the Creative Commons Attribution 4.0 License.
the Creative Commons Attribution 4.0 License.
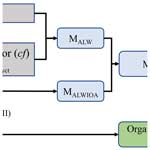
Technical note: Quantified organic aerosol subsaturated hygroscopicity by a simple optical scatter monitor system through field measurements
Jie Zhang
Tianyu Zhu
Alexandra Catena
Yaowei Li
Margaret J. Schwab
Pengfei Liu
Akua Asa-Awuku
James Schwab
The hygroscopicity of organic aerosol (κOA) plays a crucial role in cloud droplet activation and aerosol–radiation interactions. This study investigated the viability of an optical scatter monitor system, featuring two nephelometric monitors (pDR-1500), to determine κOA after knowing the aerosol chemical composition. This system was operated during a mobile lab deployment on Long Island in the summer of 2023, which was executed to coordinate with the Atmospheric Emissions and Reactions Observed from Megacities to Marine Areas (AEROMMA) field campaign. The derived κOA under subsaturated high-humidity conditions (RH between 85 % and 95 %) were categorized based on different aerosol sources, including wildfire aerosol, urban aerosol, and aerosol from rural conditions. The κOA and the OA O:C ratio exhibited linear positive relationships for the urban aerosol and the aerosol from rural conditions, with a much higher slope (0.50 vs. 0.24) for the latter. However, there was no clear relationship between κOA and the OA O:C ratio observed during each period affected by wildfire plumes. The system proposed here could be widely applied alongside the current aerosol component measurement systems, providing valuable insights into the large-scale spatial and temporal variations in OA hygroscopicity.
- Article
(2209 KB) - Full-text XML
-
Supplement
(1832 KB) - BibTeX
- EndNote
Aerosol hygroscopic growth under subsaturated high humidity remains one of the most important research topics in aerosol hygroscopicity (Liu et al., 2018; Wang et al., 2022). This phenomenon can directly determine aerosol liquid water (ALW), which can in turn impact the chemical composition and optical properties of aerosols through aqueous reactions and enhanced light scattering under ambient conditions (Ervens et al., 2011). Additionally, it plays a crucial role in the aerosol's ability to form cloud condensation nuclei (CCN), which can significantly influence cloud formation, related indirect radiative forcing, and in-cloud aqueous chemistry (Seinfeld and Pandis, 2016; Pöhlker et al., 2023). The hygroscopicity parameter under subsaturated conditions (κsub, hereafter “κ” for simplicity) is commonly used to represent aerosol hygroscopic activity and growth (Petters and Kreidenweis, 2007). In addition, κ can be further divided into the inorganic aerosol hygroscopicity (κIOA), which can be inferred from aerosol inorganic compound mass concentration, temperature, and RH (Lance et al., 2013; Cerully et al., 2015), and organic aerosol hygroscopicity (κOA), which is still poorly characterized due to limited knowledge of organic species sources and formation pathways (Jimenez et al., 2009; Shrivastava et al., 2017).
The most common method for deriving κOA involves (1) estimating κ from the hygroscopic growth factor (HGF) measured by the humidified tandem differential mobility analyzers (HTDMAs) (Petters and Kreidenweis, 2007; Wu et al., 2013) and (2) calculating κIOA from the inorganic aerosol mass concentration measured by the co-located aerosol mass spectrometer (AMS) (Zhang et al., 2007) or aerosol chemical speciation monitor (ACSM) (Ng et al., 2011) through the thermodynamic equilibrium model (Fountoukis and Nenes, 2007). However, the combination of these two complicated and expensive instruments (HTDMA and AMS or ACSM) significantly limited their widespread application for κOA estimation on both spatial and temporal scales. Numerous studies have reported positive correlations between κOA and the aerosol oxidation state (e.g., O:C ratio) (Chang et al., 2010; Massoli et al., 2010; Cappa et al., 2011; Lambe et al., 2011; Kuwata et al., 2013; Rickards et al., 2013) and have suggested a potential method to estimate κOA based on the measured O:C ratio. However, significant discrepancies exist in these relationships, underscoring the critical need for developing a simplified method or a system to obtain κOA with the potential for long-term and widespread application to explore these relationships.
A combination of dry and wet nephelometers has been used to estimate (1) aerosol liquid water content (ALW) (Guo et al., 2015; Kuang et al., 2018) and hygroscopicity (Kuang et al., 2017), relying on the measured aerosol light scattering enhancement factor (fRH) (Fierz-Schmidhauser et al., 2010; Titos et al., 2016). When combined with aerosol chemical composition data, this approach also allows for the determination of κOA (Kuang et al., 2020a; Kuang et al., 2021). These advancements have significantly promoted the application of nephelometers in aerosol hygroscopicity studies, and they also open up possibilities for using currently very popular and inexpensive optical scatter particle monitors for same purpose (e.g., Thermo pDR-1500, priced around USD 5000; there are also even more affordable options like the Purple Air, which costs a few hundred USD, and the Plantower PMS series, which is available for tens of USD). These inexpensive devices, based on single-wavelength nephelometric technology, could potentially be used to infer aerosol hygroscopicity and associated ALW. However, unlike the commonly dry and wet nephelometers that measure particle scattering coefficients to calculate fRH, these inexpensive particle monitors directly report particle mass concentration as a bulk measurement, essentially functioning as “black boxes”. Unfortunately, there are very few studies that explore the potential of these optical particle monitors for such applications.
Zhang et al. (2020) demonstrated the quantitative relationship between the response of the Thermo pDR-1500 (hereafter referred to as “pDR”, a type of optical scatter particle monitors, based on single-wavelength nephelometric technology) under subsaturated high-relative-humidity (RH) conditions and ALW. Building on this, this study extends the application of the optical scatter instrument system introduced by Zhang et al. (2020) to estimate ALW based on the 2023 summer field measurements. ALW is further used to estimate the ALWOA based on the aerosol chemical composition measured by an AMS and subsequently used to estimate κOA. The derived κOA was categorized based on the different aerosol sources, and its relationship with the measured organic aerosol ratio was discussed. Additionally, a comparison with previous studies is conducted to validate the feasibility of this system.
2.1 Field campaigns
The field measurements were conducted from 21 June 2023 to 7 September 2023 in Long Island, NY, utilizing our Atmospheric Sciences Research Center (hereafter ASRC) mobile lab. The data collection involved a combination of on-road measurements for some special case days and off-road measurements while parked beside the Flax Pond Marine Laboratory, Stony Brook University. The ASRC mobile lab is a well-equipped platform featuring an aerosol high-resolution time-of-flight aerosol mass spectrometer (HR-ToF-AMS) for aerosol chemical component mass concentration, two pDRs (one for dry aerosol and one for wet aerosol, as described in Fig. 1), a condensation particle counter (CPC) for aerosol number concentration, several gas monitors (i.e., O3, NO2, CO2, HCHO, CH4, etc.), and an Airmar meteorological monitor. Further details about the ASRC mobile lab can be found in Zhang et al. (2018). In this study, the measurements from AMS and the two pDRs were used with a time-averaging period of 1 h.
