the Creative Commons Attribution 4.0 License.
the Creative Commons Attribution 4.0 License.
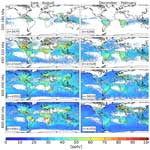
Vertical profiles of global tropospheric nitrogen dioxide (NO2) obtained by cloud slicing the TROPOspheric Monitoring Instrument (TROPOMI)
Rebekah P. Horner
Robert G. Ryan
Viral Shah
Routine observations of the vertical distribution of tropospheric nitrogen oxides (NOx ≡ NO + NO2) are severely lacking, despite the large influence of NOx on climate, air quality, and atmospheric oxidants. Here, we derive vertical profiles of global seasonal mean tropospheric NO2 by applying the cloud-slicing method to TROPOspheric Monitoring Instrument (TROPOMI) columns of NO2 retrieved above optically thick clouds. The resultant NO2 is provided at a horizontal resolution of 1° × 1° for multiple years (June 2018 to May 2022), covering five layers of the troposphere: two layers in the upper troposphere (180–320 hPa and 320–450 hPa), two layers in the middle troposphere (450–600 hPa and 600–800 hPa), and the marine boundary layer (800 hPa to the Earth's surface). NO2 in the terrestrial boundary layer is obtained as the difference between TROPOMI tropospheric columns and the integrated column of cloud-sliced NO2 in all layers above the boundary layer. Cloud-sliced NO2 typically ranges from 20–60 pptv throughout the free troposphere, and spatial coverage ranges from > 60 % in the mid-troposphere to < 20 % in the upper troposphere and boundary layer. When both datasets are abundant and sampling coverage is commensurate, our product is similar (within 10–15 pptv) to NO2 data from NASA DC-8 aircraft campaigns. However, such instances are rare. We use cloud-sliced NO2 to critique current knowledge of the vertical distribution of global NO2, as simulated by the GEOS-Chem chemical transport model, which has been updated to include peroxypropionyl nitrate (PPN) and aerosol nitrate photolysis, liberating NO2 in the lower troposphere and mid-troposphere for aerosol nitrate photolysis and in the upper troposphere for PPN. Multiyear GEOS-Chem and cloud-sliced means are compared to mitigate the influence of interannual variability. We find that for cloud-sliced NO2, interannual variability is ∼ 10 pptv over remote areas and ∼ 25 pptv over areas influenced by lightning and surface sources. The model consistently underestimates NO2 across the remote marine troposphere by ∼ 15 pptv. At the northern midlatitudes, GEOS-Chem overestimates mid-tropospheric NO2 by 20–50 pptv as NOx production per lightning flash is parameterised to be almost double that of the rest of the world. There is a critical need for in situ NO2 measurements in the tropical terrestrial troposphere to evaluate cloud-sliced NO2 there. The model and cloud-sliced NO2 discrepancies identified here need to be investigated further to ensure confident use of models to understand and interpret factors affecting the global distribution of tropospheric NOx, ozone, and other oxidants.
- Article
(11635 KB) - Full-text XML
- BibTeX
- EndNote
In the troposphere, nitrogen oxides (NOx ≡ NO + NO2) influence the formation of tropospheric ozone (O3), a greenhouse gas, and the hydroxyl radical (OH), the main atmospheric oxidant (Atkinson, 2000; Bloss et al., 2005). Due to its influence on OH, NOx also indirectly affects the lifetime and abundance of the potent greenhouse gas methane (Wild et al., 2001) and non-methane volatile organic compounds that contribute to O3 and particulate matter pollution (Crutzen and Andreae, 1990; Karl et al., 2007; Marais et al., 2016). NOx is directly emitted from the high-temperature combustion of fossil fuels, from open and domestic burning of biomass, and from natural processes such as lightning and bacteria in soils (Dignon, 1992; Pickering et al., 1998; Jain et al., 2006; Vinken et al., 2014). NOx also enters the upper layers of the troposphere via downwelling from the stratosphere (Poulida et al., 1996). The distribution of NOx varies throughout the troposphere as a result of these sources and due to the recycling of NOx via oxidation, photolysis, and the thermal decomposition of gas- and aerosol-phase reservoirs of nitrogen (Chatfield, 1994; Moxim et al., 1996; Kotamarthi et al., 2001; Scharko et al., 2014). In the warm lower troposphere, where anthropogenic sources dominate, the lifetime of NOx is a few hours. This increases with altitude to several days in the cold, dry upper troposphere, where NOx is present mostly as NO (Travis et al., 2016), reservoir compounds dominate, and terminal loss of NOx via wet deposition in the form of nitric acid (HNO3) is limited (Jaeglé et al., 1998).
Knowledge of the vertical distribution of tropospheric NOx has been largely informed by in situ instruments on research and commercial aircraft (Crawford et al., 1996; Brenninkmeijer et al., 1999; Bradshaw et al., 2000; Emmons et al., 2000; Petzold et al., 2015; Stratmann et al., 2016). These aircraft campaigns are few in time and space. The instruments used to measure NO2 are also susceptible to interference from the decomposition of thermally unstable reservoir compounds of NOx (Bradshaw et al., 2000; Browne et al., 2011; Reed et al., 2016). This interference is most severe in the upper troposphere and in remote marine regions, where thermally labile NOx reservoir compounds are abundant and decomposition of these compounds is promoted by the warm instrument inlet (Murphy et al., 2004; Nault et al., 2015; Shah et al., 2023). Studies now supplement these measurements with calculated daytime NO2 concentrations as NO and NO2 can be assumed to be in a photochemical steady state (PSS) (Davis et al., 1993; Crawford et al., 1996).
Networks of ground-based remote sensing instruments, such as Multi-AXis Differential Optical Absorption Spectroscopy (MAX-DOAS) and direct-sun Pandora instruments, have expanded globally. Still, geographic coverage for both is mostly in the Northern Hemisphere (Verhoelst et al., 2021). For Pandora, only the total tropospheric column can be derived from measurements of the total atmospheric column (Pinardi et al., 2020). MAX-DOAS, under ideal conditions, can retrieve up to four independent layers in the troposphere, though the vertical extent at most sites excludes the upper troposphere (Tirpitz et al., 2021). Space-based remote sensing observations used to retrieve vertical column densities (VCDs) of tropospheric NO2 address the limited spatial sampling of commercial and research aircraft, as well as that of the Pandora and MAX-DOAS networks, by offering daily global coverage but providing only one piece of vertical information in the troposphere (Ryan et al., 2023). These satellite observations are also impacted by biases in modelled vertical profiles of NO2 required to retrieve VCDs (Verhoelst et al., 2021), particularly in the upper troposphere, where satellite observations are most sensitive to tropospheric NO2 (Boersma et al., 2004; Travis et al., 2016; Silvern et al., 2018; Shah et al., 2023).
