the Creative Commons Attribution 4.0 License.
the Creative Commons Attribution 4.0 License.
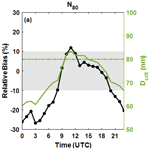
CCN estimations at a high-altitude remote site: role of organic aerosol variability and hygroscopicity
Andrea Casans
Marta Via
Juan Andrés Casquero-Vera
Sonia Castillo
Hassan Lyamani
Alberto Cazorla
Elisabeth Andrews
Daniel Pérez-Ramírez
Andrés Alastuey
Francisco Javier Gómez-Moreno
Lucas Alados-Arboledas
Francisco José Olmo
Gloria Titos
High-altitude remote sites are unique places to study aerosol–cloud interactions, since they are located at the altitude where clouds may form. At these remote sites, organic aerosols (OAs) are the main constituents of the overall aerosol population, playing a crucial role in defining aerosol hygroscopicity (κ). To estimate the cloud condensation nuclei (CCN) budget at OA-dominated sites, it is crucial to accurately characterize OA hygroscopicity (κOA) and how its temporal variability affects the CCN activity of the aerosol population, since κOA is not well established due to the complex nature of ambient OA. In this study, we performed CCN closures at a high-altitude remote site during summer to investigate the role of κOA in predicting CCN concentrations under different atmospheric conditions. In addition, we performed an OA source apportionment using positive matrix factorization (PMF). Three OA factors were identified from the PMF analysis: hydrocarbon-like OA (HOA), less-oxidized oxygenated OA (LO-OOA), and more-oxidized oxygenated OA (MO-OOA), with average contributions of 5 %, 36 %, and 59 % of the total OA, respectively. This result highlights the predominance of secondary organic aerosol (SOA) with a high degree of oxidation at this high-altitude site. To understand the impact of each OA factor on the overall OA hygroscopicity, we defined three κOA schemes that assume different hygroscopicity values for each OA factor. Our results show that the different κOA schemes lead to similar CCN closure results between observations and predictions (slope and correlation ranging between 1.08–1.40 and 0.89–0.94, respectively). However, the predictions were not equally accurate across the day. During the night, CCN predictions underestimated observations by 6 %–16 %, while, during morning and midday hours, when the aerosol was influenced by vertical transport of particles and/or new particle formation events, CCN concentrations were overestimated by 0 %–20 %. To further evaluate the role of κOA in CCN predictions, we established a new OA scheme that uses the OA oxidation level (parameterized by the f44 factor) to calculate κOA and predict CCN. This method also shows a large bias, especially during midday hours (up to 40 %), indicating that diurnal information about the oxygenation degree does not improve CCN predictions. Finally, we used a neural network model with four inputs to predict CCN: N80 (number concentration of particles with diameter > 80 nm), OA fraction, f44, and solar global irradiance. This model matched the observations better than the previous approaches, with a bias within ± 10 % and with no daily variation, reproducing the CCN variability throughout the day. Therefore, neural network models seem to be an appropriate tool to estimate CCN concentrations using ancillary parameters accordingly.
- Article
(2103 KB) - Full-text XML
-
Supplement
(867 KB) - BibTeX
- EndNote
Cloud condensation nuclei (CCN) are those aerosol particles that act as the seeds for cloud droplet activation. The number of CCN in the atmosphere determines the number of cloud droplets that form. This in turn affects cloud properties such as reflectivity and lifetime (Twomey, 1977; Albrecht, 1989), playing a critical role in the regulation of Earth's energy balance, climate, and hydrological cycle (Lohmann and Feichter, 2005).
The radiative forcing associated with the indirect effect of aerosols through aerosol–cloud interaction is larger (−1.0 ± 0.7 W m−2) than the direct effect of aerosol through aerosol–radiation interaction (−0.25 ± 0.25 W m−2) (Forster et al., 2021). Therefore, understanding physicochemical properties of aerosol particles that can act as CCN could minimize CCN prediction errors, which are essential to reducing the uncertainty of global aerosol–cloud interactions (Seinfeld et al., 2016). For that reason, the spatial and temporal variation in CCN, together with parameters controlling CCN concentrations, has been studied intensively around the world in the last decades (Deng et al., 2018; Paramonov et al., 2015; Rose et al., 2010; Salma et al., 2021; Schmale et al., 2018; Park et al., 2023; Kulkarni et al., 2023; Rejano et al., 2021; Che et al., 2016).
If ambient conditions that regulate water vapor supersaturation (SS) are disregarded, the main aerosol properties influencing the CCN activity are particle size, chemical composition, and mixing state (Dusek et al., 2006; Cubison et al., 2008; Wang et al., 2010; Deng et al., 2018; Kuang et al., 2020b). To assess how these aerosol properties control the CCN activity under different ambient aerosol composition and mixing conditions, closure studies (i.e., comprehensive evaluation and comparison of measurements from different instruments or methodologies that aim to measure the same or related parameters) have proven to be very useful (Cai et al., 2022; Crosbie et al., 2015; Ervens et al., 2010; Jurányi et al., 2010; Ren et al., 2018; Kulkarni et al., 2023).
Particle number size distribution (PNSD) is the main factor controlling CCN estimations (Crosbie et al., 2015; Dusek et al., 2006). Many studies assume an activation threshold diameter from which all particles are considered activated (Asmi et al., 2011; Cho Cheung et al., 2020; Hoyle et al., 2016; Rose et al., 2017; Casquero-Vera et al., 2023). However, reducing aerosol–cloud interaction uncertainties requires more accurate CCN predictions, which, in turn, requires knowledge about the aerosol chemical composition (Che et al., 2016, 2017).
The effect of chemical composition in CCN activity is usually treated through the hygroscopicity parameter κ (Petters and Kreidenweis, 2007), which can be obtained using bulk or size-resolved chemical composition measurements through a simple volume mixing rule (Petters and Kreidenweis, 2007). However, while the aerosol hygroscopicity of inorganic substances is well characterized, the quantification of organic aerosol (OA) hygroscopicity (κOA) remains challenging. This is due to the large variety of organic compounds within OA, resulting in a wide range of hygroscopicity values that introduce large uncertainties in CCN predictions (Casans et al., 2023; Hallquist et al., 2009; Jimenez et al., 2009; Zhang et al., 2007). It has been proven that CCN predictions are very sensitive to κOA and that a poor knowledge of κOA variability leads to large biases in CCN closures, especially at OA-dominated sites (Cai et al., 2022; Deng et al., 2019; Thalman et al., 2017; Gunthe et al., 2009; Liu and Wang, 2010).
To obtain an accurate estimation of κOA, knowledge of OA sources and their time variability is required with high time resolution (Wu et al., 2016; Deng et al., 2019; Ren et al., 2023; Cai et al., 2018). Positive matrix factorization (PMF) has proven to be a powerful tool for identifying the main OA components by using the organic mass spectra (Via et al., 2021; Minguillón et al., 2015; Crippa et al., 2013). Previous studies explained κOA variability in terms of OA sources assuming specific hygroscopicity values for each source (Cai et al., 2022; Cerully et al., 2015; Deng et al., 2019; Thalman et al., 2017) or established κOA parameterizations based on the oxidation degree (Duplissy et al., 2011; Mei et al., 2013; Wu et al., 2016; Chen et al., 2017). However, assumptions about κOA needed for accurate CCN predictions vary greatly among studied sites (Ervens et al., 2010; Cubison et al., 2008; Tao et al., 2021; Kuang et al., 2020b), due to the wide variety of sources and atmospheric processes affecting OA.
Organic aerosol usually dominates aerosol mass concentration in the fine fraction in high-altitude environments (e.g., Fröhlich et al., 2015; Ripoll et al., 2015; Zhang et al., 2023). In addition, since cloud formation conditions can occur at these sites, high-altitude sites are unique locations for studying aerosol–cloud interactions (Friedman et al., 2013; Li et al., 2020; Iwamoto et al., 2021; Jurányi et al., 2011). Moreover, these sites are often exposed to free-troposphere conditions, where the submicron aerosol population tends to be an internal mixture of background particles. In this case, satisfactory CCN predictions can be obtained using simple assumptions about aerosol chemical composition (Jurányi et al., 2010; Duan et al., 2023). However, during some conditions, such as thermally driven upslope flow, high-altitude sites might be influenced by planetary boundary layer (PBL) air with pollution particles being efficiently transported to high-altitude sites and affecting CCN activity (Jayachandran et al., 2018; Rejano et al., 2021). Also, at these sites, high-insolation conditions during midday hours promote photochemical processes that can lead to new particle formation (NPF) events, completely transforming the background aerosol population from a homogeneous aerosol population to a complex mixture of particles with different chemical and microphysical characteristics (Friedman et al., 2013; Rose et al., 2017; Shang et al., 2018). During these more complex conditions, when NPF and/or PBL transport affect the aerosol population, simple approaches for CCN predictions tend to overpredict the observations (Asmi et al., 2012; Che et al., 2017; Hu et al., 2020; Zhang et al., 2017). Further investigation on how the changes in aerosol composition and hygroscopicity affect CCN variability at these sites is required.
In this study, we investigate OA sources, their temporal variability, and their influence on CCN predictions at a high-altitude mountain site during an intensive summer field campaign. To understand the influence of aerosol composition on CCN, we calculate the overall aerosol hygroscopicity from bulk chemical composition measurements and then assume different OA schemes to retrieve κOA. We focus the analysis on the influence that OA might have on the CCN predictions under different atmospheric conditions throughout the day. Additionally, a non-analytical model approach using neural networks was developed to predict CCN concentrations based on ancillary information on particle number concentration, OA mass fraction and oxygenation degree, and solar global irradiance.
2.1 Experimental site
Aerosol measurements presented in this study were conducted at the Sierra Nevada station (SNS) from 8 June to 13 July 2021 in the frame of the BioCloud field campaign (Jaén et al., 2023). The main objective of the campaign was to evaluate the impact of biogenic and anthropogenic emissions on the CCN budget at this high-altitude mountain site. SNS is located in the Sierra Nevada mountain range in southeastern Spain (37.10° N, 3.39° W; 2500 ) and is part of the Andalusian Global Observatory of the Atmosphere (AGORA). Measurements at SNS are performed following the Aerosol, Cloud and Trace Gases Research Infrastructure (ACTRIS; http://actris.eu, last access: 12 December 2024) standards for in situ measurements at high-altitude observatories (Pandolfi et al., 2018), and the station is part of the NOAA Federated Aerosol Network (NFAN; Andrews et al., 2019).
SNS is located at a horizontal distance of 21 km and has an altitude difference of 1820 m from the city of Granada, which is located downslope of the mountains in a valley. Granada is a medium-sized city with a population of 232 208 (https://www.ine.es, last access: 12 December 2024), which increases up to 530 000 if the wider metropolitan area is considered. The main local aerosol source in Granada is road traffic, including both motor vehicle exhaust and the re-suspension of particulate material from the roadways (Casquero-Vera et al., 2021; Rejano et al., 2023; Titos et al., 2014). These pollutants emitted in the Granada area can influence the aerosol properties observed in Sierra Nevada (Rejano et al., 2023). Atmospheric aerosol at SNS has been reported to be affected by the transport of particles from the Granada metropolitan area because of planetary boundary layer (PBL) growth and the mountain–valley breeze phenomenon (Rejano et al., 2021; Jaén et al., 2023; Casquero-Vera et al., 2020). Aerosol sources at SNS during summer are primarily related to the transport of pollutants from lower altitudes and regional transport, biogenic emissions from the vegetation, and desert dust transported from the Sahara (Jaén et al., 2023). Furthermore, new particle formation (NPF) events are relatively frequent at midday, representing another important source of aerosol particles at this site (Casquero-Vera et al., 2020; Rejano et al., 2021; de Arruda Moreira et al., 2019).
2.2 Aerosol sampling and instrumentation
Sample air for all instruments was obtained through a stainless-steel tube located in the rooftop of the observatory, which is a three-storey building. Inside this tube there are several smaller stainless-steel pipes, which provide sample air to the different instruments (Baron and Willeke, 2005). All measurements reported here refer to ambient conditions and were performed without aerosol size cut. Further information about the observatory and experimental conditions can be found in previous studies performed at SNS (Casquero-Vera et al., 2020; Rejano et al., 2021; Jaén et al., 2023). In the following we describe the instruments used in this study.
A time-of-flight aerosol chemical speciation monitor (ToF-ACSM; Aerodyne Research Inc., Billerica, USA; Fröhlich et al., 2013) was deployed to measure the mass concentration and chemical composition of non-refractory submicron aerosol particles (NR-PM1) with a 10 min time resolution. The chemical species determined by the instrument were OA, , , , and Cl−. The instrument was operated at a flow rate of 3 L min−1, and the air sample passed through a Nafion dryer, maintaining the incoming relative humidity below 40 %. During the campaign, the sample flow into the instrument was 0.108 L min−1. A PM1 standard aerodynamic lens focused the sample flow into a narrow beam and transmitted particles with a vacuum aerodynamic diameter between 70 and 700 nm (Liu et al., 2007). Non-refractory particles were flash vaporized at 600 °C with a tungsten vaporizer and were ionized by electron impact at 70 eV. The instrument was equipped with a capture vaporizer that enhanced vaporization and gave a collection efficiency of 1. After sample ionization, the ions were introduced into a time-of-flight mass spectrometer (ETOF, Tofwerk Inc.), where they were orthogonally extracted and separated according to their mass-to-charge ratio (). The mass spectra were obtained for ions ranging from 12 to 200 Th. Finally, the mass spectral signals were converted to mass concentration (in µg m−3) using the ionization efficiency calculated from calibration curves of known reference species (Fröhlich et al., 2013).
Flow calibrations for the ToF-ACSM were performed before and after the BioCloud field campaign. The relative ionization efficiency (RIE) calibrations for and were performed before the campaign using dry, size-selected 300 nm particles of ammonium nitrate and ammonium sulfate generated by an Aerosol Generator atomizer (TSI 3076). For more details about the ToF-ACSM calibrations, see Fröhlich et al. (2013). Data processing was performed using the data analysis package “Tofware” (version 2.5.13, https://www.tofwerk.com/software/tofware/, last access: 12 December 2024) running in the Igor Pro 7 environment (Wavemetrics Inc., Oregon, USA). During the campaign, data were corrected for changes in the sample flow rate and the N2 signal ( 28), which is assumed to be constant in the atmosphere.
The CCN measurements were performed using a cloud condensation nuclei counter (CCNc; Droplet Measurement Technologies, model CCN-200), which is based on a cylindrical continuous-flow thermal-gradient diffusion chamber where constant temperature gradients are applied, generating different SS conditions (Roberts and Nenes, 2005). One of the columns sampled polydisperse particles, while the other column was connected to a differential mobility analyzer (DMA) to measure size-resolved CCN. For both columns, CCN concentrations were measured at four SS values, 0.2 %, 0.4 %, 0.6 %, and 0.8 %, taking 10 min at each SS value. Only polydisperse measurements at 0.2 %, 0.4 %, and 0.6 % SS are shown in this study. To ensure data quality due to instabilities of the instrument at each SS, CCN concentrations were filtered according to the criteria of Rejano et al. (2021) to ensure that CCN number concentrations (NCCN) measured at SS that differed by more than 20 % from the SS set point were disregarded. The total flow rate of the instrument was fixed at 0.5 L min−1 with an aerosol flow of 0.05 L min−1 and a sheath flow of 0.45 L min−1. The flow rates were calibrated on site before and after the campaign and were checked regularly during the campaign. SS calibration using monodisperse ammonium sulfate was also performed on site at the beginning and at the end of the campaign following the procedure described in ACTRIS guidelines (http://actris.nilu.no/Content/SOP, last access: 12 December 2024). Both calibrations provided satisfactory results and showed no change in instrument performance.
