniform Deposit Impactor–Droplet Freezing technique). Filters were collected and compared for consecutive 8 h. Online INP measurements are compared within a time span of 10 min. PINE partly joined the offline intercomparison, measuring at a constant temperature during the 8 h. Online instruments measured partly behind the Portable Fine Particle Concentrator (PFPC; Gute et al., 2019).
2.2 Online measurement techniques
Three different online INP instruments were operated behind the WAI in parallel for several hours per day. INP concentrations were determined for single particles activating in a temperature range between ∼ −20 and −30 °C, in the condensation and/or immersion freezing mode (via controlling processing relative humidity). All INP concentrations are referenced to standard litres sampled. For the intercomparison of these instruments, INP concentrations are only considered when measured within ±1 °C and within ±10 min. This aims to reduce any potential impact of a change in the sampled INP population at presumably nearly identical sampling conditions. Based on the PINE (Portable Ice Nucleation Experiment) data collected during this campaign, an average increase of 1.7 in INP concentration was observed for an increase in nucleation temperature by 1 °C. This factor of ∼1.7 is below the chosen factor of 2 to determine a good agreement between the online instruments. We acknowledge that a ±1 °C range can lead to variations in detected INP concentrations; however, a more restrictive approach would further limit the number of comparable data points.
During specific periods, online INP measurements were conducted downstream of the Portable Fine Particle Concentrator (PFPC; Gute et al., 2019), which is optimized for concentrating aerosol particles >0.1 µm. The PFPC was deployed at a separate inlet and used an impactor with a 50 % size cut at 2.5 µm. The inlet and outlet flow of the PFPC were kept at the same values, as described by Gute et al. (2019), i.e. 250 and 10 L min−1, respectively. Aerosol particles are concentrated with factors of ∼20 for particles >0.5 µm and with lower values for smaller particles. Since the INPs can be of sizes below and above 0.5 µm, INPs can be concentrated with variable factors (INP concentration factor). For the intercomparison between the online INP instruments, the same INP concentration factors were applied for simultaneous measurements. This did not have an impact on the instruments' comparability, given that the instruments did not use additional impactors smaller than the PFPC's impactor, with a size cut of 2.5 µm. The INP concentration factor used for the online intercomparison is thereby a campaign average of 11.4 and has a standard deviation of 1.7. This INP concentration factor was inferred by consecutive measurements with the concentrator turned on and off sequentially, using CSU-CFDC, which performed such measurements most frequently. The average concentration factor derived with PINE was similar (campaign average 10.9) but with a higher standard deviation (5.8), which might arise from the fact that PINE does not use an impactor when not sampling at the concentrator, such that larger particles that are ice-active can enter the instrument and contribute to more variation in the measured INP concentrations. For the comparison to the filter-based offline INP concentrations, a daily average INP concentration factor from CSU-CFDC was used to convert concentrated to ambient INP concentrations when sampling was from the PFPC. This daily average INP concentration factor ranged from values of 8.5 to 16.5, reflecting the variability in the sizes of INPs present at different times.
The instrument specifications are summarized in Table 1 and are explained in more detail in the following.
Table 1Specifications of the online instruments.
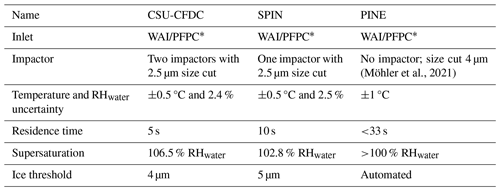
* Online instrument always sampled at the same inlet.
2.2.1 The Colorado State University Continuous Flow Diffusion Chamber (CSU-CFDC)
The CSU-CFDC is the longest-existing instrument for online detection of ambient INPs, with a legacy of versions for ground- and aircraft-based measurements starting from the late 1980s (Rogers, 1988; Rogers et al., 2001; DeMott et al., 2018). Its working principle is based on the establishment of supersaturated water and ice conditions in flowing air between two ice-coated walls of cylindrical shape in a vertical orientation. Those walls are held at different temperatures, and while the air temperature in the central lamina region is a linear function between these temperatures, the water vapour pressure is a non-linear function of temperature, resulting in a supersaturated region with respect to ice and water between the walls. The aerosol lamina is surrounded by particle-free sheath air through this region, where particles can activate into water droplets and ice crystals. While cloud droplets are evaporated downstream using an evaporation section, the remaining ice crystals are detected by their larger size using an OPC (Climet CI-3100). The size threshold to determine ice crystals was thereby 4 µm. The CSU-CFDC uses a pair of single-jet impactors upstream of the chamber for this study, with inserts defining 50 % aerodynamic size cuts at 2.5 µm, such that effectively only aerosol particles smaller than this size enter the system. This allows ice crystals to be differentiated from larger ambient aerosol. The measurement uncertainties at −30 °C with regard to temperature and relative humidity with respect to water are stated as ±0.5 °C and 2.4 %, respectively (DeMott et al., 2015). The residence times of aerosols in the supersaturated region are 5 s for the flow rate used (1.5 L min−1). For this study, water supersaturation was controlled to be sufficiently high to promote comparison to the results of immersion freezing methods (DeMott et al., 2017). The mean and median supersaturations employed for this study were both equal to 6.5 % (i.e. 106.5 % relative humidity with respect to water, RHwater), with a standard deviation of 1.4 %. At this value, it is likely that maximum INP concentrations are not captured, although underestimations would be expected to be less than the factor of 3 noted for mineral dusts in comparing data collected at 105 % versus 109 % in DeMott et al. (2015). The 1 Hz data were accumulated and averaged over a time period of 1 min for this study. CSU-CFDC is typically operated for ∼4 h before refreshing the ice surfaces on the walls. Operation times in excess of 4 h can result in an increase in background ice counts (due to frost) in the chamber and thereby degrade the signal-to-noise ratio. CFDC background corrections are needed to account for INP signal contamination that may come in the form of frost crystals flaking from the ice walls (Rogers et al., 2001). Infrequent, high-concentration bursts may occur, typically in the time just following wall icing or after a number of hours of operation. These are accounted for with a data pre-screening method to search for outliers in ice crystal arrival rates at the optical particle counter (Moore, 2020). The more common intermittent, low-concentration frost events are corrected for by comparing ambient measurements with measurements of high-efficiency particulate absorbing (HEPA)-filtered air. For PICNIC, these filter periods were 5 min long, thus bookending each 10 min ambient air sample period. The correction for intermittent frost events has recently been modified to improve the estimates of statistical significance and confidence intervals over previous techniques, following Krishnamoorthy and Lee (2013). The background INP counts from filter periods that bracket each ambient measurement are combined into a single Poisson distribution with a characteristic rate parameter. The difference between the ice crystal arrival rates during the ambient measurement and the combined filter period is used to calculate the background-corrected INP concentrations (Moore, 2020). Statistical significance and confidence intervals for each ambient measurement are determined using the moment-based Z statistic defined in Krishnamoorthy and Lee (2013).
2.2.2 The Spectrometer for Ice Nuclei (SPIN)
SPIN is a commercially available CFDC-style instrument developed by Droplet Measurement Technologies (Garimella et al., 2016). It is based on the design of the laboratory instrument ZINC (Zurich Ice Nucleation Chamber; Stetzer et al., 2008) and its mobile version PINC (Portable Ice Nucleation Chamber; Chou et al., 2011). Briefly, two parallel flat plates are separated by 1 cm and each coated with 1 mm of ice prior to experiments. A temperature gradient between the two plates establishes a supersaturation with respect to ice and potentially liquid water. The supersaturation employed for this study was 2.8±1.9 % (102.8 % RHwater ± 2.5 %), with an uncertainty in temperature of ±0.5 °C. Aerosols are fed into the chamber at a sampling rate of 1 L min−1 and constrained to a lamina centreline with 9 L min−1 of sheath air. The residence time of the particles in the chamber is 10 s. An impactor with a 50 % size cut at 2.5 µm (BGI Inc., SCC1.062 Triplex) was installed before the SPIN inlet. Activated INPs are detected using a light-depolarization OPC (Garimella et al., 2016; Droplet Measurement Technologies). Due to the sigmoidal shape of the impactor's size cut, OPC counts larger than 5 µm in diameter were considered to be activated INPs. Although SPIN is operated at a lower supersaturation as compared to the CSU-CFDC, the ice crystals have a longer residence time (10 s), such that they grow to sizes larger than 5 µm.
Aerosol spreading due to turbulence at the inlet can cause some sampled aerosol to spread outside of the aerosol lamina, where they are exposed to a lower relative humidity. This phenomenon is ordinarily accounted for by applying measurable correction factors to the CSU-CFDC and SPIN data (DeMott et al., 2015; Garimella et al., 2017; Wolf et al., 2019). The degree of aerosol lamina spreading, and therefore the correction factor applied to observed INP concentrations, depends on several variables such as inlet pressure, chamber temperature, and degree of supersaturation. The correction factor for SPIN has been quantified to vary from approximately 1.5 to 9.5 for immersion freezing conditions (Garimella et al., 2017). As the degree of aerosol lamina spreading was not quantified in this study, no correction factor was applied.
The uncertainty in INP concentration for SPIN represents the standard deviation during a 10 min sampling period. SPIN's limit of detection is dependent on background ice concentrations resulting from ice shed from the walls. Backgrounds were measured for 5 min on both sides of a 10 min sampling period. Average backgrounds before and after a sampling period were subtracted from the average measured INP concentration. Only data from when backgrounds were less than half of measured INP concentrations are reported. The campaign-averaged background concentration was ∼3 L−1. The limit of detection of SPIN sampling at the concentration is lower (∼0.6 INP L−1) when compared to not sampling at the concentrator (∼6 INP L−1), as more sampled air is analysed, while the ice background counts remain the same. SPIN can typically be operated for 4 to 6 h before backgrounds are too high to prevent measurement of ambient INP concentrations. Beside the results from SPIN presented in this article, focusing on mixed-phase cloud conditions, SPIN also measured cirrus-relevant INP concentrations, which are discussed elsewhere (Wolf et al., 2020).
2.2.3 The Portable Ice Nucleation Experiment (PINE)
The PINE is a new type of mobile instrument to measure INPs (Möhler et al., 2021). It is based on the AIDA (Aerosol Interaction and Dynamics in the Atmosphere) chamber and mimics cloud formation upon air mass lifting by expansion. The instrument is fully automated and can be operated continuously. During the PICNIC campaign, the PINE-1A version was deployed. This version consists of a 7 L cylindrical chamber, which is cooled by an external ethanol cooling chiller (Lauda RP 855; Lauda-Königshofen, Germany). PINE operates in a cycled mode of flush, expansion, and refill. During the so-called flush mode, aerosol particles are guided through the chamber at a flow rate of 2 L min−1 for 5 min. Before entering the chamber, the sampled air is dried to a frost point temperature of below °C, which avoids accumulation of ice on the chamber wall. An OPC (welas® 2500, Palas GmbH, Karlsruhe, Germany) attached to the outlet of PINE counts larger unactivated aerosol particles. The flush mode is followed by the expansion mode when a valve upstream of the chamber is closed while the volumetric flow out of the chamber is set to a constant value of 3 L min−1. Please note that the inlet flow rate during the expansion is maintained by a bypass flow, which is the same as the flush flow rate, such that no change in the sampling flow at the WAI occurs. A total pressure reduction of ∼300 mbar is thereby induced over a time of ∼50 s. During this expansion, the air temperature in the chamber is decreased by expansion cooling. As the wall and air temperatures are below the frost point temperature, the chamber is ice-saturated at the start of the expansion and achieves supersaturation with respect to ice and water during the course of the expansion, such that cloud droplets (on cloud condensation nuclei) and ice crystals (on INPs) can form. The temperature during one expansion typically decreases by 6 °C. The coldest temperature is thereby used as the nucleation temperature for each experiment, as it is assumed that the coldest temperature dominates the INP number concentration. After completing an expansion, the chamber is set to the refill mode, where the chamber is refilled with filtered sample air to reach ambient pressure conditions. Then another cycle of flush, expansion, and refill is started.
During the expansion, the ice crystals are detected by their comparably large optical size in the OPC, which makes a distinction with cloud droplets possible. As the OPC has a sideward-scattering geometry, aspherical ice crystals are detected with a higher scattering intensity than spherical cloud droplets of the same volume and refractive index. No ice background correction is needed for the INP measurements, since no ice crystals form from frost forming on the walls, which is confirmed by regular background experiments when the sampled air is passed over a filter to remove all particles before entering the chamber for several consecutive expansions.
In the PINE instrument, the residence time of aerosol particles at supersaturated conditions or in supercooled droplets is more variable compared to CFDC instruments. The time during which cloud droplets are present during an expansion is 33 s. However, it should be noted that this is an upper limit for the residence time, as ice crystals formed by INPs are detected during the whole expansion period, and each INP has its own trajectory within the cloud chamber. In the presented study, the INP concentrations are averaged over two consecutive experiments (two cycles of flush, expansion, and refill) to increase the detection limit for INPs. During the course of one expansion, about 2 L of air are continuously taken out of the chamber and analysed for forming ice crystals. The welas® 2500 OPC has an optical detection volume of 10 % and thus has a limit of detection of 2.5 INP per litre for two consecutive experiments. The uncertainty for the INP concentration is 20 %, which is an upper estimate from the uncertainties in the determination of the optical detection volume. The uncertainty in temperature is ±1 °C (see Möhler et al., 2021, for further details about the specifications of PINE).
The majority of aerosol particles with an aerodynamic diameter of <2 µm are sampled with PINE (80 %), which decreases to <50 % for particles with an aerodynamic diameter of >4 µm. No impactors were used with the PINE instruments. However, when sampling at the PFPC, which is operated with an impactor with a 50 % size cut at 2.5 µm, the sampled particle size was limited to this size.
In order to compare the PINE measurements to the offline methods for a perfect time overlap, PINE joined the offline intercomparison times for some nighttime measurements and measured at a constant temperature during the 8 h.