Mixing ratios of NO2 in distinct layers of the troposphere can be retrieved using so-called cloud slicing. This technique targets partial columns (stratospheric + tropospheric) above clouds that are sufficiently optically thick, allowing UV–visible instruments to observe discrete layers in the troposphere. Cloud slicing was first applied by Ziemke et al. (2001) to O3 columns to derive seasonal multiyear mean upper-tropospheric O3 mixing ratios in the tropics. Cloud slicing has since been used to retrieve seasonal mean concentrations of NO2 from the Ozone Monitoring Instrument (OMI) in both the mid-troposphere (900–650 hPa, or 2–4 km) and upper troposphere (450–280 hPa, or 6–11 km) at 5° latitude × 8° longitude (500 km × 800 km), as well as at six pressure levels (centred at 280, 380, 500, 620, 720, and 820 hPa) at a resolution of 2° × 2° (Choi et al., 2014; Belmonte Rivas et al., 2015; Marais et al., 2018). The OMI cloud-sliced NO2 data provide useful information at very coarse scales (20° × 32°; seasonal) (Marais et al., 2018) but have been hindered by large data loss since 2007, when many satellite pixels became obscured by the row anomaly (Torres et al., 2018). More recently, the higher-spatial-resolution TROPOspheric Monitoring Instrument (TROPOMI) has been used to derive NO2 mixing ratios in the upper troposphere (450–180 hPa, or 6–12 km) at finer scales (1° × 1°, or ∼ 100 km) than were possible with OMI (Marais et al., 2021). Cloud-sliced NO2 from TROPOMI has so far only been derived for a single year as, previously, frequent updates to the retrieval led to inconsistencies in the TROPOMI NO2 VCDs used for cloud slicing. TROPOMI NO2 data have since been reprocessed to obtain a consistent data record starting in May 2018.
Evaluation of cloud-sliced NO2 data products is very limited as the coincidence of satellite observations and aircraft campaigns is rare. Choi et al. (2014) found that the NASA OMI mid-tropospheric product is similar to coincident research aircraft campaign observations (< 10 % difference), limited to Texas and the region of the Pacific Ocean west of North America. Marais et al. (2021) intercompared seasonal mean cloud-sliced upper-tropospheric NO2 from TROPOMI and the NASA OMI product to identify that TROPOMI background values routinely exceed OMI values by 12–26 pptv. Given these product disparities, an independent evaluation of cloud-sliced NO2 mixing ratios is crucial. Past (2006–2013) NASA DC-8 aircraft campaigns and the more recent (2016–2018) NASA DC-8 Atmospheric Tomography Mission (ATom) measurement campaign sampled the troposphere from close to the surface to the upper layers of the troposphere, offering the opportunity to evaluate cloud-sliced NO2 mixing ratios over the remote Pacific and Atlantic oceans (ATom) (Thompson et al., 2022); the Canadian Arctic during the Arctic Research of the Composition of the Troposphere from Aircraft and Satellites (ARCTAS) campaign (Jacob et al., 2010); the eastern US during the Intercontinental Chemical Transport Experiment – North America Phases A and B (INTEX-A and INTEX-B) (Singh et al., 2006, 2009) and during the Studies of Emissions and Atmospheric Composition, Clouds and Climate Coupling by Regional Surveys (SEAC4RS) (Toon et al., 2016) campaign; and the northern Pacific during INTEX-B.
Here, we derive a global dataset consisting of 4 years of seasonal multiyear mean concentrations of NO2 for five discrete vertical layers of the troposphere, from the planetary boundary layer to the upper troposphere. We evaluate our dataset against directly measured and calculated (PSS) NO2 from multiple NASA DC-8 aircraft campaigns and go on to use the cloud-sliced data to assess the current understanding of the global vertical distribution of tropospheric NOx, as simulated by the GEOS-Chem chemical transport model.
2.1 Cloud slicing TROPOMI NO2 columns
TROPOMI was launched in October 2017 aboard the Sentinel-5P satellite. TROPOMI's initial nadir spatial resolution of 7.2 km × 3.5 km was enhanced to 5.6 km × 3.5 km in August 2019 (Liu et al., 2021). The swath width is 2600 km, resulting in daily global coverage at an Equator crossing time of 13:30 local solar time (LST). To derive our cloud-sliced product, we use TROPOMI Level-2 swaths retrieved using a consistent algorithm (version 2.3.1). Data are available as the reprocessed Product Algorithm Laboratory (PAL) product from 1 June 2018 to 14 November 2021 (TROPOMI, 2018) and as the offline (OFFL) product from 14 November 2021 to 31 May 2022 (TROPOMI, 2021). The cloud-slicing approach was first applied to TROPOMI by Marais et al. (2021) to derive NO2 mixing ratios in the upper troposphere over a broad pressure range of 450 to 180 hPa. We apply this cloud-slicing approach, with updates detailed below, to the entire troposphere to derive vertical profiles of seasonal mean NO2 at the same 1° × 1° resolution as that used by Marais et al. (2021) for multiple years (2018–2022) across five pressure ranges: one in the boundary layer below 800 hPa (< ∼ 2 km), two in the mid-troposphere at 800–600 hPa (∼ 2–4 km) and 600–450 hPa (∼ 4–6 km), and two in the upper troposphere at 450–320 hPa (∼ 6–9 km) and 320–180 hPa (∼ 9–12 km).