The on-road measurement field campaigns were executed as the “2023 Mobile Laboratory Measurements of the Atmospheric Chemical Evolution in Urban Outflow Plumes and their Interplay with Coastal Meteorology over Long Island” project. This project aims to study the ozone–aerosol chemistry dynamics in the urban plume in the lowest layer under the influence of the coastal meteorology over Long Island, urban heat waves, and other extreme events. It is also designed to fully coordinate with and complement other comprehensive field campaigns during the 2023 summer over NYC and its downwind regions, including Long Island, i.e., Atmospheric Emissions and Reactions Observed from Megacities to Marine Areas (AEROMMA), the New York City region for the Coastal Urban Plume Dynamics Study (CUPiDS), and the Synergistic TEMPO Air Quality Science (STAQS). More detailed information about the above campaigns can be found at https://csl.noaa.gov/projects/aeromma (last access: 12 March 2024).
Throughout the measurement period, several periods were significantly influenced by urban plumes from the eastern coastal urban regions, rural plumes from the remote region, or wildfire plumes transported from western Canada. The days with similar aerosol sources will be classified into one group, with a total of three different groups identified in this study. All of these provided a unique opportunity to explore the variation of κOA in each group and its relationship with the measured O:C ratio of organic aerosol from each source.
2.2 System setup
A schematic of the setup for κOA estimation used in the ASRC mobile lab is depicted in Fig. 1, comprising two pDRs, one Aerodyne high-resolution time-of-flight aerosol mass spectrometer (HR-ToF-AMS, hereafter AMS), and one TSI silica dryer (Diffusion Dryer 3062). During the measurements, one pDR was installed downstream of the silica dryer for the dry-aerosol mass concentration (hereafter pDRdry), and one pDR was directly connected to the ambient air under ambient RH conditions for the wet-aerosol mass concentration (hereafter pDRwet). The AMS was used to measure the non-refractory submicrometer particles (NR-PM1) chemical component mass concentration (including organic, sulfate, nitrate, ammonia, and chlorine), and the O:C ratio, and it was also used as the reference aerosol mass concentration instrument to calibrate the pDR measurements. Meanwhile, data collected under the lowest relative humidity conditions reported by pDRwet (RH < 45 %) were utilized to generate a self-correlation scatterplot between the two pDRs (Fig. S1 in the Supplement), which was applied to all data from pDRwet before all further data analysis. During the deployment, the RH in pDRdry ranged between 30 % and 45 %. We used 45 % as the upper RH threshold for self-calibration based on the following considerations: (1) ISORROPIA II model calculations indicate that aerosol liquid water associated with inorganics (ALWIOA) is zero for all data below 45 % RH, and (2) submicrometer internally mixed inorganic–organic particles do not exhibit hygroscopic growth until they reach their deliquescence point, which occurs at approximately 77 % RH (Pope et al., 2010; Jing et al., 2016; Bouzidi et al., 2020). In the mobile lab setup, ambient air was drawn at a flow rate of around 56 L min−1 (liters per minute) into a stainless steel tube with a 2.5 cm diameter equipped with a PM cyclone (URG-2000-30EC) designed to filter particles larger than 2 µm. The TSI silica dryer and pDRwet were linked to the sampling duct of the stainless steel tube via black conductive tubing with an internal diameter of 4.5 mm. The tubing lengths were approximately 0.3 m for the TSI silica dryer and 1 m for the pDRwet. After The TSI silica dryer (roughly 0.5 m long), the pDRdry and AMS were parallelly connected to the dryer output through 0.2 m black tubing. Varied lengths of black tubing were employed to maintain a roughly consistent total airflow path to the pDRdry, pDRwet, and AMS. The airflow was expected to be turbulent based on the calculated Reynolds number (RN = 30 234, as determined from https://www.omnicalculator.com/physics/reynolds-number, last access: 12 March 2024), and the estimated particle loss of the ambient aerosol, with a size between 100 to 1000 nm, from the van inlet to each instrument was less than 1 % (https://www.mpic.de/4230607/particle-loss-calculator-plc, last access: 12 March 2024).
The selection of pDR is based on its capability to report both the temperature and RH of the aerosol flow, along with the aerosol mass concentration. The pDR is a type of nephelometric monitor that utilizes an LED light source with a wavelength of 880 nm. It measures particle scattering within a forward scattering angle range of 60 to 80°. The device converts the intensity of the scattered light it detects into mass concentration values based on the factory calibration, which was aligned using gravimetric-standard Arizona Test Dust (Zhang et al., 2018). The calibration factor for the pDR, defined as the ratio of the aerosol mass concentration reported by the pDR to that of a reference instrument, was shown to be directly proportional to the relative scattering intensity calculated using Mie theory (Zhang et al., 2018) based on the lab tests for the mono-disperse particles (90, 173, 304, 490, and 1030 nm of polystyrene latex sphere (PSL) particles) and for the poly-disperse particles with four different chemical compositions (NaNO3, (NH4)2SO4, sucrose, and adipic acid). Based on laboratory tests and ambient measurements, the pDR exhibited a unimodal distribution for its calibration factor, peaking around 500 nm. This peak was larger than that of another nephelometric monitor tested in parallel, the TSI DRX (operating at a 660 nm wavelength and 90° scattering angle), which peaked at 300–400 nm. The higher peak for the pDR is attributed to its use of a longer wavelength. However, the precise value of the calibration factor is further influenced by aerosol composition, which affects the refractive index and consequently the relative scattering intensity. These findings raise concerns about the calibration of widely used low-cost particle sensors based on single-wavelength nephelometric technology. Generally speaking, the relative scattering intensity, which will be proportional to the report aerosol mass concentration from these low-cost particle sensors, is influenced by particle size, composition, instrument properties (such as light wavelength and scattering angles), and ambient RH as a factor influencing ALW (an important focus of this study). It is challenging to apply simple calibration factors derived from laboratory tests on specific aerosol species to fully correct low-cost sensors. Additionally, the calibration factor for one type of monitor cannot simply be applied to another monitor with different properties (e.g., light wavelength and scattering angles). Addressing these limitations will require further research and targeted calibration efforts specific to each monitor's characteristics.
Furthermore, it was demonstrated that the calibration factor was almost independent of the aerosol wet and dry conditions and was minimally affected by RH variations within the range of 45 % to 95 %, maintaining an accuracy with an error margin of less than 5 %. This is due to the minimal variation in relative scattering intensity caused by aerosol in this RH range after considering the influence of ALW. It should be noted that a minimally affected calibration factor means that the ratio of the calibrated dry-aerosol mass concentration to the monitor-reported value at 45 % RH is very similar to the ratio of the calibrated wet-aerosol mass concentration to the monitor-reported value at 95 % RH. However, the values for wet aerosol (both the calibrated mass concentration and the monitor-reported value) will be larger than those for dry aerosol due to the presence of aerosol liquid water (ALW) under higher-humidity conditions, which will be further discussed below using pDR as an example.
In this way, the aerosol mass concentration reported by pDRwet (hereafter , given in units of µg m−3) can be calibrated based on the calibration factor derived from the aerosol mass concentration measured from pDRdry (hereafter MpDRdry, given in units of µg m−3) and from the reference instrument (AMS in this study, MAMS for the measured mass concentration, given in units of µg m−3). Any increase in the mass concentration measured by the calibrated pDRwet compared to that of the calibrated pDRdry can be attributed solely to the presence of ALW (Zhang et al., 2020).