2.3 Offline measurement techniques
For offline INP analysis, aerosol particles were collected simultaneously with the different sampling set-ups during 8 h intervals. All INP concentrations are given with reference to standard litres sampled. Here, we present results from day- and nighttime sampling periods (10:00 to 18:00 LT (local time) and 22:00 to 06:00 LT, respectively) from 7 to 20 October 2018 (Table 1). Only during 18 to 19 October was the sampling time increased to 24 h. The particles were collected on filters, either behind the WAI (no additional impactor used) inside the laboratory or directly on the rooftop (Fig. 1). After collection, the samples were transported frozen or refrigerated to the respective laboratories, and particles were resuspended from the filters to analyse their ice nucleation activity in the immersion freezing mode. The comparison of the INP freezing spectra derived with the different methods is done at 1 °C intervals. A total of seven offline methods were deployed during PICNIC, which are described in the following sections, and their specifications regarding filter collection and freezing analysis are summarized in Table 1.
The cumulative INP concentration calculation as a function of the nucleation temperature cINP(T) for all offline techniques follows the well-established Vali (1971) equation:
where Vdrop is the droplet volume, Nl is the number of liquid and thus unfrozen droplets, while Nall is the number of the total droplets containing the aerosol suspension. The calculation thereby considers the volume of water used to extract the sample (suspension; Vsus) and the volume of air sampled Vair (considering the filter collection time and the applied flow rate). The number of total droplets from background measurements (Nall, BG) and the number of liquid droplets from background measurements Nl, BG(T) are inferred from the freezing curves of field blank filters, which were handled the same way as the sample filters, except that no airflow was guided over the blank filter. The INP errors are indicated by the use of two-tailed, 95 % confidence intervals for binomial sampling, based on Agresti and Coull (1998).
2.3.1 FRankfurt Ice nucleation Deposition freezinG Experiment (FRIDGE)
For the FRIDGE measurements, aerosol particles were collected in the laboratory from the WAI inlet. Aerosol was collected by the use of a custom-built semi-automated multi-filter sampling device. The unit consists of eight individual filter holders, the 45.7 cm housing, valves, a pump, and electronics. The sampling time of each filter can be programmed separately. The flow rate through the filters was determined to be 4.8±0.4 SD L min−1 on average. This is more than 50 % lower than the flow rate that was originally targeted due to a miscalibration and a leakage in the system. Accordingly, the flow rate needed to be corrected to the above-mentioned value and carries a rather high uncertainty. Aerosol particles were collected onto 47 mm hydrophobic PTFE Fluoropore membrane filter of 220 nm pore size (Merck Millipore). Filters were not pre-cleaned in any way. It was decided to limit the sampling time for FRIDGE to 4 h during daytime (10:00–14:00 LT; i.e. termination in the middle of the total sampling time of other instruments), as we initially expected higher INP concentrations compared to nighttime sampling, and to better capture potential variability in INP concentrations. The nighttime sample was the same as for the other groups (8 h). Moreover, on 18 October, the sampling time was not increased to 24 h, as for other methods. Filters were stored frozen at −18 °C after collection at the site. The samples were not actively cooled during transport; however, given the relatively short travel time of ∼8 h to the laboratory in Frankfurt, we do not consider that this impacts the results, but it cannot be excluded for certain (Beall et al., 2020). After transport, they were stored in a refrigerator at 4–7 °C until freezing measurements were performed. The analysis was performed using the droplet freezing mode of FRIDGE (Hiranuma et al., 2015). Before starting a measurement, a filter containing the sampled aerosol was placed in a sterile Eppendorf tube, which was filled with 5 mL of ultrapure water (ROTIPURAN® Ultra, Carl Roth). Particles were then extracted into the ultrapure water by repeated steady shaking for several minutes, without dilutions. Using an Eppendorf Reference 2 pipette, a total of about 200 (184–231) 2.5 µL droplets were manually pipetted onto a 45 mm silanized (dichlordimethylsilan) silicon wafer substrate placed on a cold stage inside a 500 cm3 measurement cell. About 65 droplets of 2.5 µL fit onto the substrate at a time; therefore, three individual runs per sample were performed to improve the freezing statistics. Before and after each measurement run, the substrate was thoroughly cleaned with pure non-denatured ethanol (ROTIPURAN®, >99.8 %, Carl Roth). During the experiment, the measurement cell was constantly flushed with dry synthetic air at 1 L min−1 to prevent condensation and riming. The temperature was decreased at a constant rate of 1 °C min−1 until every droplet was frozen, using a proportional–integral–derivative (PID)-controlled Peltier element. An ethanol cryostat cooling system supported the Peltier by dissipating the heat. The surface temperature was measured with a Pt100 sensor, which has an accuracy of ±0.2 °C. A camera saved measurement images every 10 s, and a change in brightness was detected when droplets were freezing. A detailed description of the FRIDGE immersion freezing method can be found in Schrod et al. (2020).
2.3.2 Ice Nucleation Droplet Array (INDA)
For analysis with INDA and also the below-discussed LINA (Leipzig Ice Nucleation Array; see Sect. 2.3.5), three different types of filters and two different samplers were deployed, with both samplers operating in parallel. All filters were taken at the WAI. Quartz fibre filters (Munktell, MK 360; 47 mm diameter) were used for sampling, as well as polycarbonate filters (Nuclepore, Whatman, 47 mm diameter with pore sizes of 200 or 800 nm). One sampler was a simple standard filter holder. The sampling flow was deliberately set to different values for different sampling periods, varying between 12 and 37 L min−1 and resulting in total collected air volumes between 6 and 18 m3. The other sampler was HERA (High Volume Aerosol Sampler; Hartmann et al., 2020; Grawe et al., 2023), which was developed for airborne sampling and enables the subsequent sampling of six filters. For HERA, the sampling flow varied between 15 and 41 L min−1, resulting in collected air volumes between 7 and 20 m3. All samples and blank filters were stored in separate Petri dishes right after sampling and stored and shipped frozen until the analysis was done at the laboratory in Leipzig.
INDA is based on a measurement technique that was introduced by Conen et al. (2012) and modified as suggested by Hill et al. (2014). A suspension is obtained by washing particles off a polycarbonate filter. For this, the filters are put in 3 mL of ultrapure water, followed by shaking for 15 min in a flask shaker. Subsequently, typically 0.1 mL of the suspension is used for a LINA experiment (Sect. 2.3.5). Then 3.1 mL of ultrapure water is added, and 50 µL droplets of this suspension are placed into 96 wells of a polymerase chain reaction (PCR) tray. For the quartz filter samples, each well is filled with 50 µL of ultrapure water, together with a 1 mm diameter filter punch from the quartz fibre filter. The PCR tray is then immersed in a temperature-controlled cooling bath of a thermostat and is illuminated from below. During cooling, typically done at 1 °C min−1, a picture is taken every 6 s from above. Changes in the colour of wells occur during freezing and are automatically detected. More information can be found in Gong et al. (2020) for the INP analysis of quartz fibre filters and in Hartmann et al. (2020) for polycarbonate filters.
2.3.3 The Colorado State University Ice Spectrometer (IS)
The Colorado State University (CSU) Ice Spectrometer (IS) analyses arrays of liquid suspensions from filter samples to quantify immersion freezing INP concentrations (e.g. DeMott et al., 2018). Aerosol filter samples were collected on the roof of the laboratory using precleaned (5 % H2O2, followed by two 100 nm filtered deionized (DI) water rinses), 200 nm pore diameter, 47 mm diameter Nuclepore polycarbonate filter membranes (Whatman, GE Healthcare) held in open-faced sterile Nalgene sampling heads. Mass flow rates (at 101.3 kPa and 0 °C) were recorded at the start and end of the sample period to calculate the total volume filtered. The average sample volume collected was 6 m3. Filter samples were immediately placed into sterile Petri dishes (Pall) and stored and transported frozen until analysis of INPs in Fort Collins, Colorado.
For analysis, 10 mL of 0.1 µm-filtered (Whatman Puradisc, PTFE membrane) DI water was added to a pre-rinsed polypropylene 50 mL tube (Corning) and shaken in a Roto-Torque rotator (Cole-Parmer) for 20 min to create a suspension. For each sample, serial 20-fold dilutions were made to 8000-fold. Next, 32 50 µL aliquots of each sample, with corresponding dilutions, and a 0.1 µm-filtered DI water blank were dispensed into 96-well PCR trays (OPTIMUM® µLTRA Brand, Life Science Products) in a laminar flow hood. The trays were then placed into aluminium blocks in the IS and cooled at a rate of ∼0.33 °C min−1. Freezing was detected by a CCD camera and the corresponding temperature was recorded with a LabVIEW interface. Frozen fraction results were corrected for the number of INPs in the DI water blank, resulting in the lowest freezing temperature achievable (generally between −27 and −30 °C). Temperature uncertainty is estimated at <±0.5 °C. The proportion of frozen wells was converted to a number of INPs per millilitre of suspension, using Eq. (13) in Vali (1971), and subsequently scaled to the number of INPs per filter. The average number of INPs on three field blanks (cleaned, handled, transported, and processed in the same way, with the exception of airflow) was subtracted from all samples before conversion to INPs per litre of air, considering the volume collected. Two-tailed 95 % confidence intervals for binomial sampling were calculated, based on Agresti and Coull (1998). Some samples of IS were investigated for the size of INPs by filtering the suspensions at 3 or 0.8 µm.
2.3.4 The Ice Nucleation Spectrometer of the Karlsruhe Institute of Technology (INSEKT)
INSEKT is a rebuild of the IS freezing method (e.g. Schneider et al., 2021). During PICNIC, aerosol particles were collected in the laboratory via the WAI with a standard filter holder. The aerosol particles were sampled with a flow rate of 11.3 (±0.2) SD L min−1 on 47 mm diameter Nuclepore filters (Whatman), with a pore size of 200 nm. The filters were pre-cleaned (10 % H2O2 solution) and kept frozen after aerosol particle collection and during transport until analysed in the laboratory in Karlsruhe. For INSEKT analysis, aerosol particles are washed off the filter using 8 mL filtered nanopure water (100 nm pore diameter filter and 18 MΩ deionized water) and shaken in a rotator for 20 min to ensure the release of all particles from the filter. The resulting suspension is then diluted by factors of 1, 15, and 225, and volumes of 50 µL are placed in the wells of a sterile PCR tray, alongside filtered nanopure water samples, to determine its freezing behaviour for a background correction. The PCR tray is then placed in an aluminium block cooled with an ethanol cooling bath (Lauda RP 890; Lauda-Königshofen, Germany). From a starting temperature of 0 °C, the wells are cooled down at a rate of 0.33 °C min−1. Four Pt100 temperature sensors are placed inside the aluminium blocks for each PCR tray, measuring with an accuracy of ±0.1 °C and a deviation to the edges of the wells of ±0.1 °C, resulting in an uncertainty in temperature of ±0.2 °C. A camera detects brightness changes in the wells that correspond to their freezing.
Washing water from handling filter blanks that were taken prior to the 8 October 2018 started to freeze at −7 °C, which was traced back to using non-powder-free gloves during the filter handling procedure at the Puy de Dôme, which was changed thereafter, demonstrating the need to work cleanly (Barry et al., 2021). Therefore, filters handled with non-powder-free gloves had to be disregarded. Moreover, filters containing parts of insects, which were sampled due to a leak in the WAI mesh, were excluded from the analysis.
2.3.5 The Leipzig Ice Nucleation Array (LINA)
LINA is based on a method described by Budke and Koop (2015). The filters were sampled as described in Sect. 2.3.2; however, only polycarbonate filters were analysed in LINA using washed suspensions. Of the resulting suspensions from the filter washing water, 90 droplets with a volume of 1 µL are pipetted onto a hydrophobic glass plate, which is placed on a Peltier element. Each droplet is contained in a separate compartment, which is covered by a second glass slide. Droplets are illuminated by a ring of light, which, together with a camera, is installed above. During the cooling process, typically done at 1 °C min−1, a picture is taken every 6 s from above. Changes in the reflection of the light by the droplets related to freezing are automatically detected. A more detailed description can be found in Gong et al. (2019).
2.3.6 The LED-based Ice Nucleation Detection Apparatus (LINDA)
The LED-based Ice Nucleation Detection Apparatus (LINDA) is an immersion freezing detection device that allows automatic detection of freezing in closed tubes by light transmission and is described in detail by Stopelli et al. (2014). Quartz filters (15 cm diameter) were used for analysis with LINDA and taken with a high-volume sampler at the rooftop, with a sample flow of 500 L min−1. The filters were stored in the freezer at −20 °C until analysis in the laboratory of LaMP close to the Puy de Dôme.
For analysis, four circular samples (1.2 cm diameter) were extracted from each filter and were washed in a 25 mL solution of 0.9 % NaCl for 20 min; then, 200 µL of the resulting solution was introduced to each of the 52 tubes. The array of tubes is placed in a cooling bath, with a Pt100 temperature probe at each corner of the array. A camera placed above the array detects the freezing of the tubes through the variation in the intensity of the transmitted light through the tubes. Error bars were calculated from freezing events from background filters and the NaCl solution.
INP concentration measurements from LINDA were already presented by Bras et al. (2022) to investigate the seasonal variability. Here, we focus on the comparison to other INP concentration measurements.
2.3.7 The Universidad Nacional Autónoma de México–Micro-Orifice Uniform Deposit Impactor–Droplet Freezing Technique (UNAM–MOUDI–DFT)
Aerosol particle collection was carried out by an inertial cascade impactor (MOUDI 100NR, MSP Corporation), which divides the particles according to their aerodynamic diameter in each of its eight stages (cut sizes of 0.18, 0.32, 0.56, 1.0, 1.8, 3.2, 5.6, and 10.0 µm). For this study, particles impacted on stages 2 to 7 were used. Hydrophobic glass coverslips (Hampton Research) were used as substrates in each of the eight stages. During PICNIC, the collection of particles was done in the laboratory via the WAI at a flow rate of 30 L min−1. After particle collection, the samples were stored in 60 mm Petri dishes and refrigerated at ∼4 °C for transport to the laboratory in Mexico City, where the analysis using the droplet freezing technique (DFT) was performed. We note that storing samples for a longer transportation time might impact the INP concentration (e.g. Beall et al., 2020). Although we did our best to keep the samples below 0 °C by transporting them in a freezer with ice packs, it is very likely that the samples may have experienced temperatures slightly above 0 °C right before reaching their final destination.