The first application of cloud slicing to TROPOMI NO2 is described in detail in Marais et al. (2021). We mostly follow this approach. Pixels of individual swaths are filtered to isolate observations obtained above optically thick clouds (cloud radiance fraction > 0.7). These are binned by cloud-top pressures within the five targeted pressure ranges on a fixed 1° × 1° grid. The stratospheric component of the total VCDs is corrected for a 13 % underestimate in variance, as identified by Marais et al. (2021) from comparison with ground-based direct-sun-photometer Pandora measurements at the high-altitude (4.2 km) Mauna Loa site. The corrected stratospheric VCDs are multiplied by the reported stratospheric air mass factors (AMFs) to calculate stratospheric slant columns. The stratospheric slant columns are then subtracted from the total slant columns to estimate the tropospheric slant columns, which are converted to tropospheric VCDs using a geometric AMF. Only clusters of total above-cloud VCDs with a relatively uniform stratosphere are retained for cloud slicing. These are identified as clusters of 1° × 1° pixels, with the relative standard deviation of the stratospheric column being < 0.02. A uniform stratosphere ensures that variability in partial NO2 columns above optically thick clouds is dominated by variability in the troposphere. Cloud slicing also requires that each cluster has a representative range of cloud-top pressures (Choi et al., 2014). To ensure this is achieved, we remove clusters with cloud pressure ranges corresponding to < 60 % of the pressure range of each layer (for example, a 120 hPa threshold for the 800–600 hPa layer) and that have a large standard deviation (≥ 30 hPa), which is consistent with cloud slicing performed by Choi et al. (2014) and Marais et al. (2018, 2021).
Next, we regress cloud-top pressures against above-cloud NO2 VCDs for clusters with at least 10 satellite pixels. We replace the reduced-major-axis (RMA) regression fit originally used by Marais et al. (2021) with Theil regression as this reduces the influence from outliers and is better suited to data that are not always normally distributed (Theil, 1950; Sen, 1968). The regression slope (in molec. cm−2 hPa−1) is converted to NO2 volume mixing ratios (in pptv), as described in Eq. (5) of Choi et al. (2014). The updated Theil regression fit addresses the 12–26 pptv overestimate in background values of cloud-sliced upper-tropospheric NO2 identified by Marais et al. (2021) from comparison with the OMI upper-tropospheric product. It also negates the need for the large bias correction of the TROPOMI free-tropospheric NO2 column, which Marais et al. (2021) used to resolve an apparent overestimate in TROPOMI compared to free-tropospheric NO2 columns derived with measurements from Pandora and MAX-DOAS instruments at the high-altitude Izaña site. We also find that the outlier filter used by Marais et al. (2021) for cloud-sliced NO2 > 200 pptv is no longer needed as it has negligible impact on seasonal mean cloud-sliced NO2 when using our updated approach. As an initial assessment, we compare upper-tropospheric cloud-sliced NO2 from our updated cloud-sliced approach to that from Marais et al. (2021). To ensure a consistent comparison, we recompute our updated cloud-sliced NO2 to cover the same pressure range (450–180 hPa) and time period (June 2019 to May 2020) as those reported by Marais et al. (2021) and only compare 1° × 1° grids with five or more cloud-sliced data points in each data product.
The use of a geometric AMF to convert slant columns to vertical columns assumes the vertical distribution of NO2 within each layer is relatively constant. Belmonte Rivas et al. (2015) estimated that the difference between the geometric AMF and an AMF that accounts for surface reflectivity, the vertical NO2 profile, and atmospheric scattering is < 10 % in all layers, with the exception of the lowest layer in that work (770–870 hPa). In this lowest layer, equivalent to the top half of the boundary layer in our work, the difference in the AMFs is up to ∼ 30 %. The largest differences occur over land, where NOx emissions from sources such as urban traffic, industry, soils, and open burning of biomass cause an exponential increase in NO2 with pressure, unlike over the oceans, where the NO2 profile is relatively uniform (Schreier et al., 2015; Wang et al., 2019; Kang et al., 2021; Shah et al., 2023). Given the steep vertical gradient in NO2 in the terrestrial boundary layer, we instead derive NO2 mixing ratios for the lowest layer over terrestrial regions as the difference between seasonal mean cloud-free TROPOMI tropospheric NO2 columns and free-tropospheric columns obtained by integrating cloud-sliced NO2 over the four layers above the boundary layer (800–180 hPa). Integration is only performed if data are available in all four overlying layers.
Cloud fraction and cloud-top height data are from the improved Fast Retrieval Scheme for Clouds from the Oxygen A band (FRESCO) algorithm, called FRESCO-wide (Eskes and Eichmann, 2023). FRESCO-wide minimises the difference between measured and simulated spectra between 757–758, 760–761, and 765–770 nm and is named as such because the third spectral window is wider than the 765–766 nm window used in the previous FRESCO-S algorithm (Wang et al., 2008; Van Geffen et al., 2022). The cloud-top pressure retrieved with FRESCO-wide corresponds to an altitude ∼ 1 km lower than the physical cloud-top height as the cloud-top height retrieval assumes that clouds are uniform reflective boundaries (Choi et al., 2014; Loyola et al., 2018). Marais et al. (2021) showed that cloud-sliced NO2 is relatively insensitive to the choice of TROPOMI cloud product. Their use of the TROPOMI Retrieval of Cloud Information using Neural Networks Clouds-As-Layers (ROCINN-CAL) product yielded upper-tropospheric NO2 values that were only 4–9 pptv greater than those from the FRESCO-S product. The small difference results from an extratropical latitude-dependent divergence in cloud-top heights between the two products. The reprocessed TROPOMI NO2 product (v2.3.1) includes data from two cloud retrieval algorithms, FRESCO-wide and the O2–O2 cloud (O22CLD) product. FRESCO-wide is used here as we find that it yields greater data density than the O22CLD product, and differences in NO2 between the two products for coincident grids are small (< 10 %). As of August 2023, ROCINN-CAL had not been reprocessed to obtain a consistent record, so it is not used.