Both pDR devices were fitted with a “Blue Cyclone” and had their flow rates set to 1.5 LPM, achieving an aerosol diameter 50 % cut point of 2.5 µm. This cut point was chosen to be 2.5 µm instead of 1 µm (the upper size limit of the AMS) to accommodate the enlargement of aerosols under high-RH conditions when using pDRwet. However, the difference in the size range between the pDR devices and the AMS introduced a level of uncertainty into the proposed method, which will be addressed in the following discussion. Aside from the uncertainty due to size differences, the AMS only measures non-refractory aerosols and has limited sensitivity to refractory aerosols (e.g., sea salt), which introduces additional uncertainty and will be discussed further in Sect. 2.3. It is also important to note that the temperature and RH obtained from pDRwet were measured inside of pDRwet and could be affected by the inside temperature of the mobile lab, and the calculated ALW may not accurately represent the real ALW of the ambient aerosol.
As shown in Fig. 1 and described more fully in our previous study (Zhang et al., 2020), the mass of ALW (hereafter MALW, given in units of µg m−3) can be obtained from the subtraction of the calibrated aerosol mass concentration of pDRdry (hereafter MpDRdryc) from the calibrated aerosol mass concentration of pDRwet (hereafter MpDRwetc), as shown in Eq. (1):
Here MpDRdryc was set as equal to the aerosol mass concentration measured by AMS (MAMS), and a calibration factor (cf = ) was applied to to obtain MpDRwetc (). The difference between MpDRwetc and MpDRdryc is attributed to ALW based on the consideration that the only increasing element in the dry aerosol under high RH would be the concentration of the water being absorbed (MALW). Here the cf, obtained from the ratio of MpDRdry to MAMS, was applied to determine the calibrated mass concentration of the wet aerosol () given that the calibration factor is almost independent of the aerosol wet and dry conditions (as described above), as shown in Eq. (2):
Here Mwet is the calibrated mass concentration of the wet aerosol () and is MpDRwetc in Eq. (1).
The thermodynamic equilibrium model ISORROPIA II (Fountoukis and Nenes, 2007) was used to estimate the ALW taken up by the inorganic aerosol compounds (hereafter MALWIOA) based on (1) the inorganic aerosol compound concentrations (, , ) measured by AMS with all other metal ions set to 0 and (2) the RH and temperature measured inside of pDRwet. The calculated MALWIOA is then subtracted from MALW to obtain ALW caused by the organic aerosol compounds (hereafter MALWOA, as shown in Eq. 3):
Following this, the κOA can be inferred from MALWOA following Eq. (4) (Nguyen et al., 2016):
where RH is the relative humidity reported by pDRwet, mOA is the AMS-measured organic aerosol mass concentration, ρw is the water density (1.0 g cm−3), and ρOA is the organic aerosol density. In this study, we used 1.4 g cm−3 for ρOA as this is the commonly used value (Hallquist et al., 2009; Shakya and Griffin, 2010; Nguyen et al., 2016; Riva et al., 2017; Jiang et al., 2019). However, the ρOA can vary significantly depending on the sources and formation pathways of organic aerosols, with a range between 1.2 and 1.6 g cm−3 based on a recent chamber study (El Mais et al., 2023), introducing some uncertainty into our results. In this study, only the data with RH between 85 % and 95 % were considered for estimating κOA in order to (1) match the RH used in HTDMA; (2) reduce the uncertainty of aerosol mass concentration measured by pDRwet under the RH over 95 %, which is suggested by the pDR user manual; and (3) ensure the inorganic aerosol is in an aqueous state. The derived κOA and ambient temperature and RH can be further used to estimate ambient ALW through the inverse calculations based on Eqs. (4) and (3), and the information of ambient ALW can be very useful for the study of aqueous secondary organic aerosol (SOA) formation and evolution. Meanwhile, it also emphasized the possibility of using this system for direct ambient measurements, very similar to the innovative outdoor dry and wet nephelometer system described by Qiao et al. (2024) without drying aerosols first before analysis using the HTDMAs (Tang et al., 2019) and without worrying about altering their actual phase state in ambient air (Qiao et al., 2024).
2.3 Method uncertainty and limitations
In this study, using AMS as the reference instrument for pDRdry could introduce a certain level of uncertainty for the ALW estimation due to (1) the AMS's limited sensitivity to refractory aerosols (e.g., sea salt) and (2) the discrepancy size range detected by the pDRdry and AMS. The coarse-mode particles (including the coarse-mode refractory aerosols) with a diameter between 1 and 2.5 µm detected by pDRdry will not be captured by the AMS. The basic assumption here is that the chemical compositions of the coarse and fine modes are similar to each other throughout the study (Sun et al., 2020) and that the ratio of particle water in the fine and coarse modes will be equal the ratio of fine- and coarse-mode dry mass concentration. This means that the estimated MALW, which is here based on the calibrated aerosol mass concentration from the pDRs using AMS as reference, can represent the liquid water in non-refractory PM1. However, significant uncertainty will be introduced in the estimation of κOA, particularly due to the presence of sea salt and other high-κ refractory components in coarse aerosols (AzadiAghdam et al., 2019), which can greatly increase their hygroscopicity. Due to the limited information on the chemical composition (including refractory components) of fine and coarse aerosols, we can only provide a rough estimate of this uncertainty as a bulk measurement, as shown below. Additionally, this uncertainty was further magnified when calculating MALWOA based on the estimate of MALWIOA from ISORROPIA II. The absence of measurements for metal ions necessitated the assumption of “0” for all such ions in the ISORROPIA II inputs, further compounding the inherent uncertainties of the ISORROPIA II model itself. Moreover, the uncertainty in calculating κOA also comes from using the empirical equation Eq. (4) and the assumed value for the density of organic compounds.
To approximate the uncertainty associated with this proposed method, we categorized the measured O:C ratio into bins with an increment of 0.05, ranging from 0.4 to 1.0, for each group with a different aerosol source. We then assumed that the standard deviation of κOA within each bin reflects the uncertainty in the estimated κOA based on the assumption κOA is linearly related to O:C ratio for each specific aerosol source group. The maximum standard deviation of κOA across all bins of the identified three groups was determined to be 0.08, with the mean value of κOA for this bin set as 0.18, which was expected as the upper limit of the uncertainty for κOA. More detailed information about the distribution of κOA in each bin for each group with different aerosol sources and its relationship with the measured O:C ratio is discussed in Sect. 2.5. Meanwhile, it is crucial to acknowledge that this study does not account for the impact of black or brown carbon on the results, as both the pDR devices and the AMS do not detect black or brown carbon.
It is important to note that the derived κOA values in this study were not continuous, as we could only obtain them under high-relative-humidity (RH) conditions (85 % to 90 %). Additionally, our current inability to maintain aerosol under such high-RH conditions limited the laboratory calibration and verification of this method using substances with known hygroscopic parameters (Fierz-Schmidhauser et al., 2010; Zieger et al., 2013; Han et al., 2022), despite this method being theoretically feasible. To resolve this issue, one possible update of this system could be adding a humidifier system to the pDR to get wet aerosol with RH between 85 % to 95 %, and the possible setup for the humidifier system could include a Perma Pure MH Series humidifier, water pumps, and tanks (dashed red box in Fig. 2). This will make this system more similar to the widely used humidified nephelometer system (Guo et al., 2015; Burgos et al., 2019; Fierz-Schmidhauser et al., 2010; Kuang et al., 2017, 2018, 2020b, 2021).