The DFT, built at the Institute for Atmospheric Science and Climate Change at UNAM (Córdoba et al., 2021), is based on the design by Mason et al. (2015) and determines the concentration of INPs as a function of temperature and aerodynamic particle size via immersion freezing. Each substrate is isolated in a temperature-controlled cell. Supersaturated conditions with respect to water are generated to trigger cloud droplet formation on the aerosol particles deposited on the substrate. The typical size of the droplets is around 100 µm, and 30 to 40 droplets are formed in the study area (1.2 mm2). The experiment is monitored in real time with an optical microscope (Axiolab, Zeiss, Germany) with a 5× /0.12 magnification objective coupled to a video camera (MC500-W, JVLAB). Droplets are subsequently cooled down from 0 to −40 °C at a cooling rate of 10 °C min−1. The temperature at which each droplet freezes is determined when the temperatures from the cold cell (monitored with a resistance temperature detector, RTD; ±0.1 °C uncertainty) and the videos are integrated. The INP concentration is derived from the following expression from Mason et al. (2015):
where [INPs(T)] is the INP concentration, Nu(T) is the unfrozen droplets (L−1) at a certain temperature T (°C), N0 is the total number of droplets analysed, Adeposit is the total area where the aerosol was deposited on the MOUDI hydrophobic glass coverslips (cm2), ADFT is the area analysed by the DFT, V is the volume of air sampled by the MOUDI (L), fnu is a correction factor (dimensionless) that takes into account changes in deposit inhomogeneity in a range between 0.25–0.10 mm in each of MOUDI sample, and fne is a correction factor that varies between 1.2 and 4.7 and that takes the uncertainty associated with the number of nucleation events in each experiment into account.
3.1 Intercomparison of online instruments
INP concentrations as measured with CSU-CFDC, SPIN, and PINE were typically intercompared from the morning hours to the late afternoon at ice nucleation temperatures (Tnucleation) from −20 to −30 °C. Measurements were performed either directly at the WAI or downstream of the PFPC attached to the WAI when INP concentrations were calculated back to ambient conditions (see Sect. 2.2). As an example, Fig. 2 shows a typical day of intercomparison, 11 October. In the morning hours, the instruments were set to the start conditions ( °C), which was changed consecutively for every few hours by 2 to 5 °C. As seen from this intercomparison day, the instruments measure similar INP concentrations at similar Tnucleation, with deviations within the same order of magnitude.
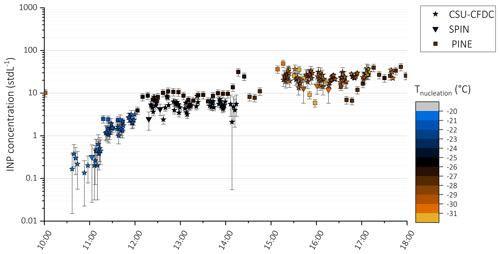
Figure 2Time series of INP concentration above liquid water saturation, as measured with CSU-CFDC (star), SPIN (triangle), and PINE (square) during 11 October 2018. The colour scale represents Tnucleation. INP concentrations are measured with a time resolution of ∼1 min (CSU-CFDC) and ∼10 min (PINE, SPIN).
To identify potential systematic deviations between the three instruments, the results from all intercomparison experiments are investigated, using the CSU-CFDC as a reference instrument, given its long history of operation and good characterization. CSU-CFDC has been used extensively in laboratory intercomparisons (e.g. DeMott et al., 2011; Hiranuma et al., 2015; DeMott et al., 2018) and in a large number of field measurement studies in surface- and aircraft-based campaigns (e.g. within the last 5 years; DeMott et al., 2018; McCluskey et al., 2018; Cornwell et al., 2019; Hiranuma et al., 2019; Kanji et al., 2019; Levin et al., 2019; Schill et al., 2020; Barry et al., 2021; Knopf et al., 2021; Twohy et al., 2021) over a period of more than 25 years. However, it should also be noted that the CSU-CFDC might not measure the total ambient INP concentration, due to aerosol lamina properties and size cuts, which will be discussed below in more detail, and that can lead to an underestimation of the INP concentration. For the comparison with the SPIN and PINE, the CSU-CFDC data that have the highest time resolution of 1 min were integrated on the time grid of the other instruments. Moreover, only measurements within ±1 °C were considered. INP concentrations as measured with SPIN (Fig. 3a) and PINE (Fig. 3b) are compared against CSU-CFDC at a large dynamic range of INP concentrations (0.1–100 INP SD L−1). This comparison reveals that SPIN observed lower INP concentrations, independently of Tnucleation. While only 35 % of the data are within a factor of 2, 80 % are still within a factor of 5 (Table 3a). It should be noted that only 20 data points could be compared here due to the mentioned temperature and time restraints. A possible explanation for this systematic deviation could be related to the aerosol lamina properties. Previous studies have found that the aerosol particles in at least some CFDCs are likely spreading beyond the lamina, such that 100 % of particles are not in the lamina where they are exposed to the targeted supersaturation condition (DeMott et al., 2015; Garimella et al., 2017; Wolf et al., 2019). The issue of lamina spreading is likely variable and depends on the CFDC geometry, the flow conditions, and the temperature gradients between the walls, which is creating the supersaturation; ultimately, this may be an issue with how the central lamina is introduced to the chamber and how the thermal gradients and non-laminar flow impact their spreading at the location where the aerosols are entering the chamber. Aerosol spreading causes aerosol particles to experience lower supersaturations than the target supersaturation, resulting in either a non-activation into cloud droplets and ice crystals (immersion freezing mode) or an activation into ice crystals that are not growing to sizes within the residence time in the chamber to be detected by the OPC (above the ice threshold). SPIN was operated at a lower supersaturation (2.8±1.9 %) compared to CSU-CFDC (6.5±1.4 %). Thus, it is expected that SPIN underestimates INP concentration by up to a factor of 10 (Garimella et al., 2017; Wolf et al., 2020).
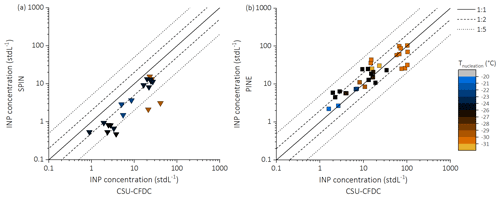
Figure 3Comparison of INP concentrations measured with SPIN (a) and PINE (b) to CSU-CFDC. INP measurements are selected for cases that fall within ±1 °C and have an overlapping sampling time. The measurements are corrected for the use of the aerosol concentrator when instruments are sampled on it by applying a correction factor of 11.4, which is the campaign average determined by CSU-CFDC.
Moreover, SPIN also used a larger ice threshold in the OPC of 5 µm, compared to 4 µm from CSU-CFDC, which has been found to impact INP concentration measurements (Jones et al., 2011). Thus, it is possible that due to a larger ice threshold size, fewer particles in SPIN were encapsulated in the intended conditions and were less likely to reach the critical size threshold. The impact of aerosol spreading was not quantified during the campaign, and data reported for the CSU-CFDC and SPIN instruments here remain original to account for this phenomenon. Moreover, no laboratory-derived calibration factors to account for a possible underestimation were applied, as the aim was to investigate such potential deviations amongst instruments using ambient aerosol particles. Please note that the residence time of SPIN is longer (10 s) compared to CSU-CFDC (5 s); however, we believe that other factors such as the difference in supersaturation are more important here.
The comparison between CSU-CFDC and the expansion chambers in PINE shows that the majority of the compared data fall within a factor of 2 (71 %) and 5 (100 %; Fig. 3b; Table 3b). As seen in Fig. 3b, no trend for under- or over-counting is observed for PINE relative to the CSU-CFDC. However, it should be noted that agreement between the measurements does not necessarily imply that both instruments can quantify the true ambient INP concentration. As stated before, the INP concentration using the CFDCs could be underestimated due to the incomplete activation of INPs in the aerosol lamina. The expansion chamber PINE could also systematically underestimate INP concentrations, as it is possible that not all sampled aerosols are activating into cloud droplets, e.g. by being poor cloud condensation nuclei. More laboratory experiments will be performed in future studies to identify such a possible low bias. In addition, the residence time of particles in PINE is not as well quantified as in the CSU-CFDC and might be longer (maximum 33 s), which might impact INP concentrations. It should also be pointed out that, due to a temperature calibration performed after the PICNIC campaign, PINE had fewer overlapping measurements with CSU-CFDC than initially targeted.
It should be noted that differences between the online instruments might arise from the difference in impactors. CSU-CFDC is operated with two single-jet 2.5 µm impactors, while SPIN is using only one, and PINE is operated without an impactor and thus has a 50 % aerodynamic size cut at 4 µm due to the loss of particles in its inlet.
3.2 Intercomparison of offline methods
INP concentrations were determined based on 8 h day- and nighttime filter samples during the campaign, using seven different freezing methods. Due to the difference in sampled volume and thus detection limit (see Table 2), the probability for the detection very rare INPs at temperatures above °C varies amongst instruments. The time series of INP concentrations from those measurements are presented in Fig. 4 at key temperatures at which many methods determined INP concentrations. Over temperatures ranging from −10 °C (Fig. 4a), −15 °C (Fig. 4b), and −20 °C (Fig. 4c), INP concentrations vary over 3 orders of magnitude, yet the measurements with a number of the different methods at single temperatures are within the error bars of each other most of the time. One clear systematic difference is that the rooftop INP concentrations (IS and LINDA) were systematically higher than those behind the WAI (all the other measurements), whereas the measurements taken from behind the inlet were generally within the quoted error bars. In order to get a more detailed picture of the results from the offline methods, the freezing spectra from each method for all day- and nighttime samples are shown in Figs. 5, 6, and 7 (alongside the online data). The INP concentrations from the offline methods were determined between and −30 °C, and span a range from below 0.001 to above 100 INP SD L−1. For most sampling intervals, the methods show good agreement, and the INP concentration and the shape of the freezing spectra are within a factor of 10. This is an indication of the general suitability of the different analysis procedures to determine INP concentrations (droplet freezing on cold stages, freezing of suspensions, and using different cooling rates) and that the different filter holders (standard filter holders, FRIDGE custom-built semi-automated sampler, open-faced disposable Nalgene units, MOUDI sampler, and HERA) and the filter materials (PTFE fluoropore membrane filters, quartz filters, hydrophobic glass coverslips, and polycarbonate filters (200 and 800 nm pore diameters; see also Sect. 3.2.2) can be used for INP collection. As previously mentioned, IS and LINDA tend to measure higher INP concentrations, which appear to be associated with their filter sampling location on the rooftop rather than from the WAI. Moreover, the INP concentration determined with online instruments generally agrees with the offline freezing spectra (Figs. 5, 6, and 7) when sampling from the WAI, which will be discussed in more detail in Sect. 3.3.
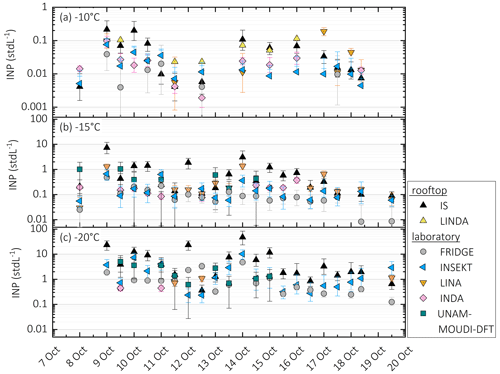
Figure 4Time series of INP concentrations at −10 °C (a), −15 °C (b), and −20 °C (c) as measured with the offline techniques on the rooftop (IS and LINDA) and in the laboratory at the WAI (FRIDGE, INSEKT, LINA, INDA, and UNAM–MOUDI–DFT).
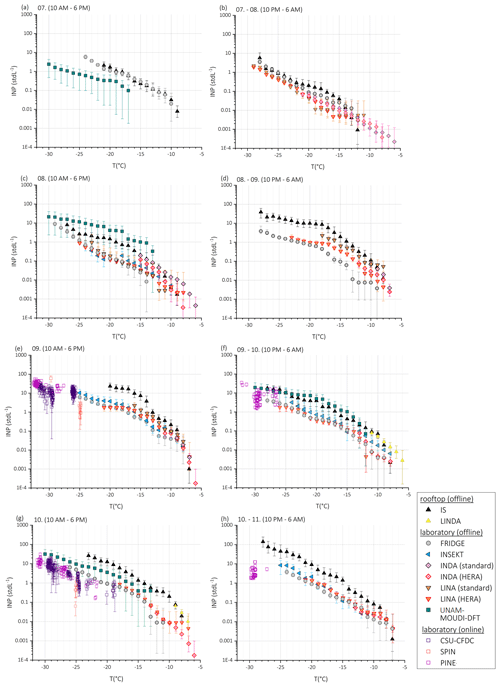
Figure 5INP freezing spectra of the offline and online methods during the sampling time from 7 to 10 October 2018. The filters for the offline INP analysis were taken during an 8 h interval, except for FRIDGE during the daytime samples (10:00–14:00 LT). INP concentrations with the online instruments were determined within the same sampling period but with a higher time resolution of minutes. Particles were collected on quartz filters for INDA and LINA using the standard filter holder (e–h).