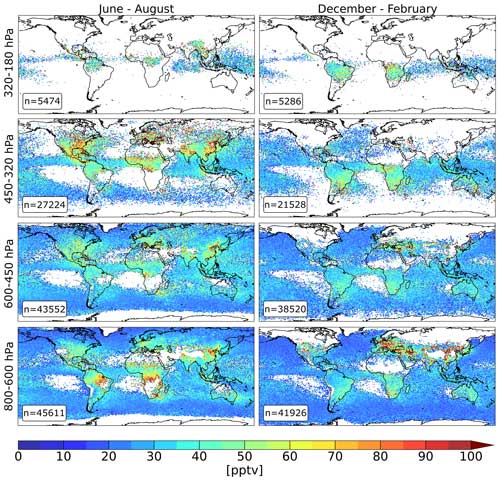
Figure 1Seasonal mean NO2 in the free troposphere obtained by cloud slicing TROPOMI. Columns are June–August (JJA; left) and December–February (DJF; right) multiyear means (2018–2021 for JJA and 2018–2022 for DJF) at 1° × 1°. Rows, from top to bottom, correspond to 320–180, 450–320, 600–450, and 800–600 hPa. Inset boxes each show the number of filled 1° × 1° grids. Data for the boundary layer (below 800 hPa) are shown in Fig. 2.
2.2 NASA DC-8 aircraft observations used to evaluate cloud-sliced NO2
We evaluate our cloud-sliced NO2 against NASA DC-8 campaign data. To mitigate interference from the decomposition of NOx reservoir compounds on measured NO2 over remote regions, we calculate PSS NO2 for ATom measurements obtained over remote oceans and for all measurements made in the upper troposphere. The PSS NO2 calculation assumes a dynamic daytime equilibrium between NO and NO2 resulting from the balance between photolysis of NO2 yielding NO and the reaction of NO with oxidants regenerating NO2. Silvern et al. (2018) estimated, using GEOS-Chem, that the oxidation of NO in the upper troposphere over the southeastern US was mostly (75 %) due to O3, followed by the hydroperoxy radical (HO2; 15 %). The remaining 10 % was due to oxidation by the methyl peroxy radical (CH3O2) and halogen monoxides. Given the dominance of O3 and HO2 and the availability of measurements of these for almost all campaigns used, we calculate PSS NO2 as follows:
where is the NO2 photolysis frequency (in s−1) and k is the rate constant for the oxidation of NO by O3 (k1) and by HO2 (k2) (in cm3 molec. −1 s−1). The square brackets denote concentrations of O3 and HO2 in molec. cm−3. NO and NO2 are expressed in pptv. Values of , NO, [O3], and [HO2] are from direct measurements, and k1 and k2 are calculated using the temperature-dependent Arrhenius equations documented in publication no. 19 of the Jet Propulsion Laboratory's Chemical Kinetics and Photochemical Data for Use in Atmospheric Studies (Burkholder et al., 2020). For cold upper-tropospheric temperatures (∼ 220 K), these values correspond to cm3 molec.−1 s−1 and cm3 molec.−1 s−1. Only aircraft data obtained between 12:00 and 15:00 LST, i.e. 1.5 h around the TROPOMI overpass time of 13:30 LST, are used to ensure consistent sampling of the midday atmosphere and that the PSS assumption is valid. We remove aircraft data influenced by stratospheric air, identified as > 1.25 mol mol−1. We also only use aircraft NO data to calculate PSS NO2 if the NO measured is double the NO instrument detection limit of 6 pptv. This ensures that the measurements used are distinct from background noise in our PSS calculation (Ryerson et al., 2000; Yang et al., 2023).
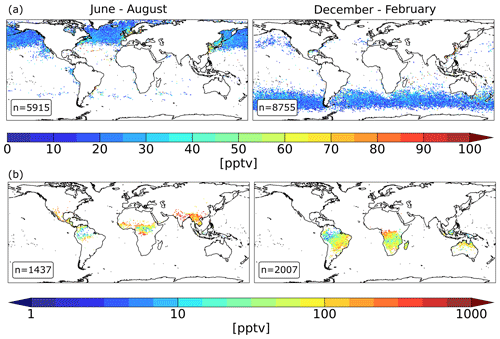
Figure 2As in Fig. 1 but for the boundary layer (below 800 hPa). Panels show NO2 derived using cloud slicing over oceans (a) and from the differencing approach over land (b) (see Sect. 2.1 for details). Note that the colour bar ranges differ in panels (a) and (b) and that panel (b) is illustrated on a log scale.
NASA DC-8 aircraft campaigns with direct observations of NO2 and observations needed to calculate PSS NO2 include INTEX-A for summer 2004 over the United States (INTEX-A Science Team, 2006); INTEX-B for spring 2006 over the eastern US, the Gulf of Mexico, and the northern Pacific Ocean (INTEX-B Science Team, 2011); ARCTAS for spring and summer 2008 over the Canadian Arctic (ARCTAS Science Team, 2011); SEAC4RS for summer and autumn 2013 over the southeastern US (SEAC4RS Science Team, 2014); and ATom, which took place once per season from 2016 to 2018, following the same pole-to-pole flight path over the Atlantic and Pacific oceans (ATom Science Team, 2021). Direct NO2 measurements are from thermal-dissociation laser-induced fluorescence (TD-LIF) (Di Carlo et al., 2013) for INTEX-A and INTEX-B and from chemiluminescence (Ryerson et al., 2000) for all other campaigns. There are other DC-8 aircraft campaigns, such as the Subsonic Assessment Ozone and Nitrogen Oxide Experiment (SONEX), over the North Atlantic, and the Deep Convective Clouds and Chemistry (DC3) campaign, over the eastern US. These are not included in our comparison because SONEX was heavily influenced by stratospheric air (Fuelberg et al., 2000) and because DC3 targeted thunderstorms with large concentrations of NOx from lightning, so it is not representative of a standard atmosphere (Singh et al., 1999; Barth et al., 2015; Nault et al., 2016). Measurements of HO2 are not available for SEAC4RS, so the PSS NO2 calculation for this campaign uses average upper-tropospheric [HO2] from the other three campaigns. We find that INTEX-A measurements of NO yield median PSS NO2 values at 450–180 hPa that are anomalously large (150–450 pptv) in comparison to PSS NO2 values from SEAC4RS (30–130 pptv), so no upper-tropospheric (450–180 hPa) INTEX-A values are used.
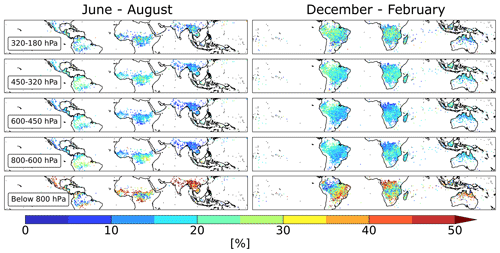
Figure 3Seasonal mean percentage contribution of NO2 in each cloud-sliced layer to the tropospheric column. Columns represent June–August (JJA; left) and December–February (DJF; right). Rows, from top to bottom, correspond to 320–180, 450–320, 600–450, 800–600, and below 800 hPa. Data are presented as multiyear means at a resolution of 1° × 1°.