The proposed instrument setup for lab calibration will include an atomizer to produce aerosol, which will be dried through the dryer. Following this, the Differential Mobility Analyzer (DMA) will pick up the different sizes of aerosols, with one branch of the aerosol flowing into the humidifier system to get wet and then becoming pDRwet and another three branches of aerosol flow for pDRdry, AMS, and the condensation particle counter (CPC), respectively. The substances and aerosols for testing will include the organic aerosols with known hygroscopic parameters (Han et al., 2022), inorganic aerosols (i.e., (NH4)2SO4, Fierz-Schmidhauser et al., 2010), and mixing solutions of organic and inorganic aerosols. Due to limited resources, this proposed instrument setup is not feasible at this moment, and the lab calibration is not included in this study. However, we hope this will inspire other research groups with this setup to conduct these lab tests to better quantify the uncertainty of this method for pDRs. Given that the pDR is a type of single-wavelength nephelometric monitor, it is logical to consider that other brands of commonly used low-cost nephelometric monitors (e.g., Purple Air, Plantower PMS series) might offer similar capabilities, and related lab tests of these instruments are also highly recommended.
3.1 Overview of measurements
The time series of all calibrated aerosol mass concentrations measured by the pDRs are shown in Fig. 3a, revealing significant discrepancies between pDRdryc and pDRwetc under high-RH conditions (Fig. 3b). This highlights the contribution of ALW to the response of the pDRwetc. As shown in Fig. 3b, the mass growth factor () was mainly between 2 and 4 under an RH range of 90 % to 100 %, with an averaged value of 2.5, which was generally higher than the value under the RH range of 80 % to 90 % with an averaged value of 1.3. Notably, there were several points with growth factors around 1.3 under their RH range of 90 % to 100 %, suggesting their weaker hygroscopicity compared to the points with a growth factor between 2 and 4. This discrepancy also implies different sources for these two distinct RH ranges.
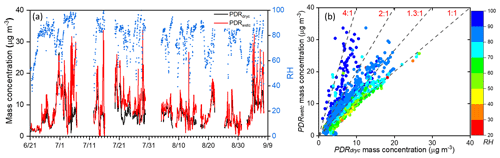
Figure 3(a) The time series of 1 h average aerosol mass concentration measured by pDRwetc and pDRdryc and (b) the correlation scatterplot of pDRwetc and pDRdryc colored by RH. The dashed lines represent the ratio lines of MpDRwetc to MpDRdryc at 1:1, 1.3:1, 2:1, and 4:1.
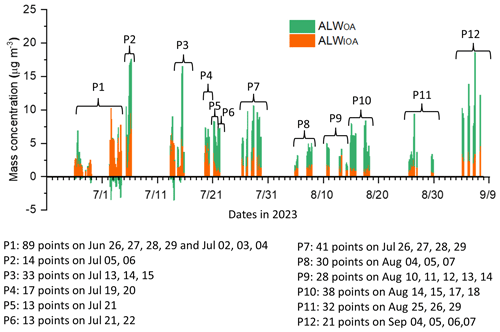
Figure 4The time series of stacked column of the ALWOA and ALWIOA with a time resolution of 1 h. P1–P12 denote the different subperiods mentioned in the text, with the data points and time periods of each subperiod indicated.
As described in the Sect. 2, only the pDRwetc with RH between 85 % and 95 % was considered for κ estimation, and the calculated MALWIOA, MALWOA, and MALW () values are shown in Fig. 4. ALW could be as high as about 18.6 µg m−3 with a related mass growth factor around 2.9. During the initial half of the deployment, there were some points with MALWOA below 0, and this occurred when MALWIOA, as estimated from the ISORROPIA II model, exceeded the total MALW derived from the two pDR measurements. Such negative values can be attributed to previously discussed uncertainties in either the calibration of the pDR devices or the estimations made by the ISORROPIA II model and will also result in negative κOA values, as described below. When considering all the points with MALWOA over 0, ALWOA showed significant contributions to the total wet-aerosol mass concentration, with an average fraction of 27 % and a range of 15 % to 39 % within the 25th to 75th percentiles of the dataset. This underscores the necessity of obtaining accurate κOA values to better obtain ALWOA and evaluate its impact on aerosol evolution.
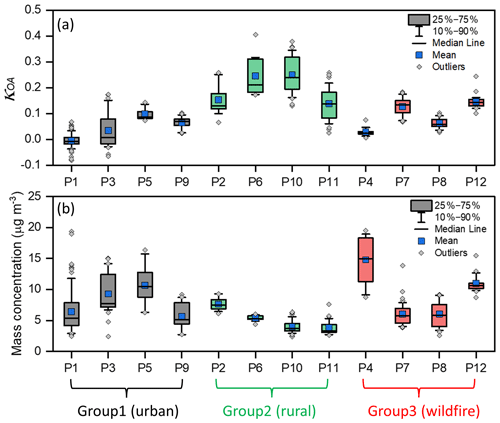
Figure 5The box-and-whisker distribution of κOA and aerosol total mass concentration for each subperiod. The time resolution of each data point is 1 h. The subperiods being affected by urban plumes are marked in grey and categorized as Group 1 (urban), the ones being affected by rural environments are marked in green and categorized as Group 2 (rural), and the ones being affected by wildfire plumes are marked in red and categorized as Group 3 (wildfire).
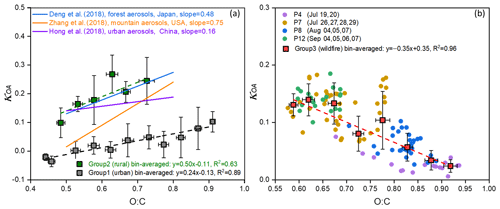
Figure 6The relationship between κOA and O:C of each group. (a) The relationship between κOA and O:C for Group 1 (urban) (marked in grey) and Group 2 (rural) (marked in green). Data points located in each O:C bin are averaged to obtain the bin-averaged κOA and O:C, with the error bar showing their standard deviation in each bin. The bin-averaged κOA and O:C are fitted using the linear regression fit, with the fitting line shown as a dashed line. Meanwhile, the fitted slopes between κOA and O:C from previous studies are presented as solid lines and are used for comparison with the results of this study and to verify the feasibility of the proposed method. (b) The relationship between bin-averaged κOA and O:C for the aerosols affected by the wildfire transports (red squares, with their standard deviation shown as an error bar) and the relationship between κOA and O:C of each subperiod of Group 3 (wildfire) with a time resolution of 1 h.
