the Creative Commons Attribution 4.0 License.
the Creative Commons Attribution 4.0 License.
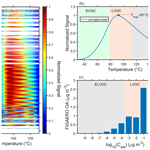
Enhanced daytime secondary aerosol formation driven by gas–particle partitioning in downwind urban plumes
Mingfu Cai
Chenshuo Ye
Shan Huang
E Zheng
Suxia Yang
Zelong Wang
Yi Lin
Tiange Li
Weiwei Hu
Wei Chen
Qicong Song
Wei Li
Yuwen Peng
Baoling Liang
Qibin Sun
Duohong Chen
Jiaren Sun
Zhiyong Yang
Min Shao
Anthropogenic emissions from city clusters can significantly enhance secondary organic aerosol (SOA) formation in the downwind regions, while the mechanism is poorly understood. To investigate the effect of pollutants within urban plumes on organic aerosol (OA) evolution, a field campaign was conducted at a downwind site of the Pearl River Delta region of China in the fall of 2019. A time-of-flight chemical ionization mass spectrometer coupled with the Filter Inlet for Gases and Aerosols (FIGAERO–CIMS) was used to probe the gas- and particle-phase molecular composition and thermograms of organic compounds. For air masses influenced by urban pollution, strong daytime SOA formation through gas–particle partitioning was observed, resulting in higher OA volatility. The obvious SOA enhancement was mainly attributed to the gas–particle partitioning of high-volatility (semi-volatile organic compounds + intermediate volatility organic compounds + volatile organic compounds, µg m−3) organic vapors. Using the equilibrium equation could underestimate the contribution of high-volatility organic vapors, since the volatility of these species in the particle phase was lower than that in the gas phase. We speculated that the elevated NOx concentration could suppress the formation of highly oxidized products, resulting in a smooth increase of low-volatility (extremely low volatility organic compounds + low volatility organic compounds, µg m−3) organic vapors. Evidence has shown that urban pollutants (NOx and VOCs) could enhance the oxidizing capacity, while the elevated VOCs were mainly responsible for promoting daytime SOA formation by increasing the RO2 production rate. Our results highlight the important role of urban anthropogenic pollutants in SOA control in the suburban region.
- Article
(6540 KB) - Full-text XML
-
Supplement
(12371 KB) - BibTeX
- EndNote
As a major concern of air pollution, aerosol particles are known to have significant impacts on public health and climate (Apte et al., 2018; Arias et al., 2024). Primary particulate matter (PM) in China has shown a remarkable reduction since 2013, owing to strict clean-air policies implemented by the Chinese government (Zhang et al., 2019). Despite the effective reduction of primary emissions in the past 10 years, secondary organic aerosol (SOA) remains at high levels and is mainly responsible for the haze development in China (Huang et al., 2014). SOA is thought to be formed through the oxidation of volatile organic compounds (VOCs) and atmospheric aging processes of primary organic aerosol (POA). However, models are especially challenged in reproducing SOA concentration and properties, since the formation mechanisms and gas precursors of SOA remain poorly characterized (Hodzic et al., 2010).
Gas–particle partitioning of organic vapors is found to be the important formation pathway of SOA worldwide (Nie et al., 2022; Hallquist et al., 2009; Lanzafame et al., 2021). Nie et al. (2022) suggested that the contribution of the condensation of organic vapors to the SOA mass growth ranged from about 38 %–71 % in China megacities. Photochemically produced SOA via gas-phase chemistry is usually related to a higher volatility and a lower oxidation degree than that formed in the aqueous phase (Ervens et al., 2011; Saha et al., 2017). The condensation processes of organic vapors are determined by their volatility, which is closely related to oxidation state, functional groups, and the number of atomic carbons. Laboratory studies revealed that high nitrogen oxide (NOx) concentration can suppress the production of molecules with a high oxidation degree by inhibiting autoxidation (Rissanen, 2018; Peng et al., 2019), which is considered to be an important pathway of low-volatility vapor formation (Praske et al., 2018). Such compounds have been shown to play a vital role in the SOA formation and growth of newly formed particles (Mutzel et al., 2015; Bianchi et al., 2019; Mohr et al., 2019). On the other hand, it is shown that the increase of oxidants owing to elevated NOx concentration can offset the decrease of autoxidation efficiency, leading to a higher production of oxygenated organic vapors (Pye et al., 2019), highlighting the complexity of SOA formation. However, the lack of a molecular dataset of SOA and gas precursors hinders the understanding of the SOA formation mechanism.
Recently, a chemical ionization time-of-flight mass spectrometer coupled with the Filter Inlet for Gases and Aerosols (FIGAERO–CIMS) has been employed to measure gas- and particle-phase oxygenated organic compounds worldwide (Chen et al., 2020; Buchholz et al., 2020; Masoud et al., 2022). Using the FIGAERO–CIMS, Cai et al. (2023) showed that a heterogeneous reaction might have an important role in the secondary formation of particle-phase oxidized organic nitrogen. The volatility of organic aerosol (OA) can provide information about the formation and aging processes of OA, given that it is strongly affected by chemical composition. In past decades, a thermodenuder (TD) coupled with aerosol detection instruments (e.g., aerosol mass spectrometer and condensation particle counter) was widely used in the estimation of OA volatility (Philippin et al., 2004; Lee et al., 2010). Cai et al. (2022) found that the OA volatility was higher at a particle size range of 30 to 200 nm during daytime, suggesting that the SOA formation through gas–particle partitioning could generally occur at all particle sizes. However, this method failed to provide the volatility information of different molecules of OA. In recent years, the FIGAERO–CIMS was developed to characterize the volatility of oxygenated organic molecules in the particle phase (Ren et al., 2022; Ylisirniö et al., 2020). Wang and Hildebrandt Ruiz (2018) showed that the thermal desorption products of SOA can be separated into different groups on a two-dimensional thermogram measured by the FIGAERO–CIMS. Ren et al. (2022) investigated the relationship between the molecular formulae of OA components and their volatilities and suggested that the volatility of OA compounds was strongly affected by the O-to-C ratio. These results provide valuable insights into the SOA formation mechanisms. However, as of yet, few FIGAERO–CIMS field studies are available in the literature in China (Ye et al., 2021; Salvador et al., 2021), especially in urban downwind areas.
Observational studies have demonstrated that anthropogenic emissions can significantly affect SOA formation in the downwind region. Fry et al. (2018) observed an enhancement of organic nitrate aerosol formed through NO3+ isoprenes in a power plant plume during nighttime, which was mainly attributed to NOx emissions from the power plant. The results from Liu et al. (2018) suggested that the OH concentrations increased by at least 250 % under polluted conditions, which might promote the daytime SOA formation. A field measurement in the Amazon forest by de Sá et al. (2018) showed that the enhancement of OA (about 30 %–171 %) in urban plumes was mainly contributed by SOA. A recent study found that anthropogenic emissions of NOx from urban areas could enhance oxidant concentrations, thereby promoting daytime SOA formation (Shrivastava et al., 2019).
In this study, we investigate the SOA formation through photochemical reactions at a typical downwind site in the Pearl River Delta (PRD) region using the FIGAERO–CIMS along with a suite of other online instruments. The volatility of OA and its relationship with identified OA sources during long-range transport, urban air mass, and coastal air mass periods are discussed. The formation mechanisms of daytime SOA formation within the urban plume are investigated based on online measurements of gas- and particle-phase organic compounds, gaseous pollutants, and aerosol physicochemical properties. The impact of urban pollutants on SOA formation will be discussed.
2.1 Field measurement
The campaign was conducted at the Heshan supersite in the PRD region during the fall of 2019 (29 September to 17 November 2019). The Heshan supersite, surrounded by farms and villages, is located southwest (22°42 N, 112°55 E, at an altitude of about 40 m) of the PRD region and about 70 km southwest of the city of Guangzhou (Fig. S1 in the Supplement). During the measurement, the sampling site was mainly influenced by the air masses from the center of the PRD region (Fig. S2a). All instruments were placed in an air-conditioned room on the top floor of the supersite. A detailed description of the site and experimental setup can be found in Cai et al. (2021).
2.2 Instrumentation
2.2.1 FIGAERO–CIMS
The FIGAERO–CIMS coupled with an X-ray source was employed to measure organic compounds in the gas- and particle-phase using I− as the chemical ionization reagent. The particle sampling inlet of the FIGAERO–CIMS was equipped with a PM2.5 cyclone and a Nafion dryer (model PD-07018T-12MSS, Perma Pure, Inc., USA). The principle of the instrument can be found in Lopez-Hilfiker et al. (2014) and Le Breton et al. (2018). In general, the operation settings and data processing were the same as in Cai et al. (2023) and Ye et al. (2021). Here, only a brief description relevant to the measurement is given. The instrument was worked in a cycle pattern of 1 h, with 24 min of gas-phase measurements and particle collection (sampling mode), followed by a 36 min particle-phase analysis (desorption mode). In the sampling mode, ambient gas was measured in the first 21 min, followed by a 3 min zero air background. At the same time, ambient particles were collected on a PTFE membrane filter. In the desorption mode, the collected particles were desorbed by heated N2. The temperature of the N2 was linearly ramped from indoor temperature (∼25 °C) to ∼ 175 °C in 12 min and held for 24 min. The data processing steps in this campaign were the same as in Ye et al. (2021). A few chemicals were calibrated before and after the measurement. For uncalibrated species, a voltage scanning method was employed to obtain their sensitivities (referred to as semi-quantified species) (Ye et al., 2021; Iyer et al., 2016; Lopez-Hilfiker et al., 2016).
2.2.2 SP-AMS
The PM1 chemical composition was measured by a soot particle aerosol mass spectrometer (SP-AMS, Aerodyne Research, Inc., USA). The details of the operation and data analysis can be found in Kuang et al. (2021). Source apportionment was performed for organic aerosols in the bulk PM1 using positive matrix factorization (PMF). The organic aerosol could be divided into six components, including two primary OA factors and four secondary OA factors. The mass spectral profiles of six OA factors are shown in Fig. S3. The time series and diurnal variation of these factors are presented in Fig. S4.
