the Creative Commons Attribution 4.0 License.
the Creative Commons Attribution 4.0 License.
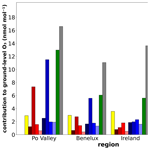
Ozone source attribution in polluted European areas during summer 2017 as simulated with MECO(n)
Markus Kilian
Volker Grewe
Patrick Jöckel
Astrid Kerkweg
Andreas Zahn
Helmut Ziereis
Emissions of land transport and anthropogenic non-traffic emissions (e.g. industry, households and power generation) are significant sources of nitrogen oxides, carbon monoxide and volatile organic compounds (VOCs). These emissions are important precursors of tropospheric ozone and affect air quality. The contribution of the emission sectors to ozone cannot be measured directly but can only be calculated using sophisticated atmospheric chemistry models. For this study we apply the MECO(n) model system (MESSy-fied ECHAM and COSMO models nested n times) equipped with a source attribution method to investigate the contribution of various sources to ground-level ozone in Europe. Compared to previous source apportionment studies for Europe, for the first time we apply a combined NOx–VOC tagging implemented in an online nested global–regional chemistry–climate model to achieve a finer resolution over central Europe (12 km) but concurrently incorporating the effect of long-range transport. We distinguish 10 different source sectors and 4 geographical source regions, analysing especially the contribution from the land transport sector. Our analysis focuses on large ozone events during summer in four different regions, two major polluted regions (Po Valley and Benelux) and two more remote regions (Iberian Peninsula and Ireland). The analysis concentrates on results for summer 2017, during which measurement campaign EMeRGe took place. Measurement data from this campaign are used for model evaluation. Our analysis shows that European land transport emissions contribute largely (42 % and 44 %, respectively) to ground-level NOy mixing ratios over Benelux and the Po Valley. Due to the overall lower ozone production efficiency over Benelux compared to the Po Valley, however, the contributions to ground-level ozone are larger in the Po Valley (12 %) compared to Benelux (8 %). In line with previous publications using different source apportionment methods, our results underline the large importance of long-range transport of ozone, especially from North America (Benelux, Ireland), but also from Africa (Iberian Peninsula), and provide additional information about the sectoral contribution not available before. Our analysis shows that the contributions of European emissions from land transport and anthropogenic non-traffic sectors strongly increase with increasing values of MDA8 (daily maximum 8 h average) ozone over the Po Valley and in the Benelux region. Accordingly, these two sectors drive large MDA8 values in these regions. Inter-comparisons of results for 2018 and with a coarser model resolution (50 instead of 12 km) show that these results are robust with respect to inter-annual variability and model resolution. Comparing our results with results from other source attribution methods we find that the contributions to ozone from individual sectors, which have large NOx but rather low VOC emissions, are estimated to be lower, if their emissions of NOx and VOCs are regarded concurrently.
- Article
(5429 KB) - Full-text XML
-
Supplement
(50420 KB) - BibTeX
- EndNote
Tropospheric ozone contributes to global warming by absorption of radiation (Myhre et al., 2013), and it is harmful for human health and plants (World Health Organisation, 2003; Jimenez-Montenegro et al., 2021). Extreme ozone events mostly occur during heat waves in major polluted areas and have detrimental effects on human health. The most important sources of tropospheric ozone are the downward transport from the stratosphere and the in situ production from precursors such as carbon monoxide (CO), methane (CH4), nitrogen oxides (NOx = NO2 + NO) and volatile organic compounds (VOCs; Haagen-Smith, 1952; Monks, 2005). VOCs comprise a wide range of compounds, which contain carbon and hydrogen atoms, where the non-methane compounds are summarized as non-methane hydrocarbons (NMHCs). The ozone precursors arise from anthropogenic sources, such as land transport (railway, inland navigation and road traffic; Mertens et al., 2020b; Hoor et al., 2009), industry (Ou et al., 2020) and shipping (e.g. Jonson et al., 2020; Matthias et al., 2016; Aulinger et al., 2016; Eyring et al., 2010) as well as from natural sources, such as lightning (Hauglustaine et al., 2001; Schumann and Huntrieser, 2007), wildfires (Di Carlo et al., 2015) and soil bacteria (Yienger and Levy, 1995; Vinken et al., 2014). In particular, the natural sources are subject to large uncertainties because emissions can not be directly measured, and processes determining the emission fluxes have not yet been fully understood (Tost et al., 2007; Mebust et al., 2011; Yienger and Levy, 1995; Vinken et al., 2014). Since the ozone chemistry is non-linear (Seinfeld and Pandis, 2006), the contribution of different precursors to ozone can only be estimated with the help of numerical models.
Typically, two different methods are applied to answer two different scientific questions with respect to the effect of emissions from different sectors on tropospheric ozone. The perturbation method investigates the change of ozone due to an emission reduction (or increase) by comparing the results of a reference simulation with those of a simulation with changed emissions. This yields the impact of the reduced/increased emission sector on ozone. The source attribution method (called tagging), in contrast, decomposes ozone and ozone precursors into the shares from various emission sources. This share is the contribution of the emission sector. Due to their different concepts, these methods answer different questions (see also Mertens et al., 2020b, Table 1). Wang et al. (2009), Grewe et al. (2010) and Clappier et al. (2017) compared the source attribution method with the perturbation method. They found that the perturbation method is inappropriate for source attribution because it quantifies the change of O3 due to an emission change. In contrast, the source attribution method does not provide information about the sensitivity of O3 to emission change. For this reason, the perturbation method is more appropriate to address future emission policies, rather than calculating the sector-wise contributions to tropospheric O3. Thus, for studies which aim to distinguish between different emission sources and the spatial origin of the emissions, the source attribution method is indispensable.
Various studies have analysed the effects of specific sources or geographical origins of ozone precursors on European ozone levels, focusing for example on anthropogenic sources in general (e.g. Tagaris et al., 2015; Karamchandani et al., 2017; Pay et al., 2019), long-range transport (e.g. Fiore et al., 2009; Derwent et al., 2015; Jonson et al., 2018), biogenic sources (e.g. Simpson, 1995; Solmon et al., 2004) and shipping (e.g. Matthias et al., 2010; Aulinger et al., 2016; Jonson et al., 2020; Fink et al., 2023).
Despite the importance of land transport emissions for ground-level ozone over Europe, few studies have focused on this sector mainly analysing the impact of changed land transport emission on ozone using global models. These studies showed a significant impact of land transport emissions on ground-level ozone over Europe (Granier and Brasseur, 2003; Matthes et al., 2007; Hoor et al., 2009; Mertens et al., 2018). Fewer studies investigated the contribution of land transport emissions to ground-level ozone based on a source attribution method in global models (e.g. Dahlmann et al., 2011; Grewe et al., 2017; Mertens et al., 2018) or using various methods with regional models focusing on Europe (Tagaris et al., 2015; Karamchandani et al., 2017; Pay et al., 2019). However, all of these regional studies have only applied source apportionment in the regional model; a sectoral apportionment of ozone from long-distance transport has not been addressed.
Lupaşcu and Butler (2019) overcame this limitation and performed a source attribution for Europe including various geographical source regions in Europe and the global source region. For this they applied boundary conditions to the regional model from a global model simulation including a source attributions method. They found that local emission sources account for 41 % and 38 % to MDA8 ozone exceedance days in the Po Valley and in Germany, respectively. Lupaşcu and Butler (2019), however, combined anthropogenic emissions from traffic, industry, power generation, etc. into one sector and therefore did not provide source attribution results for traffic emissions separately. In comparison to this, Mertens et al. (2020b) investigated the contribution of land transport emissions to ground-level ozone, with a focus on the transport sector, accounting for contributions from land transport emissions in Europe and from other regions of the world. Especially in the Po Valley, they report large contributions from land transport emissions. Further, they showed that during events with large ozone mixing ratios, the contribution of land transport peaks at up to 28 %. Mertens et al. (2020b), however, did not perform a geographical source attribution for land transport emissions, i.e. they could not discriminate between contributions from European land transport emissions and emissions from other regions of the world.
To overcome this limitation, our study adds to the results of Mertens et al. (2020b) by the following:
-
by separating anthropogenic non-traffic from land transport emissions, by further separating those between four geographical source regions (Europe, North America, east Asia and the rest of the world);
-
by applying a more recent emission inventory (EDGAR 5) and considering more recent years (emissions for 2015);
-
by applying a finer spatial resolution for Europe (0.11° instead of 0.44°); and
-
by investigating also MDA8 ozone values.
In comparison to Lupaşcu and Butler (2019), we apply a different approach for ozone source attribution, accounting for contributions of NOx and VOC precursors concurrently, instead of tagging precursors from NOx only. In addition, we focus on the specific role of land transport emissions because these emissions are an important source of ozone precursors over Europe.
By providing the shares of different emission sectors and regions to ground-level ozone and its precursors this work helps to better understand the origin of large ozone events. Specifically, the following scientific questions are investigated:
-
How do the various emission sectors contribute to NOy and O3 in the major polluted regions Benelux and the Po Valley and how does this differ in comparison to more remote regions such as the Iberian Peninsula and Ireland?
-
How large are the contributions from European emissions compared to the contributions from long-range-transported emissions to ground-level O3?
Thereby, we are interested in those emission sectors with the largest ground-level ozone share because mitigating the emissions of these sectors has the largest potential in reducing ozone. These shares provide some preliminary hints on mitigation potentials but not the effects of mitigation measures. Due to the non-linear responses of ozone chemistry on emission changes perturbation simulations are required to analyse the effect of emission changes on ozone. Such simulations are not considered in the present study.
To answer the questions, we apply the MECO(n) (MESSy-fied ECHAM and COSMO models nested n times) model. MECO(n) is an online-coupled global–regional chemistry–climate model, which allows regionally finer resolutions in order to understand regional processes better. The global model is important, to consistently represent the long-range transport across the boundaries of the embedded regional model. Moreover, we use the same chemical mechanism and the same source attribution method in the global and all regional model instances, namely the tagging method described by Grewe et al. (2017) and Rieger et al. (2018), which allows us to quantify the contributions of reactive nitrogen (NOy; see Sect. S1 in the Supplement for a definition), CO and VOC emissions to O3.
This paper is organized as follows: Sect. 2 gives an overview of the modelling system and explains the model setup used for the simulations. In Sect. 3 we present an evaluation of the MECO(n) data with other model data, air quality station measurements and measurements from the German research aircraft HALO. The source attribution results are presented in Sects. 4 to 6. Finally, the results are discussed in detail in Sect. 7, and Sect. 8 summarizes the most important results and answers the research questions.
2.1 Model description
For the present study we apply the MECO(n) model system, which couples two model components online: the global chemistry–climate model EMAC (Jöckel et al., 2010, 2016) and the regional chemistry–climate model COSMO-CLM/MESSy (version COSMO 5.0.0_clm16; Kerkweg and Jöckel, 2012). The core atmospheric model used in COSMO-CLM/MESSy is the COSMO-CLM model (Rockel et al., 2008), a regional atmospheric climate model, which is based on the COSMO (Consortium for Small-scale Modelling) model and jointly further developed by the CLM-Community. We use EMAC (ECHAM5 version 5.3.02) in the T42L90MA resolution, i.e. with a spherical truncation of T42 (corresponding to a quadratic Gaussian grid of approximately 2.8° × 2.8° in latitude and longitude) with 90 vertical hybrid pressure levels up to 0.01 hPa. EMAC is operated with a time step length of 720 s. For the simulations we used MESSy in the version 2.55.2-1913. We applied a MECO(2) setup featuring one COSMO/MESSy instance over Europe with a resolution of 0.44° × 0.44° (≈ 50 km, named CM50) and a further instance nested in CM50 covering central Europe with a resolution of 0.11° × 0.11° (≈ 12 km, named CM12). The time step length of CM50 is 240 s, and that of CM12 is 120 s. Both COSMO-CLM/MESSy instances have 40 vertical model levels (terrain following) with geometric height as the vertical coordinate. The height of the uppermost model level is ≈ 22 km; the damping zone starts at 11 km, and the lowest model layer is ≈ 20 m thick. The boundary conditions for CM50 are provided by EMAC, and the boundary conditions for CM12 are provided by CM50 (Mertens et al., 2020a). To facilitate a one-to-one comparison with observations, EMAC is “nudged” by a Newtonian relaxation of the temperature, the divergence, the vorticity and the logarithm of surface pressure (Jöckel et al., 2006) towards ERA5 reanalysis data (Hersbach et al., 2020). Sea surface temperature and sea ice coverage are prescribed as boundary conditions for the simulation setup from ERA5 as well. Due to the MESSy infrastructure, the same diagnostics and chemical process descriptions are applied in all model instances.
