the Creative Commons Attribution 4.0 License.
the Creative Commons Attribution 4.0 License.
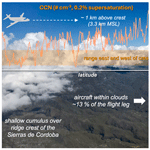
Large spatiotemporal variability in aerosol properties over central Argentina during the CACTI field campaign
Jerome D. Fast
Adam C. Varble
Mikhail Pekour
Jason Tomlinson
Alla Zelenyuk
Art J. Sedlacek III
Maria Zawadowicz
Louisa Emmons
Few field campaigns with extensive aerosol measurements have been conducted over continental areas in the Southern Hemisphere. To address this data gap and better understand the interactions of convective clouds and the surrounding environment, extensive in situ and remote sensing measurements were collected during the Cloud, Aerosol, and Complex Terrain Interactions (CACTI) field campaign conducted between October 2018 and April 2019 over the Sierras de Córdoba range of central Argentina. This study describes measurements of aerosol number, size, composition, mixing state, and cloud condensation nuclei (CCN) collected on the ground and from a research aircraft during 7 weeks of the campaign. Large spatial and multiday variations in aerosol number, size, composition, and CCN were observed due to transport from upwind sources controlled by mesoscale to synoptic-scale meteorological conditions. Large vertical wind shears, back trajectories, single-particle measurements, and chemical transport model predictions indicate that different types of emissions and source regions, including biogenic emissions and biomass burning from the Amazon and anthropogenic emissions from Chile and eastern Argentina, contribute to aerosols observed during CACTI. Repeated aircraft measurements near the boundary layer top reveal strong spatial and temporal variations in CCN and demonstrate that understanding the complex co-variability of aerosol properties and clouds is critical to quantify the impact of aerosol–cloud interactions. In addition to quantifying aerosol properties in this data-sparse region, these measurements will be valuable to evaluate predictions over the midlatitudes of South America and improve parameterized aerosol processes in local, regional, and global models.
- Article
(14386 KB) - Full-text XML
-
Supplement
(8683 KB) - BibTeX
- EndNote
Earth system models (ESMs), high-resolution models, and observations are key tools for improving our understanding of the natural and human-influenced atmospheric processes affecting Earth's climate. Despite recent scientific advances, models still contain biases arising from knowledge gaps and imperfect parameterizations of important atmospheric processes. The impacts of these biases are multifaceted, but they make important contributions to uncertainties in the net change of the Earth system energy balance between preindustrial and present-day periods. Much of this uncertainty has been attributed to current understanding and/or representation of aerosol–cloud interaction (ACI) processes, and the magnitude of this uncertainty among ESM predictions has remained unchanged for Intergovernmental Panel on Climate Change (IPCC) assessments since 1995 (Seinfeld et al., 2016; Carslaw et al., 2018).
Aerosols are known to perturb cloud hydrometeors, albedo, growth, dissipation, lifetime, and precipitation (Twomey, 1974; Albrecht, 1989; Rosenfeld et al., 2014) that subsequently influence climate over long time scales. ACI processes depend on the co-variability of aerosol and cloud properties. While the impact of aerosols on shallow marine stratocumulus has been studied extensively (e.g., Twohy et al., 2005; Wood et al., 2011; Feingold et al., 2024), there are few measurements that characterize the spatiotemporal variability of key aerosol properties in the presence of convective cloud populations that can be observed routinely by satellites. One challenge for models is that the spatiotemporal variability of aerosols and convective clouds is often subgrid scale (e.g., Fast et al., 2022). While models often contain parameterizations of subgrid-scale variability for certain cloud types, they usually assume aerosols are constant within a grid cell, which could lead to erroneous estimates of the impact of ACI. In addition to the model resolution of intersecting aerosol and cloud properties, there are complex ACI pathways for convective clouds that are still highly uncertain (Fan et al., 2016; Varble et al., 2023) for many reasons.
Aircraft in situ and remote sensing data provide key spatiotemporal measurements of aerosol properties needed to develop an improved understanding of aerosol processes and evaluate and improve models. Aircraft campaigns are usually relatively short (i.e., a few weeks or less) and do not have the global coverage of satellite and ground measurements. Most aircraft field campaigns that include aerosol measurements have been conducted over the Northern Hemisphere (Reddington et al., 2017; Watson-Parris et al., 2019). Field campaigns in the Southern Hemisphere have been conducted in the tropics, such as the Green Ocean Amazon Experiment (GoAmazon; Martin et al., 2017), or over the Southern Hemisphere ocean, such as the VAMOS Ocean-Cloud-Atmosphere-Land Study Regional Experiment (VOCALS-REx; Wood et al., 2011); the Southern Ocean Clouds, Radiation, Aerosol Transport Experimental Study (SOCRATES; McFarquhar et al., 2021); and the combined CLoud–Aerosol–Radiation Interaction and Forcing for Year 2017 (CLARIFY-2017), ObseRvations of Aerosols above CLouds and their intEractionS (ORACLES), and Layered Atlantic Smoke and Interactions with Clouds (LASIC) experiments conducted over the southeast Atlantic Ocean (Barrett et al., 2022). Global aircraft campaigns, such as the HIAPER Pole-to-Pole Observations (HIPPO; Wofsy et al., 2011) and the Atmospheric Tomography Mission (AToM; Brock et al., 2019), obtained snapshots of aerosol measurements over both hemispheres, primarily over the ocean. Few aerosol field campaigns with extensive aerosol measurements, however, have been conducted over subtropical and midlatitude continental areas in the Southern Hemisphere.
To address this data gap and better understand the interactions of convective clouds and the surrounding environment, extensive in situ and remote sensing measurements were collected during the Cloud, Aerosol, and Complex Terrain Interactions (CACTI) field campaign conducted between October 2018 and April 2019 over the Sierras de Córdoba range of central Argentina (Varble et al., 2021). The U.S. Department of Energy's Atmospheric Radiation Measurement (ARM) mobile facility (AMF; Mather and Voyles, 2013) and G-1 research aircraft (Schmid et al., 2014) were deployed during CACTI. The field campaign was designed to address science questions related to how orographic convective clouds interact with and depend on environmental conditions, thermodynamics, aerosols, and surface properties. CACTI occurred at the same time and in the same region as the National Science Foundation (NSF)-led Remote sensing of Electrification, Lightning, And Mesoscale/microscale Processes with Adaptive Ground Observations (RELAMPAGO) field campaign (Nesbitt et al., 2021). Observations from CACTI have been used to characterize the range of deep convective cloud life cycles and their relationships with ambient environments (Feng et al., 2022, 2023), determine the environmental conditions favorable for deep convection initiation (Marquis et al., 2021, 2023; Nelson et al., 2022), identify factors affecting rain rates in warm clouds (Borque et al., 2022), examine the relationship between the depth of convective cores and aerosol concentrations (Veals et al., 2022), evaluate the ability of kilometer-scale simulations to represent the characteristics of mesoscale convective systems (Zhang et al., 2021), and determine the influence of the South American low-level jet on the convective environment (Sasaki et al., 2024).
In contrast with the previous studies that focus on clouds, this paper describes measurements of aerosol number, size, composition, mixing state, and cloud condensation nuclei (CCN) collected by the AMF and G-1 platforms during the CACTI campaign. It is important to understand the spatiotemporal variation of aerosol properties before assessing how those properties influence convective clouds forming along the Sierras de Córdoba (SDC) range. CACTI measurements are analyzed to demonstrate the multiday and diurnal variations in aerosol properties on the ground as well as the vertical and horizontal variations of aerosols aloft. As will be shown later, large multiday variations in aerosol number, size, composition, and CCN were observed due to regional to long-range transport from upwind sources that is controlled by mesoscale to synoptic-scale meteorological processes. Since repeated aircraft measurements near the boundary layer top reveal strong spatial and temporal variations in CCN, inferring the impact of aerosols on convective clouds over the region will be challenging.
2.1 Ground measurements
A wide range of continuous meteorological, radiation, and aerosol measurements were collected on the ground by the ARM mobile facility (AMF; Mather and Voyles, 2013) during CACTI between 15 October 2018 and 30 April 2019. As shown in Fig. 1a, the AMF was located along the eastern slope of the SDC at an elevation of 1141 m a.m.s.l. The mountains along the continuous ∼ 300 km long north–south crest of the SDC are as high as ∼ 2790 m a.m.s.l. About 100 km northeast of the AMF site is Córdoba, the largest city in the region, with an urban population of ∼ 2.1 million. Rio Cuarto, ∼ 130 km southeast of the AMF site, is the second largest city in the region, with a population of nearly 180 000. Alta Gracia and Rio Tercero are ∼ 60 km to the northeast and east, respectively, with each having a population of ∼ 45 000, while Villa Carlos Paz, 80 km to the north, has a population of 75 000. Villa Dolores, 50 km to the west on the other side of the SDC crest, has a population of 30 000. The SDC and the lower slopes surrounding mountain range, however, are much less populated, with no more than a handful of towns having populations near 10 000.
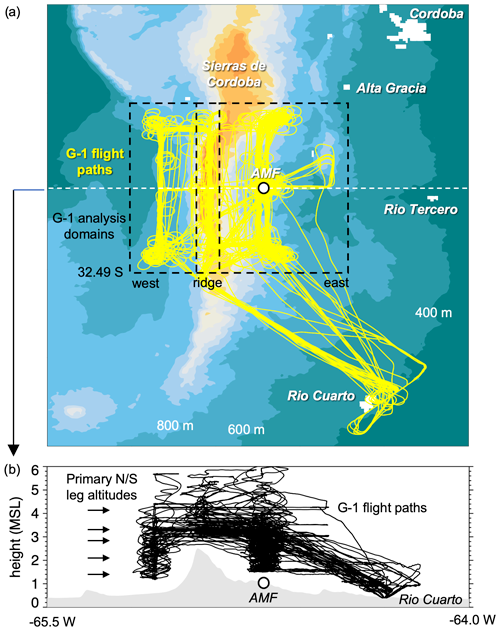
Figure 1(a) Topography of Sierras de Córdoba and the location of the AMF ground site and horizontal G-1 flight paths and (b) west–east cross section of topography at the AMF latitude and the altitude of the G-1 flight paths. In panel (a), the white cells denote urban areas and dashed magenta lines denote three analysis domains for the G-1 data.
Near-surface winds (Kyrouac et al., 2018) during the CACTI campaign were predominately from the northeast; otherwise, the winds were usually from the east to southeast (Fig. S1a in the Supplement). At about 2.5 km a.m.s.l., which is often within the afternoon convective boundary layer, winds obtained from the radiosondes (3–5 d−1; Keeler et al., 2018) were mostly from the north to northeast (Fig. S1b). Winds were usually from the northwest in the transition zone between the daytime convective boundary layer and free troposphere at 3 km a.m.s.l. (Fig. S1c) and were predominantly from the west at higher altitudes such as those at 4.5 km a.m.s.l. (Fig. S1d). The lower frequency of easterly winds suggests that aerosols originating from the most populated areas of Argentina near Buenos Aires are not often directly transported to the AMF site. However, they may be transported to the site by more complex circulations. The common directional vertical wind shear indicates that aerosols measured at the AMF site could originate from different locations at the same time, as will be discussed later.
Table 1Aerosol measurements, instruments, and sampling rates between 15 October 2018 and 30 April 2019 at the AMF site.
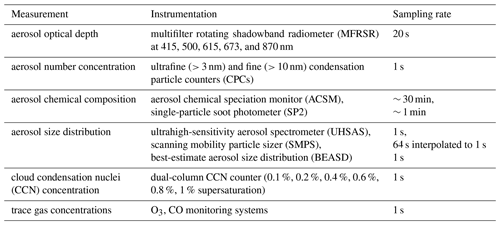
The AMF aerosol measurements used in this study are listed in Table 1. Measurements of aerosol optical depth at five wavelengths, total aerosol number concentration, aerosol chemical composition, aerosol size distribution, and CCN concentrations at six supersaturations were collected during the 6.5-month period. Measurements of scattering (nephelometer) and absorption (particle soot absorption photometer) by aerosols were also collected but are not used in this study. Aerosol optical depth at five wavelengths is derived from the multifilter rotating shadowband radiometer (MFRSR) during the day when the sky is relatively free of clouds (Koontz et al., 2018a). Two particle condensation counters (CPCs) obtained the total aerosol number concentration for particle diameters greater than 3 (Koontz et al., 2018b) and 10 nm (Koontz et al., 2018c). The aerosol chemical speciation monitor (ACSM; Ng et al., 2011) measures bulk non-refractory organic matter, sulfate, nitrate, ammonium, and chloride for particle sizes less than 1 µm (Zawadowicz et al., 2018). Refractory black carbon (rBC) concentrations and size distributions are measured by a single-particle soot photometer (SP2; Schwarz et al., 2006). The scanning mobility particle sizer (SMPS) obtained aerosol concentration for 106 size bins ranging from 10.9 to 495.8 nm (Kuang et al., 2018). The ultrahigh-sensitivity aerosol spectrometer (UHSAS) collected aerosol concentrations for 299 size bins between 55.8 and 985.5 nm (Uin et al., 2018). The SMPS and UHSAS size distribution was combined to obtain a single aerosol size distribution, with the SMPS and UHSAS values merged around diameters of 260 nm. The first column of the CCN counter cycles through six supersaturations, with measurements at each supersaturation lasting ∼ 10 min (Koontz et al., 2018d), and the second column continuously samples CCN at 0.4 % supersaturation (Koontz et al., 2018e). Trace gas measurements consisted of carbon monoxide (CO; Koontz et al., 2018f) and ozone (O3; Springston et al., 2018) and did not include aerosol precursor gas-phase species. Additional details on these instruments and a description of the meteorological and radiation measurements are presented in Varble et al. (2019, 2021).
While the surface measurements were collected between 15 October 2018 and 30 April 2019, we analyze only those measurements a few days before and after the aircraft sampling period. Describing the seasonal variability of the aerosol properties is not a focus of this study.
2.2 Aircraft measurements
The G-1 aircraft (Schmid et al., 2014) collected a wide range of meteorological, radiation, trace gas, and aerosol measurements around the AMF site over 79.4 h on 22 d between 4 November and 8 December 2018. Flight durations were usually ∼ 4 h, although flight durations on a few days were closer to ∼ 2 h. All flights were conducted between 09:15 and 17:15 LT, and flights were during either the morning, midday, or the afternoon. All the flight paths that originated at the Rio Cuarto airport where aircraft operations were based are depicted in Fig. 1a. Most of the science flight time was spent along north–south transects at constant altitudes over the AMF site, over the crest of the mountain range, and over the western slope of the of the SDC. The constant altitude flight legs were conducted below ∼ 4 km a.m.s.l., although some profiles were made up to 6 km a.m.s.l. as shown in Fig. 1b. These aircraft measurements describe the spatiotemporal variability of aerosol properties in the boundary layer and free troposphere over the same region where deep convection frequently forms (Feng et al., 2022).
One of the objectives of CACTI was to determine how environmental conditions (including aerosol properties) influence convective cloud life cycles and how those convective clouds in turn alter aerosol properties; therefore, many of the constant altitude flight legs were at or just below cloud base. Cloud sampling was usually done within shallow cumulus or cumulus congestus, most frequently observed to form along the crest of the SDC. Three out of the 22 G-1 flights were conducted on clear-sky days to sample boundary layer and lower-free-troposphere aerosol properties.