The primary OA factors include hydrocarbon-like OA (HOA), mainly contributed by traffic and cooking emissions, and biomass-burning OA (BBOA) originating from biomass-burning combustion. The HOA was identified by hydrocarbon ions CxH. Owing to the prominent hydrocarbon ions and low O:C value (0.10), HOA could be attributed to primary emissions from cooking and traffic. The BBOA was recognized by the markers C2H4O ( 60.022, 0.5 %) and C3H5O ( 73.029, 0.4 %), which are considered tracers of biomass-burning OA (Ng et al., 2011).
The SOA factors include biomass-burning SOA (BBSOA) likely formed from oxidation of biomass-burning emissions, less oxygenated OA (LOOA) provided by strong daytime photochemical formation, more oxygenated OA (MOOA) related to regional transport, and nighttime-formed OA (night-OA) contributed by secondary formation during nighttime. The BBSOA was likely formed through oxidation of biomass-burning precursors, which was supported by the evening peak at about 19:00 LT (Fig. S4). BBSOA showed a similar variation trend with C6H2NO, which might be contributed by oxidation of gaseous precursors from biomass-burning emissions (Wang et al., 2019; Bertrand et al., 2018). The significant afternoon peak of LOOA indicates its formation through photochemical reactions, which will be discussed in detail in Sect. 3.1. The negligible diurnal variation and the highest O:C value (1.0) of MOOA suggested that it could be aged OA resulting from long-range transport. Night-OA was formed through NO3 nighttime chemistry, supported by a pronounced evening elevation and positive correlation with nitrate (R=0.67). The detailed determination of PMF factors has been found in Kuang et al. (2021) and Luo et al. (2022).
2.2.3 Particle number size distribution measurements
Particle number size distribution in a size range of 1 nm–10 µm was measured by a diethylene glycol scanning mobility particle sizer (DEG-SMPS, model 3938E77, TSI Inc., USA), a SMPS (model 3938L75, TSI Inc., USA), and an aerodynamic particle sizer (APS, model 3321, TSI Inc., USA). All sample particles first passed through a Nafion dryer (Model MD-700, Perma Pure Inc., USA) to reduce relative humidity (RH) lower than 30 %. A detailed description of these instruments can be found in Cai et al. (2021).
2.2.4 Other parameters
The non-methane hydrocarbons (NMHCs) were measured by an online gas chromatography–mass spectrometry/flame ionization detector (GC-MS/FID, Wuhan Tianhong Co., Ltd, China). The concentrations of oxygenated VOCs, including formaldehyde (HCHO) and acetaldehyde (CH3CHO), were measured using a high-resolution proton transfer reaction time-of-flight mass spectrometer (PTR-ToF-MS, Ionicon Analytik, Austria). HONO was detected by the gas and aerosol collector (GAC) instrument (Dong et al., 2012). Trace gases, including O3, NOx, and CO, were measured by gas analyzers (model 49i, 42i, and 48i, Thermo Scientific, USA). Meteorological parameters (i.e., wind speed, wind direction, and temperature) were measured by a weather station (Vantage Pro 2, Davis Instruments Co., USA).
2.3 Methodology
2.3.1 Estimation of the volatility of particle- and gas-phase organic compounds
During the heating processes, the FIGAERO–CIMS simultaneously measured the desorbing compounds of the collected particles. Thus, the volatility information of particles can be obtained by investigating the relationship between the measured signals and desorption temperature. The temperature of the peak desorption signal (Tmax) has a nearly linear relationship with the natural logarithm of saturation vapor pressure (Psat) of the respective compound (Lopez-Hilfiker et al., 2014):
where a and b are fitting coefficients. Thus, the saturation vapor concentration (C∗, µg m−3) can be obtained:
where Mw is the molecular weight of the compound (determined by the FIGAERO–CIMS), R is the universal gas constant (8.314 J mol−1 K−1), and T is the thermodynamic temperature in kelvin (298.15 K).
We used a series of polyethylene glycol (PEG 5–8) compounds to calibrate the Tmax and obtained the fitting parameters a and b. The PEG standards were prepared in a mixture of acetonitrile and then atomized with a homemade atomizer. The atomized particles are classified by a differential mobility analyzer (DMA, model 3081 L, TSI Inc., USA) at two diameters (100 and 200 nm). The selected particles were then split into two paths: one to a condensation particle counter (CPC, model 3775, TSI Inc., USA) for measuring the particle concentration and another one to the particle inlet of the FIGAERO–CIMS. The collected concentration can be calculated based on the selected particle diameter, particle number concentration, flow rate of the particle inlet of the FIGAERO–CIMS, and collection time. The calibration results and corresponding fitting parameters can be found in Fig. S5 and Table S1 in the Supplement. Note that the Tmax can vary with mass loading, and it is necessary to consider this for estimation of the relationship between Tmax and C∗ (Wang and Hildebrandt Ruiz, 2018). Our calibration results demonstrated that the correlation between Tmax shift and mass loading was not linear, which may be attributed to matrix or saturation effects (Huang et al., 2018). During the measurement, the collected mass loading centered at about 620 ng, and the particle volume size distribution (PVSD) centered at about 400 nm (Fig. S6). Thus, the fitting parameters ( and a=3.732) of the calibration experiment with a diameter of 200 nm and mass loading of 407 ng were adopted in the C∗ calculation, since the mass loading and diameter are the closest to the ambient samples.
For gas-phase organic compounds (organic vapors), we first divided them into two groups based on their oxidation pathways (multi-generation OH oxidation and autoxidation; solid line in Fig. S7) and then used different parameters in their volatility estimation. The classification of pathways was based on the molecular characteristics of oxidation products of aromatics and monoterpene, respectively (Wang et al., 2020). In general, their saturation vapor concentration (C∗, at 300 K) can be estimated as follows:
where nc, nO, and nN are the numbers of carbon, oxygen, and nitrogen atoms in each compound. For oxidation products formed from the multi-generation OH oxidation (aging) pathway, the volatility parameters bC, bO, bCO, and bN were assumed to be 0.475, 2.3, −0.3, and 2.5, respectively (Donahue et al., 2011). For oxidation products formed from the autoxidation pathway, the modified parameterization is used, with bC=0.475, bO=0.2, bCO=0.9, and bN=2.5 (Bianchi et al., 2019). It should be noted that this method can only roughly distinguish the formation pathways of ambient organic vapors, since it is based on the oxidation products of specific species in a laboratory study.
2.3.2 Calculation of oxidation state () of CxHyOz and CxHyN1,2Oz compounds
For CxHyOz compounds, the can be estimated as
For CxHyN1,2Oz compounds, the can be calculated from the following equation:
where x is the valence state of N atoms, which is dependent on functional groups. Several assumptions were adopted to classify them:
-
N-containing functional groups were nitro (−NO2, ) or nitrate (−NO3, 5) in our measurement.
-
N-containing aromatics contain nitro moieties, while N-containing aliphatic hydrocarbons contain nitrate moieties.
-
N-containing aromatics have 6–9 carbon atoms and fewer hydrogen atoms than aliphatic hydrocarbons with the same number of carbon atoms.
2.3.3 Estimation of condensation sink
The condensation sink (CS) represents the condensing vapor captured by pre-existing particles and can be calculated from the following equation:
where D is the diffusion coefficient of the H2SO4 vapor ( m2 s−1); is the transitional regime correction factor, which can be calculated from the Knudsen number (Fuchs and Sutugin, 1971); and represents the particle number concentration at Dp.
2.3.4 Estimation of OA contributed by high-volatility organic vapors
Organic vapors with higher volatility (SVOC+IVOC+VOC, µg m−3) can easily reach an equilibrium between the gas and particle phase. Thus, the contribution of high-volatility organic vapors to OA concentration (OAHVgas) through gas–particle partitioning can be estimated as follows:
where Ci,g is the gas-phase concentration of species i. fi is the fraction of species i in the particle phase and is defined as
where COA is the concentration of OA measured by the SP-AMS, and is the saturation concentration of species i at temperature (T). The temperature-dependent was obtained by (Nie et al., 2022)
where ΔHvap,i is the enthalpy of vaporization and can be estimated based on .
2.3.5 Estimation of the production rate of RO2 and OH
A zero-dimensional box model (0-D Atmospheric Modeling, F0AM; Wolfe et al., 2016) based on the Master Chemical Mechanism (MCM v3.1.1, https://mcm.york.ac.uk/MCM, last access: September 2023) was used to simulate the production rate of OH in this study. The F0AM box model has been widely used in investigating chemical reactions of VOCs, NOx, and ROx radicals (including OH, HO2, and RO2) in field and laboratory research (Baublitz et al., 2023; Yang et al., 2022; D'Ambro et al., 2017). The simulation was constrained with the observation data of non-methane hydrocarbons (NMHCs), HCHO, CH3CHO, NO, CO, HONO, and meteorological parameters (RH, temperature, photolysis rates, and pressure). The background concentration of CH4 was set to 1.8 ppm (Wang et al., 2011). The simulation time step was set to 5 min. With respect to the integrity and temporal coverage of the observation data, the simulation period was from 16 October to 16 November 2019. Further details on model settings can be found in Yang et al. (2022).
The empirical kinetic modeling approach (EKMA) is applied to investigate the sensitivity of the production rate of RO2 and OH to the variation of NOx and VOCs. The base case was simulated based on the observation of average conditions. Sensitivity tests are performed by adjusting NOx or VOCs by a ratio ranging from 0.1 to 2.0 without changing other parameters.
3.1 Overview
Figure 1 shows the temporal profile of particle number size distribution (PNSD) and condensation sink (CS) during the measurement (Fig. 1a), one-dimensional thermograms and Tmax measured by the FIGAERO–CIMS (Fig. 1b), bulk PM1 chemical composition measured by the SP-AMS and PM1 concentration (Fig. 1c), deconvolved OA factors from the PMF analysis (Fig. 1d), and wind speed and direction (Fig. 1e). Note that all measurements started on 2 October 2019. As shown in Fig. 1a, new particle formation (NPF) events occurred frequently along with relatively low CS values during the measurement period (44.4 %, 20 out of 45 d). The Tmax mainly varied in two temperature ranges: 80–95 °C and 110–120 °C (Fig. 1b). The lower Tmax was usually accompanied by high desorption signals peaked at 80–95 °C (Fig. 1b), a higher fraction of LOOA (Fig. 1d), and an obvious wide accumulation mode in PNSD (Fig. 1a).