2.2 Methodology
In this study we use the TAGGING submodel developed by Grewe et al. (2017) and Rieger et al. (2018). The tagging method applies the combinatorical approach described by Grewe (2013). The TAGGING method tags CO, PAN, O3, OH, HO2, and the two families of NOy and NMHCs. The family approach for NOy and NMHCs is chosen for a reduction of computational demands. Detailed definitions of the families are provided by Grewe et al. (2017). The source attribution method allows a separation of the emissions by their emission sector and geographical origin. We differentiate 10 sources of ozone (anthropogenic and natural emission sectors or processes). In addition we subdivide the emission sectors land transport and anthropogenic non-traffic into four geographical source regions: Europe (EU), North America (NA), east Asia (EA) and the rest of the world (ROW) to distinguish between O3 from regional sources (i.e. same continent) and from long-range transport. In total this gives us 16 tagging categories into which ozone and its precursors are decomposed. Table 1 lists the tagging categories in detail. The geographical distribution of the source attribution regions is shown in Fig. S18 in the Supplement, and details on the technical implementation of the geographical source attribution are given in Sect. S3 in the Supplement.
Table 1Description of the different tagging categories applied in this study following Grewe et al. (2017). Please note that some tagging categories summarize different emission sectors (see description). The last column shows the nomenclature of the tagged ozone tracers as used in this study. The nomenclature of other species is accordingly.
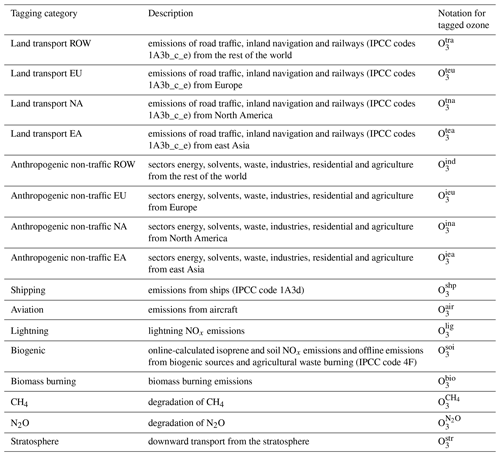
The MECO(2) simulation was performed in the QCTM (quasi-chemistry-transport model) mode, which means that the chemistry does not affect the meteorology in global and regional model instances (Deckert et al., 2011; Mertens et al., 2016). The method ensures the same simulated meteorological conditions if different emission inventories are used. The usage of the QCTM mode is important for follow-up studies with different emission inventories but not of concern for the present study.
The chemical mechanism applied with the submodel MECCA (Module Efficiently Calculating the Chemistry of the Atmosphere) considers the basic gas-phase chemistry of ozone, methane, odd nitrogen and other reactants, as described by Sander et al. (2011) and Jöckel et al. (2016). We use the CCMI2-base-02-tag.bat mechanism, which is based on the mechanism described by Jöckel et al. (2016). It includes basic NOx-CO-CH4-O3 chemistry including the chemistry of isoprene C5H8 and non-methane hydrocarbons (NMHCs) up to 4 carbon atoms. The halogen chemistry includes bromine and chlorine species. The chemistry of sulfur is also considered. In comparison to Jöckel et al. (2016) the mechanism has been slightly extended including additional halocarbons and reactions of acetonitrile (CH3CN) with OH, O1D and Cl). The chemical mechanisms for gas-phase and aqueous-phase chemistry (the latter required for scavenging and wet deposition as described by Tost et al., 2006, 2010) are included in the Supplement.
We calculate emissions of NOx by lightning only on the global scale, using the parametrization by Grewe et al. (2001). In CM50 and CM12 we use the emissions from EMAC (i.e. with the same geographical, vertical and temporal distribution), by transforming the emission flux online onto the grids of CM50 and CM12, respectively. This approach allows us to use the same amount and the same spatial–temporal distribution of the lightning NOx emissions in all model instances (see also Mertens et al., 2016).
For anthropogenic emissions we applied the EDGAR (Emissions Database for Global Atmospheric Research, version 5.0) inventory for the year 2015 with a monthly time resolution (Crippa et al., 2019b, 2020). The EDGARv5.0 inventory is based on international energy balances, agricultural statistics of the FAO (Food and Agriculture Organization of the United Nations), and regional or national assumptions on technology use and emission control standards (Crippa et al., 2019b). The data set is calculated using a consistent bottom-up approach. In our own pre-processing the EDGAR emissions are vertically distributed after Mailler et al. (2013), which is based on Bieser et al. (2011). The detailed description of the vertical distribution is given in Sect. S4 in the Supplement.
The biomass burning emissions are included using the Copernicus Atmosphere Monitoring Service Global Fire Assimilation System (CAMS GFAS, version 1.2) data set from ECMWF (European Centre for Medium-Range Weather Forecasts; Di Giuseppe et al., 2018). In order to represent the vertical distribution of the wildfire emissions in an appropriate way, the data were pre-processed and vertically distributed onto six height levels after Dentener et al. (2006) depending on the geographical region (see Sect. S5 in the Supplement). Soil NOx and biogenic isoprene emissions are calculated by the submodel ONline EMISsions (ONEMIS; Kerkweg et al., 2006)) following the parametrizations of Yienger and Levy (1995) and Guenther et al. (2006), respectively. Table S1 in the Supplement lists the annual and summer totals of NO emissions in EMAC for the used emission inventories and parametrizations (e.g. lightning).
The simulation period for EMAC and CM50 spans December 2016 until February 2019. The finer nested instance CM12 was applied only for the summer months of June, July and August (JJA) 2017 and 2018 as well as March and April 2018. These time periods were selected to match two aircraft measurement campaigns, EMeRGe (July 2017) and EMeRGe Asia (March 2018; Andrés Hernández et al., 2022), which provide valuable observation data for the model evaluation.
The MECO(n) model has been evaluated in detail by Hofmann et al. (2012) and Mertens et al. (2016). Therefore, here we focus on a short evaluation of the model performance for the period of interest.
We evaluated the model results using the seasonal ozone daily maximum 8 h mixing ratio (OSMDA8) data product based on ground-level observations and results of chemistry–climate models by Delang et al. (2021), ground-level observations of ozone and NOx, and aircraft in situ observations of NOy and O3 during the HALO EMeRGe Europe measurement campaign (Andrés Hernández et al., 2022).
The comparison of the model results to the observations and model-based data fusion product by Delang et al. (2021) reveals that OSMDA8 is overestimated systematically by 16–20 nmol mol−1 in rural regions like the Alps and parts of the Iberian Peninsula and the Balkan region. The bias of the OSMDA8 in polluted areas, like the Ruhr area, Benelux, parts of France and the Po Valley, is in the range of 5–10 nmol mol−1 (see Sect. S6.1 in the Supplement).
The comparison with the ground-level observations from the AirBase network in Europe shows an overall mean bias across all stations for NOx and O3 of −3.3 µg (NO2) m−3 and 23.5 µg m−3, respectively. The root mean square errors (RMSEs) for NOx and O3 are 12.9 µg (NO2) m−3 and 35.9 µg m−3, respectively (see Sect. S6.2 in the Supplement). The mean bias and RMSE for ozone are comparable to previous evaluations of MECO(n) (see Table 7 by Mertens et al., 2020b).
The flight measurement campaign EMeRGe Europe took place in July 2017 with HALO flights across Europe. The goal was to measure emission plumes from major polluted regions and to study their transport and transformation (Andrés Hernández et al., 2022). Since the focus of our study is on ozone and NOy, the respective in situ measurement data from EMeRGe Europe were used for comparison. A detailed description of the instruments can be found in Andrés Hernández et al. (2022) and Ziereis et al. (2022). In our study, three flights (11, 20 and 26 July 2017) are analysed because these flights took place within our study areas (Po Valley and Benelux). For this inter-comparison, the model data are sampled online along the flight track at the highest possible frequency, i.e. at every model time step (for more details see submodel S4D described by Jöckel et al., 2010).
Scatter plots for NOy and O3 comparing the in situ observations with the CM12 data at flight level are shown in Fig. 1. These indicate that the model performance, compared to the observations, varies strongly depending on the specific flight. Some are in rather good agreement with observations for NOy and O3, while other flights (especially the flight on the 11 July 2017) show a positive ozone bias of 10–15 nmol mol−1. A more detailed inter-comparison between aircraft in situ measurements and model data is limited. Specific features simulated by the model could be shifted in time (or space) compared to the observations. As an example, for some flights/chemical species, models and observations agree quite well, but the plumes in the model have a slightly different location. Therefore, Sect. S6.3 in the Supplement presents a more detailed analysis discussing how specific patterns might be represented or not represented by the model.
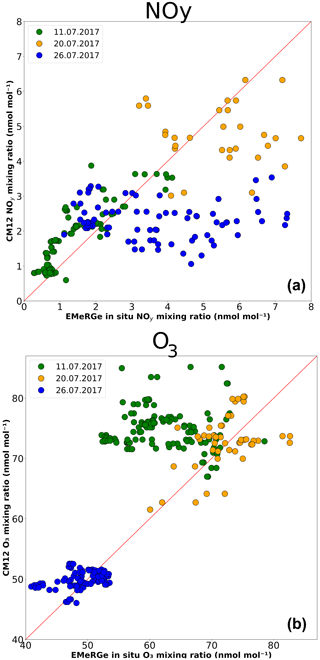
Figure 1Comparison of NOy (a) and O3 (b) mixing ratios (nmol mol−1) between the CM12 model results (vertical axis) and the HALO in situ measurements on the horizontal axis for all three flight dates, 11, 20 and 26 July 2017.
Overall, the evaluation shows that the chosen MECO(n) setup is able to reproduce ground-level and free-tropospheric NOx, NOy and O3. However, the results also show a positive bias of tropospheric ozone and a negative bias for NOx. The model biases are in the typical range of comparable model configurations (see detailed discussion in Mertens et al., 2020b). Reasons for these ozone biases have been discussed in previous publications (Mertens et al., 2016, 2021). One main reason for the positive ground-level-ozone bias is vertical mixing that is too strong during the night, mixing in ozone-rich air from the free troposphere to the boundary layer. This is a common problem in many models (Travis and Jacob, 2019). Moreover, free-tropospheric ozone is also biased high (see discussion by, for example, Jöckel et al., 2016). As a consequence, simulated contributions from the stratosphere, from lightning and from N2O decomposition to ground-level ozone are likely biased high, and contributions from the ground level are likely biased low. The main reasons for the underestimations of NOx are the horizontal resolution of the model, leading to a dilution of emissions over a large area and uncertainties of the emission inventories.
Contributions to ozone from land transport, anthropogenic non-traffic and biogenic emissions are the sectors with the largest share to ground-level O3 in Europe (e.g. Karamchandani et al., 2017; Mertens et al., 2018; Butler et al., 2018; Lupaşcu and Butler, 2019; Mertens et al., 2020b). In more detail, Mertens et al. (2020b) report contributions of these sectors during summer of up to 16 % (land transport), 20 % (biogenic) and 30 % (anth. non-traffic). Therefore, other emission sectors are either summarized as “rest” or not shown/discussed in the present paper.
Table 2Definition of the regions analysed in this study. The last column lists the type of the chemical regime.
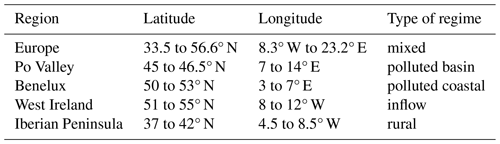
For the analysis we focus on five different study areas with rather large and rather low air pollution in Europe. Besides Europe (whole domain), the Po Valley, Benelux, a region on the Iberian Peninsula and West Ireland are considered. The latter two are chosen to represent a rural region (Iberian Peninsula) and a region that is dominated by inflow (West Ireland; see Table 2 and Fig. S19 in the Supplement)
The focus is on results for JJA 2017. Further analysis of inter-annual variability is presented in Sect. 5.3.