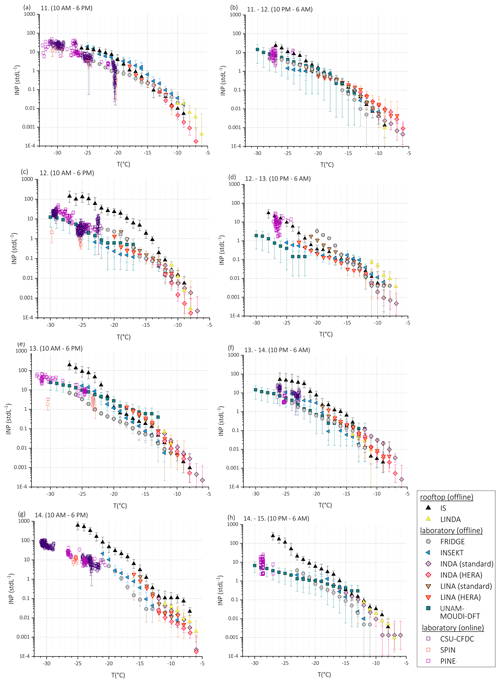
Figure 6INP freezing spectra of the offline and online methods during the sampling time from 11 to 14 October 2018 (see the caption of Fig. 5). Particles were collected on quartz filters for INDA and LINA using the standard filter holder (a).
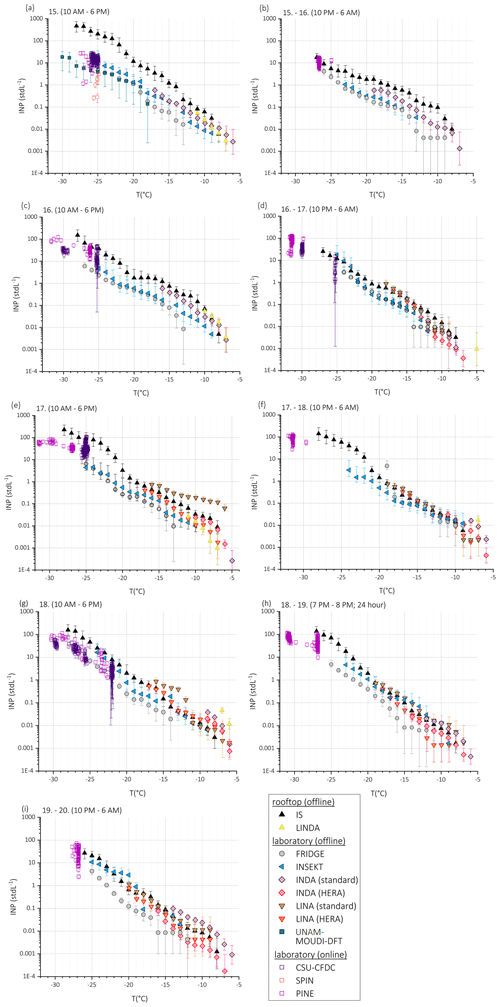
Figure 7INP freezing spectra of the offline and online methods during the sampling time 15 to the 19 October 2018 (see description of Fig. 5). The standard filter holder and HERA for analysis with INDA and LINA were equipped with quartz fibre filters (a–c) and polycarbonate filters with a pore size of 800 nm (d–g).
In order to gain better insight into the agreement during the whole campaign, we present the freezing spectra from each method compared against the INSEKT measurements as a reference (Fig. 8). This method was chosen since the filter collection for INSEKT was performed in the laboratory at the WAI inlet, similar to that for most of the other methods, and since it covers a large temperature range (approximately from −8 to −25 °C) of INP measurement. Figure 8 includes only data for INDA and LINA obtained from the standard filter holder, as no influence from the two different samplers (standard and HERA) was observed (see Figs. 5–7). Comparisons to INSEKT results on an instrument-by-instrument basis reveal that the methods used for sampling filters at the WAI on average agree with INSEKT for >45 % of the data within a factor of 2 and for >77 % within a factor of 5 (Table 2b). The FRIDGE method (Fig. 8a) has a slight tendency (still within factors of 2 and 5) to measure lower INP concentrations over the full temperature range compared to INSEKT. Recall that the flows for the FRIDGE filter collection were associated with a higher degree of uncertainty due to a miscalibration of the flows and the occurrence of a leak (see Sect. 2.3.1), which might have caused this difference. In addition, the methods use different suspension volumes for the INP detection. However, measurements with INSEKT and FRIDGE at the Jungfraujoch show a good agreement (Lacher et al., 2021), which indicates that the larger uncertainty in the present study was not caused by the different suspension volumes but rather arises from the larger uncertainty in the sample flow from FRIDGE.
As shown in Fig. 8b, the UNAM–MOUDI–DFT tends to measure higher INP concentrations compared to INSEKT. This bias may be coming from the method used to capture the particles. While for the INSEKT samples Nuclepore filters were used, in UNAM–MOUDI–DFT, particles were impacted on glass coverslips. A possible explanation is that not all particles are released from the Nuclepore filters. If so, this may relate to the aerosols sampled at Puy de Dôme, as this bias was not seen in some prior comparisons (e.g. Mason et al., 2015). Moreover, UNAM–MOUDI–DFT is the method using the fastest cooling rates of 10 °C min−1, such that an effect of a time dependency of ice nucleation might have impacted the results (e.g. Hoose and Möhler, 2012; Budke and Koop, 2015). However, this would have led to an underestimation of INP concentration, such that we conclude that the ambient INP concentration is not considerably controlled by stochastic variation or that other instrumental properties of sample collection and analysis with UNAM–MOUDI–DFT are dominant.
Again, IS and LINDA, with sampling filters on the rooftop, tend to measure higher INP concentrations (Fig. 8e, f), and only 27 % and 19 % are within a factor of 2 of the INSEKT measurements, respectively. As INSEKT is a re-built version of IS, differences due to their set-up is unlikely. A possible explanation is that filter measurements for offline INP analysis using standard inlet systems could systematically lose aerosol particles which are crucial for INP measurements. This could be supermicron particles that are lost by impaction in bends or might not be sampled especially under high-wind conditions and nanometre-sized particles that are lost by diffusion. The ability of nanoparticles to nucleate ice is not well investigated, but it is suggested that pollen particles can release ice-active nanoscale particles (Duan et al., 2023). Larger particles are often associated with dust or pollen, which are known to be efficient INPs (e.g. Murray et al., 2012). Calculations of the size-dependent inlet transmission efficiency indicate that the majority (84 %) of 10 µm particles were still sampled via the WAI at a wind speed of 10 m s−1 and 63 % at a wind speed of 15 m s−1 (Hangal and Willeke, 1990), which is an upper value measured during the campaign.
To investigate this further, IS sample suspensions were size-segregated (Fig. 9) for three cases when there was a discrepancy to the measurements at the WAI (12th, 14th, and 15th daytime; Fig. 9a, b, c) and one case when there was a good agreement (16th daytime; Fig. 9d). Those experiments reveal that the ice nucleation efficiency was not reduced significantly by filtering particles to <3 and <0.8 µm within the measurement uncertainties. Only on 12 and 16 October did a difference between the unamended and filtered samples occur. In fact, in some cases, the filtered experiments reproduced the unamended results. This indicates that the discrepancy between rooftop and WAI samples not only arises from a non-sampling of larger INPs, at least not from those INPS that remain in the liquid suspensions after the first freezing experiment was performed. Another source of discrepancy could be that fragmentation or disaggregation of especially larger particles when placed in suspensions lead to a high bias in INP concentrations, as discussed already by DeMott et al. (2017). Indeed, the open-faced Nalgene sampler can sample larger particle fragments, which could release multiple aerosols once suspended in water.
Whether this is only an issue for ground-based sampling locations but not for aircraft measurements due to, for example, typical decreases in large particle concentrations with altitude, needs to be investigated in future studies.
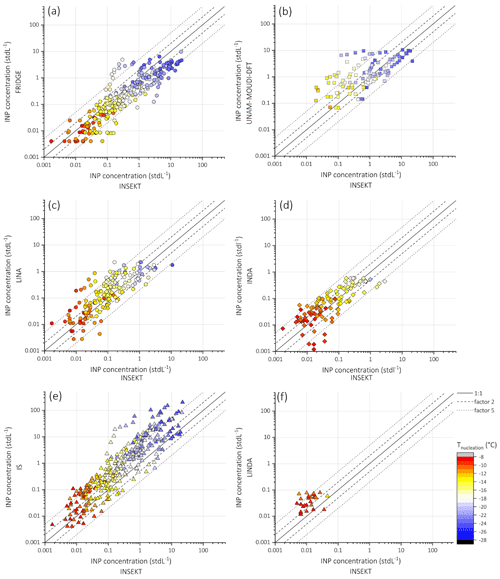
Figure 8INP concentrations measured with FRIDGE (a), UNAM–MOUDI–DFT (b), LINA (c; standard filter holder), INDA (d; standard filter holder), IS (e; filter taken on rooftop), and LINDA (f; filters taken on the rooftop) as a function of INP concentrations measured with INSEKT; colour-coding represents nucleation temperature.
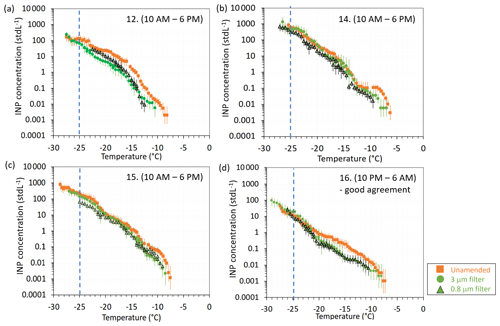
Figure 9Size-segregated INP concentration as measured from filtering IS liquid suspensions, with unamended freezing solutions (orange squares), solutions including particles <3 µm (green circles), and <0.8 µm (green triangles), are analysed for the daytime sampling period of 12 (a), 14 (b), 15 (c), and 16 October (d). Only on 16 October does IS show a good agreement to the INP concentration measurements at the WAI. The blue line indicates the freezing temperature at −25 °C.
3.2.1 Investigation of INP differences using aerosol particle measurements
A wider spread between the methods based on filters collected at the rooftop and in the laboratory via the WAI is observed during many sampling intervals. In order to gain better insight into this deviation, the time series of the difference between the INP concentration measurements from the IS (rooftop) and INSEKT (laboratory) is investigated in relation to the wind velocity and the concentration of aerosol particles. Those freezing methods were selected as they are based on the same freezing analysis principle and both span a large range of Tnucleation. As seen in Fig. 10, the difference between the INP concentration measurements from the IS and the INSEKT at −10, −15, and −20 °C, given as the lognormal difference, is sometimes occurring during elevated wind velocities (Fig. 10b), which can decrease the transmission efficiency, especially of larger particles, as discussed earlier. No relation between the difference between IS and INSEKT is observed to the total particle number concentration and the particle number concentration 0.1–0.5 µm (Fig. 10c, d). Moreover, a higher ratio between IS and INSEKT is not observed during times of higher concentrations of particles between 0.5 and 2.5 µm and 1 and 2.5 µm (Fig. 10d), which would have been an indication for a generally higher concentration of larger particles in the ambient air and which might be preferentially lost in the inlet prior to the INSEKT filter samples. At the same time, all the aerosol particle concentration measurements (total, 0.1–0.5, 0.5–2.5, and 1–2.5 µm) are especially higher in the second period of the campaign, starting from 13 October, when a higher discrepancy between IS and INSEKT is observed. This might indicate that the aerosol population changed and could have caused this discrepancy, e.g. by an increased presence of larger particles that are not sampled at the WAI, or could have caused particle fragmentation in IS. This potential cause of the discrepancy depends on the assumption that especially the larger fraction of the aerosol particle population dominated the INP population. A study performed at the same location using a stage impactor for size-segregated measurements indeed revealed that INPs are mostly super-micrometre particles (Bras et al., 2022). It should be noted that the size distribution measurements were conducted at the WAI; thus, the interpretation of the presented time series of aerosol particles during those high-wind velocity times is limited. In order to precisely identify such an impact, more intensive measurements need to be conducted by, for example, having aerosol particle size distribution measurements at the rooftop and in the laboratory simultaneously.
3.2.2 Comparison of INP concentrations using quartz fibre and polycarbonate filters
A subsample of the datasets was designed to test a possible influence of using different filter materials (quartz fibre versus polycarbonate filters). For this comparison, HERA and the standard sampler from TROPOS (Leibniz Institute for Tropospheric Research) were operated in parallel, using different filter materials. For the analysis, INDA and LINA, both operated at TROPOS, were used for evaluation. For the comparison shown here, HERA was equipped with polycarbonate filters (200 nm pore diameter), and the standard sampler with the quartz filter. Figure 11 shows results from sampling intervals between 9 (daytime) to the 11 (daytime) October. While both LINA and INDA can analyse particles collected with polycarbonate filters (creation of solution using the washing water), only INDA can analyse quartz fibre filter punches that are immersed in ultraclean water. No systematic difference between the INP concentrations using those different filter materials is observed, showing a good agreement between INDA and LINA, as previously reported (e.g. Knackstedt et al., 2018; Hartmann et al., 2019; Gong et al., 2020), which gives confidence that both materials can be used within the processing temperature ranges shown ( °C).
Moreover, quartz fibre filters and polycarbonate filters with a different pore size (800 nm) were used simultaneously in the TROPOS standard filter holder and in HERA for the analysis with INDA and LINA during some sampling intervals. Quartz fibre filters were used from the 14th nighttime (Fig. 6h) to the 16th daytime sample (Fig. 7a–c) and 800 nm polycarbonate filters for the sampling intervals from the 16th (nighttime) to the 18th (daytime; Fig. 7d–g). When comparing with the overall INP measurements from the other methods, there was no noticeable influence of using quartz fibre filters or polycarbonate filters with 800 nm pores, as compared to measurements using Nuclepore filters with a pore size of 200 nm. This shows that filters with a pore size of 800 nm and applied flow rate still have a sufficiently high collection efficiency for the majority of atmospheric INPs present during the PICNIC study. This is in agreement with Soo et al. (2016), who examined the collection efficiencies of a range of different filter materials and pore sizes for test particles with rather small sizes between 10 and 412 nm. They reported that the collection efficiency for polycarbonate filters with 800 nm pore sizes and the flow rates used here (>11 L min−1) are above 97 % for all particles in the examined size range (10–412 nm).