2.3 The GEOS-Chem chemical transport model
We use GEOS-Chem to evaluate contemporary knowledge of tropospheric NOx by comparing it to our cloud-sliced NO2 vertical profiles. For this, we use version 13.3.4 of GEOS-Chem (The International GEOS-Chem User Community, 2021) to calculate 4-year seasonal mean NO2 covering the same vertical ranges as the cloud-sliced NO2. The model years sampled (1 December 2015 to 30 November 2019) differ from those for TROPOMI due to a lag in the availability of emission inventory data. The model is driven with NASA Modern-Era Retrospective analysis for Research and Applications (version 2; MERRA-2) reanalysis meteorology at a horizontal resolution of 2° × 2.5° over 47 vertical layers (30–35 in the troposphere), extending to 0.01 hPa.
Global emissions from all anthropogenic sources, except aircraft, are from the Community Emissions Data System (CEDS) version 2 for 2015 to 2019 (McDuffie et al., 2020). Aircraft emissions of NOx are from the Aviation Emissions Inventory Code (AEIC) for 2005 (Stettler et al., 2011). We use offline, grid-independent soil NOx emission data from Weng et al. (2020); the online Global Fire Emissions Database (version 4 with small fires; GFED4s) inventory (van der Werf et al., 2017) for open burning of biomass; and offline, grid-independent lightning NOx emission data prepared by Meng et al. (2021) using the parameterisation detailed in Murray et al. (2012).
GEOS-Chem exhibits a known underestimate in tropospheric NO2 over global oceans, as evidenced by past studies (Travis et al., 2020; Guo et al., 2023; Shah et al., 2023). We address this by updating the GEOS-Chem chemical mechanism to include the photolysis of particle-phase nitrates (pNO3), liberating NOx as NO2 and as the reservoir compound nitrous acid (HONO), followed by its prompt photolysis to form NO (Ye et al., 2017; Kasibhatla et al., 2018; Romer et al., 2018; Andersen et al., 2023). Photolysis of pNO3 is implemented in GEOS-Chem by scaling the photolysis of nitric acid (HNO3) by an enhancement factor (EF). The EF is 100 for coarse-mode pNO3 and is scaled down using the relative molar concentrations of pNO3 and sea salt aerosol, as in Shah et al. (2023) for fine-mode pNO3. This increases lower-tropospheric (< 6 km) NO2 over the remote ocean by up to 15 pptv but has a smaller effect (an increase of < 10 pptv) above 6 km, where pNO3 is much less abundant (Shah et al., 2023). Photolysis of the NOx reservoir compound peroxypropionyl nitrate (PPN; C2H5C(O)OONO2), leading to the formation of NO2, occurs in the atmosphere, but this photolysis is absent in GEOS-Chem. There are no reported laboratory measurements of NO2 quantum yields from PPN. According to the Harwood et al. (2003) laboratory study, PPN absorption cross-sections and quantum yields of the nitrate radical (NO3) are within 10 % of peroxyacetyl nitrate (PAN; CH3C(O)OONO2) values, so we use PAN quantum yields and cross-sections from Burkholder et al. (2020) to represent PPN photolysis in GEOS-Chem.
For a consistent comparison of the model to cloud-sliced NO2, GEOS-Chem is sampled around the TROPOMI overpass (12:00–15:00 LST), following a 3-month spin-up from 1 September to 30 November 2015 for the chemical initialisation of the 4-year simulation. Tropospheric NO2 in GEOS-Chem is identified using MERRA-2 tropopause heights, and additional filtering is applied to remove stratospheric intrusions ( > 1.25 mol mol−1). All-sky model scenes are sampled. Marais et al. (2021) determined by applying cloud slicing to synthetic columns of NO2 simulated with GEOS-Chem that the difference between NO2 under very cloudy conditions and NO2 under all-sky conditions is small (< 17 %). The TROPOMI cloud-sliced data are gridded to the GEOS-Chem grid for the comparison, and only grid cells with at least 10 cloud-sliced data points are compared. We use a threshold of 10 to ensure that meaningful comparisons can be made between GEOS-Chem and cloud-sliced data without excluding a large number of cloud-sliced data points.
3.1 Vertical distribution of tropospheric NO2 from cloud slicing TROPOMI
Figure 1 shows the spatial distribution of cloud-sliced NO2 in the free troposphere during June–August (JJA) 2018–2021 and December–February (DJF) 2018–2022, and Fig. 2 shows boundary-layer NO2 (below 800 hPa) for the same seasons and years, obtained using cloud slicing over the ocean and differencing over land (Sect. 2.1). The percentage of filled global 1° × 1° grids is similar in both seasons, albeit with expected seasonal shifts in regions covered due to seasonal variations in the locations of clouds associated with convective features, such as the Intertropical Convergence Zone (ITCZ), and an absence of clouds over regions of persistent subsidence west of southern Africa and South America. Coverage is greatest in the mid-troposphere and least at 320–180 hPa. The average percentage coverage over JJA and DJF is 63 % of grid cells for 600–450 hPa and 68 % for 800–600 hPa, covering most of the tropics, subtropics, and midlatitudes. There are slightly fewer (38 %) grid cells at 450–320 hPa, decreasing to 8 % at 320–180 hPa. The few grid squares filled at this height mostly occur in the tropics due to the higher tropopause and greater abundance of optically thick clouds (Wang et al., 1996). In the boundary layer (Fig. 2), a total of ∼ 14 % of the grids are filled, with ∼ 11 % from direct cloud slicing and ∼ 3 % from differencing. The occurrence of data obtained from the differencing approach is restricted to locations over land, due to limited coverage of cloud-sliced NO2 in the top upper-troposphere layer. Per-layer percentages of filled grids are similar for March–May and September–November.