4.1 Contribution of different emission sectors to ground-level ozone
The subject of this section is to examine which emission sectors contribute most to ground-level ozone in the specified regions during JJA 2017. Of particular importance is the distinction between the geographical origin of emissions to differentiate between the contributions attributed to long-range transport and regional emission sources.
The largest contributions of land transport and anthropogenic non-traffic emissions to ground-level NOy in Europe are simulated in the Benelux region with up to 2–9 and 2–8 nmol mol−1, respectively (the range always indicates the geographical variation of the JJA average). The contributions in the Po Valley are 2–10 nmol mol−1 for land transport emissions and 1–4 nmol mol−1 for anthropogenic non-traffic emissions (geographical distribution is given in Fig. S16 in the Supplement). Most of the contributions to ground-level NOy in Europe come from European emissions; only a very small part is attributed to long-range transport, which is a direct consequence of the rather short lifetime of NOy.
For NMHCs, the anthropogenic non-traffic sector (30–120 nmol mol−1) and the biogenic sector (up to 30 nmol mol−1) are the largest contributors to ground-level NMHCs in the Benelux region and the Po Valley. Contributions from land transport emissions are in the range of 3–15 nmol mol−1 (see Fig. S17 in the Supplement for a geographical distribution). Given the NMHC lifetime, also here, long-range transport is not important.
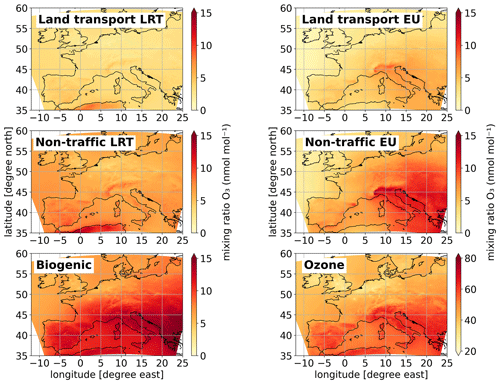
Figure 2Seasonal (JJA 2017) mean ozone mixing ratio (lower right) and absolute contributions (both in nmol mol−1) of ground-level O3 from long-range-transported (LRT: ROW + NA + EA), biogenic and European land transport as well as anthropogenic non-traffic emissions as simulated with CM12. Please note the different scaling of the total ozone mixing ratios and the contributions.
Given the complex and non-linear ozone chemistry, the contributions of ozone strongly differ from the contributions of the precursors NOy and NMHCs. Figure 2 depicts the absolute contributions to ozone as simulated by CM12 in central Europe for JJA 2017. In general, the emissions from European anthropogenic non-traffic emissions (O), from European land transport (O) and from biogenic emissions (O) are the largest contributors to ground-level O3 in Europe. These contributions also show a positive gradient in a north-west to south-east direction. The distribution of the contribution to ozone from long-range-transported emissions (anthropogenic non-traffic and land transport) is more homogeneous and largest in south Europe. Reasons for the peak over south Europe are transport of air masses from the African continent (tagged as ROW) to Europe (especially southern Spain) and descent of air masses transported from North America over the Mediterranean (Stohl et al., 2002; Eckhardt et al., 2004).
The source attribution method yields contributions to ozone of the individual emissions sources and calculates the ozone production and loss rates for each emission sector, from which we calculate the net ozone production for each emission sector (i) defined as
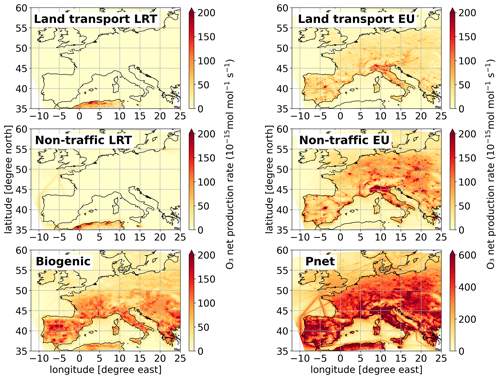
Figure 3Seasonal (JJA 2017) mean of the ground-level ozone net production in pmol mol−1 s−1 from long-range-transported (LRT: ROW + NA + EA), biogenic, and European anthropogenic non-traffic and land transport emissions. The lower-right panel shows the total net production with a different scale.
Figure 3 shows total PO3net and PO3 for the most important emission sectors (land transport, anthropogenic non-traffic and biogenic) separated between contributions from European emissions (EU) and emissions from other regions (LRT = NA + EA + ROW). Total PO3net shows a clear north–south gradient, indicating much larger net ozone production in southern Europe than in northern Europe. Accordingly, also, PO3net in the Po Valley is much larger than in Benelux. Ozone production from European land transport emissions peaks in the Po Valley and some larger cities in southern Europe (Madrid, Rome, Naples). Similarly, PO3 from European anthropogenic non-traffic emissions also peaks in the Po Valley and around hotspots, mainly in south and eastern Europe. Ozone production from biogenic sources is largest over the Iberian Peninsula. In situ production from anthropogenic precursors over Europe from LRT plays almost no role in Europe. Over land and in particular towards eastern Europe, the net production of ozone from LRT is slightly negative because loss processes of ozone from LRT are larger than the production from LRT precursors. Only along the ship lanes in the Atlantic does the net ozone production from LRT take place. This production is due to reactions of NOy from shipping with NMHC emissions from evaporation of gas/oil transported with ships (not the shipping emissions itself). This NMHC evaporation is categorized as anthropogenic non-traffic emissions from the rest of the world (see Fig. S17 in the Supplement) as it takes place over the oceans and is not directly linked to the ship operation.
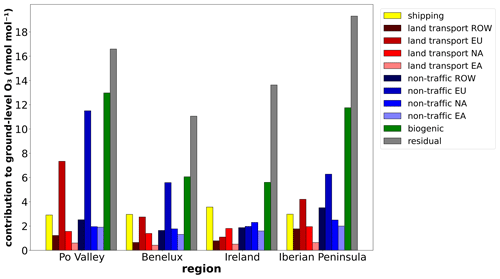
Figure 4Seasonal (JJA 2017) mean absolute contribution (in nmol mol−1) of different emissions sectors and regions to ground-level ozone in the four regions of the Po Valley, Benelux, West Ireland and Iberian Peninsula, as simulated with CM12.
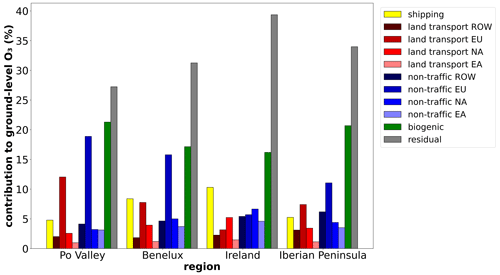
Figure 5Seasonal (JJA 2017) mean relative contribution (in %) of different emissions sectors and regions to ground-level ozone in the four regions of the Po Valley, Benelux, West Ireland and Iberian Peninsula, as simulated with CM12.
Figures 4 and 5 show the area-averaged absolute and relative contributions to ground-level ozone for each study area for JJA 2017. We focus on contributions of land transport (reddish colours) and anthropogenic non-traffic (bluish colours) emissions from different regions of the world, shipping and biogenic emissions. All other categories are summarized as “others”. This category is responsible for 27 %–39 % (12–19 nmol mol−1) of ground-level ozone in the different study areas. The largest contributors to “others” differ between the regions, but degradation of CH4 and lightning are important contributors in all regions. A detailed breakdown of the contributions from the “others” category is shown in the Supplement (Fig. S21). For the “inflow” region Ireland, emissions from North America are the largest contributor to the emission sectors anthropogenic non-traffic and land transport. Accordingly, changes of anthropogenic emissions in North America might heavily influence ozone over Ireland, which is in agreement with previous studies using the perturbation approach (e.g. Jonson et al., 2018) and the NOx tagging results by Lupaşcu and Butler (2019).
Besides long-range transport, the shipping sector in particular is an important contributor to ground-level ozone, which confirms results from global model studies by, for example, Mertens et al. (2018), Butler et al. (2020) and Mertens et al. (2024) showing large contributions of shipping emissions to ground-level ozone in the eastern Atlantic. Accordingly, the Benelux region also shows larger relative contributions of the shipping sector compared to the Iberian Peninsula or the Po Valley. Absolute contributions, however, are larger over the Iberian Peninsula than over Benelux, due to the overall larger ozone values over the Iberian Peninsula.
In all other regions, i.e. the Po Valley, Iberian Peninsula and Benelux, European emissions from land transport and anthropogenic non-traffic sectors have larger absolute and relative contributions compared to the anthropogenic non-traffic/land transport emissions from other regions. Generally, the relative/absolute contribution of European land transport emissions varies between 12 %/7 nmol mol−1 (Po Valley) and 7 % (Benelux and Iberian Peninsula)/3 nmol mol−1 (Benelux). Contributions from European anthropogenic non-traffic emissions are larger than from land transport in all regions and range between relative/absolute contributions of 19 % (Po Valley)/12 nmol mol−1 (Po Valley) and 11 % (Iberian Peninsula)/6 nmol mol−1 (Benelux and Iberian Peninsula).
The relative importance of land transport and anthropogenic non-traffic European emissions compared to other emission origins (i.e. the ratio of the contribution from European emissions from one sector to the contribution of land transport from all regions), however, differs strongly between the regions. This ratio is smallest over the Iberian Peninsula, over which anthropogenic non-traffic and land transport emissions from other regions also contribute more compared to Benelux and the Po Valley. This is consistent with results by Pay et al. (2019), who report that long-range transport is an important source for ground-level ozone over the Iberian Peninsula.
In the Po Valley, the contributions from European land transport and anthropogenic non-traffic emissions are the largest compared to the other regions. This is consistent with the net ozone production, which is largest in the Po Valley (see Fig. 3), due to large emissions and meteorological conditions favourable for ozone production.
Similarly, relative and absolute contributions from biogenic emissions are also largest in the Po Valley, even though soil NOx emissions are largest over Benelux, and isoprene emissions are largest over the southern Iberian Peninsula (see Figs. S1 and S2 and Table S2 in the Supplement for emission totals and their geographical distribution). Since our tagging mechanism combines these different ozone precursors, no differentiation can be made between O from soil NOx and isoprene emissions.
So far, our analyses show that the mitigation potential for the European anthropogenic sector over the Iberian Peninsula and the Benelux region is more limited than in the Po Valley because much less ozone is produced in situ from regional emissions. Instead, ozone is more dominated by long-range transport and by biogenic emissions (Iberian Peninsula).
Especially for human health, periods of enhanced ozone concentrations are most harmful. Such large ozone concentrations can occur, for example, under stagnant conditions during heat waves. During these periods, ozone contributions can differ strongly from seasonal mean values (e.g. Mertens et al., 2020b; Lupaşcu et al., 2022). Therefore, we investigate the contributions at the 95th, 90th and 75th percentiles of ozone and for maximum MDA8 conditions to extend previous analyses by considering (1) contributions of land transport emissions and (2) various geographical origins of emissions. The analyses are performed for the whole of Europe and for the four study regions defined in Table 2.
5.1 Contributions to large ozone mixing ratio percentiles
Figure 6 shows the absolute contributions of land transport, anthropogenic non-traffic and biogenic emissions at different percentiles of ozone (relative contributions are given in Fig. S22 in the Supplement). For this analysis, we first calculated the respective percentiles of ozone and then quantified the contributions of ozone at this percentile (see Mertens et al., 2020b, for more details on the technical realization). The ozone percentiles in the various regions have a large geographical spread; therefore the analyses are presented as box-and-whisker plots. The range of the whiskers indicates the geographical spread among the regions.
