the Creative Commons Attribution 4.0 License.
the Creative Commons Attribution 4.0 License.
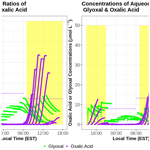
Process analysis of elevated concentrations of organic acids at Whiteface Mountain, New York
Christopher Lawrence
Mary Barth
John Orlando
Paul Casson
Richard Brandt
Daniel Kelting
Elizabeth Yerger
Sara Lance
Organic acids represent an important class of compounds in the atmosphere, but there is limited research investigating their chemical production, particularly in the northeast United States. To improve our understanding of organic acid sources, a modeling analysis was performed for air masses reaching the summit of Whiteface Mountain (WFM), New York, where measurements of organic acids in cloud water have been collected. The analysis focuses on a pollution event associated with a heat wave that occurred on 1–2 July 2018 that exhibited unusually high concentrations of formic (HCOOH), acetic (CH3COOH), and oxalic (OxAc) acid in cloud water. The gas-phase production of organic acids for this pollution event was modeled using a combination of the regional transport model Weather Research and Forecasting Model with Chemistry (WRF-Chem), which gives information on transport and environmental factors affecting air parcels reaching WFM, and the Lagrangian chemical box model BOXMOX, which allows analysis of chemistry with different chemical mechanisms. Two chemical mechanisms are used in BOXMOX: (1) the Model for Ozone and Related chemical Tracers (MOZART T1) and (2) the Master Chemical Mechanism (MCM) version 3.3.1. The WRF-Chem results show that air parcels sampled during the pollution event at WFM originated in central Missouri, which has strong biogenic emissions of isoprene. Many air parcels were influenced by emissions of nitrogen oxides (NOx) from the Chicago metropolitan area. The gas-phase oxidation of isoprene and its related oxidation products was the major source of HCOOH and CH3COOH, but both mechanisms substantially underproduced both acids compared to observations. A simple gas–aqueous mechanism was included to investigate the role of aqueous chemistry in organic acid production. Aqueous chemistry did not produce more HCOOH or CH3COOH, suggesting missing chemical sources of both acids. However this aqueous chemistry was able to explain the elevated concentrations of OxAc. Anthropogenic NOx emissions from Chicago had little overall impact on the production of all three organic acids. Further studies are required to better constrain gas and aqueous production of low-molecular-weight organic acids.
- Article
(5934 KB) - Full-text XML
-
Supplement
(6659 KB) - BibTeX
- EndNote
Organic acids are an important class of compounds in the atmosphere that can represent an important fraction of organic aerosol, comprising up to 52 % of the water-soluble organic carbon mass (Sorooshian et al., 2007; Miyazaki et al., 2009; Kawamura and Bikkina, 2016; Kawamura et al., 2017). Organic acids can also contribute a large fraction of the acidity in cloud water and rainwater, particularly in remote and rural regions (Pye et al., 2020), and may contribute to new particle formation (Zhang et al., 2004, 2017; Kumar et al., 2019). Additionally, there is growing evidence that organic acids are important in partitioning ammonia (NH3) into ambient aerosol (Tao and Murphy, 2019; Li et al., 2021) and cloud water (Lawrence et al., 2023). Organic acids are ubiquitously found throughout the atmosphere, measured in locations including the Arctic (Mungall et al., 2018; Feltracco et al., 2021), urban environments (Souza et al., 1999; Avery et al., 2001), biomass burning smoke plumes (Chaliyakunnel et al., 2016), and forested areas (Fulgham et al., 2019; Eger et al., 2020). Despite their ubiquity and their growing chemical importance in many regions around the world, organic acids are often not routinely included in studies monitoring the chemical composition of cloud water and rainwater and are rarely investigated in detail within modeling studies in either the gas or aqueous phase. To contribute to the limited body of research, this study investigates the key processes in both the gas and aqueous phases that led to unusually high concentrations of organic acids measured in Whiteface Mountain (WFM) cloud water on 1 July 2018.
Formic (HCOOH) and acetic (CH3COOH) acids are typically the most abundant monocarboxylic acids found in the atmosphere (Paulot et al., 2011; Link et al., 2020). Primary sources of HCOOH and CH3COOH include soil emissions (Mielnik et al., 2018), biomass burning (Chaliyakunnel et al., 2016), and even certain species of ants (Graedel and Eisner, 1988; Legrand et al., 2012). HCOOH and CH3COOH are also produced from the atmospheric oxidation of volatile organic compounds (VOCs; Fig. 1). It is thought HCOOH and CH3COOH are largely biogenic in origin but are also known to have important anthropogenic sources regionally including fossil fuel combustion and volatile chemical products. In particular, the oxidation of isoprene and its related oxidation products are considered the most important precursor VOCs. Even though these acids are commonly found in the atmosphere, they are typically underpredicted by current gas-phase mechanisms, especially HCOOH (Millet et al., 2015; Yuan et al., 2015; Chen et al., 2021), with the underlying causes remaining unclear.
More recent work has revealed that cloud droplets may act as an important medium for the formation of organic acids. Volatile but highly water-soluble gases like glyoxal can dissolve into cloud droplets, where they subsequently oxidize to form dicarboxylic organic acids such as oxalic acid (OxAc) (Fig. 1) that remain within the particle phase after the cloud droplets evaporate (Blando and Turpin, 2000; Lim et al., 2005; Warneck, 2005; Ervens et al., 2003; Sorooshian et al., 2006; Carlton et al., 2007; Tan et al., 2010; Tilgner and Herrmann, 2010). This process is especially important for the formation of dicarboxylic acids like OxAc as they have no known secondary gas-phase sources, while primary emissions cannot explain their atmospheric concentrations (Yao et al., 2004). Despite the prevalence of this chemistry, these processes are often ignored or are oversimplified in chemical transport models.
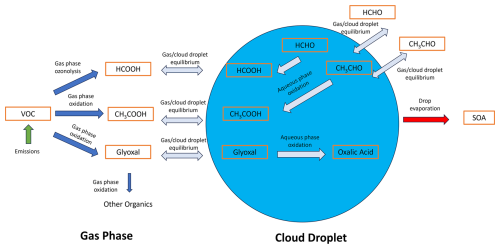
Figure 1Summary of the major processes controlling organic acid production including emissions of VOCs; gas-phase oxidation to form HCOOH, CH3COOH, and the important precursor glyoxal; gas–cloud equilibrium partitioning; and the aqueous oxidation that either produces or removes organic acids. Important secondary organic aerosol chemistry is ignored to maintain simplicity of the schematic.
At the summit of WFM in upstate New York, there is a historic cloud water monitoring program that has been operating since 1994. This program was initially focused on investigating the formation of two acid deposition species, sulfate (SO) and nitrate (NO), and was subsequently funded to monitor progress of the Clean Air Act Amendments of 1990. In more recent years, as the prevalence of acid deposition has decreased at WFM and throughout the United States, attention has shifted toward the organic fraction of cloud water (Schwab et al., 2016; Lawrence et al., 2023). Starting in 2018, organic acids were added to the suite of regularly measured chemical species within cloud water which include HCOOH, CH3COOH, and OxAc.
On 1–2 July 2018, collected cloud samples exhibited unusually high concentrations of these organic acids with the underlying causes remaining unexplored. As the influence from SO and NO in cloud water has decreased at WFM at the same time that the influence from organic carbon has increased (Lawrence et al., 2023), the importance of organic acid contributions to the chemical system has grown, requiring a better characterization of their underlying chemistry. Chemical transport models can be used to study the production of organic acids. However, it is challenging to investigate the major chemistry involved in their production upwind of a given location. Chemical box modeling can be used for a detailed look at the chemistry of organic acid production, but the initial conditions and emissions of many chemical species, particularly VOCs, are limited both spatially and temporally. To overcome these limitations, a combination of chemical transport modeling and Lagrangian chemical box modeling can be used to investigate organic acid production.
The current study used a combination of the chemical transport model Weather Research and Forecasting Model with Chemistry (WRF-Chem; Grell et al., 2005; Fast et al., 2006) and the gas-phase chemical box model BOXMOX (Knote et al., 2015) to evaluate the gas-phase chemistry affecting the high concentrations of organic acids at WFM during this pollution event. WRF-Chem simulations were performed for the heat wave and pollution event to provide the necessary meteorological and chemical input data to conduct Lagrangian chemical box modeling. BOXMOX was subsequently used for a detailed assessment of the gas-phase chemistry involved in organic acid production. Gas-phase box modeling results are compared to cloud water measurements made at WFM. Additionally, a simple gas–aqueous box model was employed to determine if cloud chemistry contributed to overall organic acid concentrations. Finally, the impacts of anthropogenic emissions on organic acid production will be discussed.
The 1–2 July 2018 pollution event was chosen as a case study to investigate the chemical production of organic acids. This event impacted much of the northeast United States, including WFM, coinciding with a regional heat wave with temperatures reaching 35 °C (Fig. S1 in the Supplement) in several locations. Many locations, particularly the New York City metropolitan area, saw O3 mixing ratios exceeding National Ambient Air Quality Standards, with mixing ratios reaching over 100 ppbv (Tian et al., 2020; Tran et al., 2023).
2.1 WFM observations
At WFM, concentrations of several chemical species including organic acids both in cloud water and in the gas phase were considerably greater than normal during this event. Information about cloud water collection protocols at WFM can be found in Lawrence et al. (2023). Briefly, an automated Mohnen omni-directional cloud water collector is used to collect warm cloud water (i.e., >0 °C) from non-precipitating clouds between the months of June and September. Samples were collected in a refrigerated accumulator that dumps into a refrigerated sample bottle every 12 h. Samples were then analyzed for sulfate (SO), nitrate (NO), ammonium (NH), calcium (Ca2+), magnesium (Mg2+), potassium (K+), sodium (Na+), chloride (Cl−), pH, conductivity, water-soluble organic carbon (WSOC), and organic acids including HCOOH, CH3COOH, and OxAc. Organic acids were measured by the Adirondack Watershed Institute using a Lachat QC 8500 ion chromatograph, along with SO and Cl−. A paper focusing on the organic acid measurement methods and observations will be submitted separately. The current work focuses on three of the measured organic acids, HCOOH, CH3COOH, and OxAc, as these are the three most common organic acids found in cloud water at WFM and other locations (Herckes et al., 2013). While the exact detection limits of the organic acid analysis is currently being determined, a conservative estimate of 50 µg L−1 for all three organic acids is used, based on the lowest-concentration calibration standard. It is worth noting that the concentrations of the three organic acids investigated in this study are well above this conservative detection limit, with concentrations of 113, 111, and 23× greater than the lowest-concentration standard used in the calibrations respectively. Trace gases are measured continuously year-round, with chemical species including ozone (O3), oxides of nitrogen (NO, NO2, and NOy), and sulfur dioxide (SO2). More information about the gas-phase dataset can be found in Brandt et al. (2016).
The pollution event consisted of some of the highest concentrations of the season for SO, NH, WSOC, HCOOH, CH3COOH, and OxAc (Fig. 2), with individual samples of HCOOH and CH3COOH exhibiting concentrations greater than 100 µeq L−1 and contributing to approximately 30 % of measured anions. Additionally, O3 and NOy mixing ratios were above the 90th percentile of mixing ratios for this event, as compared to the rest of the 2018 summer season (June through September), coinciding with the highest temperatures of the cloud collection season (Fig. S2). The relatively high mixing ratios of these trace gases may indicate significant anthropogenic influence. The cloud event focused on two cloud samples collected between 30 June 2018 at 20:00 EST to 1 July 2018 at 10:00 EST, with cloud liquid water content (LWC) values reaching up to 1.25 g m−3 (Fig. S4). The 1 July event was chosen for the modeling study as the duration of this cloud event was substantially longer than the event on 2 July, making it better-suited for modeling.
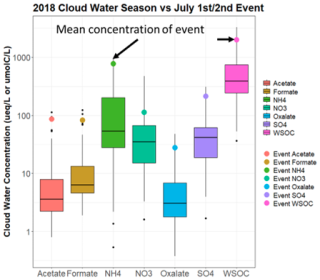
Figure 2Cloud water concentrations of acetate (CH3COOH), formate (HCOOH), NH, NO, oxalate (OxAc), SO, and WSOC from the June–September 2018 cloud water season. WSOC is reported in units of micromoles of carbon per liter (µmol C L−1), whereas all other analytes are reported in units of micro equivalents per liter (µeq L−1). The 25th, 50th, and 75th percentiles are marked by the colored boxes, the vertical lines represent 1.5× the interquartile range, and the black dots represent values outside the vertical lines.