Profiles of aerosol properties in the vicinity of the AMF site were likely influenced by vertical wind shears, boundary layer mixing, convective updrafts and downdrafts, and cloud processing. However, few true profiles through the depth of the boundary layer and lower free troposphere were made since the flight paths usually consisted of constant altitude legs connected by short vertical ascents/descents between those legs. As described later, the aircraft sampling strategy combined with the large spatiotemporal variability of aerosol properties complicates the interpretation of vertical profiles of aerosol properties within a short time window.
Table 2G-1 aircraft aerosol measurements, instrumentation, and sampling rate between 4 November and 8 December 2018.
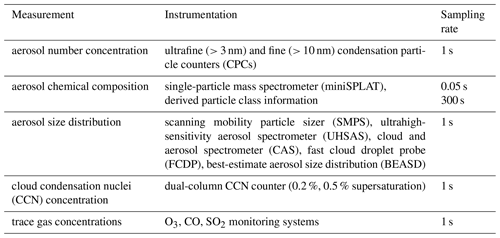
Table 2 lists the G-1 aircraft aerosol measurements used in this study. Two CPC instruments, with 3 and 10 nm lower cutoffs, were deployed on the aircraft to provide the same type of total aerosol number concentrations as the ground site (Koontz et al., 2018g, h). The miniSPLAT (Zelenyuk et al., 2015) instrument was deployed to measure the size and chemical composition of thousands of individual particles with diameters from 50 to 2000 nm. miniSPLAT detects 50 % of 85 nm diameter particles and 100 % of spherical particles in the size range 125 to 600 nm. While miniSPLAT does not produce bulk concentrations of aerosol composition like a high-resolution time-of-flight aerosol mass spectrometer (HR-ToF-AMS; DeCarlo et al., 2006), it does provide information on size and composition of individual aerosol particles, which determine aerosol activation into cloud droplets (e.g., Saliba et al., 2023). miniSPLAT measures the size, nonrefractory composition, and refractory composition (e.g., soot, sea salt, dust) of several hundreds of individual particles per minute to obtain information on aerosol mixing state. The single-particle mass spectra were classified into hundreds of clusters, which for simplicity have been subsequently combined into 14 distinct, physically meaningful, aerosol types or classes (Zelenyuk et al., 2015). Aerosol size distribution was obtained from SMPS (Mei and Pekour, 2018), UHSAS (Tomlinson, 2018), passive-cavity aerosol spectrometer probe (PCASP; Marinovici and Tomlinson, 2018), cloud and aerosol spectrometer (CAS; Cromwell et al., 2018), and fast cloud droplet probe (FCDP; Mei et al., 2018) instruments. CAS and FCDP are usually used to obtain droplet size distribution, but they also provide coarse-mode aerosol number concentration outside of clouds with additional data processing. The best-estimate aerosol size distribution (BEASD) ARM data product (Pekour and Ermold, 2023) merges data from these four instruments to produce 35 size bins from 15 nm to 9.69 µm and is used for our analyses of aircraft aerosol size distributions. In contrast to the ground CCN instrument, continuous measurements of CCN were obtained at 0.2 % and 0.5 % supersaturations (Koontz et al., 2018i, j). Trace gas measurements included sulfur dioxide (SO2; Burk et al., 2018a) in addition to CO (Burk and Ermold, 2018) and O3 (Burk et al., 2018b). The SO2 monitor was able to detect large sulfur dioxide plumes greater than ∼ 1 ppb. SO2 data below ∼ 1 ppb were quite noisy and cannot be used to examine spatial variability at part per trillion (ppt) levels. As with the ground instrumentation, there were no measurements of trace gas aerosol precursors. The 1 s sampling rate combined with a G-1 flight speed of 100 m s−1 results in aerosol measurements over 100 m distances.
2.3 CAM-Chem description
The Community Atmosphere Model with chemistry (CAM-chem), a component of the Community Earth System Model (Danabasoglu et al., 2020), is used to illustrate transport pathways of smoke during CACTI. This version of the model uses the MOZART-TS1 chemical mechanism (Emmons et al., 2020) and the Modal Aerosol Model version 4 (MAM4; Liu et al., 2016; Tilmes et al., 2019). The meteorological fields are nudged to the Modern-Era Retrospective analysis for Research and Applications, Version 2 (MERRA-2) meteorological analyses. Simulation output with a horizontal grid spacing of 1.25 × 0.94° is available (Tilmes et al., 2022) at 6 h intervals from 2001–2020.
3.1 Multiday and diurnal variability of ground aerosol measurements
Even though the SDC is not heavily populated, Fig. 2a shows large multiday variations in aerosol mass for particles less than 1 µm in diameter (PM1) from the ACSM and black carbon from the SP2. For example, PM1 concentrations were as high as 15.8 µg m−3 on 2 November and close to zero on days with rain (25–26 October, 11–12, 26–27 November).
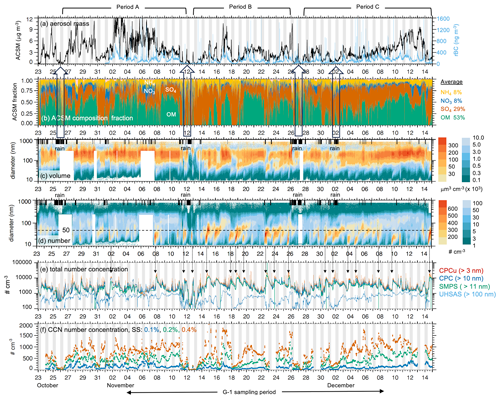
Figure 2Time series of (a) PM1 and rBC concentration, (b) ACSM composition fraction, (c) aerosol volume distribution, (d) aerosol number distribution, (e) total number concentration, and (f) CCN concentration at three supersaturations, focusing on G-1 flights during the first 2 months of CACTI.
To compare the overall multiday variability in aerosol properties during the G-1 sampling period, much of the subsequent analysis averages aerosol properties over three periods denoted as A, B, and C in Fig. 2. The heaviest rainfall (Fig. S2a) marks the beginning and end of periods A and B, which also reflect changes in the synoptic conditions over the AMF site. Intermittent rain events occur on several days during periods A and C, but it rarely rained at the AMF site during period B. As will be shown later, average aerosol properties differ between these periods. For example, ultrafine-particle concentrations are the lowest and highest during periods A and B, respectively. The highest PM1 concentrations occurred between the rain events on 25–26 October and 11–12 November (period A, Fig. S2a and b). Between the 11–12 and 26–27 November rain events (period B), the lowest PM1 concentrations occurred. After 27 November (period C), PM1 concentrations increase somewhat but are still lower than during period A. In addition to wet scavenging, the large day-to-day variability is likely due to interactions of synoptic and terrain-induced circulations that transport biogenic, anthropogenic, and biomass burning aerosols from different source regions to the site.
The temporal variation of PM1 is somewhat correlated with AOD (r = 0.56 and 0.52 at 415 and 500 nm, respectively, Fig. S3a), suggesting that aerosols in the boundary layer contribute to a large fraction of the column integrated extinction. Note that AOD measurements are missing on 43 % of the daytime periods due to partly to mostly cloudy conditions as indicated by the KAZR-ARSCL (Ka-band ARM Zenith Radar Active Remote Sensing of CLouds) ARM product (Johnson et al., 2018; Fig. S3b). Relatively high and low PM1 concentrations and AOD are often associated with northerly and southerly winds, respectively, as will be shown later. While multiday trends in PM1 and AOD are similar, they differ over several hours on some days (e.g., 23 November), indicating the presence of aerosol layers in the free troposphere that significantly contribute to column extinction.
Figure 2b shows that most of the PM1 mass is comprised of organic matter (OM; 53 % on average) and sulfate (SO4; 29 % on average). The relative contribution of OM is larger on days with relatively high PM1 concentrations, while the relative contribution of SO4 is usually larger on days when PM1 concentrations are less than 1 µg m−3. Nitrate (NO3) and ammonium (NH4) each comprise 8 % of the PM1 concentrations on average between 23 October and 15 December. While the fraction of NH4 does not vary significantly during the campaign, the fraction of NO3 varies substantially. Though NO3 is very small on many days, it contributes to over 20 % of the total mass during 6 % of this measurement period. OM, SO4, NH4, and NO3 concentrations all exhibited similar variations to PM1 among periods A, B, and C (Fig. S2a–e).
Temporal variations in the volume size distribution (Fig. 2c) are consistent with the variability in PM1, with volume decreasing dramatically during rain events. Outside of rain events, peak aerosol volume usually occurs between 200 and 300 nm. The 25th to 75th percentiles of accumulation mode particles (> 100 nm) are the largest during periods A and C and the lowest during period B (Fig. S2f), consistent with the variations in PM1 from the ACSM. Nevertheless, there are a few times during period B with the highest accumulation mode number concentrations as indicated by the largest 95th percentile. Temporal variations in aerosol number distributions (Fig. 2d) and total aerosol number concentrations (Fig. 2e) reveal that new particle formation (NPF) events occur on many days that produce large numbers of ultrafine particles (UFPs, diameter < 50 nm). While rain removes a large fraction of accumulation mode particles, smaller particles are not removed entirely by wet scavenging. In addition to differences in the meteorology and trace gas precursors between the rain events, NPF and growth are mostly likely controlled by the presence or absence of accumulation mode aerosols. Since gas-phase aerosol precursors preferentially condense on the largest particles, the higher overall PM1 concentrations during period A (Fig. S2f) suppress the formation and growth of UFP (Fig. S2g). Conversely, the lower PM1 concentrations during period B (Fig. S2f) permit more small particles to form (Fig. S2g) and grow by condensation of trace gas precursors. This effect is illustrated in Fig. S4, which indicates that peak number concentrations (dominated by UFPs) occur during periods with relatively low surface area (dominated by accumulation mode particles); therefore, there are fewer accumulation mode particles to compete as a condensational sink. While NPF events resume a few days after the rain on 26 and 27 November, accumulation mode aerosols and PM1 concentrations slowly increase after 6 December and suppress the formation and growth of UFP. Thus, UFP and Aitken mode number concentrations during period C are somewhat lower than during period B but higher than during period A (Fig. S2g).
Concentrations of CCN are a function of aerosol number, size, and hygroscopicity (Kohler, 1936; Petters and Kreidenweis, 2007). As with PM1 and accumulation mode aerosols, CCN concentrations decrease dramatically during rain events (Fig. 2f). The temporal variations in CCN are similar to variations in number concentrations of particles larger than 100 nm with a correlation coefficient (r) of 0.68, 0.88, and 0.86 and a linear regression slope of 0.18, 0.63, and 1.10 for supersaturations 0.1 %, 0.2 %, and 0.4 %, respectively. Therefore, differences in CCN concentrations (Fig. S5a) among the three periods are similar to those from the accumulation mode aerosols (Fig. S2e); however, the 95th percentile for CCN at 0.4 % supersaturation is the highest during period B because particles with smaller diameters (usually with higher concentrations) can activate. The ARM CCNKAPPA product (Kulkarni et al., 2018), which combines Kohler theory with CCN and SMPS measurements, is used to examine the critical diameter for activation and aerosol hygroscopicity (kappa, κ) between 23 October and 15 December. As expected, critical diameters for activations decrease with increasing supersaturation. The median critical diameters for 0.1 %, 0.2 %, and 0.4 % supersaturation are 195 to 210, 118 to 126, and 76 to 82 nm, respectively (Fig. S5b); however, the interquartile variations among periods A, B, and C are usually small (less than 7 %). Hygroscopicity is also relatively low and invariant during the campaign period (Fig. S5c), with median values ranging from 0.19 to 0.29. As indicated by the 95th percentiles, hydrophilic aerosols occurred most often during period B followed by periods A and C. Determining the relative importance of aerosol number, size, hygroscopicity, and mixing state factors on the temporal variations in CCN seen in Fig. 2f will require a closure study (e.g., Kulkarni et al., 2023).
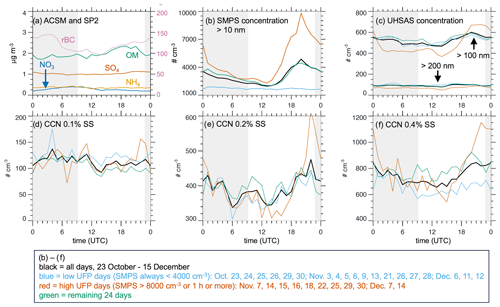
Figure 3Diurnal variability in (a) aerosol composition; (b) aerosol number concentration from SMPS; (c) aerosol number concentration from UHSAS; and CCN at (d) 0.1 %, (e) 0.2 %, and (f) 0.4 % supersaturation.
Figure 3a shows the average diurnal variations in aerosol composition, which reflect the impact of local processes such as boundary layer mixing and photochemistry. SO4 and NH4 have little diurnal variability during this 7-week period, suggesting multiday transport may be the dominant process influencing those concentrations over the AMF site. NO3 has peak concentrations around sunrise that are twice as high (0.4 µg m−3) as those during the late afternoon (0.2 µg m−3). This diurnal variability is likely due to temperature-dependent condensation/evaporation processes because the lowest temperatures occur at sunrise, and NO3 tends to evaporate during warmer temperatures. Peak OM concentrations usually occur during the afternoon, probably due to secondary organic aerosol (SOA) formation that depends on a wide range of photochemical processes. The net increase in surface OM suggests that photochemistry and/or entrainment of higher OM from the free troposphere more than compensates for the effects of growing boundary layer dilution. The peak rBC concentration at night is more difficult to explain since the local emissions of black carbon in the vicinity of the AMF site are likely to be very low. There is one rural road adjacent to the AMF site, the closest town with a population of ∼ 1000 is about 2 km to the northwest, towns with populations of ∼ 10 000 are located at lower elevations 10 km or more to the east, and larger cities with populations of 50 000 or more are more than 30 km away. Since rBC is chemically inert and can be treated as a passive tracer, the diurnal variability is due to the combination of horizontal transport and the effects of boundary layer mixing.
Ultrafine-particle number concentrations from the SMPS (Fig. 3b) show maximum concentrations occurring during the late afternoon at 20:00 UTC (17:00 LT). The diurnal variations in the UHSAS (Fig. 3c) also indicate modest concentration increases during the later afternoon for particle diameters greater than 100 nm; however, there is no diurnal variability for particle diameters greater than 200 nm. This indicates that most of the diurnal variability in aerosol number is driven by ultrafine and Aitken mode aerosols. Since NPF events vary during the sampling period (Fig. 2d), we divide the diurnal variability in aerosol number concentration into 19 d with concentrations always lower than 4000 cm−3 (low UFP), 11 d (1 during period A, 6 during period B, 4 during period C) with number concentrations greater than 8000 cm−3 for at least 1 h (high UFP), and the 24 remaining days that fall in between the low-UFP and high-UFP days. The high-UFP days exhibit the largest diurnal variability, the low-UFP days exhibit no diurnal variability, and the remaining 24 d have modest diurnal variability that is closer to the average over the entire period.