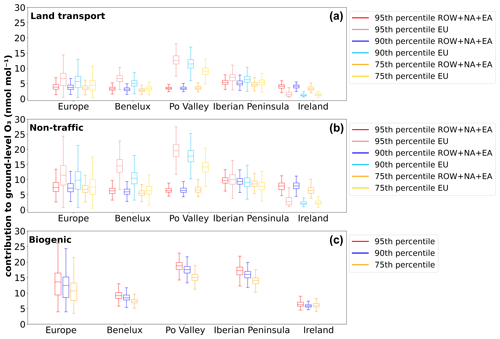
Figure 6Box-and-whisker plot showing the contributions (in nmol mol−1) of the most important emission sources for the 95th, 90th and 75th percentiles of ground-level ozone as simulated by CM12 for JJA 2017. Panel (a) shows the regional absolute contributions of O (labelled EU) and the sum of long-range-transported absolute contributions of O, O and O (labelled ROW+NA+EA). Panel (b) shows the absolute contributions of O (labelled EU) and the sum of long-range-transported absolute contributions of O, O and O (labelled ROW + NA + EA). Panel (c) shows the absolute contributions of O. The range indicated by the boxes and whiskers indicates the geographical spread within the corresponding region. The lower and upper ends of the boxes indicate the 25th and 75th percentiles, respectively; the bar shows the median; and the whiskers are defined as ±1.5 times the inter-quartile range of the contributions of all grid boxes within the geographical region.
Due to the large spatial variation of O3 (see Fig. 2), the contributions averaged over the European area show a large spread, but mean and maximum values show a tendency towards larger contributions of ozone from European land transport, from European anthropogenic non-traffic and from biogenic emissions, with increasing ozone percentiles. This result is in accordance with results by Mertens et al. (2020b). Compared to Mertens et al. (2020b), our additional information about the geographical origins of the emissions shows that the larger contributions of land transport and anthropogenic emissions at larger ozone values are caused by emissions from within Europe.
The larger contributions of land transport and anthropogenic non-traffic emission to ground-level ozone with increasing ozone percentiles are largest over Benelux and the Po Valley. Ireland and the Iberian Peninsula show no or only a little increase in the contribution of these sectors for increasing ozone values. In addition, the contribution of ozone from long-range transport is important at all ozone percentiles, especially over the Iberian Peninsula and Ireland. The results for the Iberian Peninsula confirm contribution analyses of peak ozone values by Pay et al. (2019) and ozone source attribution for the Madrid region by de la Paz et al. (2024). However, our results show in addition information on the sectoral attribution instead of attributing long-range transport only to boundary conditions of the regional model.
This analysis shows that in particular in the Po Valley, but to a limited extent also over Benelux, European anthropogenic emissions drive large ozone values. The reduction in European emissions therefore has the potential to reduce ozone peak values, especially in the Po Valley. Here and over the Iberian Peninsula, however, the contributions of biogenic emissions also strongly increase with increasing ozone levels. Competing effects, for example, the increase in contribution from natural emissions if anthropogenic emissions are reduced, as reported by Mertens et al. (2021), could therefore counteract ozone reductions measures.
5.2 Contributions to MDA8 ozone
An important metric for ozone exceedance is MDA8; therefore we also investigate ozone contributions to MDA8. To do so, we first calculate MDA8 for JJA 2017. Based on the MDA8 values, we calculate mean, minimum and maximum MDA8 values for JJA 2017 (geographical distributions are shown in Fig. S19 in the Supplement). For these maximum, minimum and mean values of MDA8, the contributions are analysed for the study regions defined in Table 2. Similar to the percentiles, the contributions are analysed as box-and-whisker plots to indicate the geographical spread among the regions in Fig. 7.
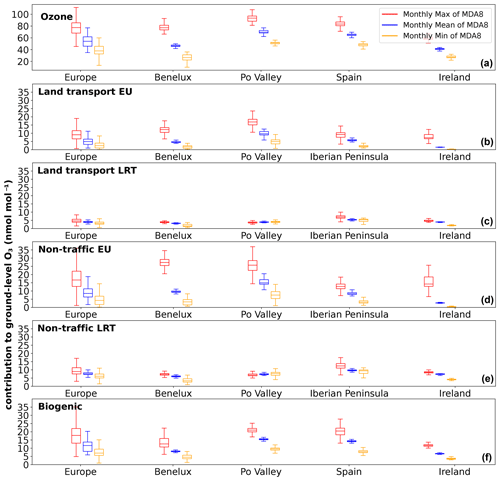
Figure 7Box-and-whisker plot showing MDA8 ozone and the absolute MDA8 ozone contributions of the most important European emission sectors at ground-level as simulated by CM12 for JJA 2017. Panel (a) shows the MDA8 ozone, and panels (b) and (c) show the contributions for European (EU) and long-range-transported (LRT) land transport emissions, respectively. Panels (d) and (e) show the contributions for European (EU) and long-range-transported (LRT) anthropogenic non-traffic emissions, respectively. Panel (f) shows the contribution of biogenic emissions. Units in each panel are nmol mol−1; the minimum, mean and maximum of MDA8 ozone (a) or minimum, mean and maximum of the absolute contributions to MDA8 ozone are shown (b–f). All results are based on 1-hourly model output. The range indicated by the boxes and whiskers indicates the geographical spread within the considered region. The lower and upper ends of the boxes indicate the 25th and 75th percentiles, respectively; the bar shows the median; and the whiskers are defined as ±1.5 times the inter-quartile range of the contributions of all grid boxes within the geographical region.
The monthly maxima of MDA8 over Europe range from 40–100 nmol mol−1 (Fig. 7, upper panel). Contributions to the maximum MDA8 from European land transport emissions range between 2–20 nmol mol−1, contributions from European anthropogenic non-traffic between 2–35 nmol mol−1 and contributions from biogenic emissions between 5–30 nmol mol−1. The analysis largely confirms the findings based on the analysis of the percentiles. We see that especially over Benelux and the Po Valley, the contributions of European land transport and anthropogenic non-traffic emissions are larger for larger MDA8 values. Accordingly, European emissions from these two sectors drive maximum MDA8 values in the two major polluted regions. This confirms the findings by Lupaşcu and Butler (2019) gained using a NOx tagging method but here with a tagging method considering NOx and VOCs concurrently. Moreover, here we quantify the role of land transport emissions in the MDA8 trends separately, instead of considering all anthropogenic emissions together.
In particular over the Iberian Peninsula, but also in Ireland (and only very limited in Benelux and the Po Valley), the absolute contributions of emissions from the land transport and anthropogenic non-traffic sectors from other source regions are larger for larger MDA8 values. Accordingly, long-range transport is also an important source, especially over the Iberian Peninsula during high MDA8 values. This large importance of long-range transport is also in accordance with previous studies (Pay et al., 2019; de la Paz et al., 2024). However, our results in addition provide a sectoral attribution for the long-range aspect instead of reporting contributions from boundary conditions only.
5.3 Inter-annual variability
Tropospheric ozone has a strong inter-annual variability; therefore we want to compare the results for JJA 2017 with results for JJA 2018. Overall, the ozone abundance in summer 2018 was larger than in summer 2017. Therefore, JJA mean absolute contributions in 2018 are generally larger than in 2017, but the JJA mean relative contributions are similar, with a tendency towards larger relative contributions of ozone from European emissions compared to long-range transport in 2018 compared to 2017. Our analysis for the MDA8 values and their contributions in 2018 also show that our results are virtually unaffected by inter-annual variability. Although the maximum MDA8 values differ between the 2 years, with larger maximum values in the Benelux area (≈90 nmol mol−1 in 2018 and ≈78 nmol mol−1 in 2017) and slightly lower values in the Po Valley (≈90 nmol mol−1 in 2018 and ≈95 nmol mol−1 in 2017), the described relation can also be observed for 2018 MDA8 values. The contributions of traffic and anthropogenic non-traffic emissions from long-range transport remain very similar for max, min and mean MDA8 values, while the contributions from European anthropogenic (non-traffic and traffic) and biogenic emissions increase with increasing MDA8 values. Corresponding figures depicting ground-level ozone and the contributions to ground-level ozone in 2018 are part of the Supplement (Sect. S7 therein).
5.4 Dependence on model resolution
The effect of the model resolution on the simulated ozone mixing ratios is well known. Therefore, Mertens et al. (2020a) investigated the effect of the model resolution on simulated ozone contributions. According to this analysis simulated ozone contributions in specific regions, e.g. the Po Valley, can differ strongly between a coarse-resolved global model and a finer-resolved regional model. A further increase in the model resolution, i.e. from 50 to 12 km resolution, however, only had a small influence on seasonal-mean ozone contributions. This also holds for the results of the present study. Generally, CM12 simulates lower JJA average ozone mixing ratios in many regions and, accordingly, slightly lower absolute contributions too. The overall geographical distribution agrees well. In polluted regions, especially in the Po Valley, CM12 tends to simulate larger contributions compared to CM50 (see Fig. S20 in the Supplement). For large ozone values, however, the effect of the resolution is even more important; therefore we also compare the MDA8 values between CM50 and CM12. The results show that CM50 simulates slightly larger maximum and mean MDA8 median values averaged over the considered regions and, accordingly, also slightly larger contributions from European land transport, from anthropogenic non-traffic and from biogenic emissions. The finer resolution of CM12, however, leads to a larger geographical variability in the considered regions (indicated by the spread of the whiskers). In the Po Valley the finer resolution even leads to larger maximum values (indicated by the upper whiskers) in CM12. The larger geographical spread can be expected given the finer resolution. In general, the comparison of CM12 and CM50 shows that for larger geographical regions, such as Benelux and the Po Valley, even for source attribution of ozone MDA8, the results are quite robust between 12 and 50 km horizontal resolution. However, it remains to be tested if further resolution increases to 4 km and below will give results that differ more from 50 km. The resolution of around 4 km is of interest because previous publications (e.g. Tie et al., 2010; Markakis et al., 2015) reported an increase in the model skill to simulate ozone mixing ratios in comparison to measurements in this range.
To understand the results of the ozone source attribution analysed in the previous sections in more detail, we next analyse the ozone chemistry using the two metrics ozone production efficiency (OPE) and the ratio of production of H2O2 and HNO3 (called SR). These quantities are defined as
In these definitions, P* denotes the diagnosed production rates from the indicated chemical reactions. OPE is a measure of the number of ozone production cycles of one NOx molecule before it reacts to HNO3. Here, we follow the definition of the OPE (see Chap. 12 of Jacob, 1999), which assumes that reactions of RO2 with NO have the same reaction rate as reactions of HO2 with NO. SR is a measure to assess whether the ozone chemistry is NOx- or VOC-limited (see Sillman, 1999), with smaller values indicating a tendency towards a VOC-limited regime.
Table 3Seasonal means (JJA 2017) of the average anthropogenic NOx emission flux (in ng m−2 s−1), the absolute and relative NOy and O3 contributions from European land transport emissions (teu) and from regional anthropogenic emissions (reg.-anth., O and O), and averages of the OPE and the SR. Please note that OPE and SR have been calculated for daylight conditions only.

Table 3 summarizes the JJA average absolute and relative contributions of NOy and O3 (of land transport emissions and of all regional anthropogenic emissions). Moreover, the table lists the calculated OPE and SR as well as average anthropogenic NOx emission fluxes in the specified regions. A more detailed breakdown of the emissions in the regions is also given in Table S2 in the Supplement.
The results show that the (compared to other regions) much larger anthropogenic NOx emissions over Benelux lead to a chemical regime, which tends to be rather VOC-limited. In addition, the large NOx mixing ratios lead to a low ozone production efficiency. Moreover, reduced UV radiation over Benelux compared to the Po Valley inhibits ozone production (e.g. Real et al., 2024).
In the Po Valley, the average NOx emission flux is lower than in the Benelux, and the ozone production efficiency is larger due to less NOx but also due to meteorological conditions, which are more favourable for ozone production compared to Benelux. These differences explain the larger production of ozone from regional emissions in the Po Valley compared to Benelux. The two remote regions (Iberian Peninsula and Ireland) show similar SR, but the OPE over Ireland is much smaller compared to that over the Iberian Peninsula, due to the overall less favourable conditions for ozone formation.