Throughout the free troposphere in all seasons (Fig. 1), cloud-sliced NO2 is typically in the range of 20–60 pptv. In the upper troposphere, lightning NOx emissions and the photolysis of NOx reservoir compounds sustain NO2 concentrations of 20–70 pptv over the oceans and NO2 concentrations > 90 pptv over the continents in JJA at 450–320 hPa. NO2 concentrations exceeding 70 pptv in JJA at 450–320 hPa over North America, China, and the Indian subcontinent are due to the combination of lightning and the convective uplift of surface anthropogenic pollution (Bertram et al., 2007; Hudman et al., 2007). NO2 persists for longer in the cold, dry upper troposphere (Ehhalt et al., 1992; Jaeglé et al., 1998; Grewe et al., 2001) than in the mid-troposphere below, so NO2 concentrations are 20 pptv greater over Europe and North America at 450–320 hPa than at 600–450 hPa. NO2 over the open oceans is similar (25–50 pptv) throughout the free troposphere and is mostly due to lightning and continental outflow (Kawakami et al., 1997; Zien et al., 2014). NO2 concentrations in excess of 55 pptv over South America and 80 pptv over central Africa at 800–600 hPa result from a mix of intense continental lightning and seasonal open burning of biomass (Andreae et al., 2001; Christian et al., 2003; Duncan et al., 2003). The burning season in South America starts in July and occurs throughout JJA in southern Africa and throughout DJF in Africa north of the tropics (Van der Werf et al., 2006; Castellanos et al., 2014; Van der Velde et al., 2021). NO2 is longer-lived in winter due to cold conditions and slow photolysis (Dickerson et al., 1982; Kenagy et al., 2018), so over continental Europe, large surface sources of anthropogenic NOx and limited lightning activity, especially in comparison to the US, contribute to 80 pptv more NO2 in DJF than in JJA at 800–600 hPa.
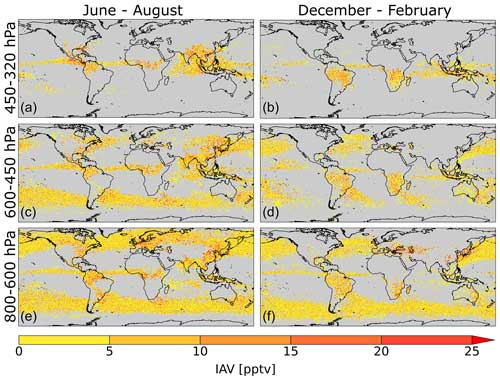
Figure 4Interannual variability (IAV) in free-tropospheric NO2. Panels show single-year NO2 IAV obtained as the absolute difference between single-year cloud-sliced NO2 (a, c, e: JJA 2021; b, d, f: DJF 2020–2021) and multiyear mean cloud-sliced NO2 for the three layers with the greatest geographic coverage. Only grid squares with at least five cloud-sliced data points in the single-year means are compared.
In the marine boundary layer (Fig. 2a), the typical range in NO2 concentrations is similar to that of the layers above, except near coastlines influenced by the continental outflow of anthropogenic pollution and local NOx production from busy harbours. Along the east coast of China, for example, NO2 concentrations are > 90 pptv, compared to 25–35 pptv over the remote ocean east of China. NO2 coverage in the terrestrial boundary layer, shown in Fig. 2b, is limited to the tropics in JJA and to the tropics and southern subtropics in DJF, when cloud-sliced NO2 data are available in all four overlying layers (Fig. 1). In the terrestrial boundary layer, NO2 concentrations exceed 30 pptv and peak at 600 pptv over eastern Brazil in DJF, central Africa in both seasons, and southeastern Asia and the Indo-Gangetic Plain (IGP) in JJA. The peaks in Brazil and central Africa are due to biomass burning, whereas the peaks for southeastern Asia and the IGP are associated with large urban and industrial sources (Giglio et al., 2010; Ghude et al., 2013; Lu et al., 2024). Steep latitudinal gradients in NO2 exceeding 100 pptv, obtained with the differencing approach for NO2 covering Amazonia and central Africa, are due to the influence of the intense seasonal burning of savanna-type vegetation bordering dense tropical forests (Chen et al., 2013; Ossohou et al., 2019; Jin et al., 2021; Van der Velde et al., 2021).
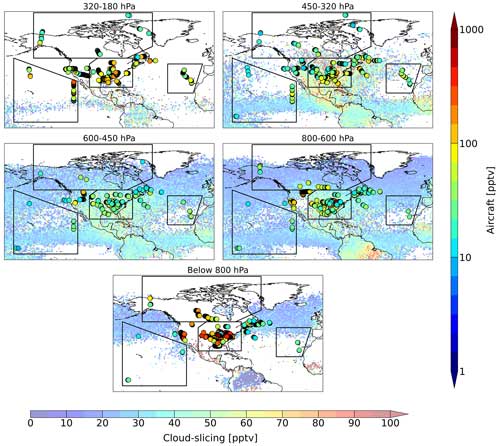
Figure 5Maps of tropospheric NO2 over the western Northern Hemisphere in June–August for the five cloud-slicing pressure ranges. Filled circles represent DC-8 NO2 data obtained along DC-8 flight tracks (Sect. 2.2). Background values are cloud-sliced NO2. Polygons show the regions sampled for the comparison of aircraft and cloud-sliced NO2 shown in Figs. 6 and 7. These are the North Atlantic, the Canadian Arctic, the eastern United States, and the Pacific.
The seasonal mean cloud-sliced NO2 at 450–180 hPa obtained by Marais et al. (2021), which we compare to our data for the same vertical extent and time period (Sect. 2.1), ranges from > 80 pptv over terrestrial regions to < 50 pptv over remote oceans. The two datasets are spatially consistent in all seasons, yielding Pearson's correlation coefficients (R) of 0.74 in JJA, 0.70 in September–November (SON), 0.64 in DJF, and 0.65 in March–May (MAM). Marais et al. (2021) found that NO2 concentrations are, on average, 26 % greater than those obtained with our updated cloud slicing. This difference, decomposed into variance and background using RMA regression, presents as 25 %–37 % more variance and 17–22 pptv less background NO2 in our data across all four seasons. The greater background values in Marais et al. (2021) are attributed to the susceptibility of their approach to outliers (Sect. 2.1).