The diurnal variations in CCN shown in Fig. 3d–f are closely related to the diurnal variability in aerosol number. Both particles with diameters greater than 200 nm and CCN at 0.1 % supersaturation exhibited no diurnal variability (Fig. 3c and d). Smaller particles start to activate at 0.2 % supersaturation (Fig. 3e); therefore, CCN at this supersaturation exhibits a modest diurnal variation on average, with afternoon concentrations ∼ 28 % higher than earlier in the day. However, CCN concentrations during the late afternoon on high-UFP days are twice as high as those around 15:00 UTC (12:00 LT). Finally, particles smaller than 100 nm start to activate CCN at 0.4 % supersaturation so that CCN concentrations at this supersaturation (Fig. 3f) are about double those at 0.2 % supersaturation with a similar late afternoon peak. Note that for both 0.2 % and 0.4 % supersaturations, peak CCN concentrations on high-UFP days occur ∼ 2 h later in the day and closer to sunset than aerosol number concentrations. This suggests growth in the aerosol size distribution during the day influences CCN, consistent with the daily growth seen in Fig. 2d.
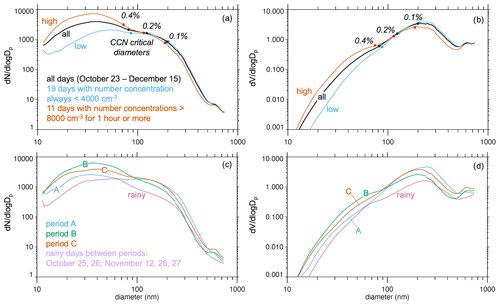
Figure 4Average size distributions at the ground site between 23 October and 15 December for days with high and low UFP concentration (a, b) and periods A, B, and C (c, d).
Figure 3c also shows that concentrations of particles with diameters greater than 200 nm on high-UFP days are ∼ 30 % lower than on low-UFP days. Figure 4a and b illustrate the average aerosol number and volume size distribution for 23 October to 15 December along with the averages for the low-UFP and high-UFP days. The differences in the number and volume distribution for particle diameters < 100 nm reflect the definition of these days and are consistent with the differences in CCN among the three supersaturations. Peak number concentrations for high-UFP days occur between 30–40 nm, while those on low-UFP days occur between 50–60 nm. In addition, high-UFP days have lower concentrations for particle diameters between 150–600 nm, likely reflecting the time between days to grow particles from UFP to accumulation mode size as indicated in Fig. 2d. Differences in the aerosol size distribution lead to differences in the critical particle diameter for CCN activation which are largest for 0.4 % supersaturation. The average aerosol number and volume size distributions for periods A, B, and C are shown in Fig. 4c and d. Periods A and B have the lowest and highest average UFP concentrations, respectively, since period B also contains the most high-UFP days. Conversely, periods A and B have the highest and lowest accumulation mode concentrations, respectively. The average aerosol size distribution for period C is between those for periods A and B and similar to the average size distribution between the whole 23 October and 15 December period in Fig. 4a and b. As expected, the rainy days at the AMF site have the lowest aerosol concentrations for all particle sizes.
Understanding the aerosol–cloud interactions in the region will depend on the intersection of cloud formation and growth with the growth rates of the aerosol size distribution and diurnal variability in CCN concentrations such as those shown in Figs. 2 and 3. Over the crest of the SDC, shallow convection typically forms by the late morning. Feng et al. (2022) show that deep convective cells preferentially form east of the crest between 15:00 and 19:00 UTC (12:00 and 16:00 LT), growing and intensifying until about 21:00 UTC (18:00 LT). This coincides with the increase in CCN concentrations at 0.2 % and 0.4 % supersaturations which is most pronounced on high-UFP days, although peak CCN concentrations occur after most of these deep convective events.
3.2 Sources of aerosols and trace gases
To explore the possible sources of aerosols over the AMF, we next compare aerosol composition with trace gases measured at the AMF site. Not surprisingly, O3 concentrations are relatively low (always < 45 ppb) at this remote site as shown in Fig. 5a. OM and O3 are very weakly correlated (r = 0.15) even though both depend on photochemical production. In contrast, the temporal variability in CO and rBC concentrations over the sampling period are moderately correlated (r = 0.59) as shown by the time series (Fig. 5b) and scatter plot of 30 min averages (Fig. 5c). The temporal variability of CO and rBC is similar because they are usually co-emitted from the same anthropogenic and/or biomass burning sources, although the ratio of CO to rBC mass emitted varies from source to source. Correlations between CO and rBC often approach 1 near the emission source; therefore, the lower correlations suggest mixing of many emission sources during long-range transport and/or cloud processing of rBC containing particles that leads to wet removal of rBC. OM is also moderately correlated with rBC (r = 0.56, Fig. 5d) for the same reason as CO and rBC; however, biogenic sources that do not emit BC can also contribute to a significant fraction of OM. Since the near-surface winds are usually from the north to northeast (Fig. S1a and b), it is possible that a large fraction of OM, rBC, and CO originates from Córdoba and/or smoke in the Amazon and La Plata basins (Fig. S6a) that is transported by the low-level jet to AMF site (e.g., Mulena et al., 2024). The scatter plots in Fig. 5c and d are color coded by northerly (330 to 30°), northeasterly (30 to 60°), and southerly (150 to 210°) wind directions and show that the highest BC concentrations occur during northerly winds.
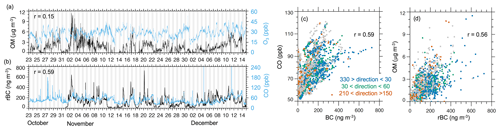
Figure 5Time series of (a) OM and O3 and (b) CO and rBC between 23 October and 15 December along with a scatter plot of (c) CO vs. rBC and (d) OM vs. rBC. Gray dots in panels (c) and (d) indicate wind directions that are not northerly, northeasterly, or southerly.
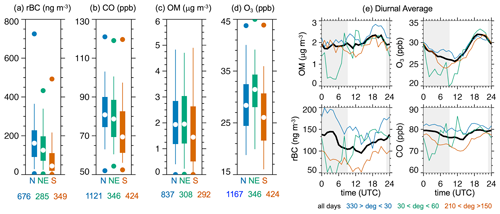
Figure 6Percentiles of (a) rBC, (b) CO, (c) OM, and (d) O3 as a function of wind direction along with (e) the diurnal variability of these quantities as a function of wind direction.
The effects of wind direction are further illustrated in Fig. 6. The magnitude of the interquartile range of rBC and CO is highest for northerly winds (Fig. 6a and b), followed closely by northeasterly winds. Southerly winds have the lowest concentrations and a substantially lower magnitude of the interquartile range. For OM, the interquartile ranges for northerly and northeasterly winds are similar, while southerly winds have somewhat lower concentrations (Fig. 6c). In contrast, northeasterly winds are associated with the highest magnitude in the interquartile range for O3, possibly due to transport of O3 produced by urban emissions from Córdoba (Fig. 6d). Despite the low correlation between OM and O3, they have a similar average diurnal variability (Fig. 6e). This suggests the low correlation is due primarily to multiday variability. Maximum OM and O3 concentrations during the afternoon are ∼ 25 % and ∼ 23 % higher than at sunrise on average, respectively. OM diurnal variability is also similar to the diurnal variability of CCN at 0.2 % supersaturation (Fig. 3e). The average diurnal variations for rBC and CO are similar, with the highest values at night and lowest values a few hours after sunrise. While the diurnal variability for northerly and southerly winds is similar, the concentrations are significantly higher for northerly winds. OM, O3, rBC, and CO concentrations are all lowest at night for northeasterly winds.
The CAM-Chem model is now used to illustrate transport pathways of biomass burning aerosols suggested by the relationships in Figs. 5 and 6. The largest CO emissions from smoke during the campaign period occur over the western Amazon basin as well as southern Brazil (Fig. S6a). Smaller fires occur across most regions outside of Patagonia and the Atacama Desert. In addition to primary carbonaceous particles, fires emit gas-phase aerosol precursors, including SO2 (Fig. S6b), with emissions rates that are 2 to 3 orders of magnitude lower than CO. These SO2 emissions ultimately become sulfate and affect CCN concentrations since sulfate is more hygroscopic than OM and rBC.
CAM-Chem predictions of CO and wind direction are evaluated with G-1 measurements as shown in Figs. S7 and S8, respectively. The vertical and temporal variations in the vicinity of the AMF site are in reasonable agreement with G-1 data, even though the coarse spatial grid does not resolve local measured variations. High CO concentrations are simulated during periods of northerly and northwesterly winds, suggesting that smoke from fires north of the AMF was transported over the site. Conversely, CO concentrations are lower when the winds were from the south. Fires and anthropogenic emissions of CO are both lower over the less populated areas of southern Argentina (Fig. S6a).
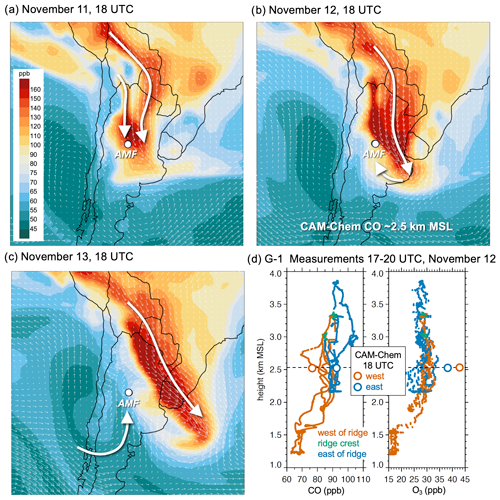
Figure 7CAM-Chem simulations of CO at ∼ 2.5 km a.m.s.l. at 18:00 UTC on (a) 11 November, (b) 12 November, and (c) 13 November along with (d) vertical profiles of CO measured by the G-1 aircraft between 17:00 and 20:00 UTC on 12 November.
Since CAM-Chem is in reasonable agreement with the G-1 CO measurements, Fig. 7 shows horizontal cross sections of CO at ∼ 2.5 km a.m.s.l. to illustrate transport during an episode between 11 and 13 November. Figure 7a shows the transport of CO from the western Amazon to the AMF site by a low-level jet. A trough pushes the low-level jet and plume of CO towards the east on 12 November (Fig. 7b), somewhat reducing the CO concentrations. While the winds have become southerly near the AMF site, CAM-Chem suggests local recirculation on the western side of the CO plume keeps the concentrations relatively high. As the trough continues to propagate to the east on November 13 (Fig. 7c), lower CO from the Pacific Ocean is transported over the AMF. Vertical profiles of CO from the G-1 on 12 November in Fig. 7d show that the highest concentrations occur east of the SDC. This is similar to the horizontal gradient in CO at 2.5 km a.m.s.l. produced by CAM-Chem that shows the CO plume east of the AMF site (Fig. 7b).
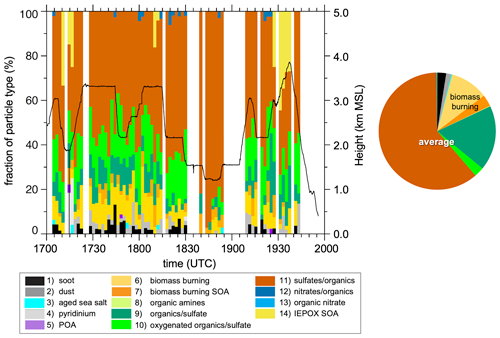
Figure 8Particle classes derived from the aircraft miniSPLAT mixing state measurements on 12 November.
Single-particle measurements from miniSPLAT from the 12 November G-1 flight shown in Fig. 8 reveal that ∼ 12 % of the particles originate from fires. The rest of the particles are composed of organics mixed with varying amounts of sulfate. A large portion of the organic material not identified as biomass burning aerosols is oxygenated organics, likely from biogenic sources in the Amazon that are transported by the same winds to the AMF site. As noted earlier, PM1 measured by the ground ACSM was relatively low between 11 and 12 November because of wet scavenging by rain and changing synoptic conditions. PM1 concentrations from the ACSM increased from 0.3 to 2.5 µg m−3 during the 3 h G-1 flight. This increase in aerosol mass occurs shortly after rain ends at the AMF site (Fig. 2a). Average PM1 concentrations during this 3 h period were 1.2 µg m−3, comprised of 54 % OM, 19 % SO4, 11 % NO3, and 16 % NH4. While bulk sulfate mass was 19 % on average, the miniSPLAT data shed light on the aerosol mixing state that suggests it was mixed with ∼ 80 % of the particles. The three particle classes that have the largest fraction of particles are made up of organics and sulfate at various ratios. This variability in aerosol mixing state could impact CCN concentrations and aerosol optical properties (e.g., Ching et al., 2017; Saliba et al., 2023).
The circulations depicted in Fig. 7 are repeated on many days during CACTI, indicating that the AMF site is periodically influenced by biomass burning. Thus, the sources of aerosols change from day to day.
3.3 Aircraft measurements
The evolving size distribution of ground-measured particles suggest that NPF events are followed by multiday growth to larger sizes. However, it is not clear whether these NPF events occur near the surface or aloft (either in the upper boundary layer or lower free troposphere) with mixing to the surface as has been observed at other locations (Chen et al., 2018; O'Donnell et al., 2023; Wang et al., 2023). Therefore, it is important to examine the vertical variations in aerosol properties that are available from the G-1 aircraft.
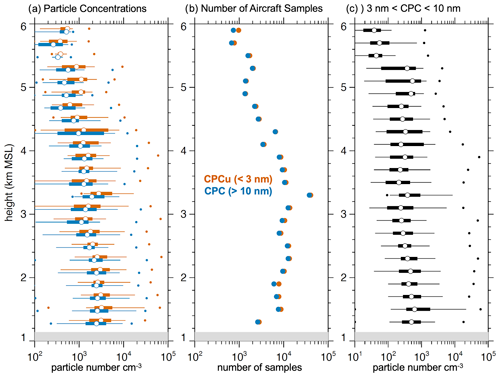
Figure 9(a) Particle number concentration percentiles as a function of height from all the G-1 measurements during CACTI along with (b) the number of samples at each altitude bin. Panel (c) is the same as panel (a), except for particle diameters between 3 and 10 nm.
The overall particle number concentrations observed by the two CPC instruments on flight days between 4 November and 8 December are shown in Fig. 9. Median CPC concentrations are highest within 1 km of the ground and then gradually become smaller with height, up to a factor of ∼ 2.2 smaller by 3 km a.m.s.l. (Fig. 9a). Median concentrations increase somewhat at 3.3 km a.m.s.l. and then continue to gradually decrease with height so that values at 6 km a.m.s.l. are almost an order of magnitude smaller than on the ground. Similar trends are produced for the interquartile and 5th to 95th percentile ranges; however, maximum concentrations that are between 10 000 and 100 000 cm−3 occur up to ∼ 4 km a.m.s.l. before decreasing with height. This suggests that high concentrations of UFP could occasionally be entrained into the growing daytime boundary layer and mixed to the surface. Note that number concentration percentiles at 3.3 km a.m.s.l. are slightly different than those at adjacent altitudes because the number of samples at that altitude are much larger than any other altitude (Fig. 9b). While there are large temporal variations in UFP concentrations within the G-1 flight periods as will be shown later, under-sampling is not likely to affect the overall vertical variations. In addition, the number of samples above 4.5 km a.m.s.l. are much lower than at other altitudes, so caution is warranted in interpreting the vertical variations at those altitudes. Particle concentrations smaller than 10 nm, obtained by computing the differences between the two CPC instruments, are similar up to 5.5 km a.m.s.l. (Fig. 9c).