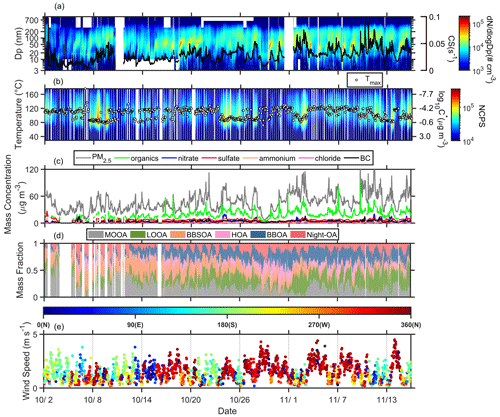
Figure 1Temporal profile of the measured variables during the campaign: (a) particle number size distribution and condensation sink (black line); (b) one-dimensional thermograms of organic compounds (ions containing C, H, and O atoms, referred to as sum thermogram) and the Tmax values (white dots) measured by the FIGAERO–CIMS; (c) bulk PM1 chemical composition measured by SP-AMS and PM1 concentration; (d) mass fraction of six OA factors from PMF analysis of SP-AMS data; and (e) wind speed and wind direction. The color in (b) represents the normalized count per second (ncps) of oxygenated organic compounds calculated based on total count per second (cps) of oxygenated organic compounds at all (total cps), 127 (cps127), and 145 (cps145) measured by the FIGAERO–I–CIMS, ncps. The OA factors included more oxygenated OA (MOOA), less oxygenated OA (LOOA), aged biomass-burning OA (BBSOA), hydrocarbon-like OA (HOA), biomass-burning OA (BBOA), and nighttime OA (night-OA).
The evening peak of hydrocarbon-like OA (HOA) and biomass-burning OA (BBOA) was related to local anthropogenic activities (e.g., biomass burning, cooking, and traffic; Fig. 2). The less oxygenated OA (LOOA) and biomass-burning SOA (BBSOA) showed afternoon peaks (Fig. 2), which could be attributed to secondary organic aerosol (SOA) formation through daytime photochemical reactions. LOOA showed a noticeable increase corresponding to the particle surface area (Fig. S8), while we did not observe such a correlation for other SOA factors (MOOA and BBSOA). Furthermore, LOOA exhibited a stronger positive correlation with organic vapors measured by the FIGAERO–CIMS compared to other OA factors (Fig. S9). These results suggested that the daytime formation of LOOA was attributed to gas–particle partitioning. The Ox (Ox=O3+NO2) had a strong a correlation with organic vapors in the afternoon (10:00–16:00 LT; Fig. S10), highlighting an important role of photochemical reaction in the formation of LOOA.
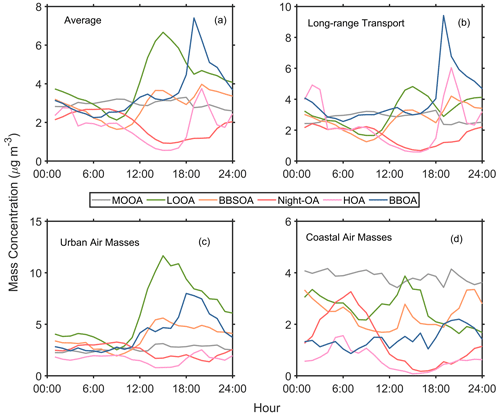
Figure 2Average diurnal variation of six OA PMF factors during (a) the whole campaign, (b) long-range transport, (c) urban air mass, and (d) coastal air mass periods.
The high desorption signal at a lower temperature range suggested that the volatility of OA could be higher, which could be associated with the formation of LOOA. Coincidently, either NPF events or a higher fraction of LOOA could only be observed during the period prevalent with north wind direction (Fig. 1e), when the measurement site was affected by the pollutant from the city cluster around the city of Guangzhou. It indicates that the urban pollutants might promote particle formation and growth and daytime SOA formation by increasing oxidants and acting as precursor gases. Xiao et al. (2023) suggested that fresh urban emissions could enhance NPF, while NPF was suppressed in aged urban plumes. Shrivastava et al. (2019) found that urban emissions, including NOx and oxidants, could significantly enhance the SOA formation in the Amazon rainforest. Three periods were classified based on the combination of wind direction and the analysis of backward trajectories to further investigate the impact of urban pollutants on this downwind site, which were long-range transport, urban air mass, and coastal air mass periods (Fig. S2 and Table S2). The long-range transport period was related to long-range transport masses from northeast inland. The urban air mass period was mainly affected by regional urban air masses from the PRD region. The coastal air mass period was associated with air masses from the South China Sea and the northeastern coast.
A significant daytime peak of LOOA (10.4 µg m−3) was shown during the urban air mass period (Fig. 2c), while the enhancement of BBSOA was inapparent. It suggests that the contribution of gas–particle reactions to SOA formation was enhanced when the site was affected by urban plumes. The Ox concentration in the afternoon during the urban air mass period was higher than that during the long-range transport period (Fig. S11), which might be able to explain the significant enhancement of LOOA for the urban air mass period. These results imply that urban pollution plumes could promote the formation of SOA in the downwind region by increasing the oxidant concentration.
3.2 The daytime formation of FIGAERO OA
As mentioned before, the increase of LOOA was usually along with the significant desorption signals measured by the FIGAERO–CIMS at a low temperature range (80–95 °C), suggesting that OA volatility could be higher. The average two-dimensional thermograms of all calibrated and semi-quantified species and an example of a one-dimensional thermogram of levoglucosan can be found in Fig. 3a and b, respectively. According to Eqs. (1) and (2), we calculated the C∗ value of all calibrated and semi-quantified species based on their Tmax and constructed the volatility distribution as a volatility basis set (VBS; Fig. 3c). The Tmax of each species is obtained based on their average thermogram. These 12 VBS bins were classified into 3 groups (Donahue et al., 2012): semi-volatile organic compounds (SVOCs, 0.3 < 3 × 102 µg m−3), less-volatile organic compounds (LVOCs, 0.3 µg m−3), and extremely low-volatility organic compounds (ELVOCs, µg m−3). In general, most species measured by the FIGAERO–CIMS fall into LVOC groups (Fig. S12). Note that the decomposition of organic compounds was ignored in this method, which could affect thermogram peaks in some cases and the measurement of low-volatility compounds (Wang and Hildebrandt Ruiz, 2018). Furthermore, the fraction of SVOC might be underestimated owing to its high volatility. As a result, fast evaporation could occur during the collection on the filter and the shift from sampling mode to desorption mode.
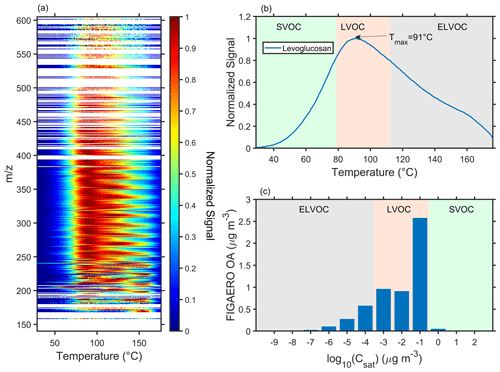
Figure 3The average (a) two-dimensional thermograms of all calibrated and semi-quantified species, (b) one-dimensional thermogram of levoglucosan, and (c) volatility distribution of all calibration and semi-quantified species in the particle phase measured by the FIGAERO–CIMS (referred as FIGAERO OA). The Tmax was converted to the C∗ according to Eqs. (1) and (2).
During the urban air mass period, the FIGAERO–CIMS measured significant signals at a desorption temperature range of SVOC and LVOC (Fig. S13) in the afternoon (12:00–16:00 LT), indicating that the OA volatility could be higher. The SVOC+LVOC in the FIGAERO OA increased from 5.2 µg m−3 (8:00 LT) to 16.29 µg m−3 (15:00 LT) during the urban air mass period (Fig. 4a), which was coincident with an enhancement of LOOA (Fig. 2c). It suggested that daytime enhancement of the SVOC+LVOC in the FIGAERO OA was closely related to the obvious LOOA formation. The FIGAERO OA during the urban air mass period was systemically higher than that during the long-range transport period, with a significantly higher concentration of LVOC group (Fig. 4b), especially the portion with a volatility log10C∗ of −1. Table 1 investigated the relationship between SVOC+LVOC and six OA factors. The SVOC+LVOC in FIGAERO OA had a significant positive correlation (R=0.72–0.85) with the LOOA, especially during the urban air mass period (R=0.85; Fig. S14 and Table 1), suggesting that the LOOA formation was mainly responsible for the increase of OA volatility.
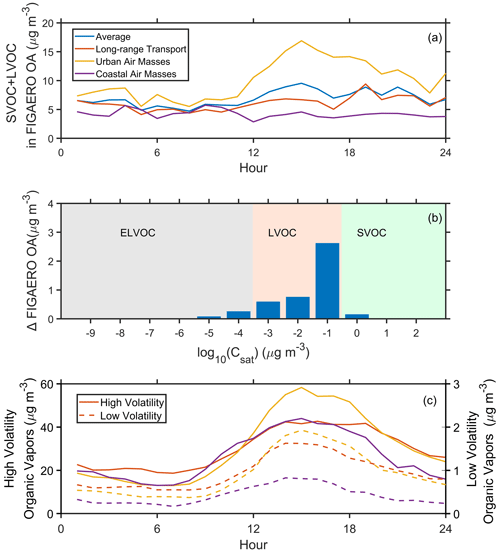
Figure 4Diurnal variation of (a) SVOC+LVOC in FIGAERO OA, (b) the difference of FIGAERO OA between the urban air mass and long-range transport periods, and (c) low-volatility organic vapors (ELVOC+LVOC; solid lines) and high-volatility organic vapors (SVOC+IVOC+VOC; dashed lines) during the whole campaign and three selected periods.