3.2 Variation of κOA with different aerosol sources
The box-and-whisker distribution of the derived κOA based on Eq. (4) for each subperiod is shown in Fig. 5a alongside the HR-ToF-AMS-measured PM1 mass concentration in Fig. 5b. Subperiods were categorized based on the back trajectories of each subperiod (Figs. S2–S7 in the Supplement). They were divided into three groups with different aerosol sources based on their similar back trajectories, mass concentration, and κOA, including one group with aerosol that have urban sources (marked in grey in Fig. 5, which are hereafter referred to as “Group 1 (urban)”; see Fig. S2 for their back trajectories), one group with aerosol that have rural sources (marked in green in Fig. 5, which are hereafter referred to as “Group 2 (rural)”; see Fig. S3 for their back trajectories), and one group with aerosol affected by the wildfire plumes (marked in red in Fig. 4, which are hereafter referred to as “Group 3 (wildfire)”; see Figs. S4–S7 for their back trajectories). Generally speaking, Group 1 (urban) showed relatively high mass concentrations and lower κOA, which agrees with findings from previous studies that the urban aerosol generally has low hygroscopicity activity (Wu et al., 2016; Hong et al., 2018). At the same time, the points with κOA below 0 were predominantly found in P1 of Group 1 (urban), with values dipping to as low as −0.08, and these negative values fell within the expected upper limited uncertainty of κOA of 0.08 given the averaged κOA of P1 being around 0. The relatively low κOA in P1 followed their low O:C ratio, as shown in Fig. S8 in the Supplement. Conversely, Group 2 (rural) exhibited higher values of κOA compared to other groups, which can be attributed to their exposure to long-term transport and reactions and consequently stronger hygroscopicity activity. Meanwhile, the data points of the subperiods (P10 and P11) of Group 2 (rural) were highly scattered, especially for plume back trajectories over the ocean, highlighting the uncertainty caused by the marine sea salt aerosol. Group 3 (wildfire) demonstrated a large range of κOA, with the subperiod “P4” having the lowest κOA (an averaged value of 0.02 near hydrophobic organics, Kuang et al., 2020a; Han et al., 2022) and the highest mass concentration. Additionally, the subperiod P4 exhibited the most notable transport pathway from western Canada to the NYC metro region (Fig. S4) compared to other wildfire plume cases (Figs. S5–S7). Considering that all four of these cases of wildfire aerosol have an original wildfire source in western Canada, it is reasonable to infer that the wildfire κOA could be strongly affected by the burning time of the original forests, the related burning conditions (i.e., smoldering vs. flaming, etc.), and the transport time from west to east (among other elements; Garofalo et al., 2019), resulting in significant variation between different cases and warranting further investigation.
The derived subsaturated hygroscopicity of organic compounds in both Group 1 (urban) and the Group 2 (rural) exhibited a tight relationship with their O:C ratio, with the κOA increasing as the O:C level rose while distinct slopes for each group, as shown in Figs. 6a and S8. The urban aerosol showed a much smaller linear slope (∼ 0.24) between κOA and O:C compared to the rural aerosol, which had a steeper linear slope of 0.50. The fitted linear slopes of this study closely resembled previous studies that have similar organic aerosol sources. This supports previous findings that the hygroscopicity of urban organic aerosols is much less sensitive to variations in oxidation level than rural organic aerosols (Wu et al., 2016; Hong et al., 2018). Figure 6a also presents the derived slope from previous studies for various atmospheric conditions using more precise instruments for κOA, with an HTDMA being used for the urban aerosol in China by Hong et al. (2018) and the forest aerosols in Japan by Deng et al. (2019), while a CCN counter (CCNc) was used for the rural mountain aerosols in the USA by Zhang et al. (2019). The slope of 0.24 of Group 1 (urban) was close to the value reported in Guangzhou, China, by Hong et al. (2018), and the slope of 0.50 aligned with findings from the forest and mountain aerosols (Deng et al., 2019; Zhang et al., 2019). The close alignment between the results of this study and those from previous research underscores the viability of this simpler system to offer reasonable estimates of κOA in comparison to more precise and costly instruments, such as an HTDMA or CCNc. Meanwhile, the near-constant trends of κOA are shown for each period affected by the wildfire plumes (Fig. 6b), and it is shown that there were no clear linear relationships between κOA and O:C for each period affected by the wildfire. This could be related to the complexity of the wildfire plumes and their long-term transport from west to east. More specifically, they showed a negative relationship when combining all four wildfire periods (Fig. 6b), and further studies will need to verify this and investigate the possible reasons behind this relationship.
Once again, the distinctly different relationships between κOA and O:C between these three groups of organic aerosols indicate the substantial uncertainty in describing the hygroscopicity using a simplified average O:C ratio without considering the possible organic aerosol sources (Kuang et al., 2020a, b; Han et al., 2022) and also highlights the necessity of deriving κOA based on direct measurements.
An inexpensive single-wavelength nephelometer system containing two pDRs (one for dry aerosol and one for wet aerosol) was used to derive the organic aerosol hygroscopicity parameter (κOA) under subsaturated high-humidity conditions (RH between 85 % and 95 %) after knowing the aerosol chemical compound mass concentrations. The derived κOA for the measurement period was largely dependent on the aerosol sources and showed different relationships with the organic aerosol oxidant level (i.e., O:C ratio in this study) for each classification of the aerosol source. In addition, κOA showed a positive linear relationship with O:C ratio for the urban aerosol and the rural aerosol, with a much higher slope being present for the latter (0.24 urban vs. 0.50 rural). Meanwhile, the magnitude of κOA for rural aerosol is much higher than the value for urban aerosol. The fitted relationships agreed well with previous studies, supporting the feasibility of this simple system for estimating κOA. No clear relationship was shown for each period when the organic aerosol was influenced by the transported wildfire plumes. These greatly different κOA vs. O:C relationships for each group, including both slopes and magnitudes, imply the necessity of estimation of κOA through direct measurements, rather than through a simple dependent relationship based on one kind of aerosol property (i.e., O:C ratio).
This approach offers a cost-effective alternative (given that two pDRs cost around USD 10 000) for estimating the κOA of ambient aerosols during field campaigns, especially when utilizing AMS or ACSM to measure the mass concentration of aerosol chemical compounds in situations where HTDMAs or the dry and wet conventional nephelometer systems are not available. Another possible more broad application of this system could be for use with the U.S. EPA Chemical Speciation Network (CSN) for period-averaged κOA after knowing the time-averaged mass concentration of each chemical compound. Further studies for this method using even more affordable options like Purple Air and Plantower PMS series are warranted, and results from these further investigations would largely enhance the role of this kind of optical particle monitor in aerosol hygroscopicity studies, especially given the growing popularity of these monitors in community disadvantage studies, where most projects rely on such devices to monitor PM2.5. The potential widespread use of this method is expected to enhance our understanding of κOA variations and their influence on CCN activities across various spatial and temporal scales. Moreover, it enables the calculation of ambient ALW from the derived κOA, taking into account ambient temperature and RH, which is particularly valuable for studies on atmospheric aqueous phases and the formation of secondary organic aerosols.
The dataset is available upon request from the corresponding author.
The supplement related to this article is available online at: https://doi.org/10.5194/acp-24-13445-2024-supplement.
JZ performed the calculations and data analyses. TZ and AC helped with the data collection. YL, MS, PL, AA, and JS helped interpret the results and revised the manuscript. JZ wrote the paper with contributions from all coauthors.
The contact author has declared that none of the authors has any competing interests.
Publisher's note: Copernicus Publications remains neutral with regard to jurisdictional claims made in the text, published maps, institutional affiliations, or any other geographical representation in this paper. While Copernicus Publications makes every effort to include appropriate place names, the final responsibility lies with the authors.
This work has been supported through the New York State Energy Research and Development Authority (NYSERDA) contract no. 183868. We acknowledge the support and assistance of New York State Department of Environmental Conservation (NYSDEC), Stony Brook University, and in particular the curator of the Flax Pond Marine Laboratory, Stephen Abrams.
This research has been supported by the New York State Energy Research and Development Authority (grant no. 183868).
This paper was edited by Dantong Liu and reviewed by Rodney Weber and one anonymous referee.