Even though the contributions cannot directly be translated into ozone reductions in case of emissions changes, they provide valuable insights for potential mitigation options. Given the overall lower ozone over Benelux compared to the Po Valley, especially NOx emissions should be decreased in order to improve air quality (by reducing NO2). However, the low SR indicates that ozone might increase or change only very little. The larger OPE and the larger contributions of ozone from European anthropogenic emissions over the Po Valley compared to other European regions indicate that reductions of these emissions can help to reduce ozone levels. However, additional perturbation studies are needed to quantify the achievable ozone reductions. Combining these simulations with the source attribution technique would help to understand the ozone response of the changed emissions (e.g. Mertens et al., 2018).
As shown, emissions from land transport and anthropogenic non-traffic sources are important for NOx (here analysed as NOy) over Europe, while for VOCs, biogenic and anthropogenic non-traffic sources are most important. The regions with the largest contributions of land transport and anthropogenic non-traffic emissions to O3, however, are not always identical with the regions of the largest contributions to NOx and NOy. Mertens et al. (2020a) already stated that large amounts of NOx emissions do not necessarily lead to large O3 mixing ratios. The reasons are the non-linearity of the ozone chemistry, depending on the availability of VOCs (Sillman, 1999), and the strong dependence of the ozone mixing ratio on the meteorological conditions, affecting deposition and transport (Vieno et al., 2010; Francis et al., 2011; Logan, 1985).
The analysed contributions, however, depend on the applied emission inventories. The model results of NOx and NOy show a good agreement with the observational data, but locally, NOy is underestimated (Sect. 3). The uncertainty estimate for a previous version (4.3.2) of the EDGAR emissions by Crippa et al. (2018) indicates uncertainties of NOx emissions of 17 %–69 % depending on the country. For EU-28 in 2012 (the most recent year covered in that analysis), uncertainties of 51 % are reported. Besides the estimates of anthropogenic emissions, estimates of biogenic and natural emissions are also uncertain; for example, estimates of the emissions of NOx from soil range from 4 to 15 Tg (N) a−1 (Vinken et al., 2014), and emissions from lightning NOx range from 2 to 7 Tg (N) a−1 (Schumann and Huntrieser, 2007).
Mertens et al. (2020b)Mertens et al. (2020b)Mertens et al. (2020b)Table 4Comparison of absolute and relative contributions for the sectors land transport, anthropogenic non-traffic and biogenic between results by Mertens et al. (2020b) and results from the present study. Please note that the region and sector definitions are not identical between the two studies. We compare our values for Benelux with their values for mid-Europe, and our values for West Ireland correspond to values of their inflow region. Moreover, the definition of the region Iberian Peninsula differs between the two studies. Sector-wise, we compare our values for anthropogenic non-traffic to their values for anthropogenic (including anthropogenic non-traffic, shipping and aviation). The range of the values by Mertens et al. (2020b) shows the range of the two different emissions inventories. All values are for JJA (2017 in our study; 2008–2010 in the study by Mertens et al., 2020b).
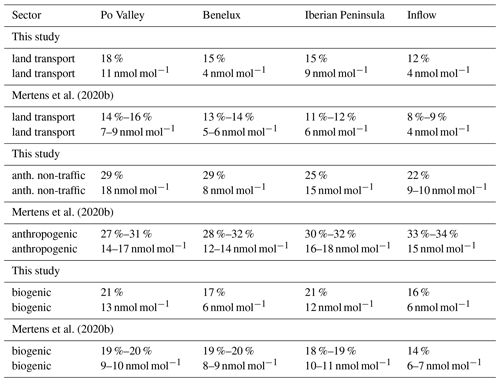
Mertens et al. (2020b), however, showed that the contributions to ground-level ozone over Europe change only slightly for two different anthropogenic emission inventories over Europe. Therefore, we expect that results change only slightly, as long as the ratio of the emission strengths and the order of magnitude of specific emissions do not change drastically. To quantify the effect of different emissions in more detail, we compare the results of our study with the results of Mertens et al. (2020b). They used the same model and tagging method but different emission inventories on a global and regional scale. In addition, Mertens et al. (2020b) analysed the years 2008–2010 and did not distinguish different geographical regions. Therefore, we summed up the contributions from different geographical regions in our study to compare the results roughly with those by Mertens et al. (2020b). This comparison is summarized in Table 4, indicating that the results are relatively robust with respect to different emission inventories and years.
The source attribution method applied in our study is subject to some simplifications, as discussed by Grewe et al. (2017). As Mertens et al. (2020a) already clarified, the mathematical method itself is accurate, but the implementation in the model requires some simplifications such as the introduction of chemical families (e.g. NOy, NMHCs). This simplification can lead to small artificial contributions. Such an example is NMHCs from the lightning category, which are created from decomposition of PAN from lightning into NOy and NMHCs. PAN from lightning is created from reactions of NOy from lightning with NMHCs from other emission categories (Grewe et al., 2017; Butler et al., 2018). In order to quantify the influence of simplifications and also the influence of different tagging approaches on the source attribution results, we further compare our results with results from other source attribution studies.
To investigate the influence of different tagging approaches, we compare our results with publications using the tagging approach described by Butler et al. (2018). In this approach, ozone is attributed – in different simulations – to either emissions of NOx or VOCs, while our approach considers NOx and VOCs concurrently. Even though a detailed inter-comparison between our results and the results from Butler et al. (2018, 2020) is limited due to different years analysed, different emission inventories applied and differently used definitions of the tagged categories, the comparison shows that sources with large amounts of NOx emissions, but only low VOC emissions, are estimated to contribute more in the NOx-only tagging approach of Butler et al. (2018). This has been discussed already by Butler et al. (2020) for shipping emissions. As example, in our simulation we estimate a global mean tropospheric burden of ozone from shipping emissions for 2017 on the order of 10 Tg for 5.8 Tg (N) a−1 shipping emissions, while Butler et al. (2020) report around 20 Tg for 4.3 Tg (N) a−1. Moreover, Butler et al. (2018) diagnosed contributions from anthropogenic NOx sources in the range of 25–35 nmol mol−1 for July 2010. In our global model instance, contributions in the range of 15–35 nmol mol−1 during July 2017 (see Fig. S23 in the Supplement) are simulated. Accordingly, Butler et al. (2020) report ground-level ozone contributions from north-west European (local) NOx emissions of 10–13 nmol mol−1 in JJA 2010, which is larger than our result of 8 nmol mol−1 for the Benelux region in JJA 2017.
Lupaşcu and Butler (2019) applied the Butler et al. (2018) tagging method within the WRF-Chem model (Weather Research and Forecasting (WRF) model coupled with Chemistry model) that attributes O3 concentrations in several European receptor regions to NOx emissions. They calculated in their GEN (Germany, Belgium, the Netherlands and Luxembourg) region means of regional contribution from anthropogenic (land transport and non-traffic) emissions for July to September 2010 of 25 % (their Fig. 7). Compared to this, we find a contribution of 23 % from anthropogenic emissions from Europe in the Benelux region for JJA 2017.
In summary, as discussed in previous studies (Butler et al., 2020), our approach of tagging NOx and VOCs concurrently provides lower contributions from anthropogenic sources, which mainly comprise NOx but not that much in terms of VOC emissions, compared to a separate tagging of NOx or VOCs. However, more detailed model inter-comparison exercises are needed to better quantify the differences between these approaches.
Other tagging methods, often applied on the regional scale (Dunker et al., 2002; Kwok et al., 2015) check if the ozone chemistry is either NOx- or VOC-limited and attribute ozone production accordingly to either NOx or VOCs. Karamchandani et al. (2017) applied such a source attribution method in the Comprehensive Air quality Model with Extensions (CAMx) and estimated the ozone contribution from road transport emissions for different metropolitan regions across Europe. They found contributions to MDA8 during summer of 19 % in Amsterdam and 11 % in London. Our results for Amsterdam are 9 % and 7 %–8 % for London and therefore lower in both cases. With a similar approach Pay et al. (2019) used the source attribution method with the CMAQ model and estimated a relative ozone contribution from road transport emissions of 11 %–16 % in most parts of Spain during ozone exceedances in summer, which is in a similar order of magnitude compared to our JJA mean contributions (see Fig. 5).
A limitation of our study is the choice of the three tagging regions, which are large-scale and represent almost entire continents. Thus, no country-wise source–receptor relationships have been able to be established so far. Therefore, the tagging regions could be further refined within Europe or in other regions (e.g. country by country) in order to enable the source attribution to each country (e.g. by Lupaşcu and Butler, 2019). This approach is mainly limited by the amount of memory per computing task due to the large amount of additional tracers. Nevertheless, it could be worthwhile for the assessment of mitigation strategies on a national scale.
In the present study we investigate the contributions of anthropogenic (land transport and non-traffic) and biogenic emissions to ground-level ozone over Europe. By means of simulations with the MECO(n) model system we analyse contributions in several regions in detail. The model system allows an online coupling of the global chemistry–climate model EMAC with the regional chemistry–climate model COSMO-CLM/MESSy. In order to quantify the contributions of land transport, anthropogenic non-traffic and biogenic emissions to ozone and its precursors, a tagging method for source attribution is used, which takes into account NOx and VOCs concurrently. To distinguish between regional and long-range-transported contributions, we define four different geographical source regions, Europe, North America, east Asia and the rest of the world. Our tagging method fully decomposes the budgets of ozone and ozone precursors into contributions from various emission sources (and regions) and is applied consistently in the global and regional model instances. The study is based on a previous assessment (Mertens et al., 2020b) and adds the following new aspects:
-
Land transport and anthropogenic non-traffic emissions are further separated between four geographical source regions (Europe, North America, east Asia and the rest of the world).
-
We apply a more recent emission inventory (EDGAR 5), analyse a more recent year (2017) and consider a finer spatial resolution (0.11° instead of 0.44°).
Compared to other studies we apply a source attribution method that takes into account NOx and VOCs concurrently. Moreover, we apply the tagging method on the global and on the regional scale and therefore also provide a sectoral source attribution of long-range transport.
Our analysis shows that land transport emissions contribute strongly (42 % and 44 %, respectively) to the ground-level NOy mixing ratios for JJA 2017 in the most polluted regions Benelux and the Po Valley, with larger absolute contributions in the Po Valley. However, due to the different meteorological conditions and the overall larger NOx emissions in the Benelux compared to the Po Valley, the contributions of European land transport (12 % in the Po Valley and 8 % in the Benelux) and other anthropogenic non-traffic emissions (19 % in the Po Valley and 15 % in the Benelux, respectively) are smaller over the Benelux compared to the Po Valley during JJA 2017. In line with previous studies, our results show large contributions from biogenic emissions across Europe.
Our results show additionally that long-range transport of ozone, especially formed from land transport and other anthropogenic emissions, has a larger relative contribution to ground-level ozone over Ireland, the Benelux countries and the Iberian Peninsula compared to the Po Valley. The absolute ozone levels over Benelux and Ireland, however, are low, and accordingly the absolute contributions from long-range transport are largest over the Iberian Peninsula. The main geographical source regions for Benelux and Ireland are emissions from North America, while for the Iberian Peninsula the contributions of anthropogenic emissions from the rest of the world are also important. These contributions can mainly be associated with ozone originating from emissions in north Africa.
For the upper percentile of ozone and MDA8 ozone over the Benelux countries and the Po Valley, our results clearly show that the larger ozone mixing ratios are determined by European anthropogenic and land transport emissions as well as by biogenic emissions. Accordingly, a reduction of regional emissions can potentially lower the high ozone levels. This confirms similar previous analyses but with a different methodology. Moreover, we were able to quantify the role of the land transport emissions separately. In contrast to the other regions long-range transport contributions to extreme values are likewise important for the Iberian Peninsula, suggesting that a reduction of European emissions only has a limited effect on extreme ozone mixing ratios. An inter-comparison between 2017 and 2018 shows that these main findings also hold for 2018. Moreover, they also hold for the coarser-resolution (50 km) domain.
The comparison with results from studies applying ozone source attribution to either NOx or VOC shows that our approach tends to simulate lower contributions of sources with strong NOx but low VOC emissions. Follow-up studies need to quantify this difference in more detail by applying both methods with the same simulation setup and (if possible) the same model system. Further, we intend to further refine the source regions for the apportionment (tagging) in order to allow a country-by-country attribution (e.g. as in Lupaşcu and Butler, 2019) in subsequent studies. This is a prerequisite to assessing the potential benefit of national mitigation regulations.