2.2 Determining total organic acid mixing ratios from cloud water observations
Currently at WFM, organic acids are measured only within cloud water. However, substantial concentrations of low-molecular-weight organic acids have been previously shown to be in the gas phase (Khwaja, 1995). Gas-phase and total mixing ratios of organic acids can be estimated, assuming the organic acid is in equilibrium with the atmosphere, as a function of the acid's Henry's law constant, cloud LWC, temperature, pressure, and pH of the cloud droplets using the following equation:
where OrgAcidtot is the calculated sum of gas-phase and aqueous-phase organic acid mixing ratios in parts per trillion by volume (pptv), 1012 is a conversion factor to convert the mixing ratio to parts per trillion by volume, QLWC is the cloud LWC (in L m−3), R is the universal gas constant (8.314 m3 Pa K−1 mol−1), T is the ambient temperature (in K), P is the ambient pressure (in Pa), OrgAcidaq is the concentration of the specific organic acid measured in the cloud water (in mol L−1), Patm is the ambient atmospheric pressure (in atm), and KHeff is the temperature- and pH-dependent effective Henry's law constant for the given organic acid (in mol atm−1). The pH dependency of KHeff for monocarboxylic acids can be calculated by
while for dicarboxylic acids, KHeff can be calculated by
where KH is the standard Henry's law constant of the organic acid, Ka is the acid dissociation constant for monocarboxylic acids, and are the first and second dissociation constants for dicarboxylic acids, and [H+] is the acidity of the cloud droplets. The temperature dependence of the Henry's law constant is
where T2 is the ambient temperature, T1 is the reference temperature of 298.15 K, and ΔHs is enthalpy of dissolution described in Sander (2023). The values used for the above calculations can be found in Table S1 in the Supplement. KH values of HCOOH, CH3COOH, and OxAc are taken from Sander (2023), while Ka values were taken from Seinfeld and Pandis (2016). The associated pH values of the two cloud samples used in this study are 4.50 and 4.56, while the temperatures are 292.17 and 292.12 K respectively.
This work uses a combination of modeling techniques, including ensembles of HYbrid Single-Particle Lagrangian Integrated Trajectory (HYSPLIT) back trajectories (Stein et al., 2015), the WRF-Chem chemical transport model, gas-phase box modeling, and the box modeling of gas and aqueous chemistry. This methodology is used to allow for more detailed investigation of the underlying chemistry impacting organic acid formation. It is challenging to investigate chemical processing of an air mass upwind of a location in detail using chemical transport models alone. A Lagrangian approach coupled with a chemical box model allows for the detailed investigation of the underlying chemistry involved in the production of organic acids. Figure 3 summarizes the step-by-step procedure for this modeling process.
3.1 HYSPLIT back-trajectory analysis
A 3 d ensemble back-trajectory analysis was conducted to determine the source location of the pollution event using the (HYSPLIT) model (Stein et al., 2015). The receptor site for the trajectories is the summit of WFM (44.37° N, 73.9° W; 1500 m above sea level). The meteorological data used for these calculations were the North American Mesoscale (NAM) 12 km × 12 km dataset (more information on the meteorology data can found at https://www.ready.noaa.gov/archives.php, last access: 8 July 2022). The trajectories consistently flew near the surface in central Missouri near Jefferson City approximately 2 d prior to the pollution event at WFM (Fig. 4). This location was therefore chosen to launch the WRF-Chem forward trajectories.
3.2 WRF-Chem
3.2.1 Model run description
The chemical transport model used for these simulations was the Weather Research and Forecasting Model with Chemistry (WRF-Chem) v4.0.3 (Grell et al., 2005; Fast et al., 2006). Multiphase chemistry including gas, aerosol, clouds, and rain was included within the simulation. A 5 d simulation was performed from 27 June 2018 at 00:00 UTC to 2 July 2018 at 12:00 UTC with a 12 km × 12 km horizontal grid resolution and 43 vertical layers from the surface to 50 hPa. A detailed description of the WRF-Chem simulation parameters and a map of the WRF-Chem domain can be found in Sect. S3 and Fig. S3 of the Supplement.
3.2.2 WRF-Chem evaluation
O3 and PM 2.5 data collected by the EPA's Air Quality System (AQS) monitoring program (U.S. EPA, 2024) were used to evaluate the capabilities of WRF-Chem to represent the pollution event. The air mass associated with this pollution event was characterized by a combination of high temperatures over the Great Plains region that moved eastward towards the Great Lakes region before reaching the northeast under the influence of a large high-pressure system. The air mass associated with this pollution event was characterized by high temperatures over the Great Plains. A high-pressure system formed a ridge over much of the Great Lakes and Ontario, Canada, with the air mass moving from Missouri across Illinois and Michigan before reaching New York (Fig. S5). WRF-Chem properly captured the warm temperatures that moved across the midwest into the northeast (Fig. S5). These meteorological conditions contributed to O3 mixing ratios in excess of 70 ppbv over large portions of the midwest on 29 June at 20:00 UTC before spreading to the northeast United States including WFM on 1 July at 20:00 UTC. Additionally, PM2.5 levels rose to levels >15 µg m−3 throughout much of the eastern United States on 1 July 2018, including WFM (Fig. 5). There was potential evidence for an influence from wildfire activity from the southeast United States according to the WRF-Chem simulations, but it was unclear if emissions from these fires contributed significantly to the pollution event. To determine potential fire impact, a WRF-Chem simulation was run that did not include any biomass burning emissions for the same time interval as the original simulations. Comparisons of these simulations found virtually no contribution of biomass burning emissions to PM2.5 mass concentrations, O3 mixing ratios, or trace gases important in the formation of organic acids (Fig. S6), indicating this pollution event was primarily driven by biogenic and/or anthropogenic emissions.
Modeled O3 exhibited a strong positive linear correlation (r>0.8) with observations across the model domain but consistently exhibited a mean bias error (MBE) of more than 10 ppbv on 29 June and 1 July (Figs. S7 and S8). This high bias in O3 has been reported in other recent works (Travis et al., 2016; Schwantes et al., 2020; Place et al., 2023), which may be due to overestimated NOx emissions and/or improper representation of gas-phase organic chemistry. Note that the 2017 EPA National Emissions Inventory (NEI) used in this study is appropriate for a typical summer day and will likely not represent the actual emissions of the heat wave period caused by the stagnation event. Heat waves can increase demand on the grid (Maia-Silva et al., 2020; Stone et al., 2023) and therefore increase NOx emissions due to greater combustion of fossil fuels from power generation (Chen et al., 2015), which are not represented in the 2017 NEI. Given the potential low bias in modeled NOx emissions, the high bias in modeled O3 is even more perplexing, highlighting the complex chemistry involved in O3 production.
Importantly, the modeled MBE for O3 is < 10 ppbv for central Missouri on 29 June and western New York on 1 July, locations that were upwind of WFM according to the HYSPLIT trajectories. This indicates that O3 chemistry was well represented in the air mass that traveled to WFM. PM2.5 model predictions performed worse compared to O3, with many linear correlation values exhibiting null or negative values and MBE exceeding 10 µg m−3. Similar to O3, model MBE was < 10 µg m−3 for Missouri and much of Chicago on June 29th and western New York on 1 July.
Three air quality monitoring sites in New York measuring O3, PM2.5, and 2 m temperature were chosen for time-series evaluations of WRF-Chem, including Pinnacle State Park (PSP) in the Southern Tier of New York, Queens College, New York City, and measurements at the old ski lodge below the summit of WFM (Fig. S9). More information about the data collected at these sites can be found in Brandt et al. (2016) and Ninneman et al. (2020), while Pearson correlation values and MBE statistics can be found in Fig. S9. WFM tends to show the lowest linear correlation with observations. This is likely due to WRF-Chem underestimating the elevation of WFM (1483 m) by over 700 m and therefore not properly accounting for the topography in the region (Fig. S10). By using a 12 km × 12 km horizontal grid mesh in WRF-Chem, the topography is not well represented, resulting in the modeled WFM summit being underestimated by approximately 700 m and affecting the capabilities of WRF-Chem to represent mountain and valley winds and the timing of when the summit is above and within the planetary boundary layer (Giovannini et al., 2020). PSP shows the lowest MBE values with high correlation coefficients (r>0.7) for O3 and 2 m temperature. Finally, Queens College saw the strongest correlation coefficients for O3 and 2 m temperature (r>0.85) but exhibited large positive biases for O3 and PM2.5. The causes behind these overpredictions remain unclear but are beyond the scope of this work.
3.2.3 Forward-trajectory ensemble analysis
A feature of WRF-Chem is to monitor air masses through forward trajectories. With an input file, trajectories can be launched at specified latitude–longitude–height locations and times. The trajectory code uses resolved winds (u, v, w) to determine the location of the air mass at each time step. Several variables can be monitored along the trajectory including prognostic and diagnostic information (https://www2.acom.ucar.edu/sites/default/files/documents/Trajectory.desc_.pdf, last access: 10 October 2024). During the WRF-Chem simulation, 10 sets of 75 forward trajectories were launched near Jefferson City, Missouri, at 38.5° N and 92.5° W. This location was chosen based on the HYSPLIT back-trajectory analysis. The starting latitude and longitude of the trajectories was perturbed by ± 0.1 and ± 0.2°, and they were launched at three starting heights of 750, 1000, and 1250 m every 2 h starting on 28 June 2018 at 22:00 UTC and ending on 29 June 2018 at 16:00 UTC. To limit the analysis to trajectories that influenced WFM, only trajectories that flew within 1° latitude and longitude and below 3000 m a.g.l. were considered for chemical box modeling. Of the 750 trajectories launched, 556 trajectories (74.1 %) reached WFM.
3.2.4 Chemical box modeling
The chemical box model BOXMOX was used to simulate the gas-phase chemistry along the trajectory pathways. BOXMOX uses a Kinetic PreProcessor with a Rosenbrock ODE solver (Knote et al., 2015). The necessary box model input parameters were obtained from the output data from the WRF-Chem forward trajectories, providing information for initial conditions, emissions (biogenic, anthropogenic, and biomass burning), background conditions, photolysis rate constants, and environmental conditions (temperature, pressure, planetary boundary layer height). Initial conditions are determined by using the mixing ratios at time 0 of the launch locations of the given trajectory. Photolysis rates were provided at a 15 min time resolution, while emissions, environmental conditions, and background conditions were provided at a 1 h time resolution. Emissions were assumed to be zero if the trajectory height was above the top of the boundary layer. In order to account for the entrainment of background air into the air parcel, a first-order mixing rate constant was set to 1.17 × 10−5 s−1, associated with a dilution time of approximately 24 h, consistent with values used in other works (Wolfe et al., 2016; Decker et al., 2019). A sensitivity analysis of this dilution constant in Sect. S7 reveals that while there were noticeable impacts on organic acid production, the conclusions of this work were not impacted (Fig. S11), as will be discussed further in Sect. 4. Background air is determined by 60 km × 60 km WRF-Chem average mixing ratios of the chemical species of interest at the height of the trajectory.
Two gas-phase mechanisms were used for the BOXMOX simulations: the Model for Ozone and Related chemical Tracers (MOZART) version T1 and the Master Chemical Mechanism (MCM) version 3.3.1. Two mechanisms were chosen to determine if a simpler mechanism is sufficient in simulating organic acid chemistry that is more explicitly represented in the more complex mechanism of MCM v3.3.1. MOZART T1 contains 151 chemical species and 352 gas-phase reactions, as described in Emmons et al. (2020). MCM is a highly detailed chemical mechanism containing 142 emitted non-methane VOC species and nearly 17 000 reactions (Jenkin et al., 2015). The MOZART T1 mechanism simplifies the chemistry of larger VOC species by grouping their chemistry into categories of lumped species. These VOCs include BIGALK (alkane species with more than three carbons), BIGENE (alkenes with more than three carbons), and XYLENES (all xylene species and alkyl benzene species but not toluene or benzene). However, the individual VOCs that make up these lumped species are directly represented in MCM v3.3.1 and need to be translated into realistic atmospheric mixing ratios. Initially, this was done by using whole air sampler VOC data collected by UC Irvine during the KORUS-AQ field campaign to determine if the average fraction of the lumped species was represented by an individual species. However, a sensitivity study using MCM v3.3.1 was conducted by setting initial conditions and emissions of the lumped species to 0 to determine if they have a significant role in organic acid production (Fig. S12). The results showed that there were virtually no differences in organic acid mixing ratios when removing the lumped species from the simulations, and therefore the contributions of their chemistry are assumed to be negligible.