Next, we examine the vertical profiles of particle concentrations in relation to the low- and high-UFP days as determined by the surface CPC measurements. The median particle number concentrations for the seven G-1 flights associated with high-UFP days were higher than the median among all the G-1 flights up to ∼ 3 km a.m.s.l. (Fig. S9). Conversely, the four G-1 flights on the low-UFP days were lower than the median among all the G-1 flights up to ∼ 3 km a.m.s.l. When the G-1 flights are divided into periods A, B, and C determined by the surface CPC measurements, period B has the highest median concentration near the surface, followed by periods C and A, as well as the rainy days. The results in Fig. S9 suggest that the boundary layer particle number concentrations are consistent with the broad variations seen on the ground. However, it is not surprising that the trends in the surface number concentrations are not representative of the temporal variability in the upper boundary layer and free troposphere.
A summary of the vertical profiles of CCN concentration for all the aircraft flights is shown in Fig. 10a. The median CCN at 0.2 % supersaturation between the surface and 3.5 km a.m.s.l. varies between 255 and 335 cm−3. At 0.5 % supersaturation, CCN concentrations are about twice as high as those at 0.2 % and vary between 546 and 724 cm−3. Above 3.5 km a.m.s.l., concentrations drop to below 100 cm−3 at 4 and 5.5 km a.m.s.l. for 0.2 % and 0.5 % supersaturations, respectively. The median cloud base height of 2.6 km a.m.s.l. and the interquartile range from KAZR-ARSCL computed during the G-1 flight periods (Fig. 10b) illustrate the relevant altitudes in which CCN can be entrained into clouds. The median CCN concentrations at the median cloud base height are similar to those at the surface, suggesting a well-mixed boundary layer. However, additional analyses are needed to determine whether surface CCN measurements are representative of the conditions at cloud base at the same time as when the G-1 flew directly over the AMF site.
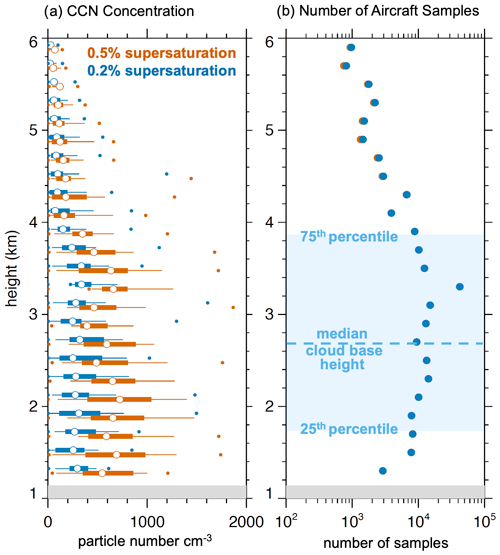
Figure 10(a) CCN concentration percentiles as a function of height from all the G-1 measurements during CACTI along with (b) the number of samples at each altitude bin. Light blue shading in panel (b) denotes the 25th to 75th percentile of cloud base height observed over the AMF site.
Median CCN concentrations for low-UFP days below 4.5 km a.m.s.l. are 20 %–25 % lower than the median for all the flights at 0.2 % supersaturation (Fig. S10a) and up to 70 % lower at 0.5 % supersaturation (Fig. S10b). Differences in the median CCN concentrations are also produced among periods A, B, and C; however, the vertical variations are noisy and suggest that additional flights are needed to account for the strong spatiotemporal variations in CCN aloft. While there are only two aircraft flights on rainy days, they indicate CCN concentrations are lower than all other flights within 0.3 and 1 km of the surface for 0.2 % and 0.5 % supersaturations, respectively. Above that altitude, median CCN concentrations are similar to the median of all the aircraft flights.
3.4 3 December case study
The percentiles in Figs. 9 and 10 reflect large spatial and temporal variations in CPC and CCN concentrations around the AMF site. In this section we analyze data from 3 December to demonstrate the spatiotemporal variability of aerosol number, size distribution, CCN, and trace gases for a particular flight on a partly cloudy day. This day was chosen because (1) the observed wind shears were similar to many other days, (2) surface PM1 concentrations were around 2 to 3 µg m−3 and not extremely low or high, and (3) relatively high ultrafine-particle number concentrations were observed at the surface and aloft. We note that the measurements on this day, however, are not representative of the entire G-1 mission since the aircraft also flew on clear-sky days and days where shallow cumulus transitioned to deeper convection.
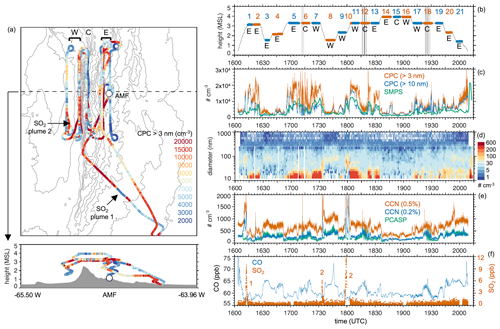
Figure 11(a) Spatial variations in particle number concentration (> 3 nm) for the 3 December aircraft flight along with temporal variations in (b) altitude, (c) particle number concentrations from CPC and SMPS instruments, (d) aerosol number distribution from BEASD, (e) CCN and particle number concentrations from PCASP, and (f) CO and SO2 concentrations. E, C, and W in panel (b) denote legs east of the crest, over the crest, and west of the crest as shown in panel (a).
Measurements during the afternoon on 3 December are shown in Fig. 11 to demonstrate the spatiotemporal variability of aerosol number, size distribution, CCN, and trace gases for a particular flight. The flight path color coded by CPC concentrations indicates that particle number varied by over an order of magnitude both horizontally and vertically during the 4 h flight period (Fig. 11a). By comparing the flight altitude (Fig. 11b), the time series of particle number concentration (Fig. 11c), and the aerosol size distribution (Fig. 11d), one can see that the highest UFP number concentrations with diameters < 30 nm occurred along ∼ 3.3 km a.m.s.l. flight legs (1, 2, 5–7, 11–13, 17–19). While clear skies were observed directly over the AMF site on this day, a line of orographic cumulus formed over the crest of the SDC. While there was widespread cumulus over the ridge, the time spent flying through clouds was less than 2.7 % total time at 3.3 km a.m.s.l. There were few ultrafine particles (diameters < 30 nm) for flight legs at lower (legs 3, 4, 8, 9, 20, 21) and higher (legs 14–16) altitudes. For the lower- and higher-altitude legs, the highest particle concentrations occurred at diameters of ∼ 80–100 and ∼ 30–60 nm, respectively. The spatiotemporal variability in CCN concentrations (Fig. 11e) is similar to the accumulation mode aerosols, with the highest CCN concentrations occurring for the lowest flight legs where the highest concentrations of larger particles occur. Outside of the Rio Cuarto airport, the highest CO concentrations occur along the lowest flight legs (Fig. 11f), and spatiotemporal variations in CO are similar to spatiotemporal variations in accumulation mode aerosols.
On this day, the G-1 encountered two SO2 plumes, with concentrations as high as 12 ppb denoted as plume no. 1 and no. 2 in Fig. 11a and f. The G-1 passed through plume no. 2 twice at different altitudes. The narrow plume width and high concentrations suggest that a local source is responsible for these two plumes. Local maxima in particle number and CCN concentrations occurred at the same location as the SO2 plume, suggesting that SO4 was also present. SO4 is more hydrophilic than other aerosol species, which may be why CCN concentrations are higher within the plume. Other than one other small plume of SO2 (∼ 3 ppb) on 3 December, no other SO2 plumes of this magnitude were observed by the G-1 during the campaign. The detection limit of the instrument is too high to describe variability of SO2 at the ppt level.
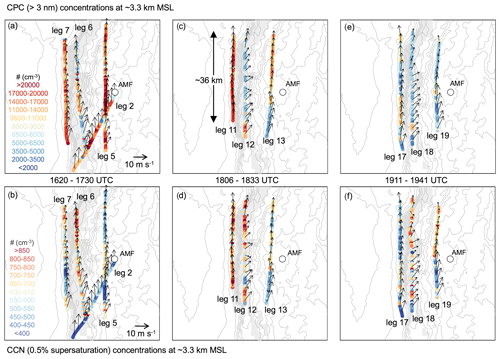
Figure 12Spatial variations in particle number concentrations > 3 nm (a, c, e) and CCN concentrations (b, d, f) for all the ∼ 3.3 km a.m.s.l. constant altitude transects on 3 December. Blue arrows depict wind speed and direction at 30 s intervals. Gray shading denotes cloud optical depth > 2 obtained from the GOES satellite ( km).
The advantage of the multiple constant altitude flight legs is that they can be compared to determine how aerosol properties aloft evolve in time. Figure 12 depicts the variations in total particle number and CCN concentrations for three periods: 16:20–17:30, 18:06–18:33, and 19:11–19:14 UTC, each with flight legs west of, over, and east of the crest of the SDC. During the first period between 16:20 and 17:30 UTC, particle concentrations exceed 15 000 cm−3 along most of the four flight legs (Fig. 12a). CCN concentrations vary by a factor of 3 (300 to 900 cm−3) over the region during the same period (Fig. 12b). The observed winds at this altitude are southerly and usually between 8 to 10 m s−1.
The second period between 18:06 and 18:33 UTC took place 36 to 133 min after the first period; therefore, aerosols sampled along legs 2, 5, 6, and 7 would be transported ∼ 17–80 km to the north by the time the aircraft conducted legs 11 to 13. Since the flight legs are ∼ 40 km long, aerosols measured during the second period are not likely to be the same as those sampled during the first period. During the second period, most of the particle concentrations remain above 15 000 cm−3 for the flight legs west and east of the SDC crest (Fig. 12c). However, lower concentrations between 2000 to 5000 cm−3 are being transported by southwesterly winds towards the AMF site along the southern third of leg 13. At this time, CCN concentrations are the highest for legs 11 and 12 and lower along leg 13 closer to the AMF site (Fig. 12d). Interestingly, winds over the crest along legs 6 and 12 switched from southerly to southwesterly, and particle number concentrations became a factor of 2 lower between period one and period two. As will be shown in more detail later, this is likely due to both the growing boundary layer and subsidence that transports lower particle number concentrations to this altitude. While winds in the lower boundary layer along legs 3 and 8 and from the AMF radiosonde at 18:00 UTC (not shown) are from the north to northeast, southwesterly winds observed near the top of the boundary layer top along leg 12 and from the 18:00 UTC AMF radiosonde at 3.3 km reflect the wind direction shear between the boundary layer and free troposphere.
The same wind pattern for period two persists through period three between 19:11–19:41 UTC. Strong gradients in total aerosol number and CCN concentrations are observed for all three flight legs during this period (Fig. 12e and f). CCN concentrations are the highest along leg 18 over the crest during period three. It is possible that recycling of aerosols through clouds changes the size and hygroscopicity of aerosol populations and thus CCN in this region, but that requires further analysis.
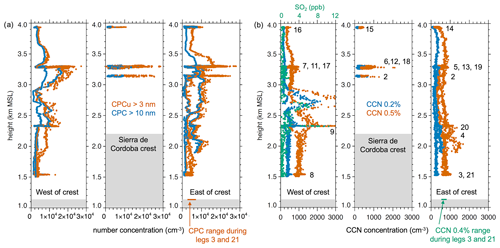
Figure 13Vertical variations in (a) particle number concentrations and (b) CCN concentrations on 3 December divided into transects that are west of, over, and east of the Sierras de Córdoba crest. Green dots in panel (b) denote SO2 concentrations west of the crest on 3 December. Gray shading denotes average terrain elevation, and the range of surface concentrations from the AMF site during the aircraft flight period is denoted on the bottom of the east of the crest panels.
While Fig. 12 illustrates the strong spatial and temporal variations in aerosol properties at 3.3 km a.m.s.l. on 3 December, Fig. 13 shows the vertical variations in number and CCN concentrations that are divided into flight paths west of, over, and east of the crest of the SDC. West of the crest, the highest number concentrations occur in two layers, one between 2.3 and 2.7 km a.m.s.l. and the other between 2.9 and 3.3 km a.m.s.l. (Fig. 13a). Note that the smallest particle (CPC > 3 nm) concentrations vary for a given altitude due to both spatial and temporal variability along the flight legs. The differences for the larger particles > 10 nm are much smaller at these altitudes; however, there are larger differences below 2.3 km a.m.s.l. Thus, the spatial variability for UFP and larger particles is not necessarily the same. East of the crest, a layer of high number concentrations occurred between 3.1 and 3.5 km a.m.s.l.; however, there is not a distinct second layer as seen west of the crest, and the two CPC instruments have similar spatiotemporal variability below 3.1 km a.m.s.l. While the spatial variability in aerosol number is similar west of, over, and east of the crest at 3.3 km a.m.s.l., the variability at 3.9 km a.m.s.l. west of the crest is lower than over and east of the crest. In general, Fig. 13 illustrates that aerosol number concentrations and variability can be different west and east of the SDC crest.
As shown in Fig. 13b, there are also differences in CCN concentrations between the west and east sides of the mountain range. There are two layers of high CCN concentrations that were sampled by the aircraft west of the crest: one between 2.6 and 2.9 km a.m.s.l. and the other between 2.3 and 2.5 km a.m.s.l. The highest SO2 concentrations also occur within these layers, suggesting that SO4 produced by SO2 plume no. 2 (Fig. 11a and f) leads to higher number concentrations and more hydrophilic aerosols. SO2 concentrations were low at all altitudes east of the crest. Note that the layer between 2.6 and 2.9 km a.m.s.l. occurs between the constant altitude transects; therefore, the spatial extent of this layer cannot be determined, and other layers could be missed between the constant altitude transects. In addition, CCN concentration profiles at both 0.2 % and 0.5 % supersaturations are somewhat lower east of the crest below 2.5 km a.m.s.l. and somewhat higher east of the crest above 3.5 km a.m.s.l. Thus, CCN also exhibits differences west and east of the crest.
To examine whether the aerosol size distribution is different across the SDC, the average number and volume distributions as functions of altitude and location relative to the crest are shown in Fig. 14. For the highest aircraft constant altitude flight legs at 3.9 km a.m.s.l., the number and volume distributions west of, over, and east of the crest are very similar. At 3.3 km a.m.s.l., particle number concentrations and volumes for diameters less than 40 nm are the lowest over the crest and highest west of the crest. But for particles greater than 80 nm in diameter, particle number concentrations are highest over the crest, and the size distributions for the flight legs west and east of the crest are similar. For flight legs below 2.65 km a.m.s.l., there are significant differences in the number and volume distributions west and east of the crest, and those differences vary with height. At the lowest flight legs at 1.5 km a.m.s.l. (∼ 0.4 km above ground east of the crest), the particle number concentrations and volumes for particle diameters greater than 80 nm are very similar to those measured at the ground AMF site. The differences for particles smaller than 80 nm indicate large spatial variability in UFP concentrations in the region. Differences between the AMF and aircraft size distributions at higher altitudes suggest that the ground measurements are not representative of conditions aloft. The spatiotemporal variability in size distribution likely contributes to variability in CCN concentrations, similar to the differences in critical diameters shown in Fig. 4.