The Modular Earth Submodel System (MESSy) is continuously further developed and applied by a consortium of institutions. The usage of MESSy and access to the source code is licensed to all affiliates of institutions which are members of the MESSy Consortium. Institutions can become a member of the MESSy Consortium by signing the MESSy Memorandum of Understanding. More information can be found on the MESSy Consortium website (http://www.messy-interface.org, last access: 3 December 2024). The code applied here has been based on MESSy version d2.55.2-1913 and will be available in the next official release (not yet scheduled). The simulation results used here are archived at the German Climate Computing Center (DKRZ) and are available on request. The EDGAR 5.0 emission data set is available at http://data.europa.eu/89h/377801af-b094-4943-8fdc-f79a7c0c2d19 (Crippa et al., 2019a). The tool CDO used for data processing is available at https://code.mpimet.mpg.de/projects/cdo (Lang, 2024).
The supplement related to this article is available online at: https://doi.org/10.5194/acp-24-13503-2024-supplement.
MK, MM and PJ built the model setup and performed the simulations at the DKRZ. AK co-developed MECO(n) and helped during the study with model updates, bug fixes and definition of the model setup. MK analysed the results and drafted the manuscript. VG supported the interpretation of the source attribution results. HZ and AZ provided the in situ data from the EMeRGe Europe campaign. All authors contributed to the interpretation of the results and to the revision of the manuscript.
At least one of the (co-)authors is a member of the editorial board of Atmospheric Chemistry and Physics. The peer-review process was guided by an independent editor, and the authors also have no other competing interests to declare.
Publisher's note: Copernicus Publications remains neutral with regard to jurisdictional claims made in the text, published maps, institutional affiliations, or any other geographical representation in this paper. While Copernicus Publications makes every effort to include appropriate place names, the final responsibility lies with the authors.
This article is part of the special issue “The Modular Earth Submodel System (MESSy) (ACP/GMD inter-journal SI)”. It is not associated with a conference.
We acknowledge the use of the tool CDO for the processing of data. Further, we acknowledge the EDGAR 5.0 emission data set (Crippa et al., 2019a). In addition, we are very thankful to the CLM-Community (http://clm-community.eu, last access: 13 March 2023) for providing and maintaining the COSMO-CLM model. For our study we used resources of the Deutsches Klimarechenzentrum (DKRZ) granted by its Scientific Steering Committee (WLA) under project ID bd1063. We thank Heidi Huntrieser (DLR) and two anonymous reviewers for very valuable comments that improved the manuscript.
This research has been supported by the DLR transport program (projects Data and Model-based Solutions for the Transformation of Mobility – DATAMOST – and Transport and Climate – TraK), the DLR impulse project ELK (EmissionsLandKarte), and the Helmholtz-Gemeinschaft (“Advanced Earth System Modelling Capacity (ESM)” project). The HALO deployment during EMeRGe was funded by a consortium comprising the German Research Foundation (DFG) Priority Program HALO-SPP 1294, the Institute of Atmospheric Physics of DLR, the Max Planck Society (MPG), and the Helmholtz Association.
The article processing charges for this open-access publication were covered by the German Aerospace Center (DLR).
This paper was edited by Tao Wang and reviewed by two anonymous referees.
Andrés Hernández, M. D., Hilboll, A., Ziereis, H., Förster, E., Krüger, O. O., Kaiser, K., Schneider, J., Barnaba, F., Vrekoussis, M., Schmidt, J., Huntrieser, H., Blechschmidt, A.-M., George, M., Nenakhov, V., Harlass, T., Holanda, B. A., Wolf, J., Eirenschmalz, L., Krebsbach, M., Pöhlker, M. L., Kalisz Hedegaard, A. B., Mei, L., Pfeilsticker, K., Liu, Y., Koppmann, R., Schlager, H., Bohn, B., Schumann, U., Richter, A., Schreiner, B., Sauer, D., Baumann, R., Mertens, M., Jöckel, P., Kilian, M., Stratmann, G., Pöhlker, C., Campanelli, M., Pandolfi, M., Sicard, M., Gómez-Amo, J. L., Pujadas, M., Bigge, K., Kluge, F., Schwarz, A., Daskalakis, N., Walter, D., Zahn, A., Pöschl, U., Bönisch, H., Borrmann, S., Platt, U., and Burrows, J. P.: Overview: On the transport and transformation of pollutants in the outflow of major population centres – observational data from the EMeRGe European intensive operational period in summer 2017, Atmos. Chem. Phys., 22, 5877–5924, https://doi.org/10.5194/acp-22-5877-2022, 2022. a, b, c, d
Aulinger, A., Matthias, V., Zeretzke, M., Bieser, J., Quante, M., and Backes, A.: The impact of shipping emissions on air pollution in the greater North Sea region – Part 1: Current emissions and concentrations, Atmos. Chem. Phys., 16, 739–758, https://doi.org/10.5194/acp-16-739-2016, 2016. a, b
Bieser, J., Aulinger, A., Matthias, V., Quante, M., and Denier van der Gon, H.: Vertical emission profiles for Europe based on plume rise calculations, Environ. Pollut., 159, 2935–2946, https://doi.org/10.1016/j.envpol.2011.04.030, 2011. a
Butler, T., Lupascu, A., Coates, J., and Zhu, S.: TOAST 1.0: Tropospheric Ozone Attribution of Sources with Tagging for CESM 1.2.2, Geosci. Model Dev., 11, 2825–2840, https://doi.org/10.5194/gmd-11-2825-2018, 2018. a, b, c, d, e, f, g
Butler, T., Lupascu, A., and Nalam, A.: Attribution of ground-level ozone to anthropogenic and natural sources of nitrogen oxides and reactive carbon in a global chemical transport model, Atmos. Chem. Phys., 20, 10707–10731, https://doi.org/10.5194/acp-20-10707-2020, 2020. a, b, c, d, e, f
Clappier, A., Belis, C. A., Pernigotti, D., and Thunis, P.: Source apportionment and sensitivity analysis: two methodologies with two different purposes, Geosci. Model Dev., 10, 4245–4256, https://doi.org/10.5194/gmd-10-4245-2017, 2017. a
Crippa, M., Guizzardi, D., Muntean, M., Schaaf, E., Dentener, F., van Aardenne, J. A., Monni, S., Doering, U., Olivier, J. G. J., Pagliari, V., and Janssens-Maenhout, G.: Gridded emissions of air pollutants for the period 1970–2012 within EDGAR v4.3.2, Earth Syst. Sci. Data, 10, 1987–2013, https://doi.org/10.5194/essd-10-1987-2018, 2018. a
Crippa, M., Guizzardi, D., Muntean, M., Schaaf, E., and Oreggioni, G.: EDGAR v5.0 Global Air Pollutant Emissions, European Commission, Joint Research Centre (JRC) [data set], http://data.europa.eu/89h/377801af-b094-4943-8fdc-f79a7c0c2d19 (last access: 13 March 2023), 2019a. a, b
Crippa, M., Oreggioni, G., Guizzardi, D., Muntean, M., Schaaf, E., Lo Vullo, E., Solazzo, E., Monforti-Ferrario, F., Olivier, J., and Vignati, E.: Fossil O2 and GHG emissions of all world countries, Tech. Rep. KJ-NA-29849-EN-N (online), KJ-NA-29849-EN-C (print), Luxembourg, ISBN 978-92-76-11100-9 (online), ISBN 978-92-76-11025-5 (print), https://doi.org/10.2760/687800, 2019b. a, b
Crippa, M., Solazzo, E., Huang, G., Guizzardi, D., Koffi, E., Muntean, M., Schieberle, C., Friedrich, R., and Janssens-Maenhout, G.: High resolution temporal profiles in the Emissions Database for Global Atmospheric Research, Sci. Data, 7, 121, https://doi.org/10.1038/s41597-020-0462-2, 2020. a
Dahlmann, K., Grewe, V., Ponater, M., and Matthes, S.: Quantifying the contributions of individual NOx sources to the trend in ozone radiative forcing, Atmos. Environ., 45, 2860–2868, https://doi.org/10.1016/j.atmosenv.2011.02.071, 2011. a
Deckert, R., Jöckel, P., Grewe, V., Gottschaldt, K.-D., and Hoor, P.: A quasi chemistry-transport model mode for EMAC, Geosci. Model Dev., 4, 195–206, https://doi.org/10.5194/gmd-4-195-2011, 2011. a
Delang, M., Becker, J. S., Chang, K., Serre, M. L., Cooper, O. R., Schultz, M. G., Schröder, S., Lu, X., Zhang, L., Deushi, M., Josse, B., Keller, C. A., Lamarque, J., Lin, M., Liu, J., Marécal, V., Strode, S. A., Sudo, K., Tilmes, S., Zhang, L., Cleland, S. E., Collins, E. L., Brauer, M., and West, J. J.: Mapping Yearly Fine Resolution Global Surface Ozone through the Bayesian Maximum Entropy Data Fusion of Observations and Model Output for 1990–2017, Environ. Sci. Technol., 55, 4389–4398, https://doi.org/10.1021/acs.est.0c07742, 2021. a, b
de la Paz, D., Borge, R., de Andrés, J. M., Tovar, L., Sarwar, G., and Napelenok, S. L.: Summertime tropospheric ozone source apportionment study in the Madrid region (Spain), Atmos. Chem. Phys., 24, 4949–4972, https://doi.org/10.5194/acp-24-4949-2024, 2024. a, b
Dentener, F., Drevet, J., Lamarque, J. F., Bey, I., Eickhout, B., Fiore, A. M., Hauglustaine, D., Horowitz, L. W., Krol, M., Kulshrestha, U. C., Lawrence, M., Galy-Lacaux, C., Rast, S., Shindell, D., Stevenson, D., Van Noije, T., Atherton, C., Bell, N., Bergman, D., Butler, T., Cofala, J., Collins, B., Doherty, R., Ellingsen, K., Galloway, J., Gauss, M., Montanaro, V., Müller, J. F., Pitari, G., Rodriguez, J., Sanderson, M., Solmon, F., Strahan, S., Schultz, M., Sudo, K., Szopa, S., and Wild, O.: Nitrogen and sulfur deposition on regional and global scales: A multimodel evaluation, Global Biogeochem. Cy., 20, GB4003, https://doi.org/10.1029/2005GB002672, 2006. a
Derwent, R. G., Utembe, S. R., Jenkin, M. E., and Shallcross, D. E.: Tropospheric ozone production regions and the intercontinental origins of surface ozone over Europe, Atmos. Environ., 112, 216–224, https://doi.org/10.1016/j.atmosenv.2015.04.049, 2015. a
Di Carlo, P., Aruffo, E., Biancofiore, F., Busilacchio, M., Pitari, G., Dari-Salisburgo, C., Tuccella, P., and Kajii, Y.: Wildfires impact on surface nitrogen oxides and ozone in Central Italy, Atmos. Pollut. Res., 6, 29–35, https://doi.org/10.5094/APR.2015.004, 2015. a
Di Giuseppe, F., Rémy, S., Pappenberger, F., and Wetterhall, F.: Using the Fire Weather Index (FWI) to improve the estimation of fire emissions from fire radiative power (FRP) observations, Atmos. Chem. Phys., 18, 5359–5370, https://doi.org/10.5194/acp-18-5359-2018, 2018. a