Figure 3 shows the relative contribution of individual layers to the tropospheric column, obtained by summing the column densities of cloud-sliced NO2 in each layer for grid cells with data in all layers. This limits coverage to the tropics and subtropics. As expected, the boundary-layer contribution is the greatest, typically exceeding 55 % in locations influenced by intensive anthropogenic activity and biomass burning (Sahu and Sheel, 2014; Beirle et al., 2019; Keita et al., 2021). The relative contribution from layers above the boundary layer exhibits zonal and meridional variability but remains relatively constant with altitude, amounting to ∼ 20 % over central Africa and ∼ 10 % over southern Asia.
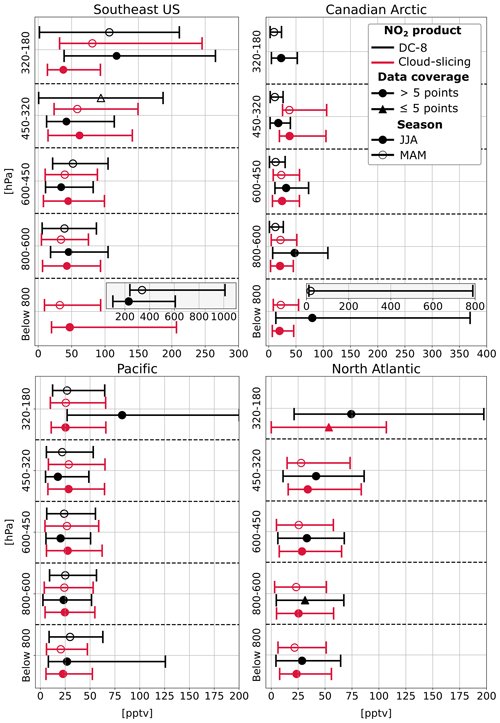
Figure 6Comparison of seasonal mean vertical profiles of DC-8 and cloud-sliced tropospheric NO2. Symbols represent median values for the sampling domains shown in Fig. 5 for MAM (unfilled symbols) and JJA (filled symbols). Symbol shapes for both the DC-8 and cloud-slicing datasets differentiate between medians obtained with fewer than six data points (triangles) and those obtained with more than five data points (circles). Error bars represent interquartile ranges (IQRs). The NO2 concentration scales differ, and inset boxes (in the top row) show boundary-layer NO2 exceeding the x-axis range.
We also examine the size of interannual variability (IAV) in tropospheric NO2 according to our cloud-sliced data. This is shown in Fig. 4 for JJA and DJF in a selected year (2021 for JJA and December 2020 to February 2021 for DJF) and is calculated as the absolute difference between cloud-sliced NO2 in these years and the multiyear mean (Fig. 1). Only three of the five layers are shown as coverage is poor for individual years for the other two layers. IAV data are obtained for < 1 % of all 1° × 1° grid cells at 180–320 hPa and for just 2 % in the boundary layer. IAV in the layers shown in Fig. 4 is typically ∼ 10 pptv over the remote ocean and ∼ 25 pptv over continental regions (the eastern US, Europe, and the tropics). The greater IAV over the continents is due to the influence of anthropogenic, biomass burning, and lightning NOx emissions. NO2 IAV corresponds to about 20 %–50 % of the variability in the multiyear means shown in Figs. 1 and 2. Relatively large NO2 IAV over the remote oceans is restricted to the edges of sampled areas in the subtropics that have low data density, due to proximity to regions of persistent subsidence, where retrievals from cloud slicing are not always successful.
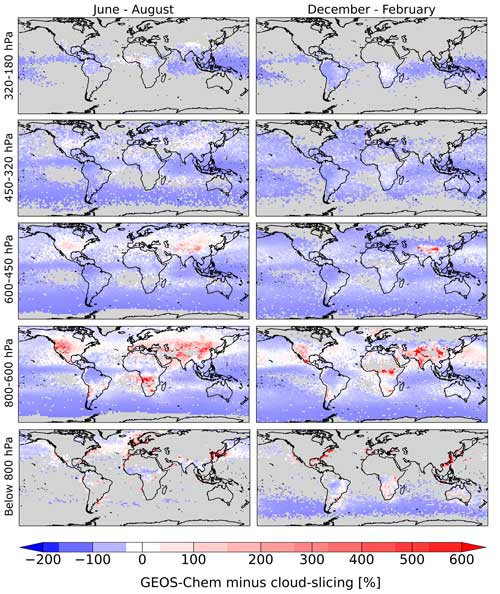
Figure 8Percentage difference between cloud-sliced and GEOS-Chem vertical profiles of tropospheric NO2. Maps are presented at a resolution of 2° × 2.5°. Blue (red) indicates that the model's values are smaller (greater) than the values of cloud-sliced NO2. The percentage difference is calculated by taking the difference between the GEOS-Chem and cloud-sliced values and dividing the result by the cloud-sliced values. It is determined for all regridded cloud-sliced 2° × 2.5° grid squares filled in each sampled year.
3.2 Evaluation of cloud-sliced NO2 with observed and calculated (PSS) NO2
Figure 5 shows the regions selected to intercompare cloud-sliced and DC-8 NO2 obtained from direct measurements and PSS NO2 (Sect. 2.2). Selected regions include the North Atlantic Ocean, sampled during ATom; the Canadian Arctic, sampled during ARCTAS and ATom; the eastern United States, sampled during SEAC4RS, INTEX-A, and INTEX-B; and the Pacific Ocean, sampled during ATom and INTEX-B. These regions were chosen to optimise the coincidence of aircraft data in all five layers. In many instances, though, coincidence is over a limited extent of the sampling domain, especially in the upper troposphere across almost all domains and in the Pacific Ocean across all layers. Domains sampled in all seasons due to the ATom campaign include the Canadian Arctic and the Pacific and Atlantic oceans. The most sampled time period is JJA, the greatest regional coverage is over the eastern US, and the mid-tropospheric layers (800–600 and 600–450 hPa) have the most DC-8 data. According to the DC-8 NO2 data, hotspots (where NO2 is > 200 pptv) occur over the US terrestrial boundary layer, where there are large surface NOx emissions. Much lower concentrations of < 25 pptv over the remote ocean are due to an absence of large local sources.