3.3 Comparison of online and offline methods
The comparisons presented in Figs. 5, 6, and 7 also include the measurements obtained from the CSU-CFDC, SPIN, and PINE. They are measured within the same time period of the filter collection but represent the instruments' specific time resolution, which is ∼1 min for CSU-CFDC and ∼10 min for SPIN and PINE. The measurements with PINE cover the full 8 h filter collection time, with a few exceptions.
Generally, the INP concentrations from the online instruments compare well with the offline techniques and are within the range of the offline-determined INP concentrations measured at the WAI. There is a slight tendency for the online instruments to measure lower INP concentrations, especially on 10 October (day- and nighttime; Fig. 5g, h). This low bias might be explained by the limitations of the instruments to measure only particles below 2.5 µm by the use of impactors (CFDCs) or below 4 µm due to the natural loss in the tubes for the PINE instruments (Möhler et al., 2021). Thus, it might be possible that the filters used for the offline INP analysis sampled a higher fraction of larger aerosol particles that were ice-active. Moreover, the good agreement of online and offline INP measurements at the WAI indicates that the potential disaggregation of aerosol particles into many INPs in liquid solutions via the bulk immersion freezing techniques is not of major importance, at least for the measured size distribution at the WAI.
In general, the measurements from the online INP instruments reveal that INP concentrations at a given temperature vary up to an order of magnitude during the sampling interval of 8 h, a variability that cannot be detected by the offline methods. A combination of both online and offline techniques is therefore of great advantage to capture both the INP concentration over a wide temperature range and their variability at single temperatures.
During the PICNIC campaign in October 2018, a suite of online and offline INP measurement techniques were operated simultaneously to compare the temperature-dependent INP concentrations relevant to the formation of mixed-phase clouds. The methods were deployed in their typical operation configuration without equalizing measurement set-ups. Two CFDCs (CSU-CFDC and SPIN) and an expansion chamber (PINE) measured INP concentrations in the temperature range from −20 to −30 °C. INP concentrations were compared within ±10 min and ±1 °C to ensure that sampling and nucleation conditions were as close as possible. PINE agreed well with CSU-CFDC, and most INP concentration measurements were within a factor of 2 (71 %). During the cloud formation process in PINE it is conceivable that not all aerosol particles are activating into cloud droplets during the expansion-induced cooling process, which can cause a low bias of immersion freezing INPs. Also, in CFDCs, it is possible that not all aerosol particles under investigation are exposed to targeted supersaturation conditions due to aerosol spreading beyond the aerosol lamina (DeMott et al., 2015; Garimella et al., 2017). Indeed, the comparison of CSU-CFDC and SPIN reveals that SPIN measured lower INP concentrations (only 35 % of the data are within a factor of 2), which could arise from different degrees of aerosol spreading beyond the lamina. The supersaturation was lower in SPIN (2.8±1.9 %) than in CSU-CFDC (6.5±1.4 %), and the instrument-specific size threshold to identify ice crystals was larger in SPIN (5 µm) than in CSU-CFDC (4 µm). Therefore, it is conceivable that fewer particles in SPIN were activated into cloud droplets and ice crystals, or they were not growing to ice crystals large enough to be classified as ice. More specific tests to characterize the effect of aerosol spreading beyond the lamina during field studies, as well as laboratory characterization of the established supersaturation conditions and hence cloud droplet and ice crystal activation, should be performed in future studies. More such intensive INP intercomparisons, resulting in a larger dataset, should be conducted in the future to better understand discrepancies amongst the online instruments and to guide potential technical mitigation.
INP filter sampling was performed during day- and nighttime for 8 h and analysed with FRIDGE, INDA, IS, INSEKT, LINA, LINDA, and UNAM–MOUDI–DFT. The filters for IS and LINDA were collected directly in ambient air on the rooftop of the laboratory, while the other filters were collected behind the WAI in the laboratory. The methods using filters collected at the WAI generally show good agreement over the investigated temperature range when compared to INSEKT as a reference, as >45 % are within a factor of 2. This indicates that, with attention to protocols for filter handling and analysis, not only with respect to the different freezing procedures (droplet freezing, freezing of suspensions) but also the sampling devices (standard filter holders, FRIDGE custom-built semi-automated sampler, open-faced Nalgene units, MOUDI, and HERA) and sampling substrates (PTFE fluoropore membrane, quartz filters, hydrophobic glass coverslips, and polycarbonate filters (200 and 800 nm pore diameters)) can be used together to provide generally consistent and reliable measurements of INP concentrations. It should be pointed out that the faster cooling rate (10 °C min−1) of the UNAM–MOUDI–DFT did not lead to lower INP concentrations compared to the other methods, indicating that the time dependence of nucleation is of secondary importance for immersion freezing on ambient particles in this study. IS and LINDA sometimes measured higher INP concentrations, and compared to the INSEKT method, only 27 % and 19 % of the data derived with IS and LINDA are within a factor of 2, respectively. This occurred sometimes during high-wind conditions and might be explained by losses of super-micrometre aerosol particles and INPs in the WAI. Calculations of particle transmission efficiencies reveal that the majority (>90 %) of 10 µm particles are sampled at the WAI. Next to this potential non-sampling of larger aerosol particles, it is also conceivable that in-suspension fragmentation/disaggregation of especially larger particles, which were more often sampled on the rooftop, results in an elevated INP concentration, as discussed in DeMott et al. (2017). It should be noted that such a fragmentation is leading to an artificially high INP concentration, as the initial particle would only lead to the freezing of one cloud droplet in which it is immersed. Moreover, most ambient INP measurements are performed behind aerosol inlets, and a systematic undercounting or overestimation should be investigated in future studies. For example, the aerosol particle transmission efficiency should be measured during different sampling conditions with regard to meteorology and the presence of particles in the size range relevant to ice nucleation. Moreover, specific experiments for a potential particle fragmentation and increase in INP number concentration should be conducted by measuring aerosol particles and INPs impacted and directly counted on a substrate and after re-suspending the impacted aerosol in solution. In addition, different rooftop configurations could be tested in parallel using no inlet and different PM inlets (e.g. PM10, PM2.5).
The INP measurements of the online instruments that were performed within the same sampling intervals of the filter collection time agreed well with the results from the offline methods. The online instruments showed a slight tendency to measure lower INP concentrations during some sampling intervals, which might be caused by the restriction of the online instruments for sampling aerosol particles smaller than 2.5 µm, which is needed to avoid the misclassification of unactivated aerosol particles as ice crystals. Nevertheless, we conclude that the presented methods here are suitable for combination with offline methods, which is required in order to capture the complete temperature range relevant for heterogeneous nucleation in the mixed-phase cloud regime. In addition, based on the finding of a good agreement between online and offline methods at the WAI, we conclude that the potential breakup of aerosol particles that passes through the WAI into many INPs via the bulk immersion freezing technique is of minor importance for particles below approximately 10 µm.
Especially in light of ongoing efforts for INP monitoring networks, we recommend that such intensive INP intercomparison measurements are repeated frequently, during different seasons, and at measurement sites characterized by different aerosol particle sources and properties. Ambient INP intercomparison campaigns are useful in addition to laboratory campaigns, where specific aerosol particles are used as test material. Such efforts are needed to ensure accurate INP concentration measurements, which is required to better understand and represent INPs in the atmospheric system.
For a better understanding of the formation and evolution of ice in clouds, it is essential to integrate observations of INPs in numerical models (e.g. Coluzza et al., 2017; Burrows et al., 2022). This requires a certain precision in INP measurement technique, as studies have shown that variations in the modelled INP concentration can lead to changes in the balance between ice and supercooled water, with a significant impact on the radiative properties of clouds and precipitation processes (e.g. Tan et al., 2016; Vergara-Temprado et al., 2018; French et al., 2018). Phillips et al. (2003) found a significant impact on modelled microphysical processes and precipitation rates when varying INP concentrations by factors of 10, indicating that observational errors need to be smaller than this factor. Ervens et al. (2011) investigated the partitioning between ice and water in Arctic mixed-phase clouds and only revealed an effect when INP concentrations were increased by a factor of 5. The results from this ambient intercomparison campaign revealed that the majority of the data from the different INP measurement techniques, in their original configuration, are within a factor of 5, which generally demonstrate their suitability to derive model-relevant INP data.
The data used in this study are available via the KITopen data repository (https://doi.org/10.35097/1876, Lacher et al., 2024).
LL wrote the paper, with contributions from YB, PJD, LAL, CRR, JS, HW, MW, and EF. CSU-CFDC measurements and analysis were provided by KB, PJD, EJTL, and KAM. DJC, MG, and MW conducted the SPIN measurements and analysis. PINE measurements were performed by LL, MA, and OM. CB, NB, RF, BJM, JN, and TP contributed to the instrument set-up and data analysis. HB, DC, SR, JS, and ET performed the FRIDGE filter measurements and data analysis. Filter sampling for INDA and LINA and data analysis were conducted by CJ, SM, FS, and HW. KB, PJD, TCJH, and EJTL performed and analysed the IS measurements. INSEKT measurements were performed and analysed by BB, LL, KH, and OM. YB and EF provided the measurements from LINDA. UNAM–MOUDI–DFT measurements and analysis were conducted by LAL, CRR, DP and KS. MR provided logistical support. EF provided the meteorological measurements at the Puy de Dôme station and coordinated the PICNIC campaign.
At least one of the (co-)authors is a member of the editorial board of Atmospheric Chemistry and Physics. The peer-review process was guided by an independent editor, and the authors also have no other competing interests to declare.
Publisher’s note: Copernicus Publications remains neutral with regard to jurisdictional claims made in the text, published maps, institutional affiliations, or any other geographical representation in this paper. While Copernicus Publications makes every effort to include appropriate place names, the final responsibility lies with the authors.
We thank the technical team from the Puy de Dôme/OPGC (l'Observatoire de Physique du Globe) for support and service during the campaign. We acknowledge the KIT technical team, with our special thanks to Steffen Vogt. The authors gratefully acknowledge CNRS-INSU for supporting measurements performed at the SI-COPDD and those within the long-term monitoring aerosol programme SNO-CLAP, both of which are components of the ACTRIS French Research Infrastructure and whose data are hosted at the AERIS data centre (https://www.aeris-data.fr/, last access: 21 February 2024).
This research has received funding from the European Commission under the Horizon 2020 – Research and Innovation Framework Programme via the ACTRIS-2 Trans-National Access and from the ANR-CHAIN (project no. ANR-14-CE01-0003-01). Larissa Lacher has received funding from the KIT Technology Transfer Project N059. Barbara Bertozzi has received funding from the European Union's Horizon 2020 research and innovation programme under the Marie Skłodowska-Curie Actions (grant no. 764991). Martin Wolf and Daniel Cziczo have received funding from the U.S. National Science Foundation (grant no. AGS-1838429), a supplement to Collaborative Research: A Closure Study of Mixed Phase Clouds at Storm Peak (grant no. AGS-1749851). Colorado State University co-authors have received partial funding support from U.S. National Science Foundation Award (grant no. 1660486). Luis Ladino and Carolina Ramirez have received partial funding from Conacyt (grant no. CB-285023). Conrad Jentzsch and Stephan Mertes have been supported by the German Research Foundation (DFG) through SPP 1294 (grant no. 316508271) and have received funding for the campaign from the ACTRIS-2 Trans-National Access. Stephan Mertes has also received funding from the German Research Foundation (DFG) (grant no. 268020496 – TRR 172), within the Transregional Collaborative Research Centre “ArctiC Amplification: Climate Relevant Atmospheric and SurfaCe Processes, and Feedback Mechanisms (AC)3.” Erik Thomson and Dimitri Castarede have been supported by the Swedish Research Councils, VR (grant nos. 2013-05153 and 2020-03497) and FORMAS (grant no. 2017-00564), and by the Swedish Strategic Research Area MERGE. Benjamin J. Murray and Mike Adams have received funding from the European Research Council (grant no. 648661; MarineIce).
The article processing charges for this open-access publication were covered by the Karlsruhe Institute of Technology (KIT).
This paper was edited by Alex Huffman and reviewed by two anonymous referees.
Agresti, A. and Coull, B. A.: Approximate is Better than “Exact” for Interval Estimation of Binomial Proportions, The American Statistician, 52, 119–126, https://doi.org/10.1080/00031305.1998.10480550, 1998.
Asmi, E., Freney, E., Hervo, M., Picard, D., Rose, C., Colomb, A., and Sellegri, K.: Aerosol cloud activation in summer and winter at puy-de-Dôme high altitude site in France, Atmos. Chem. Phys., 12, 11589–11607, https://doi.org/10.5194/acp-12-11589-2012, 2012.
Atkinson, J. D., Murray, B. J., Woodhouse, M. T., Whale, T. F., Baustian, K. J., Carslaw, K. S., Dobbie, S., O/'Sullivan, D., and Malkin, T. L.: The importance of feldspar for ice nucleation by mineral dust in mixed-phase clouds, Nature, 498, 355–358, https://doi.org/10.1038/nature12278, 2013.
Baray, J.-L., Deguillaume, L., Colomb, A., Sellegri, K., Freney, E., Rose, C., Van Baelen, J., Pichon, J.-M., Picard, D., Fréville, P., Bouvier, L., Ribeiro, M., Amato, P., Banson, S., Bianco, A., Borbon, A., Bourcier, L., Bras, Y., Brigante, M., Cacault, P., Chauvigné, A., Charbouillot, T., Chaumerliac, N., Delort, A.-M., Delmotte, M., Dupuy, R., Farah, A., Febvre, G., Flossmann, A., Gourbeyre, C., Hervier, C., Hervo, M., Huret, N., Joly, M., Kazan, V., Lopez, M., Mailhot, G., Marinoni, A., Masson, O., Montoux, N., Parazols, M., Peyrin, F., Pointin, Y., Ramonet, M., Rocco, M., Sancelme, M., Sauvage, S., Schmidt, M., Tison, E., Vaïtilingom, M., Villani, P., Wang, M., Yver-Kwok, C., and Laj, P.: Cézeaux-Aulnat-Opme-Puy De Dôme: a multi-site for the long-term survey of the tropospheric composition and climate change, Atmos. Meas. Tech., 13, 3413–3445, https://doi.org/10.5194/amt-13-3413-2020, 2020.