Dunker, A. M., Yarwood, G., Ortmann, J. P., and Wilson, G. M.: Comparison of Source Apportionment and Source Sensitivity of Ozone in a Three-Dimensional Air Quality Model, Environ. Sci. Technol., 36, 2953–2964, https://doi.org/10.1021/es011418f, 2002. a
Eckhardt, S., Stohl, A., Wernli, H., James, P., Forster, C., and Spichtinger, N.: A 15-Year Climatology of Warm Conveyor Belts, J. Climate, 17, 218–237, https://doi.org/10.1175/1520-0442(2004)017<0218:AYCOWC>2.0.CO;2, 2004. a
Eyring, V., Isaksen, I. S., Berntsen, T., Collins, W. J., Corbett, J. J., Endresen, O., Grainger, R. G., Moldanova, J., Schlager, H., and Stevenson, D. S.: Transport impacts on atmosphere and climate: Shipping, Atmos. Environ., 44, 4735–4771, https://doi.org/10.1016/j.atmosenv.2009.04.059, 2010. a
Fink, L., Karl, M., Matthias, V., Oppo, S., Kranenburg, R., Kuenen, J., Moldanova, J., Jutterström, S., Jalkanen, J.-P., and Majamäki, E.: Potential impact of shipping on air pollution in the Mediterranean region – a multimodel evaluation: comparison of photooxidants NO2 and O3, Atmos. Chem. Phys., 23, 1825–1862, https://doi.org/10.5194/acp-23-1825-2023, 2023. a
Fiore, A. M., Dentener, F. J., Wild, O., Cuvelier, C., Schultz, M. G., Hess, P., Textor, C., Schulz, M., Doherty, R. M., Horowitz, L. W., MacKenzie, I. A., Sanderson, M. G., Shindell, D. T., Stevenson, D. S., Szopa, S., Van Dingenen, R., Zeng, G., Atherton, C., Bergmann, D., Bey, I., Carmichael, G., Collins, W. J., Duncan, B. N., Faluvegi, G., Folberth, G., Gauss, M., Gong, S., Hauglustaine, D., Holloway, T., Isaksen, I. S. A., Jacob, D. J., Jonson, J. E., Kaminski, J. W., Keating, T. J., Lupu, A., Marmer, E., Montanaro, V., Park, R. J., Pitari, G., Pringle, K. J., Pyle, J. A., Schroeder, S., Vivanco, M. G., Wind, P., Wojcik, G., Wu, S., and Zuber, A.: Multimodel estimates of intercontinental source-receptor relationships for ozone pollution, J. Geophys. Res., 114, D04301, https://doi.org/10.1029/2008JD010816, 2009. a
Francis, X., Chemel, C., Sokhi, R., Norton, E., Ricketts, H., and Fisher, B.: Mechanisms responsible for the build-up of ozone over South East England during the August 2003 heatwave, Atmos. Environ., 45, 6880–6890, https://doi.org/10.1016/j.atmosenv.2011.04.035, 2011. a
Granier, C. and Brasseur, G. P.: The impact of road traffic on global tropospheric ozone, Geophys. Res. Lett., 30, 1086, https://doi.org/10.1029/2002GL015972, 2003. a
Grewe, V.: A generalized tagging method, Geosci. Model Dev., 6, 247–253, https://doi.org/10.5194/gmd-6-247-2013, 2013. a
Grewe, V., Brunner, D., Dameris, M., Grenfell, J. L., Hein, R., Shindell, D., and Staehelin, J.: Origin and variability of upper tropospheric nitrogen oxides and ozone at northern mid-latitudes, Atmos. Environ., 35, 3421–3433, https://doi.org/10.1016/S1352-2310(01)00134-0, 2001. a
Grewe, V., Tsati, E., and Hoor, P.: On the attribution of contributions of atmospheric trace gases to emissions in atmospheric model applications, Geosci. Model Dev., 3, 487–499, https://doi.org/10.5194/gmd-3-487-2010, 2010. a
Grewe, V., Tsati, E., Mertens, M., Frömming, C., and Jöckel, P.: Contribution of emissions to concentrations: the TAGGING 1.0 submodel based on the Modular Earth Submodel System (MESSy 2.52), Geosci. Model Dev., 10, 2615–2633, https://doi.org/10.5194/gmd-10-2615-2017, 2017. a, b, c, d, e, f, g
Guenther, A., Karl, T., Harley, P., Wiedinmyer, C., Palmer, P. I., and Geron, C.: Estimates of global terrestrial isoprene emissions using MEGAN (Model of Emissions of Gases and Aerosols from Nature), Atmos. Chem. Phys., 6, 3181–3210, https://doi.org/10.5194/acp-6-3181-2006, 2006. a
Haagen-Smith, A. J.: Chemistry and Physiology of Los Angeles smog, Ind. Eng. Chem., 44, 1342–1346, https://doi.org/10.1021/ie50510a045, 1952. a
Hauglustaine, D., Emmons, L., and Newchurch, M. E. A.: GOn the Role of Lightning NOx in the Formation of Tropospheric Ozone Plumes: A Global Model Perspective, J. Atmos. Chem., 38, 3277–3294, https://doi.org/10.1023/A:1006452309388, 2001. a
Hersbach, H., Bell, B., Berrisford, P., Hirahara, S., Horányi, A., Muñoz-Sabater, J., Nicolas, J., Peubey, C., Radu, R., Schepers, D., Simmons, A., Soci, C., Abdalla, S., Abellan, X., Balsamo, G., Bechtold, P., Biavati, G., Bidlot, J., Bonavita, M., De Chiara, G., Dahlgren, P., Dee, D., Diamantakis, M., Dragani, R., Flemming, J., Forbes, R., Fuentes, M., Geer, A., Haimberger, L., Healy, S., Hogan, R. J., Hólm, E., Janisková, M., Keeley, S., Laloyaux, P., Lopez, P., Lupu, C., Radnoti, G., de Rosnay, P., Rozum, I., Vamborg, F., Villaume, S., and Thépaut, J.-N.: The ERA5 global reanalysis, Q. J. Roy. Meteor. Soc., 146, 1999–2049, https://doi.org/10.1002/qj.3803, 2020. a
Hofmann, C., Kerkweg, A., Wernli, H., and Jöckel, P.: The 1-way on-line coupled atmospheric chemistry model system MECO(n) – Part 3: Meteorological evaluation of the on-line coupled system, Geosci. Model Dev., 5, 129–147, https://doi.org/10.5194/gmd-5-129-2012, 2012. a
Hoor, P., Borken-Kleefeld, J., Caro, D., Dessens, O., Endresen, O., Gauss, M., Grewe, V., Hauglustaine, D., Isaksen, I. S. A., Jöckel, P., Lelieveld, J., Myhre, G., Meijer, E., Olivie, D., Prather, M., Schnadt Poberaj, C., Shine, K. P., Staehelin, J., Tang, Q., van Aardenne, J., van Velthoven, P., and Sausen, R.: The impact of traffic emissions on atmospheric ozone and OH: results from QUANTIFY, Atmos. Chem. Phys., 9, 3113–3136, https://doi.org/10.5194/acp-9-3113-2009, 2009. a, b
Jacob, D.: Introduction to Atmospheric Chemistry, Princeton University Press, Princeton, New Jersey, ISBN 0691001855, 1999. a
Jimenez-Montenegro, L., López-Fernández, M., and Gimenez, E.: Worldwide Research on the Ozone Influence in Plants, Agronomy, 11, 1504, https://doi.org/10.3390/agronomy11081504, 2021. a
Jöckel, P., Tost, H., Pozzer, A., Brühl, C., Buchholz, J., Ganzeveld, L., Hoor, P., Kerkweg, A., Lawrence, M. G., Sander, R., Steil, B., Stiller, G., Tanarhte, M., Taraborrelli, D., van Aardenne, J., and Lelieveld, J.: The atmospheric chemistry general circulation model ECHAM5/MESSy1: consistent simulation of ozone from the surface to the mesosphere, Atmos. Chem. Phys., 6, 5067–5104, https://doi.org/10.5194/acp-6-5067-2006, 2006. a
Jöckel, P., Kerkweg, A., Pozzer, A., Sander, R., Tost, H., Riede, H., Baumgaertner, A., Gromov, S., and Kern, B.: Development cycle 2 of the Modular Earth Submodel System (MESSy2), Geosci. Model Dev., 3, 717–752, https://doi.org/10.5194/gmd-3-717-2010, 2010. a, b
Jöckel, P., Tost, H., Pozzer, A., Kunze, M., Kirner, O., Brenninkmeijer, C. A. M., Brinkop, S., Cai, D. S., Dyroff, C., Eckstein, J., Frank, F., Garny, H., Gottschaldt, K.-D., Graf, P., Grewe, V., Kerkweg, A., Kern, B., Matthes, S., Mertens, M., Meul, S., Neumaier, M., Nützel, M., Oberländer-Hayn, S., Ruhnke, R., Runde, T., Sander, R., Scharffe, D., and Zahn, A.: Earth System Chemistry integrated Modelling (ESCiMo) with the Modular Earth Submodel System (MESSy) version 2.51, Geosci. Model Dev., 9, 1153–1200, https://doi.org/10.5194/gmd-9-1153-2016, 2016. a, b, c, d, e
Jonson, J. E., Schulz, M., Emmons, L., Flemming, J., Henze, D., Sudo, K., Tronstad Lund, M., Lin, M., Benedictow, A., Koffi, B., Dentener, F., Keating, T., Kivi, R., and Davila, Y.: The effects of intercontinental emission sources on European air pollution levels, Atmos. Chem. Phys., 18, 13655–13672, https://doi.org/10.5194/acp-18-13655-2018, 2018. a, b
Jonson, J. E., Gauss, M., Schulz, M., Jalkanen, J.-P., and Fagerli, H.: Effects of global ship emissions on European air pollution levels, Atmos. Chem. Phys., 20, 11399–11422, https://doi.org/10.5194/acp-20-11399-2020, 2020. a, b
Karamchandani, P., Long, Y., Pirovano, G., Balzarini, A., and Yarwood, G.: Source-sector contributions to European ozone and fine PM in 2010 using AQMEII modeling data, Atmos. Chem. Phys., 17, 5643–5664, https://doi.org/10.5194/acp-17-5643-2017, 2017. a, b, c, d
Kerkweg, A. and Jöckel, P.: The 1-way on-line coupled atmospheric chemistry model system MECO(n) – Part 2: On-line coupling with the Multi-Model-Driver (MMD), Geosci. Model Dev., 5, 111–128, https://doi.org/10.5194/gmd-5-111-2012, 2012. a
Kerkweg, A., Buchholz, J., Ganzeveld, L., Pozzer, A., Tost, H., and Jöckel, P.: Technical Note: An implementation of the dry removal processes DRY DEPosition and SEDImentation in the Modular Earth Submodel System (MESSy), Atmos. Chem. Phys., 6, 4617–4632, https://doi.org/10.5194/acp-6-4617-2006, 2006. a
Kwok, R. H. F., Baker, K. R., Napelenok, S. L., and Tonnesen, G. S.: Photochemical grid model implementation and application of VOC, NOx, and O3 source apportionment, Geosci. Model Dev., 8, 99–114, https://doi.org/10.5194/gmd-8-99-2015, 2015. a
Lang, J.-P.: Climate Data Operators, Max-Planck-Institut für Meteorologie [code], https://code.mpimet.mpg.de/projects/cdo, last access: 4 December 2024. a
Logan, J. A.: Tropospheric ozone: Seasonal behavior, trends, and anthropogenic influence, J. Geophys. Res.-Atmos., 90, 10463–10482, https://doi.org/10.1029/JD090iD06p10463, 1985. a
Lupaşcu, A. and Butler, T.: Source attribution of European surface O3 using a tagged O3 mechanism, Atmos. Chem. Phys., 19, 14535–14558, https://doi.org/10.5194/acp-19-14535-2019, 2019. a, b, c, d, e, f, g, h, i
Lupaşcu, A., Otero, N., Minkos, A., and Butler, T.: Attribution of surface ozone to NOx and volatile organic compound sources during two different high ozone events, Atmos. Chem. Phys., 22, 11675–11699, https://doi.org/10.5194/acp-22-11675-2022, 2022. a
Mailler, S., Khvorostyanov, D., and Menut, L.: Impact of the vertical emission profiles on background gas-phase pollution simulated from the EMEP emissions over Europe, Atmos. Chem. Phys., 13, 5987–5998, https://doi.org/10.5194/acp-13-5987-2013, 2013. a
Markakis, K., Valari, M., Perrussel, O., Sanchez, O., and Honore, C.: Climate-forced air-quality modeling at the urban scale: sensitivity to model resolution, emissions and meteorology, Atmos. Chem. Phys., 15, 7703–7723, https://doi.org/10.5194/acp-15-7703-2015, 2015. a
