the Creative Commons Attribution 4.0 License.
the Creative Commons Attribution 4.0 License.
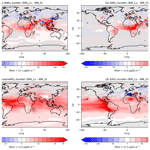
Quantifying the impact of global nitrate aerosol on tropospheric composition fields and its production from lightning NOx
Anthony C. Jones
Jonathan M. Wilkinson
Several global modelling studies have explored the effects of lightning-generated nitrogen oxides (LNOx) on gas-phase chemistry and atmospheric radiative transfer, but few have quantified LNOx's impact on aerosol, particularly when nitrate aerosol is included. This study addresses two key questions: (1) how does including nitrate aerosol affect properties such as tropospheric composition, and (2) how do these effects depend on lightning parameterisation and LNOx levels? Using the Met Office's Unified Model–United Kingdom Chemistry and Aerosol (UM–UKCA) global chemistry–climate model, which now includes a modal nitrate aerosol scheme, we investigate these effects with two lightning-flash-rate parameterisations. Our findings show that both nitrate aerosol and LNOx significantly impact tropospheric composition and aerosol responses. Including nitrate aerosol reduces global mean tropospheric OH by 5 %, decreases the tropospheric ozone burden by 4 %–5 %, increases methane lifetime by a similar amount, and alters the top-of-atmosphere (TOA) net downward radiative flux by −0.4 W m−2. The inclusion of nitrate also shifts the aerosol size distribution, particularly in the Aitken and accumulation modes. A 5.2 Tg N yr−1 increase in LNOx from a zero baseline results in global aerosol increases of 2.8 % in NH4, 4.7 % in fine NO3, 12 % in coarse NO3, and 5.8 % in SO4 mass burdens. This much LNOx increase causes relatively small positive changes in aerosol optical depth, TOA radiative flux, and cloud droplet number concentration compared to when nitrate is included. The results, based on a fast uptake rate for HNO3 to produce NH4NO3, likely represent an upper limit on nitrate effects.
- Article
(8621 KB) - Full-text XML
-
Supplement
(2111 KB) - BibTeX
- EndNote
Liquid or solid particles in the atmosphere, called aerosols, have a considerable impact on Earth's energy budget and thus the climate system and also impact air quality. Aerosols can be emitted directly from both anthropogenic and natural sources (termed primary aerosols), or they can be chemically produced in the atmosphere from precursor gases by condensation of vapours on pre-existing particles or by nucleation of new particles (termed secondary aerosols). Although atmospheric aerosol can comprise many different species, the main groups include sulfate (, or simply SO4), nitrate (, or simply NO3), ammonium (, or simply NH4), carbonaceous aerosols (e.g. organic carbon and black carbon), sea salt, and mineral dust, with their sizes varying from nanometres to tens of micrometres depending on the formation process (Szopa et al., 2021). Apart from exerting a direct radiative forcing (i.e. a change in the energy budget at the top of the atmosphere), aerosols also impact clouds through aerosol–cloud interactions, which in turn impact radiative forcing indirectly.
Sulfate and nitrate are predominantly secondary inorganic aerosols produced from atmospheric oxidation and are often the major components of fine-mode aerosol (Haywood and Boucher, 2000). They are strong scatterers of the incoming solar energy (the “aerosol direct effect”) and thus exert a negative radiative forcing on climate (meaning a cooling of the atmosphere). These aerosols also act as the cloud condensation nuclei (CCN) and modify cloud properties, such as cloud albedo, persistence or lifetime of clouds, and the precipitation rate (the “aerosol indirect effect”).
Sulfate is formed by oxidation of precursor sulfur dioxide (SO2) in the gas phase and in the aqueous phase in cloud droplets. The main precursor species for the chemical formation of nitrate aerosol are reactive gases of nitric acid (HNO3) and ammonia (NH3). HNO3 is the oxidation product of nitrogen oxides (NOx). NOx is a mixture of nitric oxide (NO) and nitrogen dioxide (NO2), and it is primarily emitted from anthropogenic burning of fossil fuel and from natural sources, such as biomass burning, soil emissions, and lightning. It is also an important air pollutant by itself, and it further impacts air pollution and radiation, for example, through net tropospheric ozone (O3) production and changes in tropospheric methane lifetime caused by changes in the hydroxyl radical (OH). Major emissions of NH3 gas include agricultural sources, which include volatilisation of livestock manure and mineral fertiliser application. Sulfate aerosol also indirectly influences nitrate aerosol and O3 levels through changes in oxidation rates and by eroding ammonia concentrations.
Sulfate and nitrate particles (or sulfate and nitrate in particles) can be dry or in aqueous solution, and their production takes place through complex chemical pathways (Finlayson-Pitts and Pitts, 2000). In short, SO2 and NOx are oxidised into sulfuric acid (H2SO4) (liquid aerosol droplets) and HNO3 (atmospheric gas), respectively. H2SO4 reacts with NH3 to produce aerosol of ammonium sulfate (NH4HSO4 and (NH4)2SO4). Thus, tropospheric sulfate aerosol may be considered to consist of sulfuric acid particles that are partially or totally neutralised by NH3. After H2SO4 is neutralised, any excess NH3 then combines with HNO3 to form aerosol of ammonium nitrate (NH4NO3). Low temperature, high relative humidity, and elevated fine particulate matter favour nitrate production (Szopa et al., 2021, and references therein).
Ammonium and nitrate aerosols formed through these gas-to-particle reactions constitute a large portion of fine-mode particles (with a diameter < 1 µm), affecting both climate and air quality, particularly over populous regions in the Northern Hemisphere (Szopa et al., 2021). In addition to fine-mode particles, coarse-mode nitrate aerosol is formed when HNO3 condenses irreversibly onto existing sea-salt and dust aerosols to produce sodium nitrate (NaNO3) and calcium nitrate (Ca(NO3)2) salts, respectively (Li and Shao, 2009). The coarse-mode nitrate aerosol dominates the global mass burden of nitrate, which may be important from the perspective of air quality but has little radiative effect on the solar spectrum compared to the fine-mode nitrate (Bian et al., 2017; Hauglustaine et al., 2014).
Apart from its direct effects on climate and air quality, nitrate aerosol, through its deposition, also plays a part in constraining net primary productivity, thus altering the sequestration of carbon and having ecological effects (Bian et al., 2017). Nitrate aerosol is expected to become even more important in the future atmosphere due to the continued increase in nitrate precursor emissions (e.g. NH3 and NOx) and the decline in SO2 due to stricter emissions regulations (Bellouin et al., 2011; Hauglustaine et al., 2014). For example, this could be due to increasing use of ammonia-based fertilisers and the potential use of ammonia as an effective medium for storing and transporting hydrogen as a fuel in a competitive net-zero-carbon economy. Although nitrate aerosol has been included in some global models, such as the chemical transport model GEOS-Chem (e.g. Park et al., 2004) and the chemistry–climate models GISS (e.g. Bauer et al., 2007) and GFDL (e.g. Paulot et al., 2016), it is often ignored in global chemistry–climate models (Tost, 2017). This may be partly due to the computational cost of simulating nitrate, combined with the chemical complexity of its formation and the semi-volatile nature of ammonium nitrate, which can re-evaporate into the atmosphere (e.g. Stelson et al., 1979). In fact, out of the 10 global Earth system models with atmospheric chemistry that participated in the Aerosol Chemistry Model Intercomparison Project (AerChemMIP) under the Coupled Model Intercomparison Project Phase 6 (CMIP6), which aims to assess the effects of reactive gases and aerosols on Earth's climate, only the GISS and GFDL models explicitly treated nitrate aerosol along with an interactive stratospheric and tropospheric chemistry scheme (Thornhill et al., 2021).
As mentioned above, lightning is a major source of NOx, particularly in the tropical–subtropical middle to upper troposphere where lightning is mostly discharged (Murray, 2016; Bucsela et al., 2019). Although lightning-generated NOx (abbreviated as LNOx) constitutes only about 10 % of the total NOx source emissions globally, it has an inordinately large effect on tropospheric composition (e.g. Murray, 2016; Luhar et al., 2021), such as the OH and O3 mixing ratios. To give an example, whilst NOx emissions from lightning are comparable in magnitude to those from soils or biomass burning, they contribute about 3 times as much to the total tropospheric O3 column (Dahlmann et al., 2011). This is because, in the middle to upper troposphere where lightning NOx is released, the O3 production efficiency per unit of NOx is significantly higher (∼ 100 molecules of O3 per molecule of NOx) compared to near the surface (∼ 10–30 molecules of O3 per molecule of NOx) due to the higher amount of UV radiance, lower concentrations and longer lifetimes of NOx (days instead of hours), and cooler temperatures affecting ozone loss chemistry at such altitudes (Dahlmann et al., 2011).
Previous global modelling studies have demonstrated the importance of LNOx for atmospheric gas-phase chemistry and oxidation capacity (see, for example, Labrador et al., 2005; Schumann and Huntrieser, 2007; Finney et al., 2016; Gressent et al., 2016; Gordillo-Vázquez et al., 2019; and Luhar et al., 2021) and also its impact on cloud cover and atmospheric radiative transfer (e.g. Luhar et al., 2022). Even though these modelling studies did not explicitly include nitrate aerosol processes, an indirect impact of LNOx on aerosol is implicit through perturbations to the oxidation capacity of the atmosphere (e.g. via changes in OH and O3), for example enhancement of new particle formation and aerosol abundances stemming from faster oxidation rates of gas-phase sulfur-to-sulfate conversion (Murray, 2016; Tost, 2017; Luhar et al., 2022).
The area of quantifying the role of LNOx production in aerosol, particularly with nitrate aerosol included, has only received very limited attention compared to its role in gaseous atmospheric composition, and this could be due to reasons such as the inference that the low LNOx emission (∼ 12 %) compared to anthropogenic and biomass burning NOx sources contributes negligibly to nitrate concentrations. To our knowledge, the global modelling by Tost (2017), which involves a modal aerosol scheme with nitrate included, is the only study to explicitly examine the impact of LNOx on aerosol. It shows that LNOx (parameterised via the Price and Rind (1992) (PR92) scheme described below) is a significant source of nitrate in the upper troposphere and influences the aerosol size distribution and radiation. It is reported that chemical conversion of LNOx into HNO3 is more favourable in the middle to upper troposphere, where lightning NOx mostly occurs, as compared to within the atmospheric boundary layer (where the dominant NOx and NH3 sources are located) due to differences in chemical composition, chemical reactivity, and loss processes (Tost, 2017). Tost (2017) points to observational support for the occurrence of both NH3 and NO3 aerosol in convective outflows, meaning that the formation of NH4NO3 is likely, aided by the low temperatures in the upper troposphere. Therefore, LNOx can change the spatial distribution of nitrate concentrations and concomitant climate impacts. Given the emerging importance of nitrate as sulfate concentrations wane, it is important to assess the relative importance of all nitrate sources. At the same time, how modelled global atmospheric composition is impacted when nitrate is accounted for needs to be quantified.
A more comprehensive study involving the sensitivity of tropospheric composition (including aerosol) to global lightning parameterisation with and without nitrate is currently lacking, and the present paper aims to address this. Recently, a nitrate scheme was included in the modal aerosol scheme of the Met Office's Unified Model (UM)–United Kingdom Chemistry and Aerosol (UKCA) global chemistry–climate model, hereafter denoted UM-GA8.0-UKCA or UM–UKCA, where GA8.0 refers to the Global Atmosphere configuration in the UM (Jones et al., 2021). The aerosol scheme in UM–UKCA is a prognostic double-moment scheme (Mann et al., 2010) which transports both the particle number and the mass concentrations in size classes. In this paper, we apply UM–UKCA to study the sensitivity of tropospheric composition and radiation to the lightning parameterisation with and without nitrate aerosol included.
Ultimately, the questions we seek to answer using UM–UKCA are (1) what is the impact of adding nitrate to UM–UKCA on tropospheric composition fields (such as O3, OH, NOx, methane lifetime, and aerosol), aerosol optical depth (AOD), and radiation and (2) what is the dependency of these impacts on the lightning parameterisation in the model? For this purpose, we apply UM–UKCA without and with nitrate and vary LNOx through the use two empirical parameterisations of the lightning flash rate: (a) the PR92 parameterisations for ocean and land which are a function of convective cloud-top height and which most global chemistry–climate models use (Tost, 2017; Archibald et al., 2020) and (b) the flash-rate parameterisations of Luhar et al. (2021) (termed Lu21), which improve upon the PR92 parameterisation for the ocean. Simulations are also conducted with no LNOx. Our modelling can be considered a study of sensitivity of global fields of interest to changes in lightning NOx without and with nitrate aerosol. An outline of the paper is as follows. In Sect. 2, we provide details of the LNOx parameterisations used; Sect. 3 briefly describes the global UM–UKCA model configuration used, with some extra detail about the model's aerosol scheme with nitrate and the model simulation setup; this is followed by results and discussion in Sect. 4 and then conclusions in Sect. 5.
In traditional lightning parameterisations used in general circulation models, the amount of LNOx is estimated as
where PNO is the amount of NO produced per flash. The flash rate F is computed for every model time step and each grid column.
In global chemical transport and chemistry–climate models (including most CMIP6 models), F (flashes per minute) is most commonly parameterised in terms of convective cloud-top height using Price and Rind's (1992) (PR92) formulas for land (FL) and ocean (FO) as follows:
where the convective cloud-top height (H, in km) is diagnosed on a time-step basis from the UM's convection scheme and a minimum cloud depth of 5 km is required for LNOx emissions to be activated. A spatial calibration factor is applied to adjust the above flash-rate formulas for given horizontal model resolutions (Price and Rind, 1994; Luhar et al., 2021). The oceanic flash rates obtained from Eq. (3) are about 2 to 3 orders of magnitude smaller compared to those computed using Eq. (2) for overland clouds.
The calculated flash rate (FL or FO) is divided into intracloud (IC) and cloud-to-ground (CG) components, FIC and FCG, and respective emission factors for the amount of NO released per IC and CG flash, PNO,IC and PNO,CG, are applied; i.e.