3.2.5 Gas–aqueous chemical box model
In addition to the gas-phase box modeling, a simplified gas–aqueous box model was introduced to study the effects of aqueous chemistry on organic acid concentrations for the analyzed pollution event. Detailed information on the aqueous box model can be found in Li et al. (2017) and Barth et al. (2021). Briefly, the gas–aqueous box model contains a simplified gas-phase mechanism with 64 reactants and 168 reactions. Gas–aqueous phase partitioning of low-solubility or slow-reacting species is controlled by their Henry's law coefficients, while high-solubility species (such as HNO3) or fast-reacting species (OH, HO2, NO3 radicals) are controlled by the resistance model developed by Schwartz (1986). The aqueous mechanism contains 45 reactions including conversion of sulfur dioxide (SO2) to SO via hydrogen peroxide (H2O2) and O3, and the oxidation of C1–C3 carbonyls and organic acids via the OH radical.
A limitation of these simulations is that the forward trajectories produced by WRF-Chem contained no cloud LWC, preventing the inclusion of cloud water chemistry along the trajectories despite the observed cloud event at WFM. Therefore, a set of stationary aqueous box model simulations were run at the summit of WFM. Hourly meteorological measurements at the summit (including LWC, temperature, and sea-level pressure) were used to constrain these aqueous simulations. A complication of stationary box models is the need to account for advection of air upwind of a given location. To minimize the potential influence of changing air masses, model runs were limited to 3 h, with 30 min of gas-phase-only chemistry at the beginning of each simulation, assuming negligible advection and emissions in this time frame. The 3 h simulations were run each hour from 30 June 2018 at 12:00 EST to 1 July 2018 at 13:00 EST including periods before, during, and after the polluted cloud event at WFM. Initial conditions of gas-phase species were provided from hourly averaged mixing ratios from the BOXMOX MOZART T1 results within 1° latitude and longitude of WFM. The authors emphasize that while these aqueous modeling methods are highly simplified, the purpose of the aqueous modeling is to determine whether clouds were likely to have had an appreciable impact on organic acid mixing ratios for this pollution event rather than to try to precisely quantify the impact of cloud chemical processing on organic acid concentrations.
4.1 Forward trajectories
There is very little temporal variability in the WRF-Chem trajectory ensembles during the pollution event based on the median trajectory positions for each launch time, consistent with the HYSPLIT back-trajectory results (Fig. 6a). Median trajectories rather than mean values are used, as median values tend to be less sensitive to outliers than mean values (Wilcox, 2012). The ensemble trajectories indicate that many trajectories are within the boundary layer and are influenced by NOx emissions from the Chicago metropolitan area (Fig. 6c). The full set of trajectory ensembles can be found in Fig. S13. The trajectories largely travel eastward, with little horizontal variation between the trajectories at each launch date, indicating minimal uncertainty in the forward-trajectory analysis. Many trajectories experience significant increases in NOx of up to 4 ppbv as the air masses advect over the Chicago metropolitan area, the likely source of the anthropogenic influence on the air mass impacting WFM. Some trajectories (particularly those launched from 29 June 2018 at 10:00 and 12:00 UTC) are also influenced by emissions from Toronto, Ontario, Canada.
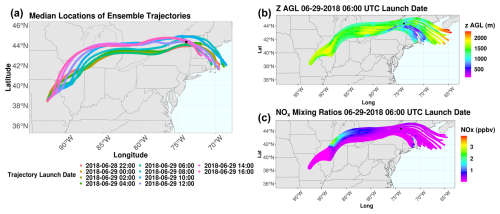
Figure 6(a) Median locations of forward-trajectory ensembles launched in WRF-Chem, colored by launch date. Forward-trajectory ensembles for trajectories launched on 29 June 2018 at 06:00 UTC colored by (b) trajectory height above ground level (m) and (c) NOx mixing ratios. The date format is year–month–day.
Time series of O3 and NOx for each of the 10 launch dates reveal good model agreement between MOZART T1 and MCM v3.3.1 results, indicating that the simpler chemistry within MOZART T1 is sufficient in capturing O3 mixing ratios, which vary only slightly (45–60 ppbv) but typically increase as the simulations progress (Fig. S14). Many of the trajectories launched from Missouri show enhanced mixing ratios of isoprene, with median mixing ratios of up to 5 ppbv (Fig. S15). This is consistent with previous work within the Ozark region of Missouri (Carlton and Baker, 2011; Schwantes et al., 2020) and is exhibited by the WRF-Chem simulations (Fig. S16).
4.2 Formic and acetic acid
4.2.1 HCOOH production
There is significant net production of HCOOH by both chemical mechanisms (MOZART T1 and MCM) for all of the trajectory launch dates, particularly for trajectories launched on 28 June at 22:00 UTC, 29 June at 00:00 UTC, and 29 June at 10:00 UTC, peaking at mixing ratios of 300 pptv (Fig. 7). For all simulations, both mechanisms are in near agreement, with strong production for many sets of trajectories being confined to early in the simulations before mixing ratios become more controlled by background conditions as emitted VOC precursors are exhausted. HCOOH for both mechanisms is almost entirely produced by the ozonolysis of isoprene and isoprene oxidation products, mainly methyl vinyl ketone (MVK) and methacrolein (MACR) (Fig. S17). At low mixing ratios of isoprene (< 500 pptv), ethene (C2H4) becomes the dominant source of HCOOH in MOZART T1, but in these instances, dilution is the major controlling factor. It is worth noting that background mixing ratios of HCOOH are about lower than the peak mixing ratios within the box model simulations, decreasing HCOOH mixing ratios to 100–150 pptv as background air is entrained into the air parcel. The low HCOOH mixing ratios in the background data files are caused by the ozonolysis of isoprene, MVK, and MACR not producing HCOOH within WRF-Chem's MOZART–MOSAIC chemistry mechanism. Using the more comprehensive gas-phase chemistry in the MOZCART mechanism within WRF-Chem (i.e., MOZART T1 plus the GOCART aerosol scheme) increases mixing ratios of HCOOH up to 150 pptv (Fig. S18). The MOZART–MOSAIC chemistry module was used to simulate aerosol and cloud chemistry for this study to have a more complete aerosol and cloud chemistry representation that the MOZCART chemistry option does not include. Since the background files are extracted from WRF-Chem using the MOZART–MOSAIC module, this contributes to a low bias of HCOOH within the box model simulations compared to using the MOZCART mechanism, as discussed in Sect. 4.2.3.
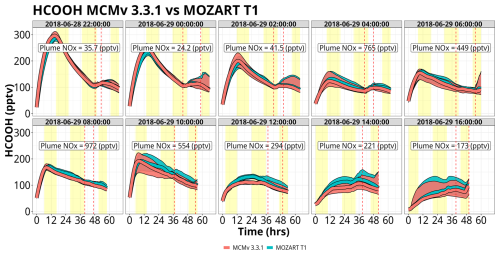
Figure 7Simulation time series of HCOOH mixing ratios for Mozart T1 (blue) and MCM (red) for the WRF-Chem forward-trajectory ensembles, separated by launch time. Red and blue lines represent the median value for the ensemble with the shading representing the interquartile range. Yellow shading represents daylight hours. Vertical dashed lines represent the range of times that the trajectories approach WFM. Plume NOx represents the median NOx mixing ratios when the trajectories are above the Chicago metropolitan area. The date format is year–month–day.
4.2.2 CH3COOH production
The mixing ratios of CH3COOH reach values >1500 pptv, up to 5× greater than those of HCOOH (Fig. 8). MCM produces more CH3COOH than MOZART T1 by up to 500 pptv, with the largest differences occurring within the first few sets of trajectories, i.e., trajectories launched on 28 June at 22:00 UTC, 29 June at 00:00 UTC, and 29 June at 02:00 UTC. However, the disagreement between the two chemical mechanisms largely disappears in the later set of trajectories, particularly for the ensembles influenced by higher NOx mixing ratios (specifically ensembles on 29 June from 04:00–10:00 UTC). The major production pathway (greater than 90 %) for CH3COOH is the reaction of the acetyl peroxy radical (CH3CO3) plus the hydroperoxy radical (HO2) or organic peroxy radicals (RO2). For low-NOx environments, these peroxy radicals can out-compete reactions with NO, increasing the prevalence of this reaction pathway and increasing CH3COOH production (Fig. S19). There are subtle differences in the chemistry between the two mechanisms that contribute to the overall greater production of CH3COOH in MCM. During the first 20 h of all sets of trajectories, mixing ratios of CH3CO3 were approximately the same between the two mechanisms (Fig. S20). However, there are important differences in the reactivity of CH3CO3 within these simulations, particularly as it relates to RO2 radicals. While the overall reactivity of CH3CO3 with RO2 radicals is greater in MOZART T1 (as shown in Fig. S21), a larger proportion of reactions from RO2 radicals in MCM result in CH3COOH formation. MCM treats the rate constant and the yield of CH3COOH from CH3CO3 + RO2 as the methyl peroxy radical (CH3O2), while MOZART T1 has only two RO2 species, CH3O2 and MCO3, that contribute notably to CH3COOH production. Beyond 20 h, CH3CO3 mixing ratios are up to 2 pptv greater in MCM. This is due to 2× greater methylglyoxal production within MCM versus MOZART T1, an important precursor for CH3CO3 from both photolysis and OH (Fig. S22). Disagreements in the rate coefficient for the reaction of OH with peracetic acid also contribute to these discrepancies. Peracetic acid (CH3CO3H) is not a direct source of CH3CO3 but rather serves as a chemical reservoir. The CH3CO3H + OH rate constant is 3.7× greater in MCM compared to MOZART T1, forcing more CH3CO3H to shift back to CH3CO3 and hence more CH3COOH. There is evidence that this reaction's rate constant is even slower than what is used in either model, indicating that CH3CO3H is in reality even more of a permanent sink for CH3CO3 and thus that both mechanisms may overestimate CH3COOH from this pathway (Berasategui et al., 2020).
4.2.3 Comparison of gas-phase chemistry to cloud water observations
In this section we validate the performance of the gas-phase chemical box model by comparing the box model results within 1° latitude and longitude of WFM to the derived gas–aqueous-phase organic acids (Fig. 9). It is assumed that HCOOH and CH3COOH measured in the cloud water were produced entirely in the gas phase and partitioned into cloud droplets rather than being produced in the aqueous phase, already existing within the aerosol that the cloud droplets activated on, or being directly emitted. It is also important to note that bulk cloud water may deviate from Henry's law even if individual cloud droplets may be in equilibrium with the atmosphere. This can be due to differences in pH of individual cloud droplets, mass transfer limitations (especially for highly soluble or reactive species), and changes in equilibrium due to competing reactions (Pandis and Seinfeld, 1991; Winiwarter et al., 1994; Wang et al., 2020). Despite these uncertainties, comparing the BOXMOX results with observations can indicate if the current chemistry represented in the mechanisms can properly model organic acids in the air masses arriving at WFM. Average HCOOH mixing ratios increased from 100 to 200 pptv over the course of the simulations, using both mechanisms, while CH3COOH mixing ratios largely remained constant at approximately 1000 pptv. In spite of the substantial disagreements in gas-phase production between the two mechanisms, MCM exhibited only 100–200 pptv more CH3COOH than MOZART T1 when it arrived at WFM.