Baron, P. A. and Willeke, K.: Aerosol Measurement: Principles, Techniques, and Applications, John Wiley & Sons, New York, ISBN 978-0471356363, 2002.
Barry, K. R., Hill, T. C. J., Jentzsch, C., Moffett, B. F., Stratmann, F., and DeMott, P. J.:Pragmatic protocols for working cleanly when measuring ice nucleating particles, Atmos. Res., 250, 105419, https://doi.org/10.1016/j.atmosres.2020.105419, 2021.
Beall, C. M., Lucero, D., Hill, T. C., DeMott, P. J., Stokes, M. D., and Prather, K. A.: Best practices for precipitation sample storage for offline studies of ice nucleation in marine and coastal environments, Atmos. Meas. Tech., 13, 6473–6486, https://doi.org/10.5194/amt-13-6473-2020, 2020.
Boose, Y., Kanji, Z. A., Kohn, M., Sierau, B., Zipori, A., Crawford, I., Lloyd, G., Bukowiecki, N., Herrmann, E., Kupiszewski, P., Steinbacher, M., and Lohmann, U.: Ice Nucleating Particle Measurements at 241 K during Winter Months at 3580 m MSL in the Swiss Alps, J. Atmos. Sci., 73, 2203–2228, https://doi.org/10.1175/JAS-D-15-0236.1, 2016a.
Boose, Y., Welti, A., Atkinson, J., Ramelli, F., Danielczok, A., Bingemer, H. G., Plötze, M., Sierau, B., Kanji, Z. A., and Lohmann, U.: Heterogeneous ice nucleation on dust particles sourced from nine deserts worldwide – Part 1: Immersion freezing, Atmos. Chem. Phys., 16, 15075–15095, https://doi.org/10.5194/acp-16-15075-2016, 2016b.
Bras, Y., Freney, L., Bouvier, L., Pichon, J.-M., Picard, D., Amato, P., Cruz Minguillón, M., and Sellegri, K.: Seasonal Variations, Origin and Parameterization of Ice-Nucleating Particles at a Mountain Station in Central France, ESS Open Archive, https://doi.org/10.1002/essoar.10511724.1, 2022.
Brasseur, Z., Castarède, D., Thomson, E. S., Adams, M. P., Drossaart van Dusseldorp, S., Heikkilä, P., Korhonen, K., Lampilahti, J., Paramonov, M., Schneider, J., Vogel, F., Wu, Y., Abbatt, J. P. D., Atanasova, N. S., Bamford, D. H., Bertozzi, B., Boyer, M., Brus, D., Daily, M. I., Fösig, R., Gute, E., Harrison, A. D., Hietala, P., Höhler, K., Kanji, Z. A., Keskinen, J., Lacher, L., Lampimäki, M., Levula, J., Manninen, A., Nadolny, J., Peltola, M., Porter, G. C. E., Poutanen, P., Proske, U., Schorr, T., Silas Umo, N., Stenszky, J., Virtanen, A., Moisseev, D., Kulmala, M., Murray, B. J., Petäjä, T., Möhler, O., and Duplissy, J.: Measurement report: Introduction to the HyICE-2018 campaign for measurements of ice-nucleating particles and instrument inter-comparison in the Hyytiälä boreal forest, Atmos. Chem. Phys., 22, 5117–5145, https://doi.org/10.5194/acp-22-5117-2022, 2022.
Budke, C. and Koop, T.: BINARY: an optical freezing array for assessing temperature and time dependence of heterogeneous ice nucleation, Atmos. Meas. Tech., 8, 689–703, https://doi.org/10.5194/amt-8-689-2015, 2015.
Burkert-Kohn, M., Wex, H., Welti, A., Hartmann, S., Grawe, S., Hellner, L., Herenz, P., Atkinson, J. D., Stratmann, F., and Kanji, Z. A.: Leipzig Ice Nucleation chamber Comparison (LINC): intercomparison of four online ice nucleation counters, Atmos. Chem. Phys., 17, 11683–11705, https://doi.org/10.5194/acp-17-11683-2017, 2017.
Burrows, S. M., McCluskey, C. S., Cornwell, G., Steinke, I., Zhang, K., Zhao, B., Zawadowicz, M., Raman, A., Kulkarni, G., China, S., Zelenyuk, A., and DeMott, P. J.: Ice-Nucleating Particles That Impact Clouds and Climate: Observational and Modeling Research Needs, Rev. Geophys., 60, e2021RG000745, https://doi.org/10.1029/2021RG000745, 2022.
Campbell, J. R. and Shiobara, M.: Glaciation of a mixed-phase boundary layer cloud at a coastal arctic site as depicted in continuous lidar measurements, Polar Sci., 2, 121–127, https://doi.org/10.1016/j.polar.2008.04.004, 2008.
Carlsen, T. and David, R. O.: Spaceborne Evidence That Ice-Nucleating Particles Influence High-Latitude Cloud Phase, Geophys. Res. Lett., 49, e2022GL098041, https://doi.org/10.1029/2022GL098041, 2022.
Chou, C., Stetzer, O., Weingartner, E., Jurányi, Z., Kanji, Z. A., and Lohmann, U.: Ice nuclei properties within a Saharan dust event at the Jungfraujoch in the Swiss Alps, Atmos. Chem. Phys., 11, 4725–4738, https://doi.org/10.5194/acp-11-4725-2011, 2011.
Coluzza, I., Creamean, J., Rossi, M. J., Wex, H., Alpert, P. A., Bianco, V., Boose, Y., Dellago, C., Felgitsch, L., Fröhlich-Nowoisky, J., Herrmann, H., Jungblut, S., Kanji, Z. A., Menzl, G., Moffett, B., Moritz, C., Mutzel, A., Pöschl, U., Schauperl, M., Scheel, J., Stopelli, E., Stratmann, F., Grothe, H., and Schmale, D. G.: Perspectives on the Future of Ice Nucleation Research: Research Needs and Unanswered Questions Identified from Two International Workshops, Atmosphere, 8, 138, https://doi.org/10.3390/atmos8080138, 2017.
Conen, F., Henne, S., Morris, C. E., and Alewell, C.: Atmospheric ice nucleators active ≥ −12 °C can be quantified on PM10 filters, Atmos. Meas. Tech., 5, 321–327, https://doi.org/10.5194/amt-5-321-2012, 2012.
Córdoba, F., Ramírez-Romero, C., Cabrera, D., Raga, G. B., Miranda, J., Alvarez-Ospina, H., Rosas, D., Figueroa, B., Kim, J. S., Yakobi-Hancock, J., Amador, T., Gutierrez, W., García, M., Bertram, A. K., Baumgardner, D., and Ladino, L. A.: Measurement report: Ice nucleating abilities of biomass burning, African dust, and sea spray aerosol particles over the Yucatán Peninsula, Atmos. Chem. Phys., 21, 4453–4470, https://doi.org/10.5194/acp-21-4453-2021, 2021.
Cornwell, G. C., McCluskey, C. S., Levin, E. J. T., Suski, K. J., DeMott, P. J., Kreidenweis, S. M., and Prather, K. A.: Direct online mass spectrometry measurements of ice nucleating particles at a California coastal site. J. Geophys. Res.-Atmos., 124, 12157–12172, https://doi.org/10.1029/2019JD030466, 2019.
Creamean, J. M., Kirpes, R. M., Pratt, K. A., Spada, N. J., Maahn, M., de Boer, G., Schnell, R. C., and China, S.: Marine and terrestrial influences on ice nucleating particles during continuous springtime measurements in an Arctic oilfield location, Atmos. Chem. Phys., 18, 18023–18042, https://doi.org/10.5194/acp-18-18023-2018, 2018.
Creamean, J. M., Barry, K., Hill, T. C. J., Hume, C., DeMott, P. J., Shupe, M. D., Dahlke, S., Willmes, S., Schmale, J., Beck, I., Hoppe, C. J. M., Fong, A., Chamberlain, E., Bowman, J., Scharien, R., and Persson, O.: Annual cycle observations of aerosols capable of ice formation in central Arctic clouds, Nat. Commun., 13, 3537, https://doi.org/10.1038/s41467-022-31182-x, 2022.
Cziczo, D. J., Ladino, L., Boose, Y., Kanji, Z. A., Kupiszewski, P., Lance, S., Mertes, S., and Wex, H.: Measurements of Ice Nucleating Particles and Ice Residuals, Meteorol. Monogr., 58, 8.1–8.13, https://doi.org/10.1175/amsmonographs-d-16-0008.1, 2017.
DeMott, P. J., Möhler, O., Stetzer, O., Vali, G., Levin, Z., Petters, M. D., Murakami, M., Leisner, T., Bundke, U., Klein, H., Kanji, Z. A., Cotton, R., Jones, H., Benz, S., Brinkmann, M., Rzesanke, D., Saathoff, H., Nicolet, M., Saito, A., Nillius, B., Bingemer, H., Abbatt, J., Ardon, K., Ganor, E., Georgakopoulos, D. G., and Saunders, C.: Resurgence in Ice Nuclei Measurement Research, B. Am. Meteorol. Soc., 92, 1623–1635, https://doi.org/10.1175/2011bams3119.1, 2011.
DeMott, P. J., Prenni, A. J., McMeeking, G. R., Sullivan, R. C., Petters, M. D., Tobo, Y., Niemand, M., Möhler, O., Snider, J. R., Wang, Z., and Kreidenweis, S. M.: Integrating laboratory and field data to quantify the immersion freezing ice nucleation activity of mineral dust particles, Atmos. Chem. Phys., 15, 393–409, https://doi.org/10.5194/acp-15-393-2015, 2015.
DeMott, P. J., Hill, T. C. J., Petters, M. D., Bertram, A. K., Tobo, Y., Mason, R. H., Suski, K. J., McCluskey, C. S., Levin, E. J. T., Schill, G. P., Boose, Y., Rauker, A. M., Miller, A. J., Zaragoza, J., Rocci, K., Rothfuss, N. E., Taylor, H. P., Hader, J. D., Chou, C., Huffman, J. A., Pöschl, U., Prenni, A. J., and Kreidenweis, S. M.: Comparative measurements of ambient atmospheric concentrations of ice nucleating particles using multiple immersion freezing methods and a continuous flow diffusion chamber, Atmos. Chem. Phys., 17, 11227–11245, https://doi.org/10.5194/acp-17-11227-2017, 2017.
DeMott, P. J., Möhler, O., Cziczo, D. J., Hiranuma, N., Petters, M. D., Petters, S. S., Belosi, F., Bingemer, H. G., Brooks, S. D., Budke, C., Burkert-Kohn, M., Collier, K. N., Danielczok, A., Eppers, O., Felgitsch, L., Garimella, S., Grothe, H., Herenz, P., Hill, T. C. J., Höhler, K., Kanji, Z. A., Kiselev, A., Koop, T., Kristensen, T. B., Krüger, K., Kulkarni, G., Levin, E. J. T., Murray, B. J., Nicosia, A., O'Sullivan, D., Peckhaus, A., Polen, M. J., Price, H. C., Reicher, N., Rothenberg, D. A., Rudich, Y., Santachiara, G., Schiebel, T., Schrod, J., Seifried, T. M., Stratmann, F., Sullivan, R. C., Suski, K. J., Szakáll, M., Taylor, H. P., Ullrich, R., Vergara-Temprado, J., Wagner, R., Whale, T. F., Weber, D., Welti, A., Wilson, T. W., Wolf, M. J., and Zenker, J.: The Fifth International Workshop on Ice Nucleation phase 2 (FIN-02): laboratory intercomparison of ice nucleation measurements, Atmos. Meas. Tech., 11, 6231–6257, https://doi.org/10.5194/amt-11-6231-2018, 2018.
Desai, N., Chandrakar, K. K., Kinney, G., Cantrell, W., and Shaw, R. A.: Aerosol-Mediated Glaciation of Mixed-Phase Clouds: Steady-State Laboratory Measurements, Geophys. Res. Lett., 46, 9154–9162, https://doi.org/10.1029/2019gl083503, 2019.
Duan, P., Hu, W., Wu, Z., Bi, K., Zhu, J., and Fu, P.: Ice nucleation activity of airborne pollen: A short review of results from laboratory experiments, Atmos. Res., 285, 106659, https://doi.org/10.1016/j.atmosres.2023.106659, 2023.
Emersic, C., Connolly, P. J., Boult, S., Campana, M., and Li, Z.: Investigating the discrepancy between wet-suspension- and dry-dispersion-derived ice nucleation efficiency of mineral particles, Atmos. Chem. Phys., 15, 11311–11326, https://doi.org/10.5194/acp-15-11311-2015, 2015.
Ervens, B., Feingold, G., Sulia, K., and Harrington, J.: The impact of microphysical parameters, ice nucleation mode, and habit growth on the ice/liquid partitioning in mixed-phase Arctic clouds, J. Geophys. Res.-Atmos., 116, D17205, https://doi.org/10.1029/2011JD015729, 2011.
Fan, J., Leung, L. R., Rosenfeld, D., and DeMott, P. J.: Effects of cloud condensation nuclei and ice nucleating particles on precipitation processes and supercooled liquid in mixed-phase orographic clouds, Atmos. Chem. Phys., 17, 1017–1035, https://doi.org/10.5194/acp-17-1017-2017, 2017.
Farah, A., Freney, E., Chauvigné, A., Baray, J.-L., Rose, C., Picard, D., Colomb, A., Hadad, D., Abboud, M., Farah, W., and Sellegri, K.: Seasonal Variation of Aerosol Size Distribution Data at the Puy de Dôme Station with Emphasis on the Boundary Layer/Free Troposphere Segregation, Atmosphere, 9, 244, https://doi.org/10.3390/atmos9070244, 2018.