Table 1The correlation coefficient between SVOC+LVOC in FIGAERO OA and six OA factors in AMS OA during different periods.
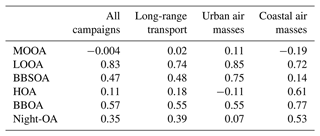
Interestingly, the high-volatility organic vapors (SVOC+IVOC+VOC, µg m−3) dramatically increased in the afternoon during the urban air mass period, while we did not observe such a phenomenon for low-volatility (ELVOC+LVOC, µg m−3) organic vapors (Fig. 4c). The concentration of low-volatility organic vapors in the afternoon (12:00–16:00 LT) did not show a significant difference (1.76 and 1.84 µg m−3) between the long-range transport and urban air mass periods, indicating that the irreversible condensation of low-volatility organic vapors could not fully explain the enhancement of LOOA during the urban air mass period (Wang et al., 2022). However, the high-volatility organic vapors had a notably higher concentration (51.69 µg m−3) during the urban air mass period than that (41.70 µg m−3) during the long-range transport period. This implies that the significant enhancement of LOOA during the urban air mass period might be mainly attributed to the equilibrium partitioning of high-volatility organic vapors, which could also increase the volatility of total OA.
Here we selected a typical day (2 November 2019) of the urban air mass period for further investigation. The measurement site was affected by the urban plume from the city cluster in the PRD region on this day (Fig. S15). A wide accumulation mode centered at about 180 nm in PNSD was observed, with a significant desorption signal measured by the FIGAERO–CIMS in the afternoon and weak north wind (Fig. S16). As shown in Fig. 5a, the desorption signals of organic compounds increased from 09:00 LT and reached their peak at 14:00 LT, suggesting a significant daytime SOA formation. The variation of OA volatility distribution and mean C∗ is shown in Fig. 5b. The showed an afternoon peak (0.021 µg m−3) at 15:00 LT, suggesting a higher OA volatility in the afternoon. An evident enhancement of OA with a volatility log10C∗ of −1 was observed in the afternoon, aligning with the formation of LOOA (Fig. 5c), which primarily contributes to higher OA volatility. Combined with the volatility distribution analysis in Fig. 4b, the positive correlation between LOOA and OA with a volatility log10C∗ of −1 indicated that the main components of LOOA have a volatility log10C∗ of −1. Interestingly, the Tmax value of the sum thermogram (Fig. 5a) increased from 81 °C at 09:00 LT to 96 °C at 17:00 LT, implying that the OA volatility decreased during the daytime owing to the daytime aging processes. However, the value consistently increased from 06:00 LT until 15:00 LT and then began to decrease, which was in conflict with the increasing Tmax. One possible reason is that species in the FIGAERO OA fell into a specific Tmax range (about 11 °C) and were categorized into different C∗ bins by a factor of 10. Thus, the slight variation of Tmax might not affect the estimated volatility distribution of FIGAERO OA. The other possible reason is that the volatility distribution of FIGAERO OA was estimated based on the Tmax value of calibrated and semi-quantified species, while the sum thermograms contained all organic compounds containing C, H, and O atoms. There could be some organic compounds formed through aging processes that were not included in the C∗ estimation.
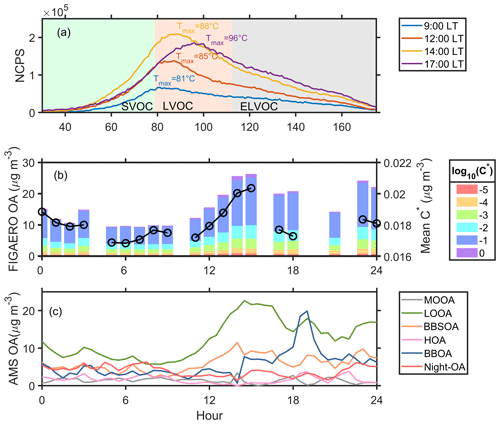
Figure 5(a) The sum thermograms at 9:00, 12:00, 14:00, and 17:00 LT; (b) variation of FIGAERO OA volatility presented in a volatility range from 10−5 to 100 µg m−3 and mean C∗; and (c) variation of six OA factors from PMF analysis on 2 November 2019. The mean is estimated as , where fi is the mass fraction of OA with a volatility .
3.3 The contribution of high-volatility organic vapors to SOA formations
In the previous section, we found that the significant enhancements in LOOA during the urban air mass period might be attributed to the high-volatility organic vapors through gas–particle partitioning. The contribution of high-volatility organic vapors to the OA concentration via equilibrium partitioning can be estimated based on Eq. (7). Our results show that the estimated contribution of high-volatility organic vapors (estimated OAHVgas) was higher (peaked at about 1.17 µg m−3) during the urban air mass period (Fig. 6a). Correspondingly, we observed an enhancement in the measured concentration of these species in the particle phase (measured OAHVgas, peaked at about 10.32 µg m−3; Fig. 6b). This implies that the increase in high-volatility organic vapors might significantly contribute to the daytime SOA formation during the urban air mass period. However, the estimated contribution was much lower than the measured value. It suggests that using the equilibrium equation might not be able to fully explain the increase of LOOA contributed by the high-volatility organic vapors during the urban air mass period. Nie et al. (2022) indicated that the estimation of OA contribution through the equilibrium equation can be easily disturbed by varied meteorological processes, which would lead to uncertainties in the calculations.
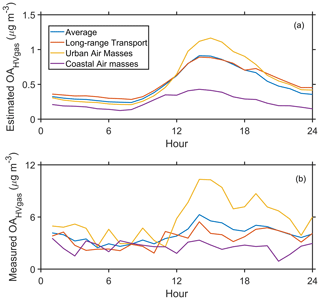
Figure 6The diurnal variation of (a) the estimated contribution of high-volatility organic vapors to the OA (estimated OAHVgas) and (b) the total concentration of corresponding species in the particle phase measured by the FIGAERO CIMS.
Moreover, the gas–particle equilibrium theory assumes that particles are droplets and that the high-volatility species in the particle phase could reach a reversible equilibrium with the gas-phase concentration. However, some studies indicate that this assumption significantly overestimates the volatility of these species in the particle phase and underestimates the contribution of high-volatility organic vapors to the SOA concentration (Kolesar et al., 2015; Cappa and Wilson, 2011). This is because particles might exist in a glassy state rather than a liquid state. This was consistent with the difference of the volatility distribution of these species between the particle and gas phase (Fig. 7a). The volatility in the particle phase was centered at a log10C∗ of −1, while that in the gas phase showed a higher concentration of log–8 µg m−3, implying that the volatility of these compounds in the particle phase could be lower than that in the gas phase.
Another possible explanation is that the corresponding species in the particle phase could be the decomposition products of low-volatility compounds, leading to a higher concentration than expected. We further investigate the difference between the measured and estimated concentration of different high-volatility species (Fig. 7b). The measured concentration was systematically higher than the estimated value. The higher measured concentration of C2H2O4I− could be due to the decomposition of low-volatility spices, as the desorption signal peaked at the ELVOC region (Fig. 7c). However, for higher-molecular-weight compounds, the corresponding Tmax values were in the LVOC region, suggesting that these species might not be the decomposition products. This implies that the decomposition products might play a minor effect in the difference between the measured and estimated concentration.
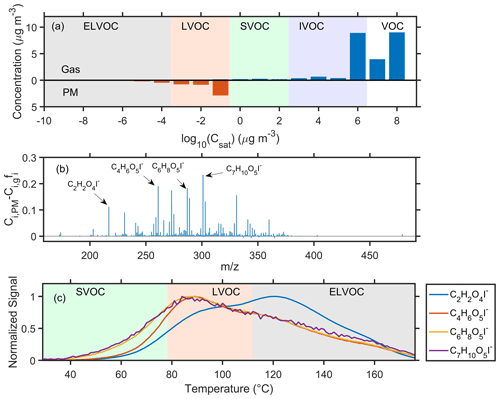
Figure 7(a) The average volatility distribution of high-volatility organic vapors in the gas phase and particle phase. (b) The average difference between the measured concentration in the particle phase (Ci,PM) and the estimated concentration (Ci,gfi) of different compounds in the high-volatility organic vapors. (c) The average thermograms of C2H2O4I−, C4H6O5I−, C6H8O5I−, and C7H10O5I−.
Taken together, these results suggest the increase in high-volatility organic vapors could promote the daytime enhancement of SOA during urban air mass periods. However, this contribution might be underestimated using gas–particle equilibrium theory, since the volatility of organic aerosol may differ significantly from the volatility determined by the equilibrium theory.
3.4 Enhancement of SOA formation by urban pollutants
As mentioned before, the significant enhancement of high-volatility organic vapors was observed during the urban air mass period. Figure 8 compares the difference in organic vapors in the carbon oxidation state () in the afternoon (12:00–16:00 LT) between the long-range transport and urban air mass periods. A higher concentration of organic vapors with a low () was observed during the urban air mass period, while this trend overturned for high () organic vapors. This suggests that the oxidation degree of organic vapors was lower during the urban air mass period, even though the Ox concentration was higher (Fig. S11). This trend was more significant for compounds with carbon numbers between 2 and 5, indicating a higher concentration of small molecules with low during the urban air mass period. The of major C5 compounds was about −1.33, which was mainly contributed by C5H8NOI−, highlighting the role of NOx chemistry. The oxygenated organic vapor production rates depend on oxidant and precursor concentration, and the mechanism of significant enhancement of high-volatility organic vapors remains unclear. We speculated that it could be partly attributed to the elevated NOx concentration in the afternoon during the urban air mass period (Fig. S17). NOx was found to have a detrimental effect on the production of highly oxidized products and thus on the formation of low-volatility vapors (Rissanen, 2018), which might be responsible for the smooth increase of low-volatility organic vapors. Previous studies have found that the increase of NOx could lead to higher OH production, which would offset decreases in the autoxidation efficiency and further result in enhanced SOA formation (Liu et al., 2021; Pye et al., 2019). During the urban air mass period, both low-volatility and high-volatility CHON compounds increased in the afternoon, implying the effect of NOx on the photochemical reactions (Fig. S18a and b). That was further evidenced by the higher fraction of CHON compounds in the FIGAERO OA (Fig. S18f). This result was consistent with Schwantes et al. (2019), who reported that low-volatility organic nitrates might have a significant contribution to SOA under high NOx conditions. Interestingly, in contrast with the higher fraction of low-volatility CHON compounds in the afternoon, the fraction of high-volatility CHON compounds was lower at the same time (Fig. S18d and e), indicating that the effect of high NOx concentration on photochemical oxidation goes beyond the formation of CHON compounds for high-volatility species.