Matthes, S., Grewe, V., Sausen, R., and Roelofs, G.-J.: Global impact of road traffic emissions on tropospheric ozone, Atmos. Chem. Phys., 7, 1707–1718, https://doi.org/10.5194/acp-7-1707-2007, 2007. a
Matthias, V., Bewersdorff, I., Aulinger, A., and Quante, M.: The contribution of ship emissions to air pollution in the North Sea regions, Environ. Pollut., 158, 2241–2250, https://doi.org/10.1016/j.envpol.2010.02.013, 2010. a
Matthias, V., Aulinger, A., Backes, A., Bieser, J., Geyer, B., Quante, M., and Zeretzke, M.: The impact of shipping emissions on air pollution in the greater North Sea region – Part 2: Scenarios for 2030, Atmos. Chem. Phys., 16, 759–776, https://doi.org/10.5194/acp-16-759-2016, 2016. a
Mebust, A. K., Russell, A. R., Hudman, R. C., Valin, L. C., and Cohen, R. C.: Characterization of wildfire NOx emissions using MODIS fire radiative power and OMI tropospheric NO2 columns, Atmos. Chem. Phys., 11, 5839–5851, https://doi.org/10.5194/acp-11-5839-2011, 2011. a
Mertens, M., Kerkweg, A., Jöckel, P., Tost, H., and Hofmann, C.: The 1-way on-line coupled model system MECO(n) – Part 4: Chemical evaluation (based on MESSy v2.52), Geosci. Model Dev., 9, 3545–3567, https://doi.org/10.5194/gmd-9-3545-2016, 2016. a, b, c, d
Mertens, M., Grewe, V., Rieger, V. S., and Jöckel, P.: Revisiting the contribution of land transport and shipping emissions to tropospheric ozone, Atmos. Chem. Phys., 18, 5567–5588, https://doi.org/10.5194/acp-18-5567-2018, 2018. a, b, c, d, e
Mertens, M., Kerkweg, A., Grewe, V., Jöckel, P., and Sausen, R.: Are contributions of emissions to ozone a matter of scale? – a study using MECO(n) (MESSy v2.50), Geosci. Model Dev., 13, 363–383, https://doi.org/10.5194/gmd-13-363-2020, 2020a. a, b, c, d
Mertens, M., Kerkweg, A., Grewe, V., Jöckel, P., and Sausen, R.: Attributing ozone and its precursors to land transport emissions in Europe and Germany, Atmos. Chem. Phys., 20, 7843–7873, https://doi.org/10.5194/acp-20-7843-2020, 2020b. a, b, c, d, e, f, g, h, i, j, k, l, m, n, o, p, q, r, s, t, u, v, w, x
Mertens, M., Jöckel, P., Matthes, S., Nützel, M., Grewe, V., and Sausen, R.: COVID-19 induced lower-tropospheric ozone changes, Environ. Res. Lett., 16, 064005, https://doi.org/10.1088/1748-9326/abf191, 2021. a, b
Mertens, M., Brinkop, S., Graf, P., Grewe, V., Hendricks, J., Jöckel, P., Lanteri, A., Matthes, S., Rieger, V. S., Righi, M., and Thor, R. N.: The contribution of transport emissions to ozone mixing ratios and methane lifetime in 2015 and 2050 in the Shared Socioeconomic Pathways (SSPs), Atmos. Chem. Phys., 24, 12079–12106, https://doi.org/10.5194/acp-24-12079-2024, 2024. a
Monks, P.: Gas-Phase Radical Chemistry in the Troposphere, Chem. Soc. Rev., 34, 376–395, https://doi.org/10.1039/b307982c, 2005. a
Myhre, G., Shindell, D., Bréon, F.-M., Collins, W., Fuglestvedt, J., Huang, J., Koch, D., Lamarque, J.-F., Lee, D., Mendoza, B., Nakajima, T., Robock, A., Stephens, G., Takemura, T., and Zhang, H.: Anthropogenic and natural radiative forcing, Cambridge University Press, Cambridge, UK, 659–740, https://doi.org/10.1017/CBO9781107415324.018, 2013. a
Ou, J., Huang, Z., Klimont, Z., Jia, G., Zhang, S., Li, C., Meng, J., Mi, Z., Zheng, H., Shan, Y., Louie, P., Zheng, J., and Guan, D.: Role of export industries on ozone pollution and its precursors in China, Nat. Commun., 11, 5492, https://doi.org/10.1038/s41467-020-19035-x, 2020. a
Pay, M. T., Gangoiti, G., Guevara, M., Napelenok, S., Querol, X., Jorba, O., and Pérez García-Pando, C.: Ozone source apportionment during peak summer events over southwestern Europe, Atmos. Chem. Phys., 19, 5467–5494, https://doi.org/10.5194/acp-19-5467-2019, 2019. a, b, c, d, e, f
Real, E., Megaritis, A., Colette, A., Valastro, G., and Messina, P.: Atlas of ozone chemical regimes in Europe, Atmos. Environ., 320, 120323, https://doi.org/10.1016/j.atmosenv.2023.120323, 2024. a
Rieger, V. S., Mertens, M., and Grewe, V.: An advanced method of contributing emissions to short-lived chemical species (OH and HO2): the TAGGING 1.1 submodel based on the Modular Earth Submodel System (MESSy 2.53), Geosci. Model Dev., 11, 2049–2066, https://doi.org/10.5194/gmd-11-2049-2018, 2018. a, b
Rockel, B., Will, A., and Hense, A.: The regional climate model COSMO-CLM (CCLM), Meteorol. Z., 17, 347–348, https://doi.org/10.1127/0941-2948/2008/0309, 2008. a
Sander, R., Baumgaertner, A., Gromov, S., Harder, H., Jöckel, P., Kerkweg, A., Kubistin, D., Regelin, E., Riede, H., Sandu, A., Taraborrelli, D., Tost, H., and Xie, Z.-Q.: The atmospheric chemistry box model CAABA/MECCA-3.0, Geosci. Model Dev., 4, 373–380, https://doi.org/10.5194/gmd-4-373-2011, 2011. a
Schumann, U. and Huntrieser, H.: The global lightning-induced nitrogen oxides source, Atmos. Chem. Phys., 7, 3823–3907, https://doi.org/10.5194/acp-7-3823-2007, 2007. a, b
Seinfeld, J. and Pandis, S.: Atmospheric Chemistry and Physics: From Air Pollution to Climate Change, John Wiley and Sons, New York, ISBN 978-0-471-72017-1, 2006. a
Sillman, S.: The relation between ozone, NOx and hydrocarbons in urban and polluted rural environments, Atmos. Environ., 33, 1821–1845, https://doi.org/10.1016/S1352-2310(98)00345-8, 1999. a, b
Simpson, D.: Biogenic emissions in Europe: 2. Implications for ozone control strategies, J. Geophys. Res.-Atmos., 100, 22891–22906, https://doi.org/10.1029/95JD01878, 1995. a
Solmon, F., Sarrat, C., Serça, D., Tulet, P., and Rosset, R.: Isoprene and monoterpenes biogenic emissions in France: Modeling and impact during a regional pollution episode, Atmos. Environ., 38, 3853–3865, https://doi.org/10.1016/j.atmosenv.2004.03.054, 2004. a
Stohl, A., Eckhardt, S., Forster, C., James, P., and Spichtinger, N.: On the pathways and timescales of intercontinental air pollution transport, J. Geophys. Res.-Atmos., 107, ACH 6-1–ACH 6-17, https://doi.org/10.1029/2001JD001396, 2002. a
Tagaris, E., Sotiropoulou, R. E. P., Gounaris, N., Andronopoulos, S., and Vlachogiannis, D.: Effect of the Standard Nomenclature for Air Pollution (SNAP) Categories on Air Quality over Europe, Atmosphere, 6, 1119, https://doi.org/10.3390/atmos6081119, 2015. a, b
Tie, X., Brasseur, G., and Ying, Z.: Impact of model resolution on chemical ozone formation in Mexico City: application of the WRF-Chem model, Atmos. Chem. Phys., 10, 8983–8995, https://doi.org/10.5194/acp-10-8983-2010, 2010. a
Tost, H., Jöckel, P., Kerkweg, A., Sander, R., and Lelieveld, J.: Technical note: A new comprehensive SCAVenging submodel for global atmospheric chemistry modelling, Atmos. Chem. Phys., 6, 565–574, https://doi.org/10.5194/acp-6-565-2006, 2006. a
Tost, H., Jöckel, P., and Lelieveld, J.: Lightning and convection parameterisations – uncertainties in global modelling, Atmos. Chem. Phys., 7, 4553–4568, https://doi.org/10.5194/acp-7-4553-2007, 2007. a
Tost, H., Lawrence, M. G., Brühl, C., Jöckel, P., The GABRIEL Team, and The SCOUT-O3-DARWIN/ACTIVE Team: Uncertainties in atmospheric chemistry modelling due to convection parameterisations and subsequent scavenging, Atmos. Chem. Phys., 10, 1931–1951, https://doi.org/10.5194/acp-10-1931-2010, 2010. a
Travis, K. R. and Jacob, D. J.: Systematic bias in evaluating chemical transport models with maximum daily 8 h average (MDA8) surface ozone for air quality applications: a case study with GEOS-Chem v9.02, Geosci. Model Dev., 12, 3641–3648, https://doi.org/10.5194/gmd-12-3641-2019, 2019. a
Vieno, M., Dore, A. J., Stevenson, D. S., Doherty, R., Heal, M. R., Reis, S., Hallsworth, S., Tarrason, L., Wind, P., Fowler, D., Simpson, D., and Sutton, M. A.: Modelling surface ozone during the 2003 heat-wave in the UK, Atmos. Chem. Phys., 10, 7963–7978, https://doi.org/10.5194/acp-10-7963-2010, 2010. a
Vinken, G. C. M., Boersma, K. F., Maasakkers, J. D., Adon, M., and Martin, R. V.: Worldwide biogenic soil NOx emissions inferred from OMI NO2 observations, Atmos. Chem. Phys., 14, 10363–10381, https://doi.org/10.5194/acp-14-10363-2014, 2014. a, b, c
Wang, Z. S., Chien, C.-J., and Tonnesen, G. S.: Development of a tagged species source apportionment algorithm to characterize three-dimensional transport and transformation of precursors and secondary pollutants, J. Geophys. Res.-Atmos., 114, D21206, https://doi.org/10.1029/2008JD010846, 2009. a
World Health Organisation (WHO): Health aspects of air pollution with particulate matter, ozone and nitrogen dioxide: report on a WHO working group, Bonn, Germany, 13–15 January 2003, World Health Organisation, https://iris.who.int/handle/10665/107478, 2003. a
Yienger, J. J. and Levy II, H.: Empirical model of global soil-biogenic NOx emissions, J. Geophys. Res.-Atmos., 100, 11447–11464, https://doi.org/10.1029/95JD00370, 1995. a, b, c
Ziereis, H., Hoor, P., Grooß, J.-U., Zahn, A., Stratmann, G., Stock, P., Lichtenstern, M., Krause, J., Bense, V., Afchine, A., Rolf, C., Woiwode, W., Braun, M., Ungermann, J., Marsing, A., Voigt, C., Engel, A., Sinnhuber, B.-M., and Oelhaf, H.: Redistribution of total reactive nitrogen in the lowermost Arctic stratosphere during the cold winter 2015/2016, Atmos. Chem. Phys., 22, 3631–3654, https://doi.org/10.5194/acp-22-3631-2022, 2022. a
- Abstract
- Introduction
- Model simulations
- Model evaluation
- Source attribution results
- Contributions during periods of large ozone mixing ratios
- Ozone production efficiency
- Discussion
- Conclusions
- Code and data availability
- Author contributions
- Competing interests
- Disclaimer
- Special issue statement
- Acknowledgements
- Financial support
- Review statement
- References
- Supplement
- Abstract
- Introduction
- Model simulations
- Model evaluation
- Source attribution results
- Contributions during periods of large ozone mixing ratios
- Ozone production efficiency
- Discussion
- Conclusions
- Code and data availability
- Author contributions
- Competing interests
- Disclaimer
- Special issue statement
- Acknowledgements
- Financial support
- Review statement
- References
- Supplement