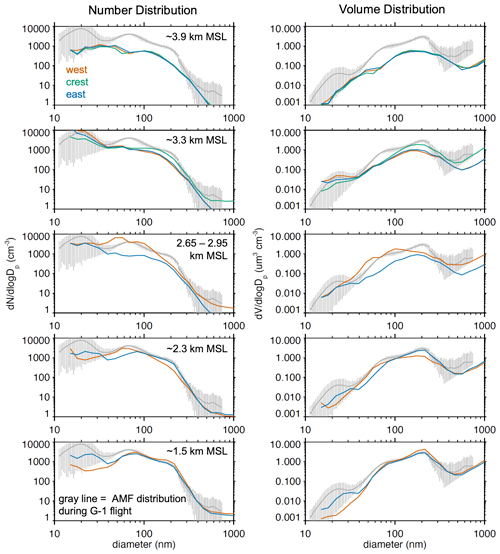
Figure 14Number and volume of aerosol distributions at five altitudes that are west of, over, and east of the Sierras de Córdoba crest on 3 December. Gray line denotes average ground measurements during the aircraft flight.
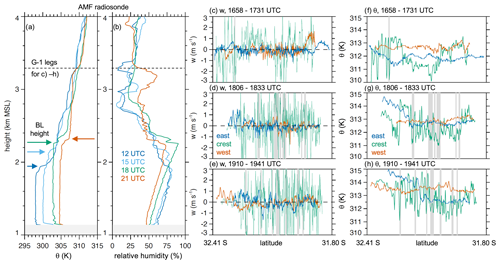
Figure 15Radiosonde profiles of (a) potential temperature and (b) relative humidity at the AMF site on 3 December along with the spatial variations in vertical velocity (c–e) and potential temperature (f–h) for G-1 flight legs at ∼ 3.3 km a.m.s.l. divided transects that are west of, over, and east of the Sierras de Córdoba crest. Gray shading in panels (c–h) denotes aircraft sampling within clouds.
To further understand the role of boundary layer growth over the crest, we next examine variations in potential temperature and vertical velocity among the 3.3 km a.m.s.l. aircraft flight legs in relation to the AMF radiosonde profiles as shown in Fig. 15. Between 12:00 and 21:00 UTC, the convective boundary layer at the AMF site grows from 1.9 to 2.3 km a.m.s.l. (Fig. 15a, b). At 21:00 UTC, the inflection of potential temperature at ∼ 3.3 km a.m.s.l. and the higher relative humidity and southwesterly winds just below that level reflect the advection of the boundary layer air from the higher terrain towards the AMF site that produces a layer of constant potential temperature between 2.3 and 3.2 km a.m.s.l. The increase in potential temperature between 15:00 to 18:00 UTC just above 3.3 km a.m.s.l. is likely due to subsidence as southwesterly air is transported across the leeward side of the crest.
For the flight legs between 16:58 and 17:31 UTC at 3.3 km a.m.s.l., variability in vertical velocity (Fig. 15c) and potential temperature (Fig. 15f) is small in the free troposphere west and east of the crest. During this time period, the aircraft flew just above most of the growing clouds along the ridge (leg 6). Larger variations in both quantities are measured over the crest, reflecting turbulent motions generated by clouds just above the growing boundary layer and terrain variability. By the second period between 18:06 and 18:33 UTC, spatiotemporal variations in vertical velocity (Fig. 15d) and potential temperature (Fig. 15g) increase over the crest as the boundary layer grew and increased the intensity of turbulent eddies. Some of the anomalies with lower potential temperatures within clouds are due to updrafts that reduce the potential temperatures near the boundary layer top. High-frequency variability in vertical velocity and potential temperature remains low west and east of the crest, suggesting the convective boundary layer does not reach 3.3 a.m.s.l. for those transects over lower terrain elevations. The variability during the third period between 19:10–19:41 UTC (Fig. 15e and h) is similar to the previous period. While there are few high-frequency variations in potential temperature west and east of the crest, there are larger-scale variations likely due to larger-scale horizontal advection. For example, the southerly winds along the southern third of leg 12 (Fig. 12c) between 18:06 and 18:33 UTC coincide with both higher potential temperature and lower particle number concentrations, suggesting a different air mass. The higher potential temperatures progress northward by the third period between 19:20–19:41 UTC.
CCN concentrations shown in Fig. 16 further illustrate spatial variabilities within and among the legs west of, over, and east of the mountain range crest. During the first period between 16:58–17:31 UTC, CCN concentrations at 0.2 % supersaturation are higher over the crest than those along the flight legs west and east of the crest (Fig. 16a). There are also larger spatial fluctuations along portions over the crest that might be tied to the larger vertical velocity variations (Fig. 15c). High-frequency fluctuations in CCN increase as the boundary layer grows over the crest and envelopes the flight leg over the crest between 18:06–18:33 UTC (Fig. 16b). CCN concentrations at 0.2 % supersaturation are still the highest, with the largest amount of spatial variability during the third period between 19:10–19:41 UTC (Fig. 16c). In the free troposphere, the overall CCN concentrations west of the crest remain stable, while there are large-scale variations east of the crest. CCN at 0.5 % supersaturation still exhibits differences between the legs west of, over, and east of the crest; however, CCN concentrations are not consistently higher or lower across the crest during the three time periods (Fig. 16d–f). CCN concentrations are also lower along the southern third of legs 13 and 19 east of the crest, consistent with the lower particle number concentrations transported northward along legs 13 and 19 during the second and third time periods.

Figure 16Spatial variations in 0.2 % CCN (a–c) and 0.5 % CCN (d–f) for G-1 flight legs at ∼ 3.3 km a.m.s.l. that are west of, over, and east of the Sierras de Córdoba crest. Gray shading denotes aircraft sampling within clouds.
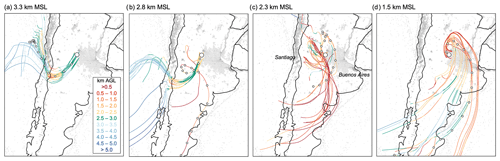
Figure 17Back trajectories originating at (a) 3.3 km a.m.s.l., (b) 2.8 km a.m.s.l., (c) 2.3 km a.m.s.l., and (d) 1.5 km a.m.s.l. over the AMF site on 3 December during the G-1 flight between 16:00 and 20:00 UTC. Dots denote 6 h periods along one trajectory for each height.
Figures 1–16 demonstrate that local meteorological processes affect the variability in observed aerosol properties. Back trajectories are used in Fig. 17 to illustrate long-range transport pathways and possible sources of aerosols transported over the AMF site on 3 December. The HYSPLIT model (Rolph et al., 2017; Stein et al., 2015) is used to compute 4 d back trajectories originating over the AMF site at 1.5, 2.3, 2.8, and 3.3 km a.m.s.l. levels and at hourly intervals between 16:00 and 20:00 UTC during the G-1 aircraft flight period. Back trajectories are also computed at the corners of a 1° wide box around the AMF site at the same altitudes and times. The trajectories are driven by winds from the National Centers for Environmental Prediction's Global Data Assimilation System at 0.5° grid spacing.
Back trajectories arriving over the AMF site at 3.3 km a.m.s.l. (Fig. 17a) suggest that air in the lower free troposphere over the Pacific Ocean is transported by northerly to northwesterly winds toward the coast of Chile. As the trajectories pass over the Andes, they become closer to the terrain (within 1.5 km a.m.s.l.). It is possible that anthropogenic aerosols and aerosol precursors emitted over Chile and transported by upslope flows could be mixed with this air mass over the Andes. Westerly winds then transport these aerosols and precursors over the Andes into Argentina, and they are subsequently transported by southwesterly winds towards the AMF site. A similar transport pattern is produced for back trajectories originating at 2.8 km a.m.s.l. (Fig. 17b), except that the air passes over Chile further to the south and farther from the largest anthropogenic emissions. Some trajectories suggest that low-emission regions over Argentina could be lifted to that altitude. In contrast, back trajectories starting at 2.3 km a.m.s.l. near the top of the growing boundary layer are more complex (Fig. 17c). Some trajectories passing over southern Argentina, where the anthropogenic emission rates are relatively low, are transported over the eastern slopes of the Andes, where they are lofted to higher altitudes and then transported by westerly winds to the AMF site. Other trajectories exhibit a counterclockwise circulation so that air from southern Argentina is transported east and north of the AMF site, and northerly winds finally transport the air masses to over the AMF site. Finally, for trajectories arriving near the surface at 1.5 km a.m.s.l. (Fig. 17d), almost all the back trajectories exhibit the counterclockwise circulation, suggesting that the lower atmosphere over the AMF is a mixture of cleaner air passing over southern Argentina and higher concentrations of aerosols originating from more populated regions along the Paraná River between Sante Fe and Buenos Aires.
Even though back trajectories do not pass over the Amazon, miniSPLAT measurements on this day suggest that ∼ 14 % of the particles originate from biomass burning at all altitudes (Fig. S11). This is nearly the same percentage as on 12 November, when smoke was transported from more distant sources in the Amazon. However, the combination of back trajectories, wind directions (Fig. S8), and low CO concentrations (Fig. 11f) suggests that biomass burning from the Amazon did not contribute significantly to aerosols on this day. Fire emission inventories indicate smaller fires occurred over eastern Argentina and along the coast of Chile 3 d prior to 3 December (not shown); therefore, the source of biomass burning aerosols over the AMF site was different on 12 November and 3 December. These results are consistent with Schill et al. (2020), who found biomass burning aerosols were present nearly everywhere in the remote troposphere, often comprising ∼ 25 % of the accumulation mode aerosol number. The transport time also suggests that primary and secondary aerosols originating from the populated regions over eastern Argentina would be aged by up to 1.5 d. In addition, the types of organic mixtures for these days were very different. On 3 December, the fraction of particle class 10 containing oxygenated organics and sulfate mixtures was far less, particle class 11 containing the highest ratio of sulfate to organics was higher on 3 December, and particle class 14 containing IEPOX SOA was very small relative to 12 November. While many of the back trajectories passed over southern Argentina, dust contributed to less than 1 % of the particles smaller than 1 µm in diameter on 3 December.
Figure 17 illustrates the impact of vertical wind shear and mountain venting processes on the transport of aerosols and their precursors. The back trajectories are consistent with AMF radiosonde wind directions that are northerly in the boundary layer, westerly near the boundary layer top, and southwesterly in the lower free troposphere (Fig. S8). Mountain venting of aerosols and precursors into the lower free troposphere results from a combination of upslope flows and detrainment from the boundary layer over the highest terrain (De Wekker and Kossmann, 2015) that subsequently produces layers in the lower free troposphere transported far downwind. Thus, the combination of local lofting of aerosols over the Sierras de Córdoba range and upwind lofting over the Andes mountains likely contributes to variability in aerosol properties. Mountain venting processes and their impact on pollutant transport have been observed in many regions of the world, including the Alps (e.g., Henne et al., 2005; Kossmann et al., 1999; Nyeki et al., 2000), central Mexico (Fast and Zhong, 1998), California (e.g., Fast et al., 2014; Lu and Turco, 1994; Langford et al., 2010), and the Andes (e.g., Lopere et al., 2021). It is important to note that the 0.5° grid spacing in GDAS is not likely to resolve local terrain-driven circulations and around the SDC and the Andes, which introduces uncertainties in these trajectories.
In situ measurements of aerosol properties are needed to evaluate and improve air quality, chemical transport, and climate model predictions and to better understand complex aerosol–cloud interaction processes. While surface monitoring networks and aircraft field campaigns have collected aerosol measurements in the Northern Hemisphere over the past several decades, few field campaigns with extensive aerosol measurements have been conducted over subtropical and midlatitude continental areas in the Southern Hemisphere. This study analyzes a wide range of surface and aircraft measurements collected over a 7-week period during the recent CACTI field campaign in central Argentina.
CACTI surface observations show large multiday variations in aerosol number, mass, composition, and size distribution. On average, PM1 aerosol mass obtained from the ACSM instrument (3.7 µg m−3) was comprised of 53 % OM, 29 % SO4, 8 % NO3, and 8 % NH4; however, the fraction of SO4 was significantly larger when PM1 mass was low (< 1 µg m−3). While multiday trends in PM1 and AOD are qualitatively similar and suggest most aerosol mass occurs in the boundary layer, on some days these trends differ over several hours, indicating that aerosol layers in the free troposphere can significantly contribute to column extinction. As expected, days with the highest precipitation (25–26 October, 11–12 November, and 26–27 November) have the lowest PM1 mass concentrations due to wet removal and/or changing regional air masses. These rainy days divide the campaign into three periods: period A between 27 October and 10 November with the highest aerosol mass and lowest aerosol number concentrations, period B between 13 and 25 November with the lowest aerosol mass and highest aerosol number concentrations, and period C after 28 November with mass concentrations similar to period A but with aerosol number concentrations between those from periods A and B. The high ultrafine-particle concentrations during period B, and to a lesser extent period C, suggest that new particle formation occurred over or upwind of the AMF site. Average size distributions are also different among these three periods. All these measurements suggest that changing mesoscale to synoptic-scale meteorology alters transport patterns as well as local aerosol formation and growth processes.
Diurnal variation in aerosol composition, number, and CCN can be attributed to local meteorological and chemical processes. While SO4 and NH4 showed no diurnal variability on average, peak OM, NO3, and rBC concentrations occurred during the midafternoon, around sunrise, and at night, respectively. The daily peak in OM is likely due to photochemistry associated with SOA, and the daily peak of NO3 at sunrise when the temperatures are the coldest likely inhibits partitioning of NO3 to the gas phase. Particles greater than 200 nm in diameter exhibit no diurnal variations, which is consistent with the weak diurnal variability in PM1 mass. Conversely, smaller particles exhibit diurnal variations with a peak at ∼ 20:00 UTC (17:00 LT), and the strongest diurnal variations are for the smallest particles less than 10 nm in diameter. No diurnal variability in CCN at 0.1 % supersaturation was observed, consistent with little diurnal variability in accumulation mode aerosols. CCN at higher supersaturations have diurnal variations with peak concentrations at 22:00 UTC, reflecting the growth of aerosols during the afternoon.
Potential sources of aerosols can be determined by correlating aerosol composition with trace gases and wind directions. Since rBC and CO are often co-emitted, observed rBC and CO exhibit similar diurnal variability and are temporally correlated (r = 0.59). The concentrations of both quantities are largest during northerly winds, followed by northeasterly, with the lowest concentrations during southerly winds. OM concentrations are largest for northerly and northeasterly winds, with somewhat lower concentrations during southerly winds. O3 is the largest during northeasterly winds and therefore may originate from anthropogenic emissions in Córdoba. The CAM-Chem global chemical transport model and single-particle instrument data show that biomass burning aerosols from the Amazon are frequently transported by the South American low-level jet to the AMF region. Single-particle measurements show that ∼ 12 % of particles during one of the transport events are from biomass burning particles. Aged biogenic OM may also be transported by the same winds. While SO4 is 29 % of the PM1 mass on average on the ground, it is important to note that single-particle measurements reveal it is mixed with organics at various ratios, illustrating a more complex mixing state than from bulk measurements.