AzadiAghdam, M., Braun, R. A., Edwards, E.-L., Bañaga, P. A., Cruz, M. T., Betito, G., Cambaliza, M. O., Dadashazar, H., Lorenzo, G. R., and Ma, L.: On the nature of sea salt aerosol at a coastal megacity: Insights from Manila, Philippines in Southeast Asia, Atmos. Environ., 216, 116922, https://doi.org/10.1016/j.atmosenv.2019.116922, 2019.
Bouzidi, H., Zuend, A., Ondráček, J., Schwarz, J., and Ždímal, V.: Hygroscopic behavior of inorganic–organic aerosol systems including ammonium sulfate, dicarboxylic acids, and oligomer, Atmos. Environ., 229, 117481, https://doi.org/10.1016/j.atmosenv.2020.117481, 2020.
Burgos, M. A., Andrews, E., Titos, G., Alados-Arboledas, L., Baltensperger, U., Day, D., Jefferson, A., Kalivitis, N., Mihalopoulos, N., Sherman, J., Sun, J., Weingartner, E., and Zieger, P.: A global view on the effect of water uptake on aerosol particle light scattering, Scientific Data, 6, 157, https://doi.org/10.1038/s41597-019-0158-7, 2019.
Cappa, C., Che, D., Kessler, S., Kroll, J., and Wilson, K.: Variations in organic aerosol optical and hygroscopic properties upon heterogeneous OH oxidation, J. Geophys. Res., 116, D15204, https://doi.org/10.1029/2011jd015918, 2011.
Cerully, K. M., Bougiatioti, A., Hite Jr., J. R., Guo, H., Xu, L., Ng, N. L., Weber, R., and Nenes, A.: On the link between hygroscopicity, volatility, and oxidation state of ambient and water-soluble aerosols in the southeastern United States, Atmos. Chem. Phys., 15, 8679–8694, https://doi.org/10.5194/acp-15-8679-2015, 2015.
Chang, R. Y.-W., Slowik, J. G., Shantz, N. C., Vlasenko, A., Liggio, J., Sjostedt, S. J., Leaitch, W. R., and Abbatt, J. P. D.: The hygroscopicity parameter (κ) of ambient organic aerosol at a field site subject to biogenic and anthropogenic influences: relationship to degree of aerosol oxidation, Atmos. Chem. Phys., 10, 5047–5064, https://doi.org/10.5194/acp-10-5047-2010, 2010.
Deng, Y., Yai, H., Fujinari, H., Kawana, K., Nakayama, T., and Mochida, M.: Diurnal variation and size dependence of the hygroscopicity of organic aerosol at a forest site in Wakayama, Japan: their relationship to CCN concentrations, Atmos. Chem. Phys., 19, 5889–5903, https://doi.org/10.5194/acp-19-5889-2019, 2019.
El Mais, A. E. R., D'Anna, B., Drinovec, L., Lambe, A. T., Peng, Z., Petit, J.-E., Favez, O., Aït-Aïssa, S., and Albinet, A.: Insights into secondary organic aerosol formation from the day- and nighttime oxidation of polycyclic aromatic hydrocarbons and furans in an oxidation flow reactor, Atmos. Chem. Phys., 23, 15077–15096, https://doi.org/10.5194/acp-23-15077-2023, 2023.
Ervens, B., Turpin, B. J., and Weber, R. J.: Secondary organic aerosol formation in cloud droplets and aqueous particles (aqSOA): a review of laboratory, field and model studies, Atmos. Chem. Phys., 11, 11069–11102, https://doi.org/10.5194/acp-11-11069-2011, 2011.
Fierz-Schmidhauser, R., Zieger, P., Wehrle, G., Jefferson, A., Ogren, J. A., Baltensperger, U., and Weingartner, E.: Measurement of relative humidity dependent light scattering of aerosols, Atmos. Meas. Tech., 3, 39–50, https://doi.org/10.5194/amt-3-39-2010, 2010.
Fountoukis, C. and Nenes, A.: ISORROPIA II: a computationally efficient thermodynamic equilibrium model for K+–Ca2+–Mg2+––Na+–––Cl−–H2O aerosols, Atmos. Chem. Phys., 7, 4639–4659, https://doi.org/10.5194/acp-7-4639-2007, 2007.
Garofalo, L. A., Pothier, M. A., Levin, E. J. T., Campos, T., Kreidenweis, S. M., and Farmer, D. K.: Emission and Evolution of Submicron Organic Aerosol in Smoke from Wildfires in the Western United States, ACS Earth Space Chem., 3, 1237–1247, https://doi.org/10.1021/acsearthspacechem.9b00125, 2019.
Guo, H., Xu, L., Bougiatioti, A., Cerully, K. M., Capps, S. L., Hite Jr., J. R., Carlton, A. G., Lee, S.-H., Bergin, M. H., Ng, N. L., Nenes, A., and Weber, R. J.: Fine-particle water and pH in the southeastern United States, Atmos. Chem. Phys., 15, 5211–5228, https://doi.org/10.5194/acp-15-5211-2015, 2015.
Hallquist, M., Wenger, J. C., Baltensperger, U., Rudich, Y., Simpson, D., Claeys, M., Dommen, J., Donahue, N. M., George, C., Goldstein, A. H., Hamilton, J. F., Herrmann, H., Hoffmann, T., Iinuma, Y., Jang, M., Jenkin, M. E., Jimenez, J. L., Kiendler-Scharr, A., Maenhaut, W., McFiggans, G., Mentel, Th. F., Monod, A., Prévôt, A. S. H., Seinfeld, J. H., Surratt, J. D., Szmigielski, R., and Wildt, J.: The formation, properties and impact of secondary organic aerosol: current and emerging issues, Atmos. Chem. Phys., 9, 5155–5236, https://doi.org/10.5194/acp-9-5155-2009, 2009.
Han, S., Hong, J., Luo, Q., Xu, H., Tan, H., Wang, Q., Tao, J., Zhou, Y., Peng, L., He, Y., Shi, J., Ma, N., Cheng, Y., and Su, H.: Hygroscopicity of organic compounds as a function of organic functionality, water solubility, molecular weight, and oxidation level, Atmos. Chem. Phys., 22, 3985–4004, https://doi.org/10.5194/acp-22-3985-2022, 2022.
Hong, J., Xu, H., Tan, H., Yin, C., Hao, L., Li, F., Cai, M., Deng, X., Wang, N., Su, H., Cheng, Y., Wang, L., Petäjä, T., and Kerminen, V.-M.: Mixing state and particle hygroscopicity of organic-dominated aerosols over the Pearl River Delta region in China, Atmos. Chem. Phys., 18, 14079–14094, https://doi.org/10.5194/acp-18-14079-2018, 2018.
Jiang, X., Tsona, N. T., Jia, L., Liu, S., Zhang, H., Xu, Y., and Du, L.: Secondary organic aerosol formation from photooxidation of furan: effects of NOx and humidity, Atmos. Chem. Phys., 19, 13591–13609, https://doi.org/10.5194/acp-19-13591-2019, 2019.