The particle number size distribution (PNSD) was measured in the mobility diameter range between 12–535 nm every 5 min using a scanning mobility particle sizer (SMPS; TSI model 3938) composed of a differential mobility analyzer (DMA; TSI 3081) and a condensation particle counter (CPC; TSI 3750). The aerosol flow rate was 1 L min−1, and the sheath flow was 5 L min−1. The quality of the SMPS measurements was assured by frequently checking the flow rates and performing 203 nm PSL checks, following the ACTRIS and Global Atmospheric Watch (GAW) recommendations (Wiedensohler et al., 2012).
An aethalometer (Model AE-33, Magee Scientific) was used to determine the equivalent black carbon (eBC) mass concentration with a time resolution of 1 min. The aethalometer draws the ambient air at a constant flow rate of 4 L min−1. The eBC was determined from the aerosol absorption coefficient at 880 nm using a mass absorption cross-section of 7.77 m2 g−1 as recommended by the manufacturer. The PM1 mass concentration was estimated as the sum of the mass of non-refractory components obtained by ToF-ACSM and eBC mass concentration measured by the aethalometer as suggested by the second deliverable of the COST Action CA16109 COLOSSAL.
Finally, a Hukseflux LP02-05 pyranometer was used to measure the horizontal solar global irradiance with 5 min resolution.
3.1 Source apportionment of organic aerosol
The source apportionment of organic aerosol was performed using the positive matrix factorization (PMF) method (Paatero and Tapper, 1994) using the multilinear engine ME-2 (Paatero, 1999). The PMF is a multivariate factor analysis technique that allows the decomposition of the measured OA mass spectral matrix (X), where the matrix columns are the variables ( ions) and the matrix rows are the observations (ToF-ACSM timestamps), into two matrices, the factors or source profiles matrix (F) and the contributions matrix (G):
where eij represents the elements of the residual matrix (E), accounting for unexplained information of X in the p factors solution. The number of PMF factors, p, is a pre-set parameter that must be established. Once the number of factors is fixed, the algorithm solves Eq. (3) iteratively, minimizing the Q function, which is defined as
where σij is the measurement uncertainties corresponding to the xij input data. The solution with the correct number of factors should give near unity, with Qexp being the expected value of Q, and is calculated as Qexp = , with n being the number of observations and m being the number of variables.
To improve the source apportionment characterization and achieve environmentally meaningful solutions, the ME-2 methodology allows the establishment of a priori mass profiles of known OA sources, the so-called anchor profiles, based on previous scientific knowledge at an experimental site (Canonaco et al., 2013) or on chamber data. The strength of this a priori constraint is modulated through the a-value approach (Paatero and Hopke, 2009; Brown et al., 2012). The a-value establishes how much deviation from the anchor profile the model allows to the solution factor. Thus, a fully constrained factor has an a-value equal to 0, whereas for unconstrained factors the a-value is equal to 1. The ME-2 engine initialization and the results analysis were done using the SoFi v.8 toolkit (Source Finder; Canonaco et al., 2013) for Igor Pro environment. The PMF was run for a range of solutions from three to five factors, and the mass spectra considered ranged between 12 and 120 Th, since higher ions contribute only marginally to the mass spectra and exhibit a low signal-to-noise ratio (SNR < 0.2).
3.2 CCN estimations and activation properties using κ-Köhler theory
The κ-Köhler theory establishes a mathematical relation between the water vapor supersaturation ratio, the droplet diameter (D), the dry particle size (Ddry) acting as CCN, and the κ parameter (Petters and Kreidenweis, 2007) as follows:
where ρw and Mw are the density and molar mass of water, respectively; R is the universal gas constant; T is the absolute temperature; and is the surface tension of the solution–air interface (assumed to be equal to the surface tension of pure water). Therefore, from the overall κ of an aerosol population, we can estimate the Dcrit, which is the threshold size at which particles become CCN, at a certain SS using κ-Köhler theory. This method assumes a homogenous aerosol population mixture (internally mixed) where all particles larger than this cutoff diameter activate (Jurányi et al., 2011). Thus, NCCN is estimated by summing up the PNSD from Dcrit to the upper limit of the size distribution as follows:
Alternatively, we can perform the inverse calculation integrating the PNSD from its upper limit to the diameter at which the integral value equals the simultaneously measured NCCN(SS) with the CCNc. Then, the effective hygroscopicity parameter can be retrieved using the κ-Köhler equation from aerosol size distribution and CCN concentration measurements (Jurányi et al., 2011), substituting Ddry with Dcrit in Eq. (3), being by definition the maximum of the curve of the instrument SS at which Dcrit was calculated. These CCN-derived κ values (κCCN) quantify the effective hygroscopicity of activated particles in the CCNc and exhibit a dependency on SS (Kammermann et al., 2010).
3.3 Estimation of aerosol hygroscopicity from chemical composition measurements
One of the most commonly used approaches to estimate the total aerosol hygroscopicity from chemical composition measurements is based on the Zdanovskii–Stokes–Robinson (ZSR) approach. By regarding ambient aerosols as a mixture of individual compounds, the hygroscopicity parameter can be retrieved using a mixing rule in terms of the volume fractions of the chemical species (Petters and Kreidenweis, 2007) as follows:
where εi is the volume fraction of each chemical species and κi its corresponding hygroscopicity. This approximation provides a successful explanation of observations as shown in previous studies (Bougiatioti et al., 2009, 2016; Rose et al., 2010; Wang et al., 2010). The summation is performed over all chemical species considered for the calculation of the κchem parameter. In this study we have considered three main terms in Eq. (3): OA, inorganic aerosol (IA), and eBC. Thus, κchem can be estimated as follows:
where κOA (εOA), κIA (εIA), and κBC (εBC) are the hygroscopicity parameters (volume fractions) of organic aerosols, inorganic aerosols, and BC, respectively. The contribution of IA to κchem considers some inorganic salts (ammonium nitrate, ammonium sulfate, ammonium bisulfate, and sulfuric acid) present in the atmosphere. The volume fractions of these inorganic salts were obtained by the simplified ion pairing scheme presented by Gysel et al. (2007) using the inorganic species measured by the ToF-ACSM (, , and ions). The density and hygroscopicity parameters for each inorganic salt were taken from previous studies (Kuang et al., 2020b; Wu et al., 2016) and are summarized in Table S1 in the Supplement.
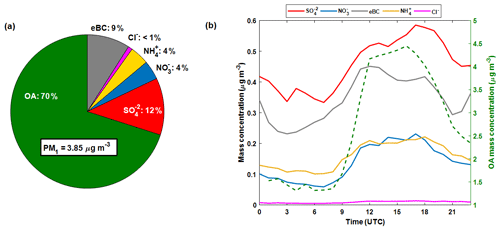
Figure 1(a) Pie chart of PM1 inorganic species (, , , Cl−), organic aerosol, and eBC mass concentration averaged over the BioCloud campaign and (b) mean diurnal pattern evolution for each species.
The inorganic contribution to κchem is assumed to be a well-defined term in Eq. (5). We assumed that BC particles were completely hydrophobic (κBC=0) for calculating κchem, which is a reasonable assumption, as suggested in previous studies (Deng et al., 2019; Kuang et al., 2020b; Schmale et al., 2018). Unlike inorganic species that exhibit a well-characterized hygroscopic behavior, the water uptake capacity of OA species is poorly understood because of the presence of diverse organic species (Casans et al., 2023; Hallquist et al., 2009; Kanakidou et al., 2005; Rastak et al., 2017). This diversity makes determining κOA extremely challenging (Kuang et al., 2020a). In Sect. 4.2, we present different OA schemes in terms of the PMF solution to estimate κOA, assuming different density and hygroscopicity values for each OA source.
3.4 Performing non-analytical solutions for CCN predictions: neural networks
Apart from analytical solutions based on predefined relationships between variables, non-analytical solutions like machine learning techniques have become a powerful alternative to predict certain variables using ancillary information as input. Indeed, neural networks have been applied with remarkable success in recent years for regression problems in the framework of atmospheric sciences (Biancofiore et al., 2017; Comrie, 1997; Spellman, 1999) to relate atmospheric variables with non-linear and highly complex behavior.
For our regression problem, we built a neural network which uses four input parameters and has NCCN as the output parameter. Our neural network consists of a two-layer feed-forward network with sigmoid hidden neurons and linear output neurons. We chose a hidden layer of 10 neurons after verifying that results did not improve with more neurons. We used the back-propagation algorithm (Rumelhart et al., 1986) with Bayesian regularization (Foresee and Hagan, 1997) for training the network. Data were split into training, validation, and test, using 55 % of the data for training, 20 % of the data to halt training when generalization stops improving (neural network validation), and the remaining 25 % of data for testing. Each subset of data was obtained by a random selection of observations. The entire modeling process was performed using the Neural Net Fitting tool of MATLAB.
4.1 BioCloud field campaign overview
In this section we present an overview of the PM1 chemical composition including identification of OA sources and the analysis of CCN activation properties from 8 June to 13 July 2021 within the framework of the BioCloud field campaign.
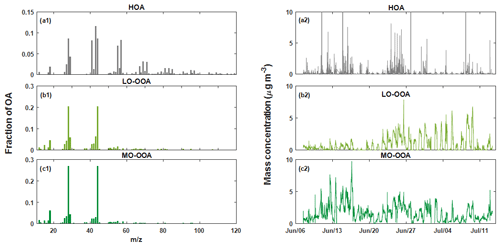
Figure 2Mass spectra of the three OA factors (left panels) and their time series evolution (right panels) during the BioCloud field campaign.
4.1.1 Submicron aerosol chemical composition and source apportionment
The average PM1 concentration during the campaign was 3.85 ± 2.88 µg m−3, with 10 min average concentrations ranging from 0.15 to 15.3 µg m−3. Figure 1a shows the mean PM1 concentration and relative contribution of the considered species (OA, , , , Cl−, eBC) to the total PM1. On average, the most abundant aerosol component is OA (2.68 µg m−3), followed by (0.46 µg m−3) and eBC (0.33 µg m−3), with relative contributions of 70 %, 12 %, and 9 %, respectively. Inorganic components (, , , Cl−) represent 20 % of the total PM1 concentration on average, indicating the large contribution of organics at this high-altitude remote site during summer. Similar OA dominance is observed in remote sites worldwide in summer (Fröhlich et al., 2015; Heikkinen et al., 2020; Jimenez et al., 2009; Ripoll et al., 2015; Zhang et al., 2007). The most abundant inorganic component is , which is expected during summer with high temperature and insolation conditions that favor the formation of this compound (Pey et al., 2009; Titos et al., 2014). and species exhibit similar low mass concentrations (0.15 µg m−3), probably due to the high summer temperatures that favor the instability of ammonium nitrate. Cl− shows a negligible concentration, near to the detection limit of the instrument. The mean eBC mass concentration (0.33 µg m−3) is in the range of those previously observed at SNS (Rejano et al., 2021) and in the range of values reported at other high-altitude remote sites during summer, with values ranging between 0.2 and 0.5 µg m−3 across all sites (Ripoll et al., 2015; Zeb et al., 2020; Gramsch et al., 2020).
To gain insight into the local and regional aerosol sources and the underlying atmospheric aerosol processes that control aerosol evolution, diurnal variations in the mass concentration of the measured aerosol species were investigated (Fig. 1b). The mass concentration of inorganic species exhibited an increase throughout the day, starting at 08:00 UTC (local time minus 2 h). , , and mass concentrations followed a similar diurnal pattern. Based on these diurnal patterns, inorganic species are most likely transported from the Granada urban area due to upslope mountain breezes and the increase in the PBL height during the daytime. OA also increased at midday, but the increase was sharper, reaching a maximum between 12:00–16:00 UTC. OA exhibits a larger increase in concentration at midday hours compared to the other species (Fig. 1b), which might suggest the influence of upslope transport but also additional sources of OA in the vicinity of the measurement site (such as local emissions or secondary processes like nucleation). Finally, eBC mass concentration increased more gradually, starting at 03:00 UTC and reaching a maximum at 11:00 UTC. The earlier increase in eBC with respect to IA and OA species might be related to some local primary emissions during the early morning, although most of the eBC observed at SNS is due to upslope transport (Rejano et al., 2021).
By analyzing the OA mass spectra using PMF methodology, it is possible to infer whether the OA origin is locally formed and/or transported. To further explore the phenomenology of OA, the OA mass concentration was separated into different OA factors according to the PMF analysis. According to the values and the physical interpretation of the PMF solution, the most reliable solution was the three-factor solution with the following OA sources: hydrocarbon-like OA (HOA), less-oxidized oxygenated OA (LO-OOA), and more-oxidized oxygenated OA (MO-OOA). Once the three OA sources were identified, a new constrained PMF solution was obtained to improve the source apportionment. We constrained the HOA factor to the Crippa et al. (2013) anchor profile, which is considered the standard mass profile for HOA, with an a-value equal to 0.1. The LO-OOA and MO-OOA factors were kept unconstrained to adapt better to the site-specific aerosol characteristics.
The mass spectra profiles and the time series for each OA factor are presented in Fig. 2. As mentioned above, the first factor was constrained to the standard HOA profile; therefore, the obtained mass spectrum has a high contribution of fragments ( 41, 43, 55, 57, 69, 71; Fig. 2a1), also known as aliphatic hydrocarbons. These ions are typically related to primary emissions of diesel exhaust (Canagaratna et al., 2010; Crippa et al., 2013). The other two factors accounted for virtually all OA at SNS (around 95 %) and were resolved freely by the model. Both secondary factors (LO-OOA and MO-OOA) are quite oxidized, with large contributions of 28 and 44 (Fig. 2b1 and c1). OA at this site is mostly composed of oxygenated OA, which agrees with previous observations at mountain sites during summer, when secondary organic aerosol (SOA) formation through photochemical oxidation is more efficient (Ripoll et al., 2015). The fraction of 43 (C2H3O+) and 44 () ions relative to the whole mass spectra (f43 and f44, respectively) indicates the aerosol oxidation degree and allows differentiation of the OOA into less-oxidized OOA (i.e., LO-OOA with a higher ratio) and more oxidized OOA (i.e., MO-OOA with a lower ratio) (Fröhlich et al., 2015; Ng et al., 2010).
The results of the PMF show average contributions of 5 %, 36 %, and 59 % of HOA, LO-OOA, and MO-OOA, respectively, to the total OA concentrations during the measurement campaign. The low contribution of the HOA factor (which represents 3.5 % of the total PM1 during the campaign) highlights the absence of important local primary OA (POA) sources close to the measurement site. However, sporadic peaks were observed throughout the field campaign (Fig. 2a2), probably related to occasional local combustion emissions (Jaén et al., 2023). The first half of the campaign (before 26 June) was characterized by a higher contribution of MO-OOA (mean values for this period were 2.0 ± 1.4 and 0.7 ± 0.8 µg m−3 for MO-OOA and LO-OOA, respectively), while LO-OOA became more relevant during the second half of the campaign (mean values for this period were 1.1 ± 0.9 and 1.2 ± 1.6 µg m−3 for MO-OOA and LO-OOA, respectively) (Fig. 2). The higher abundance of MO-OOA in the first half of the campaign might be associated with less efficient transport and the predominance of stagnant conditions favoring the presence of aged aerosols, while the higher LO-OOA concentration might be associated with more efficient transport to SNS due to vertical transport of particles and gaseous precursors from lower altitudes by orographic buoyant upward flow. These differences in the OA origin during each period can be related to different meteorological conditions for the two periods.