While the impact of the South American low-level jet on convection is well known (e.g., Sasaki et al., 2024), its impact on trace gas and aerosol transport has not been studied extensively (Martins et al., 2018). Since the South American low-level jet occurs frequently, it may be an important mechanism that transports biogenic, biomass burning, and anthropogenic aerosols into Argentina. The CACTI data set provides a means to estimate that impact; however, additional careful analyses are needed since the SDC range may perturb the low-level jet, vertical wind shears, and coupling with the surface. These local effects likely vary from day to day. A more thorough analysis of transport by the low-level jet is left to future studies.
The aircraft measurements show that the largest total aerosol number concentrations usually occur at the surface and decrease by an order of magnitude by 5 km a.m.s.l. on average. Statistics of all the flights illustrate that aerosol number concentrations vary significantly (by an order of magnitude or more for 5th to 95th percentiles) both temporally (multiday and within 4 h flight periods) and horizontally within 50 km of the AMF site at all altitudes. In contrast, average CCN concentrations remain relatively constant from the surface up to 3.5 km a.m.s.l. and then gradually decrease to small values by 5 km a.m.s.l. The percentiles of CCN also show temporal and horizontal variability (factor of ∼ 2 for 5th to 95th percentiles), but that variability becomes small above 5 km a.m.s.l.
Since the aircraft data reveal large variations in aerosol properties aloft on many days, we focus on the 3 December measurements as a case study. On this day, repeated constant altitude flights reveal large spatial gradients in aerosol number and CCN concentrations that change from hour to hour. Two small plumes of SO2 with high aerosol number concentrations were observed; however, it is possible that the aircraft could easily miss other small, transient plumes even with repeated patterns at multiple altitudes. While the aerosol size distribution from the lowest flight transects east of the mountain crest and within the boundary layer was similar to the ground measurements, it changed with height and was also different west of, over, and east of the crest of the Sierras de Córdoba range. Some flight tracks over the mountain crest occurred just as the growing boundary layer intersected those altitudes, illustrating the effects of aerosol entrainment across the top of the boundary layer. It is possible that cloud processing also affects the size, composition, and hygroscopicity of aerosols over the mountain crest, but further analysis is needed to examine this process.
Strong vertical wind shear at the AMF site results in transport pathways of aerosol sources that vary with height. Back trajectories on 3 December indicate that aerosols within the boundary layer likely originated from the more populous regions of eastern Argentina with anthropogenic and biomass burning contributions. At higher altitudes, mountain venting processes could produce lofting of aerosols and aerosol precursors emitted from Chile or western Argentina on either side of the Andes that are subsequently transported by westerly winds over the AMF site. A similar, smaller-scale process may operate along the Sierras de Córdoba crest since the aircraft measurements indicate aerosols entrained into the free troposphere are transported eastward over the AMF site.
In addition to quantifying aerosol properties in a data-sparse region, the aerosol property measurements presented in this study will be valuable to evaluate predictions over the midlatitudes of South America and improve parameterized aerosol processes in local, regional, and global models. For aerosol–cloud interaction studies, the measurements clearly show that accounting for the co-variabilities of aerosol properties and convective cloud populations over the Sierras de Córdoba range will be critical. Knowing aerosol properties just below and surrounding clouds is important because aerosols are entrained into the base of clouds by convective updrafts. In addition to cloud base entrainment, aerosols with different properties at higher altitudes are also entrained into the sides of clouds as they grow vertically. As clouds evaporate, the size and composition of aerosol particles that were within cloud droplets can be different than aerosol populations surrounding clouds because of cloud chemistry and coalescence of cloud droplets. Thus, the properties of aerosol populations near the top of the boundary layer and around clouds will change in time as aerosols are cycled through clouds multiple times over several hours. Given the large observed variations in both aerosol and cloud properties and the complexity of their interactions, it will be challenging to develop a robust statistical signal of aerosol–cloud interactions from all the measurements. Thus, studying impacts of aerosols on cloud properties and impacts of clouds on aerosol properties on a case-by-case basis will provide critical insights.
In addition to citations given in the text, all CACTI data are available through links provided at https://www.arm.gov/research/campaigns/amf2018cacti (Varble, 2019). Citations for individual data sets are provided throughout the text. This includes https://doi.org/10.5439/1488849 (Burk and Ermold, 2018), https://doi.org/10.5439/1488850 (Burk et al., 2018a), https://doi.org/10.5439/1488851 (Burk et al., 2018b), https://doi.org/10.5439/1438488 (Cromwell et al., 2018), https://doi.org/10.5439/1228769 (Johnson et al., 2018), https://doi.org/10.5439/1595321 (Keeler et al., 2018), https://doi.org/10.5439/1356805 (Koontz et al., 2018a), https://doi.org/10.5439/1228061 (Koontz et al., 2018b), https://doi.org/10.5439/1352536 (Koontz et al., 2018c), https://doi.org/10.5439/1323892 (Koontz et al., 2018d), https://doi.org/10.5439/1323893 (Koontz et al., 2018e), https://doi.org/10.5439/1250819 (Koontz et al., 2018f), https://doi.org/10.5439/1349237 (Koontz et al., 2018g), https://doi.org/10.5439/1373109 (Koontz et al., 2018h), https://doi.org/10.5439/1349242 (Koontz et al., 2018i), https://doi.org/10.5439/1349243 (Koontz et al., 2018j), https://doi.org/10.5439/1476898 (Kuang et al., 2018), https://doi.org/10.5439/1729907 (Kulkarni et al., 2018), https://doi.org/10.5439/1786358 (Kyrouac et al., 2018), https://doi.org/10.5439/1245509 (Marinovici and Tomlinson, 2018), https://doi.org/10.5439/1961525 (Mei and Pekour, 2018), https://doi.org/10.5439/1417472 (Mei et al., 2018), https://doi.org/10.5439/1905541 (Pekour and Ermold, 2023), https://doi.org/10.5439/1346692 (Springston et al., 2018), https://doi.org/10.5439/1524780 (Tomlinson, 2018), https://doi.org/10.5439/1409033 (Uin et al., 2018), and https://doi.org/10.5439/1558768 (Zawadowicz et al., 2018).
The supplement related to this article is available online at: https://doi.org/10.5194/acp-24-13477-2024-supplement.
JDF performed the data analyses and wrote the manuscript. ACV was the PI of the CACTI campaign, assisted in the design and interpretation of the data analyses, and contributed to the manuscript. FM, MP, JT, AZ, AJS III, and MZ were instrument mentors during CACTI and provided comments on the manuscript where they are first mentioned. LE is the creator of the CAM-Chem simulations and provided comments on the manuscript.
The contact author has declared that none of the authors has any competing interests.
Publisher's note: Copernicus Publications remains neutral with regard to jurisdictional claims made in the text, published maps, institutional affiliations, or any other geographical representation in this paper. While Copernicus Publications makes every effort to include appropriate place names, the final responsibility lies with the authors.
Ground and aircraft measurements used in this study were provided by the Atmospheric Radiation Measurement (ARM) Climate Research Facility – a DOE user facility sponsored by BER. The Pacific Northwest National Laboratory (PNNL) is operated for DOE by Battelle Memorial Institute under contract DE-AC06-76RL01830. The authors gratefully acknowledge the NOAA Air Resources Laboratory (ARL) for the provision of the HYSPLIT transport and dispersion model as well as the READY website (https://www.ready.noaa.gov, last access: 26 November 2024) used in this publication. CAM-Chem simulations were supported by the NSF National Center for Atmospheric Research, which is a major facility sponsored by the U.S. National Science Foundation under cooperative agreement no. 1852977.
This research was supported by the U.S. Department of Energy (DOE) Office of Science Office of Biological and Environmental Research (BER) through the Atmospheric System Research (ASR) program under project KP1701010/57131.
This paper was edited by Eleanor Browne and reviewed by two anonymous referees.
Albrecht, B. A.: Aerosols, cloud microphysics, and fractional cloudiness, Science, 245, 1227–1230, https://doi.org/10.1126/science.245.4923.1227, 1989.
Barrett, P. A., Abel, S. J., Coe, H., Crawford, I., Dobracki, A., Haywood, J., Howell, S., Jones, A., Langridge, J., McFarquhar, G. M., Nott, G. J., Price, H., Redemann, J., Shinozuka, Y., Szpek, K., Taylor, J. W., Wood, R., Wu, H., Zuidema, P., Bauguitte, S., Bennett, R., Bower, K., Chen, H., Cochrane, S., Cotterell, M., Davies, N., Delene, D., Flynn, C., Freedman, A., Freitag, S., Gupta, S., Noone, D., Onasch, T. B., Podolske, J., Poellot, M. R., Schmidt, S., Springston, S., Sedlacek III, A. J., Trembath, J., Vance, A., Zawadowicz, M. A., and Zhang, J.: Intercomparison of airborne and surface-based measurements during the CLARIFY, ORACLES and LASIC field experiments, Atmos. Meas. Tech., 15, 6329–6371, https://doi.org/10.5194/amt-15-6329-2022, 2022.
Borque, P. A., Varble, A. C., and Hardin, J.: Peak rain rate sensitivity to observed cloud condensation nuclei and turbulence in continental warm shallow clouds during CACTI, J. Geophys. Res., 127, E2022JD036864, https://doi.org/10.1029/2022JD036864, 2022.
Brock, C. A., Williamson, C., Kupc, A., Froyd, K. D., Erdesz, F., Wagner, N., Richardson, M., Schwarz, J. P., Gao, R.-S., Katich, J. M., Campuzano-Jost, P., Nault, B. A., Schroder, J. C., Jimenez, J. L., Weinzierl, B., Dollner, M., Bui, T., and Murphy, D. M.: Aerosol size distributions during the Atmospheric Tomography Mission (ATom): methods, uncertainties, and data products, Atmos. Meas. Tech., 12, 3081–3099, https://doi.org/10.5194/amt-12-3081-2019, 2019.
Burk, K. and Ermold, B.: Carbon Monoxide – Airborne (AAFCO), Atmospheric Radiation Measurement (ARM) User Facility [data set], https://doi.org/10.5439/1488849, 2018.
Burk, K., Ermold, B., and Trojanowski, R.: Sulfur Dioxide Monitor aboard Aircraft (AAFSO2), Atmospheric Radiation Measurement (ARM) User Facility [data set], https://doi.org/10.5439/1488850, 2018a.
Burk, K., Ermold, B., and Trojanowski, R.: Ozone Monitor aboard Aircraft (AAFO3), Atmospheric Radiation Measurement (ARM) User Facility [data set], https://doi.org/10.5439/1488851, 2018b.
Carslaw, K. S., Lee, L. A., Regayre, L. A., and Johnson, J. S.: Climate models are uncertain, but we can do something about it, Eos, 99, https://doi.org/10.1029/2018EO093757, 2018.
Chen, H., Hodshire, A. L., Ortega, J., Greenberg, J., McMurry, P. H., Carlton, A. G., Pierce, J. R., Hanson, D. R., and Smith, J. N.: Vertically resolved concentration and liquid water content of atmospheric nanoparticles at the US DOE Southern Great Plains site, Atmos. Chem. Phys., 18, 311–326, https://doi.org/10.5194/acp-18-311-2018, 2018.
Ching, J., Fast, J., West, M., and Riemer, N.: Metrics to quantify the importance of mixing state for CCN activity, Atmos. Chem. Phys., 17, 7445–7458, https://doi.org/10.5194/acp-17-7445-2017, 2017.
Cromwell, E., Tomlinson, J., and Pekour, M.: Cloud and Aerosol Spectrometer aboard aircraft (AAFCAS), Atmospheric Radiation Measurement (ARM) User Facility [data set], https://doi.org/10.5439/1438488, 2018.
Danabasoglu, G., Lamarque, J.-F., Bachmeister, J., Bailey, D. A., DuVivier, A. K., Edwards, J., Emmons, L. K., Fasullo, J., Garcia, R., Gettelman, A., Hannay, C., Holland, M. M., Large, W. G., Lawrence, D. M., Lenaerts, J. T. M., Lindsay, K., Lipscomb, W. H., Mills, M. J., Neale, R., Oleson, K. W., Otto-Bliesner, B., Phillips, A. S., Sacks, W., Tilmes, S., van Kampenhout, L., Vertenstein, M., Bertini, A., Dennis, J., Deser, C., Fischer, C., Fox-Kember, B., Kay, J. E., Kinnison, D., Kushner, P. J., Long, M. C., Mickelson, S., Moore, J. K., Nienhouse, E., Polvani, L., Rasch, P. J., and Strand, W. G.: The Community Earth System Model version 2 (CESM2), J. Adv. Model. Earth Sys., 12, e2019MS001916, https://doi.org/10.1029/2019MS001916, 2020.
DeCarlo, P. F., Kimmel, J. R., Trimborn, A., Northway, M. J., Jayne, J. T., Aiken, A. C., Gonin, M., Fuhrer, K., Horvath, T., Docherty, K. S., Worsnop, D. R., and Jimenez, J. L.: Field-deployable, high-resolution, time-of-flight aerosol mass spectrometer, Anal. Chem., 78, 8281–8289, https://doi.org/10.1021/ac061249n, 2006.
De Wekker, S. J. F. and Kossman, M.: Convective boundary layer heights over mountainous terrain – A review of concepts, Front. Earth Sci., 3, 77, https://doi.org/10.3389/feart.2015.00077, 2015.
Emmons, L. K., Schwantes, R. H.,Orlando, J. J., Tyndall, G., Kinnison, D., Lamarque, J.-F., Daniel Marsh, D., Mills, M. J., Tilmes, S., Bardeen, C., Buchholz, R. R., Conley, A., Gettelman, A., Garcia, R., Simpson, I., Blake, D. R., Meinardi, S., and Pétron, G.: The chemistry mechanism in the Community Earth System Model version 2 (CESM2), J. Adv. Model. Earth Sys., 12, e2019MS001882, https://doi.org/10.1029/2019MS001882, 2020.
Fan, J., Wang, Y., Rosenfeld, D., and Liu, Y.: Review of aerosol–cloud interactions: Mechanisms, significance, and challenges, J. Atmos. Sci., 73, 4221–4252, https://doi.org/10.1175/JAS-D-16-0037.1, 2016.
Fast, J. D. and Zhong, S. Y.: Meteorological factors associated with inhomogeneous ozone concentrations within the Mexico City basin, J. Geophys. Res., 103, 18927–18946, https://doi.org/10.1029/98JD01725, 1998.
Fast, J. D., Allan, J., Bahreini, R., Craven, J., Emmons, L., Ferrare, R., Hayes, P. L., Hodzic, A., Holloway, J., Hostetler, C., Jimenez, J. L., Jonsson, H., Liu, S., Liu, Y., Metcalf, A., Middlebrook, A., Nowak, J., Pekour, M., Perring, A., Russell, L., Sedlacek, A., Seinfeld, J., Setyan, A., Shilling, J., Shrivastava, M., Springston, S., Song, C., Subramanian, R., Taylor, J. W., Vinoj, V., Yang, Q., Zaveri, R. A., and Zhang, Q.: Modeling regional aerosol and aerosol precursor variability over California and its sensitivity to emissions and long-range transport during the 2010 CalNex and CARES campaigns, Atmos. Chem. Phys., 14, 10013–10060, https://doi.org/10.5194/acp-14-10013-2014, 2014.