The fraction of CG lightning flashes is determined based on cold-cloud thickness, following an empirical relationship developed by Price and Rind (1993), where cold-cloud thickness is further parameterised as a function of latitude. The remaining fraction is then equal to the IC flash fraction. These fractions multiplied with the calculated flash rate (FL or FO) give FCG and FIC, respectively. The calculated NO at a specific location and time step is distributed vertically in the grid column using a linear distribution in log(pressure) coordinates. For IC flashes, this extends from 500 hPa to the cloud top, and for CG flashes, it extends from 500 hPa to the surface (Archibald et al., 2020; Luhar et al., 2021). Equation (3) is known to greatly underpredict flash rates over the ocean. Luhar et al. (2021) tested the PR92 flash-rate formulas in the Australian Community Climate and Earth System Simulator–UKCA (or ACCESS–UKCA) global chemistry–climate model without nitrate aerosol (which is essentially UM–UKCA with the UM at vn8.4) using satellite-based lightning data and concluded that whilst the PR92 formula for land (Eq. 2) performs satisfactorily, the oceanic formula, Eq. (3), predicts a mean global flash rate that is smaller by a factor of approximately 30 compared to the observed rate (a predicted global oceanic average of 0.33 flashes s−1 compared to the observed 9.16 flashes s−1) and thus results in a proportional underestimation of LNOx over the ocean.
Luhar et al. (2021) formulated the following updated flash-rate parameterisations following the scaling relationships between thunderstorm electrical generator power and storm geometry developed by Boccippio (2002), coupled with available data:
In the study by Luhar et al. (2021), Eq. (5) performed very similarly to Eq. (2) in estimating the spatial pattern of the global continental lightning flash rate, giving a global mean value of 35.9 flashes s−1 compared to the satellite climatological value of 38.5 flashes s1. The updated oceanic parameterisation (Eq. 6) simulated the oceanic (and thus the global total ) flash-rate data much better, giving a mean global oceanic flash rate of 9.1 flashes s−1 compared to 7.7 flashes s−1 derived from the satellite climatology. However, there was still an underestimation of flash frequencies in the extratropics, which is probably an inherent limitation of the convective cloud-top-height approach to parameterising F. The updated flash-rate parameterisations resulted in an increase in the global LNOx by ∼ 40 % (from the default value of 4.8 Tg N yr−1), causing a significant change in the tropospheric composition compared to that obtained from the default model run.
There is large uncertainty in the global LNOx amounts. For example, Schumann and Huntrieser (2007) cite an uncertainty of 2–8 Tg N yr−1. Other estimates include 4–8 Tg N yr−1 (Martin et al., 2007) and ∼ 9.0 Tg N yr−1 (Nault et al., 2017). The range of global LNOx in five CMIP6 Earth system models for the present-day conditions ranged from 3.2 to 7.6 Tg N yr−1 (Griffiths et al., 2021; Szopa et al., 2021). A major reason for this is due to the poor constraining of the quantity of NO produced per flash. The default in the UM–UKCA model, as used by Luhar et al. (2021), is × 1026 molecules of NO produced per flash, where it is assumed that the scaling factor (Sf) equals 2. This is equal to PNO=330 mol NO produced per flash, which is close to the middle of the large range of 70–665 mol NO per flash reported in the scientific literature and based on observations (Luhar et al., 2021). Here, we use Sf=1.7, i.e. PNO= 280 mol NO per flash, which can be compared with the 280±80 mol NO produced per flash obtained by Marais et al. (2018) using satellite-based lightning data and the Ozone Monitoring Instrument (OMI) NO2 columns coupled with the GEOS-Chem global chemical transport model and the 310 mol NO per flash suggested by Miyazaki et al. (2014) based on assimilation into a global chemical transport model (CTM) of satellite observations of atmospheric composition and flash distribution.
Because PNO is assumed independent of the IC and CG components in our model, the partitioning of the flash rate into the IC and CG flash rates only affects the shape of the vertical profile of the LNOx distribution. The total amount of LNOx released remains independent of this partitioning.
We use the Met Office Unified Model (UM), which includes global atmosphere and coupled modelling systems from weather to climate timescales. The latest available release (at the time of our research) of the UM (vn13.2) involving the science configurations Global Atmosphere vn8.0 (GA8.0) and Global Land vn9.0 (GL9.0) of the Joint UK Land Environment Simulator (JULES) land surface model was selected. (The new nitrate aerosol scheme was included in the UM at vn11.8 (Jones et al., 2021) with the corresponding science configurations GA7.1 and GL7.0 as described by Walters et al. (2019) and updates between GA7.0 and GA8.0 pertinent to aerosols described in Jones et al. (2022).)
The horizontal resolution of the model is 1.875° longitude ×1.25° latitude, and there are 85 staggered hybrid-height levels which extend from the surface to 85 km in the vertical (the so-called N96L85 climate configuration). The resolution in the vertical becomes coarser, with the lowest 50 levels under 18 km altitude. The model was run in atmosphere-only mode with a dynamical time step of 20 min. Monthly climatologies of sea-surface temperature and sea-ice fields for the year 2000 are prescribed, which are generated by averaging over the 1995–2004 time series data created for CMIP6 atmosphere-only model simulations. This is the same model setup as that of Jones et al. (2021), albeit using the GA8.0 rather than GA7.1 atmosphere model.
Atmospheric composition in the UM is described by the UKCA model (https://www.ukca.ac.uk, last access: 10 December 2024), with the model's chemical solver called every 60 min. The UKCA configuration used here employs a combined stratosphere–troposphere chemistry scheme (namely, StratTrop1.0) (Archibald et al., 2020), which also contains the multi-modal, multi-component, double-moment Global Model of Aerosol Processes-mode (GLOMAP-mode) aerosol microphysics scheme as described by Mann et al. (2010) and Mulcahy et al. (2020). GLOMAP-mode coupled to UKCA chemistry is termed UKCA-mode.
With 84 species and 291 chemical reactions, the StratTrop1.0 scheme in UKCA simulates the chemical cycles of Ox, HOx, NOx, and halogenic compounds; the oxidation of carbon monoxide (CO), methane (CH4), and other volatile organic compounds (VOCs); and heterogeneous chemistry of polar stratospheric clouds and tropospheric aerosols. The Fast-JX scheme (see Telford et al., 2013) is used to model interactive photolysis. Gaseous wet deposition and dry deposition are included.
Atmospheric radiative transfer is described via a two-stream approximation, with nine longwave bands and six shortwave bands (Manners et al., 2023). The radiation changes include both direct aerosol radiative forcing and indirect radiative effects of clouds and atmospheric composition changes. A prognostic cloud fraction and prognostic condensate scheme (PC2) is used to model large-scale clouds (Wilson et al., 2008), and the cloud microphysics is simulated using a single-moment scheme (Wilson and Ballard, 1999) with extensive revisions (as included in GA8.0). The convection scheme is based on a mass flux approach (Gregory and Rowntree, 1990) with several extensions to include convective momentum transport and downdraughts (Walters et al., 2019).
The full modelling system we use is termed UM-GA8.0GL9.0-UKCA, which in this paper is referred to as UM–UKCA.
3.1 UKCA-mode aerosol scheme with nitrate
UKCA-mode as used here is a double-moment aerosol microphysics scheme that transports the aerosol number and mass concentrations in lognormal size modes (four soluble and one insoluble) (Mann et al., 2010; Mulcahy et al., 2020). This is default UKCA-mode setup 2 (see Table 1). For each mode, the median aerosol dry radius is allowed to evolve within specified size ranges, but the lognormal standard deviation (i.e. “mode width”) is kept fixed. This scheme thus resolves the differential growth of particles and their composition across the aerosol size range including internal mixtures. The default UKCA-mode configuration involves sulfate (SO4), organic matter (OM), black carbon (BC), and sea salt (SS). Species in each mode are considered an internal mixture. On the other hand, mineral dust is treated outside of UKCA-mode and is represented by a six-bin scheme developed as part of the older single-moment Coupled Large-scale Aerosol Simulator for Studies in Climate (CLASSIC) framework (Woodward, 2001; Bellouin et al., 2013).
Table 1Aerosol size distribution used in the UKCA-mode aerosol scheme. Species are sulfate (SO4), black carbon (BC), organic matter (OM), sea salt (SS), ammonium (NH4), nitrate (NO3), and coarse nitrate (coarseNO3) (adapted from Jones et al., 2021).
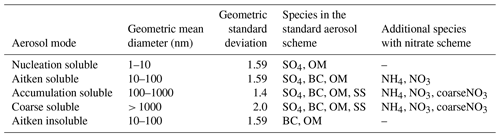
With the new nitrate scheme (Jones et al., 2021), which follows Hauglustaine et al. (2014) and Rémy et al. (2019), ammonium (NH4), nitrate (NO3), and coarse nitrate (denoted coarseNO3) are added to the standard aerosols, namely SO4, OM, BC, and SS, and the new UKCA-mode setup consists of 28 aerosol tracers (23 mass + 5 number concentrations) in total (Table 1) (this UKCA-mode setup together with the CLASSIC dust scheme is referred to as setup 10). Note that here “NO3” refers exclusively to semi-volatile NO3 linked with NH4, whereas “coarseNO3” pertains to stable NO3 related to sea salt and dust. NH4 and NO3 mass is introduced into the Aitken soluble and accumulation soluble modes, and it may be moved to the coarse soluble mode through coagulation and mode merging, whereas coarseNO3 is restricted to the accumulation soluble and coarse soluble modes. Only nitrate condensing on bins 2–6 (with bin 2 and half of bin 3 mapped to the accumulation mode and the other half of bin 3 and bins 4, 5, and 6 mapped to the coarse mode) of the CLASSIC mineral dust scheme is considered. The nitrate scheme requires NH3 and HNO3 to be available (emission of NH3 is prescribed, while gaseous HNO3 is produced by various chemical reactions in the atmosphere). Full detail of the methodology is reported by Jones et al. (2021).
The component of the new nitrate scheme dealing with the formation of fine-mode NH4NO3 from the condensation of HNO3 and NH3 is numerically solved first, prior to the condensation of HNO3 on coarse aerosols (i.e. dust and sea salt). Most fine-mode nitrate schemes assume that NH4NO3 concentrations reach thermodynamic equilibrium instantaneously, without accounting for the kinetic limitations on the condensation of HNO3 or NH3 onto existing aerosol particles. Instead, our quasi-instantaneous thermodynamic equilibrium scheme assumes an exponential decay of the gas phase towards equilibrium, using an equilibration timescale (τe). This approach is based on Schwartz's (1986) first-order uptake theory and incorporates correction factors from Fuchs and Sutugin (1970) to account for molecular effects and limitations in interfacial mass transport. τe is a function of the HNO3 condensation or uptake rate coefficient (γ), a key parameter in the first-order uptake theory and defined as the number of gas molecules condensing on a particle divided by the number impacting onto the particle surface. The higher the uptake coefficient, the smaller the equilibration timescale. The benefit of using such a scheme is that it realistically constrains the rate at which NH4NO3 concentrations achieve equilibrium.
Jones et al. (2021) tested the sensitivity of NH4NO3 aerosol concentrations to the HNO3 uptake coefficient for the NH3–HNO3 uptake on Aitken and accumulation soluble particles (Table 1) with two values selected from the literature, γ=0.193 (FAST) and 0.001 (SLOW), representing fast and slow uptake rates, respectively. They found that, generally, the fast uptake value shows a higher spatial correlation with measured nitrate surface concentrations, whereas the slow value simulates their magnitudes better. These authors also found that compared to FAST, the SLOW value led to a 58 % and 52 % reduction in the global near-surface concentration and burden of fine particulate nitrate, respectively. The reductions in NH4 were 24 % and 15 %, while coarseNO3 remained almost unchanged. Aerosol optical depth decreased by 6 %, and the magnitude of the top-of-atmosphere (TOA) net downward radiative flux changed by 63 %. This sensitivity test showed that despite a 200-fold variation in the uptake rate, the model's response was nonlinear and perhaps less sensitive than expected. In this study, we use the FAST value γ=0.193, which is currently the default in UKCA-mode. Jones et al. (2021) showed that this value produces similar results globally to the widely utilised assumption of instantaneous thermodynamic equilibrium. This suggests that our results likely represent the upper end of efficiency of NH4 and NO3 production and its impact in the UM. Jones et al. (2021) recognised that rather than being globally invariant, γ may vary with aerosol composition, temperature, and relativity humidity and needs better constraining; thus this needs further research and future model development outside the scope of the present study.
In our nitrate scheme, coarse nitrate is present in the accumulation and coarse soluble modes. Following NH4NO3 production and the associated update to HNO3 concentrations, the first-order uptake parameterisation is further employed to model the irreversible uptake of HNO3 on sea salt and dust to produce NaNO3 and Ca(NO3)2, respectively. This reaction is slower than ammonium nitrate production; therefore numerically ammonium nitrate production is solved first. The HNO3 uptake coefficients for CLASSIC dust and sea salt are relative-humidity-dependent variables based on measurements from Fairlie et al. (2010) and Sander et al. (2011), respectively. Dust is assumed to uniformly constitute 5 % Ca2+ by mass (Jones et al., 2021).
The model's radiation scheme and treatment of hygroscopic growth have been modified to include the direct radiative effects of nitrate aerosol and the indirect impacts on clouds, enabling a comprehensive nitrate impact assessment in future UM studies (Jones et al., 2021).
3.2 Model simulation setup
We conducted six UM–UKCA simulations for various combinations of the LNOx and nitrate schemes, including no-nitrate and no-LNOx cases (Table 2). The model experimental setup is very similar to that by Jones et al. (2021). All simulations are initialised with results from a previously spun-up model experiment and are run for the model years 1989–2008. To further eliminate any spin-up effects, model output from only the last 15 years (1994–2008) is used for analysis. The model is forced by prescribed sea-surface temperature and sea-ice fields specified as monthly varying climatologies for the “year 2000”, which are essentially averages over the 1995–2004 time series data developed for CMIP6 atmosphere-only simulations. No meteorological nudging is used so as to allow feedback mechanisms to create an individual climate state. The use of perpetual year-2000 conditions in our simulation design follows standard simulation protocol for the development of the UKCA model in the Met Office. For statistical robustness of the feedbacks included in the model, instead of examining individual years of the simulations, we present results that are averages over the 15-year simulation period.