Figure S1 in the Supplement shows the time series of meteorological variables (temperature, pressure, and relative humidity) during the campaign. The second half of the campaign is characterized by higher temperatures, higher pressure, and lower relative humidity compared to the first half. In addition, we observed that the diurnal pattern of wind speed is very similar during the whole campaign (with higher wind speeds during the evening and lower wind speeds in the central hours of the day), but the second half of the campaign shows higher values of the wind speed with respect to the first half of the campaign (Fig. S2 in the Supplement). The wind direction was also predominantly from the west for the whole campaign, but we observed that the first half of the campaign shows a more pronounced diurnal pattern and more influence of other wind directions (Fig. S2). During the second half of the campaign, a more constant wind direction is observed, suggesting a continuous transport of aerosol from the valley to the mountain. The significant difference between the meteorological conditions in both halves of the campaign can be associated to a more efficient transport of aerosol from the valley to the mountain during the second half of the campaign, which would involve differences in the aerosol physicochemical properties and, in particular, in the predominance of the OA factors (50 % contribution of LO-OOA and 48 % contribution of MO-OOA during the second half compared to 26 % and 72 % during the first half). A detailed analysis of the air masses and wind influence in aerosol composition during the BioCloud campaign is shown in Jaén et al. (2023).
To check the effectiveness of aerosol transport due to the mountain–valley breeze regime, eBC concentration can be used as a tracer of transported aerosols from lower altitudes, due to absence of local BC sources. During the second half of the campaign, eBC shows a more pronounced diurnal pattern, reaching higher concentrations during midday hours compared with the first half of the campaign (Fig. S3 in the Supplement); however, differences between both diurnal patterns are not enough to assure us that the predominance of each OA factor is related to different atmospheric conditions.
4.1.2 CCN activation properties
Aerosol chemical composition plays an important role in defining aerosol hygroscopicity and CCN activation properties (Svenningsson et al., 2006; Liu et al., 2018). In this sub-section, we link some aerosol physical properties which are directly related to the CCN activity, such as total particle concentration (Ntot), nucleation mode particle concentration (Nnucl; defined as the concentration of particles below 25 nm), Aitken mode particle concentration (NAit; diameters between 25 and 100 nm), accumulation mode particle concentration (Nacc; defined as the particle concentration above 100 nm), and some activation parameters (NCCN, Dcrit, κCCN) at different SS values with the submicron chemical composition described previously. A statistical overview of these parameters (mean, median, standard deviation, and percentiles 25 and 75) is shown in Table S2 in the Supplement. The mean NCCN values range from 320 ± 280cm−3 at SS = 0.2 % to 800 ± 700 cm−3 at SS = 0.6 %. The mean Dcrit value at SS = 0.2 % is 111 ± 21 nm, indicating that particle activation is limited to accumulation mode particles. At higher SS, some Aitken mode particles start to contribute to NCCN, since mean Dcrit values decrease with SS (72 ± 18 nm at 0.4 % and 58 ± 16 nm at 0.6 %). In contrast, CCN-derived κ values (κCCN) are mainly constrained to the range between 0.1–0.25 (which is the interquartile range for all SS; see Table S2), showing little dependence on SS, with median values of 0.18, 0.15, and 0.13 at SS = 0.2 %, 0.4 %, and 0.6 %, respectively. Overall, the aerosol activation properties agree with previous observations of these parameters at SNS (Rejano et al., 2021; Casquero-Vera et al., 2020) and with those reported at other mountain sites during summer (Asmi et al., 2012; Georgakaki et al., 2021; Jurányi et al., 2011; Rejano et al., 2021).
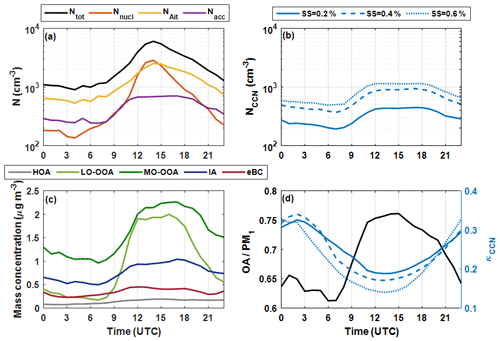
Figure 3Mean diurnal pattern of (a) particle number concentration and each aerosol mode (Ntot, Nnucl, NAit, Nacc), (b) CCN concentrations, (c) OA factors and IA species mass concentration, and (d) OA and PM1 ratio and CCN-derived kappa.
To evaluate the influence of chemical species on the activation properties, Fig. 3 shows the mean diurnal patterns of OA factors, IA, eBC, and particle concentration for each aerosol mode (Nnuc, NAit, and Nacc), along with κCCN and particle and CCN number concentrations. All variables exhibit a clear diurnal pattern but with some differences among them. To prove the significance of the difference between night and day hours for all variables presented in Fig. 3, we performed the Wilcoxon rank-sum test (also called Mann–Whitney U). The test demonstrated the significance of the difference between day and night data for all variables (NCCN, Ntot, Nnucl, NAitk, Nacc, κ, and chemical species mass concentrations) with a p-value lower than 10−10, in all cases. Regarding particle number concentration in the different modes, Nnucl exhibits a clear and sharp peak around midday hours (maximum at 14:00 UTC) due to the impact of new particle formation (NPF) events (Fig. 3a). Nacc exhibits a flatter pattern, with the increase in concentrations observed at midday mostly associated with vertical transport due to the mountain–valley breeze regime and PBL height increase throughout the day. NCCN at all SS values follows a similar diurnal evolution (Fig. 3b) to Nacc, with maximum CCN concentrations observed during the midday hours and minimum concentrations during the night.
The overall hygroscopicity of the activated particles (κCCN) exhibits an inverse diurnal pattern to the other aerosol variables (Fig. 3d), with a decrease during morning and midday hours coinciding with the NCCN increase. This decrease in κCCN is accompanied by an increase in the OA contribution to PM1 (Fig. 3d); however, it is not directly related because κCCN starts to decrease around 03:00 UTC and the OA/PM1 ratio starts to increase around 06:00 UTC. The ratio maximum (values higher than 0.75) was observed between 12:00–15:00 UTC due to the higher relative increase in LO-OOA and MO-OOA with respect to IA and eBC during those hours (Fig. 3c), coinciding with the κCCN minimum between 13:00–14:00 UTC for all SS. Figure 3c reveals that all species are affected by vertical upslope transport during morning and midday hours; however, LO-OOA can also be affected during midday hours by SOA formation linked to photochemical oxidation induced by high solar irradiance values together with high temperatures (Fig. S4b in the Supplement) and a high concentration of O3 and NOx (Fig. S4a) (Minguillón et al., 2016; Via et al., 2021). During the night, we observed the highest values of κCCN; this is probably related to the large contribution of inorganics to PM1 in this period, since IA species have the highest hygroscopicity values. At all SS investigated, κCCN values are very similar during the night (around 0.32), while κCCN differences among SS values are enhanced during midday hours (Fig. 3d). This difference is likely due to the aerosol population becoming more dominated by OA (mainly LO-OOA) at midday and requiring higher SS to activate less hygroscopic particles. Note that the diurnal pattern of κCCN at all SS is constrained between 0.15 to 0.3, which is in the typical range for hygroscopic organic species (Kuang et al., 2020a), in agreement with the predominance of MO-OOA in our PM1 measurements. These observations indicate that OA and its oxygenation degree (higher or lower contribution of MO-OOA/LO-OOA) might be an important factor controlling the overall aerosol hygroscopicity at SNS during the day.
4.2 Predicting CCN concentration: the role of organic aerosol
In the previous section, we observed that the diurnal variability in the κ parameter might be related to both the OA content and its oxidation degree. In this section, we apply different approaches to predict CCN concentrations and evaluate the impact of OA sources in the overall performance of the closure scheme depending on the underlying assumptions. We use the total aerosol hygroscopicity calculated from PM1 chemical composition measurements (κchem) using three different organic hygroscopicity schemes for CCN calculation and discuss the degree of agreement of the different CCN closures under different atmospheric conditions. Then, another approach to estimate κOA in terms of the f44 parameter is presented to link the hygroscopicity changes with the aerosol oxidation degree. Finally, a neural-network-based approach using ancillary parameters is used to predict the CCN concentrations at Sierra Nevada.
4.2.1 Using different OA hygroscopicity schemes
Using the bulk chemical composition measurements, we estimated the overall κchem as explained in Sect. 3.3 to predict NCCN using κ-Köhler theory and PNSD data. For the IA contribution to κchem, the Cl− species was neglected due to its low contribution at SNS (Cl− concentrations were very close to the detection limit of the instrument), as shown in Sect. 4.1.1. In this study we used different κ values for the obtained OA factors (HOA, LO-OOA, and MO-OOA) to compute the overall κchem in three different ways:
-
Scheme 1. We assume that , which is the typical value observed for κOA in a wide variety of environments (Gunthe et al., 2009; Jurányi et al., 2011; Rose et al., 2010; Schmale et al., 2018).
-
Scheme 2. We assume that HOA are hydrophobic particles, κHOA=0 (Cappa et al., 2011; Jimenez et al., 2009; Kanakidou et al., 2005; Thalman et al., 2017), and that LO-OOA and MO-OOA components have a constant κ value of 0.1.
-
Scheme 3. Since the level of oxidation of OA affects its hygroscopicity, we assume specific hygroscopicity values for LO-OOA and MO-OOA (κLO-OOA=0.08 and κMO-OOA=0.16), as reported by Cerully et al. (2015). HOA is again assumed to be non-hygroscopic (κHOA=0).
Table 1 summarizes the densities and hygroscopicity values of HOA, LO-OOA, and MO-OOA used for calculating the κchem value for the different OA schemes. The volume fractions of OA components were obtained assuming the density of OOA as 1.4 g m−3, and for HOA the typical POA density of 1 g m−3 was assumed (Kuang et al., 2020a; Wu et al., 2016).
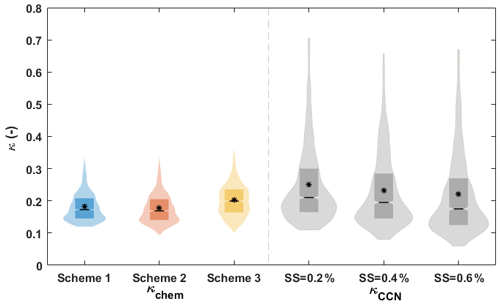
Figure 4Violin plot of κ distribution data for the chemical schemes (κchem) and the CCN calculation at different SS values (κCCN). The boxes represent the interquartile distance, and the asterisk is the mean value. The shaded area for each variable represents the probability density function (PDF).
Figure 4 shows the violin plots of the retrieved κ values for each OA scheme, κchem, and the calculated κ values from the CCNc measurements, κCCN, at different SS. The κchem values exhibit lower variability (ranging from 0.1 to 0.35) compared to the κCCN values (from 0.06 to 0.7). The probability density function (PDF) values of κchem for schemes 1 and 2 are very similar, with a maximum around 0.14. The main difference in the data distribution between both schemes is observed at low hygroscopicity values, which have been identified as periods of higher HOA contribution (i.e., during HOA peak events), as can be observed in Fig. S5 in the Supplement. Scheme 3 exhibits a clearly different data distribution compared to schemes 1 and 2 due to the assumption of different κ values for the LO-OOA and MO-OOA factors. In general, scheme 3 results in higher κchem values (mean and median values are 0.20), since we assumed a higher hygroscopicity for the MO-OOA factor, which is the main factor controlling OA at SNS. Also, Fig. 4 shows that the data are more homogenously distributed around the mean value for scheme 3, while the distributions for schemes 1 and 2 are skewed towards lower values.
The κCCN values exhibit very different data distributions relative to the κchem values. All κCCN PDFs show a clear maximum and positive skewness with some outlier observations (higher mean than median values). This is likely due to the larger variability in the parameters used to retrieve aerosol hygroscopicity in the supersaturated regime (i.e., NCCN and PNSD via κ-Köhler theory) compared to smaller changes in chemical composition. This is particularly important in the case of SNS, since the submicron chemical composition is dominated by OA, and, despite the changes in hygroscopicity among OA constituents, the range of change in κchem is quite limited because κchem is less sensitive to temporal changes in composition. As anticipated, higher SS values result in a shift to lower values in the data distribution due to activation of less hygroscopic particles. Across all SS values, mean κCCN values are higher than κchem for the different OA schemes. However, the differences between κCCN and κchem median values are minimal. It is important to note that the κCCN accounts only for activated particles in the CCNc, whereas κchem accounts only for aerosol particles in the size range allowed by the aerodynamic lens in the ToF-ACSM. Moreover, both methods assume internally mixed particles to estimate the overall κ, which is an important limitation in the case of externally mixed particles (Wang et al., 2010; Ren et al., 2018; Kulkarni et al., 2023). In this study, we would expect that, during midday hours at SNS, the aerosol population would be more externally mixed due to the influence of NPF events and the vertical transport of particles; however, with the instrumentation and methods used here, we cannot give conclusive information about the aerosol mixing state and its impact on the different scheme's performance.
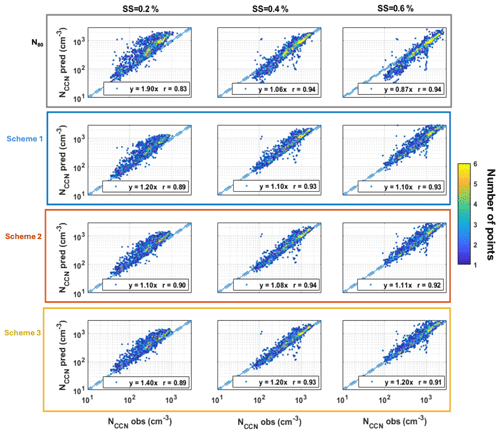
Figure 5Log–log scatter plot of predicted CCN concentrations (NCCN pred) as a function of observed CCN concentrations (NCCN obs) using the four prediction schemes. The solid blue line represents the 1:1 line, and the dashed lines are the ± 10 %. The linear equation and Pearson correlation coefficient (r) are also included.
Based on the calculated κchem values, we retrieved Dcrit at a specific SS using Eq. (3) and then estimated NCCN by integrating the PNSD using Eq. (4) with a time resolution of 30 min. In addition, we used a simpler approach to estimate NCCN from PNSD data, which consists of assuming that particles above a certain size are activated. In this case, we selected 80 nm as the fixed activation diameter, and N80 (number concentration of particles with a diameter larger than 80 nm) is used as a proxy for NCCN. This threshold diameter has been selected because, at medium SS values (0.4 %–0.5 %), the Dcrit for a wide variety of aerosol types is constrained between 70–90 nm (Rejano et al., 2023). The comparison between predicted and measured NCCN at the different SS values for the different OA schemes is shown in Fig. 5. The results show that CCN closure dependence depends on SS when the N80 approach is used. This is expected, since this simple approach does not include the Dcrit dependence on SS. In this case, the predicted NCCN values overestimate the measurements at low SS (mean Dcrit is 111 ± 21 nm) and underestimate the measurements at high SS (mean Dcrit is 58 ± 16 nm). At SS = 0.4 % the mean Dcrit is 72 ± 18 nm, and, therefore, despite the diurnal and day-to-day variability in Dcrit which might hamper the predictions using N80, the N80 proxy very accurately explains the NCCN observations at this specific SS with the best correlation coefficient (r=0.94) and slope of the regression (1.06).
For the chemical CCN closure approach (OA schemes 1–3), all the schemes overestimate the CCN observations with slope values ranging from 1.08 to 1.4 and correlation coefficients between 0.89–0.94 (Fig. 5), indicating similar CCN closure for all SS and schemes. A slightly worse agreement between predictions and observations is observed at SS = 0.2 % probably due to the higher discrepancy between κCCN and κchem at this SS, as previous studies have pointed out for low SS values (Cai et al., 2018; Mei et al., 2013). Closure results for schemes 1 and 2 are very similar, despite the observed difference in κchem values between both schemes. This similarity is due to the low contribution of HOA at SNS. For scheme 3, the results indicate that, assuming a lower/higher κ for LO-OOA/MO-OOA, respectively, rather than the standard κOA=0.1, leads to a larger overestimation of the predicted NCCN (especially at SS = 0.2). Moreover, scheme 3 results show no improvement in the correlation coefficients compared to the other OA schemes. Despite the large variability observed in the OA components, our results demonstrate that the simple approach of assuming a constant κOA of 0.1, even for a complex environment dominated by OA, seems to provide satisfactory predictions of CCN concentration.