The gas-phase box modeling with both MOZART T1 and MCM substantially underestimated both HCOOH and CH3COOH measured in cloud water by approximately an order of magnitude, implying a large missing source of organic acids, which may be from gas, particle, or aqueous phases. As mentioned in Sect. 4.2.1, there is a low bias in the background conditions from the WRF-Chem simulations due to missing ozonolysis reactions of isoprene, MACR, and MVK. However, even the inclusions of the chemistry in the WRF-Chem simulations cannot explain the order-of-magnitude underestimation of HCOOH in the BOXMOX results. These results are consistent with other modeling work investigating organic acids, as gas-phase box models typically underestimate HCOOH and CH3COOH production, implying that gas-phase chemistry alone is not sufficient to properly model these organic acids (Paulot et al., 2011; Millet et al., 2015; Jones et al., 2017). However, the particular reasons remain unclear for these underestimates. Work by Link et al. (2021) found that ecosystems dominated by isoprene produced greater mixing ratios of organic acids than monoterpene-dominated ecosystems, implying that isoprene chemistry not represented in models might be a missing source of HCOOH and CH3COOH. There is also emerging evidence that cloud droplets may play a unique role in the formation of HCOOH that is not being accounted for in these gas-phase simulations. For example, formaldehyde (HCHO) dissolves into cloud droplets, hydrolyzing to form a methanediol, which then partitions back to the gas phase and oxidizes to form HCOOH (Franco et al., 2021). A similar process with other larger aldehydes may be possible, potentially acting as additional sources of larger organic acids.
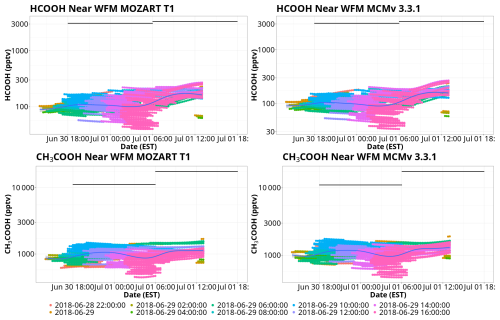
Figure 9Comparisons of model and observational mixing ratios of HCOOH and CH3COOH for MOZART T1 and MCM. Points represent modeled mixing ratios from the trajectory ensembles within 1° of WFM, colored by trajectory launch date. Black lines represent the total (gas–aqueous) mixing ratio estimates derived from 12 h bulk cloud water samples collected at the summit of WFM. The blue line represents a trend line of the BOXMOX results fitted using a generalized additive model. The date format is year–month–day.
4.3 Comparison of gas–aqueous chemistry to cloud water observations
Cloud chemistry can alter organic acid concentrations as distinct from gas-phase chemistry alone. This section examines the impacts of aqueous chemistry by investigating both total mixing ratios and aqueous concentrations of HCOOH and CH3COOH using mixing ratios near WFM to initialize the model (Fig. 10). The total mixing ratios are useful to show the overall change in organic acid concentrations resulting from chemistry in both phases, while the aqueous-phase concentrations can be used to directly compare them with cloud water measurements. Despite large concentrations of CH3COOH in the aqueous phase, CH3COOH mixing ratios change by less than 1 % throughout these simulations, indicating a limited role of chemistry (within the gas or aqueous phase) on the overall CH3COOH produced within these gas–aqueous simulations. However, HCOOH is almost completely depleted within the aqueous phase, driven largely by the ionic HCOO− reacting with the aqueous-phase OH radical, with limited aqueous production from HCHO + OH unable to replace HCOOH. The majority of HCOOH depletion occurs from photochemistry during the daytime, including hours 0–7 and 16–25 of the simulations. Both HCOOH and CH3COOH are greatly underestimated compared to cloud water measurements, similar to the gas-phase-only results. Model and observational discrepancies are also made worse by the aqueous depletion of HCOOH, suggesting an even greater missing source of gas-phase HCOOH, unrepresented aqueous or heterogeneous HCOOH production pathways, or some combination of these processes. These model results imply that gas-to-droplet partitioning is the major source of HCOOH and CH3COOH in cloud water rather than chemical production within cloud droplets. This is confirmed by comparing the rate of gas-to-droplet partitioning to aqueous production, which is 100× and 10 000× greater for HCOOH and CH3COOH respectively.
The depletion of HCOOH deviates from a previous cloud chemistry modeling study at WFM (Barth et al., 2021). The same aqueous chemical mechanism found strong production of HCOOH within cloud water, while a more complex aqueous mechanism, CAPRAM 4.0α, exhibited even stronger production due to reactions involving the aqueous oxidation of CH3CO3H, which is not included in the model used in this study. The differences in model results on different dates imply that cloud water chemistry can be either a net source or net sink of HCOOH depending on the given scenario. The reason for HCOOH depletion in this modeling study remains unclear but likely is related to missing reactions in one or both of the gas and aqueous phases, whose explanation is beyond the scope of this work.
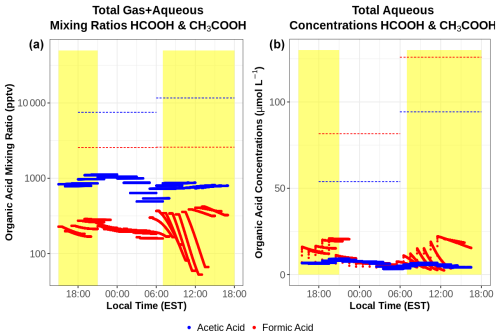
Figure 10Total (gas–aqueous) mixing ratios (a) and aqueous-phase concentrations (b) of HCOOH (red) and CH3COOH (blue) from the simple gas–aqueous box model run at the summit of WFM during a cloud event that occurred from 30 June to 1 July 2018. Dashed horizontal lines represent cloud water concentrations measured at WFM during this period. Total mixing ratios in (a) were derived from the cloud water measurements using Eqs. (1)–(3).
Neither MOZART T1 nor MCM produces OxAc despite its known prevalence, as there is no known gas-phase chemistry that produces OxAc. Current research points to aqueous chemistry being its dominant source, with glyoxal serving as an important precursor (Sorooshian et al., 2006; Lee et al., 2011). Since glyoxal serves as an important precursor gas for organic acid production, it is worth investigating the gas-phase chemistry controlling glyoxal production.
5.1 Glyoxal production
Glyoxal shows complex differences between the two gas-phase mechanisms (Fig. 11). In the first two sets of trajectories, MCM produces up to 2× more glyoxal than MOZART T1, but for later sets of trajectories, such as 29 June 2018 at 08:00 and 10:00 UTC, MOZART T1 produces up to 50 pptv more glyoxal than MCM. The higher glyoxal mixing ratios within MOZART T1 are associated with higher daytime isoprene mixing ratios (greater than 1 ppbv) coupled with higher NOx mixing ratios over the Chicago metropolitan area. Further investigation of the major chemical production pathways between the two mechanisms reveals that MCM predicts considerable ozonolysis chemistry of isoprene oxidation products (including a strong source from the ozonolysis of a hydroperoxy aldehyde or C5HPALD2 in MCM v3.3.1), a source that is not included in MOZART T1 (shown in Fig. S23). Trajectories launched on 28 June 2018 at 22:00 UTC show the strongest nocturnal production within MCM v3.3.1 as the simulation starts towards the end of the day. Photochemistry only has a few hours to oxidize nearly 5 ppbv of isoprene and as a result only produces typically short-lived second-generation oxidation products such as C5HPALD2 (with a chemical lifetime of 1 h when OH = 5×106 molec. cm−3 s−1), which then strongly reacts with O3 at night to form glyoxal.
In trajectories influenced by anthropogenic NOx, such as ensembles launched on 29 June 2018 at 06:00 and 10:00 UTC, a major glyoxal production pathway in MOZART T1 is the reaction of a lumped peroxy radical (XO2) with NO, where XO2 is a lumped species representing peroxy radicals formed in the oxidation of isoprene by-products, including isoprene epoxydiol (IEPOX), hydroperoxy aldehyde (HPALD), and an unsaturated hydroxy hydroperoxide (ISOPOOH), and represents the daytime chemistry that leads to greater glyoxal production in MOZART T1 compared to MCM v3.3.1. Similar to CH3COOH, the disagreements between the two mechanisms largely disappear for glyoxal when trajectories arrive at WFM, as primary VOCs are depleted and glyoxal is oxidized or the air parcel entrains background air (Fig. S24).
5.2 Oxalic acid cloud chemistry
Results of the gas–aqueous modeling find substantial aqueous-phase production of OxAc that corresponds with a sharp aqueous-phase depletion of glyoxal (Fig. 12). OxAc production is confined to the daytime, as the OH radical is the major driver of OxAc production chemistry within the model. The concentrations of OxAc are well within an order of magnitude of measured cloud water concentrations. These simulations suggest that aqueous chemistry of small carbonyl compounds such as glyoxal can largely explain the observed concentration of organic acids such as OxAc. It is important to note that this is a simplified aqueous box model that focuses on two- or three-carbon organic acid chemistry that is better suited for chemical transport models. There are aqueous chemical mechanisms that contain larger organic compounds and more aqueous-phase reactions that likely better capture the chemical complexity in cloud droplets and wet aerosol (McNeill et al., 2012; Mouchel-Vallon et al., 2017; Bräuer et al., 2019). Additionally, other types of chemistry such a transition-metal ion chemistry (Zuo and Hoigne, 1992; Sorooshian et al., 2013) or reactions involving organic nitrogen or organic sulfur compounds (Pratt et al., 2013; Lim et al., 2016) are not included in this mechanism that could have direct or indirect impacts on organic acid formation. Uncertainties of Henry's law for OxAc and precursor gases may also contribute to uncertainties in overall OxAc production. Despite these uncertainties, the model results provide strong evidence that under atmospherically relevant conditions, aqueous chemistry can have major impacts on concentrations of organic acids like OxAc and HCOOH.
6.1 Influence of anthropogenic NOx emissions on organic acid formation
Strong isoprene emissions from Missouri are a major contributor to all three organic acids discussed in this work. However, several air parcels modeled in this study are also influenced by anthropogenic NOx emissions from the Chicago metropolitan area, which impacted the oxidation pathway of isoprene in these simulations. A high-NOx versus low-NOx chemical regime for specific VOCs is often defined by whether RO2 predominately reacts with NO or HO2, which can change the overall oxidation pathway of the VOC. The ratio can serve as a useful proxy for the NOx regime to explore the impacts of anthropogenic NOx on organic acid production. The impact of NOx emissions from the Chicago metropolitan area on HCOOH production is subtle, as the dominant production pathway of HCOOH is from isoprene ozonolysis. NOx, coupled with warm temperatures, is directly related to O3 production, and high NOx could therefore contribute indirectly to HCOOH formation. However there is very little connection between ratios with HCOOH production rates in these simulations (Fig. S25), as the vast majority of HCOOH production in all trajectory ensembles occurred during the first 10–15 h of the simulation – before trajectories reached the Chicago metropolitan area – and the primary VOCs responsible for HCOOH production (mainly isoprene) are largely exhausted. NOx emissions have a more direct impact on CH3COOH production, particularly within MCM, with the production of CH3COOH being reduced by up to 3× for ratios greater than 10 (Fig. S26). This reduction is caused by NO out-competing HO2 and RO2 to react with CH3CO3 due to elevated anthropogenic NOx emissions from the Chicago metropolitan area, thus reducing the major production pathway of CH3COOH. However, like HCOOH, the majority of production of CH3COOH occurs before the trajectories arrive in Chicago, muting the NOx impact on overall production.
It is not possible to directly investigate the role of anthropogenic NOx on OxAc using these simulations, as there is no gas-phase production of OxAc in either mechanism. Instead, glyoxal's NOx dependency can be examined as a proxy for OxAc. Both gas-phase mechanisms show glyoxal production increasing with ratios, with a stronger relationship within MOZART T1 simulations due to the parameterized XO2 + NO reaction (Fig. S27). The timing of the NOx emissions is as important as the strength of the emission sources as it relates to glyoxal. The trajectory ensemble launched on 29 June 2018 at 08:00 UTC exhibited some of the highest NOx mixing ratios (>2 ppbv) in the simulations (Fig. S14), but these emissions arrived mostly at night, muting the impact they could have on glyoxal production. Compare this to the trajectories launched on 29 June 2018 at 10:00 UTC, where anthropogenic NOx contributes to a glyoxal production rate 2× greater than the trajectories from 29 June 2018 at 08:00 UTC in the first 40 h of the simulations despite NOx mixing ratios being approximately 2× smaller. These results indicate that the daytime anthropogenic influence increased overall glyoxal production and its likely oxidation products such as oxalic acid, but this influence was decreased due to the timing of the NOx emissions.