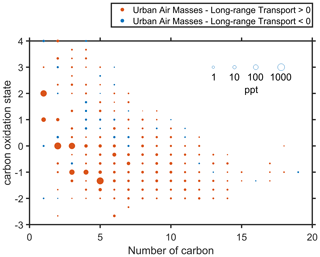
Figure 8Difference in the carbon oxidation state () in the gas phase in the afternoon (12:00–16:00 LT) between the long-range transport and urban air mass periods. The symbol sizes are proportional to the logarithm of concentration. The symbol colors represent that the concentration during the urban air mass period was higher (red) or lower (blue) than that during the long-range transport period.
To further understand how the urban plumes affect the SOA formation, we used an observation-constrained box model to simulate the production rate of organic peroxy radicals (RO2) and OH with different NOx and VOC concentrations (Fig. 9). The detailed description of the box model is described in Sect. 2.3.4. In general, the production rates of OH (P(OH)) were close to the transition regime during three selected periods (Fig. 9a), where the P(OH) is sensitive to both VOC and NOx variation. Further, the P(OH) tended to be in the NOx-limited regime during the coastal air mass period. The emission of NOx might enhance the atmospheric oxidation capacity, consistent with the results from other observations (Shrivastava et al., 2019; Pye et al., 2019). Interestingly, the sensitivity regime of P(OH) changed to the VOCs limited during the urban air mass period, suggesting that the production of OH would be suppressed with continued increases in NOx. During the urban air mass period, the concentration of NOx and VOCs was noticeably increased compared to the coastal air mass period, leading to a significant increase of P(OH).
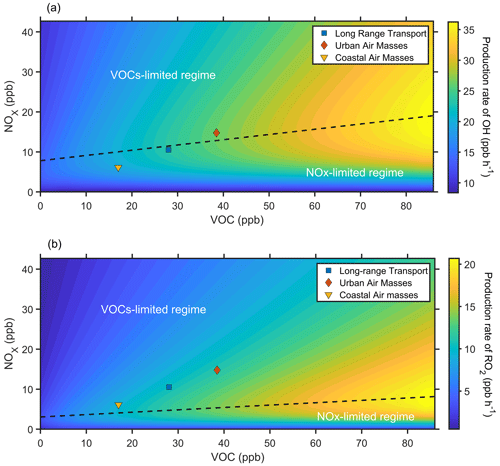
Figure 9The simulated production rate of OH (a) and RO2 (b) with NOx and VOC concentration predicted by an observation-constrained box model under campaign average conditions. The blue square, red diamond, and yellow triangle represent the average conditions during the long-range transport, urban air mass, and coastal air mass periods, respectively.
Recent studies show that autoxidation of RO2 can result in highly oxygenated molecules (O:C ≥ 0.7) and promote SOA formation (Pye et al., 2019, 2015). In general, the production rate of RO2 (P(RO2)) was in the VOC-limited regime during three selected periods (Fig. 9b), where the P(RO2) increased with the increase of VOCs. It suggests that the production of RO2 was suppressed with the increase in NOx. During the urban air mass period, the concentration of VOCs was noticeably increased compared to the coastal air mass period, leading to a significant increase of P(RO2). The model results indicate that urban pollutants, including NOx and VOCs, could enhance the oxidizing capacity, while the increase of VOCs was mainly responsible for significant daytime SOA formation.
In this study, we demonstrated that daytime SOA formation could be enhanced when the rural site was affected by pollutants from the city region, which could be partly attributed to the high concentration of oxidants in urban pollution. A higher volatility of OA was observed during the urban air mass period, which was mainly contributed by the component with a volatility log10C∗ of −1. The significant increase of SVOC+LVOC in FIGAERO OA in the afternoon was associated with enhanced LOOA formation. Similar to other measurements, the daytime formation of LOOA was mainly through gas–particle partitioning of organic vapors, supported by a significant positive relationship between the LOOA and organic vapors. We observed a dramatic increase in the high-volatility organic vapors in the afternoon during the urban air mass period, while low-volatility organic vapors did not exhibit a similar growth trend. This indicated that the rapid increase of LOOA during the urban air mass period was mainly contributed by the gas–particle partitioning of high-volatility organic vapors. However, this contribution was underestimated using equilibrium theory, since the the volatility of “high-volatility” organic vapors in the particle phase was significantly lower than that in the gas phase.
The high NOx might also suppress the formation of highly oxidized products. Thus, the elevated NOx in the urban plume might be able to explain the smooth increase in low-volatility organic vapors and a higher concentration of organic vapors with a low . A box model simulation showed that the P(OH) was close to the transition regime during three selected periods, indicating that the elevated NOx and VOCs in urban plumes can increase the oxidizing capacity. However, the P(RO2) was in the VOC-limited regime, suggesting that the increase in VOCs was mainly responsible for the daytime enhancement of SOA. Further investigations on the effect of urban pollutants on SOA formation on the regional scale are still needed for formulating air pollution control strategies.
Data from the measurements are available at https://doi.org/10.6084/m9.figshare.25376059 (Cai, 2024).
The supplement related to this article is available online at: https://doi.org/10.5194/acp-24-13065-2024-supplement.
MC, YC, and BY designed the research. MC, YC, BY, SH, EZ, ZW, YL, TL, WH, WC, QS, WL, YP, BL, QS, and JZ performed the measurements. MC, YC, BY, SH, EZ, SY, ZW, YL, TL, WH, WC, QS, WL, YP, BL, QS, and JZ analyzed the data. MC, YC, and BY wrote the paper with contributions from all co-authors.
The contact author has declared that none of the authors has any competing interests.
Publisher's note: Copernicus Publications remains neutral with regard to jurisdictional claims made in the text, published maps, institutional affiliations, or any other geographical representation in this paper. While Copernicus Publications makes every effort to include appropriate place names, the final responsibility lies with the authors.
Additional support from the crew of the Heshan supersite and Guangdong Environmental Monitoring Center is greatly acknowledged.
This work was supported by the National Key Research and Development Program of China (grant no. 2023YFC3706200), National Natural Science Foundation of China (grant nos. 42305123, 42275103, 42121004), Guangdong Basic and Applied Basic Research Foundation (grant nos. 2023A1515012240, 2024A1515030221), the Research Fund Program of Guangdong-Hong Kong-Macau Joint Laboratory of Collaborative Innovation for Environmental Quality (grant no.GHML2022-005).
This paper was edited by Quanfu He and reviewed by four anonymous referees.
Apte, J. S., Brauer, M., Cohen, A. J., Ezzati, M., and Pope, C. A., III: Ambient PM2.5 Reduces Global and Regional Life Expectancy, Environ. Sci. Technol. Lett., 5, 546–551, https://doi.org/10.1021/acs.estlett.8b00360, 2018.
Arias, P. A., Bellouin, N., Coppola, E., Jones, R. G., Krinner, G., Marotzke, J., Naik, V., Palmer, M. D., Plattner, G. K., Rogelj, J., Rojas, M., Sillmann, J., Storelvmo, T., Thorne, P. W., Trewin, B., Achuta Rao, K., Adhikary, B., Allan, R. P., Armour, K., Bala, G., Barimalala, R., Berger, S., Canadell, J. G., Cassou, C., Cherchi, A., Collins, W., Collins, W. D., Connors, S. L., Corti, S., Cruz, F., Dentener, F. J., Dereczynski, C., Di Luca, A., Diongue Niang, A., Doblas-Reyes, F. J., Dosio, A., Douville, H., Engelbrecht, F., Eyring, V., Fischer, E., Forster, P., Fox-Kemper, B., Fuglestvedt, J. S., Fyfe, J. C., Gillett, N. P., Goldfarb, L., Gorodetskaya, I., Gutierrez, J. M., Hamdi, R., Hawkins, E., Hewitt, H. T., Hope, P., Islam, A. S., Jones, C., Kaufman, D. S., Kopp, R. E., Kosaka, Y., Kossin, J., Krakovska, S., Lee, J. Y., Li, J., Mauritsen, T., Maycock, T. K., Meinshausen, M., Min, S. K., Monteiro, P. M. S., Ngo-Duc, T., Otto, F., Pinto, I., Pirani, A., Raghavan, K., Ranasinghe, R., Ruane, A. C., Ruiz, L., Sallée, J. B., Samset, B. H., Sathyendranath, S., Seneviratne, S. I., Sörensson, A. A., Szopa, S., Takayabu, I., Tréguier, A. M., van den Hurk, B., Vautard, R., von Schuckmann, K., Zaehle, S., Zhang, X., and Zickfeld, K.: Technical Summary, in: Climate Change 2021: The Physical Science Basis. Contribution of Working Group I to the Sixth Assessment Report of the Intergovernmental Panel on Climate Change, edited by: Masson-Delmotte, V., Zhai, P., Pirani, A., Connors, S. L., Péan, C., Berger, S., Caud, N., Chen, Y., Goldfarb, L., Gomis, M. I., Huang, M., Leitzell, K., Lonnoy, E., Matthews, J. B. R., Maycock, T. K., Waterfield, T., Yelekçi, O., Yu, R., and Zhou, B., Cambridge University Press, Cambridge, United Kingdom and New York, NY, USA, 33−-144, https://doi.org/10.1017/9781009157896.002, 2021.