Fast, J. D., Bell, D. M., Kulkarni, G., Liu, J., Mei, F., Saliba, G., Shilling, J. E., Suski, K., Tomlinson, J., Wang, J., Zaveri, R., and Zelenyuk, A.: Using aircraft measurements to characterize subgrid-scale variability of aerosol properties near the Atmospheric Radiation Measurement Southern Great Plains site, Atmos. Chem. Phys., 22, 11217–11238, https://doi.org/10.5194/acp-22-11217-2022, 2022.
Feingold, G., Ghate, V. P., Russell, L. M., Blossey, P., Cantrell, W., Christensen, M. W., Diamond, M. S., Gettelman, A., Glassmeier, F., Gryspeerdt, E., Haywood, J., Hoffmann, F., Kaul, C. M., Lebsock, M., McComiskey, A. C., McCoy, D. T., Ming, Y., Mülmenstädt, J., Possner, A., Prabhakaran, P., Quinn, P. K., Schmidt, K. S., Shaw, R. A., Singer, C. E., Sorooshian, A., Toll, V., Wan, J. S., Wood, R., Yang, F., Zhang, J., and Zheng, X.: Physical science research needed to evaluate the viability and risks of marine cloud brightening, Sci. Adv., 10, eadi8594, https://doi.org/10.1126/sciadv.adi8594, 2024.
Feng, Z., Varble, A. C., Hardin, J. C., Marquis, J. N., Hunzinger, A., Zhang, Z., and Thieman, M.: Deep convection initiation, growth and environments in the complex terrain of central Argentina during CACTI, Mon. Weather Rev., 150, 1135–1155, https://doi.org/10.1175/MWR-D-21-0237.1, 2022.
Feng, Z., Hardin, J., Barnes, H. C., Li, J., Leung, L. R., Varble, A., and Zhang, Z.: PyFLEXTRKR: a flexible feature tracking Python software for convective cloud analysis, Geosci. Model Dev., 16, 2753–2776, https://doi.org/10.5194/gmd-16-2753-2023, 2023.
Henne, S., Dommen, J., Neininger, B., Reimann, S., Staehelin, J., and Prévôt, A. S. H.: Influence of mountain venting in the Alps on the ozone chemistry of the lower free troposphere and the European pollution export, J. Geophys. Res., 110, D22307, https://doi.org/10.1029/2005JD005936, 2005.
Johnson, K., Jensen, M., and Giangrande, S.: Active Remote Sensing of CLouds (ARSCL) product using Ka-band ARM Zenith Radars (ARSCLKAZRBND1KOLLIAS), Atmospheric Radiation Measurement (ARM) User Facility [data set], https://doi.org/10.5439/1228769, 2018.
Keeler, E., Burk, K., and Kyrouac, J.: Balloon-Borne Sounding System (SONDEWNPN), Atmospheric Radiation Measurement (ARM) User Facility [data set], https://doi.org/10.5439/1595321, 2018.
Kohler, H.: The nucleus in and the growth of hygroscopic droplets, Trans. Farad. Soc., 32, 1152–1161, 1936.
Koontz, A., Flynn, C., and Shilling, J.: Aerosol Optical Depth (AOD) derived from MFRSR measurements (MFRSRAOD1MICH), Atmospheric Radiation Measurement (ARM) User Facility [data set], https://doi.org/10.5439/1356805, 2018a.
Koontz, A., Flynn, C., Kuang, C., Andrews, E., Hayes, C., Singh, A., and Salwen, C.: Condensation Particle Counter (AOSCPCU), Atmospheric Radiation Measurement (ARM) User Facility [data set], https://doi.org/10.5439/1228061, 2018b.
Koontz, A., Kuang, C., Andrews, E., Hayes, C., Singh, A., and Salwen, C.: Condensation Particle Counter (AOSCPCF), Atmospheric Radiation Measurement (ARM) User Facility [data set], https://doi.org/10.5439/1352536, 2018c.
Koontz, A., Uin, J., Andrews, E., Enekwizu, O., Hayes, C., and Salwen, C.: Cloud Condensation Nuclei Particle Counter (AOSCCN2COLA), Atmospheric Radiation Measurement (ARM) User Facility [data set], https://doi.org/10.5439/1323892, 2018d.
Koontz, A., Uin, J., Andrews, E., Enekwizu, O., Hayes, C., and Salwen, C.: Cloud Condensation Nuclei Particle Counter (AOSCCN2COLB), Atmospheric Radiation Measurement (ARM) User Facility [data set], https://doi.org/10.5439/1323893, 2018e.
Koontz, A., Springston, S., and Trojanowski, R.: Carbon Monoxide Analyzer (AOSCO), Atmospheric Radiation Measurement (ARM) User Facility [data set], https://doi.org/10.5439/1250819, 2018f.
Koontz, A., Mei, F., Stephenson, J., and Pekour, M.: Condensation particle counter aboard aircraft (AAFCPCU), Atmospheric Radiation Measurement (ARM) User Facility [data set], https://doi.org/10.5439/1349237, 2018g.
Koontz, A., Mei, F., Stephenson, J., and Pekour, M.: Condensation particle counter aboard aircraft (AAFCPCFISO), Atmospheric Radiation Measurement (ARM) User Facility [data set], https://doi.org/10.5439/1373109, 2018h.
Koontz, A., Mei, F., and Pekour, M.: Cloud Condensation Nuclei Particle Counter aboard Aircraft (AAFCCN2COLA), Atmospheric Radiation Measurement (ARM) User Facility [data set], https://doi.org/10.5439/1349242, 2018i.
Koontz, A., Mei, F., and Pekour, M.: Cloud Condensation Nuclei Particle Counter aboard Aircraft (AAFCCN2COLB), Atmospheric Radiation Measurement (ARM) User Facility [data set], https://doi.org/10.5439/1349243, 2018j.
Kossmann, M., Corsmeier, U., de Wekker, S. F. J., Fiedler, F., Vögtlin, R., Kalthoff, N., Güsten, H., and Neininger, B.: Observations of handover processes between the atmospheric boundary layer and the free troposphere over mountainous terrain, Contr. Atmos. Phys., 72, 329–350, 1999.
Kuang, C., Singh, A., Howie, J., Salwen, C., and Hayes, C.: Scanning mobility particle sizer (AOSSMPS), Atmospheric Radiation Measurement (ARM) User Facility [data set], https://doi.org/10.5439/1476898, 2018.
Kulkarni, G., Levin, M., and Shilling, J.: CCN Counter derived hygroscopicity parameter kappa (AOSCCNSMPSKAPPA), Atmospheric Radiation Measurement (ARM) User Facility [data set], https://doi.org/10.5439/1729907, 2018.
Kulkarni, G., Mei, F., Shilling, J., Wang, J., Reveggino, R., Flynn, C., Zelenyuk, A., and Fast, J. D.: Cloud condensation nuclei closure study Using airborne measurements over the southern Great Plains, J. Geophys. Res., 128, e2022JD037964, https://doi.org/10.1029/2022JD037967, 2023.
Kyrouac, J., Shi, Y., and Tuftedal, M.: Surface Meteorological Instrumentation (MET), Atmospheric Radiation Measurement (ARM) User Facility [data set], https://doi.org/10.5439/1786358, 2018.
Langford, A. O., Senff, C. F., Alvarez, R. J., Banta, R. M., and Hardesty, R. M.: Long-range transport of ozone from the Los Angeles Basin: A case study, Geophy. Res. Lett., 37, L06807, https://doi.org/10.1029/2010GL042507, 2010.
Lopere, R., Muller, S., Menut, L., and Huneeus, N.: Pathways for wintertime deposition of anthropogenic light-absorbing particles on the Central Andes cryosphere, Environ. Pollution, 272, 115901, https://doi.org/10.1016/j.envpol.2020.115901, 2021.
Lu, R. and Turco, R. P.: Air pollutant transport in a coastal environment, J. Atmos. Sci., 51, 2285–2308, https://doi.org/10.1175/1520-0469(1994)051<2285:APTIAC>2.0.CO;2, 1994.
Liu, X., Ma, P.-L., Wang, H., Tilmes, S., Singh, B., Easter, R. C., Ghan, S. J., and Rasch, P. J.: Description and evaluation of a new four-mode version of the Modal Aerosol Module (MAM4) within version 5.3 of the Community Atmosphere Model, Geosci. Model Dev., 9, 505–522, https://doi.org/10.5194/gmd-9-505-2016, 2016.
Marinovici, C. and Tomlinson, J.: Passive cavity aerosol spectrometer aboard aircraft (AAFPCASP), Atmospheric Radiation Measurement (ARM) User Facility [data set], https://doi.org/10.5439/1245509, 2018.
Marquis, J. N., Varble, A. C., Robinson, P., Nelson, T. C., and Friedrich, K.: Low-level mesoscale and cloud-scale interactions promoting deep convection initiation, Mon. Weather Rev., 149, 2473–2495, https://doi.org/10.1175/MWR-D-20-0391.1, 2021.
Marquis, J. N., Feng, Z., Varble, A. C., Nelson, T. C., Houston, A., Peters, J. M., Mulholland, J. P., and Hardin, J.: Near-cloud atmospheric ingredients for deep convection initiation, Mon. Weather Rev., 51, 1247–1267, https://doi.org/10.1175/MWR-D-22-0243.1, 2023.
Martin, S., Artaxo, P., Machado, L., Manzi, A., Souza, R., Schumacher, C., Wang, J., Brito, J., Jardine, K., Medeiros, A., de Sa, T. Biscaro, S., Calheiros, A., Potela, B., Adachi, K., Aiken, A., Albrecht, R., Alexander, L., Andreae, R., Barbosa, H., Buseck, P., Chand, D., Comstock, J., Dubey, M., Fan, J., Fast, J., Fisch, G., Fortner, E., Giagrande, S., Gilles, M., Goldstein, A., Guenther, A., Hubbe, J., Jensen, M., Jimenez, J., Keutsch, F., Kim, S., Kuang, C. Laskin, A., McKinney, K., Mei, F., Miller, M., Nascimento, R., Pauliquevis, T., Pekour, M., Peres, J., Petaja, T., Pohlder, C., Poschl, U., Rizzo, L., Schmid, B., Shilling, J., Dias, M. A. S., Smith, J., Tomlinson, J., Tota, J., and Wendisch, M.: The green ocean Amazon experiment (GoAmazon2014/5) observes pollution affecting gases, aerosols, clouds, and rainfall over the rain forest, B. Am. Meteorol. Soc., 98, 981–997, https://doi.org/10.1175/BAMS-D-15-00221.1, 2017.
Martins, L. D., Hallak, R., Alves, R. C., de Almeida, D. S., Squizzato, R., Moreira, C. A. B., Beal, A., da Silva, I., Rudke, A., and Martins, J. A.: Long-range Transport of Aerosols from Biomass Burning over Southeastern South America and their Implications on Air Quality, Aerosol Air Qual. Res., 18, 1734–1745, https://doi.org/10.4209/aaqr.2017.11.0545, 2018.
Mather, J. H. and Voyles, J. W.: The ARM Climate Research Facility: A review of structure and capabilities, B. Am. Meteorol. Soc., 94, 377–392, https://doi.org/10.1175/BAMS-D-11-00218.1, 2013.
McFarquhar, G. M., Bretherton, C. S., Marchand, R., Protat, A., DeMott, P. J., Alexander, S. P., Roberts, G. C., Twohy, C. H., Toohey, D., Siems, S., Huang, Y., Wood, R., Rauber, R. M., Lasher-Trapp, S., Jensen, J., Stith, J. L., Mace, J., Um, J., Järvinen, E., Schnaiter, M., Gettelman, A., Sanchez, K. J., McCluskey, C. S., Russell, L. M., McCoy, L. L., Atlas, R. L., Bardeen, C. G., Moore, K. A., Hill, T. C. J., Humphries, R. S., Keywood, M. D., Ristovski, Z., Cravigan, L., Schofield, R., Fairall, C., Mallet, M. S., Kreidenweis, S. M., Rainwater, B., D'Alessandro, J., Wang, Y., Wu, W., Saliba, G., Levin, E. J. T., Ding, S., Lang, F., Truong, S. C. H., Wolff, C., Haggerty, J., Harvey, M. J., Klekociuk, A. R., and McDonald, A.: Observations of Clouds, Aerosols, Precipitation, and Surface Radiation over the Southern Ocean: An Overview of CAPRICORN, MARCUS, MICRE, and SOCRATES, B. Am. Meteorol. Soc., 102, E894-E928, https://doi.org/10.1175/BAMS-D-20-0132.1, 2021.
Mei, F. and Pekour, M.: Scanning mobility particle sizer aboard aircraft (AAFSMPS), Atmospheric Radiation Measurement (ARM) User Facility [data set], https://doi.org/10.5439/1961525, 2018.
Mei, F., Burk, K., Ermold, B., and Zhang, D.: Fast Cloud Droplet Probe aboard aircraft (AAFFCDP), Atmospheric Radiation Measurement (ARM) User Facility [data set], https://doi.org/10.5439/1417472, 2018.
Mulena, G. C., Asmi, E. M., Ruiz, J. J., Pallotta, J. V., and Jin, Y.: Biomass burning aerosol observations and transport over northern and central Argentina: A case study, Remote Sens., 16, 1780, https://doi.org/10.3390/rs16101780, 2024.
Nelson, T. C., Marquis, J., Peters, J., and Friedrich, K.: Environmental controls on simulated deep moist convection initiation occurring during RELAMPAGO-CACTI, J. Atmos. Sci., 79, 1941–1964, https://doi.org/10.1175/JAS-D-21-0226.1, 2022.
Nesbitt, S. W., Salio, P. V., Ávila, E., Bitzer, P., Carey, L., Chandrasekar, V., Deierling, W., Dominguez, F., Dillon, M. E., Garcia, C. M., Gochis, D., Goodman, S., Hence, D. A., Kosiba, K. A., Kumjian, M. R., Lang, T., Luna, T. M., Marquis, J., Marshall, R., McMurdie, L. A., de Lima Nascimento, E., Rasmussen, K. L., Roberts, R., Rowe, A. K., Ruiz, J. J., São Sabbas, E. F. M. T., Saulo, A. C., Schumacher, R. S., Skabar, Y. G., Machado, L. A. T., Trapp, R. J., Varble, A. C., Wilson, J., Wurman, J., Zipser, E. J., Arias, I., Bechis, H., and Grover, M. A.: A storm safari in subtropical South America: Proyecto RELAMPAGO, B. Am. Meteorol. Soc., 102, E1621–E1644, https://doi.org/10.1175/BAMS-D-20-0029.1, 2021.