Table 2Details of six UM–UKCA simulations performed in this study. The indicated lightning schemes are Price and Rind (1992) (PR92) and Luhar et al. (2021) (Lu21). The uncertainty corresponds to a 1σ standard deviation calculated from annual means over 15 years of simulation after linear detrending (sample size = 15).
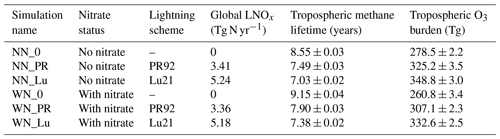
The gaseous and aerosol emissions are mainly prescribed from the CMIP6 historical emissions inventory as monthly fields, and they include anthropogenic, biomass burning, and natural categories. Jones et al. (2021) provide further details on these emissions including the global total for each UKCA chemical species. In our simulations, monthly emissions for the year 2000, which are generated by averaging over the 1995–2004 time series data, are used and the emissions are computed for every model time step of 20 min. The only extra species emission used by the model when the nitrate scheme is invoked is that of NH3, whose global total was 53.6 Tg N yr−1 (Table 3).
The global total NOx emission (excluding LNOx) was 49.5 Tg N yr−1 (Table 3), of which the soil, anthropogenic surface, biomass burning, and aircraft components were 11.1 %, 72.3 %, 15.2 %, and 1.4 %, respectively. Note that the LNOx emission is not included in the input emissions database and is generated interactively as described in Sect. 2.
Concentrations of the long-lived species CO2, CH4, N2O, and O3-depleting substances are prescribed as lower boundary conditions at the surface (Archibald et al., 2020). All simulations used the optional two-layered oceanic process-based scheme proposed by Luhar et al. (2018) (configuration corresponding to Ranking 1 in their Table 1) for dry deposition ozone to the ocean.
In the following section, we present the impact of LNOx on tropospheric composition and radiation with and without nitrate aerosol.
4.1 Modelled lightning flash rate and LNOx
Luhar et al. (2021) previously evaluated the flash-rate parameterisations Eqs. (2)–(6) within their ACCESS–UKCA model (which is essentially UM–UKCA vn8.4 (with GA4.0) and which does not have nitrate aerosol) using the Lightning Imaging Sensor (LIS)/Optical Transient Detector (OTD) satellite data of global flash-rate distribution (Cecil et al., 2014). Here, we have used the above flash-rate parameterisations in UM–UKCA at vn13.2 (with GA8.0), both with and without nitrate – this model version has basically the same convection scheme as in vn8.4 and therefore results in similar global flash-rate distributions. Here, we only do a limited comparison of the simulated flash-rate density with the LIS/OTD climatology since a comprehensive evaluation (including a seasonal comparison) has already been performed by Luhar et al. (2021).
The LIS/OTD flash density climatology in Fig. 1a shows high overland values in the tropics and subtropics, as well as in lower mid-latitudes. Some relatively high levels are also observed over water at these latitudes, especially over the western Atlantic, the Pacific, the western Indian Ocean close to southern Africa, and the waters around the maritime continent. The modelled flash density distribution in Fig. 1b obtained using the PR92 scheme is able to reproduce the broad observed distribution at low latitudes over land (barring parts of India); however, it is apparent that it does not simulate the extension of the observed flash density over the temperate latitudes well, especially in the Northern Hemisphere (NH). It is also clear that the PR92 scheme predicts nearly zero marine flash density, contrary to the observations. On the other hand, the Lu21 scheme reproduces the observed distribution of lightning flash density over the ocean considerably better than the PR92 scheme does (Fig. 1b), although some significant spatial differences are visible (for example, high bias over the Pacific Ocean and equatorial Indian Ocean and low bias over western Indian Ocean close to southern Africa) compared to the LIS/OTD climatology. The simulated overland distributions in Fig. 1b and c are very similar.
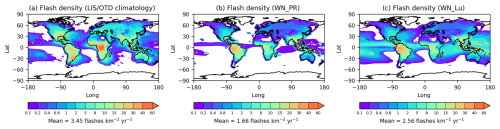
Figure 1Global distribution of the mean lightning flash density (flashes km−2 yr−1): (a) the LIS/OTD satellite climatology, (b) the modelled distribution using the PR92 flash-rate parameterisations, and (c) the modelled distribution using the Lu21 flash-rate parameterisations (both simulations with nitrate aerosol included).
The simulated flash-rate distributions in Fig. 1b and c show a few small areas with a relatively high flash rate in the western equatorial Pacific Ocean (i.e. mostly areas east of the Philippines), and this is most likely because the land–sea mask used at N96 resolution in the present model to distinguish between land and ocean for flash-rate calculation treats these grid point areas as land. Noting that there do not seem to be corresponding high-flash-rate spots in the observations (Fig. 1a), we recommend that these grid points be treated as water in the land–sea mask in future versions of UM–UKCA (or alternatively a minimum land fraction threshold for lightning onset could be applied).
With the present UM–UKCA, the total global lightning flash frequency obtained from the PR92 scheme without nitrate is 27.7 flashes s−1, of which 27.2 flashes s−1 is over the land and 0.5 flashes s−1 is over the ocean. The corresponding values for the Lu21 scheme are 42.5, 30.9, and 11.6 flashes s−1. For comparison, the values obtained from the LIS/OTD climatology are 46.3, 38.5, and 7.7 flashes s−1 (Cecil et al., 2014; Luhar et al., 2021), respectively, showing a considerable improvement in the flash-rate estimate by the Lu21 scheme over the ocean. In the study by Luhar et al. (2021) using UM vn8.4, the same PR92 scheme yielded 32.9, 32.5, and 0.4 flashes s−1, respectively, and the same Lu21 scheme yielded 45.0, 35.9, and 9.1 flashes s−1, respectively. These differences in the flash rate between the two model versions show the impact of incremental changes made in the model, although the approach used in the convection scheme remains the same in both model versions.
When nitrate is explicitly added to the model, the PR92 scheme gives 27.3 flashes s−1 and the Lu21 scheme gives 42.0 flashes s−1 for the globe. Therefore, there is a very small decrease (∼ 1.3 %) in flash frequency for both the PR92 and the Lu21 schemes with nitrate in UM–UKCA, which demonstrates how inclusion of nitrate aerosol may influence the lightning flash rate itself. While this is interesting, we have not investigated this further given that these are atmospheric-only simulations and ocean feedbacks may change the impact of nitrate on flash rate. Note that this nitrate–lightning feedback may be due to indirect effects of nitrate aerosol through cloud microphysics and radiation that impact model's meteorology and convection scheme (also note that there is high sensitivity of the parameterised flash rate to cloud-top height due to its almost fifth-power dependence on the latter, so even minute variations in cloud-top height can cause significant changes in the calculated flash rate). In this context, there is some work performed by Wang et al. (2018) on the effects of aerosols on NOx production by lightning via direct and indirect aerosol effects.
Since PNO has a fixed value, the above differences in the modelled flash rate are reflected in a nearly linear proportion in the global LNOx amounts obtained from the various model runs (see Table 2).
4.2 Impact of nitrate aerosol on gas-phase tropospheric composition
The importance of LNOx for gas-phase tropospheric composition has previously been demonstrated by several studies (e.g. Labrador et al., 2005; Gordillo-Vázquez et al., 2019; and Luhar et al., 2021). Below, we examine the impact of inclusion of nitrate on the modelled gas-phase tropospheric composition, viz. total O3, NOx, OH, and CO and methane lifetime, by considering the “no-nitrate” (NN_Lu) and “with-nitrate” (WN_Lu) runs involving the Lu21 flash-rate parameterisation. (Table 4 summarises the global averages of various atmospheric fields obtained from the no-nitrate and with-nitrate simulations for the three lightning NOx options. The change per Tg N yr−1 of LNOx based on the slopes of linear least-squares fits is also given).
Table 4Modelled global averages of various atmospheric variables obtained from the no-nitrate (NN) and with-nitrate (WN) simulations for three lightning NOx setup options: no LNOx, Price and Rind's (1992) (PR92 or PR) lightning scheme, and Luhar et al. (2021) (Lu21 or Lu) lightning scheme. All species are tropospheric averages, aerosol no. is aerosol number concentration, nu denotes nucleation soluble mode, Ai denotes Aitken soluble mode, Ai (in) denotes Aitken insoluble mode, ac denotes accumulation soluble mode, co denotes coarse soluble mode, and CDNC denotes cloud droplet number concentration.
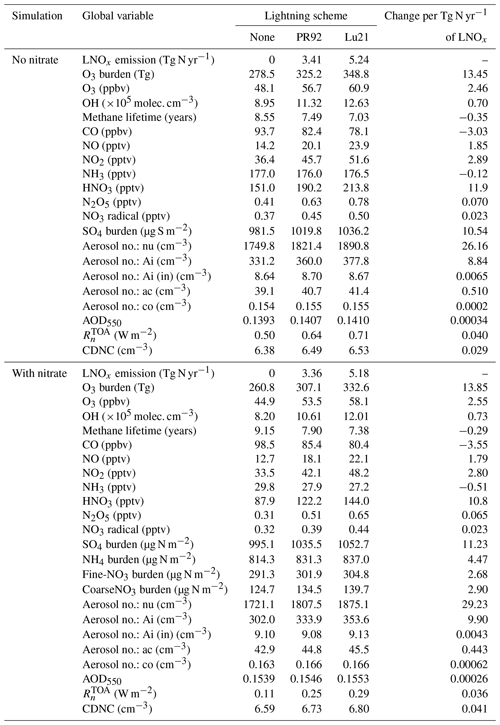
The modelled near-surface (at the lowest 20 m model level) O3 mixing ratio (ppbv) distribution in Fig. 2a and b suggests that both NN_Lu and WN_Lu cases look qualitatively very similar, showing relatively high levels in the Northern Hemisphere, particularly within 0–50° N, which can be associated with the higher precursor emissions in these regions. However, it is apparent from the difference plot (Fig. 2c) that there is a global decrease in near-surface O3 when nitrate is included. This decrease is as much as 3 ppbv within the above-mentioned latitudinal region, and on average it is 4.3 % (from the global mean of 29.9 ppbv). Annually averaged data from our 15-year model simulation (sample size of 15) were used in a t test to determine whether the means of two populations differed significantly at a 95 % confidence level. Figure 2c also displays hatched areas of statistically insignificant difference (so significance level less than 5 %), which suggest that the differences are significant over most of the globe.
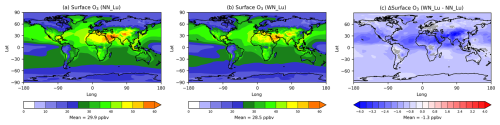
Figure 2Near-surface ozone concentration (ppbv) modelled (a) without (NN_Lu) and (b) with (WN_Lu) the nitrate scheme. The difference (WN_Lu − NN_Lu) is shown in (c). The Lu21 lightning-flash-rate parameterisation was used. The difference plot (c) also shows hatched areas representing statistically insignificant difference (significance level less than 5 %).
In the model, the tropopause is defined as follows. In the extratropics (latitude °), the tropopause is the pressure level of the 2 PVU (potential vorticity units) surface, and in the tropics (latitude °) it is the pressure level of the 380 K potential temperature isentropic surface. Between the two latitudes, a weighted average of the two definitions is used following the method of Hoerling et al. (1993). The zonal-mean tropospheric O3 levels from the two runs also look very similar qualitatively (Fig. 3a and b) with less O3 in the lower troposphere (altitudes < 5 km) over the Southern Hemisphere (SH) compared to that over the Northern Hemisphere. Inclusion of nitrate results in O3 decreases throughout the troposphere (Fig. 3c) with the biggest decreases being located in the middle to upper troposphere in the Northern Hemisphere. The volume-weighted global mean tropospheric O3 obtained with nitrate is 58.1 ppbv, which is 4.6 % lower than with no nitrate. Similarly, the tropospheric ozone burden decreases by the same relative amount (by 16 Tg from 348.8 to 332.8 Tg) with nitrate (see Table 2). Recently, an evaluation study by Russo et al. (2023) involving UM–UKCA (without nitrate) and focusing on the North Atlantic region suggests a considerable model positive bias for ozone in the tropical upper troposphere. These authors attribute this to shortcomings of the model's convection and lightning parameterisations (the latter are based on the convective cloud-top-height approach), which underestimate lightning flashes in mid-latitudes relative to the tropics; this was also evident in the study by Luhar et al. (2021). However, it is clear from Fig. 3c that the inclusion of nitrate in the model may alleviate the model's positive ozone bias in the tropical upper troposphere to some extent.
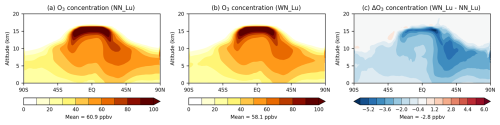
Figure 3Zonal-mean tropospheric ozone (ppbv) modelled (a) without and (b) with the nitrate scheme. The difference between (b) and (a) is shown in (c). The Lu21 lightning-flash-rate parameterisation was used.
The OH radical is the controlling oxidant in the global atmosphere and governs the chemical lifetime of most anthropogenic and natural gases, for example CH4 and CO. Concentration of OH in the troposphere is governed by a complex set of chemical reactions involving species such as tropospheric O3, CH4, CO, nonmethane VOCs, and NOx, as well as by the amount of humidity and solar radiation (e.g. Naik et al., 2013). The nitrate aerosol mechanism is linked to some of these reactions (e.g. those involving HNO3), which leads to changes tropospheric OH. The simulated zonal-average tropospheric OH in Fig. 4a shows high OH levels in the tropical lower troposphere, particularly near the surface within 10–30° N with values as high as (20–25) × 105 molec. cm−3. The OH concentrations decline with height, but a secondary maximum in the tropical upper troposphere (at ∼ 13 km) is apparent, likely linked to LNOx emissions. The corresponding plot in Fig. 4b with nitrate indicates some decrease in OH, and this is more apparent in the difference plot in Fig. 4c, where there is a decrease in OH everywhere with the largest changes being as much as 1.3×105 molec. cm−3 within 0–40° N in the Northern Hemisphere. Overall, there is a decline of 5.0 % in the global tropospheric OH when nitrate is included in the model.