Baublitz, C. B., Fiore, A. M., Ludwig, S. M., Nicely, J. M., Wolfe, G. M., Murray, L. T., Commane, R., Prather, M. J., Anderson, D. C., Correa, G., Duncan, B. N., Follette-Cook, M., Westervelt, D. M., Bourgeois, I., Brune, W. H., Bui, T. P., DiGangi, J. P., Diskin, G. S., Hall, S. R., McKain, K., Miller, D. O., Peischl, J., Thames, A. B., Thompson, C. R., Ullmann, K., and Wofsy, S. C.: An observation-based, reduced-form model for oxidation in the remote marine troposphere, P. Natl. Acad. Sci. USA, 120, e2209735120, https://doi.org/10.1073/pnas.2209735120, 2023.
Bertrand, A., Stefenelli, G., Jen, C. N., Pieber, S. M., Bruns, E. A., Ni, H., Temime-Roussel, B., Slowik, J. G., Goldstein, A. H., El Haddad, I., Baltensperger, U., Prévôt, A. S. H., Wortham, H., and Marchand, N.: Evolution of the chemical fingerprint of biomass burning organic aerosol during aging, Atmos. Chem. Phys., 18, 7607–7624, https://doi.org/10.5194/acp-18-7607-2018, 2018.
Bianchi, F., Kurtén, T., Riva, M., Mohr, C., Rissanen, M. P., Roldin, P., Berndt, T., Crounse, J. D., Wennberg, P. O., Mentel, T. F., Wildt, J., Junninen, H., Jokinen, T., Kulmala, M., Worsnop, D. R., Thornton, J. A., Donahue, N., Kjaergaard, H. G., and Ehn, M.: Highly Oxygenated Organic Molecules (HOM) from Gas-Phase Autoxidation Involving Peroxy Radicals: A Key Contributor to Atmospheric Aerosol, Chem. Rev., 119, 3472–3509, https://doi.org/10.1021/acs.chemrev.8b00395, 2019.
Buchholz, A., Ylisirniö, A., Huang, W., Mohr, C., Canagaratna, M., Worsnop, D. R., Schobesberger, S., and Virtanen, A.: Deconvolution of FIGAERO–CIMS thermal desorption profiles using positive matrix factorisation to identify chemical and physical processes during particle evaporation, Atmos. Chem. Phys., 20, 7693–7716, https://doi.org/10.5194/acp-20-7693-2020, 2020.
Cai, M.: Enhanced daytime secondary aerosol formation driven by gas-particle partitioning in downwind urban plumes, figshare [data set], https://doi.org/10.6084/m9.figshare.25376059.v1, 2024.
Cai, M., Liang, B., Sun, Q., Liu, L., Yuan, B., Shao, M., Huang, S., Peng, Y., Wang, Z., Tan, H., Li, F., Xu, H., Chen, D., and Zhao, J.: The important roles of surface tension and growth rate in the contribution of new particle formation (NPF) to cloud condensation nuclei (CCN) number concentration: evidence from field measurements in southern China, Atmos. Chem. Phys., 21, 8575–8592, https://doi.org/10.5194/acp-21-8575-2021, 2021.
Cai, M., Huang, S., Liang, B., Sun, Q., Liu, L., Yuan, B., Shao, M., Hu, W., Chen, W., Song, Q., Li, W., Peng, Y., Wang, Z., Chen, D., Tan, H., Xu, H., Li, F., Deng, X., Deng, T., Sun, J., and Zhao, J.: Measurement report: Distinct size dependence and diurnal variation in organic aerosol hygroscopicity, volatility, and cloud condensation nuclei activity at a rural site in the Pearl River Delta (PRD) region, China, Atmos. Chem. Phys., 22, 8117–8136, https://doi.org/10.5194/acp-22-8117-2022, 2022.
Cai, Y., Ye, C., Chen, W., Hu, W., Song, W., Peng, Y., Huang, S., Qi, J., Wang, S., Wang, C., Wu, C., Wang, Z., Wang, B., Huang, X., He, L., Gligorovski, S., Yuan, B., Shao, M., and Wang, X.: The important contribution of secondary formation and biomass burning to oxidized organic nitrogen (OON) in a polluted urban area: insights from in situ measurements of a chemical ionization mass spectrometer (CIMS), Atmos. Chem. Phys., 23, 8855–8877, https://doi.org/10.5194/acp-23-8855-2023, 2023.
Cappa, C. D. and Wilson, K. R.: Evolution of organic aerosol mass spectra upon heating: implications for OA phase and partitioning behavior, Atmos. Chem. Phys., 11, 1895–1911, https://doi.org/10.5194/acp-11-1895-2011, 2011.
Chen, Y., Takeuchi, M., Nah, T., Xu, L., Canagaratna, M. R., Stark, H., Baumann, K., Canonaco, F., Prévôt, A. S. H., Huey, L. G., Weber, R. J., and Ng, N. L.: Chemical characterization of secondary organic aerosol at a rural site in the southeastern US: insights from simultaneous high-resolution time-of-flight aerosol mass spectrometer (HR-ToF-AMS) and FIGAERO chemical ionization mass spectrometer (CIMS) measurements, Atmos. Chem. Phys., 20, 8421–8440, https://doi.org/10.5194/acp-20-8421-2020, 2020.
D'Ambro, E. L., Møller, K. H., Lopez-Hilfiker, F. D., Schobesberger, S., Liu, J., Shilling, J. E., Lee, B. H., Kjaergaard, H. G., and Thornton, J. A.: Isomerization of Second-Generation Isoprene Peroxy Radicals: Epoxide Formation and Implications for Secondary Organic Aerosol Yields, Environ. Sci. Technol., 51, 4978–4987, https://doi.org/10.1021/acs.est.7b00460, 2017.
de Sá, S. S., Palm, B. B., Campuzano-Jost, P., Day, D. A., Hu, W., Isaacman-VanWertz, G., Yee, L. D., Brito, J., Carbone, S., Ribeiro, I. O., Cirino, G. G., Liu, Y., Thalman, R., Sedlacek, A., Funk, A., Schumacher, C., Shilling, J. E., Schneider, J., Artaxo, P., Goldstein, A. H., Souza, R. A. F., Wang, J., McKinney, K. A., Barbosa, H., Alexander, M. L., Jimenez, J. L., and Martin, S. T.: Urban influence on the concentration and composition of submicron particulate matter in central Amazonia, Atmos. Chem. Phys., 18, 12185–12206, https://doi.org/10.5194/acp-18-12185-2018, 2018.
Donahue, N. M., Epstein, S. A., Pandis, S. N., and Robinson, A. L.: A two-dimensional volatility basis set: 1. organic-aerosol mixing thermodynamics, Atmos. Chem. Phys., 11, 3303–3318, https://doi.org/10.5194/acp-11-3303-2011, 2011.
Donahue, N. M., Kroll, J. H., Pandis, S. N., and Robinson, A. L.: A two-dimensional volatility basis set – Part 2: Diagnostics of organic-aerosol evolution, Atmos. Chem. Phys., 12, 615–634, https://doi.org/10.5194/acp-12-615-2012, 2012.
Dong, H.-B., Zeng, L.-M., Hu, M., Wu, Y.-S., Zhang, Y.-H., Slanina, J., Zheng, M., Wang, Z.-F., and Jansen, R.: Technical Note: The application of an improved gas and aerosol collector for ambient air pollutants in China, Atmos. Chem. Phys., 12, 10519–10533, https://doi.org/10.5194/acp-12-10519-2012, 2012.
Ervens, B., Turpin, B. J., and Weber, R. J.: Secondary organic aerosol formation in cloud droplets and aqueous particles (aqSOA): a review of laboratory, field and model studies, Atmos. Chem. Phys., 11, 11069–11102, https://doi.org/10.5194/acp-11-11069-2011, 2011.
Fry, J. L., Brown, S. S., Middlebrook, A. M., Edwards, P. M., Campuzano-Jost, P., Day, D. A., Jimenez, J. L., Allen, H. M., Ryerson, T. B., Pollack, I., Graus, M., Warneke, C., de Gouw, J. A., Brock, C. A., Gilman, J., Lerner, B. M., Dubé, W. P., Liao, J., and Welti, A.: Secondary organic aerosol (SOA) yields from NO3 radical + isoprene based on nighttime aircraft power plant plume transects, Atmos. Chem. Phys., 18, 11663–11682, https://doi.org/10.5194/acp-18-11663-2018, 2018.
Fuchs, N. and Sutugin, A.: High Dispersed Aerosols, Topics in Current Aerosol Research, in: International Reviews in Aerosol Physics and Chemistry, Pergamon Press Oxford, 5–60 pp., 1971.
Hallquist, M., Wenger, J. C., Baltensperger, U., Rudich, Y., Simpson, D., Claeys, M., Dommen, J., Donahue, N. M., George, C., Goldstein, A. H., Hamilton, J. F., Herrmann, H., Hoffmann, T., Iinuma, Y., Jang, M., Jenkin, M. E., Jimenez, J. L., Kiendler-Scharr, A., Maenhaut, W., McFiggans, G., Mentel, Th. F., Monod, A., Prévôt, A. S. H., Seinfeld, J. H., Surratt, J. D., Szmigielski, R., and Wildt, J.: The formation, properties and impact of secondary organic aerosol: current and emerging issues, Atmos. Chem. Phys., 9, 5155–5236, https://doi.org/10.5194/acp-9-5155-2009, 2009.
Hodzic, A., Jimenez, J. L., Madronich, S., Canagaratna, M. R., DeCarlo, P. F., Kleinman, L., and Fast, J.: Modeling organic aerosols in a megacity: potential contribution of semi-volatile and intermediate volatility primary organic compounds to secondary organic aerosol formation, Atmos. Chem. Phys., 10, 5491–5514, https://doi.org/10.5194/acp-10-5491-2010, 2010.
Huang, R.-J., Zhang, Y., Bozzetti, C., Ho, K.-F., Cao, J.-J., Han, Y., Daellenbach, K. R., Slowik, J. G., Platt, S. M., Canonaco, F., Zotter, P., Wolf, R., Pieber, S. M., Bruns, E. A., Crippa, M., Ciarelli, G., Piazzalunga, A., Schwikowski, M., Abbaszade, G., Schnelle-Kreis, J., Zimmermann, R., An, Z., Szidat, S., Baltensperger, U., Haddad, I. E., and Prevot, A. S. H.: High secondary aerosol contribution to particulate pollution during haze events in China, Nature, 514, 218–222, https://doi.org/10.1038/nature13774, 2014.