Ng, N. L., Herndon, S. C., Trimborn, A., Canagaratna, M. R., Croteau, P. L., Onasch, T. B., Sueper, D., Worsnop, D. R., Zhang, Q., Sun, Y. L., and Jayne, J. T.: An aerosol chemical speciation monitor (ACSM) for routine monitoring of the composition and mass concentrations of ambient aerosol, Aerosol Sci. Tech., 45, 780–794, https://doi.org/10.1080/02786826.2011.560211, 2011.
Nyeki, S., Kalberer, M., Colbeck, I., De Wekker, S., Furger, M., Gäggeler, H. W., Kossmann, M., Lugauer, M., Steyn, D., Weingartner, E., Wirth, M., and Baltensperger, U.: Convective boundary layer evolution to 4 km asl over High-alpine terrain: Airborne lidar observations in the Alps, Geophys. Res. Lett., 27, 689–692, https://doi.org/10.1029/1999GL010928, 2000.
O'Donnell, S. E., Akherati, A., He, Y., Hodshire, A. L., Shilling, J. E., Kuang, C., Fast, J. D. Fast, Mei, F., Schobesberger, S., Thornton, J. A., Smith, J. N., Jathar, S. H., and Pierce, J. R.: Look up: Probing the vertical profile of new particle formation and growth in the planetary boundary layer with models and observations, J. Geophys. Res., 128, e2022JD037525, https://doi.org/10.1029/2022JD037525, 2023.
Pekour, M. and Ermold, B.: Aerosol Size Distribution Best Estimate from sensors aboard aircraft (AAFMERGEDAEROSOLSD), Atmospheric Radiation Measurement (ARM) User Facility [data set], https://doi.org/10.5439/1905541, 2023.
Petters, M. D. and Kreidenweis, S. M.: A single parameter representation of hygroscopic growth and cloud condensation nucleus activity, Atmos. Chem. Phys., 7, 1961–1971, https://doi.org/10.5194/acp-7-1961-2007, 2007.
Reddington, C. L., Carslaw, K. S., Stier, P., Schutgens, N., Coe, H., Liu, D., Allan, J., Browse, J., Pringle, K. J., Lee, L. A., Yoshioka, M., Johnson, J. S., Regayre, L. A., Spracklen, D. V., Mann, G. W., Clarke, A., Hermann, M., Henning, S., Wex, H., Kristensen, T. B., Leaitch, W. R., Pöschl, U., Rose, D., Andreae, M. O., Schmale, J., Kondo, Y., Oshima, N., Schwarz, J. P., Nenes, A., Anderson, B., Roberts, G. C., Snider, J. R., Leck, C., Quinn, P. K., Chi, X., Ding, A., Jimenez, J. L., and Zhang, Q.: The Global Aerosol Synthesis and Science Project (GASSP): Measurements and modeling to reduce uncertainty, B. Am. Meteorol. Soc., 98, 1857–1877, https://doi.org/10.1175/bams-d-15-00317.1, 2017.
Rolph, G., Stein, A., and Stunder, B.: Real-time Environmental Applications and Display sYstem: READY, Environ. Model. Softw., 95, 210–228, https://doi.org/10.1016/j.envsoft.2017.06.025, 2017.
Rosenfeld, D., Andreae, M. D., Chin, A. A. M., de Leeuw, G., Donovan, D. P., Kahn, R., Kinne, S., Kivekäs, N., Kulmala, M., Lau, W., Schmidt, K. S., Suni, T., Wagner, T., Wild, M., and Quaas, J.: Global observations of aerosol-cloud-precipitation-climate interactions, Rev. Geophys., 52, 750–808, https://doi.org/10.1002/2013RG000441, 2014.
Saliba, G., Bell, D., Suski, K., Fast, J., Imre, D., Kulkarni, G., Mei, F., Muelmenstaedt, J. H., Pekour, M., Shilling, J. E., Tomlinson, J., Varble, A. C., Thornton, J. A., and Zelenyuk, A.: Aircraft measurements of single particle size and composition reveal aerosol size and mixing state dictate their activation into cloud droplets, Environ. Sci. Atmos., 3, 1352, https://doi.org/10.1039/D3EA00052D, 2023.
Sasaki, C. R. S., Rowe, A. K., McMurdie, L. A., Varble, A. C., and Zhang, Z.: Influences of the South American low-level jet on the convective environment in central Argentina using a convection-Permitting simulation, Mon. Weather Rev., 152, 629–648, https://doi.org/10.1175/MWR-D-23-0122.1, 2024.
Schill, G. P., Froyd, K. D., Bian, H., Kupc, A., Williamson, C., Brock, C. A., Ray, E., Hornbrook, R. S., Hills, A. J., Apel, E. C., Chin, M., Colarco, P. R., and Murphy, D. M.: Widespread biomass burning smoke throughout the remote troposphere, Nat. Geosci., 13, 422–427, https://doi.org/10.1038/s41561-020-0586-1, 2020.
Schmid, B., Tomlinson, J. M., Hubbe, J. M., Comstock, J. M., Mei, F., Chand, D., Pekour, M. S., Kluzek, C. D., Andrews, E., Biraud, S. C., and McFarquhar, G. M.: The DOE ARM Aerial Facility, B. Am. Meteorol. Soc., 95, 723–742, https://doi.org/10.1175/BAMS-D-13-00040.1, 2014.
Schwarz, J. P., Gao, R. S., Fahey, D. W., Thomson, D. S., Watts, L. A., Wilson, J. C., Reeves, J. M., Darbeheshti, M., Baumgardner, D. G., Kok, G. L., Chung, S. H., Schulz, M., Hendricks, J., Lauer, A., Kärcher, B., Slowik, J. G., Rosenlof, K. H., Thompson, T. L., Langford, A. O., Loewenstein, M., and Aikin, K. C.: Single-particle measurements of midlatitude black carbon and light-scattering aerosols from the boundary layer to the lower stratosphere, J. Geophys. Res., 111, D16207, https://doi.org/10.1029/2006JD007076, 2006.
Seinfeld, J. H., Bretherton, C., Carslaw, K. S., Coe, H., DeMott, P. J. Dunlea, E. J., Feingold, G., Ghan, S., Guenther, A. B., Kahn, R., Kraucunas, I., Kreidenweis, S. M., Molina, M. J., Nenes, A., Penner, J. E., Prather, K. A., Ramanathan, V., Ramaswamy, V., Rasch, P. J., Ravishankara, A. R., Rosenfeld, D., Stephens, G., and Wood, R.: Improving our fundamental understanding of the role of aerosol–cloud interactions in the climate system, P. Natl. Acad. Sci. USA, 113, 5781–5790, https://doi.org/10.1073/pnas.1514043113, 2016.
Springston, S., Koontz, A., and Trojanowski, R.: Ozone Monitor (AOSO3), Atmospheric Radiation Measurement (ARM) User Facility [data set], https://doi.org/10.5439/1346692, 2018.
Stein, A. F., Draxler, R. R., Rolph, G. D., Stunder, B. J. B., Cohen, M. D., and Ngan, F.: NOAA's HYSPLIT atmospheric transport and dispersion modeling system, B. Am. Meteorol. Soc., 96, 2059–2077, https://doi.org/10.1175/BAMS-D-14-00110.1, 2015.
Tilmes, S., Hodzic, A., Emmons, L. K., Mills, M. J., Gettelman, A., Kinnison, D. E., Park, M., Lamarque, J.-F., Vitt, F., Shrivastava, M., Campuzano Jost, P., Jimenez, J., and Liu, X.: Climate forcing and trends of organic aerosols in the Community Earth System Model (CESM2), J. Adv. Model Earth Sys., 11, 4323–4351, https://doi.org/10.1029/2019MS001827, 2019.
Tilmes, S., Emmons, L. K., Buchholz, R. R., and the CESM2 development team: CESM2.2 CAM-chem as Boundary Conditions, Research Data Archive at the National Center for Atmospheric Research, Computational and Information Systems Laboratory [data set], https://doi.org/10.5065/XS0R-QE86, 2022.
Tomlinson, J.: Ultra high sensitivity aerosol spectrometer aboard aircraft (UHSAS-air), Atmospheric Radiation Measurement (ARM) User Facility [data set], https://doi.org/10.5439/1524780, 2018.
Twohy, C. H., Petters, M. D., Snider, J. R., Stevens, B., Tahnk, W., Wetzel, M., Russell, L., and Burnet, F.: Evaluationof the aerosol indirect effect in marine stratocumulus clouds: Droplet number, size, liquid water path, and radiative impact, J. Geophys.Res., 110, D08203, https://doi.org/10.1029/2004JD005116, 2005.
Twomey, S.: Pollution and the planetary albedo, Atmos. Environ., 8, 1251–1256, https://doi.org/10.1016/0004-6981(74)90004-3, 1974.
Uin, J., Senum, G., Koontz, A., Flynn, C., Salwen, C., and Hayes, C.: Ultra-High Sensitivity Aerosol Spectrometer (AOSUHSAS), Atmospheric Radiation Measurement (ARM) User Facility [data set], https://doi.org/10.5439/1409033, 2018.
Varble, A., Nesbitt, S. W., Salio, P., Avila, E., Borque, P., DeMott, P., McFarquhar, G., van den Heever, S., Zipser, E., Gochis, D., Houze, R., Jensen, M., Kollias, P., Kreidenweis, S., Leung, R., Rasmussen, K., Romps, D., and Williams, C.,: Cloud, Aerosol, and Complex Terrain Interactions (CACTI) field campaign report, Tech. Doc. DOE/SC-ARM-19-028, http://www.arm.gov/publications/programdocs/doe-sc-arm-19-028.pdf (last access: 26 November 2024), 2019.
Varble, A., Nesbitt, S. W., Salio, P., Hardin, J.C., Bharadwaj, N., Borque, P., DeMott, P., Feng, Z., Hill, T., Marquis, J., Matthews, A., Mei, F., Oktem, R., Castro, V., Goldberger, L., Hunzinger, A., Barry, K., Kreidenweis, S., McFarquhar, G. M., McMurdie, L., Pekour, M., Powers, H., Romps, D. M., Saulo, C., Schmid, B., Tomlinson, J., van den Heever, S., Zelenyuk, A., Zhang, Z., and Zipser, E.: Utilizing a storm-generating hotspot to study convective cloud transitions: The CACTI experiment, B. Am. Meteorol. Soc., 102, E1597–E1620, https://doi.org/10.1175/BAMS-D-20-0030.1 2021.
Varble, A. C.: Cloud, Aerosol, and Complex Terrain Interactions (CACTI) Field, Atmospheric Radiation Measurement (ARM) User Facility [data set], https://ww.arm.gov/research/campaigns/amf2018cacti (last access: 2 December 2024), 2019.
Varble, A. C., Igel, A. L., Morrison, H., Grabowski, W. W., and Lebo, Z. J.: Opinion: A critical evaluation of the evidence for aerosol invigoration of deep convection, Atmos. Chem. Phys., 23, 13791–13808, https://doi.org/10.5194/acp-23-13791-2023, 2023.
Veals, P., Varble, A. C., Russell, J. O. H., Hardin, J. C., and Zipser, E. J.: A decrease in the depth of deep convective cores with increasing aerosol concentration during the CACTI campaign, J. Atmos. Sci., 79, 705–722, https://doi.org/10.1175/JAS-D-21-0119.1, 2022.
Wang, Y., Bagya Ramesh, C., Giangrande, S. E., Fast, J., Gong, X., Zhang, J., Tolga Odabasi, A., Oliveira, M. V. B., Matthews, A., Mei, F., Shilling, J. E., Tomlinson, J., Wang, D., and Wang, J.: Examining the vertical heterogeneity of aerosols over the Southern Great Plains, Atmos. Chem. Phys., 23, 15671–15691, https://doi.org/10.5194/acp-23-15671-2023, 2023.
Watson-Parris, D., Schutgens, N., Reddington, C., Pringle, K. J., Liu, D., Allan, J. D., Coe, H., Carslaw, K. S., and Stier, P.: In situ constraints on the vertical distribution of global aerosol, Atmos. Chem. Phys., 19, 11765–11790, https://doi.org/10.5194/acp-19-11765-2019, 2019.
Wofsy, S. C., Daube, B., Jimenez, R., Kort, E., Pittman, J. V., Park, S., Commane, R., Xiang, B., Santoni, G., Jacob, D., Fisher, J., Pickett-Heaps, C., Wang, H., Wecht, K. J., Wang, Q.-Q., Stephens, B. B., Shertz, S. Romashkin, P., Campos, T., Haggerty, J., Cooper, W. A., Rogers, D., Beaton, S., Hendershot, R., Elkins, J. W., Fahey, D., Gao, R., Moore, F., Montzka, S. A., Schwarz, J., Hurst, D., Miller, B., Sweeney, C., Oltmans, S., Nance, D., Hintsa, E., Dutton, G., Watts, L., Spackman, R., Rosenlof, K., Ray, E., Zondlo, M. A., Diao, M., Keeling, R., Bent, J., Atlas, E., Lueb, R., Mahoney, M. J., Chahine, M., Olson, E., Patra, P., Ishijima, K., Engelen, R., Flemming, J., Nassar, R., Jones, D. B. A., and Fletcher, S. E. M.: HIAPER Pole-to-Pole Observations (HIPPO): Fine-grained, global scale measurements of climatically important atmospheric gases and aerosols, Philos. T. Roy. Soc., 369, 2073–2086, https://doi.org/10.1098/rsta.2010.0313, 2011.
Wood, R., Mechoso, C. R., Bretherton, C. S., Weller, R. A., Huebert, B., Straneo, F., Albrecht, B. A., Coe, H., Allen, G., Vaughan, G., Daum, P., Fairall, C., Chand, D., Gallardo Klenner, L., Garreaud, R., Grados, C., Covert, D. S., Bates, T. S., Krejci, R., Russell, L. M., de Szoeke, S., Brewer, A., Yuter, S. E., Springston, S. R., Chaigneau, A., Toniazzo, T., Minnis, P., Palikonda, R., Abel, S. J., Brown, W. O. J., Williams, S., Fochesatto, J., Brioude, J., and Bower, K. N.: The VAMOS Ocean-Cloud-Atmosphere-Land Study Regional Experiment (VOCALS-REx): goals, platforms, and field operations, Atmos. Chem. Phys., 11, 627–654, https://doi.org/10.5194/acp-11-627-2011, 2011.
Zawadowicz, M., Watson, T., Hayes, C., Allain, M., Salwen, C., and Behrens, B.: Aerosol Chemical Speciation Monitor (AOSACSM), Atmospheric Radiation Measurement (ARM) User Facility [data set], https://doi.org/10.5439/1558768, 2018.
Zelenyuk, A., Berg, L. K., Wilson, J., Zhang, Z., Wang, J., and Mueller, K., Airborne single particle mass spectrometers (SPLAT II & miniSPLAT) and new software for data visualization and analysis in a geo-spatial context, J. Am. Soc. Mass Spectrom., 26, 257–270, https://doi.org/10.1007/s13361-014-1043-4, 2015.
Zhang, Z., Varble, A. C., Feng, Z., Hardin, J., and Zipser, E.: Growth of mesoscale convective systems in observation and a seasonal convection-permitting simulation over Argentina, Mon. Weather Rev., 149, 3469–3490, https://doi.org/10.1175/MWR-D-20-0411.1, 2021.