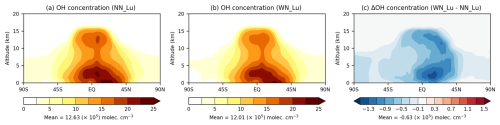
Figure 4Zonal-mean tropospheric OH (×105 molec. cm−3) simulated (a) without and (b) with the nitrate scheme. The difference between (b) and (a) is shown in (c). The Lu21 lightning-flash-rate parameterisation was used.
As a consequence of the decrease in OH with nitrate in the model, the methane lifetime () with respect to loss by OH in the troposphere increases by 5 % from 7.0 years, and on average the tropospheric CO goes up by 3.0 % from the global average value of 78.1 ppbv, as compared to the simulation with no nitrate. On the other hand, there is a decrease in NO by 7.5 % and in NO2 by 6.7 % owing to the swift removal of particulate nitrate from the atmosphere compared to gas-phase nitrogen species. In Fig. 5a, plotted as a function of LNOx for all simulations (Table 2) suggests that within the range of LNOx considered, on average, is greater by ∼ 0.4 years when nitrate is included. This approximately equates to the increase in as a result of a decrease of 1 Tg N yr−1 in LNOx (using the slopes of the linear best-fit lines in Fig. 5a). The 1σ standard deviation uncertainty in in Fig. 5a (and in Table 2) calculated from the annual means over 15 years of simulation after linear trending is on average ±0.027 years, which is much smaller than the mean decrease of ∼ 1.7 years within the range of LNOx considered. In Fig. 5b, the reduction in the ozone burden in the troposphere when using the nitrate scheme is almost uniform at 16.5 Tg O3 across the LNOx values considered, and this magnitude is comparable to about 13 Tg O3 generated per unit teragram of N per year of LNOx. This result is perhaps somewhat contrary to that reported by Tost (2017), who deduced that the nitrate formation does not have an important influence on the distribution of tropospheric ozone. The tropospheric O3 burden obtained by Luhar et al. (2020, 2021) with an older version of UM–UKCA (without nitrate) that used the Lu21 scheme (giving an LNOx of 6.6–6.9 Tg N yr−1) was 304–308 Tg O3, which is considerably lower than 348.8 Tg O3 obtained from the corresponding present simulation NN_Lu. Notwithstanding the various differences between the two simulations (differences in prescribed emissions, updated model schemes and parameters, nudging, etc.), one important reason for this difference is that the old version of UKCA StratTrop included the chemical reaction HO2+ NO → HNO3, which acts as a significant sink of HOx (OH, HO2) in the upper troposphere and thus reduces ozone. However, based on further studies (e.g. Mertens et al., 2022), it was determined that this reaction was not important, and it was omitted in later versions of StratTrop. The uncertainty in the ozone burden in Fig. 5b (and in Table 2) is on average ±2.9 Tg O3, which is much smaller than the mean increase of ∼ 70 Tg O3 within the LNOx range considered.
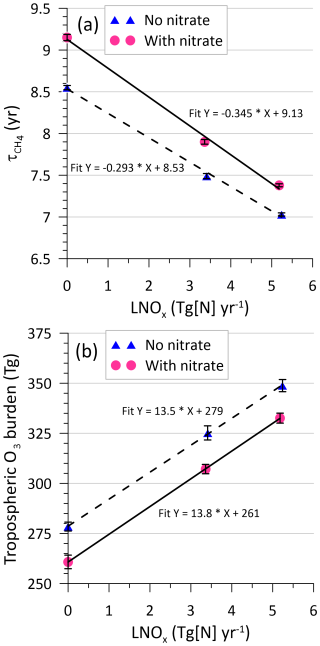
Figure 5(a) Modelled methane lifetime () due to loss by tropospheric OH, as a function of lightning-generated NOx, and (b) the same plot but for tropospheric O3 burden. The lines are linear least-squares fits, and the error bars correspond to a 1σ standard deviation.
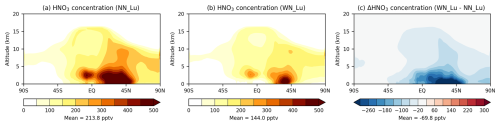
Figure 6Zonal-mean tropospheric HNO3 (pptv) modelled (a) without and (b) with the nitrate scheme. The difference between (b) and (a) is shown in (c). The Lu21 lightning-flash-rate parameterisation was used.
When nitrate aerosol is considered, there is a decrease in the HNO3 mixing ratio throughout the troposphere (Fig. 6), mostly in the lower troposphere and between 0–45° N (where most of the surface NH3 emissions are located), as part of the gas-phase HNO3 is used up in the nitrate aerosol mechanism (following neutralisation by the uptake of ammonia). On average, this reduction is 33 % compared to the no-nitrate value. The distribution of the reduction in Fig. 6c is qualitatively very similar to that in NH3, with the latter decreasing by 85 % (plot not shown) compared to the no-nitrate case. We find that on average 1 Tg N yr−1 of LNOx emission results in a 11 pptv increase in the tropospheric nitric acid mixing ratio with or without nitrate.
We have also looked at the gaseous nitrate radical (NO3) (different from nitrate aerosol) and N2O5 (dinitrogen pentoxide) mixing ratios. The NO3 radical has relatively low concentration, but it is the controlling nighttime oxidant (the counterpart of OH in the daytime) (Archer-Nicholls et al., 2023). It is generated by the reaction involving NO2 and O3 and reacts rapidly with unsaturated VOCs (e.g. isoprene and terpenes), thus affecting their atmospheric budgets as well as their degradation products (Khan et al., 2015). The NO3 radical reacts further with NO2 during the nighttime to form N2O5 (dinitrogen pentoxide), another important nighttime species whose uptake onto aerosol removes NOx from the atmosphere and produces nitric acid (Brown et al., 2006). We find that with the nitrate aerosol scheme, NO3 radical is decreased by 11.5 % overall from the tropospheric mean value of 0.5 pptv and, similarly, N2O5 goes down by 16.4 % from the tropospheric mean value of 0.78 pptv.
4.3 Impact of LNOx on aerosol mass concentration
Previous modelling studies have shown that lightning NOx does lead to a significant increase in particulate nitrate concentrations (Tost, 2017). Jones et al. (2021) have presented results with nitrate aerosol that were obtained with the PR92 scheme but without explicitly looking at sensitivity to lightning. Tost (2017) has investigated no-LNOx and LNOx (with the PR92 scheme) cases with nitrate included. We now investigate whether the changing of the LNOx parameterisation (to Lu21) significantly impacts NO3 concentrations. The three simulations (no-LNOx, PR92, and Lu91) with and without nitrate also provide three quantities of global LNOx against which the globally averaged parameter values can be plotted.
Ammonium nitrate aerosol is formed from HNO3 condensation onto pre-existing aerosols, mainly thermodynamically stabilised by ammonium (). The modelled column annual-mean mass burdens of NH4 (from both (NH4)2SO4 and NH4NO3), fine NO3 (from NH4NO3), coarseNO3 (from both NaNO3 and Ca(NO3)2), and SO4 from the PR92 and Lu21 simulations and the differences between the two simulations (Lu21 − PR92) are shown in Fig. 7 (top two rows). As fine NO3 is associated with NH4, their spatial distributions are nearly the same, with relatively high burdens over land in the Northern Hemisphere, particularly the values as high as 3 mg N m−2 in South Asia, 2 mg N m−2 in East Asia and China, and 1.1 mg N m−2 in central North America.
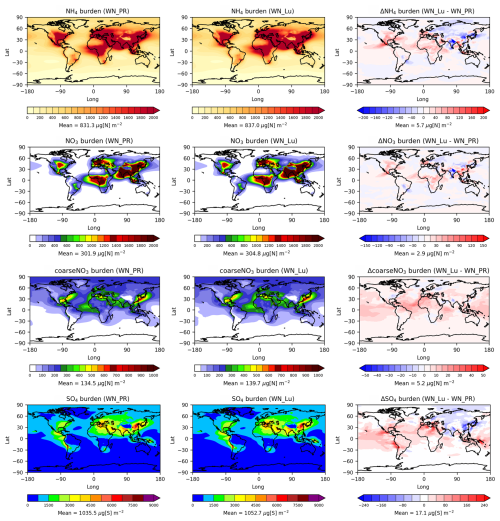
Figure 7Annual-mean tropospheric mass burdens of NH4, fine NO3, coarseNO3, and SO4 from the PR92 (left column) and Lu21 (middle column) simulations (both with nitrate) and the difference (right column) between the two simulations.
Moving from the PR92 to the Lu21 scheme, there is a very small global mean increase (∼ 1 %) in both NH4 and fine-NO3 burdens as LNOx increases from 3.36 to 5.18 Tg N yr−1. This increase is dominated by particle mass in the Aitken mode for NH4 (68 %) and accumulation mode for fine NO3 (77 %). There is a greater increase in the coarseNO3 (as NaNO3), at ∼ 4 %.
However, the global difference plots in Fig. 7 show considerable regional variations in the changes in aerosol burden, with the Lu21 scheme predicting larger fine-nitrate concentrations over south-eastern China (by 90 µg N m−2), North America and central Africa (by 40 µg N m−2), and western Europe (by 50 µg N m−2). In contrast, the Lu21-predicted nitrate is lower over India (by as much as 100 µg N m−2), north-east Asia, and eastern Europe. A very similar qualitative difference distribution is obtained for NH4. For coarse nitrate, there are increases over most of the globe, with larger magnitudes in the tropics (by ∼ 15 µg N m−2) compared to the PR92 scheme.
Sulfate aerosol is produced when OH oxidises SO2 in the presence of water vapour to form H2SO4, which either nucleates or condenses on existing particles, depending upon its concentration. The distributions of the SO4 burden in Fig. 7 (bottom panels) show a global increase of ∼ 1.7 % with the Lu21 scheme, and this increase is dominated by the particles in the Aitken mode (55 %) and accumulation mode (42 %). There are relatively large increases (∼ 70 µg S m−2) over most of the tropics, but burdens decrease over Russia, India, China, and north-east Asia.
The tropospheric burdens of NH4, fine NO3 and coarseNO3, and SO4 for the Lu21 scheme with nitrate are 0.43 Tg N, 0.16 Tg N, 0.07 Tg N, and 0.54 Tg S, respectively. These values can be compared with the global NH4, fine-mode NO3, and SO4 burdens of 0.13–0.58 Tg N, 0.03–0.42 Tg N, and 0.28–1.10 Tg S, respectively, obtained from the AeroCom phase III model intercomparison study by Bian et al. (2017).
The above differences can be compared with those between the Lu21 scheme and when LNOx is set to zero (which means the global LNOx difference between the two cases is 5.18 Tg N yr−1). Figure 8 is the same as Fig. 7 (right), except that the difference is between the Lu21 scheme and the no-LNOx case. Hatched areas representing statistically insignificant difference (significance level less than 5 %) are also plotted. On average globally, there is a mean increase of 2.8 % in NH4, 4.7 % in fine NO3, 12 % in coarseNO3, and 5.8 % in SO4. This increase is dominated by particle mass in the Aitken mode for NH4 (68 %), accumulation mode for fine NO3 (77 %), coarse mode for NaNO3 (73 %), and Aitken mode for SO4 (66 %). Taken together, Figs. 7 and 8 indicate that updating the LNOx parameterisation from PR92 to Lu21 has a substantial impact on the LNOx-mediated nitrate aerosol concentrations.
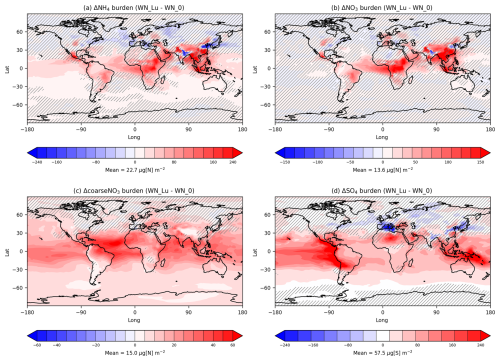
Figure 8Difference between the Lu21 and no-LNOx simulations (both with nitrate) for annual-mean tropospheric (a) NH4, (b) fine-NO3, (c) coarseNO3, and (d) SO4 burdens. The hatched areas represent statistically insignificant difference (significance level less than 5 %).
The zonal-mean vertical distributions of NH4, fine NO3, coarseNO3 (related to sea salt and dust), and SO4 mass concentrations from the Lu21 simulation (with nitrate) shown in Fig. 9 (left) suggest that these species are mostly confined to the lower troposphere (as their precursors are emitted from sources close to the surface). High concentrations are predicted near the surface between the Equator and 60° N, which corresponds to the locations of the main precursor emissions (i.e. NH3 and NOx). It is apparent that the vertical extent of fine NO3 is limited because of the rapid wet removal of NH3 gas from the atmosphere due to its high effective solubility, which in turn limits the vertical range to which NH4NO3 may be established by condensation. Conversely, near the surface over land regions, NH4NO3 production is HNO3-limited due to the much greater concentrations of NH3 from agricultural sources (Jones et al., 2021). NH4 would reach a greater altitude than fine NO3, due to its long-lived association with SO4 aerosol. The coarseNO3 (NaNO3+ Ca(NO3)2) aerosol is also confined to near the surface as they are easily removed from the atmosphere by wet deposition and gravitational settling.