Huang, W., Saathoff, H., Pajunoja, A., Shen, X., Naumann, K.-H., Wagner, R., Virtanen, A., Leisner, T., and Mohr, C.: α-Pinene secondary organic aerosol at low temperature: chemical composition and implications for particle viscosity, Atmos. Chem. Phys., 18, 2883–2898, https://doi.org/10.5194/acp-18-2883-2018, 2018.
Iyer, S., Lopez-Hilfiker, F., Lee, B. H., Thornton, J. A., and Kurtén, T.: Modeling the Detection of Organic and Inorganic Compounds Using Iodide-Based Chemical Ionization, The J. Phys. Chem. A, 120, 576–587, https://doi.org/10.1021/acs.jpca.5b09837, 2016.
Kolesar, K. R., Li, Z., Wilson, K. R., and Cappa, C. D.: Heating-Induced Evaporation of Nine Different Secondary Organic Aerosol Types, Environ. Sci. Technol., 49, 12242–12252, https://doi.org/10.1021/acs.est.5b03038, 2015.
Kuang, Y., Huang, S., Xue, B., Luo, B., Song, Q., Chen, W., Hu, W., Li, W., Zhao, P., Cai, M., Peng, Y., Qi, J., Li, T., Wang, S., Chen, D., Yue, D., Yuan, B., and Shao, M.: Contrasting effects of secondary organic aerosol formations on organic aerosol hygroscopicity, Atmos. Chem. Phys., 21, 10375–10391, https://doi.org/10.5194/acp-21-10375-2021, 2021.
Lanzafame, G. M., Srivastava, D., Favez, O., Bandowe, B. A. M., Shahpoury, P., Lammel, G., Bonnaire, N., Alleman, L. Y., Couvidat, F., Bessagnet, B., and Albinet, A.: One-year measurements of secondary organic aerosol (SOA) markers in the Paris region (France): Concentrations, gas/particle partitioning and SOA source apportionment, Sci. Total Environ., 757, 143921, https://doi.org/10.1016/j.scitotenv.2020.143921, 2021.
Le Breton, M., Wang, Y., Hallquist, Å. M., Pathak, R. K., Zheng, J., Yang, Y., Shang, D., Glasius, M., Bannan, T. J., Liu, Q., Chan, C. K., Percival, C. J., Zhu, W., Lou, S., Topping, D., Wang, Y., Yu, J., Lu, K., Guo, S., Hu, M., and Hallquist, M.: Online gas- and particle-phase measurements of organosulfates, organosulfonates and nitrooxy organosulfates in Beijing utilizing a FIGAERO ToF-CIMS, Atmos. Chem. Phys., 18, 10355–10371, https://doi.org/10.5194/acp-18-10355-2018, 2018.
Lee, B. H., Kostenidou, E., Hildebrandt, L., Riipinen, I., Engelhart, G. J., Mohr, C., DeCarlo, P. F., Mihalopoulos, N., Prevot, A. S. H., Baltensperger, U., and Pandis, S. N.: Measurement of the ambient organic aerosol volatility distribution: application during the Finokalia Aerosol Measurement Experiment (FAME-2008), Atmos. Chem. Phys., 10, 12149–12160, https://doi.org/10.5194/acp-10-12149-2010, 2010.
Liu, Y., Seco, R., Kim, S., Guenther, A. B., Goldstein, A. H., Keutsch, F. N., Springston, S. R., Watson, T. B., Artaxo, P., Souza, R. A. F., McKinney, K. A., and Martin, S. T.: Isoprene photo-oxidation products quantify the effect of pollution on hydroxyl radicals over Amazonia, Sci. Adv., 4, eaar2547, https://doi.org/10.1126/sciadv.aar2547, 2018.
Liu, Y., Nie, W., Li, Y., Ge, D., Liu, C., Xu, Z., Chen, L., Wang, T., Wang, L., Sun, P., Qi, X., Wang, J., Xu, Z., Yuan, J., Yan, C., Zhang, Y., Huang, D., Wang, Z., Donahue, N. M., Worsnop, D., Chi, X., Ehn, M., and Ding, A.: Formation of condensable organic vapors from anthropogenic and biogenic volatile organic compounds (VOCs) is strongly perturbed by NOx in eastern China, Atmos. Chem. Phys., 21, 14789–14814, https://doi.org/10.5194/acp-21-14789-2021, 2021.
Lopez-Hilfiker, F. D., Iyer, S., Mohr, C., Lee, B. H., D'Ambro, E. L., Kurtén, T., and Thornton, J. A.: Constraining the sensitivity of iodide adduct chemical ionization mass spectrometry to multifunctional organic molecules using the collision limit and thermodynamic stability of iodide ion adducts, Atmos. Meas. Tech., 9, 1505–1512, https://doi.org/10.5194/amt-9-1505-2016, 2016.
Lopez-Hilfiker, F. D., Mohr, C., Ehn, M., Rubach, F., Kleist, E., Wildt, J., Mentel, Th. F., Lutz, A., Hallquist, M., Worsnop, D., and Thornton, J. A.: A novel method for online analysis of gas and particle composition: description and evaluation of a Filter Inlet for Gases and AEROsols (FIGAERO), Atmos. Meas. Tech., 7, 983–1001, https://doi.org/10.5194/amt-7-983-2014, 2014.
Luo, B., Kuang, Y., Huang, S., Song, Q., Hu, W., Li, W., Peng, Y., Chen, D., Yue, D., Yuan, B., and Shao, M.: Parameterizations of size distribution and refractive index of biomass burning organic aerosol with black carbon content, Atmos. Chem. Phys., 22, 12401–12415, https://doi.org/10.5194/acp-22-12401-2022, 2022.
Masoud, C. G., Li, Y., Wang, D. S., Katz, E. F., DeCarlo, P. F., Farmer, D. K., Vance, M. E., Shiraiwa, M., and Hildebrandt Ruiz, L.: Molecular composition and gas-particle partitioning of indoor cooking aerosol: Insights from a FIGAERO-CIMS and kinetic aerosol modeling, Aerosol Sci. Technol., 56, 1156–1173, https://doi.org/10.1080/02786826.2022.2133593, 2022.
Mohr, C., Thornton, J. A., Heitto, A., Lopez-Hilfiker, F. D., Lutz, A., Riipinen, I., Hong, J., Donahue, N. M., Hallquist, M., Petäjä, T., Kulmala, M., and Yli-Juuti, T.: Molecular identification of organic vapors driving atmospheric nanoparticle growth, Nat. Commun., 10, 4442, https://doi.org/10.1038/s41467-019-12473-2, 2019.
Mutzel, A., Poulain, L., Berndt, T., Iinuma, Y., Rodigast, M., Böge, O., Richters, S., Spindler, G., Sipilä, M., Jokinen, T., Kulmala, M., and Herrmann, H.: Highly Oxidized Multifunctional Organic Compounds Observed in Tropospheric Particles: A Field and Laboratory Study, Environ. Sci. Technol., 49, 7754–7761, https://doi.org/10.1021/acs.est.5b00885, 2015.
Ng, N. L., Canagaratna, M. R., Jimenez, J. L., Zhang, Q., Ulbrich, I. M., and Worsnop, D. R.: Real-Time Methods for Estimating Organic Component Mass Concentrations from Aerosol Mass Spectrometer Data, Environ. Sci. Technol., 45, 910–916, https://doi.org/10.1021/es102951k, 2011.
Nie, W., Yan, C., Huang, D. D., Wang, Z., Liu, Y., Qiao, X., Guo, Y., Tian, L., Zheng, P., Xu, Z., Li, Y., Xu, Z., Qi, X., Sun, P., Wang, J., Zheng, F., Li, X., Yin, R., Dallenbach, K. R., Bianchi, F., Petäjä, T., Zhang, Y., Wang, M., Schervish, M., Wang, S., Qiao, L., Wang, Q., Zhou, M., Wang, H., Yu, C., Yao, D., Guo, H., Ye, P., Lee, S., Li, Y. J., Liu, Y., Chi, X., Kerminen, V.-M., Ehn, M., Donahue, N. M., Wang, T., Huang, C., Kulmala, M., Worsnop, D., Jiang, J., and Ding, A.: Secondary organic aerosol formed by condensing anthropogenic vapours over China's megacities, Nat. Geosci., 15, 255–261, https://doi.org/10.1038/s41561-022-00922-5, 2022.
Peng, Z., Lee-Taylor, J., Orlando, J. J., Tyndall, G. S., and Jimenez, J. L.: Organic peroxy radical chemistry in oxidation flow reactors and environmental chambers and their atmospheric relevance, Atmos. Chem. Phys., 19, 813–834, https://doi.org/10.5194/acp-19-813-2019, 2019.
Philippin, S., Wiedensohler, A., and Stratmann, F.: Measurements of non-volatile fractions of pollution aerosols with an eight-tube volatility tandem differential mobility analyzer (VTDMA-8), J. Aerosol Sci., 35, 185–203, https://doi.org/10.1016/j.jaerosci.2003.07.004, 2004.
Praske, E., Otkjær, R. V., Crounse, J. D., Hethcox, J. C., Stoltz, B. M., Kjaergaard, H. G., and Wennberg, P. O.: Atmospheric autoxidation is increasingly important in urban and suburban North America, P. Natl. Acad. Sci. USA, 115, 64–69, https://doi.org/10.1073/pnas.1715540115, 2018.
Pye, H. O. T., Luecken, D. J., Xu, L., Boyd, C. M., Ng, N. L., Baker, K. R., Ayres, B. R., Bash, J. O., Baumann, K., Carter, W. P. L., Edgerton, E., Fry, J. L., Hutzell, W. T., Schwede, D. B., and Shepson, P. B.: Modeling the Current and Future Roles of Particulate Organic Nitrates in the Southeastern United States, Environ. Sci. Technol., 49, 14195–14203, https://doi.org/10.1021/acs.est.5b03738, 2015.