6.2 Modeling uncertainties
There are several processes that may contribute to uncertainties in modeling organic acids that arise from unknowns in both gas-phase and aqueous-phase chemistry as well as the lack of measurements of a suite of trace gases and aerosol composition and concentrations. There are large disagreements between MOZART T1 and MCM v3.3.1 in the production of CH3COOH and glyoxal. While there is mechanism agreement as trajectories arrive at WFM, this agreement is caused by entrainment of background air controlling the CH3COOH and glyoxal mixing ratios rather than similar chemical production rates. Investigating the production of these gases in another location or on a different date would likely lead to different results. While changing the entrainment parameter within the box modeling did not impact the conclusions of this work, changes in this parameter did have an appreciable impact on the magnitude of the organic acid mixing ratios, thus increasing the uncertainty in modeling organic acid production. The model runs underestimating HCOOH and CH3COOH by an order of magnitude imply missing chemistry, but it is unclear if this is due to gas and/or aqueous chemistry.
While the gas–aqueous chemistry model produces measured OxAc concentrations, the model is missing known processes that could serve as OxAc sources such as the oxidation of larger organic compounds (Tilgner and Herrmann, 2010; Barth et al., 2021), sinks such as iron oxalate complexes (Zuo and Hoigne, 1992; Sorooshian et al., 2013; Mouchel-Vallon et al., 2017), or key controls of the oxidant budget like photo-fenton reactions (Deguillaume et al., 2005; Nguyen et al., 2013).
The box model simulations also lack the representation of organic aerosol, which may contribute further uncertain. Organic acids may have already existed within aerosol before cloud formation, providing a direct source of organic acids to cloud water before any chemistry has occurred. Carbonyl compounds have also been detected within aerosol samples (Liu et al., 2022; Wang et al., 2022), which can then be oxidized after cloud droplet activation to form organic acids. WSOC can serve as an important sink for aqueous-phase OH, which can either enhance or reduce organic acid production depending on the number of organic acid precursors available for reaction (Arakaki et al., 2013; Tilgner and Herrmann, 2018).
In addition to uncertainties of modeling components, the lack of field observations of both organic acids and their precursors reduces our ability to constrain organic acid production. Regular monitoring of organic acids and their precursor gases is rare in the northeast United States or elsewhere. VOCs are monitored in networks like the EPA's Photochemical Assessment Monitoring Stations (PAMSs), but they are designed to assess O3 production and are therefore constrained to more populated regions. Whiteface Mountain is the only site in the region that monitors organic acids, and there are no recent gas-phase organic acid measurements in the region, with the most recent known measurements occurring in 1991 (Khwaja, 1995).
6.3 Future work
Future work will investigate the impacts of cloud water chemistry on organic acid production in more detail. Specific attention will be paid to the aqueous-phase depletion of HCOOH and why this result differs from another WFM case study using the same mechanism (Barth et al., 2021). In addition to a more detailed look at the key chemical reactions (i.e., sinks, sources, oxidant budgets) within the simple gas–aqueous-phase mechanism, the aqueous chemistry will be expanded to include key processes that were not represented in this work, including metal–organic complexes and associated photochemistry, photo-fenton chemistry, and the inclusion of larger organic compounds in the mechanisms. This updated chemistry will then be compared to observations to see if the improved mechanism can better describe HCOOH, CH3COOH, and OxAc concentrations.
This study used a combination of WRF-Chem and Lagrangian chemical box modeling to investigate the major chemical processes that impact organic acid formation in both the gas and aqueous phases at Whiteface Mountain (WFM), New York, during a pollution event on 1 July 2018 that led to record-high organic acid concentrations. HYSPLIT ensemble back-trajectory analysis determined that WFM was influenced from central Missouri, a region with strong biogenic VOC emissions, and anthropogenic emissions from the Chicago metropolitan area. WRF-Chem simulations were used to simulate the pollution before and during the event and to launch forward trajectories based on the HYSPLIT results. WRF-Chem was then used to provide input necessary for chemical box modeling along the trajectories. To determine if gas-phase chemistry can explain the organic acid concentrations measured at WFM, the box model BOXMOX was run with two gas-phase mechanisms (the Model for Ozone and Related chemical Tracers or MOZART T1 and the Master Chemical Mechanism or MCM v3.3.1). The MOZART T1 mechanism is a condensed gas-phase mechanism, while MCM v3.3.1 is more detailed, allowing the evaluation of whether MOZART T1 can sufficiently predict organic acid production compared to MCM v3.3.1. The gas-phase box model results were then used as input for a simple gas–aqueous box model run at the summit of WFM to investigate the potential role of aqueous chemistry on organic acids. Strong biogenic emissions of isoprene from Missouri driven by a heat wave were responsible for the strong production of organic acids, with an influence from anthropogenic inputs of NOx from the Chicago metropolitan area.
The two gas-phase mechanisms used in the BOXMOX simulations showed good agreement in HCOOH production, with ozonolysis chemistry from isoprene, MACR, and MVK serving as the major sources. MCM v3.3.1 produced up to 40 % more CH3COOH than MOZART T1 under high-isoprene but low-NOx conditions due to a stronger CH3CO3 + HO2 chemical pathway. The two gas-phase mechanisms differed in their calculation of glyoxal production. MCM v3.3.1 produced more glyoxal from the nocturnal ozonolysis of hydroperoxy aldehyde or C5HPALD2, a low-NOx oxidation product of isoprene, while MOZART T1 produced more glyoxal under higher-NOx conditions where NO + XO2 dominated. The disagreements between the two mechanisms for CH3COOH and glyoxal largely disappear as they arrive at WFM, but this is due to the entrainment of background air dominating mixing ratios after emitted primary VOCs have been exhausted. Both gas-phase mechanisms greatly underpredicted HCOOH and CH3COOH by an order of magnitude in comparison to measurements made at WFM.
To learn how aqueous-phase chemistry could contribute to organic acid formation, a cloud chemistry box model was applied using a simple aqueous-phase mechanism. The gas–aqueous-phase box model shows little change in CH3COOH mixing ratios due to aqueous chemistry but exhibits a significant depletion of HCOOH, exacerbating the gas-phase underpredictions of HCOOH. Glyoxal mixing ratios showed up to 100 pptv between the two mechanisms upwind of WFM, with MCM v3.3.1 producing a large amount, 50–100 pptv, of glyoxal at nighttime from the ozonolysis of an isoprene hydroperoxy aldehyde (C5HPALD2), while MOZART T1 showed 2× greater production of glyoxal during the day from the lumped isoprene oxidation peroxy radical XO2 reaction with NO. Anthropogenic NOx emissions led to increased glyoxal production in both mechanisms, but the effect was stronger within MOZART T1. There is a strong aqueous production of OxAc from carbonyl compounds like glyoxal, with concentrations well within an order of magnitude of cloud water measurements at WFM. The gas–aqueous box modeling indicates that aqueous processing can impact organic acid concentrations.
These results contribute to the limited research indicating that biogenic VOC emissions are a major source of organic acids in the atmosphere, but gas-phase chemistry alone greatly underpredicts their atmospheric concentrations. While the addition of aqueous chemistry does not improve the model predictions of HCOOH and CH3COOH, this study provides further evidence that cloud droplets are a major source of oxalic acid under realistic atmospheric conditions. Only a limited number of modeling studies have looked explicitly at OxAc (Crahan et al., 2004; Ervens et al., 2004; Warneck, 2005; Chen et al., 2007; Myriokefalitakis et al., 2011; Zhu et al., 2020; Barth et al., 2021; Myriokefalitakis et al., 2022) despite its role as a significant component of secondary organic aerosol (SOA) mass. A major reason for this is that most chemical transport models contain either no or a crude representation of organic chemistry within cloud droplets. The lack of representation of aqueous organic chemistry risks the model developing a “clear-sky” bias, a phrase introduced in Christiansen et al. (2020), preventing proper characterization of the chemical properties of organic aerosol.
A large contributing factor to uncertainties in organic acid production is the lack of observational data, particularly organic acids in both the gas and aqueous phases. Regular observational studies over a broader range of geographical and temporal scales are required to better constrain organic acid concentrations. VOC measurements of key organic acid precursors like isoprene, methacrolein, methyl vinyl ketone, and glyoxal, especially in regions of high biogenic volatile organic compound (BVOC) emissions, are needed to better constrain organic acid production. Cloud water chemistry measurements must be expanded beyond organic acids to include key aqueous precursor gases such as glyoxal and methylglyoxal. Simultaneous gas–aqueous-phase field measurements are also necessary, as cloud water measurements alone are not sufficient to properly investigate cloud water processing of organic carbon. Finally modeling work at different temporal and geographic scales coupled with field observations is necessary for improved modeling of organic acids so that the processes governing atmospheric chemistry are better represented. The procedure of back-trajectory analysis that then initializes forward-trajectory runs within WRF-Chem (or another chemical transport model) could be automated to provide insight to researchers during field campaigns and guide laboratory analysis of collected samples to target specific chemical species or processes.
The northeast United States is a region undergoing a significant shift in condensed-phase chemical composition from a SO- and NO-dominated system to an organic-carbon-dominated system, with organic acids representing a larger fraction of total ions in cloud water and rainwater (Lawrence et al., 2023). Because of the trend towards a higher fraction of organic acids in cloud water, it is critical to better understand their production. As the world decarbonizes and anthropogenic emissions of SO2 and NOx decrease, field campaigns and modeling efforts targeting the northeast United States can serve as a blueprint for other regions of the world that are experiencing similar changes in atmospheric composition and chemistry – improving the representation in air quality and climate models of aerosol and precipitation composition – and therefore inform policy decisions.
All model simulations, including WRF-Chem, BOXMOX, and the aqueous box modeling results, are archived in the NCAR Campaign Storage file system (/glade/campaign/acom/acom-weather/barthm/WFM_2018July01case). These datasets can be accessed by contacting the corresponding author. Cloud water chemistry datasets can be accessed at http://atmoschem.asrc.cestm.albany.edu/~cloudwater/pub/Data.htm (Lance, 2024). The postprocessing analysis code can be accessed by contacting the corresponding author.
The supplement related to this article is available online at: https://doi.org/10.5194/acp-24-13693-2024-supplement.
EY and DK conducted the cloud water chemical analysis. PC, RB, CL, and SL conducted the cloud water sampling in 2018. MB and CL conducted WRF-Chem simulations. CL conducted box modeling simulations. JO consulted on box model results. CL and MB wrote the manuscript with contributions from all co-authors.
At least one of the (co-)authors is a member of the editorial board of Atmospheric Chemistry and Physics. The peer-review process was guided by an independent editor, and the authors also have no other competing interests to declare.
The New York State Energy Research and Development Authority (NYSERDA) has not reviewed the information contained herein, and the opinions expressed in this report do not necessarily reflect those of NYSERDA or the state of New York.
Publisher's note: Copernicus Publications remains neutral with regard to jurisdictional claims made in the text, published maps, institutional affiliations, or any other geographical representation in this paper. While Copernicus Publications makes every effort to include appropriate place names, the final responsibility lies with the authors.
The NSF National Center for Atmospheric Research (NCAR) is a major facility sponsored by the U.S. National Science Foundation under cooperative agreement no. 1852977. The authors would like to thank the NCAR Advanced Study Program's Graduate Visitor Program and NCAR's Atmospheric Chemistry Observations and Modeling Laboratory for providing travel funding to allow in-person collaboration between authors Christopher Lawrence, Mary Barth, and John Orlando. We would like to acknowledge the high-performance computing support from Cheyenne (https://doi.org/10.5065/D6RX99HX) provided by NSF NCAR's Computational and Information Systems Laboratory. We acknowledge the use of the WRF-Chem preprocessors tool (mozbc, fire_emiss, biogenic emissions, anthropogenic emissions), provided by NSF NCAR/ACOM. We would also like to thank James Schwab for providing trace gas data from Whiteface Mountain, Pinnacle State Park, and Queens College.