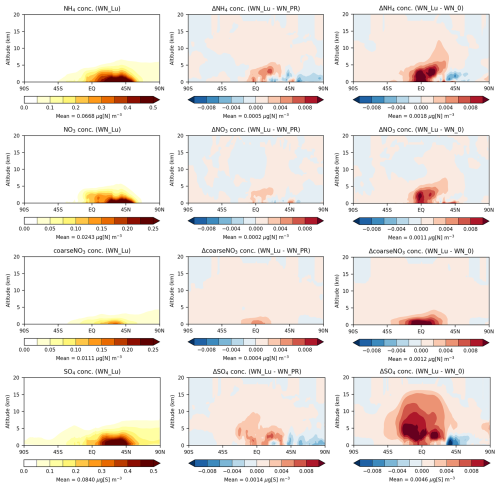
Figure 9Zonal-mean tropospheric NH4, fine-NO3, coarseNO3, and SO4 concentrations from the Lu21 simulation with nitrate (left column), the difference between the Lu21 and PR92 simulations (Lu21 − PR92) (middle column), and the difference between the Lu21 and no-LNOx simulations (Lu21 − no-LNOx) (right column).
The differences between the Lu21 and PR92 simulations (Lu21 − PR92) (Fig. 9, middle) indicate that with the Lu21 scheme there are noticeable increases in NH4 and fine-NO3 mass concentrations around the Equator below 4 km and an elevated maximum at an altitude of 3 km at around 15° N. While most of the LNOx would be distributed vertically within the middle to upper troposphere in the tropics, NH3 is mostly released at the surface between 0–60° N, and thus these are the locations within the lower troposphere where LNOx results in an optimal formation of NH4 and fine NO3. This is clearer from the difference between the Lu21 and no-LNOx simulations (Lu21 − no-LNOx) (Fig. 9, right), where the differences are much greater but the qualitative shapes of the difference patterns are very similar. This suggests that the differences in the distribution are governed by a balance between the availability of LNOx and that of NH3. There are areas of small decreases in the lower troposphere in the Northern Hemisphere beyond 40° N. With regard to the coarseNO3, the increases are mostly confined near the surface and symmetrically centred around the Equator, which suggests that the differences in the distribution are dominated by LNOx availability. The bottom plots in Fig. 9 generally show increases in SO4 with the Lu21 scheme throughout the troposphere between 40° S–40° N and areas of decrease below 3 km beyond 40° N. There is an amplification of this behaviour when looking at the differences between the Lu21 case and the no-LNOx case. The overall increase in SO4 with the Lu21 scheme is possibly due to an increase in tropospheric oxidants in response to an increase in LNOx (for example, there is a 13 % increase in OH with the Lu21 scheme compared to the PR92 scheme with nitrate included).
4.4 Impact of LNOx on aerosol number concentration
In addition to the aerosol mass concentrations above, we can also examine zonal differences in prognostic aerosol number concentrations. Figure 10 presents the modelled zonal-mean tropospheric aerosol number concentrations from the Lu21 simulation with nitrate (left), the difference between the Lu21 and PR92 simulations (Lu21 − PR92) (middle), and the difference between the Lu21 and no-LNOx simulations (Lu21 − no-LNOx) (right).
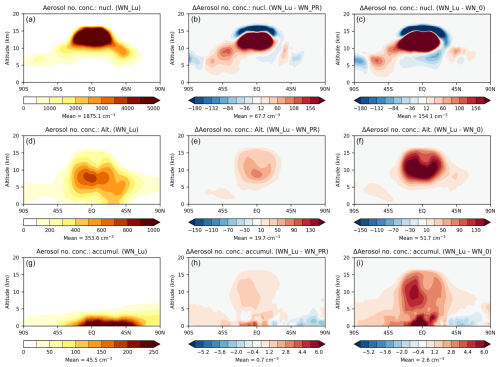
Figure 10Zonal-mean tropospheric nucleation-mode, Aitken-mode, and accumulation-mode (all soluble) aerosol number concentrations from the Lu21 simulation with nitrate (a, d, g), the difference between the Lu21 and PR92 simulations (Lu21 − PR92) (b, e, h), and the difference between the Lu21 and no-LNOx simulations (Lu21 − no-LNOx) (c, f, i).
In Fig. 10 (left), the nucleation-mode (soluble) particles are the highest in number and are mostly located in the upper troposphere within 40° S–40° N, followed by the Aitken-mode (soluble) particles with a maximum in the mid-troposphere around the Equator, and the accumulation-mode (soluble) particles in the lower troposphere between 30° S–70° N. The coarse-mode (soluble) particles (plot not shown) are the fewest in number and are confined to very close to the surface due to their effective gravitational sedimentation, with more particles in the Southern Hemisphere than in the Northern Hemisphere (probably due to a larger oceanic surface in the SH so as to cause a large sea-salt particle number concentration).
The difference plots in Fig. 10 (middle) show that the Lu21 scheme with its higher amount of LNOx leads to a greater number concentration of nucleation-mode particles (overall by about 3.6 %) compared to the PR92 scheme, although there are regions, particularly near the tropopause between 30° S–30° N, where the particle number concentration decreases with the Lu21 scheme. Both simulations include nitrate aerosol, but in the present scheme aerosol number concentrations are not modified explicitly by nitrate chemistry because of the assumption that NH4 and NO3 condense onto (and evaporate from) existing atmospheric aerosol rather than nucleating new particles. Therefore, the changes in the number of nucleation-mode particles in Fig. 10 (middle) are likely due to an indirect impact of LNOx changes on the oxidising capacity of the atmosphere (for example, via changes in OH and O3), increases in which as LNOx is increased would generally lead to enhanced formation of new particles owing to faster oxidation rates of gas-phase sulfur to sulfate conversion. (Enhancement of nucleation-mode particle numbers with lightning emissions in the upper troposphere was also predicted in the model simulations by Tost, 2017, which included nitrate.)
In Fig. 10 (middle), there is an overall increase of 5.6 % in the number of particles in the Aitken mode in the troposphere with the Lu21 scheme, and this increase is centred in the middle of the troposphere (∼ 8 km) within 30° S–30° N. The particle number in the accumulation mode (Fig. 10, bottom) is confined to the lower troposphere and is impacted much less (∼ 1.5 %) by the change in the LNOx scheme. There are negligible changes in the coarse-mode and Aitken-mode (insoluble) particle number concentrations with the changes in LNOx amounts considered (plots not shown).
The differences in the particle numbers between the Lu21 scheme and the no-LNOx case shown in Fig. 10 (right) essentially show bigger increases but with qualitatively similar patterns – the increases in particle number in the nucleation, Aitken, and accumulation modes are 8.2 %, 14.6 %, and 5.7 %, respectively.
Thus, it is apparent from Figs. 9 and 10 that with the Lu21 scheme, with its 54 % larger total LNOx compared to the PR92 scheme, there is a rise in the aerosol mass concentration (of NH4, fine NO3, coarseNO3, and SO4) in the lower troposphere, whereas there is an increase in the total aerosol number concentration, particularly in the nucleation and Aitken modes, in the middle to upper troposphere. The magnitudes of these increases are amplified when comparing the results from the Lu21 scheme with the no-LNOx case.
Wang et al. (2021) observed formation of new ultrafine particles during lightning events, resulting in large increases in nucleation- and Aitken-mode aerosols (by 18.9 and 5.6 times, respectively) coupled with ∼ 12 % intensification of nitrate aerosol signals (but it is not clear from their data if there were any nitrate signals in the nucleation mode). These increases are qualitatively consistent with the modelled differences with and without lightning shown in Fig. 10. In our model, nitrate does not participate in nucleation and the increases in particle numbers stem from LNOx enhancing the oxidising capacity of the troposphere and thus sulfate aerosol formation. Wang et al. (2021) point out that lightning channels may be conducive to an ion-induced nucleation process to generate new particles. Also, there is some evidence (e.g. Wang et al., 2020) that nitrate participates in nucleation directly. Note that any such direct new particle formation due to lightning or participation of nitrate in nucleation is not included in our model but may be considered in future versions of the UM as new observations become available.
It is also instructive to present particle number concentrations without and with nitrate. In Fig. 10, we already presented the modelled zonal-mean tropospheric aerosol number concentrations from the Lu21 simulation with the nitrate scheme. Figure 11 presents the difference between this simulation and the same simulation without the nitrate scheme. There are regions of both increased and decreased nucleation-mode (soluble) particle number concentrations from the middle to upper troposphere with nitrate on, likely as a result of changes in oxidation rates of gas-phase sulfur to sulfate conversion. But overall, there is a very small decrease of ∼ 1 % in nucleation-mode particle numbers with nitrate on. With nitrate on, there is a reduction in the Aitken-mode particle number density (by 6.4 % overall) throughout the troposphere, particularly between 10–50° N with higher concentrations in the lower troposphere. The probable reason for this is that condensational growth and altered coagulation due to the additional nitrate mass move these particles to the accumulation mode. This is evident from the particle number distribution for the accumulation mode, where there is a 10 % enhancement in the particle number concentration with nitrate (mostly confined to the lower troposphere between 10° S–50° N). Of all the modes, aerosols in the accumulation mode are of most importance as they have the greatest climate impact. Not only do they have the highest scattering efficiency, but they also have the longest atmospheric lifetime. They constitute the largest source of CCN.
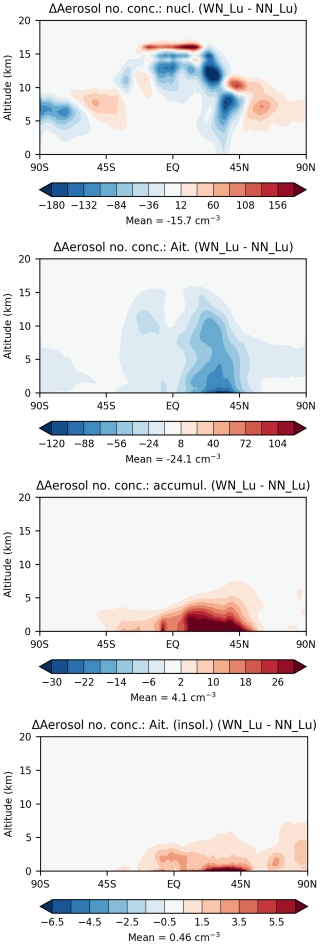
Figure 11Difference between the zonal-mean tropospheric aerosol number concentrations in various aerosol modes from the Lu21 simulation with and without the nitrate scheme.
The coarse-mode aerosol number concentrations are much smaller (global tropospheric average = 0.16 cm−3) and are confined to near the surface, and the inclusion of nitrate leads to an increase of 7.1 % over the no-nitrate value.
We also looked at how tropospheric sulfate is impacted by the inclusion of nitrate, and this is presented in the Supplement.
4.5 Impact of LNOx on aerosol optical depth (AOD), TOA radiation, and CDNC
As demonstrated earlier, significant differences in the size distribution of aerosol in the troposphere are caused by lightning. In conjunction with the inclusion of nitrate, this has ramifications for the aerosol extinction and cloud properties (Tost, 2017). In Fig. 12 (left), we present the modelled column aerosol optical depth (AOD550 at 550 nm wavelength) from the Lu21 simulation with nitrate. Large AODs over central Africa (biomass burning region), north-west Africa (dust emission region), and China and India (anthropogenic pollution emissions) are evident. These patterns are consistent with the 2003–2012 mean MODIS (Collection 6) satellite AOD550 data with a globally averaged value of 0.162 (see Jones et al., 2021), which can be compared with the present model estimate of 0.155. The differences between the Lu21 and PR92 simulations (Lu21 − PR92) (Fig. 12, middle) indicate that there is a very small (0.5 %) overall increase in the global AOD550 with the Lu21 scheme, but it is apparent there are larger differences regionally, for example an increase of 0.01–0.02 over north-western parts of Africa, western Europe, and the central Atlantic and a decrease over India. The differences in AOD550 between the Lu21 scheme and the no-LNOx case (both with nitrate) shown in Fig. 12 (right) demonstrate, as expected, bigger increases (∼ 1 %).
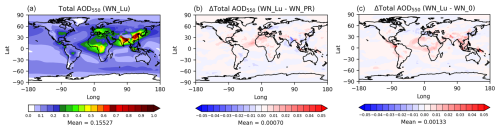
Figure 12Annual-mean aerosol optical depth (AOD550) from the Lu21 simulation with nitrate (a), the difference between the Lu21 and PR92 simulations (Lu21 − PR92) (b), and the difference between the Lu21 and no-LNOx simulations (Lu21 − no-LNOx) (c).
In Fig. 13, globally averaged AOD550 plotted as a function of LNOx for all simulations suggests that within the range of LNOx considered, on average, AOD550 is greater by ∼ 0.015 (about 11 %) when nitrate is included (this difference is almost thrice as large as that obtained by Jones et al., 2021). Based on the slopes of the linear least-squares fits, the increase in AOD550 as a result of a 1 Tg N yr−1 increase in LNOx is with nitrate and without nitrate. The 1σ standard deviation uncertainty bars (calculated from the annual means over 15 years of simulation) in Fig. 13 suggest that the mean increase in AOD550 with LNOx within the range of LNOx considered is ∼0.0015, which is comparable to or within the average AOD550 uncertainty of .
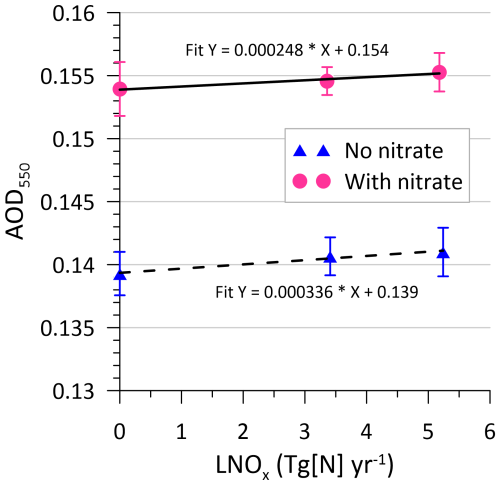
Figure 13Modelled globally averaged AOD550 as a function of lightning-generated NOx. The lines are linear least-squares fits. The error bars correspond to a 1σ standard deviation.