Pye, H. O. T., D'Ambro, E. L., Lee, B. H., Schobesberger, S., Takeuchi, M., Zhao, Y., Lopez-Hilfiker, F., Liu, J., Shilling, J. E., Xing, J., Mathur, R., Middlebrook, A. M., Liao, J., Welti, A., Graus, M., Warneke, C., de Gouw, J. A., Holloway, J. S., Ryerson, T. B., Pollack, I. B., and Thornton, J. A.: Anthropogenic enhancements to production of highly oxygenated molecules from autoxidation, P. Natl. Acad. Sci. USA, 116, 6641–6646, https://doi.org/10.1073/pnas.1810774116, 2019.
Ren, S., Yao, L., Wang, Y., Yang, G., Liu, Y., Li, Y., Lu, Y., Wang, L., and Wang, L.: Volatility parameterization of ambient organic aerosols at a rural site of the North China Plain, Atmos. Chem. Phys., 22, 9283–9297, https://doi.org/10.5194/acp-22-9283-2022, 2022.
Rissanen, M. P.: NO2 Suppression of Autoxidation–Inhibition of Gas-Phase Highly Oxidized Dimer Product Formation, ACS Earth Space Chem., 2, 1211–1219, https://doi.org/10.1021/acsearthspacechem.8b00123, 2018.
Saha, P. K., Khlystov, A., Yahya, K., Zhang, Y., Xu, L., Ng, N. L., Grieshop, A. P. J. A. C., and Physics: Quantifying the volatility of organic aerosol in the southeastern US, 17, 501–520, 2017.
Salvador, C. M. G., Tang, R., Priestley, M., Li, L., Tsiligiannis, E., Le Breton, M., Zhu, W., Zeng, L., Wang, H., Yu, Y., Hu, M., Guo, S., and Hallquist, M.: Ambient nitro-aromatic compounds – biomass burning versus secondary formation in rural China, Atmos. Chem. Phys., 21, 1389–1406, https://doi.org/10.5194/acp-21-1389-2021, 2021.
Schwantes, R. H., Charan, S. M., Bates, K. H., Huang, Y., Nguyen, T. B., Mai, H., Kong, W., Flagan, R. C., and Seinfeld, J. H.: Low-volatility compounds contribute significantly to isoprene secondary organic aerosol (SOA) under high-NOx conditions, Atmos. Chem. Phys., 19, 7255–7278, https://doi.org/10.5194/acp-19-7255-2019, 2019.
Shrivastava, M., Andreae, M. O., Artaxo, P., Barbosa, H. M. J., Berg, L. K., Brito, J., Ching, J., Easter, R. C., Fan, J., Fast, J. D., Feng, Z., Fuentes, J. D., Glasius, M., Goldstein, A. H., Alves, E. G., Gomes, H., Gu, D., Guenther, A., Jathar, S. H., Kim, S., Liu, Y., Lou, S., Martin, S. T., McNeill, V. F., Medeiros, A., de Sá, S. S., Shilling, J. E., Springston, S. R., Souza, R. A. F., Thornton, J. A., Isaacman-VanWertz, G., Yee, L. D., Ynoue, R., Zaveri, R. A., Zelenyuk, A., and Zhao, C.: Urban pollution greatly enhances formation of natural aerosols over the Amazon rainforest, Nat. Commun., 10, 1046, https://doi.org/10.1038/s41467-019-08909-4, 2019.
Wang, D. S. and Hildebrandt Ruiz, L.: Chlorine-initiated oxidation of n-alkanes under high-NOx conditions: insights into secondary organic aerosol composition and volatility using a FIGAERO–CIMS, Atmos. Chem. Phys., 18, 15535–15553, https://doi.org/10.5194/acp-18-15535-2018, 2018.
Wang, M., Chen, D., Xiao, M., Ye, Q., Stolzenburg, D., Hofbauer, V., Ye, P., Vogel, A. L., Mauldin, R. L., Amorim, A., Baccarini, A., Baumgartner, B., Brilke, S., Dada, L., Dias, A., Duplissy, J., Finkenzeller, H., Garmash, O., He, X.-C., Hoyle, C. R., Kim, C., Kvashnin, A., Lehtipalo, K., Fischer, L., Molteni, U., Petäjä, T., Pospisilova, V., Quéléver, L. L. J., Rissanen, M., Simon, M., Tauber, C., Tomé, A., Wagner, A. C., Weitz, L., Volkamer, R., Winkler, P. M., Kirkby, J., Worsnop, D. R., Kulmala, M., Baltensperger, U., Dommen, J., El-Haddad, I., and Donahue, N. M.: Photo-oxidation of Aromatic Hydrocarbons Produces Low-Volatility Organic Compounds, Environ. Sci. Technol., 54, 7911–7921, https://doi.org/10.1021/acs.est.0c02100, 2020.
Wang, Y., Zhang, Y., Hao, J., and Luo, M.: Seasonal and spatial variability of surface ozone over China: contributions from background and domestic pollution, Atmos. Chem. Phys., 11, 3511–3525, https://doi.org/10.5194/acp-11-3511-2011, 2011.
Wang, Y., Hu, M., Wang, Y., Zheng, J., Shang, D., Yang, Y., Liu, Y., Li, X., Tang, R., Zhu, W., Du, Z., Wu, Y., Guo, S., Wu, Z., Lou, S., Hallquist, M., and Yu, J. Z.: The formation of nitro-aromatic compounds under high NOx and anthropogenic VOC conditions in urban Beijing, China, Atmos. Chem. Phys., 19, 7649–7665, https://doi.org/10.5194/acp-19-7649-2019, 2019.
Wang, Y., Clusius, P., Yan, C., Dällenbach, K., Yin, R., Wang, M., He, X.-C., Chu, B., Lu, Y., Dada, L., Kangasluoma, J., Rantala, P., Deng, C., Lin, Z., Wang, W., Yao, L., Fan, X., Du, W., Cai, J., Heikkinen, L., Tham, Y. J., Zha, Q., Ling, Z., Junninen, H., Petäjä, T., Ge, M., Wang, Y., He, H., Worsnop, D. R., Kerminen, V.-M., Bianchi, F., Wang, L., Jiang, J., Liu, Y., Boy, M., Ehn, M., Donahue, N. M., and Kulmala, M.: Molecular Composition of Oxygenated Organic Molecules and Their Contributions to Organic Aerosol in Beijing, Environ. Sci. Technol., 56, 770–778, https://doi.org/10.1021/acs.est.1c05191, 2022.
Wolfe, G. M., Marvin, M. R., Roberts, S. J., Travis, K. R., and Liao, J.: The Framework for 0-D Atmospheric Modeling (F0AM) v3.1, Geosci. Model Dev., 9, 3309–3319, https://doi.org/10.5194/gmd-9-3309-2016, 2016.
Xiao, Q., Zhang, J., Wang, Y., Ziemba, L. D., Crosbie, E., Winstead, E. L., Robinson, C. E., DiGangi, J. P., Diskin, G. S., Reid, J. S., Schmidt, K. S., Sorooshian, A., Hilario, M. R. A., Woods, S., Lawson, P., Stamnes, S. A., and Wang, J.: New particle formation in the tropical free troposphere during CAMP2Ex: statistics and impact of emission sources, convective activity, and synoptic conditions, Atmos. Chem. Phys., 23, 9853–9871, https://doi.org/10.5194/acp-23-9853-2023, 2023.
Yang, S., Yuan, B., Peng, Y., Huang, S., Chen, W., Hu, W., Pei, C., Zhou, J., Parrish, D. D., Wang, W., He, X., Cheng, C., Li, X.-B., Yang, X., Song, Y., Wang, H., Qi, J., Wang, B., Wang, C., Wang, C., Wang, Z., Li, T., Zheng, E., Wang, S., Wu, C., Cai, M., Ye, C., Song, W., Cheng, P., Chen, D., Wang, X., Zhang, Z., Wang, X., Zheng, J., and Shao, M.: The formation and mitigation of nitrate pollution: comparison between urban and suburban environments, Atmos. Chem. Phys., 22, 4539–4556, https://doi.org/10.5194/acp-22-4539-2022, 2022.
Ye, C., Yuan, B., Lin, Y., Wang, Z., Hu, W., Li, T., Chen, W., Wu, C., Wang, C., Huang, S., Qi, J., Wang, B., Wang, C., Song, W., Wang, X., Zheng, E., Krechmer, J. E., Ye, P., Zhang, Z., Wang, X., Worsnop, D. R., and Shao, M.: Chemical characterization of oxygenated organic compounds in the gas phase and particle phase using iodide CIMS with FIGAERO in urban air, Atmos. Chem. Phys., 21, 8455–8478, https://doi.org/10.5194/acp-21-8455-2021, 2021.
Ylisirniö, A., Buchholz, A., Mohr, C., Li, Z., Barreira, L., Lambe, A., Faiola, C., Kari, E., Yli-Juuti, T., Nizkorodov, S. A., Worsnop, D. R., Virtanen, A., and Schobesberger, S.: Composition and volatility of secondary organic aerosol (SOA) formed from oxidation of real tree emissions compared to simplified volatile organic compound (VOC) systems , Atmos. Chem. Phys., 20, 5629–5644, https://doi.org/10.5194/acp-20-5629-2020, 2020.
Zhang, Q., Zheng, Y., Tong, D., Shao, M., Wang, S., Zhang, Y., Xu, X., Wang, J., He, H., Liu, W., Ding, Y., Lei, Y., Li, J., Wang, Z., Zhang, X., Wang, Y., Cheng, J., Liu, Y., Shi, Q., Yan, L., Geng, G., Hong, C., Li, M., Liu, F., Zheng, B., Cao, J., Ding, A., Gao, J., Fu, Q., Huo, J., Liu, B., Liu, Z., Yang, F., He, K., and Hao, J.: Drivers of improved PM2.5 air quality in China from 2013 to 2017, P. Natl. Acad. Sci. USA, 116, 24463–24469, https://doi.org/10.1073/pnas.1907956116, 2019.