These results agree with other CCN closures studies based on bulk chemical composition under varying assumptions of OA hygroscopicity (e.g., Kulkarni et al., 2023b; Meng et al., 2014; Ren et al., 2018b; Zhang et al., 2017b). Mei et al. (2013) obtained good CCN closures at OA-dominated conditions (70 %–80 % of PM1) assuming constant κOA values of 0.08 and 0.13 (which are very close to κOA=0.1 used in this study). Rose et al. (2011) reported NCCN overestimations of 20 % assuming κOA = 0.1 near the Guangzhou area (China), but better results (overestimation of 10 %) were observed when further assumptions about the hygroscopicity of low-volatility particles were included. Assuming κOA = 0.1 using both bulk and size-resolved chemical composition, Meng et al. (2014) showed at a coastal site in Hong Kong that NCCN overestimations reached values of 26 % and 10 %, respectively. These authors concluded that CCN closures can be less sensitive to hygroscopicity considerations and that some mixing state considerations may play a role. In contrast, Ren et al. (2018b) demonstrated in an urban environment that the aerosol mixing state plays a minor role in CCN prediction when κOA exceeds 0.1. They obtained good closure (closure ratios of 1.0–1.16) using bulk chemical and internal mixture assumptions in the Beijing urban area under clean conditions. Siegel et al. (2022) also obtained accurate NCCN closure results (slopes between 0.82–0.91) in the Arctic under internally mixed assumptions by characterizing very precisely the organic hygroscopicity based on laboratory experiments and field observations. When considering remote sites without the influence of local emissions, Cai et al. (2018) demonstrated that either bulk or size-resolved chemical composition measurements can achieve practically the same agreement in NCCN predictions. Therefore, the accuracy of NCCN predictions can exhibit a wide variety of results depending on the characteristics of the experimental site and the atmospheric conditions.
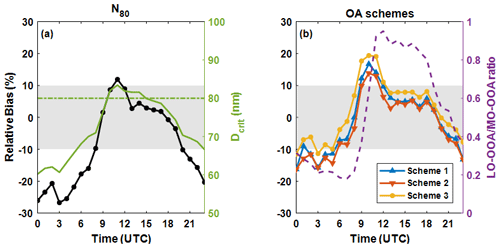
Figure 6Diurnal evolution of the median relative bias in CCN predictions at SS = 0.4 % for each prediction scheme. The gray shaded area in all panels represents the ± 10 % relative bias. The Dcrit at SS = 0.4 % is shown in panel (a), and the horizontal line represents the threshold size of 80 nm. The ratio between LO-OOA and MO-OOA mass concentrations is shown in panel (b).
To obtain a deeper understanding of the performance of CCN predictions and gain knowledge about how the differences in OA composition during the day may or may not affect the CCN predictions, we calculated the diurnal evolution of the relative bias () of the N80 approach and each OA scheme. Since the SS did not appear to cause significant differences in the estimation of the CCN among the three OA schemes, from now on, we focus the analysis at SS = 0.4 %. Figure 6 shows the median diurnal evolution of the relative bias of each scheme for SS = 0.4 %. In this analysis we consider CCN predictions to be accurate when the associated uncertainty is within the range of ± 10 % (gray shaded area in Fig. 6), which is associated with the instrument uncertainty (Schmale et al., 2017). All schemes exhibit similar diurnal patterns in relative bias with negative values during nighttime hours and positive values during midday hours. There is a clear difference between the relative bias pattern obtained by N80 and the OA schemes. Figure 6a shows both the diurnal pattern of Dcrit at 0.4 % and the threshold size of 80 nm. As expected, the difference between the observed Dcrit and the assumed threshold size (80 nm) is clearly related to the bias value, and, in general, the positive/negative bias is associated with Dcrit values larger/smaller than 80 nm. The largest deviations with respect to observations are found during nighttime hours (underestimation of between 20 %–30 %), when the Dcrit is considerably below 80 nm. Therefore, the use of this approach should be limited to situations when the Dcrit is fairly constant and restricted to a specific SS.
For the OA schemes, the diurnal evolution of the relative bias is similar to the N80 approach, with negative relative bias during the night and positive relative bias during the daytime. The relative bias ranges from −6 % to −16 % for all OA schemes during the nighttime period, which is a smaller range than observed for the Dcrit = 80 nm scheme. The nighttime period is associated with free tropospheric conditions dominated by aged aerosol (OA is dominated by MO-OOA). Conversely, during morning/midday hours, the relative bias increases from its minimum value at 06:00 UTC (3 %–8 % underestimation) to its maximum value at 10:00 UTC (14 %–20 % overestimation) (Fig. 6b). The LO-OOA / MO-OOA ratio and relative bias diurnal patterns show a similar shape (Fig. 6b) but with 1 h of delay between the maximum values for each parameter. This suggests that the largest bias occurs when the relative contribution of LO-OOA and MO-OOA starts changing. When the ratio of LO-OOA / MO-OOA is constant, the relative bias remains constant as well. These results indicate that the relative bias in CCN predictions is highly dependent on the LO-OOA and MO-OOA variability and their relative contribution to OA. Since these factors have different degrees of oxidation, in the next section we present a new OA scheme that describes κOA in terms of OA oxidation degree.
4.2.2 Parameterizing κOA in terms of OA oxidation degree using the f44 parameter
In this sub-section, we calculate κOA from Eq. (5) using the overall aerosol hygroscopicity as κCCN, κIA obtained from ToF-ACSM measurements assuming specific hygroscopicity values for each species shown in Table S1, and κBC assumed as 0 (Cerully et al., 2015; Kuang et al., 2020b; Thalman et al., 2017):
With this approach, the mean and median values of calculated κOA are 0.18 and 0.15 at SS = 0.4 %, respectively, which are values higher than the standard value of κOA=0.1 but are within the range of ambient κOA observations in the supersaturated regime (Levin et al., 2014; Gunthe et al., 2011; Kawana et al., 2016). The inferred values of κOA confirm the predominance of MO-OOA species in the activated particles at SS = 0.4 %, since it is very close to the assumed value of 0.16 for κMO-OOA in OA scheme 3 (Table 1). Figure S6 in the Supplement shows the probability density function (PDF) for the effective κOA at SS = 0.4 % retrieved with this method. The PDF distribution shows its maximum at κOA=0.11, which is similar to the assumed κOA (∼ 0.1) for scheme 1. The PDF also exhibits a clear positive skewness revealing the influence of more hygroscopic species at SS = 0.4 %
Previous studies parameterized κOA as a function of the oxidation degree using the f44 parameter (Kuang et al., 2020a). Therefore, we explore a potential improvement in the κOA calculation at SNS by establishing a new OA scheme (named here as scheme 4) based on a linear relationship between κOA and f44. This enables calculation of the κchem as follows:
where m and n are the slope and the intercept of the linear relationship between κOA and f44. To establish the parameterization, the dataset is split randomly in two subsets: the first is used to obtain the linear regression, and the second is used to check its performance for CCN calculation. Each data subset consists of 50 % of the data. In the first subset of data, we re-sampled the f44 values into 80 bins and calculated the corresponding average κOA values for each f44 bin. Then, the empirical parameterization was obtained by establishing a linear regression between the averaged κOA values and f44. As shown in Fig. 7, there is a clear linear trend between the binned values of κOA and f44. For high values of f44 (especially above 0.26), the κOA values exhibit higher dispersion. The κOA and f44 relationship obtained in this analysis (slope of 3.24) is for 0.2 < f44 < 0.32. These high values of f44 are due to the high oxidation degree of OA and the low contribution of HOA at this site. Previous studies that reported a linear relationship between κOA and f44 were developed for less-oxidized aerosol with f44 values ranging from 0.05 to 0.20 (Duplissy et al., 2011; Kuang et al., 2020b; Chen et al., 2017; Mei et al., 2013). Those studies also reported lower slopes for the κOA–f44 relationship, ranging between 2.1–2.4. Like the SNS analysis being reported on here, these studies from the literature were performed at OA-dominated sites during the warm season. However, these other sites observed lower f44 values due to a higher contribution of HOA and biomass-burning-related OA (Duplissy et al., 2011; Mei et al., 2013; Chang et al., 2010). For freshly emitted biomass burning particles, Chen et al. (2017) also obtained a lower slope value of 2.3 associated with low f44 values (f44 < 0.1). A significantly lower slope for the κOA–f44 relationship (1.04) was reported by Kuang et al. (2020a) for measurements on the North China Plain during winter where the aerosol composition was dominated by a higher contribution of HOA and coal combustion OA (f44 < 0.15). In contrast to these values reported in the literature, the κOA–f44 relationship retrieved in our study is for higher values of f44 and exhibits the largest slope. Our results therefore suggest that the fit to the κOA–f44 relationship depends on the oxygenation degree of the organic particles. However, some of the variability observed among studies in the κOA–f44 relationship might arise from differences in κOA calculation, such as the value of SS used and/or whether bulk or size-resolved measurements were available.
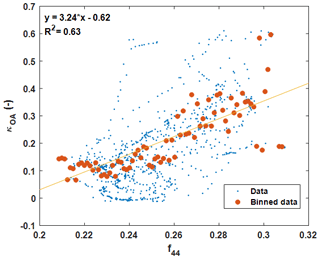
Figure 7Scatter plot of κOA at SS = 0.4 % with respect to f44. The linear regression is applied to the binned data.
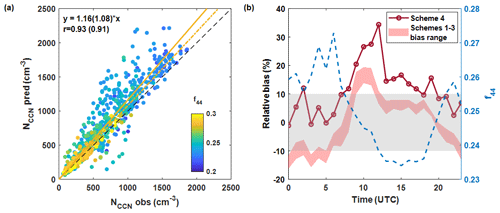
Figure 8(a) Scatter plot of predicted CCN concentrations (NCCN pred) as a function of observed CCN concentrations (NCCN obs) using OA scheme 4. Data points are color-coded by the corresponding f44 value. The dashed black line represents the 1:1 line. The linear equation and Pearson correlation coefficient (r) are also included for all data and for filtered data in parentheses (data points with f44 > 0.25). The solid and dashed yellow lines represent the linear regression of all data and filtered data, respectively. (b) Median diurnal evolution of the relative bias at SS = 0.4 % of OA scheme 4 (left y axis) and f44 (right y axis). The gray shaded area represents the ± 10 % relative bias. The red shaded area represents the relative bias range for the other OA schemes shown in Fig. 6b.
After applying scheme 4 to the other half of the dataset, the CCN closure at SS = 0.4 % exhibits a similar slope and correlation coefficient (1.13 and 0.93, respectively; see Fig. 8a) to the three other OA schemes. For high f44 values (> 0.25) the CCN closure is better (slope of 1.05), while for low f44 values (< 0.25) the CCN predictions tend to overestimate the CCN concentrations and exhibit higher data dispersion. This is also observed in the median diurnal pattern of the relative bias (Fig. 8b). During the night (when high f44 values are observed), the new OA scheme can explain the observations within the ± 10 % range and improves the CCN closure relative to the previous OA schemes. However, the relative bias increases up to 35 % (Fig. 8b) during morning and midday hours when the aerosol is characterized by lower f44 values associated with higher LO-OOA contribution. The sensitivity of κOA to changes in f44 is highly dependent on aerosol sources and atmospheric conditions, and significant deviations have been observed depending on the site (Kuang et al., 2020a). Our results are comparable with those of Zhang et al., (2016). They analyzed the impact of aerosol oxidation level on CCN predictions at a suburban site in northern China using the κOA–f44 relation presented by Mei et al. (2013). They showed that, for OA mass fractions higher than 0.6, the NCCN predictions are very sensitive to f44 values, and the best CCN closures were observed for f44 > 0.15 with a slope value around 0.94 at SS = 0.39 %. As observed in this study and in Zhang et al. (2016), accurate NCCN predictions at OA-dominated sites using the κOA–f44 relation are challenging, since both κOA and NCCN are very sensitive to f44 values.
To sum up, the new κOA calculation using f44 parameterization shows good agreement between CCN calculations and observations during the night (bias ranging between 0 %–10 % from 21:00 to 06:00 UTC); however, it results in worse predictions during morning and midday hours at SNS. After verifying that all OA schemes for calculating κOA yield nearly identical results, with the most significant biases occurring under conditions influenced by daytime vertical upslope transport of particles and/or NPF events, we conclude that using a bulk κchem to predict NCCN consistently results in discrepancies with observations. The clear diurnal variability in aerosol properties and atmospheric conditions may require size-resolved chemical composition or mixing state assumptions for the aerosol population, like externally mixed aerosol or even a combination of aerosol populations (Kulkarni et al., 2023; Zhang et al., 2017; Ren et al., 2018), to improve the results throughout the day. However, the analytical methods used in this study have limitations and do not consider aerosol mixing state information. For that reason, the next section will explore a non-analytical approach with the aim of improving the CCN prediction throughout the day.
4.2.3 Non-analytical approach for CCN prediction: neural networks
In this section, we develop a prediction scheme based on a neural network that uses four input parameters (N80, , f44, and solar global irradiance) to account for the main features affecting the CCN concentration at SNS, respectively: aerosol concentration in the CCN-active size range, OA contribution to total PM1 and its oxygenation degree (related to OA hygroscopicity), and insolation conditions that might affect secondary processes influenced by photochemistry. More details on the neural network architecture are given in Sect. 3.4.
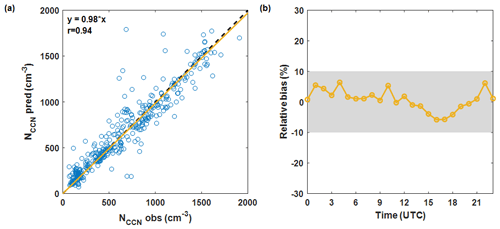
Figure 9(a) Scatter plot of predicted CCN concentrations (NCCN pred) as a function of observed CCN concentrations (NCCN obs) for the testing sub-dataset (25 % of the whole dataset) at SS = 0.4 % using the neural network approach. The linear regression and Pearson correlation coefficient (r) are also included. (b) Median diurnal evolution of the relative bias at SS = 0.4 % of the neural network model. Also, the gray shaded area represents the ± 10 % relative bias.
Figure 9 shows the performance of the neural network approach for CCN estimation at SS = 0.4 % (slope of 0.98 and correlation coefficient of 0.94). This neural network approach shows the best correlation with observations in this study. Compared with the analytical approaches using bulk chemical composition measurements, this model shows a general underestimation of measurements (slope < 1) contrasting with the overestimation obtained for all OA scheme approaches (all slopes > 1). In terms of capturing the diurnal variability, this new approach performs better, since the median diurnal pattern remains within the ± 10 % range (Fig. 9b). The neural network can describe NCCN variability throughout the day, even during morning and midday hours, when all four OA schemes exhibited the highest bias values. The neural network scheme also explains the CCN variability during the most complex aerosol conditions at SNS. The inclusion of the solar irradiance in the neural network model acts as a proxy of photochemical activity and secondary process influence which helps to diminish the overestimation peak observed for all OA schemes during the midday hours.