Lightning influences atmospheric radiation via changes in O3 levels and methane lifetime and through direct and indirect aerosol effects (e.g. Tost, 2017; Luhar et al., 2022). Figure 14 presents the modelled present-day all-sky annual-mean TOA net downward radiative flux (), incorporating both shortwave and longwave components, as a function of LNOx for both with- and without-nitrate cases. In the present model simulation, perturbation in this quality is akin to the total effective radiative forcing (ERF) (Bellouin et al., 2020). Although these results are based on only three model runs, the slopes of the linear best-fit lines in Fig. 14 indicate that there is a rise of 36 mW m−2 in with a per-teragram increase in N generated per year by lightning when nitrate is included in the model, and the value is 40 mW m−2 without nitrate. (The latter is almost the same as the 39.6 mW m−2 (Tg N yr obtained by Luhar et al. (2022) using ACCESS–UKCA (an older version (vn8.4) of UM–UKCA with somewhat different emission and model settings) without nitrate.) A decrease or negative change in signifies reduced atmospheric radiation absorption, indicating cooling conditions. In Fig. 14, increases with LNOx, which suggests that the positive radiative feedback from ozone increases outweighs the negative feedback from the reduction in the methane lifetime and rise in aerosol concentrations as LNOx increases. This holds true regardless of whether nitrate is included. However, the inclusion of nitrate results in a change of approximately −0.4 W m2 in for any given LNOx level (Fig. 14), indicating that the incorporation of nitrate in the model has a far greater impact on than the change in LNOx considered here. Note that the difference of −0.4 W m−2 is nearly twice as large as that obtained by Jones et al. (2021), and this is likely due to the various updates to the UM that have happened moving from science configurations GA7.1 and GL7.0 to GA8.0 and GL9.0 (from UM vn11.8 to vn13.2), which include changes pertinent to aerosols, such as tuning of the oceanic dimethyl sulfide emissions and cloud droplet spectral dispersion parameterisation, as well as near-surface drag improvements (Jones et al., 2022). As a comparison, in a regional model simulation study, Drugé et al. (2019) found that ammonium and nitrate aerosol caused a TOA direct radiative forcing of about −1.4 W m−2 under all-sky conditions over Europe for the period 1979–2016. The uncertainty bars in Fig. 14 indicate that the increase in with LNOx within the range of LNOx considered is ∼ 0.20 W m−2, which can be compared to the average uncertainty of W m−2.
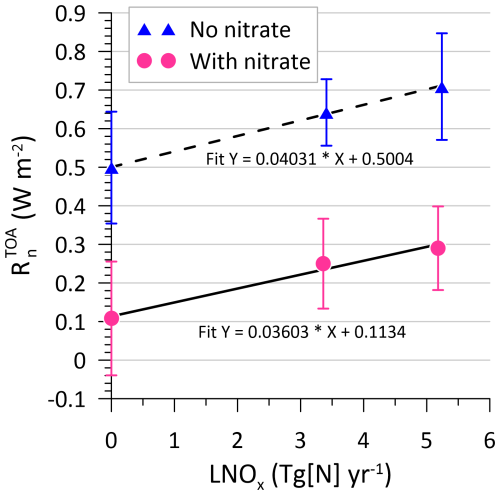
Figure 14Modelled annual-mean top-of-atmosphere (TOA) net downward radiative flux, as a function of lightning-generated NOx. The lines are linear least-squares fits. The error bars correspond to a 1σ standard deviation.
It is clear that there are greater changes when nitrate is considered compared to the effects of changes in the LNOx scheme.
We also examined the modelled tropospheric cloud droplet number concentration (CDNC) (one could also look at CCN or condensation nuclei (CN), but such output was not available from the model runs made). CDNC can be used as a proxy for CCN (the latter is a measure of the potential to form cloud droplets at the bottom of the cloud). A typical cloud droplet is 20 µm in diameter. Apart from modifying the aerosol size distribution, nitrate also modifies the chemical composition of the aerosol, which can change the CCN efficiency of the particles with ramifications for the indirect aerosol effects (Tost, 2017). The incorporation of nitrate in the model causes an average increase of ∼ 3.5 % in CDNC for any given LNOx value (Fig. 15). The average increase in CDNC per teragram of N per year of LNOx is 0.035 cm−3. Compared to the no-LNOx case, there is a ∼ 3 % increase in the mean tropospheric CDNC when LNOx is considered (via the Lu21 scheme) (see the Supplement, Sect. S2, for zonal CDNC distributions).
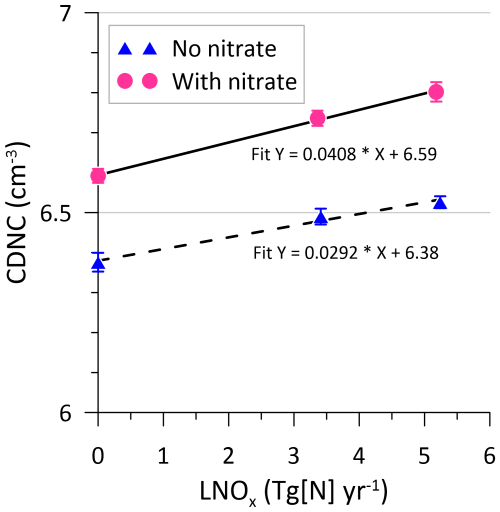
Figure 15Modelled annual-mean tropospheric cloud droplet number concentration (CDNC), as a function of lightning-generated NOx. The lines are linear least-squares fits. The error bars correspond to a 1σ standard deviation.
In this work, more complex flash-rate schemes could also be tried, for example the upward cloud-ice-flux-based method of Finney et al. (2016), which is shown to perform better in the subtropics and part of the mid-latitudes compared to the cloud-top-height-based schemes used here. However, we believe that the results on globally averaged atmospheric composition changes with LNOx obtained here are not likely to change, but there could be regional impacts.
In this sensitivity-focused study, we have essentially addressed two problems through the use of a global chemistry–climate model, UM-GA8.0-UKCA: (1) quantifying the impact of including nitrate aerosol on global mean quantities such as tropospheric composition, and (2) evaluating the dependency of these effects on lightning-generated NOx. The latter was explored by considering two empirical lightning-flash-rate parameterisations: the PR92 scheme (Price and Rind, 1992) and the Lu21 scheme (Luhar et al., 2021), with the Lu21 scheme improving upon the underestimation of the flash rate by the PR92 scheme over the ocean. In addition to the changes in the various global mean quantities without and with nitrate, changes per teragram of N per year in LNOx were also presented.
The amount of global LNOx obtained from the Lu21 scheme was about 50 % higher than that from the PR92 scheme (5.2 vs. 3.4 Tg N yr−1). This variation in LNOx, together with the simulations with zero LNOx, enabled an investigation of the change in various globally averaged modelled quantities as a function of LNOx. We found that both nitrate aerosol and changes in LNOx lead to significant changes in tropospheric composition and aerosol responses. We also found that the difference between values of the various global mean quantities obtained without and with nitrate is almost constant regardless of the value of the total global LNOx emission used.
With the inclusion of nitrate aerosol, there was a decrease (of ∼ 4 %–5 %) in the mean tropospheric O3 level, with the biggest reductions located in the middle to upper troposphere in the Northern Hemisphere. The methane lifetime increased by approximately 5 % (∼ 0.4 years) as the mean tropospheric OH concentration decreased by a similar percentage. There were reductions in NH3, HNO3, the gaseous nitrate radical, and N2O5 mixing ratios. Aerosol size distribution also changed when nitrate aerosol is included. We found that there was a very small decrease of ∼ 1 % in the aerosol number concentration in the nucleation mode, a reduction of ∼ 6.4 % in the Aitken mode, and an enhancement of ∼ 10 % in the accumulation mode. Of all the modes, aerosols in the accumulation mode are of most importance in terms of climate impact; thus this change is significant. The mean AOD550 increased by 11 % and the mean tropospheric CDNC by 3.5 %, and the change in was ∼ −0.4 W m−2. These results build on those presented in Jones et al. (2021) by determining the impact of nitrate aerosol on tropospheric composition, and they will be useful, for example, for the development of the UK Earth System Model version 2 (UKESM2).
Comparing simulations with and without LNOx emissions (corresponding to an LNOx difference of 5.2 Tg N yr−1), we show that the impact of LNOx on global-mean tropospheric aerosol composition is an increase of 2.8 % in NH4, 4.7 % in fine NO3, 12 % in coarseNO3, and 5.8 % in SO4 by mass and a small 1 % increase in AOD550 and an increase of 3 % in CDNC.
Moving from the PR92 to the Lu21 scheme (both with nitrate included), there was a very small global mean increase (∼ 1 %) in both NH4 and fine-NO3 aerosol mass burdens. On the other hand, the increase in the coarseNO3 was greater (∼ 4 %). This change in the LNOx scheme also increased the tropospheric SO4 aerosol burden by ∼ 1.7 %. These aerosol changes could be ascribed to particular aerosol modes, and they had considerable regional variations. These increases were dominated by the aerosol mass concentration in the lower troposphere. There was an increase of ∼ 3.6 % in the aerosol number concentration in the nucleation mode and an increase of ∼ 5.6 % in the Aitken mode, and these increases were dominated by increases in the middle to upper troposphere. A small decrease of ∼ 1.5 % was estimated in the accumulation mode with negligible changes in the other modes. Compared to the PR92 scheme, the Lu21 scheme yielded a very small (0.5 %) overall increase in the global AOD550. The mean increased by ∼ 0.07 W m−2, which suggests that the positive radiative feedback from an increase in ozone dominates over negative radiative feedback resulting from a reduction in the methane lifetime and an increase in aerosol concentration as LNOx is increased in the Lu21 scheme. In general, we find that the magnitude of changes in gas-phase tropospheric composition as a result of changes in LNOx going from the PR92 to the Lu21 scheme is roughly comparable to that caused by the inclusion of nitrate aerosol. But the magnitudes of changes in AOD550, , and CDNC are dominated by the inclusion of nitrate.
We assumed a single value for the HNO3 uptake coefficient (γ) to produce NH4NO3, corresponding to the FAST value (0.193) used in Jones et al. (2021), which likely represents an upper limit on nitrate effects. Given uncertainties over the composition, relative-humidity, and temperature dependence of the HNO3 uptake coefficient, it is not yet possible to perform a more comprehensive study in which the uptake rate is a dependent on these variables. Any direct new particle formation (due to lightning or otherwise) is not yet explicitly included in the nitrate scheme in UM–UKCA, which could also be important – this may be considered in future versions of the UM. The results obtained here on the degree of sensitivity to nitrate aerosol and LNOx will be useful for further LNOx and nitrate impact assessment in future UM studies. Nitrate concentrations are sensitive to precursor emissions, and we used constant emissions forcings representative of the year 2000 following CMIP6 protocol. A more comprehensive study could include transient emissions to investigate recent trends in ammonium nitrate concentrations.
In this paper, we have shown that simulating nitrate in global climate models is important for tropospheric composition, alongside radiation and cloud droplet activation. We have also shown that simulating LNOx production over the ocean (as in Lu21) produces a tangible impact on regional aerosol concentrations at the surface, though the largest impacts are at higher altitudes where LNOx and NH3 coexist. Our results could be used to infer the impact of changing lightning rates (and LNOx emissions) on nitrate concentrations under climate change.
Owing to intellectual property right restrictions, we cannot provide the source code or documentation papers for the UM. The Met Office UM is available for use under licence. A number of research organisations and national meteorological services use the UM in collaboration with the Met Office to undertake basic atmospheric process research, produce forecasts, and develop the UM code. To apply for a licence, see http://www.metoffice.gov.uk/research/modelling-systems/unified-model (Met Office, 2024a). Geospatial figures were produced using Python 3.10.8 (https://www.python.org, Python, 2024) and Iris 2.4.0 (https://scitools.org.uk, Met Office, 2024b).
Output from the model suites used here is available on MASS tape archive and can be accessed upon request by obtaining a log-in to the UK's environmental science data analysis facility at http://www.jasmin.ac.uk (Jasmin, 2024). The model suites used UM vn13.2, and the suite IDs corresponding to the simulation names NN_0, NN_PR, NN_Lu, WN_0, WN_PR, and WN_Lu used in this paper are u-cx746, u-cx815, u-cx814, u-cx745, u-cx817, and u-cx819, respectively. The LIS/OTD lightning flash data (V2.3.2015) are available from https://lightning.nsstc.nasa.gov/data/data_lis-otd-climatology.html (NASA GHRC, 2024).
The supplement related to this article is available online at: https://doi.org/10.5194/acp-24-14005-2024-supplement.
AKL designed the study, set up the modelling experiments, developed analysis scripts, analysed model output, and prepared the paper with assistance from all co-authors. ACJ contributed to setting up the modelling experiments, advised on aerosol in the model, oversaw the progress of simulations, and assisted with the development of analysis codes. JMW assisted with and carried out part of the modelling work pertaining to lightning schemes.
The contact author has declared that none of the authors has any competing interests.
Publisher’s note: Copernicus Publications remains neutral with regard to jurisdictional claims made in the text, published maps, institutional affiliations, or any other geographical representation in this paper. While Copernicus Publications makes every effort to include appropriate place names, the final responsibility lies with the authors.
Ashok Luhar acknowledges a travel award from the UM Partner Visiting Scientist Exchange (UNITE) programme to visit the Met Office, Exeter, in June–July 2023 and thanks the UNITE programme manager Joao Teixeira for the assistance provided. We acknowledge Luke Roberts of the Met Office for his assistance with setting up the computational environment on JASMIN (UK's storage and compute facility for environmental science data analysis) to carry out model output analysis and Martin Cope of CSIRO for his helpful comments on this work. We thank the editor and two anonymous reviewers for their useful comments.
This research has been supported by the CSIRO-UM Momentum Partnership Agreement project with the Met Office (CSIRO projects OD-207701, OD-237006).
This paper was edited by Kostas Tsigaridis and reviewed by two anonymous referees.