This research has been supported by NASA's Future Investigators in NASA Earth and Space Science and Technology (FINESST) program (award number 80NSSC21K1633) and a NSF's Faculty Early Career Development (CAREER) grant (award number 1945563). Cloud water measurements reported in this paper were supported by the New York State Energy Research and Development Authority (NYSERDA) contract 124461 (2018–2021). WFM trace gas and meteorological measurements were supported by NYSERDA contract 48971.
This paper was edited by Lynn M. Russell and reviewed by two anonymous referees.
Arakaki, T., Anastasio, C., Kuroki, Y., Nakajima, H., Okada, K., Kotani, Y., Handa, D., Azechi, S., Kimura, T., Tsuhako, A., and Miyagi, Y.: A General Scavenging Rate Constant for Reaction of Hydroxyl Radical with Organic Carbon in Atmospheric Waters, Environ. Sci. Technol., 47, 8196–8203, https://doi.org/10.1021/es401927b, 2013. a
Avery, G. B., Tang, Y., Kieber, R. J., and Willey, J. D.: Impact of recent urbanization on formic and acetic acid concentrations in coastal North Carolina rainwater, Atmos. Environ., 35, 3353–3359, https://doi.org/10.1016/S1352-2310(00)00328-9, 2001. a
Barth, M. C., Ervens, B., Herrmann, H., Tilgner, A., McNeill, V. F., Tsui, W. G., Deguillaume, L., Chaumerliac, N., Carlton, A., and Lance, S. M.: Box Model Intercomparison of Cloud Chemistry, J. Geophys. Res.-Atmos., 126, e2021JD035486, https://doi.org/10.1029/2021JD035486, 2021. a, b, c, d, e
Berasategui, M., Amedro, D., Vereecken, L., Lelieveld, J., and Crowley, J. N.: Reaction between CH3C(O)OOH (peracetic acid) and OH in the gas phase: a combined experimental and theoretical study of the kinetics and mechanism, Atmos. Chem. Phys., 20, 13541–13555, https://doi.org/10.5194/acp-20-13541-2020, 2020. a
Blando, J. D. and Turpin, B. J.: Secondary organic aerosol formation in cloud and fog droplets: a literature evaluation of plausibility, Atmos. Environ., 34, 1623–1632, https://doi.org/10.1016/S1352-2310(99)00392-1, 2000. a
Brandt, R. E., Schwab, J. J., Casson, P. W., Roychowdhury, U. K., Wolfe, D., Demerjian, K. L., Civerolo, K. L., Rattigan, O. V., and Felton, H. D.: Atmospheric Chemistry Measurements at Whiteface Mountain, NY: Ozone and Reactive Trace Gases, Aerosol Air Qual. Res., 16, 873–884, https://doi.org/10.4209/aaqr.2015.05.0376, 2016. a, b
Bräuer, P., Mouchel-Vallon, C., Tilgner, A., Mutzel, A., Böge, O., Rodigast, M., Poulain, L., van Pinxteren, D., Wolke, R., Aumont, B., and Herrmann, H.: Development of a protocol for the auto-generation of explicit aqueous-phase oxidation schemes of organic compounds, Atmos. Chem. Phys., 19, 9209–9239, https://doi.org/10.5194/acp-19-9209-2019, 2019. a
Carlton, A. G. and Baker, K. R.: Photochemical Modeling of the Ozark Isoprene Volcano: MEGAN, BEIS, and Their Impacts on Air Quality Predictions, Environ. Sci. Technol., 45, 4438–4445, https://doi.org/10.1021/es200050x, 2011. a
Carlton, A. G., Turpin, B. J., Altieri, K. E., Seitzinger, S., Reff, A., Lim, H.-J., and Ervens, B.: Atmospheric oxalic acid and SOA production from glyoxal: Results of aqueous photooxidation experiments, Atmos. Environ., 41, 7588–7602, https://doi.org/10.1016/j.atmosenv.2007.05.035, 2007. a
Chaliyakunnel, S., Millet, D., Wells, K., Cady-Pereira, K., and Shephard, M.: A Large Underestimate of Formic Acid from Tropical Fires: Constraints from Space-Borne Measurements, Environ. Sci. Technol., 50, 5631–5640, https://doi.org/10.1021/acs.est.5b06385, 2016. a, b
Chen, J., Griffin, R. J., Grini, A., and Tulet, P.: Modeling secondary organic aerosol formation through cloud processing of organic compounds, Atmos. Chem. Phys., 7, 5343–5355, https://doi.org/10.5194/acp-7-5343-2007, 2007. a
Chen, X., Millet, D. B., Neuman, J. A., Veres, P. R., Ray, E. A., Commane, R., Daube, B. C., McKain, K., Schwarz, J. P., Katich, J. M., Froyd, K. D., Schill, G. P., Kim, M. J., Crounse, J. D., Allen, H. M., Apel, E. C., Hornbrook, R. S., Blake, D. R., Nault, B. A., Campuzano-Jost, P., Jimenez, J. L., and Dibb, J. E.: HCOOH in the Remote Atmosphere: Constraints from Atmospheric Tomography (ATom) Airborne Observations, ACS Earth Space Chem., 5, 1436–1454, https://doi.org/10.1021/acsearthspacechem.1c00049, 2021. a
Chen, Y., Hobbs, B. F., Hugh Ellis, J., Crowley, C., and Joutz, F.: Impacts of climate change on power sector NOx emissions: A long-run analysis of the US mid-atlantic region, Energ. Policy, 84, 11–21, https://doi.org/10.1016/j.enpol.2015.04.013, 2015. a
Christiansen, A. E., Carlton, A. G., and Henderson, B. H.: Differences in fine particle chemical composition on clear and cloudy days, Atmos. Chem. Phys., 20, 11607–11624, https://doi.org/10.5194/acp-20-11607-2020, 2020. a
Crahan, K. K., Hegg, D., Covert, D. S., and Jonsson, H.: An exploration of aqueous oxalic acid production in the coastal marine atmosphere, Atmos. Environ., 38, 3757–3764, https://doi.org/10.1016/j.atmosenv.2004.04.009, 2004. a
Decker, Z. C. J., Zarzana, K. J., Coggon, M., Min, K.-E., Pollack, I., Ryerson, T. B., Peischl, J., Edwards, P., Dubé, W. P., Markovic, M. Z., Roberts, J. M., Veres, P. R., Graus, M., Warneke, C., de Gouw, J., Hatch, L. E., Barsanti, K. C., and Brown, S. S.: Nighttime Chemical Transformation in Biomass Burning Plumes: A Box Model Analysis Initialized with Aircraft Observations, Environ. Sci. Technol., 53, 2529–2538, https://doi.org/10.1021/acs.est.8b05359, 2019. a
Deguillaume, L., Leriche, M., and Chaumerliac, N.: Impact of radical versus non-radical pathway in the Fenton chemistry on the iron redox cycle in clouds, Chemosphere, 60, 718–724, https://doi.org/10.1016/j.chemosphere.2005.03.052, 2005. a
Eger, P. G., Schuladen, J., Sobanski, N., Fischer, H., Karu, E., Williams, J., Riva, M., Zha, Q., Ehn, M., Quéléver, L. L. J., Schallhart, S., Lelieveld, J., and Crowley, J. N.: Pyruvic acid in the boreal forest: gas-phase mixing ratios and impact on radical chemistry, Atmos. Chem. Phys., 20, 3697–3711, https://doi.org/10.5194/acp-20-3697-2020, 2020. a
Emmons, L. K., Schwantes, R. H., Orlando, J. J., Tyndall, G., Kinnison, D., Lamarque, J.-F., Marsh, D., Mills, M. J., Tilmes, S., Bardeen, C., Buchholz, R. R., Conley, A., Gettelman, A., Garcia, R., Simpson, I., Blake, D. R., Meinardi, S., and Pétron, G.: The Chemistry Mechanism in the Community Earth System Model Version 2 (CESM2), J. Adv. Model. Earth Sy., 12, e2019MS001882, https://doi.org/10.1029/2019MS001882, 2020. a
Ervens, B., George, C., Williams, J. E., Buxton, G. V., Salmon, G. A., Bydder, M., Wilkinson, F., Dentener, F., Mirabel, P., Wolke, R., and Herrmann, H.: CAPRAM 2.4 (MODAC mechanism): An extended and condensed tropospheric aqueous phase mechanism and its application, J. Geophys. Res.-Atmos., 108, 4426, https://doi.org/10.1029/2002JD002202, 2003. a
Ervens, B., Feingold, G., Frost, G. J., and Kreidenweis, S. M.: A modeling study of aqueous production of dicarboxylic acids: 1. Chemical pathways and speciated organic mass production, J. Geophys. Res.-Atmos., 109, https://doi.org/10.1029/2003JD004387, 2004. a
Fast, J. D., Gustafson Jr., W. I., Easter, R. C., Zaveri, R. A., Barnard, J. C., Chapman, E. G., Grell, G. A., and Peckham, S. E.: Evolution of ozone, particulates, and aerosol direct radiative forcing in the vicinity of Houston using a fully coupled meteorology-chemistry-aerosol model, J. Geophys. Res.-Atmos., 111, D21305, https://doi.org/10.1029/2005JD006721, 2006. a, b
Feltracco, M., Barbaro, E., Spolaor, A., Vecchiato, M., Callegaro, A., Burgay, F., Vardè, M., Maffezzoli, N., Dallo, F., Scoto, F., Zangrando, R., Barbante, C., and Gambaro, A.: Year-round measurements of size-segregated low molecular weight organic acids in Arctic aerosol, Sci. Total Environ., 763, 142954, https://doi.org/10.1016/j.scitotenv.2020.142954, 2021. a
Franco, B., Blumenstock, T., Cho, C., Clarisse, L., Clerbaux, C., Coheur, P.-F., De Mazière, M., De Smedt, I., Dorn, H.-P., Emmerichs, T., Fuchs, H., Gkatzelis, G., Griffith, D. W. T., Gromov, S., Hannigan, J. W., Hase, F., Hohaus, T., Jones, N., Kerkweg, A., Kiendler-Scharr, A., Lutsch, E., Mahieu, E., Novelli, A., Ortega, I., Paton-Walsh, C., Pommier, M., Pozzer, A., Reimer, D., Rosanka, S., Sander, R., Schneider, M., Strong, K., Tillmann, R., Van Roozendael, M., Vereecken, L., Vigouroux, C., Wahner, A., and Taraborrelli, D.: Ubiquitous atmospheric production of organic acids mediated by cloud droplets, Nature, 593, 233–237, https://doi.org/10.1038/s41586-021-03462-x, 2021. a
Fulgham, S. R., Brophy, P., Link, M., Ortega, J., Pollack, I., and Farmer, D. K.: Seasonal Flux Measurements over a Colorado Pine Forest Demonstrate a Persistent Source of Organic Acids, ACS Earth Space Chem., 3, 2017–2032, https://doi.org/10.1021/acsearthspacechem.9b00182, 2019. a
Giovannini, L., Ferrero, E., Karl, T., Rotach, M. W., Staquet, C., Trini Castelli, S., and Zardi, D.: Atmospheric Pollutant Dispersion over Complex Terrain: Challenges and Needs for Improving Air Quality Measurements and Modeling, Atmosphere, 11, 646, https://doi.org/10.3390/atmos11060646, 2020. a
Graedel, T. E. and Eisner, T.: Atmospheric formic acid from formicine ants: a preliminary assessment, Tellus B, 40, 335–339, https://doi.org/10.1111/j.1600-0889.1988.tb00107.x, 1988. a
Grell, G. A., Peckham, S. E., Schmitz, R., McKeen, S. A., Frost, G., Skamarock, W. C., and Eder, B.: Fully coupled “online” chemistry within the WRF model, Atmos. Environ., 39, 6957–6975, https://doi.org/10.1016/j.atmosenv.2005.04.027, 2005. a, b
Herckes, P., Valsaraj, K. T., and Collett, J. L.: A review of observations of organic matter in fogs and clouds: Origin, processing and fate, Atmos. Res., 132–133, 434–449, https://doi.org/10.1016/j.atmosres.2013.06.005, 2013. a