This newly developed model is able to manage the non-linear and time-dependent relationships between variables during the hours when aerosol population might be an external mixture of background particles, upslope transport particles, and/or particles produced during NPF events, suggesting it is a suitable approach for CCN prediction throughout the day. Park et al. (2023) also proposed machine learning approaches to develop CCN predictions based on multiple linear regression and non-negative matrix factorization techniques. They concluded that these methods are robust and capable of simulating either internal or external mixing conditions. However, the CCN predictions observed in our study are more accurate (r=0.94) than the results observed by Park et al. (2023) (R2 between 0.71–0.81). This might be due to the input parameters considered in the neural network, since Park et al. (2023) only considered aerosol size distribution measurements without any consideration of chemical composition or hygroscopicity. Nair et al. (2021) used a random forest regression model and also reported strong agreement between CCN estimations and observations during an aircraft campaign. They used model-simulated data of aerosol composition, atmospheric trace gases, and meteorological variables without aerosol size information to estimate NCCN at SS = 0.4 %, finding a Kendall correlation coefficient of 0.76.
Our analysis, along with results in the previous literature, indicates that machine learning approaches are very useful for accurately predicting NCCN under different conditions in terms of other aerosol properties. Furthermore, this suggests that CCN coverage can be improved worldwide by using machine learning and making use of more routinely measured parameters such as aerosol size distribution, OA properties, and solar global irradiance. However, further studies assessing the potential of these tools at multiple sites and during long time scales are still necessary.
We analyzed the influence of κOA on CCN estimations from bulk chemical composition measurements using different OA schemes to describe the overall aerosol hygroscopicity. We investigated the physicochemical properties and CCN activity of the aerosol population at a high-altitude mountain site (SNS station) on the southeastern Iberian Peninsula, where atmospheric conditions can allow cloud formation.
Our results show the important contribution of OA to the total PM1 mass concentration at SNS, where it represents up to the 70 % of the PM1. After applying PMF analysis, we determined that MO-OOA and LO-OOA are the main factors that control both OA and total PM1. During nighttime hours, aerosol particles are more aged and hygroscopic, with a predominant contribution of MO-OOA and inorganic species. During the morning (06:00–10:00 UTC), the aerosol population starts to be affected by orographic buoyant upward flows of aerosol from the urban area due to the mountain–valley breeze regime and PBL influence. During this time, LO-OOA and eBC make a higher relative contribution to the aerosol population, resulting in a decrease in the overall hygroscopicity. The aerosol population properties continue to change during midday hours (11:00–16:00 UTC, highest insolation hours), when the LO-OOA factor and nucleation mode particles exhibit the highest concentration of the day. This is likely caused by SOA formation through photochemical reactions during NPF events, in combination with other sources such as upslope transport.
The CCN concentration has been estimated by using different OA hygroscopicity schemes based on bulk chemical composition. CCN closure for all OA schemes exhibited slopes and correlation coefficients in the range between 1.08–1.40 and 0.89–0.94, respectively. We find that using a fixed size threshold for CCN activation exhibited a very pronounced diurnal pattern. All OA schemes investigated resulted in similar CCN closure statistics. OA schemes performed better at night (bias between −16 % and −6 %), when the OA is more oxidized and the aerosol is more aged, than during the day, when the OA is less oxidized and the aerosol is more influenced by photochemical and boundary layer processes (bias from 0 % to 20 %). We also propose a new OA scheme based on the f44 parameter, which reflects the oxidation degree of the OA and results in similar overall values to the other OA schemes for the closure slope and correlation coefficient (1.13 and 0.93, respectively). The new OA scheme did improve the closure results for more aged aerosol (f44 > 0.25), which is measured at night but not during the day (bias values up to 40 %), when the aerosol is more complex and f44 values are lower. These findings indicate that factors beyond the bulk κOA characterization must be considered when the aerosol is more complex.
We attribute the observed positive bias of all OA schemes to two main causes. Firstly, the ToF-ACSM provides information on a limited aerosol size range often dominated by accumulation particles which is more affected by inorganic species (Meng et al., 2014; Che et al., 2016, 2017); therefore, the real κchem of the whole aerosol population might be overestimated by κchem measured with the ToF-ACSM. In addition, we must consider the effect of the well-known differences in the size ranges considered between the different instruments in this field campaign: CCNc, with no size cutoff; SMPS, with a mobility diameter range of 12–535 nm; and ToF-ACSM, with an aerodynamic diameter range of 70–700 nm (associated with a mobility diameter range of 58–578 nm using an effective aerosol density of 1.47 g cm−3 and a shape factor of 1). Secondly, when the aerosol population consists of a complex mixture of particles, which at SNS can be observed during PBL influence conditions, the underlying assumptions for estimating CCN predictions based on internally mixed aerosol particles can introduce an intrinsic bias, and κOA assumptions have a secondary role. Since the methodology used here is limited and cannot directly infer the mixing state of the aerosol population, we are not able to quantify this effect on the CCN predictions. Moreover, during morning and midday hours related to more complex conditions, the relationship between variables might change over time and can have a non-linear nature. Therefore, the analytical model approaches presented here cannot explain the CCN changes throughout the day. The big takeaway is that the complexity of the aerosol should be considered when using bulk chemical composition measurements to predict CCN concentrations worldwide.
For that reason, we built a neural network approach which is able to predict CCN concentrations throughout the day. Using four input parameters for the neural network (N80, , f44, and solar global irradiance), we were able to predict NCCN accurately in all conditions throughout the day (within ± 10 % relative bias), revealing that this approach was the best for CCN predictions at this complex remote site. It is important to note that the disadvantage of predicting atmospheric parameters using neural networks is that the model is a “black box” which is trained with data of a specific site and can only forecast in that specific site or similar locations. Despite this, it may be possible to use neural networks to improve our understanding of global CCN coverage using few aerosol parameters without needing to consider details of aerosol complexity such as mixing state.
The data used in this article are available from the first author at frejano@ugr.es.
The supplement related to this article is available online at: https://doi.org/10.5194/acp-24-13865-2024-supplement.
FR performed the data harmonization, treatment, and formal analysis and wrote the article. AC, MV, JACV, FJO, and GT carried out the conceptualization and investigation, and, together with HL and EA, they carried out a thorough proofreading of the article before obtaining the final version. AA, DP, and LA assisted in the conceptualization. AC, SC, FJG, and GT provided the experimental datasets. All authors contributed to the discussion of the results and provided comments on the paper.
The contact author has declared that none of the authors has any competing interests.
Publisher's note: Copernicus Publications remains neutral with regard to jurisdictional claims made in the text, published maps, institutional affiliations, or any other geographical representation in this paper. While Copernicus Publications makes every effort to include appropriate place names, the final responsibility lies with the authors.
This work has been supported by University of Granada Plan Propio through the Visiting Scholars (PPVS2018-04) and Singular Laboratory (AGORA, LS2022-1) programs. Fernando Rejano acknowledges support from an FPU grant (FPU19/05340, Ministerio de Universidades). Elisabeth Andrews acknowledges support from NOAA cooperative agreement NA22OAR4320151.
This research was funded by the Spanish Ministry of Science and Innovation through projects NUCLEUS (grant no. PID2021-128757OB-I00) funded by MICIU/AEI/10.13039/501100011033, and ERDF – “A way of making Europe”, BioCloud (grant no. RTI2018.101154.A.I00) funded by MCIN/AEI/10.13039/501100011033 from ERDF – “A way of making Europe”, ELPIS (grant no. PID2020-120015RB-100) funded by MCIN/AEI/10.13039/501100011033, and ACTRIS-España RED2022-134824-E. Also, this research has received support from the European Union's Horizon 2020 research and innovation program through projects ACTRIS.IMP (grant no. 871115) and ATMO_ACCESS (grant no. 101008004). Andrea Casans is funded by the Spanish Ministry of Science and Innovation under the predoctoral program FPI (grant no. PRE2019-090827) funded by MCIN/AEI/10.13039/501100011033, FSE – “El FSE invierte en tu futuro”.
This paper was edited by Eija Asmi and reviewed by three anonymous referees.
Albrecht, B. A.: Aerosols, cloud microphysics, and fractional cloudiness, Science, 245, 1227–30, https://doi.org/10.1126/science.245.4923.1227, 1989.
Andrews, E., Sheridan, P. J. P. J., Ogren, J. A. J. A., Hageman, D., Jefferson, A., Wendell, J., Alástuey, A., Alados-Arboledas, L., Bergin, M., Ealo, M., Sorribas, M., Sun, J., Gannet Hallar, A., Hoffer, A., Kalapov, I., Keywood, M., Kim, J., Kim, S. W., Kolonjari, F., Labuschagne, C., Lin, N. H., Macdonald, A., Mayol-Bracero, O. L., McCubbin, I. B., Pandolfi, M., Reisen, F., Sharma, S., Sherman, J. P., Sorribas, M., and Sun, J.: Overview of the NOAA/ESRL federated aerosol network, B. Am. Meteor. Soc., 100, 123–135, https://doi.org/10.1175/BAMS-D-17-0175.1, 2019.
Asmi, A., Wiedensohler, A., Laj, P., Fjaeraa, A.-M., Sellegri, K., Birmili, W., Weingartner, E., Baltensperger, U., Zdimal, V., Zikova, N., Putaud, J.-P., Marinoni, A., Tunved, P., Hansson, H.-C., Fiebig, M., Kivekäs, N., Lihavainen, H., Asmi, E., Ulevicius, V., Aalto, P. P., Swietlicki, E., Kristensson, A., Mihalopoulos, N., Kalivitis, N., Kalapov, I., Kiss, G., de Leeuw, G., Henzing, B., Harrison, R. M., Beddows, D., O'Dowd, C., Jennings, S. G., Flentje, H., Weinhold, K., Meinhardt, F., Ries, L., and Kulmala, M.: Number size distributions and seasonality of submicron particles in Europe 2008–2009, Atmos. Chem. Phys., 11, 5505–5538, https://doi.org/10.5194/acp-11-5505-2011, 2011.
Asmi, E., Freney, E., Hervo, M., Picard, D., Rose, C., Colomb, A., and Sellegri, K.: Aerosol cloud activation in summer and winter at puy-de-Dôme high altitude site in France, Atmos. Chem. Phys., 12, 11589–11607, https://doi.org/10.5194/acp-12-11589-2012, 2012.
Baron, P. A. and Willeke, K.: Aerosol fundamentals, Aerosol measurement principles, techniques, and applications, 2nd edn., Wiley-Blackwell, ISBN 10-0471784923, 2005.
Biancofiore, F., Busilacchio, M., Verdecchia, M., Tomassetti, B., Aruffo, E., Bianco, S., Di Tommaso, S., Colangeli, C., Rosatelli, G., and Di Carlo, P.: Recursive neural network model for analysis and forecast of PM10 and PM2.5, Atmos. Pollut. Res., 8, 652–659, https://doi.org/10.1016/j.apr.2016.12.014, 2017.
Bougiatioti, A., Fountoukis, C., Kalivitis, N., Pandis, S. N., Nenes, A., and Mihalopoulos, N.: Cloud condensation nuclei measurements in the marine boundary layer of the Eastern Mediterranean: CCN closure and droplet growth kinetics, Atmos. Chem. Phys., 9, 7053–7066, https://doi.org/10.5194/acp-9-7053-2009, 2009.
Bougiatioti, A., Bezantakos, S., Stavroulas, I., Kalivitis, N., Kokkalis, P., Biskos, G., Mihalopoulos, N., Papayannis, A., and Nenes, A.: Biomass-burning impact on CCN number, hygroscopicity and cloud formation during summertime in the eastern Mediterranean, Atmos. Chem. Phys., 16, 7389–7409, https://doi.org/10.5194/acp-16-7389-2016, 2016.
Brown, S. G., Lee, T., Norris, G. A., Roberts, P. T., Collett Jr., J. L., Paatero, P., and Worsnop, D. R.: Receptor modeling of near-roadway aerosol mass spectrometer data in Las Vegas, Nevada, with EPA PMF, Atmos. Chem. Phys., 12, 309–325, https://doi.org/10.5194/acp-12-309-2012, 2012.
Cai, M., Tan, H., Chan, C. K., Qin, Y., Xu, H., Li, F., Schurman, M. I., Liu, L., and Zhao, J.: The size-resolved cloud condensation nuclei (CCN) activity and its prediction based on aerosol hygroscopicity and composition in the Pearl Delta River (PRD) region during wintertime 2014, Atmos. Chem. Phys., 18, 16419–16437, https://doi.org/10.5194/acp-18-16419-2018, 2018.
Cai, M., Huang, S., Liang, B., Sun, Q., Liu, L., Yuan, B., Shao, M., Hu, W., Chen, W., Song, Q., Li, W., Peng, Y., Wang, Z., Chen, D., Tan, H., Xu, H., Li, F., Deng, X., Deng, T., Sun, J., and Zhao, J.: Measurement report: Distinct size dependence and diurnal variation in organic aerosol hygroscopicity, volatility, and cloud condensation nuclei activity at a rural site in the Pearl River Delta (PRD) region, China, Atmos. Chem. Phys., 22, 8117–8136, https://doi.org/10.5194/acp-22-8117-2022, 2022.
Canagaratna, M. R., Onasch, T. B., Wood, E. C., Herndon, S. C., Jayne, J. T., Cross, E. S., Miake-Lye, R. C., Kolb, C. E., and Worsnop, D. R.: Evolution of Vehicle Exhaust Particles in the Atmosphere, J. Air Waste Manage., 60, 1192–1203, https://doi.org/10.3155/1047-3289.60.10.1192, 2010.
Canonaco, F., Crippa, M., Slowik, J. G., Baltensperger, U., and Prévôt, A. S. H.: SoFi, an IGOR-based interface for the efficient use of the generalized multilinear engine (ME-2) for the source apportionment: ME-2 application to aerosol mass spectrometer data, Atmos. Meas. Tech., 6, 3649–3661, https://doi.org/10.5194/amt-6-3649-2013, 2013.
Cappa, C. D., Che, D. L., Kessler, S. H., Kroll, J. H., and Wilson, K. R.: Variations in organic aerosol optical and hygroscopic properties upon heterogeneous OH oxidation, J. Geophys. Res.-Atmos., 116, D15204, https://doi.org/10.1029/2011JD015918, 2011.
Casans, A., Rejano, F., Maldonado-Valderrama, J., Casquero-Vera, J. A., Ruiz-Peñuela, S., van Drooge, B. L., Lyamani, H., Cazorla, A., Andrews, E., Lin, J. J., Mirza-Montoro, F., Pérez-Ramírez, D., Olmo, F. J., Alados-Arboledas, L., Cariñanos, P., and Titos, G.: Cloud condensation nuclei activation properties of Mediterranean pollen types considering organic chemical composition and surface tension effects, Atmos. Environ., 310, 119961, https://doi.org/10.1016/j.atmosenv.2023.119961, 2023.
Casquero-Vera, J. A., Lyamani, H., Dada, L., Hakala, S., Paasonen, P., Román, R., Fraile, R., Petäjä, T., Olmo-Reyes, F. J., and Alados-Arboledas, L.: New particle formation at urban and high-altitude remote sites in the south-eastern Iberian Peninsula, Atmos. Chem. Phys., 20, 14253–14271, https://doi.org/10.5194/acp-20-14253-2020, 2020.
Casquero-Vera, J. A., Lyamani, H., Titos, G., Minguillón, M. C., Dada, L., Alastuey, A., Querol, X., Petäjä, T., Olmo, F. J., and Alados-Arboledas, L.: Quantifying traffic, biomass burning and secondary source contributions to atmospheric particle number concentrations at urban and suburban sites, Sci. Total Environ., 768, 145282, https://doi.org/10.1016/j.scitotenv.2021.145282, 2021.