Field, P. R. and Heymsfield, A. J.: Importance of snow to global precipitation, Geophys. Res. Lett., 42, 9512–9520, https://doi.org/10.1002/2015gl065497, 2015.
French, J. R., Friedrich, K., Tessendorf, S. A., Rauber, R. M., Geerts, B., Rasmussen, R. M., Xue, L., Kunkel, M. L., and Blestrud, D. R.: Precipitation formation from orographic cloud seeding, P. Natl. Acad. Sci., 115, 1168–1173, https://doi.org/10.1073/pnas.1716995115, 2018.
Garimella, S., Kristensen, T. B., Ignatius, K., Welti, A., Voigtländer, J., Kulkarni, G. R., Sagan, F., Kok, G. L., Dorsey, J., Nichman, L., Rothenberg, D. A., Rösch, M., Kirchgäßner, A. C. R., Ladkin, R., Wex, H., Wilson, T. W., Ladino, L. A., Abbatt, J. P. D., Stetzer, O., Lohmann, U., Stratmann, F., and Cziczo, D. J.: The SPectrometer for Ice Nuclei (SPIN): an instrument to investigate ice nucleation, Atmos. Meas. Tech., 9, 2781–2795, https://doi.org/10.5194/amt-9-2781-2016, 2016.
Garimella, S., Rothenberg, D. A., Wolf, M. J., David, R. O., Kanji, Z. A., Wang, C., Rösch, M., and Cziczo, D. J.: Uncertainty in counting ice nucleating particles with continuous flow diffusion chambers, Atmos. Chem. Phys., 17, 10855–10864, https://doi.org/10.5194/acp-17-10855-2017, 2017.
Gong, X., Wex, H., Müller, T., Wiedensohler, A., Höhler, K., Kandler, K., Ma, N., Dietel, B., Schiebel, T., Möhler, O., and Stratmann, F.: Characterization of aerosol properties at Cyprus, focusing on cloud condensation nuclei and ice-nucleating particles, Atmos. Chem. Phys., 19, 10883–10900, https://doi.org/10.5194/acp-19-10883-2019, 2019.
Gong, X., Wex, H., van Pinxteren, M., Triesch, N., Fomba, K. W., Lubitz, J., Stolle, C., Robinson, T.-B., Müller, T., Herrmann, H., and Stratmann, F.: Characterization of aerosol particles at Cabo Verde close to sea level and at the cloud level – Part 2: Ice-nucleating particles in air, cloud and seawater, Atmos. Chem. Phys., 20, 1451–1468, https://doi.org/10.5194/acp-20-1451-2020, 2020.
Grawe, S., Jentzsch, C., Schaefer, J., Wex, H., Mertes, S., and Stratmann, F.: Next-generation ice-nucleating particle sampling on board aircraft: characterization of the High-volume flow aERosol particle filter sAmpler (HERA), Atmos. Meas. Tech., 16, 4551–4570, https://doi.org/10.5194/amt-16-4551-2023, 2023.
Gute, E., Lacher, L., Kanji, Z. A., Kohl, R., Curtius, J., Weber, D., Bingemer, H., Clemen, H.-C., Schneider, J., Gysel-Beer, M., Ferguson, S. T., and Abbatt, J. P. D.: Field evaluation of a Portable Fine Particle Concentrator (PFPC) for ice nucleating particle measurements, Aerosol Sci. Tech., 53, 1067–1078, https://doi.org/10.1080/02786826.2019.1626346, 2019.
Hangal, S. and Willeke, K.: Aspiration efficiency: unified model for all forward sampling angles, Environ. Sci. Technol., 24, 688-691, https://doi.org/10.1021/es00075a012, 1990.
Hartmann, M., Blunier, T., Brügger, S. O., Schmale, J., Schwikowski, M., Vogel, A., Wex, H., and Stratmann, F.: Variation of Ice Nucleating Particles in the European Arctic Over the Last Centuries, Geophys. Res. Lett., 46, 4007–4016, https://doi.org/10.1029/2019GL082311, 2019.
Hartmann, M., Adachi, K., Eppers, O., Haas, C., Herber, A., Holzinger, R., Hünerbein, A., Jäkel, E., Jentzsch, C., van Pinxteren, M., Wex, H., Willmes, S., and Stratmann, F.: Wintertime Airborne Measurements of Ice Nucleating Particles in the High Arctic: A Hint to a Marine, Biogenic Source for Ice Nucleating Particles, Geophys. Res. Lett., 47, e2020GL087770, https://doi.org/10.1029/2020GL087770, 2020.
Hill, T. C. J., Moffett, B. F., DeMott, P. J., Georgakopoulos, D. G., Stump, W. L., and Franc, G. D.: Measurement of Ice Nucleation-Active Bacteria on Plants and in Precipitation by Quantitative PCR, Appl. Environ. Microbiol., 80, 1256–1267, https://doi.org/10.1128/aem.02967-13, 2014.
Hill, T. C. J., DeMott, P. J., Tobo, Y., Fröhlich-Nowoisky, J., Moffett, B. F., Franc, G. D., and Kreidenweis, S. M.: Sources of organic ice nucleating particles in soils, Atmos. Chem. Phys., 16, 7195–7211, https://doi.org/10.5194/acp-16-7195-2016, 2016.
Hiranuma, N., Augustin-Bauditz, S., Bingemer, H., Budke, C., Curtius, J., Danielczok, A., Diehl, K., Dreischmeier, K., Ebert, M., Frank, F., Hoffmann, N., Kandler, K., Kiselev, A., Koop, T., Leisner, T., Möhler, O., Nillius, B., Peckhaus, A., Rose, D., Weinbruch, S., Wex, H., Boose, Y., DeMott, P. J., Hader, J. D., Hill, T. C. J., Kanji, Z. A., Kulkarni, G., Levin, E. J. T., McCluskey, C. S., Murakami, M., Murray, B. J., Niedermeier, D., Petters, M. D., O'Sullivan, D., Saito, A., Schill, G. P., Tajiri, T., Tolbert, M. A., Welti, A., Whale, T. F., Wright, T. P., and Yamashita, K.: A comprehensive laboratory study on the immersion freezing behavior of illite NX particles: a comparison of 17 ice nucleation measurement techniques, Atmos. Chem. Phys., 15, 2489–2518, https://doi.org/10.5194/acp-15-2489-2015, 2015.
Hiranuma, N., Adachi, K., Bell, D. M., Belosi, F., Beydoun, H., Bhaduri, B., Bingemer, H., Budke, C., Clemen, H.-C., Conen, F., Cory, K. M., Curtius, J., DeMott, P. J., Eppers, O., Grawe, S., Hartmann, S., Hoffmann, N., Höhler, K., Jantsch, E., Kiselev, A., Koop, T., Kulkarni, G., Mayer, A., Murakami, M., Murray, B. J., Nicosia, A., Petters, M. D., Piazza, M., Polen, M., Reicher, N., Rudich, Y., Saito, A., Santachiara, G., Schiebel, T., Schill, G. P., Schneider, J., Segev, L., Stopelli, E., Sullivan, R. C., Suski, K., Szakáll, M., Tajiri, T., Taylor, H., Tobo, Y., Ullrich, R., Weber, D., Wex, H., Whale, T. F., Whiteside, C. L., Yamashita, K., Zelenyuk, A., and Möhler, O.: A comprehensive characterization of ice nucleation by three different types of cellulose particles immersed in water, Atmos. Chem. Phys., 19, 4823–4849, https://doi.org/10.5194/acp-19-4823-2019, 2019.
Hoose, C. and Möhler, O.: Heterogeneous ice nucleation on atmospheric aerosols: a review of results from laboratory experiments, Atmos. Chem. Phys., 12, 9817–9854, https://doi.org/10.5194/acp-12-9817-2012, 2012.
Jones, H. M., Flynn, M. J., DeMott, P. J., and Möhler, O.: Manchester Ice Nucleus Counter (MINC) measurements from the 2007 International workshop on Comparing Ice nucleation Measuring Systems (ICIS-2007), Atmos. Chem. Phys., 11, 53–65, https://doi.org/10.5194/acp-11-53-2011, 2011.
Kalesse, H., de Boer, G., Solomon, A., Oue, M., Ahlgrimm, M., Zhang, D., Shupe, M. D., Luke, E., and Protat, A.: Understanding Rapid Changes in Phase Partitioning between Cloud Liquid and Ice in Stratiform Mixed-Phase Clouds: An Arctic Case Study, Mon. Weather Rev., 144, 4805–4826, https://doi.org/10.1175/mwr-d-16-0155.1, 2016.
Kanji, Z. A., Ladino, L. A., Wex, H., Boose, Y., Burkert-Kohn, M., Cziczo, D. J., and Krämer, M.: Overview of Ice Nucleating Particles, Meteorol. Monogr., 58, 1.1–1.33, https://doi.org/10.1175/amsmonographs-d-16-0006.1, 2017.
Kanji, Z. A., Sullivan, R. C., Niemand, M., DeMott, P. J., Prenni, A. J., Chou, C., Saathoff, H., and Möhler, O.: Heterogeneous ice nucleation properties of natural desert dust particles coated with a surrogate of secondary organic aerosol, Atmos. Chem. Phys., 19, 5091–5110, https://doi.org/10.5194/acp-19-5091-2019, 2019.
Knackstedt, K. A., Moffett, B. F., Hartmann, S., Wex, H., Hill, T. C. J., Glasgo, E. D., Reitz, L. A., Augustin-Bauditz, S., Beall, B. F. N., Bullerjahn, G. S., Fröhlich-Nowoisky, J., Grawe, S., Lubitz, J., Stratmann, F., and McKay, R. M. L.: Terrestrial Origin for Abundant Riverine Nanoscale Ice-Nucleating Particles, Environ. Sci. Technol., 52, 12358–12367, https://doi.org/10.1021/acs.est.8b03881, 2018.
Knopf, D. A., Alpert, P. A., and Wang, B.: The Role of Organic Aerosol in Atmospheric Ice Nucleation: A Review, ACS Earth Space Chem., 2, 168–202, https://doi.org/10.1021/acsearthspacechem.7b00120, 2018.
Knopf, D. A., Barry, K. R., Brubaker, T. A., Jahl, L. G., Jankowski, K. A. L., Li, J., Lu, Y., Monroe, L. W., Moore, K. A., Rivera-Adorno, F. A., Sauceda, K. A., Shi, Y., Tomlin, J. M., Vepuri, H. S. K., Wang, P., Lata, N. N., Levin, E. J. T., Creamean, J. M., Hill, T. C. J., China, S., Alpert, P. A., Moffet, R. C., Hiranuma, N., Sullivan, R. C., Fridlind, A. M., West, M., Riemer, N., Laskin, A., DeMott, P. J., and Liu, X.: Aerosol–Ice Formation Closure: A Southern Great Plains Field Campaign, B. Am. Meteorol. Soc., 102, E1952–E197, https://doi.org/10.1175/BAMS-D-20-0151.1, 2021.
Krishnamoorthy, K. and Lee, M.: New approximate confidence intervals for the difference between two Poisson means and comparison, J. Stat. Comput. Simul., 83, 2232–2243, https://doi.org/10.1080/00949655.2012.686616, 2013.
Lacher, L., Lohmann, U., Boose, Y., Zipori, A., Herrmann, E., Bukowiecki, N., Steinbacher, M., and Kanji, Z. A.: The Horizontal Ice Nucleation Chamber (HINC): INP measurements at conditions relevant for mixed-phase clouds at the High Altitude Research Station Jungfraujoch, Atmos. Chem. Phys., 17, 15199–15224, https://doi.org/10.5194/acp-17-15199-2017, 2017.
Lacher, L., Clemen, H.-C., Shen, X., Mertes, S., Gysel-Beer, M., Moallemi, A., Steinbacher, M., Henne, S., Saathoff, H., Möhler, O., Höhler, K., Schiebel, T., Weber, D., Schrod, J., Schneider, J., and Kanji, Z. A.: Sources and nature of ice-nucleating particles in the free troposphere at Jungfraujoch in winter 2017, Atmos. Chem. Phys., 21, 16925–16953, https://doi.org/10.5194/acp-21-16925-2021, 2021.
Lacher, L., Adams, M. P., Barry, K., Bertozzi, B., Bingemer, H., Boffo, C., Bras, Y., Büttner, N., Castarede, D., Cziczo, D. J., DeMott, P. J., Fösig, R., Goodell, M., Höhler, K., Hill, T. C. J., Jentzsch, C., Ladino, L. A., Levin, E. J. T., Mertes, S., Möhler, O., Moore, K. A., Murray, B. J., Nadolny, J., Pfeuffer, T., Picard, D., Ramírez-Romero, C., Ribeiro, M., Richter, S., Schrod, J., Sellegri, K., Stratmann, F., Swanson, B. E., Thomson, E., Wex, H., Wolf, M., and Freney, E.: Dataset: The Puy de Dôme ICe Nucleation Intercomparison Campaign (PICNIC): Comparison between online and offline methods in ambient air, 4RadarKit [data set], https://doi.org/10.35097/1876, 2024.
Levin, E. J. T., DeMott, P. J., Suski, K. J., Boose, Y., Hill, T. C. J., McCluskey, C. S., Schill, G. P., Rocci, K., Al-Mashat, H., Kristensen, L. J., Cornwell, G. C., Prather, K. A., Tomlinson, J. M., Mei, F., Hubbe, J., Pekour, M. S., Sullivan, R. J., Leung, L. R., and Kreidenweis, S. M.: Characteristics of ice nucleating particles in and around California winter storms, J. Geophys. Res.-Atmos., 124, 11530–11551, https://doi.org/10.1029/2019JD030831, 2019.
Mason, R. H., Chou, C., McCluskey, C. S., Levin, E. J. T., Schiller, C. L., Hill, T. C. J., Huffman, J. A., DeMott, P. J., and Bertram, A. K.: The micro-orifice uniform deposit impactor–droplet freezing technique (MOUDI-DFT) for measuring concentrations of ice nucleating particles as a function of size: improvements and initial validation, Atmos. Meas. Tech., 8, 2449–2462, https://doi.org/10.5194/amt-8-2449-2015, 2015.