Archer-Nicholls, S., Allen, R., Abraham, N. L., Griffiths, P. T., and Archibald, A. T.: Large simulated future changes in the nitrate radical under the CMIP6 SSP scenarios: implications for oxidation chemistry, Atmos. Chem. Phys., 23, 5801–5813, https://doi.org/10.5194/acp-23-5801-2023, 2023.
Archibald, A. T., O'Connor, F. M., Abraham, N. L., Archer-Nicholls, S., Chipperfield, M. P., Dalvi, M., Folberth, G. A., Dennison, F., Dhomse, S. S., Griffiths, P. T., Hardacre, C., Hewitt, A. J., Hill, R. S., Johnson, C. E., Keeble, J., Köhler, M. O., Morgenstern, O., Mulcahy, J. P., Ordóñez, C., Pope, R. J., Rumbold, S. T., Russo, M. R., Savage, N. H., Sellar, A., Stringer, M., Turnock, S. T., Wild, O., and Zeng, G.: Description and evaluation of the UKCA stratosphere–troposphere chemistry scheme (StratTrop vn 1.0) implemented in UKESM1, Geosci. Model Dev., 13, 1223–1266, https://doi.org/10.5194/gmd-13-1223-2020, 2020.
Bauer, S. E., Koch, D., Unger, N., Metzger, S. M., Shindell, D. T., and Streets, D. G.: Nitrate aerosols today and in 2030: a global simulation including aerosols and tropospheric ozone, Atmos. Chem. Phys., 7, 5043–5059, https://doi.org/10.5194/acp-7-5043-2007, 2007.
Bellouin, N., Rae, J., Jones, A., Johnson, C., Haywood, J., and Boucher, O.: Aerosol forcing in the Climate Model Intercomparison Project (CMIP5) simulations by HadGEM2-ES and the role of ammonium nitrate, J. Geophys. Res.-Atmos., 116, D20206, https://doi.org/10.1029/2011jd016074, 2011.
Bellouin, N., Mann, G. W., Woodhouse, M. T., Johnson, C., Carslaw, K. S., and Dalvi, M.: Impact of the modal aerosol scheme GLOMAP-mode on aerosol forcing in the Hadley Centre Global Environmental Model, Atmos. Chem. Phys., 13, 3027–3044, https://doi.org/10.5194/acp-13-3027-2013, 2013.
Bellouin, N., Quaas, J., Gryspeerdt, E., Kinne, S., Stier, P., Watson-Parris, D., Boucher, O., Carslaw, K. S., Christensen, M., Daniau, A.-L., Dufresne, J.-L., Feingold, G., Fiedler, S., Forster, P., Gettelman, A., Haywood, J. M., Lohmann, U., Malavelle, F., Mauritsen, T., McCoy, D. T., Myhre, G., Mülmenstädt, J., Neubauer, D., Possner, A., Rugenstein, M., Sato, Y., Schulz, M., Schwartz, S. E., Sourdeval, O., Storelvmo, T., Toll, V., Winker, D., and Stevens, B.: Bounding global aerosol radiative forcing of climate change, Rev. Geophys., 58, e2019RG000660, https://doi.org/10.1029/2019RG000660, 2020.
Bian, H., Chin, M., Hauglustaine, D. A., Schulz, M., Myhre, G., Bauer, S. E., Lund, M. T., Karydis, V. A., Kucsera, T. L., Pan, X., Pozzer, A., Skeie, R. B., Steenrod, S. D., Sudo, K., Tsigaridis, K., Tsimpidi, A. P., and Tsyro, S. G.: Investigation of global particulate nitrate from the AeroCom phase III experiment, Atmos. Chem. Phys., 17, 12911–12940, https://doi.org/10.5194/acp-17-12911-2017, 2017.
Boccippio, D. J.: Lightning scaling relations revisited, J. Atmos. Sci., 59, 1086–1104, https://doi.org/10.1175/1520-0469(2002)059<1086:LSRR>2.0.CO;2, 2002.
Brown, S. S., Ryerson, T. B., Wollny, A. G., Brock, C. A., Peltier, R., Sullivan, A. P., Weber, R. J., Dube, W. P., Trainer, M., Meagher, J. F., Fehsenfeld, F. C., and Ravishankara, A. R.: Variability in nocturnal nitrogen oxide processing and its role in regional air quality, Science, 311, 67–70, https://doi.org/10.1126/science.1120120, 2006.
Bucsela, E., Pickering, K. E., Allen, D., Holzworth, R., and Krotkov, N.: Midlatitude lightning NOx production efficiency inferred from OMI and WWLLN data. J. Geophys. Res.-Atmos., 124, 13475–13497, https://doi.org/10.1029/2019JD030561, 2019.
Cecil, D. J., Buechler, D. E., and Blakeslee, R. J.: Gridded lightning climatology from TRMM-LIS and OTD: Dataset description, Atmos. Res., 135–136, 404–414, https://doi.org/10.1016/j.atmosres.2012.06.028, 2014.
Dahlmann, K., Grewe, V., Ponater, M., and Matthes, S.: Quantifying the contributions of individual NOx sources to the trend in ozone radiative forcing, Atmos. Environ., 45, 2860–2868, https://doi.org/10.1016/j.atmosenv.2011.02.071, 2011.
Drugé, T., Nabat, P., Mallet, M., and Somot, S.: Model simulation of ammonium and nitrate aerosols distribution in the Euro-Mediterranean region and their radiative and climatic effects over 1979–2016, Atmos. Chem. Phys., 19, 3707–3731, https://doi.org/10.5194/acp-19-3707-2019, 2019.
Fairlie, T. D., Jacob, D. J., Dibb, J. E., Alexander, B., Avery, M. A., van Donkelaar, A., and Zhang, L.: Impact of mineral dust on nitrate, sulfate, and ozone in transpacific Asian pollution plumes, Atmos. Chem. Phys., 10, 3999–4012, https://doi.org/10.5194/acp-10-3999-2010, 2010.
Finlayson-Pitts, B. J. and Pitts, J. N.: Chemistry of the Upper and Lower Atmosphere: Theory, Experiments and Applications, Academic Press, San Diego, ISBN 0-12-257060-x, 969 pp., 2000.
Finney, D. L., Doherty, R. M., Wild, O., and Abraham, N. L.: The impact of lightning on tropospheric ozone chemistry using a new global lightning parametrisation, Atmos. Chem. Phys., 16, 7507–7522, https://doi.org/10.5194/acp-16-7507-2016, 2016.
Fuchs, N. A. and Sutugin, A. G.: Highly Dispersed Aerosols, Butterworth-Heinemann, Newton, Mass., USA, 105 pp., https://doi.org/10.1016/B978-0-08-016674-2.50006-6, 1970.
Gordillo-Vázquez, F. J., Pérez-Invernón, F. J., Huntrieser, H., and Smith, A. K.: Comparison of six lightning parameterizations in CAM5 and the impact on global atmospheric chemistry, Earth Space Sci., 6, 2317–2346, https://doi.org/10.1029/2019EA000873, 2019.
Gregory, D. and Rowntree, P. R.: A mass flux convection scheme with representation of cloud ensemble characteristics and stability-dependent closure, Mon. Weather Rev., 118, 1483–1506, https://doi.org/10.1175/1520-0493(1990)118<1483:AMFCSW>2.0.CO;2, 1990.
Gressent, A., Sauvage, B., Cariolle, D., Evans, M., Leriche, M., Mari, C., and Thouret, V.: Modeling lightning-NOx chemistry on a sub-grid scale in a global chemical transport model, Atmos. Chem. Phys., 16, 5867–5889, https://doi.org/10.5194/acp-16-5867-2016, 2016.
Griffiths, P. T., Murray, L. T., Zeng, G., Shin, Y. M., Abraham, N. L., Archibald, A. T., Deushi, M., Emmons, L. K., Galbally, I. E., Hassler, B., Horowitz, L. W., Keeble, J., Liu, J., Moeini, O., Naik, V., O'Connor, F. M., Oshima, N., Tarasick, D., Tilmes, S., Turnock, S. T., Wild, O., Young, P. J., and Zanis, P.: Tropospheric ozone in CMIP6 simulations, Atmos. Chem. Phys., 21, 4187–4218, https://doi.org/10.5194/acp-21-4187-2021, 2021.
Hauglustaine, D. A., Balkanski, Y., and Schulz, M.: A global model simulation of present and future nitrate aerosols and their direct radiative forcing of climate, Atmos. Chem. Phys., 14, 11031–11063, https://doi.org/10.5194/acp-14-11031-2014, 2014.
Haywood, J. and Boucher, O.: Estimates of the direct and indirect radiative forcing due to tropospheric aerosols: A review, Rev. Geophys., 38, 513–543, https://doi.org/10.1029/1999RG000078, 2000.
Hoerling, M. P., Schaack, T. K., and Lenzen, A. J.: A global analysis of stratosphere-tropospheric exchange during northern winter, Mon. Weather Rev., 121, 162–172, https://doi.org/10.1175/1520-0493(1993)121<0162:AGAOSE>2.0.CO;2, 1993.
JASMIN: JASMIN, the UK's data analysis facility for environmental science,, http://www.jasmin.ac.uk (last access: 14 December 2024), 2024.
Jones, A. C., Hill, A., Remy, S., Abraham, N. L., Dalvi, M., Hardacre, C., Hewitt, A. J., Johnson, B., Mulcahy, J. P., and Turnock, S. T.: Exploring the sensitivity of atmospheric nitrate concentrations to nitric acid uptake rate using the Met Office's Unified Model, Atmos. Chem. Phys., 21, 15901–15927, https://doi.org/10.5194/acp-21-15901-2021, 2021.
Jones, A. C., Hill, A., Hemmings, J., Lemaitre, P., Quérel, A., Ryder, C. L., and Woodward, S.: Below-cloud scavenging of aerosol by rain: a review of numerical modelling approaches and sensitivity simulations with mineral dust in the Met Office's Unified Model, Atmos. Chem. Phys., 22, 11381–11407, https://doi.org/10.5194/acp-22-11381-2022, 2022.
Khan, M. A. H., Cooke, M. C., Utembe, S. R., Archibald, A. T., Derwent, R. G., Xiao, P., Percival, C. J., Jenkin, M. E., Morris, W. C., and Shallcross, D. E.: Global modeling of the nitrate radical (NO3) for present and pre-industrial scenarios, Atmos. Res., 164–165, 347–357, https://doi.org/10.1016/j.atmosres.2015.06.006, 2015.
Labrador, L. J., von Kuhlmann, R., and Lawrence, M. G.: The effects of lightning-produced NOx and its vertical distribution on atmospheric chemistry: sensitivity simulations with MATCH-MPIC, Atmos. Chem. Phys., 5, 1815–1834, https://doi.org/10.5194/acp-5-1815-2005, 2005.
Li, W. J. and Shao, L. Y.: Observation of nitrate coatings on atmospheric mineral dust particles, Atmos. Chem. Phys., 9, 1863–1871, https://doi.org/10.5194/acp-9-1863-2009, 2009.
Luhar, A. K., Woodhouse, M. T., and Galbally, I. E.: A revised global ozone dry deposition estimate based on a new two-layer parameterisation for air–sea exchange and the multi-year MACC composition reanalysis, Atmos. Chem. Phys., 18, 4329–4348, https://doi.org/10.5194/acp-18-4329-2018, 2018.
Luhar, A. K., Galbally, I. E., Woodhouse, M. T., and Abraham, N. L.: Assessing and improving cloud-height-based parameterisations of global lightning flash rate, and their impact on lightning-produced NOx and tropospheric composition in a chemistry–climate model, Atmos. Chem. Phys., 21, 7053–7082, https://doi.org/10.5194/acp-21-7053-2021, 2021.
Luhar, A. K., Galbally, I. E., and Woodhouse, M. T.: Radiative impact of improved global parameterisations of oceanic dry deposition of ozone and lightning-generated NOx, Atmos. Chem. Phys., 22, 13013–13033, https://doi.org/10.5194/acp-22-13013-2022, 2022.
Mann, G. W., Carslaw, K. S., Spracklen, D. V., Ridley, D. A., Manktelow, P. T., Chipperfield, M. P., Pickering, S. J., and Johnson, C. E.: Description and evaluation of GLOMAP-mode: a modal global aerosol microphysics model for the UKCA composition-climate model, Geosci. Model Dev., 3, 519–551, https://doi.org/10.5194/gmd-3-519-2010, 2010.
Manners, J., Edwards, J. M., Hill, P., and Thelen, J.-C.: SOCRATES Technical Guide Suite Of Community RAdiative Transfer codes based on Edwards and Slingo, Met Office, UK, available at: https://code.metoffice.gov.uk/trac/socrates (last access: 26 February 2024), 2023.
Marais, E. A., Jacob, D. J., Choi, S., Joiner, J., Belmonte-Rivas, M., Cohen, R. C., Beirle, S., Murray, L. T., Schiferl, L. D., Shah, V., and Jaeglé, L.: Nitrogen oxides in the global upper troposphere: interpreting cloud-sliced NO2 observations from the OMI satellite instrument, Atmos. Chem. Phys., 18, 17017–17027, https://doi.org/10.5194/acp-18-17017-2018, 2018.
Martin, R. V., Sauvage, B., Folkins, I., Sioris, C. E., Boone, C., Bernath, P., and Ziemke, J.: Space-based constraints on the production of nitric oxide by lightning, J. Geophys. Res., 112, D09309, https://doi.org/10.1029/2006JD007831, 2007.
Mertens, L. A., Winiberg, F. A. F., Allen, H. M., Sander, S. P., and Okumura, M.: Yields of HONO2 and HOONO products from the reaction of HO2 and NO using pulsed laser photolysis and mid-infrared cavity-ringdown spectroscopy, J. Phys. Chem. A, 126, 7342, https://doi.org/10.1021/acs.jpca.2c04643, 2022.
Met Office: Unified Model, http://www.metoffice.gov.uk/research/modelling-systems/unified-model (last access: 14 December 2024), 2024a.
Met Office: SciTools [software], https://scitools.org.uk (last access: 27 February 2024), 2024b.