Casquero-Vera, J. A., Pérez-Ramírez, D., Lyamani, H., Rejano, F., Casans, A., Titos, G., Olmo, F. J., Dada, L., Hakala, S., Hussein, T., Lehtipalo, K., Paasonen, P., Hyvärinen, A., Pérez, N., Querol, X., Rodríguez, S., Kalivitis, N., González, Y., Alghamdi, M. A., Kerminen, V.-M., Alastuey, A., Petäjä, T., and Alados-Arboledas, L.: Impact of desert dust on new particle formation events and the cloud condensation nuclei budget in dust-influenced areas, Atmos. Chem. Phys., 23, 15795–15814, https://doi.org/10.5194/acp-23-15795-2023, 2023.
Cerully, K. M., Bougiatioti, A., Hite Jr., J. R., Guo, H., Xu, L., Ng, N. L., Weber, R., and Nenes, A.: On the link between hygroscopicity, volatility, and oxidation state of ambient and water-soluble aerosols in the southeastern United States, Atmos. Chem. Phys., 15, 8679–8694, https://doi.org/10.5194/acp-15-8679-2015, 2015.
Chang, R. Y.-W., Slowik, J. G., Shantz, N. C., Vlasenko, A., Liggio, J., Sjostedt, S. J., Leaitch, W. R., and Abbatt, J. P. D.: The hygroscopicity parameter (κ) of ambient organic aerosol at a field site subject to biogenic and anthropogenic influences: relationship to degree of aerosol oxidation, Atmos. Chem. Phys., 10, 5047–5064, https://doi.org/10.5194/acp-10-5047-2010, 2010.
Che, H. C., Zhang, X. Y., Wang, Y. Q., Zhang, L., Shen, X. J., Zhang, Y. M., Ma, Q. L., Sun, J. Y., Zhang, Y. W., and Wang, T. T.: Characterization and parameterization of aerosol cloud condensation nuclei activation under different pollution conditions, Sci. Rep., 6, 24497, https://doi.org/10.1038/srep24497, 2016.
Che, H. C., Zhang, X. Y., Zhang, L., Wang, Y. Q., Zhang, Y. M., Shen, X. J., Ma, Q. L., Sun, J. Y., and Zhong, J. T.: Prediction of size-resolved number concentration of cloud condensation nuclei and long-term measurements of their activation characteristics, Sci. Rep., 7, 5819, https://doi.org/10.1038/s41598-017-05998-3, 2017.
Chen, J., Budisulistiorini, S. H., Itoh, M., Lee, W.-C., Miyakawa, T., Komazaki, Y., Yang, L. D. Q., and Kuwata, M.: Water uptake by fresh Indonesian peat burning particles is limited by water-soluble organic matter, Atmos. Chem. Phys., 17, 11591–11604, https://doi.org/10.5194/acp-17-11591-2017, 2017.
Cheung, H. C., Chou, C. C.-K., Lee, C. S. L., Kuo, W.-C., and Chang, S.-C.: Hygroscopic properties and cloud condensation nuclei activity of atmospheric aerosols under the influences of Asian continental outflow and new particle formation at a coastal site in eastern Asia, Atmos. Chem. Phys., 20, 5911–5922, https://doi.org/10.5194/acp-20-5911-2020, 2020.
Comrie, A. C.: Comparing Neural Networks and Regression Models for Ozone Forecasting, J. Air Waste Manage., 47, 653–663, https://doi.org/10.1080/10473289.1997.10463925, 1997.
Crippa, M., DeCarlo, P. F., Slowik, J. G., Mohr, C., Heringa, M. F., Chirico, R., Poulain, L., Freutel, F., Sciare, J., Cozic, J., Di Marco, C. F., Elsasser, M., Nicolas, J. B., Marchand, N., Abidi, E., Wiedensohler, A., Drewnick, F., Schneider, J., Borrmann, S., Nemitz, E., Zimmermann, R., Jaffrezo, J.-L., Prévôt, A. S. H., and Baltensperger, U.: Wintertime aerosol chemical composition and source apportionment of the organic fraction in the metropolitan area of Paris, Atmos. Chem. Phys., 13, 961–981, https://doi.org/10.5194/acp-13-961-2013, 2013.
Crosbie, E., Youn, J.-S., Balch, B., Wonaschütz, A., Shingler, T., Wang, Z., Conant, W. C., Betterton, E. A., and Sorooshian, A.: On the competition among aerosol number, size and composition in predicting CCN variability: a multi-annual field study in an urbanized desert, Atmos. Chem. Phys., 15, 6943–6958, https://doi.org/10.5194/acp-15-6943-2015, 2015.
Cubison, M. J., Ervens, B., Feingold, G., Docherty, K. S., Ulbrich, I. M., Shields, L., Prather, K., Hering, S., and Jimenez, J. L.: The influence of chemical composition and mixing state of Los Angeles urban aerosol on CCN number and cloud properties, Atmos. Chem. Phys., 8, 5649–5667, https://doi.org/10.5194/acp-8-5649-2008, 2008.
de Arruda Moreira, G., Guerrero-Rascado, J. L., Benavent-Oltra, J. A., Ortiz-Amezcua, P., Román, R., E. Bedoya-Velásquez, A., Bravo-Aranda, J. A., Olmo Reyes, F. J., Landulfo, E., and Alados-Arboledas, L.: Analyzing the turbulent planetary boundary layer by remote sensing systems: the Doppler wind lidar, aerosol elastic lidar and microwave radiometer, Atmos. Chem. Phys., 19, 1263–1280, https://doi.org/10.5194/acp-19-1263-2019, 2019.
Deng, Y., Kagami, S., Ogawa, S., Kawana, K., Nakayama, T., Kubodera, R., Adachi, K., Hussein, T., Miyazaki, Y., and Mochida, M.: Hygroscopicity of Organic Aerosols and Their Contributions to CCN Concentrations Over a Midlatitude Forest in Japan, J. Geophys. Res.-Atmos., 123, 9703–9723, https://doi.org/10.1029/2017JD027292, 2018.
Deng, Y., Yai, H., Fujinari, H., Kawana, K., Nakayama, T., and Mochida, M.: Diurnal variation and size dependence of the hygroscopicity of organic aerosol at a forest site in Wakayama, Japan: their relationship to CCN concentrations, Atmos. Chem. Phys., 19, 5889–5903, https://doi.org/10.5194/acp-19-5889-2019, 2019.
Duan, J., Chen, Y., Zhang, X., Wang, W., Zhong, S., Li, J., Lu, G., Fang, C., Guo, L., and Fu, P.: Influence of aerosol physicochemical properties on CCN activation during the Asian winter monsoon at the summit of Mt. Lu, China, Atmos. Environ., 296, 119592, https://doi.org/10.1016/j.atmosenv.2023.119592, 2023.
Duplissy, J., DeCarlo, P. F., Dommen, J., Alfarra, M. R., Metzger, A., Barmpadimos, I., Prevot, A. S. H., Weingartner, E., Tritscher, T., Gysel, M., Aiken, A. C., Jimenez, J. L., Canagaratna, M. R., Worsnop, D. R., Collins, D. R., Tomlinson, J., and Baltensperger, U.: Relating hygroscopicity and composition of organic aerosol particulate matter, Atmos. Chem. Phys., 11, 1155–1165, https://doi.org/10.5194/acp-11-1155-2011, 2011.
Dusek, U., Frank, G. P., Hildebrandt, L., Curtius, J., Schneider, J., Walter, S., Chand, D., Drewnick, F., Hings, S., Jung, D., Borrmann, S., and Andreae, M. O.: Size matters more than chemistry for cloud-nucleating ability of aerosol particles, Science, 312, 1375–1378, https://doi.org/10.1126/science.1125261, 2006.
Ervens, B., Cubison, M. J., Andrews, E., Feingold, G., Ogren, J. A., Jimenez, J. L., Quinn, P. K., Bates, T. S., Wang, J., Zhang, Q., Coe, H., Flynn, M., and Allan, J. D.: CCN predictions using simplified assumptions of organic aerosol composition and mixing state: a synthesis from six different locations, Atmos. Chem. Phys., 10, 4795–4807, https://doi.org/10.5194/acp-10-4795-2010, 2010.
Foresee, F. D. and Hagan, M. T.: Gauss-Newton approximation to Bayesian learning, Proceedings of International Conference on Neural Networks (ICNN'97), 9–12 June 1997, Houston, Texas, USA, vol. 3, 3, 1930–1935, 1997.
Forster, P., Storelvmo, T., Armour, K., Collins, W., Dufresne, J.-L., Frame, D., Lunt, D. J., Mauritsen, T., Palmer, M. D., Watanabe, M., Wild, M., and Zhang, H.: The Earth's Energy Budget, Climate Feedbacks, and Climate Sensitivity, in: Climate Change 2021: The Physical Science Basis. Contribution of Working Group I to the Sixth Assessment Report of the Intergovernmental Panel on Climate Change, edited by: Masson-Delmotte, V., Zhai, P., Pirani, A., Connors, S. L., Péan, C., Berger, S., Caud, N., Chen, Y., Goldfarb, L., Gomis, M. I., Huang, M., Leitzell, K., Lonnoy, E., Matthews, J. B. R., Maycock, T. K., Waterfield, T., Yelekçi, O., Yu, R., and Zhou, B., Cambridge University Press, Cambridge, United Kingdom and New York, NY, USA, 923–1054, https://doi.org/10.1017/9781009157896.009, 2021.
Friedman, B., Zelenyuk, A., Beranek, J., Kulkarni, G., Pekour, M., Gannet Hallar, A., McCubbin, I. B., Thornton, J. A., and Cziczo, D. J.: Aerosol measurements at a high-elevation site: composition, size, and cloud condensation nuclei activity, Atmos. Chem. Phys., 13, 11839–11851, https://doi.org/10.5194/acp-13-11839-2013, 2013.
Fröhlich, R., Cubison, M. J., Slowik, J. G., Bukowiecki, N., Prévôt, A. S. H., Baltensperger, U., Schneider, J., Kimmel, J. R., Gonin, M., Rohner, U., Worsnop, D. R., and Jayne, J. T.: The ToF-ACSM: a portable aerosol chemical speciation monitor with TOFMS detection, Atmos. Meas. Tech., 6, 3225–3241, https://doi.org/10.5194/amt-6-3225-2013, 2013.
Fröhlich, R., Crenn, V., Setyan, A., Belis, C. A., Canonaco, F., Favez, O., Riffault, V., Slowik, J. G., Aas, W., Aijälä, M., Alastuey, A., Artiñano, B., Bonnaire, N., Bozzetti, C., Bressi, M., Carbone, C., Coz, E., Croteau, P. L., Cubison, M. J., Esser-Gietl, J. K., Green, D. C., Gros, V., Heikkinen, L., Herrmann, H., Jayne, J. T., Lunder, C. R., Minguillón, M. C., Močnik, G., O'Dowd, C. D., Ovadnevaite, J., Petralia, E., Poulain, L., Priestman, M., Ripoll, A., Sarda-Estève, R., Wiedensohler, A., Baltensperger, U., Sciare, J., and Prévôt, A. S. H.: ACTRIS ACSM intercomparison – Part 2: Intercomparison of ME-2 organic source apportionment results from 15 individual, co-located aerosol mass spectrometers, Atmos. Meas. Tech., 8, 2555–2576, https://doi.org/10.5194/amt-8-2555-2015, 2015.
Georgakaki, P., Bougiatioti, A., Wieder, J., Mignani, C., Ramelli, F., Kanji, Z. A., Henneberger, J., Hervo, M., Berne, A., Lohmann, U., and Nenes, A.: On the drivers of droplet variability in alpine mixed-phase clouds, Atmos. Chem. Phys., 21, 10993–11012, https://doi.org/10.5194/acp-21-10993-2021, 2021.
Gramsch, E., Muñoz, A., Langner, J., Morales, L., Soto, C., Pérez, P., and Rubio, M. A.: Black carbon transport between Santiago de Chile and glaciers in the Andes Mountains, Atmos. Environ., 232, 117546, https://doi.org/10.1016/j.atmosenv.2020.117546, 2020.
Gunthe, S. S., King, S. M., Rose, D., Chen, Q., Roldin, P., Farmer, D. K., Jimenez, J. L., Artaxo, P., Andreae, M. O., Martin, S. T., and Pöschl, U.: Cloud condensation nuclei in pristine tropical rainforest air of Amazonia: size-resolved measurements and modeling of atmospheric aerosol composition and CCN activity, Atmos. Chem. Phys., 9, 7551–7575, https://doi.org/10.5194/acp-9-7551-2009, 2009.
Gunthe, S. S., Rose, D., Su, H., Garland, R. M., Achtert, P., Nowak, A., Wiedensohler, A., Kuwata, M., Takegawa, N., Kondo, Y., Hu, M., Shao, M., Zhu, T., Andreae, M. O., and Pöschl, U.: Cloud condensation nuclei (CCN) from fresh and aged air pollution in the megacity region of Beijing, Atmos. Chem. Phys., 11, 11023–11039, https://doi.org/10.5194/acp-11-11023-2011, 2011.
Gysel, M., Crosier, J., Topping, D. O., Whitehead, J. D., Bower, K. N., Cubison, M. J., Williams, P. I., Flynn, M. J., McFiggans, G. B., and Coe, H.: Closure study between chemical composition and hygroscopic growth of aerosol particles during TORCH2, Atmos. Chem. Phys., 7, 6131–6144, https://doi.org/10.5194/acp-7-6131-2007, 2007.
Hallquist, M., Wenger, J. C., Baltensperger, U., Rudich, Y., Simpson, D., Claeys, M., Dommen, J., Donahue, N. M., George, C., Goldstein, A. H., Hamilton, J. F., Herrmann, H., Hoffmann, T., Iinuma, Y., Jang, M., Jenkin, M. E., Jimenez, J. L., Kiendler-Scharr, A., Maenhaut, W., McFiggans, G., Mentel, Th. F., Monod, A., Prévôt, A. S. H., Seinfeld, J. H., Surratt, J. D., Szmigielski, R., and Wildt, J.: The formation, properties and impact of secondary organic aerosol: current and emerging issues, Atmos. Chem. Phys., 9, 5155–5236, https://doi.org/10.5194/acp-9-5155-2009, 2009.
Heikkinen, L., Äijälä, M., Riva, M., Luoma, K., Dällenbach, K., Aalto, J., Aalto, P., Aliaga, D., Aurela, M., Keskinen, H., Makkonen, U., Rantala, P., Kulmala, M., Petäjä, T., Worsnop, D., and Ehn, M.: Long-term sub-micrometer aerosol chemical composition in the boreal forest: inter- and intra-annual variability, Atmos. Chem. Phys., 20, 3151–3180, https://doi.org/10.5194/acp-20-3151-2020, 2020.
Hoyle, C. R., Webster, C. S., Rieder, H. E., Nenes, A., Hammer, E., Herrmann, E., Gysel, M., Bukowiecki, N., Weingartner, E., Steinbacher, M., and Baltensperger, U.: Chemical and physical influences on aerosol activation in liquid clouds: a study based on observations from the Jungfraujoch, Switzerland, Atmos. Chem. Phys., 16, 4043–4061, https://doi.org/10.5194/acp-16-4043-2016, 2016.
Hu, D., Liu, D., Zhao, D., Yu, C., Liu, Q., Tian, P., Bi, K., Ding, S., Hu, K., Wang, F., Wu, Y., Wu, Y., Kong, S., Zhou, W., He, H., Huang, M., and Ding, D.: Closure Investigation on Cloud Condensation Nuclei Ability of Processed Anthropogenic Aerosols, J. Geophys. Res.-Atmos., 125, e2020JD032680, https://doi.org/10.1029/2020JD032680, 2020.
Iwamoto, Y., Watanabe, A., Kataoka, R., Uematsu, M., and Miura, K.: Aerosol–cloud interaction at the summit of Mt. Fuji, Japan: Factors influencing cloud droplet number concentrations, Appl. Sci.-Basel, 11, 8439, https://doi.org/10.3390/app11188439, 2021.