Mason, R. H., Si, M., Chou, C., Irish, V. E., Dickie, R., Elizondo, P., Wong, R., Brintnell, M., Elsasser, M., Lassar, W. M., Pierce, K. M., Leaitch, W. R., MacDonald, A. M., Platt, A., Toom-Sauntry, D., Sarda-Estève, R., Schiller, C. L., Suski, K. J., Hill, T. C. J., Abbatt, J. P. D., Huffman, J. A., DeMott, P. J., and Bertram, A. K.: Size-resolved measurements of ice-nucleating particles at six locations in North America and one in Europe, Atmos. Chem. Phys., 16, 1637–1651, https://doi.org/10.5194/acp-16-1637-2016, 2016.
McCluskey, C. S., Hill, T. C. J., Humphries, R. S., Rauker, A. M., Moreau, S., Strutton, P. G., Chambers, S. D., Williams, A. G., McRobert, I., Ward, J., Keywood, M. D., Harnwell, J., Ponsonby, W., Loh, Z. M., Krummel, P. B., Protat, A., Kreidenweis, S. M., and DeMott, P. J.: Observations of ice nucleating particles over Southern Ocean waters, Geophys. Res. Lett., 45, 11989–11997, https://doi.org/10.1029/2018GL079981, 2018.
Mertes, S., Verheggen, B., Walter, S., Connolly, P., Ebert, M., Schneider, J., Bower, K. N., Cozic, J., Weinbruch, S., Baltensperger, U., and Weingartner, E.: Counterflow Virtual Impactor Based Collection of Small Ice Particles in Mixed-Phase Clouds for the Physico-Chemical Characterization of Tropospheric Ice Nuclei: Sampler Description and First Case Study, Aerosol Sci. Tech., 41, 848–864, https://doi.org/10.1080/02786820701501881, 2007.
Mitts, B. A., Wang, X., Lucero, D. D., Beall, C. M., Deane, G. B., DeMott, P. J., and Prather, K. A.: Importance of Supermicron Ice Nucleating Particles in Nascent Sea Spray, Geophys. Res. Lett., 48, e2020GL089633, https://doi.org/10.1029/2020GL089633, 2021.
Möhler, O., Adams, M., Lacher, L., Vogel, F., Nadolny, J., Ullrich, R., Boffo, C., Pfeuffer, T., Hobl, A., Weiß, M., Vepuri, H. S. K., Hiranuma, N., and Murray, B. J.: The Portable Ice Nucleation Experiment (PINE): a new online instrument for laboratory studies and automated long-term field observations of ice-nucleating particles, Atmos. Meas. Tech., 14, 1143–1166, https://doi.org/10.5194/amt-14-1143-2021, 2021.
Moore, K. A.: Constraining marine ice nucleating particle parameterizations in atmospheric models using observations from the Southern Ocean, Colorado State University, Colorado State University Libraries, https://hdl.handle.net/10217/208435 (last access: 27 February 2024), 2020.
Mülmenstädt, J., Sourdeval, O., Delanoë, J., and Quaas, J.: Frequency of occurrence of rain from liquid-, mixed-, and ice-phase clouds derived from A-Train satellite retrievals, Geophys. Res. Lett., 42, 6502–6509, https://doi.org/10.1002/2015GL064604, 2015.
Murray, B. J., O'Sullivan, D., Atkinson, J. D., and Webb, M. E.: Ice nucleation by particles immersed in supercooled cloud droplets, Chem. Soc. Rev., 41, 6519–6554, https://doi.org/10.1039/C2CS35200A, 2012.
Murray, B. J. and Liu, X.: Chapter 15 – Ice-nucleating particles and their effects on clouds and radiation, in: Aerosols and Climate, edited by: Carslaw, K. S., Elsevier, 619–649, https://doi.org/10.1016/B978-0-12-819766-0.00014-6, 2022.
Ogura, I., Kotake, M., Sakurai, H., and Honda, K.: Surface-collection efficiency of Nuclepore filters for nanoparticles, Aerosol Sci. Tech., 50, 846–856, https://doi.org/10.1080/02786826.2016.1200007, 2016.
Paramonov, M., Drossaart van Dusseldorp, S., Gute, E., Abbatt, J. P. D., Heikkilä, P., Keskinen, J., Chen, X., Luoma, K., Heikkinen, L., Hao, L., Petäjä, T., and Kanji, Z. A.: Condensation/immersion mode ice-nucleating particles in a boreal environment, Atmos. Chem. Phys., 20, 6687–6706, https://doi.org/10.5194/acp-20-6687-2020, 2020.
Paukert, M. and Hoose, C.: Modeling immersion freezing with aerosol-dependent prognostic ice nuclei in Arctic mixed-phase clouds, J. Geophys. Res.-Atmos., 119, 9073–9092, https://doi.org/10.1002/2014jd021917, 2014.
Phillips, V. T. J., Choularton, T. W., Illingworth, A. J., Hogan, R. J., and Field, P. R.: Simulations of the glaciation of a frontal mixed-phase cloud with the Explicit Microphysics Model, Q. J. Roy. Meteor. Soc., 129, 1351–1371, https://doi.org/10.1256/qj.02.100, 2003.
Prenni, A., DeMott, P., Rogers, D., Kreidenweis, S. M., McFarquhar, G. M., Zhang, G., and Poellot, M. R.: Ice nuclei characteristics from M-PACE and their relation to ice formation in clouds, Tellus Ser. B, 61, 436–448, https://doi.org/10.1111/j.1600-0889.2009.00415.x, 2009.
Rogers, D. C.: Development of a continuous flow thermal gradient diffusion chamber for ice nucleation studies, Atmos. Res., 22, 149–181, https://doi.org/10.1016/0169-8095(88)90005-1, 1988.
Rogers, D. C., DeMott, P. J., and Kreidenweis, S. M.: Airborne measurements of tropospheric ice-nucleating aerosol particles in the Arctic spring, J. Geophys. Res.-Atmos., 106, 15053–15063, https://doi.org/10.1029/2000JD900790, 2001.
Schill, G. P., DeMott, P. J., Emerson, E. W., Rauker, A. M. C., Kodros, J. K., Suski, K. J., Hill, T. C. J., Levin, E. J. T., Pierce, J. R., Farmer, D. K., and Kreidenweis, S. M.: The contribution of black carbon to global ice nucleating particle concentrations relevant to mixed-phase clouds, P. Natl. Acad. Sci. USA, 117, 22705–22711, https://doi.org/10.1073/pnas.2001674117, 2020.
Schmidt, S., Schneider, J., Klimach, T., Mertes, S., Schenk, L. P., Kupiszewski, P., Curtius, J., and Borrmann, S.: Online single particle analysis of ice particle residuals from mountain-top mixed-phase clouds using laboratory derived particle type assignment, Atmos. Chem. Phys., 17, 575–594, https://doi.org/10.5194/acp-17-575-2017, 2017.
Schneider, J., Höhler, K., Heikkilä, P., Keskinen, J., Bertozzi, B., Bogert, P., Schorr, T., Umo, N. S., Vogel, F., Brasseur, Z., Wu, Y., Hakala, S., Duplissy, J., Moisseev, D., Kulmala, M., Adams, M. P., Murray, B. J., Korhonen, K., Hao, L., Thomson, E. S., Castarède, D., Leisner, T., Petäjä, T., and Möhler, O.: The seasonal cycle of ice-nucleating particles linked to the abundance of biogenic aerosol in boreal forests, Atmos. Chem. Phys., 21, 3899–3918, https://doi.org/10.5194/acp-21-3899-2021, 2021.
Schrod, J., Kleinhenz, D., Hörhold, M., Erhardt, T., Richter, S., Wilhelms, F., Fischer, H., Ebert, M., Twarloh, B., Della Lunga, D., Jensen, C. M., Curtius, J., and Bingemer, H. G.: Ice-nucleating particle concentrations of the past: insights from a 600-year-old Greenland ice core, Atmos. Chem. Phys., 20, 12459–12482, https://doi.org/10.5194/acp-20-12459-2020, 2020.
Soo, J. C., Monaghan, K., Lee, T., Kashon, M., and Harper, M.: Air sampling filtration media: Collection efficiency for respirable size-selective sampling, Aerosol Sci. Tech., 50, 76–87, https://doi.org/10.1080/02786826.2015.1128525, 2016.
Spurny, K. R. and Lodge, J. P.: Collection Efficiency Tables for Membrane Filters Used in the Sampling and Analysis of Aerosols and Hydrosols, NCAR technical note, University Corporation for Atmospheric Research, Vol. 1, NCAR/TN-77+STR, https://doi.org/10.5065/D6F769JJ, 1972.
Stetzer, O., Baschek, B., Lüönd, F., and Lohmann, U.: The Zurich Ice Nucleation Chamber (ZINC)-A New Instrument to Investigate Atmospheric Ice Formation, Aerosol Sci. Tech., 42, 64–74, https://doi.org/10.1080/02786820701787944, 2008.
Stopelli, E., Conen, F., Zimmermann, L., Alewell, C., and Morris, C. E.: Freezing nucleation apparatus puts new slant on study of biological ice nucleators in precipitation, Atmos. Meas. Tech., 7, 129–134, https://doi.org/10.5194/amt-7-129-2014, 2014.
Sze, K. C. H., Wex, H., Hartmann, M., Skov, H., Massling, A., Villanueva, D., and Stratmann, F.: Ice-nucleating particles in northern Greenland: annual cycles, biological contribution and parameterizations, Atmos. Chem. Phys., 23, 4741–4761, https://doi.org/10.5194/acp-23-4741-2023, 2023.
Tan, I., Storelvmo, T., and Zelinka, M. D.: Observational constraints on mixed-phase clouds imply higher climate sensitivity, Science, 352, 224–227, https://doi.org/10.1126/science.aad5300, 2016.
Twohy, C. H., DeMott, P. J., Russell, L. M., Toohey, D. W., Rainwater, B., Geiss, R., Sanchez, K. J., Lewis, S., Roberts, G. C., Humphries, R. S., McCluskey, C. S., Moore, K. A., Selleck, P. W., Keywood, M. D., Ward, J. P., and McRobert, I. M.: Cloud nucleating particles over the Southern Ocean in a changing climate, Earth's Future, 9, e2020EF001673, https://doi.org/10.1029/2020EF001673, 2021.
Vali, G.: Quantitative Evaluation of Experimental Results and the Heterogeneous Freezing Nucleation of Supercooled Liquids, J. Atmos. Sci., 28, 402–409, https://doi.org/10.1175/1520-0469(1971)028<0402:qeoera>2.0.co;2, 1971.
Vali, G., DeMott, P. J., Möhler, O., and Whale, T. F.: Technical Note: A proposal for ice nucleation terminology, Atmos. Chem. Phys., 15, 10263–10270, https://doi.org/10.5194/acp-15-10263-2015, 2015.
Vergara-Temprado, J., Miltenberger, A. K., Furtado, K., Grosvenor, D. P., Shipway, B. J., Hill, A. A., Wilkinson, J. M., Field, P. R., Murray, B. J., and Carslaw, K. S.: Strong control of Southern Ocean cloud reflectivity by ice-nucleating particles, P. Natl. Acad. Sci. USA, 115, 2687–2692, https://doi.org/10.1073/pnas.1721627115, 2018.
Welti, A., Müller, K., Fleming, Z. L., and Stratmann, F.: Concentration and variability of ice nuclei in the subtropical maritime boundary layer, Atmos. Chem. Phys., 18, 5307–5320, https://doi.org/10.5194/acp-18-5307-2018, 2018.
Wex, H., Augustin-Bauditz, S., Boose, Y., Budke, C., Curtius, J., Diehl, K., Dreyer, A., Frank, F., Hartmann, S., Hiranuma, N., Jantsch, E., Kanji, Z. A., Kiselev, A., Koop, T., Möhler, O., Niedermeier, D., Nillius, B., Rösch, M., Rose, D., Schmidt, C., Steinke, I., and Stratmann, F.: Intercomparing different devices for the investigation of ice nucleating particles using Snomax® as test substance, Atmos. Chem. Phys., 15, 1463–1485, https://doi.org/10.5194/acp-15-1463-2015, 2015.
Wilson, T. W., Ladino, L. A., Alpert, P. A., Breckels, M. N., Brooks, I. M., Browse, J., Burrows, S. M., Carslaw, K. S., Huffman, J. A., Judd, C., Kilthau, W. P., Mason, R. H., McFiggans, G., Miller, L. A., Najera, J. J., Polishchuk, E., Rae, S., Schiller, C. L., Si, M., Temprado, J. V., Whale, T. F., Wong, J. P. S., Wurl, O., Yakobi-Hancock, J. D., Abbatt, J. P. D., Aller, J. Y., Bertram, A. K., Knopf, D. A., and Murray, B. J.: A marine biogenic source of atmospheric ice-nucleating particles, Nature, 525, 234–238, https://doi.org/10.1038/nature14986, 2015.
Wolf, M. J., Coe, A., Dove, L. A., Zawadowicz, M. A., Dooley, K., Biller, S. J., Zhang, Y., Chisholm, S. W., and Cziczo, D. J.: Investigating the Heterogeneous Ice Nucleation of Sea Spray Aerosols Using Prochlorococcus as a Model Source of Marine Organic Matter, Environ. Sci. Technol., 53, 1139–1149, https://doi.org/10.1021/acs.est.8b05150, 2019.
Wolf, M. J., Zhang, Y., Zawadowicz, M. A., Goodell, M., Froyd, K., Freney, E., Sellegri, K., Rösch, M., Cui, T., Winter, M., Lacher, L., Axisa, D., DeMott, P. J., Levin, E. J. T., Gute, E., Abbatt, J., Koss, A., Kroll, J. H., Surratt, J. D., and Cziczo, D. J.: A biogenic secondary organic aerosol source of cirrus ice nucleating particles, Nat. Commun., 11, 4834, https://doi.org/10.1038/s41467-020-18424-6, 2020.