Miyazaki, K., Eskes, H. J., Sudo, K., and Zhang, C.: Global lightning NOx production estimated by an assimilation of multiple satellite data sets, Atmos. Chem. Phys., 14, 3277–3305, https://doi.org/10.5194/acp-14-3277-2014, 2014.
Mulcahy, J. P., Johnson, C., Jones, C. G., Povey, A. C., Scott, C. E., Sellar, A., Turnock, S. T., Woodhouse, M. T., Abraham, N. L., Andrews, M. B., Bellouin, N., Browse, J., Carslaw, K. S., Dalvi, M., Folberth, G. A., Glover, M., Grosvenor, D. P., Hardacre, C., Hill, R., Johnson, B., Jones, A., Kipling, Z., Mann, G., Mollard, J., O'Connor, F. M., Palmiéri, J., Reddington, C., Rumbold, S. T., Richardson, M., Schutgens, N. A. J., Stier, P., Stringer, M., Tang, Y., Walton, J., Woodward, S., and Yool, A.: Description and evaluation of aerosol in UKESM1 and HadGEM3-GC3.1 CMIP6 historical simulations, Geosci. Model Dev., 13, 6383–6423, https://doi.org/10.5194/gmd-13-6383-2020, 2020.
Murray, L. T.: Lightning NOx and impacts on air quality, Current Pollution Reports, 2, 115–133, https://doi.org/10.1007/s40726-016-0031-7, 2016.
Naik, V., Voulgarakis, A., Fiore, A. M., Horowitz, L. W., Lamarque, J.-F., Lin, M., Prather, M. J., Young, P. J., Bergmann, D., Cameron-Smith, P. J., Cionni, I., Collins, W. J., Dalsøren, S. B., Doherty, R., Eyring, V., Faluvegi, G., Folberth, G. A., Josse, B., Lee, Y. H., MacKenzie, I. A., Nagashima, T., van Noije, T. P. C., Plummer, D. A., Righi, M., Rumbold, S. T., Skeie, R., Shindell, D. T., Stevenson, D. S., Strode, S., Sudo, K., Szopa, S., and Zeng, G.: Preindustrial to present-day changes in tropospheric hydroxyl radical and methane lifetime from the Atmospheric Chemistry and Climate Model Intercomparison Project (ACCMIP), Atmos. Chem. Phys., 13, 5277–5298, https://doi.org/10.5194/acp-13-5277-2013, 2013.
NASA GHRC: Lightning & Atmospheric Electricity Research, https://lightning.nsstc.nasa.gov/data/data_lis-otd-climatology.html (last access: 14 December 2024), 2024
Nault, B. A., Laughner, J. L.,Wooldridge, P. J., Crounse, J. D., Dibb, J., Diskin, G., Peischl, J., Podolske, J. R., Pollack, I. B., Ryerson, T. B., Scheuer, E., Wennberg, P. O., and Cohen, R. C.: Lightning NOx emissions: reconciling measured and modeled estimates with updated NOx Chemistry, Geophys. Res. Lett., 44, 9479–9488, https://doi.org/10.1002/2017GL074436, 2017.
Park, R. J., Jacob, D. J., Field, B. D., Yantosca, R. M., and Chin, M.: Natural and transboundary pollution influences on sulfate-nitrate-ammonium aerosols in the United States: Implications for policy, J. Geophys. Res.-Atmos., 109, D15204, https://doi.org/10.1029/2003jd004473, 2004.
Paulot, F., Ginoux, P., Cooke, W. F., Donner, L. J., Fan, S., Lin, M.-Y., Mao, J., Naik, V., and Horowitz, L. W.: Sensitivity of nitrate aerosols to ammonia emissions and to nitrate chemistry: implications for present and future nitrate optical depth, Atmos. Chem. Phys., 16, 1459–1477, https://doi.org/10.5194/acp-16-1459-2016, 2016.
Price, C. and Rind, D.: A simple lightning parameterization for calculating global lightning distributions, J. Geophys. Res.-Atmos., 97, 9919–9933, https://doi.org/10.1029/92JD00719, 1992.
Price, C. and Rind, D.: What determines the cloud-to-ground lightning fraction in thunderstorms?, Geophys. Res. Lett., 20, 463– 466, https://doi.org/10.1029/93GL00226, 1993.
Price, C. and Rind, D.: Modeling global lightning distributions in a general circulation model, Mon. Weather Rev., 122, 1930–1939, https://doi.org/10.1175/1520-0493(1994)122<1930:MGLDIA>2.0.CO;2, 1994.
Python: Python [software], https://www.python.org (last access: 27 February 2024), 2024.
Rémy, S., Kipling, Z., Flemming, J., Boucher, O., Nabat, P., Michou, M., Bozzo, A., Ades, M., Huijnen, V., Benedetti, A., Engelen, R., Peuch, V.-H., and Morcrette, J.-J.: Description and evaluation of the tropospheric aerosol scheme in the European Centre for Medium-Range Weather Forecasts (ECMWF) Integrated Forecasting System (IFS-AER, cycle 45R1), Geosci. Model Dev., 12, 4627–4659, https://doi.org/10.5194/gmd-12-4627-2019, 2019.
Russo, M. R., Kerridge, B. J., Abraham, N. L., Keeble, J., Latter, B. G., Siddans, R., Weber, J., Griffiths, P. T., Pyle, J. A., and Archibald, A. T.: Seasonal, interannual and decadal variability of tropospheric ozone in the North Atlantic: comparison of UM-UKCA and remote sensing observations for 2005–2018, Atmos. Chem. Phys., 23, 6169–6196, https://doi.org/10.5194/acp-23-6169-2023, 2023.
Sander, S. P., Abbatt, J., Barker, J. R., Burkholder, J. B., Friedl, R. R., Golden, D. M., Huie, R. E., Kolb, C. E., Kurylo, M. J., Moortgat, G. K., Orkin, V. L., and Wine, P. H.: Chemical Kinetics and Photochemical Data for Use in Atmospheric Studies, Evaluation No. 17, JPL Publication 10-6, Jet Propulsion Laboratory, Pasadena, https://jpldataeval.jpl.nasa.gov/pdf/JPL 10-6 Final 15June2011.pdf (last access: 12 December 2024) 2011.
Schumann, U. and Huntrieser, H.: The global lightning-induced nitrogen oxides source, Atmos. Chem. Phys., 7, 3823–3907, https://doi.org/10.5194/acp-7-3823-2007, 2007.
Schwartz, S. E.: Mass transport considerations pertinent to aqueous phase reactions of gases in liquid-water clouds, in: Chemistry of Multiphase Atmospheric Systems, edited by: Jaeschke, W., Springer, New York, 415–471 pp., https://link.springer.com/chapter/10.1007/978-3-642-70627-1_16 (last access: 12 December 2024), 1986.
Stelson, A. W., Friedlander, S. K., and Seinfeld, J. H.: A note on the equilibrium relationship between ammonia and nitric acid and particulate ammonium nitrate, Atmos. Environ., 13, 369–371, https://doi.org/10.1016/0004-6981(79)90293-2, 1979.
Szopa, S., Naik, V., Adhikary, B., Artaxo, P., Berntsen, T., Collins, W. D., Fuzzi, S., Gallardo, L., Kiendler Scharr, A., Klimont, Z., Liao, H., Unger, N., and Zanis, P.: Short-Lived Climate Forcers, in: Climate Change 2021: The Physical Science Basis. Contribution of Working Group I to the Sixth Assessment Report of the Intergovernmental Panel on Climate Change, edited by: Masson-Delmotte, V., Zhai, P., Pirani, A., Connors, S. L., Péan, C., Berger, S., Caud, N., Chen, Y., Goldfarb, L., Gomis, M. I., Huang, M., Leitzell, K., Lonnoy, E., Matthews, J. B. R., Maycock, T. K., Waterfield, T., Yelekçi, O., Yu, R., and Zhou, B., Cambridge University Press, Cambridge, UK and New York, NY, USA, 817–922, https://doi.org/10.1017/9781009157896.008, 2021.
Telford, P. J., Abraham, N. L., Archibald, A. T., Braesicke, P., Dalvi, M., Morgenstern, O., O'Connor, F. M., Richards, N. A. D., and Pyle, J. A.: Implementation of the Fast-JX Photolysis scheme (v6.4) into the UKCA component of the MetUM chemistry-climate model (v7.3), Geosci. Model Dev., 6, 161–177, https://doi.org/10.5194/gmd-6-161-2013, 2013.
Thornhill, G. D., Collins, W. J., Kramer, R. J., Olivié, D., Skeie, R. B., O'Connor, F. M., Abraham, N. L., Checa-Garcia, R., Bauer, S. E., Deushi, M., Emmons, L. K., Forster, P. M., Horowitz, L. W., Johnson, B., Keeble, J., Lamarque, J.-F., Michou, M., Mills, M. J., Mulcahy, J. P., Myhre, G., Nabat, P., Naik, V., Oshima, N., Schulz, M., Smith, C. J., Takemura, T., Tilmes, S., Wu, T., Zeng, G., and Zhang, J.: Effective radiative forcing from emissions of reactive gases and aerosols – a multi-model comparison, Atmos. Chem. Phys., 21, 853–874, https://doi.org/10.5194/acp-21-853-2021, 2021.
Tost, H.: Chemistry–climate interactions of aerosol nitrate from lightning, Atmos. Chem. Phys., 17, 1125–1142, https://doi.org/10.5194/acp-17-1125-2017, 2017.
Walters, D., Baran, A. J., Boutle, I., Brooks, M., Earnshaw, P., Edwards, J., Furtado, K., Hill, P., Lock, A., Manners, J., Morcrette, C., Mulcahy, J., Sanchez, C., Smith, C., Stratton, R., Tennant, W., Tomassini, L., Van Weverberg, K., Vosper, S., Willett, M., Browse, J., Bushell, A., Carslaw, K., Dalvi, M., Essery, R., Gedney, N., Hardiman, S., Johnson, B., Johnson, C., Jones, A., Jones, C., Mann, G., Milton, S., Rumbold, H., Sellar, A., Ujiie, M., Whitall, M., Williams, K., and Zerroukat, M.: The Met Office Unified Model Global Atmosphere 7.0/7.1 and JULES Global Land 7.0 configurations, Geosci. Model Dev., 12, 1909–1963, https://doi.org/10.5194/gmd-12-1909-2019, 2019.
Wang, Q., Li, Z., Guo, J., Zhao, C., and Cribb, M.: The climate impact of aerosols on the lightning flash rate: is it detectable from long-term measurements?, Atmos. Chem. Phys., 18, 12797–12816, https://doi.org/10.5194/acp-18-12797-2018, 2018.
Wang, M., Kong, W., Marten, R., He, X.-C., Chen, D., Pfeifer, J., Heitto, A., Kontkanen, J., Dada, L., Kürten, A., Yli-Juuti, T., Manninen, H. E., Amanatidis, S., Amorim, A., Baalbaki, R., Baccarini, A., Bell, D. M., Bertozzi, B., Bräkling, S., Brilke, S., Murillo, L. C., Chiu, R., Chu, B., Menezes, L.-P. D., Duplissy, J., Finkenzeller, H., Carracedo, L. G., Granzin, M., Guida, R., Hansel, A., Hofbauer, V., Krechmer, J., Lehtipalo, K., Lamkaddam, H., Lampimäki, M., Lee, C. P., Makhmutov, V., Marie, G., Mathot, S., Mauldin, R. L., Mentler, B., Müller, T., Onnela, A., Partoll, E., Petäjä, T., Philippov, M., Pospisilova, V., Ranjithkumar, A., Rissanen, M., Rörup, B., Scholz, W., Shen, J., Simon, M., Sipilä, M., Steiner, G., Stolzenburg, D., Tham, Y. J., Tomé, A., Wagner, A. C., Wang, D. S., Wang, Y., Weber, S. K., Winkler, P. M., Wlasits, P. J., Wu, Y., Xiao, M., Ye, Q., Zauner-Wieczorek, M., Zhou, X., Volkamer, R., Riipinen, I., Dommen, J., Curtius, J., Baltensperger, U., Kulmala, M., Worsnop, D. R., Kirkby, J., Seinfeld, J. H., El-Haddad, I., Flagan, R. C., and Donahue, N. M.: Rapid growth of new atmospheric particles by nitric acid and ammonia condensation, Nature, 581, 184–189, https://doi.org/10.1038/s41586-020-2270-4, 2020.
Wang, H., Pei, Y., Yin, Y., Shen, L., Chen, K., Shi, Z., and Chen, J.: Observational evidence of lightning-generated ultrafine aerosols, Geophys. Res. Lett., 48, e2021GL093771, https://doi.org/10.1029/2021GL093771, 2021.
Wilson, D. R. and Ballard, S. P.: A microphysically based precipitation scheme for the UK Meteorological Office Unified Model, Q. J. Roy. Meteorol. Soc., 125, 1607–1636, https://doi.org/10.1002/qj.49712555707, 1999.
Wilson, D. R., Bushell, A. C., Kerr-Munslow, A. M., Price, J. D., and Morcrette, C. J.: PC2: A prognostic cloud fraction and condensation scheme. I: Scheme description, Q. J. Roy. Meteorol. Soc., 134, 2093–2107, https://doi.org/10.1002/qj.333, 2008.
Woodward, S.: Modeling the atmospheric life cycle and radiative impact of mineral dust in the Hadley Centre climate model, J. Geophys. Res.-Atmos., 106, 18155–18166, https://doi.org/10.1029/2000JD900795, 2001.
- Abstract
- Introduction
- Lightning-generated NOx parameterisations
- The Met Office Unified Model (UM) with global chemistry and aerosol
- Results and discussion
- Conclusions
- Code availability
- Data availability
- Author contributions
- Competing interests
- Disclaimer
- Acknowledgements
- Financial support
- Review statement
- References
- Supplement
- Abstract
- Introduction
- Lightning-generated NOx parameterisations
- The Met Office Unified Model (UM) with global chemistry and aerosol
- Results and discussion
- Conclusions
- Code availability
- Data availability
- Author contributions
- Competing interests
- Disclaimer
- Acknowledgements
- Financial support
- Review statement
- References
- Supplement