Jaén, C., Titos, G., Castillo, S., Casans, A., Rejano, F., Cazorla, A., Herrero, J., Alados-Arboledas, L., Grimalt, J. O., and van Drooge, B. L.: Diurnal source apportionment of organic and inorganic atmospheric particulate matter at a high-altitude mountain site under summer conditions (Sierra Nevada; Spain), Sci. Total Environ., 905, 167178, https://doi.org/10.1016/j.scitotenv.2023.167178, 2023.
Jayachandran, V., Nair, V. S., and Babu, S. S.: CCN activation properties at a tropical hill station in Western Ghats during south-west summer monsoon: Vertical heterogeneity, Atmos. Res., 214, 36–45, https://doi.org/10.1016/j.atmosres.2018.07.018, 2018.
Jimenez, J. L., Canagaratna, M. R., Donahue, N. M., Prevot, A. S. H., Zhang, Q., Kroll, J. H., DeCarlo, P. F., Allan, J. D., Coe, H., Ng, N. L., Aiken, A. C., Docherty, K. S., Ulbrich, I. M., Grieshop, A. P., Robinson, A. L., Duplissy, J., Smith, J. D., Wilson, K. R., Lanz, V. A., Hueglin, C., Sun, Y. L., Tian, J., Laaksonen, A., Raatikainen, T., Rautiainen, J., Vaattovaara, P., Ehn, M., Kulmala, M., Tomlinson, J. M., Collins, D. R., Cubison, M. J., Dunlea, E. J., Huffman, J. A., Onasch, T. B., Alfarra, M. R., Williams, P. I., Bower, K., Kondo, Y., Schneider, J., Drewnick, F., Borrmann, S., Weimer, S., Demerjian, K., Salcedo, D., Cottrell, L., Griffin, R., Takami, A., Miyoshi, T., Hatakeyama, S., Shimono, A., Sun, J. Y., Zhang, Y. M., Dzepina, K., Kimmel, J. R., Sueper, D., Jayne, J. T., Herndon, S. C., Trimborn, A. M., Williams, L. R., Wood, E. C., Middlebrook, A. M., Kolb, C. E., Baltensperger, U., and Worsnop, D. R.: Evolution of organic aerosols in the atmosphere, Science, 326, 1525–1529, https://doi.org/10.1126/science.1180353, 2009.
Jurányi, Z., Gysel, M., Weingartner, E., DeCarlo, P. F., Kammermann, L., and Baltensperger, U.: Measured and modelled cloud condensation nuclei number concentration at the high alpine site Jungfraujoch, Atmos. Chem. Phys., 10, 7891–7906, https://doi.org/10.5194/acp-10-7891-2010, 2010.
Jurányi, Z., Gysel, M., Weingartner, E., Bukowiecki, N., Kammermann, L., and Baltensperger, U.: A 17 month climatology of the cloud condensation nuclei number concentration at the high alpine site Jungfraujoch, J. Geophys. Res.-Atmos., 116, D10204, https://doi.org/10.1029/2010JD015199, 2011.
Kammermann, L., Gysel, M., Weingartner, E., Herich, H., Cziczo, D. J., Holst, T., Svenningsson, B., Arneth, A., and Baltensperger, U.: Subarctic atmospheric aerosol composition: 3. Measured and modeled properties of cloud condensation nuclei, J. Geophys. Res.-Atmos., 115, D04202, https://doi.org/10.1029/2009JD012447, 2010.
Kanakidou, M., Seinfeld, J. H., Pandis, S. N., Barnes, I., Dentener, F. J., Facchini, M. C., Van Dingenen, R., Ervens, B., Nenes, A., Nielsen, C. J., Swietlicki, E., Putaud, J. P., Balkanski, Y., Fuzzi, S., Horth, J., Moortgat, G. K., Winterhalter, R., Myhre, C. E. L., Tsigaridis, K., Vignati, E., Stephanou, E. G., and Wilson, J.: Organic aerosol and global climate modelling: a review, Atmos. Chem. Phys., 5, 1053–1123, https://doi.org/10.5194/acp-5-1053-2005, 2005.
Kawana, K., Nakayama, T., and Mochida, M.: Hygroscopicity and CCN activity of atmospheric aerosol particles and their relation to organics: Characteristics of urban aerosols in Nagoya, Japan, J. Geophys. Res.-Atmos., 121, 4100–4121, https://doi.org/10.1002/2015JD023213, 2016.
Kuang, Y., Xu, W., Tao, J., Ma, N., Zhao, C., and Shao, M.: A Review on Laboratory Studies and Field Measurements of Atmospheric Organic Aerosol Hygroscopicity and Its Parameterization Based on Oxidation Levels, Curr. Pollut. Rep., 6, 410–424, https://doi.org/10.1007/s40726-020-00164-2, 2020a.
Kuang, Y., He, Y., Xu, W., Zhao, P., Cheng, Y., Zhao, G., Tao, J., Ma, N., Su, H., Zhang, Y., Sun, J., Cheng, P., Yang, W., Zhang, S., Wu, C., Sun, Y., and Zhao, C.: Distinct diurnal variation in organic aerosol hygroscopicity and its relationship with oxygenated organic aerosol, Atmos. Chem. Phys., 20, 865–880, https://doi.org/10.5194/acp-20-865-2020, 2020b.
Kulkarni, G., Mei, F., Shilling, J. E., Wang, J., Reveggino, R. P., Flynn, C., Zelenyuk, A., and Fast, J.: Cloud Condensation Nuclei Closure Study Using Airborne Measurements Over the Southern Great Plains, J. Geophys. Res.-Atmos., 128, e2022JD037964, https://doi.org/10.1029/2022JD037964, 2023.
Levin, E. J. T., Prenni, A. J., Palm, B. B., Day, D. A., Campuzano-Jost, P., Winkler, P. M., Kreidenweis, S. M., DeMott, P. J., Jimenez, J. L., and Smith, J. N.: Size-resolved aerosol composition and its link to hygroscopicity at a forested site in Colorado, Atmos. Chem. Phys., 14, 2657–2667, https://doi.org/10.5194/acp-14-2657-2014, 2014.
Li, J., Zhu, C., Chen, H., Zhao, D., Xue, L., Wang, X., Li, H., Liu, P., Liu, J., Zhang, C., Mu, Y., Zhang, W., Zhang, L., Herrmann, H., Li, K., Liu, M., and Chen, J.: The evolution of cloud and aerosol microphysics at the summit of Mt. Tai, China, Atmos. Chem. Phys., 20, 13735–13751, https://doi.org/10.5194/acp-20-13735-2020, 2020.
Liu, P., Song, M., Zhao, T., Gunthe, S. S., Ham, S., He, Y., Qin, Y. M., Gong, Z., Amorim, J. C., Bertram, A. K., and Martin, S. T.: Resolving the mechanisms of hygroscopic growth and cloud condensation nuclei activity for organic particulate matter, Nat. Commun., 9, 4076, https://doi.org/10.1038/s41467-018-06622-2, 2018.
Liu, P. S. K., Deng, R., Smith, K. A., Williams, L. R., Jayne, J. T., Canagaratna, M. R., Moore, K., Onasch, T. B., Worsnop, D. R., and Deshler, T.: Transmission Efficiency of an Aerodynamic Focusing Lens System: Comparison of Model Calculations and Laboratory Measurements for the Aerodyne Aerosol Mass Spectrometer, Aerosol Sci. Technol., 41, 721–733, https://doi.org/10.1080/02786820701422278, 2007.
Liu, X. and Wang, J.: How important is organic aerosol hygroscopicity to aerosol indirect forcing?, Environ. Res. Lett., 5, 44010, https://doi.org/10.1088/1748-9326/5/4/044010, 2010.
Lohmann, U. and Feichter, J.: Global indirect aerosol effects: a review, Atmos. Chem. Phys., 5, 715–737, https://doi.org/10.5194/acp-5-715-2005, 2005.
Mei, F., Setyan, A., Zhang, Q., and Wang, J.: CCN activity of organic aerosols observed downwind of urban emissions during CARES, Atmos. Chem. Phys., 13, 12155–12169, https://doi.org/10.5194/acp-13-12155-2013, 2013.
Meng, J. W., Yeung, M. C., Li, Y. J., Lee, B. Y. L., and Chan, C. K.: Size-resolved cloud condensation nuclei (CCN) activity and closure analysis at the HKUST Supersite in Hong Kong, Atmos. Chem. Phys., 14, 10267–10282, https://doi.org/10.5194/acp-14-10267-2014, 2014.
Minguillón, M. C., Ripoll, A., Pérez, N., Prévôt, A. S. H., Canonaco, F., Querol, X., and Alastuey, A.: Chemical characterization of submicron regional background aerosols in the western Mediterranean using an Aerosol Chemical Speciation Monitor, Atmos. Chem. Phys., 15, 6379–6391, https://doi.org/10.5194/acp-15-6379-2015, 2015.
Minguillón, M. C., Pérez, N., Marchand, N., Bertrand, A., Temime-Roussel, B., Agrios, K., Szidat, S., van Drooge, B., Sylvestre, A., Alastuey, A., Reche, C., Ripoll, A., Marco, E., Grimalt, J. O., and Querol, X.: Secondary organic aerosol origin in an urban environment: influence of biogenic and fuel combustion precursors, Faraday Discuss., 189, 337–359, https://doi.org/10.1039/C5FD00182J, 2016.
Nair, A. A., Yu, F., Campuzano-Jost, P., DeMott, P. J., Levin, E. J. T., Jimenez, J. L., Peischl, J., Pollack, I. B., Fredrickson, C. D., Beyersdorf, A. J., Nault, B. A., Park, M., Yum, S. S., Palm, B. B., Xu, L., Bourgeois, I., Anderson, B. E., Nenes, A., Ziemba, L. D., Moore, R. H., Lee, T., Park, T., Thompson, C. R., Flocke, F., Huey, L. G., Kim, M. J., and Peng, Q.: Machine Learning Uncovers Aerosol Size Information From Chemistry and Meteorology to Quantify Potential Cloud-Forming Particles, Geophys. Res. Lett., 48, e2021GL094133, https://doi.org/10.1029/2021GL094133, 2021.
Ng, N. L., Canagaratna, M. R., Zhang, Q., Jimenez, J. L., Tian, J., Ulbrich, I. M., Kroll, J. H., Docherty, K. S., Chhabra, P. S., Bahreini, R., Murphy, S. M., Seinfeld, J. H., Hildebrandt, L., Donahue, N. M., DeCarlo, P. F., Lanz, V. A., Prévôt, A. S. H., Dinar, E., Rudich, Y., and Worsnop, D. R.: Organic aerosol components observed in Northern Hemispheric datasets from Aerosol Mass Spectrometry, Atmos. Chem. Phys., 10, 4625–4641, https://doi.org/10.5194/acp-10-4625-2010, 2010.
Paatero, P.: The Multilinear Engine—A Table-Driven, Least Squares Program for Solving Multilinear Problems, Including the n-Way Parallel Factor Analysis Model, J. Comput. Graph. Stat., 8, 854–888, https://doi.org/10.1080/10618600.1999.10474853, 1999.
Paatero, P. and Hopke, P. K.: Rotational tools for factor analytic models, J. Chemometr., 23, 91–100, https://doi.org/10.1002/cem.1197, 2009.
Paatero, P. and Tapper, U.: Positive matrix factorization: A non-negative factor model with optimal utilization of error estimates of data values, Environmetrics, 5, 111–126, https://doi.org/10.1002/env.3170050203, 1994.
Pandolfi, M., Alados-Arboledas, L., Alastuey, A., Andrade, M., Angelov, C., Artiñano, B., Backman, J., Baltensperger, U., Bonasoni, P., Bukowiecki, N., Collaud Coen, M., Conil, S., Coz, E., Crenn, V., Dudoitis, V., Ealo, M., Eleftheriadis, K., Favez, O., Fetfatzis, P., Fiebig, M., Flentje, H., Ginot, P., Gysel, M., Henzing, B., Hoffer, A., Holubova Smejkalova, A., Kalapov, I., Kalivitis, N., Kouvarakis, G., Kristensson, A., Kulmala, M., Lihavainen, H., Lunder, C., Luoma, K., Lyamani, H., Marinoni, A., Mihalopoulos, N., Moerman, M., Nicolas, J., O'Dowd, C., Petäjä, T., Petit, J.-E., Pichon, J. M., Prokopciuk, N., Putaud, J.-P., Rodríguez, S., Sciare, J., Sellegri, K., Swietlicki, E., Titos, G., Tuch, T., Tunved, P., Ulevicius, V., Vaishya, A., Vana, M., Virkkula, A., Vratolis, S., Weingartner, E., Wiedensohler, A., and Laj, P.: A European aerosol phenomenology – 6: scattering properties of atmospheric aerosol particles from 28 ACTRIS sites, Atmos. Chem. Phys., 18, 7877–7911, https://doi.org/10.5194/acp-18-7877-2018, 2018.
Paramonov, M., Kerminen, V.-M., Gysel, M., Aalto, P. P., Andreae, M. O., Asmi, E., Baltensperger, U., Bougiatioti, A., Brus, D., Frank, G. P., Good, N., Gunthe, S. S., Hao, L., Irwin, M., Jaatinen, A., Jurányi, Z., King, S. M., Kortelainen, A., Kristensson, A., Lihavainen, H., Kulmala, M., Lohmann, U., Martin, S. T., McFiggans, G., Mihalopoulos, N., Nenes, A., O'Dowd, C. D., Ovadnevaite, J., Petäjä, T., Pöschl, U., Roberts, G. C., Rose, D., Svenningsson, B., Swietlicki, E., Weingartner, E., Whitehead, J., Wiedensohler, A., Wittbom, C., and Sierau, B.: A synthesis of cloud condensation nuclei counter (CCNC) measurements within the EUCAARI network, Atmos. Chem. Phys., 15, 12211–12229, https://doi.org/10.5194/acp-15-12211-2015, 2015.
Park, M., Yum, S. S., Seo, P., Kim, N., and Ahn, C.: A New CCN Number Concentration Prediction Method Based on Multiple Linear Regression and Non-Negative Matrix Factorization: 1. Development, Validation, and Comparison Using the Measurement Data Over the Korean Peninsula, J. Geophys. Res.-Atmos., 128, e2023JD039189, https://doi.org/10.1029/2023JD039189, 2023.
Petters, M. D. and Kreidenweis, S. M.: A single parameter representation of hygroscopic growth and cloud condensation nucleus activity, Atmos. Chem. Phys., 7, 1961–1971, https://doi.org/10.5194/acp-7-1961-2007, 2007.
Pey, J., Pérez, N., Castillo, S., Viana, M., Moreno, T., Pandolfi, M., López-Sebastián, J. M., Alastuey, A., and Querol, X.: Geochemistry of regional background aerosols in the Western Mediterranean, Atmos. Res., 94, 422–435, https://doi.org/10.1016/j.atmosres.2009.07.001, 2009.
Rastak, N., Pajunoja, A., Acosta Navarro, J. C., Ma, J., Song, M., Partridge, D. G., Kirkevåg, A., Leong, Y., Hu, W. W., Taylor, N. F., Lambe, A., Cerully, K., Bougiatioti, A., Liu, P., Krejci, R., Petäjä, T., Percival, C., Davidovits, P., Worsnop, D. R., Ekman, A. M. L., Nenes, A., Martin, S., Jimenez, J. L., Collins, D. R., Topping, D. O., Bertram, A. K., Zuend, A., Virtanen, A., and Riipinen, I.: Microphysical explanation of the RH-dependent water affinity of biogenic organic aerosol and its importance for climate, Geophys. Res. Lett., 44, 5167–5177, https://doi.org/10.1002/2017GL073056, 2017.