Jimenez, J. L., Canagaratna, M. R., Donahue, N. M., Prevot, A. S. H., Zhang, Q., Kroll, J. H., DeCarlo, P. F., Allan, J. D., Coe, H., Ng, N. L., Aiken, A. C., Docherty, K. S., Ulbrich, I. M., Grieshop, A. P., Robinson, A. L., Duplissy, J., Smith, J. D., Wilson, K. R., Lanz, V. A., Hueglin, C., Sun, Y. L., Tian, J., Laaksonen, A., Raatikainen, T., Rautiainen, J., Vaattovaara, P., Ehn, M., Kulmala, M., Tomlinson, J. M., Collins, D. R., Cubison, M. J., Dunlea, E. J., Huffman, J. A., Onasch, T. B., Alfarra, M. R., Williams, P. I., Bower, K., Kondo, Y., Schneider, J., Drewnick, F., Borrmann, S., Weimer, S., Demerjian, K., Salcedo, D., Cottrell, L., Griffin, R., Takami, A., Miyoshi, T., Hatakeyama, S., Shimono, A., Sun, J. Y., Zhang, Y. M., Dzepina, K., Kimmel, J. R., Sueper, D., Jayne, J. T., Herndon, S. C., Trimborn, A. M., Williams, L. R., Wood, E. C., Middlebrook, A. M., Kolb, C. E., Baltensperger, U., and Worsnop, D. R.: Evolution of Organic Aerosols in the Atmosphere, Science, 326, 1525–1529, https://doi.org/10.1126/science.1180353, 2009.
Jing, B., Tong, S., Liu, Q., Li, K., Wang, W., Zhang, Y., and Ge, M.: Hygroscopic behavior of multicomponent organic aerosols and their internal mixtures with ammonium sulfate, Atmos. Chem. Phys., 16, 4101–4118, https://doi.org/10.5194/acp-16-4101-2016, 2016.
Kuang, Y., Zhao, C., Tao, J., Bian, Y., Ma, N., and Zhao, G.: A novel method for deriving the aerosol hygroscopicity parameter based only on measurements from a humidified nephelometer system, Atmos. Chem. Phys., 17, 6651–6662, https://doi.org/10.5194/acp-17-6651-2017, 2017.
Kuang, Y., Zhao, C. S., Zhao, G., Tao, J. C., Xu, W., Ma, N., and Bian, Y. X.: A novel method for calculating ambient aerosol liquid water content based on measurements of a humidified nephelometer system, Atmos. Meas. Tech., 11, 2967–2982, https://doi.org/10.5194/amt-11-2967-2018, 2018.
Kuang, Y., Xu, W., Tao, J., Ma, N., Zhao, C., and Shao, M.: A Review on Laboratory Studies and Field Measurements of Atmospheric Organic Aerosol Hygroscopicity and Its Parameterization Based on Oxidation Levels, Curr. Pollut. Rep., 6, 410–424, https://doi.org/10.1007/s40726-020-00164-2, 2020a.
Kuang, Y., He, Y., Xu, W., Zhao, P., Cheng, Y., Zhao, G., Tao, J., Ma, N., Su, H., Zhang, Y., Sun, J., Cheng, P., Yang, W., Zhang, S., Wu, C., Sun, Y., and Zhao, C.: Distinct diurnal variation in organic aerosol hygroscopicity and its relationship with oxygenated organic aerosol, Atmos. Chem. Phys., 20, 865–880, https://doi.org/10.5194/acp-20-865-2020, 2020b.
Kuang, Y., Huang, S., Xue, B., Luo, B., Song, Q., Chen, W., Hu, W., Li, W., Zhao, P., Cai, M., Peng, Y., Qi, J., Li, T., Wang, S., Chen, D., Yue, D., Yuan, B., and Shao, M.: Contrasting effects of secondary organic aerosol formations on organic aerosol hygroscopicity, Atmos. Chem. Phys., 21, 10375–10391, https://doi.org/10.5194/acp-21-10375-2021, 2021.
Kuwata, M., Shao, W., Lebouteiller, R., and Martin, S. T.: Classifying organic materials by oxygen-to-carbon elemental ratio to predict the activation regime of Cloud Condensation Nuclei (CCN), Atmos. Chem. Phys., 13, 5309–5324, https://doi.org/10.5194/acp-13-5309-2013, 2013.
Lambe, A. T., Onasch, T. B., Massoli, P., Croasdale, D. R., Wright, J. P., Ahern, A. T., Williams, L. R., Worsnop, D. R., Brune, W. H., and Davidovits, P.: Laboratory studies of the chemical composition and cloud condensation nuclei (CCN) activity of secondary organic aerosol (SOA) and oxidized primary organic aerosol (OPOA), Atmos. Chem. Phys., 11, 8913–8928, https://doi.org/10.5194/acp-11-8913-2011, 2011.
Lance, S., Raatikainen, T., Onasch, T. B., Worsnop, D. R., Yu, X.-Y., Alexander, M. L., Stolzenburg, M. R., McMurry, P. H., Smith, J. N., and Nenes, A.: Aerosol mixing state, hygroscopic growth and cloud activation efficiency during MIRAGE 2006, Atmos. Chem. Phys., 13, 5049–5062, https://doi.org/10.5194/acp-13-5049-2013, 2013.
Liu, P., Song, M., Zhao, T., Gunthe, S. S., Ham, S., He, Y., Qin, Y. M., Gong, Z., Amorim, J. C., Bertram, A. K., and Martin, S. T.: Resolving the mechanisms of hygroscopic growth and cloud condensation nuclei activity for organic particulate matter, Nat. Commun., 9, 4076, https://doi.org/10.1038/s41467-018-06622-2, 2018.
Massoli, P., Lambe, A. T., Ahern, A. T., Williams, L. R., Ehn, M., Mikkilä, J., Canagaratna, M. R., Brune, W. H., Onasch, T. B., Jayne, J. T., Petäjä, T., Kulmala, M., Laaksonen, A., Kolb, C. E., Davidovits, P., and Worsnop, D. R.: Relationship between aerosol oxidation level and hygroscopic properties of laboratory generated secondary organic aerosol (SOA) particles, Geophys. Res. Lett., 37, L24801, https://doi.org/10.1029/2010gl045258, 2010.
Ng, N. L., Herndon, S. C., Trimborn, A., Canagaratna, M. R., Croteau, P. L., Onasch, T. B., Sueper, D., Worsnop, D. R., Zhang, Q., Sun, Y. L., and Jayne, J. T.: An Aerosol Chemical Speciation Monitor (ACSM) for Routine Monitoring of the Composition and Mass Concentrations of Ambient Aerosol, Aerosol Sci. Tech., 45, 780–794, https://doi.org/10.1080/02786826.2011.560211, 2011.
Nguyen, T. K. V., Zhang, Q., Jimenez, J. L., Pike, M., and Carlton, A. G.: Liquid water: ubiquitous contributor to aerosol mass, Environ. Sci. Tech. Let., 3, 257–263, https://doi.org/10.1021/acs.estlett.6b00167, 2016.
Petters, M. D. and Kreidenweis, S. M.: A single parameter representation of hygroscopic growth and cloud condensation nucleus activity, Atmos. Chem. Phys., 7, 1961–1971, https://doi.org/10.5194/acp-7-1961-2007, 2007.
Pöhlker, M. L., Pöhlker, C., Quaas, J., Mülmenstädt, J., Pozzer, A., Andreae, M. O., Artaxo, P., Block, K., Coe, H., Ervens, B., and Gallimore, P.: Global organic and inorganic aerosol hygroscopicity and its effect on radiative forcing, Nat. Commun., 14, 6139. https://doi.org/10.1038/s41467-023-41695-8, 2023.