Jenkin, M. E., Young, J. C., and Rickard, A. R.: The MCM v3.3.1 degradation scheme for isoprene, Atmos. Chem. Phys., 15, 11433–11459, https://doi.org/10.5194/acp-15-11433-2015, 2015. a
Jones, B. T., Muller, J., O'Shea, S., Bacak, A., Allen, G., Gallagher, M., Bower, K., Le Breton, M., Bannan, T. J., Bauguitte, S., Pyle, J., Lowry, D., Fisher, R., France, J., Nisbet, E., Shallcross, D., and Percival, C.: Are the Fenno-Scandinavian Arctic Wetlands a Significant Regional Source of Formic Acid?, Atmosphere, 8, 112, https://doi.org/10.3390/atmos8070112, 2017. a
Kawamura, K. and Bikkina, S.: A review of dicarboxylic acids and related compounds in atmospheric aerosols: Molecular distributions, sources and transformation, Atmos. Res., 170, 140–160, https://doi.org/10.1016/j.atmosres.2015.11.018, 2016. a
Kawamura, K., Hoque, M. M. M., Bates, T. S., and Quinn, P. K.: Molecular distributions and isotopic compositions of organic aerosols over the western North Atlantic: Dicarboxylic acids, related compounds, sugars, and secondary organic aerosol tracers, Org. Geochem., 113, 229–238, https://doi.org/10.1016/j.orggeochem.2017.08.007, 2017. a
Khwaja, H. A.: Atmospheric concentrations of carboxylic acids and related compounds at a semiurban site, Atmos. Environ., 29, 127–139, https://doi.org/10.1016/1352-2310(94)00211-3, 1995. a, b
Knote, C., Tuccella, P., Curci, G., Emmons, L., Orlando, J. J., Madronich, S., Baró, R., Jiménez-Guerrero, P., Luecken, D., Hogrefe, C., Forkel, R., Werhahn, J., Hirtl, M., Pérez, J. L., San José, R., Giordano, L., Brunner, D., Yahya, K., and Zhang, Y.: Influence of the choice of gas-phase mechanism on predictions of key gaseous pollutants during the AQMEII phase-2 intercomparison, Atmos. Environ., 115, 553–568, https://doi.org/10.1016/j.atmosenv.2014.11.066, 2015. a, b
Kumar, M., Burrell, E., Hansen, J. C., and Francisco, J. S.: Molecular insights into organic particulate formation, Commun. Chem., 2, 1–10, https://doi.org/10.1038/s42004-019-0183-7, 2019. a
Lance, S.: Whiteface Mountain Cloud Water Chemistry Data, Atmospheric Sciences Research Center [data set], http://atmoschem.asrc.cestm.albany.edu/~cloudwater/pub/Data.htm (last access: 4 December 2024), 2024. a
Lawrence, C. E., Casson, P., Brandt, R., Schwab, J. J., Dukett, J. E., Snyder, P., Yerger, E., Kelting, D., VandenBoer, T. C., and Lance, S.: Long-term monitoring of cloud water chemistry at Whiteface Mountain: the emergence of a new chemical regime, Atmos. Chem. Phys., 23, 1619–1639, https://doi.org/10.5194/acp-23-1619-2023, 2023. a, b, c, d, e
Lee, A. K. Y., Herckes, P., Leaitch, W. R., Macdonald, A. M., and Abbatt, J. P. D.: Aqueous OH oxidation of ambient organic aerosol and cloud water organics: Formation of highly oxidized products, Geophys. Res. Lett., 38, L11805, https://doi.org/10.1029/2011GL047439, 2011. a
Legrand, M., Gros, V., Preunkert, S., Sarda‐Estève, R., Thierry, A.-M., Pépy, G., and Jourdain, B.: A reassessment of the budget of formic and acetic acids in the boundary layer at Dumont d'Urville (coastal Antarctica): The role of penguin emissions on the budget of several oxygenated volatile organic compounds, J. Geophys. Res.-Atmos., 117, D06308, https://doi.org/10.1029/2011JD017102, 2012. a
Li, Y., Barth, M. C., Patton, E. G., and Steiner, A. L.: Impact of In-Cloud Aqueous Processes on the Chemistry and Transport of Biogenic Volatile Organic Compounds, J. Geophys. Res.-Atmos., 122, 11131–11153, https://doi.org/10.1002/2017JD026688, 2017. a
Li, Y., Zhao, J., Wang, Y., Seinfeld, J. H., and Zhang, R.: Multigeneration Production of Secondary Organic Aerosol from Toluene Photooxidation, Environ. Sci. Technol., 55, 8592–8603, https://doi.org/10.1021/acs.est.1c02026, 2021. a
Lim, H.-J., Carlton, A. G., and Turpin, B. J.: Isoprene Forms Secondary Organic Aerosol through Cloud Processing: Model Simulations, Environ. Sci. Technol., 39, 4441–4446, https://doi.org/10.1021/es048039h, 2005. a
Lim, Y. B., Kim, H., Kim, J. Y., and Turpin, B. J.: Photochemical organonitrate formation in wet aerosols, Atmos. Chem. Phys., 16, 12631–12647, https://doi.org/10.5194/acp-16-12631-2016, 2016. a
Link, M. F., Nguyen, T. B., Bates, K., Müller, J.-F., and Farmer, D. K.: Can Isoprene Oxidation Explain High Concentrations of Atmospheric Formic and Acetic Acid over Forests?, ACS Earth Space Chem., 4, 730–740, https://doi.org/10.1021/acsearthspacechem.0c00010, 2020. a
Link, M. F., Brophy, P., Fulgham, S. R., Murschell, T., and Farmer, D. K.: Isoprene versus Monoterpenes as Gas-Phase Organic Acid Precursors in the Atmosphere, ACS Earth Space Chem., 5, 1600–1612, https://doi.org/10.1021/acsearthspacechem.1c00093, 2021. a
Liu, Q., Gao, Y., Huang, W., Ling, Z., Wang, Z., and Wang, X.: Carbonyl compounds in the atmosphere: A review of abundance, source and their contributions to O3 and SOA formation, Atmos. Res., 274, 106184, https://doi.org/10.1016/j.atmosres.2022.106184, 2022. a
Maia-Silva, D., Kumar, R., and Nateghi, R.: The critical role of humidity in modeling summer electricity demand across the United States, Nat. Commun., 11, 1686, https://doi.org/10.1038/s41467-020-15393-8, 2020. a
McNeill, V. F., Woo, J. L., Kim, D. D., Schwier, A. N., Wannell, N. J., Sumner, A. J., and Barakat, J. M.: Aqueous-Phase Secondary Organic Aerosol and Organosulfate Formation in Atmospheric Aerosols: A Modeling Study, Environ. Sci. Technol., 46, 8075–8081, https://doi.org/10.1021/es3002986, 2012. a
Mielnik, A., Link, M., Mattila, J., Fulgham, S. R., and Farmer, D. K.: Emission of formic and acetic acids from two Colorado soils, Environ. Sci.-Proc. Imp., 20, 1537–1545, https://doi.org/10.1039/C8EM00356D, 2018. a
Millet, D. B., Baasandorj, M., Farmer, D. K., Thornton, J. A., Baumann, K., Brophy, P., Chaliyakunnel, S., de Gouw, J. A., Graus, M., Hu, L., Koss, A., Lee, B. H., Lopez-Hilfiker, F. D., Neuman, J. A., Paulot, F., Peischl, J., Pollack, I. B., Ryerson, T. B., Warneke, C., Williams, B. J., and Xu, J.: A large and ubiquitous source of atmospheric formic acid, Atmos. Chem. Phys., 15, 6283–6304, https://doi.org/10.5194/acp-15-6283-2015, 2015. a, b
Miyazaki, Y., Aggarwal, S. G., Singh, K., Gupta, P. K., and Kawamura, K.: Dicarboxylic acids and water-soluble organic carbon in aerosols in New Delhi, India, in winter: Characteristics and formation processes, J. Geophys. Res.-Atmos., 114, D19206, https://doi.org/10.1029/2009JD011790, 2009. a
Mouchel-Vallon, C., Deguillaume, L., Monod, A., Perroux, H., Rose, C., Ghigo, G., Long, Y., Leriche, M., Aumont, B., Patryl, L., Armand, P., and Chaumerliac, N.: CLEPS 1.0: A new protocol for cloud aqueous phase oxidation of VOC mechanisms, Geosci. Model Dev., 10, 1339–1362, https://doi.org/10.5194/gmd-10-1339-2017, 2017. a, b
Mungall, E. L., Abbatt, J. P. D., Wentzell, J. J. B., Wentworth, G. R., Murphy, J. G., Kunkel, D., Gute, E., Tarasick, D. W., Sharma, S., Cox, C. J., Uttal, T., and Liggio, J.: High gas-phase mixing ratios of formic and acetic acid in the High Arctic, Atmos. Chem. Phys., 18, 10237–10254, https://doi.org/10.5194/acp-18-10237-2018, 2018. a
Myriokefalitakis, S., Tsigaridis, K., Mihalopoulos, N., Sciare, J., Nenes, A., Kawamura, K., Segers, A., and Kanakidou, M.: In-cloud oxalate formation in the global troposphere: a 3-D modeling study, Atmos. Chem. Phys., 11, 5761–5782, https://doi.org/10.5194/acp-11-5761-2011, 2011. a
Myriokefalitakis, S., Bergas-Massó, E., Gonçalves-Ageitos, M., Pérez García-Pando, C., van Noije, T., Le Sager, P., Ito, A., Athanasopoulou, E., Nenes, A., Kanakidou, M., Krol, M. C., and Gerasopoulos, E.: Multiphase processes in the EC-Earth model and their relevance to the atmospheric oxalate, sulfate, and iron cycles, Geosci. Model Dev., 15, 3079–3120, https://doi.org/10.5194/gmd-15-3079-2022, 2022. a
Nguyen, T. B., Coggon, M. M., Flagan, R. C., and Seinfeld, J. H.: Reactive Uptake and Photo-Fenton Oxidation of Glycolaldehyde in Aerosol Liquid Water, Environ. Sci. Technol., 47, 4307–4316, https://doi.org/10.1021/es400538j, 2013. a
Ninneman, M., Lu, S., Zhou, X., and Schwab, J.: On the Importance of Surface-Enhanced Renoxification as an Oxides of Nitrogen Source in Rural and Urban New York State, ACS Earth Space Chem., 4, 1985–1992, https://doi.org/10.1021/acsearthspacechem.0c00185, 2020. a
Pandis, S. N. and Seinfeld, J. H.: Should bulk cloudwater or fogwater samples obey Henry's law?, J. Geophys. Res.-Atmos., 96, 10791–10798, https://doi.org/10.1029/91JD01031, 1991. a
Paulot, F., Wunch, D., Crounse, J. D., Toon, G. C., Millet, D. B., DeCarlo, P. F., Vigouroux, C., Deutscher, N. M., González Abad, G., Notholt, J., Warneke, T., Hannigan, J. W., Warneke, C., de Gouw, J. A., Dunlea, E. J., De Mazière, M., Griffith, D. W. T., Bernath, P., Jimenez, J. L., and Wennberg, P. O.: Importance of secondary sources in the atmospheric budgets of formic and acetic acids, Atmos. Chem. Phys., 11, 1989–2013, https://doi.org/10.5194/acp-11-1989-2011, 2011. a, b
Place, B. K., Hutzell, W. T., Appel, K. W., Farrell, S., Valin, L., Murphy, B. N., Seltzer, K. M., Sarwar, G., Allen, C., Piletic, I. R., D'Ambro, E. L., Saunders, E., Simon, H., Torres-Vasquez, A., Pleim, J., Schwantes, R. H., Coggon, M. M., Xu, L., Stockwell, W. R., and Pye, H. O. T.: Sensitivity of northeastern US surface ozone predictions to the representation of atmospheric chemistry in the Community Regional Atmospheric Chemistry Multiphase Mechanism (CRACMMv1.0), Atmos. Chem. Phys., 23, 9173–9190, https://doi.org/10.5194/acp-23-9173-2023, 2023. a
Pratt, K. A., Fiddler, M. N., Shepson, P. B., Carlton, A. G., and Surratt, J. D.: Organosulfates in cloud water above the Ozarks' isoprene source region, Atmos. Environ., 77, 231–238, https://doi.org/10.1016/j.atmosenv.2013.05.011, 2013. a