Rejano, F., Titos, G., Casquero-Vera, J. A., Lyamani, H., Andrews, E., Sheridan, P., Cazorla, A., Castillo, S., Alados-Arboledas, L., and Olmo, F. J.: Activation properties of aerosol particles as cloud condensation nuclei at urban and high-altitude remote sites in southern Europe, Sci. Total Environ., 762, 143100, https://doi.org/10.1016/j.scitotenv.2020.143100, 2021.
Rejano, F., Casquero-Vera, J. A., Lyamani, H., Andrews, E., Casans, A., Pérez-Ramírez, D., Alados-Arboledas, L., Titos, G., and Olmo, F. J.: Impact of urban aerosols on the cloud condensation activity using a clustering model, Sci. Total Environ., 858, 159657, https://doi.org/10.1016/j.scitotenv.2022.159657, 2023.
Ren, J., Zhang, F., Wang, Y., Collins, D., Fan, X., Jin, X., Xu, W., Sun, Y., Cribb, M., and Li, Z.: Using different assumptions of aerosol mixing state and chemical composition to predict CCN concentrations based on field measurements in urban Beijing, Atmos. Chem. Phys., 18, 6907–6921, https://doi.org/10.5194/acp-18-6907-2018, 2018.
Ren, J., Zhang, F., Chen, L., Cao, G., Liu, M., Li, X., Wu, H., Cheng, Y., and Li, Z.: Identifying the hygroscopic properties of fine aerosol particles from diverse sources in urban atmosphere and the applicability in prediction of cloud nuclei, Atmos. Environ., 298, 119615, https://doi.org/10.1016/j.atmosenv.2023.119615, 2023.
Ripoll, A., Minguillón, M. C., Pey, J., Jimenez, J. L., Day, D. A., Sosedova, Y., Canonaco, F., Prévôt, A. S. H., Querol, X., and Alastuey, A.: Long-term real-time chemical characterization of submicron aerosols at Montsec (southern Pyrenees, 1570 ), Atmos. Chem. Phys., 15, 2935–2951, https://doi.org/10.5194/acp-15-2935-2015, 2015.
Roberts, G. C. and Nenes, A.: A continuous-flow streamwise thermal-gradient CCN chamber for atmospheric measurements, Aerosol Sci. Technol., 39, 206–221, https://doi.org/10.1080/027868290913988, 2005.
Rose, C., Sellegri, K., Moreno, I., Velarde, F., Ramonet, M., Weinhold, K., Krejci, R., Andrade, M., Wiedensohler, A., Ginot, P., and Laj, P.: CCN production by new particle formation in the free troposphere, Atmos. Chem. Phys., 17, 1529–1541, https://doi.org/10.5194/acp-17-1529-2017, 2017.
Rose, D., Nowak, A., Achtert, P., Wiedensohler, A., Hu, M., Shao, M., Zhang, Y., Andreae, M. O., and Pöschl, U.: Cloud condensation nuclei in polluted air and biomass burning smoke near the mega-city Guangzhou, China – Part 1: Size-resolved measurements and implications for the modeling of aerosol particle hygroscopicity and CCN activity, Atmos. Chem. Phys., 10, 3365–3383, https://doi.org/10.5194/acp-10-3365-2010, 2010.
Rose, D., Gunthe, S. S., Su, H., Garland, R. M., Yang, H., Berghof, M., Cheng, Y. F., Wehner, B., Achtert, P., Nowak, A., Wiedensohler, A., Takegawa, N., Kondo, Y., Hu, M., Zhang, Y., Andreae, M. O., and Pöschl, U.: Cloud condensation nuclei in polluted air and biomass burning smoke near the mega-city Guangzhou, China – Part 2: Size-resolved aerosol chemical composition, diurnal cycles, and externally mixed weakly CCN-active soot particles, Atmos. Chem. Phys., 11, 2817–2836, https://doi.org/10.5194/acp-11-2817-2011, 2011.
Rumelhart, D. E., Hinton, G. E., and Williams, R. J.: Learning representations by back-propagating errors, Nature, 323, 533–536, https://doi.org/10.1038/323533a0, 1986.
Salma, I., Thén, W., Vörösmarty, M., and Gyöngyösi, A. Z.: Cloud activation properties of aerosol particles in a continental Central European urban environment, Atmos. Chem. Phys., 21, 11289–11302, https://doi.org/10.5194/acp-21-11289-2021, 2021.
Schmale, J., Henning, S., Henzing, B., Keskinen, H., Sellegri, K., Ovadnevaite, J., Bougiatioti, A., Kalivitis, N., Stavroulas, I., Jefferson, A., Park, M., Schlag, P., Kristensson, A., Iwamoto, Y., Pringle, K., Reddington, C., Aalto, P., Äijälä, M., Baltensperger, U., Bialek, J., Birmili, W., Bukowiecki, N., Ehn, M., Fjæraa, A. M., Fiebig, M., Frank, G., Fröhlich, R., Frumau, A., Furuya, M., Hammer, E., Heikkinen, L., Herrmann, E., Holzinger, R., Hyono, H., Kanakidou, M., Kiendler-Scharr, A., Kinouchi, K., Kos, G., Kulmala, M., Mihalopoulos, N., Motos, G., Nenes, A., O'Dowd, C., Paramonov, M., Petäjä, T., Picard, D., Poulain, L., Prévôt, A. S. H., Slowik, J., Sonntag, A., Swietlicki, E., Svenningsson, B., Tsurumaru, H., Wiedensohler, A., Wittbom, C., Ogren, J. A., Matsuki, A., Yum, S. S., Myhre, C. L., Carslaw, K., Stratmann, F., and Gysel, M.: Collocated observations of cloud condensation nuclei, particle size distributions, and chemical composition, Sci. Data, 4, 170003, https://doi.org/10.1038/sdata.2017.3, 2017.
Schmale, J., Henning, S., Decesari, S., Henzing, B., Keskinen, H., Sellegri, K., Ovadnevaite, J., Pöhlker, M. L., Brito, J., Bougiatioti, A., Kristensson, A., Kalivitis, N., Stavroulas, I., Carbone, S., Jefferson, A., Park, M., Schlag, P., Iwamoto, Y., Aalto, P., Äijälä, M., Bukowiecki, N., Ehn, M., Frank, G., Fröhlich, R., Frumau, A., Herrmann, E., Herrmann, H., Holzinger, R., Kos, G., Kulmala, M., Mihalopoulos, N., Nenes, A., O'Dowd, C., Petäjä, T., Picard, D., Pöhlker, C., Pöschl, U., Poulain, L., Prévôt, A. S. H., Swietlicki, E., Andreae, M. O., Artaxo, P., Wiedensohler, A., Ogren, J., Matsuki, A., Yum, S. S., Stratmann, F., Baltensperger, U., and Gysel, M.: Long-term cloud condensation nuclei number concentration, particle number size distribution and chemical composition measurements at regionally representative observatories, Atmos. Chem. Phys., 18, 2853–2881, https://doi.org/10.5194/acp-18-2853-2018, 2018.
Seinfeld, J. H., Bretherton, C., Carslaw, K. S., Coe, H., DeMott, P. J., Dunlea, E. J., Feingold, G., Ghan, S., Guenther, A. B., Kahn, R., Kraucunas, I., Kreidenweis, S. M., Molina, M. J., Nenes, A., Penner, J. E., Prather, K. A., Ramanathan, V., Ramaswamy, V., Rasch, P. J., Ravishankara, A. R., Rosenfeld, D., Stephens, G., and Wood, R.: Improving our fundamental understanding of the role of aerosol–cloud interactions in the climate system, P. Natl. Acad. Sci. USA, 113, 5781–90, https://doi.org/10.1073/pnas.1514043113, 2016.
Shang, D., Hu, M., Zheng, J., Qin, Y., Du, Z., Li, M., Fang, J., Peng, J., Wu, Y., Lu, S., and Guo, S.: Particle number size distribution and new particle formation under the influence of biomass burning at a high altitude background site at Mt. Yulong (3410 m), China, Atmos. Chem. Phys., 18, 15687–15703, https://doi.org/10.5194/acp-18-15687-2018, 2018.
Siegel, K., Neuberger, A., Karlsson, L., Zieger, P., Mattsson, F., Duplessis, P., Dada, L., Daellenbach, K., Schmale, J., Baccarini, A., Krejci, R., Svenningsson, B., Chang, R., Ekman, A. M. L., Riipinen, I., and Mohr, C.: Using Novel Molecular-Level Chemical Composition Observations of High Arctic Organic Aerosol for Predictions of Cloud Condensation Nuclei, Environ. Sci. Technol., 56, 13888–13899, https://doi.org/10.1021/acs.est.2c02162, 2022.
Spellman, G.: An application of artificial neural networks to the prediction of surface ozone concentrations in the United Kingdom, Appl. Geogr., 19, 123–136, https://doi.org/10.1016/S0143-6228(98)00039-3, 1999.
Svenningsson, B., Rissler, J., Swietlicki, E., Mircea, M., Bilde, M., Facchini, M. C., Decesari, S., Fuzzi, S., Zhou, J., Mønster, J., and Rosenørn, T.: Hygroscopic growth and critical supersaturations for mixed aerosol particles of inorganic and organic compounds of atmospheric relevance, Atmos. Chem. Phys., 6, 1937–1952, https://doi.org/10.5194/acp-6-1937-2006, 2006.
Tao, J., Kuang, Y., Ma, N., Hong, J., Sun, Y., Xu, W., Zhang, Y., He, Y., Luo, Q., Xie, L., Su, H., and Cheng, Y.: Secondary aerosol formation alters CCN activity in the North China Plain, Atmos. Chem. Phys., 21, 7409–7427, https://doi.org/10.5194/acp-21-7409-2021, 2021.
Thalman, R., de Sá, S. S., Palm, B. B., Barbosa, H. M. J., Pöhlker, M. L., Alexander, M. L., Brito, J., Carbone, S., Castillo, P., Day, D. A., Kuang, C., Manzi, A., Ng, N. L., Sedlacek III, A. J., Souza, R., Springston, S., Watson, T., Pöhlker, C., Pöschl, U., Andreae, M. O., Artaxo, P., Jimenez, J. L., Martin, S. T., and Wang, J.: CCN activity and organic hygroscopicity of aerosols downwind of an urban region in central Amazonia: seasonal and diel variations and impact of anthropogenic emissions, Atmos. Chem. Phys., 17, 11779–11801, https://doi.org/10.5194/acp-17-11779-2017, 2017.
Titos, G., Lyamani, H., Pandolfi, M., Alastuey, A., and Alados-Arboledas, L.: Identification of fine (PM1) and coarse (PM10−1) sources of particulate matter in an urban environment, Atmos. Environ., 89, 593–602, https://doi.org/10.1016/j.atmosenv.2014.03.001, 2014.
Twomey, S.: The Influence of Pollution on the Shortwave Albedo of Clouds, J. Atmos. Sci., 34, 1149–1152, https://doi.org/10.1175/1520-0469(1977)034<1149:TIOPOT>2.0.CO;2, 1977.
Via, M., Minguillón, M. C., Reche, C., Querol, X., and Alastuey, A.: Increase in secondary organic aerosol in an urban environment, Atmos. Chem. Phys., 21, 8323–8339, https://doi.org/10.5194/acp-21-8323-2021, 2021.
Wang, J., Cubison, M. J., Aiken, A. C., Jimenez, J. L., and Collins, D. R.: The importance of aerosol mixing state and size-resolved composition on CCN concentration and the variation of the importance with atmospheric aging of aerosols, Atmos. Chem. Phys., 10, 7267–7283, https://doi.org/10.5194/acp-10-7267-2010, 2010.
Wiedensohler, A., Birmili, W., Nowak, A., Sonntag, A., Weinhold, K., Merkel, M., Wehner, B., Tuch, T., Pfeifer, S., Fiebig, M., Fjäraa, A. M., Asmi, E., Sellegri, K., Depuy, R., Venzac, H., Villani, P., Laj, P., Aalto, P., Ogren, J. A., Swietlicki, E., Williams, P., Roldin, P., Quincey, P., Hüglin, C., Fierz-Schmidhauser, R., Gysel, M., Weingartner, E., Riccobono, F., Santos, S., Grüning, C., Faloon, K., Beddows, D., Harrison, R., Monahan, C., Jennings, S. G., O'Dowd, C. D., Marinoni, A., Horn, H.-G., Keck, L., Jiang, J., Scheckman, J., McMurry, P. H., Deng, Z., Zhao, C. S., Moerman, M., Henzing, B., de Leeuw, G., Löschau, G., and Bastian, S.: Mobility particle size spectrometers: harmonization of technical standards and data structure to facilitate high quality long-term observations of atmospheric particle number size distributions, Atmos. Meas. Tech., 5, 657–685, https://doi.org/10.5194/amt-5-657-2012, 2012.
Wu, Z. J., Zheng, J., Shang, D. J., Du, Z. F., Wu, Y. S., Zeng, L. M., Wiedensohler, A., and Hu, M.: Particle hygroscopicity and its link to chemical composition in the urban atmosphere of Beijing, China, during summertime, Atmos. Chem. Phys., 16, 1123–1138, https://doi.org/10.5194/acp-16-1123-2016, 2016.
Zeb, B., Alam, K., Nasir, J., Mansha, M., Ahmad, I., Bibi, S., Malik, S. M., and Ali, M.: Black Carbon aerosol characteristics and radiative forcing over the high altitude glacier region of Himalaya-Karakorum-Hindukush, Atmos. Environ., 238, 117711, https://doi.org/10.1016/j.atmosenv.2020.117711, 2020.
Zhang, F., Li, Z., Li, Y., Sun, Y., Wang, Z., Li, P., Sun, L., Wang, P., Cribb, M., Zhao, C., Fan, T., Yang, X., and Wang, Q.: Impacts of organic aerosols and its oxidation level on CCN activity from measurement at a suburban site in China, Atmos. Chem. Phys., 16, 5413–5425, https://doi.org/10.5194/acp-16-5413-2016, 2016.
Zhang, F., Wang, Y., Peng, J., Ren, J., Collins, D., Zhang, R., Sun, Y., Yang, X., and Li, Z.: Uncertainty in Predicting CCN Activity of Aged and Primary Aerosols, J. Geophys. Res.-Atmos., 122, 711–723, 736, https://doi.org/10.1002/2017JD027058, 2017.
Zhang, Q., Jimenez, J. L., Canagaratna, M. R., Allan, J. D., Coe, H., Ulbrich, I., Alfarra, M. R., Takami, A., Middlebrook, A. M., Sun, Y. L., Dzepina, K., Dunlea, E., Docherty, K., DeCarlo, P. F., Salcedo, D., Onasch, T., Jayne, J. T., Miyoshi, T., Shimono, A., Hatakeyama, S., Takegawa, N., Kondo, Y., Schneider, J., Drewnick, F., Borrmann, S., Weimer, S., Demerjian, K., Williams, P., Bower, K., Bahreini, R., Cottrell, L., Griffin, R. J., Rautiainen, J., Sun, J. Y., Zhang, Y. M., and Worsnop, D. R.: Ubiquity and dominance of oxygenated species in organic aerosols in anthropogenically-influenced Northern Hemisphere midlatitudes, Geophys. Res. Lett., 34, L13801, https://doi.org/10.1029/2007GL029979, 2007.
Zhang, Z., Xu, W., Zhang, Y., Zhou, W., Xu, X., Du, A., Zhang, Y., Qiao, H., Kuang, Y., Pan, X., Wang, Z., Cheng, X., Liu, L., Fu, Q., Worsnop, D. R., Li, J., and Sun, Y.: Measurement report: Secondary organic aerosols at a forested mountain site in southeastern China, EGUsphere [preprint], https://doi.org/10.5194/egusphere-2023-2684, 2023.