Pope, F. D., Dennis-Smither, B. J., Griffiths, P. T., Clegg, S. L., and Cox, R. A.: Studies of single aerosol particles containing malonic acid, glutaric acid, and their mixtures with sodium chloride. I. Hygroscopic growth, J. Phys. Chem. A, 114, 5335–5341, 2010.
Qiao, H., Kuang, Y., Yuan, F., Liu, L., Zhai, M., Xu, H., Zou, Y., Deng, T., and Deng, X.: Unlocking the Mystery of Aerosol Phase Transitions Governed by Relative Humidity History Through an Advanced Outdoor Nephelometer System, Geophys. Res. Lett., 51, e2023GL107179, https://doi.org/10.1029/2023GL107179, 2024.
Rickards, A. M. J., Miles, R. E. H., Davies, J. F., Marshall, F. H., and Reid, J. P.: Measurements of the sensitivity of aerosol hygroscopicity and the κ parameter to the ratio, J. Phys. Chem. A, 117, 14120–14131, https://doi.org/10.1021/jp407991n, 2013.
Riva, M., Healy, R. M., Flaud, P.-M., Perraudin, E., Wenger, J. C., and Villenave, E.: Gas- and particle-phase products from the photooxidation of acenaphthene and acenaphthylene by OH radicals, Atmos. Environ., 151, 34–44, https://doi.org/10.1016/j.atmosenv.2016.11.063, 2017.
Seinfeld, J. H. and Pandis, S. N.: Atmospheric chemistry and physics: from air pollution to climate change, John Wiley & Sons, New Jersey, ISBN 9781118947401, 2016.
Shakya, K. M. and Griffin, R. J.: Secondary Organic Aerosol from Photooxidation of Polycyclic Aromatic Hydrocarbons, Environ. Sci. Technol., 44, 8134–8139, https://doi.org/10.1021/es1019417, 2010.
Shrivastava, M., Cappa, C. D., Fan, J., Goldstein, A. H., Guenther, A. B., Jimenez, J. L., Kuang, C., Laskin, A., Martin, S. T., Ng, N. L., Petaja, T., Pierce, J. R., Rasch, P. J., Roldin, P., Seinfeld, J. H., Shilling, J., Smith, J. N., Thornton, J. A., Volkamer, R., Wang, J., Worsnop, D. R., Zaveri, R. A., Zelenyuk, A., and Zhang, Q.: Recent advances in understanding secondary organic aerosol: implications for global climate forcing, Rev. Geophys., 55, 509–559, https://doi.org/10.1002/2016RG000540, 2017.
Sun, Y., He, Y., Kuang, Y., Xu, W., Song, S., Ma, N., Tao, J., Cheng, P., Wu, C., Su, H., Cheng, Y., Xie, C., Chen, C., Lei, L., Qiu, Y., Fu, P., Croteau, P., and Worsnop, D. R.: Chemical differences between PM1 and PM2.5 in Highly polluted environment and implications in air pollution studies, Geophys. Res. Lett., 47, e2019GL086288, https://doi.org/10.1029/2019GL086288, 2020.
Tang, M., Chan, C. K., Li, Y. J., Su, H., Ma, Q., Wu, Z., Zhang, G., Wang, Z., Ge, M., Hu, M., He, H., and Wang, X.: A review of experimental techniques for aerosol hygroscopicity studies, Atmos. Chem. Phys., 19, 12631–12686, https://doi.org/10.5194/acp-19-12631-2019, 2019.
Titos, G., Cazorla, A., Zieger, P., Andrews, E., Lyamani, H., Granados-Munoz, M. J., Olmo, F. J., and Alados-Arboledas, L.: Effect of hygroscopic growth on the aerosol light-scattering coefficient: A review of measurements, techniques and error sources, Atmos. Environ., 141, 494–507, 2016.
Wang, Y., Voliotis, A., Hu, D., Shao, Y., Du, M., Chen, Y., Kleinheins, J., Marcolli, C., Alfarra, M. R., and McFiggans, G.: On the evolution of sub- and super-saturated water uptake of secondary organic aerosol in chamber experiments from mixed precursors, Atmos. Chem. Phys., 22, 4149–4166, https://doi.org/10.5194/acp-22-4149-2022, 2022.
Wu, Z. J., Poulain, L., Henning, S., Dieckmann, K., Birmili, W., Merkel, M., van Pinxteren, D., Spindler, G., Müller, K., Stratmann, F., Herrmann, H., and Wiedensohler, A.: Relating particle hygroscopicity and CCN activity to chemical composition during the HCCT-2010 field campaign, Atmos. Chem. Phys., 13, 7983–7996, https://doi.org/10.5194/acp-13-7983-2013, 2013.
Wu, Z. J., Zheng, J., Shang, D. J., Du, Z. F., Wu, Y. S., Zeng, L. M., Wiedensohler, A., and Hu, M.: Particle hygroscopicity and its link to chemical composition in the urban atmosphere of Beijing, China, during summertime, Atmos. Chem. Phys., 16, 1123–1138, https://doi.org/10.5194/acp-16-1123-2016, 2016.
Zhang, J., Marto, J. P., and Schwab, J. J.: Exploring the applicability and limitations of selected optical scattering instruments for PM mass measurement, Atmos. Meas. Tech., 11, 2995–3005, https://doi.org/10.5194/amt-11-2995-2018, 2018.
Zhang, J., Lance, S., Brandt, R., Marto, J., Ninneman, M., and Schwab, J.: Observed below-cloud and cloud interstitial submicron aerosol chemical and physical properties at Whiteface Mountain, New York, during August 2017, ACS Earth Space Chem., 3, 1438–1450, https://doi.org/10.1021/acsearthspacechem.9b00117, 2019.
Zhang, J., Lance, S., Wang, X., Wang, J., and Schwab, J. J.: Estimation of aerosol liquid water from optical scattering instruments using ambient and dried sample streams, Atmos. Environ., 239, 117787, https://doi.org/10.1016/j.atmosenv.2020.117787, 2020.
Zhang, Q., Jimenez, J., Canagaratna, M., Allan, J., Coe, H., Ulbrich, I., Alfarra, M., Takami, A., Middlebrook, A., Sun, Y., Dzepina, K., Dunlea, E., Docherty, K., De Carlo, P., Salcedo, D., Onasch, T., Jayne, J., Miyoshi, T., Shimono, A., Hatakeyama, S., Takegawa, N., Kondo, Y., Schneider, J., Drewnick, F., Borrmann, S., Weimer, S., Demerjian, K., Williams, P., Bower, K., Bahreini, R., Cottrell, L., Griffin, R., Rautiainen, J., Sun, J., Zhang, Y., and Worsnop, D.: Ubiquity and dominance of oxygenated species in organic aerosols in anthropogenically-influenced Northern Hemisphere midlatitudes, Geophys. Res. Lett., 34, L13801, https://doi.org/10.1029/2007GL029979, 2007.
Zieger, P., Fierz-Schmidhauser, R., Weingartner, E., and Baltensperger, U.: Effects of relative humidity on aerosol light scattering: results from different European sites, Atmos. Chem. Phys., 13, 10609–10631, https://doi.org/10.5194/acp-13-10609-2013, 2013.