Pye, H. O. T., Nenes, A., Alexander, B., Ault, A. P., Barth, M. C., Clegg, S. L., Collett Jr., J. L., Fahey, K. M., Hennigan, C. J., Herrmann, H., Kanakidou, M., Kelly, J. T., Ku, I.-T., McNeill, V. F., Riemer, N., Schaefer, T., Shi, G., Tilgner, A., Walker, J. T., Wang, T., Weber, R., Xing, J., Zaveri, R. A., and Zuend, A.: The acidity of atmospheric particles and clouds, Atmos. Chem. Phys., 20, 4809–4888, https://doi.org/10.5194/acp-20-4809-2020, 2020. a
Sander, R.: Compilation of Henry's law constants (version 5.0.0) for water as solvent, Atmos. Chem. Phys., 23, 10901–12440, https://doi.org/10.5194/acp-23-10901-2023, 2023. a, b
Schwab, J. J., Casson, P., Brandt, R., Husain, L., Dutkewicz, V., Wolfe, D., Demerjian, K. L., Civerolo, K. L., Rattigan, O. V., Felton, H. D., and Dukett, J. E.: Atmospheric Chemistry Measurements at Whiteface Mountain, NY: Cloud Water Chemistry, Precipitation Chemistry, and Particulate Matter, Aerosol Air Qual. Res., 16, 841–854, https://doi.org/10.4209/aaqr.2015.05.0344, 2016. a
Schwantes, R. H., Emmons, L. K., Orlando, J. J., Barth, M. C., Tyndall, G. S., Hall, S. R., Ullmann, K., St. Clair, J. M., Blake, D. R., Wisthaler, A., and Bui, T. P. V.: Comprehensive isoprene and terpene gas-phase chemistry improves simulated surface ozone in the southeastern US, Atmos. Chem. Phys., 20, 3739–3776, https://doi.org/10.5194/acp-20-3739-2020, 2020. a, b
Schwartz, S. E.: Mass-Transport Considerations Pertinent to Aqueous Phase Reactions of Gases in Liquid-Water Clouds, in: Chemistry of Multiphase Atmospheric Systems, edited by: Jaeschke, W., NATO ASI Series, 415–471, Springer, Berlin, Heidelberg, ISBN 978-3-642-70627-1, https://doi.org/10.1007/978-3-642-70627-1_16, 1986. a
Seinfeld, J. H. and Pandis, S. N.: Atmospheric Chemistry and Physics: From Air Pollution to Climate Change, Wiley, 3rd edn., ISBN 978-1-118-94740-1, 2016. a
Sorooshian, A., Varutbangkul, V., Brechtel, F. J., Ervens, B., Feingold, G., Bahreini, R., Murphy, S. M., Holloway, J. S., Atlas, E. L., Buzorius, G., Jonsson, H., Flagan, R. C., and Seinfeld, J. H.: Oxalic acid in clear and cloudy atmospheres: Analysis of data from International Consortium for Atmospheric Research on Transport and Transformation 2004, J. Geophys. Res.-Atmos., 111, D23S45, https://doi.org/10.1029/2005JD006880, 2006. a, b
Sorooshian, A., Ng, N. L., Chan, A. W. H., Feingold, G., Flagan, R. C., and Seinfeld, J. H.: Particulate organic acids and overall water-soluble aerosol composition measurements from the 2006 Gulf of Mexico Atmospheric Composition and Climate Study (GoMACCS), J. Geophys. Res.-Atmos., 112, D13201, https://doi.org/10.1029/2007JD008537, 2007. a
Sorooshian, A., Wang, Z., Coggon, M. M., Jonsson, H. H., and Ervens, B.: Observations of Sharp Oxalate Reductions in Stratocumulus Clouds at Variable Altitudes: Organic Acid and Metal Measurements During the 2011 E-PEACE Campaign, Environ. Sci. Technol., 47, 7747–7756, https://doi.org/10.1021/es4012383, 2013. a, b
Souza, S. R., Vasconcellos, P. C., and Carvalho, L. R. F.: Low molecular weight carboxylic acids in an urban atmosphere: Winter measurements in São Paulo City, Brazil, Atmos. Environ., 33, 2563–2574, https://doi.org/10.1016/S1352-2310(98)00383-5, 1999. a
Stein, A. F., Draxler, R. R., Rolph, G. D., Stunder, B. J. B., Cohen, M. D., and Ngan, F.: NOAA's HYSPLIT Atmospheric Transport and Dispersion Modeling System, B. Am. Meteorol. Soc., 96, 2059–2077, https://doi.org/10.1175/BAMS-D-14-00110.1, 2015. a, b
Stone, B. J., Gronlund, C. J., Mallen, E., Hondula, D., O'Neill, M. S., Rajput, M., Grijalva, S., Lanza, K., Harlan, S., Larsen, L., Augenbroe, G., Krayenhoff, E. S., Broadbent, A., and Georgescu, M.: How Blackouts during Heat Waves Amplify Mortality and Morbidity Risk, Environ. Sci. Technol., 57, 8245–8255, https://doi.org/10.1021/acs.est.2c09588, 2023. a
Tan, Y., Carlton, A. G., Seitzinger, S. P., and Turpin, B. J.: SOA from methylglyoxal in clouds and wet aerosols: Measurement and prediction of key products, Atmos. Environ., 44, 5218–5226, https://doi.org/10.1016/j.atmosenv.2010.08.045, 2010. a
Tao, Y. and Murphy, J. G.: Evidence for the Importance of Semivolatile Organic Ammonium Salts in Ambient Particulate Matter, Environ. Sci. Technol., 53, 108–116, https://doi.org/10.1021/acs.est.8b03800, 2019. a
Tian, Y., LaFarr, M., Yun, J., Civerolo, K., Hao, W., Zalewsky, E., and Zhou, L.: Analyzing Meteorological and Chemical Conditions for Two High Ozone Events Over the New York City and Long Island Region, in: IGARSS 2020–2020 IEEE International Geoscience and Remote Sensing Symposium, 5537–5540, https://doi.org/10.1109/IGARSS39084.2020.9324470, 2020. a
Tilgner, A. and Herrmann, H.: Radical-driven carbonyl-to-acid conversion and acid degradation in tropospheric aqueous systems studied by CAPRAM, Atmos. Environ., 44, 5415–5422, https://doi.org/10.1016/j.atmosenv.2010.07.050, 2010. a, b
Tilgner, A. and Herrmann, H.: Tropospheric Aqueous-Phase OH Oxidation Chemistry: Current Understanding, Uptake of Highly Oxidized Organics and Its Effects, in: Multiphase Environmental Chemistry in the Atmosphere, Vol. 1299 of ACS Symposium Series, 49–85, American Chemical Society, ISBN 978-0-8412-3363-8, https://doi.org/10.1021/bk-2018-1299.ch004, 2018. a
Tran, T., Kumar, N., and Knipping, E.: Investigating sensitivity of ozone to emission reductions in the New York City (NYC) metropolitan and downwind areas, Atmos. Environ., 301, 119675, https://doi.org/10.1016/j.atmosenv.2023.119675, 2023. a
Travis, K. R., Jacob, D. J., Fisher, J. A., Kim, P. S., Marais, E. A., Zhu, L., Yu, K., Miller, C. C., Yantosca, R. M., Sulprizio, M. P., Thompson, A. M., Wennberg, P. O., Crounse, J. D., St. Clair, J. M., Cohen, R. C., Laughner, J. L., Dibb, J. E., Hall, S. R., Ullmann, K., Wolfe, G. M., Pollack, I. B., Peischl, J., Neuman, J. A., and Zhou, X.: Why do models overestimate surface ozone in the Southeast United States?, Atmos. Chem. Phys., 16, 13561–13577, https://doi.org/10.5194/acp-16-13561-2016, 2016. a
U.S. EPA: Air Quality System Data Mart, http://www.epa.gov/ttn/airs/aqsdatamar (last access: 20 September 2024), 2024. a
Wang, M., Perroux, H., Fleuret, J., Bianco, A., Bouvier, L., Colomb, A., Borbon, A., and Deguillaume, L.: Anthropogenic and biogenic hydrophobic VOCs detected in clouds at the puy de Dôme station using Stir Bar Sorptive Extraction: Deviation from the Henry's law prediction, Atmos. Res., 237, 104844, https://doi.org/10.1016/j.atmosres.2020.104844, 2020. a
Wang, Z., Chen, X., Liang, Y., and Shi, Q.: Molecular characterization of carbonyl compounds in atmospheric fine particulate matters (PM2.5) in Beijing by derivatization with Girard's reagent T combined with positive-ion ESI Orbitrap MS, Atmos. Res., 273, 106176, https://doi.org/10.1016/j.atmosres.2022.106176, 2022. a
Warneck, P.: Multi-Phase Chemistry of C2 and C3 Organic Compounds in the Marine Atmosphere, J. Atmos. Chem., 51, 119–159, https://doi.org/10.1007/s10874-005-5984-7, 2005. a, b
Wilcox, R. R.: Introduction to robust estimation and hypothesis testing, Statistical modeling and decision science, Academic Press, Amsterdam, Boston, 3rd edn., ISBN 978-0-12-386983-8, 2012. a
Winiwarter, W., Fierlinger, H., Puxbaum, H., Facchini, M. C., Arends, B. G., Fuzzi, S., Schell, D., Kaminski, U., Pahl, S., Schneider, T., Berner, A., Solly, I., and Kruisz, C.: Henry's law and the behavior of weak acids and bases in fog and cloud, J. Atmos. Chem., 19, 173–188, https://doi.org/10.1007/BF00696588, 1994. a
Wolfe, G. M., Marvin, M. R., Roberts, S. J., Travis, K. R., and Liao, J.: The Framework for 0-D Atmospheric Modeling (F0AM) v3.1, Geosci. Model Dev., 9, 3309–3319, https://doi.org/10.5194/gmd-9-3309-2016, 2016. a
Yao, X., Fang, M., Chan, C. K., Ho, K. F., and Lee, S. C.: Characterization of dicarboxylic acids in PM2.5 in Hong Kong, Atmos. Environ., 38, 963–970, https://doi.org/10.1016/j.atmosenv.2003.10.048, 2004. a
Yuan, B., Veres, P. R., Warneke, C., Roberts, J. M., Gilman, J. B., Koss, A., Edwards, P. M., Graus, M., Kuster, W. C., Li, S.-M., Wild, R. J., Brown, S. S., Dubé, W. P., Lerner, B. M., Williams, E. J., Johnson, J. E., Quinn, P. K., Bates, T. S., Lefer, B., Hayes, P. L., Jimenez, J. L., Weber, R. J., Zamora, R., Ervens, B., Millet, D. B., Rappenglück, B., and de Gouw, J. A.: Investigation of secondary formation of formic acid: urban environment vs. oil and gas producing region, Atmos. Chem. Phys., 15, 1975–1993, https://doi.org/10.5194/acp-15-1975-2015, 2015. a
Zhang, H., Kupiainen-Määttä, O., Zhang, X., Molinero, V., Zhang, Y., and Li, Z.: The enhancement mechanism of glycolic acid on the formation of atmospheric sulfuric acid–ammonia molecular clusters, J. Chem. Phys., 146, 184308, https://doi.org/10.1063/1.4982929, 2017. a
Zhang, R., Suh, I., Zhao, J., Zhang, D., Fortner, E. C., Tie, X., Molina, L. T., and Molina, M. J.: Atmospheric New Particle Formation Enhanced by Organic Acids, Science, 304, 1487–1490, https://doi.org/10.1126/science.1095139, 2004. a
Zhu, Y., Tilgner, A., Hoffmann, E. H., Herrmann, H., Kawamura, K., Yang, L., Xue, L., and Wang, W.: Multiphase MCM–CAPRAM modeling of the formation and processing of secondary aerosol constituents observed during the Mt. Tai summer campaign in 2014, Atmos. Chem. Phys., 20, 6725–6747, https://doi.org/10.5194/acp-20-6725-2020, 2020. a
Zuo, Y. and Hoigne, J.: Formation of hydrogen peroxide and depletion of oxalic acid in atmospheric water by photolysis of iron(III)-oxalato complexes, Environ. Sci. Technol., 26, 1014–1022, https://doi.org/10.1021/es00029a022, 1992. a, b