Figures 6 and 7 compare median DC-8 and cloud-sliced NO2 concentrations for MAM and JJA (Fig. 6) and SON and DJF (Fig. 7) for the polygons in Fig. 5. Cloud-slicing data are for 2018–2021 in JJA and SON, 2018–2022 in DJF, and 2019–2022 in MAM. JJA data are compared to the ARCTAS, SEAC4RS, INTEX-A, and ATom-1 campaigns, while DJF data are compared to ATom-2, and SON data are compared to ATom-3 and SEAC4RS. MAM data are compared to ATom-4, ARCTAS, INTEX-A, and INTEX-B. Only the boundary layer and mid-troposphere layers are compared for INTEX-A as PSS NO2 cannot be calculated due to issues with the NO measurements (Sect. 2.2). Vertical profiles of DC-8 NO2 are relatively stable (∼ 25–80 pptv) throughout the troposphere over the Pacific and North Atlantic oceans and increase exponentially to ∼ 75–450 pptv in the boundary layer over the southeastern US and the Canadian Arctic. Most cloud-sliced NO2 in the mid-troposphere and in the 320–450 hPa layer of the upper troposphere differs by < 15 pptv from DC-8 NO2 in the extensively sampled southeastern US and by < 25 pptv in other locations for medians obtained with more than five data points. Greater variability (i.e. wider interquartile ranges) in each layer of either dataset is typically due to fewer data points and less extensive coverage (Fig. 4).
Large differences between DC-8 and cloud-sliced NO2 occur in the boundary layer and the top tropospheric layer. In these layers, there are few coincident data points (Fig. 5). Most DC-8 data in these two layers are over land influenced by ground-based sources, such as intense biomass burning in the boundary layer (Alvarado et al., 2010; Bian et al., 2013), lightning, and the convective uplift of surface pollution in the upper troposphere, whereas most cloud-sliced NO2 in these two layers occurs over the ocean (Fig. 5). The cluster of points in the boundary layer over New England, in the northeastern US (shown in Fig. 5), has similar coverage for both datasets. These points exhibit a median of 30 pptv (IQR: 20–50 pptv) for DC-8 NO2 and a median of 25 pptv (IQR: 20–30 pptv) for cloud-sliced NO2. New England is not included in our comparison in Figs. 6 and 7 as sampling over this location is limited to JJA during INTEX-A.
3.3 Comparison of cloud-sliced vertical profiles with synthetic GEOS-Chem profiles
Figure 8 shows the percentage difference between multiyear mean GEOS-Chem and cloud-sliced NO2 for June–August and December–February, obtained after regridding the cloud-sliced NO2 to the 2° × 2.5° GEOS-Chem grid. Multiyear means for both datasets are compared to minimise the influence of the interannual variability quantified in Sect. 3.1. In general, the amount of GEOS-Chem NO2 is 30 %–80 % (10–25 pptv) less than the amount of cloud-sliced NO2 in remote locations. Specifically, this occurs above the Southern Ocean in all layers retrieved, above South America throughout the free troposphere, and for all grid cells except those over Africa in the upper troposphere. Spatial patterns and magnitudes of discrepancies similar to those plotted in Fig. 8 occur in March–May and September–November.
Inclusion of nitrate photolysis in GEOS-Chem decreases the model underestimate of NO2 over remote regions from 40–80 pptv to an average of ∼ 15 pptv in the mid-troposphere. A relatively large model underestimate of 25–40 pptv over oceans may be due to uncertainties in the enhancement factor used to parameterise nitrate photolysis (Sect. 2.3) (Shah et al., 2023). PPN photolysis is most effective at increasing NO2 in the two layers of the upper troposphere where it is abundant and thermally stable, meaning photolysis dominates its conversion to NO2. In JJA, for example, PPN photolysis contributes ∼ 65 pptv of NO2 over the northern midlatitudes, with isolated enhancements of 50–60 pptv over southeastern Asia, extending from Mozambique to Madagascar. As a result of PPN photolysis, the discrepancy between the model and cloud-sliced upper-tropospheric NO2 is relatively small (10–30 pptv) over the terrestrial northern midlatitudes. The model exceeds the cloud-sliced data by 20–50 pptv over the northern midlatitudes at 600–450 hPa during the summer lightning season north of 35° N. These are the latitudes at which lightning NOx production rates in GEOS-Chem almost double, from 260 moles per flash (mol fl−1) to the south to 500 mol fl−1 to the north (Murray et al., 2012). The effect of this on NO2 is also evident at 450–320 hPa, though the spatial extent and dataset differences are smaller in this layer. The 500 mol fl−1 rate that is applied to northern-midlatitude lightning far exceeds observationally constrained global mean estimates of ∼ 280 mol fl−1 (Marais et al., 2018), regional mean estimates of 180 mol fl−1 for the northern midlatitudes (Bucsela et al., 2019), and estimates of 230–360 mol fl−1 for the US and western Atlantic (Allen et al., 2021).
The largest differences between the two datasets occur in the boundary layer along coastlines in North America, Europe, and China, influenced by anthropogenic pollution. This may in part be due to the different years targeted. COVID lockdowns influenced the surface emissions of traffic NOx in the cloud-sliced data, and anthropogenic NOx emissions are steadily declining over North America, Europe, and China as a result of air quality regulations (Zhao et al., 2013; Lloret and Valiela, 2016; Clappier et al., 2021). Both COVID lockdowns and emission reduction policies would contribute to a model overestimate of NO2. GEOS-Chem NO2 also exceeds cloud-sliced NO2 at multiple locations in the 800–600 hPa layer. These include southern Africa in JJA and northern Africa in DJF, coinciding with the dry burning season in these regions, as well as central Asia in all seasons, where there are large sources of anthropogenic pollution. The apparent model overestimate over the western US at 600–800 hPa occurs in all seasons and may result from a combination of factors. The TROPOMI sampling period includes the high-fire year (2020) (Albores et al., 2023), while the model does not, affecting the comparison in seasons coincident with the fire season (JJA and SON). There are also relatively few cloud-sliced data points over this region of subsidence. It is difficult to diagnose discrepancies in the tropical terrestrial boundary layer as anthropogenic emission inventories are prone to misrepresenting sources unique to the tropics (Duncan et al., 2003; Marais and Wiedinmyer, 2016; Vohra et al., 2022), and there are no suitable independent in situ measurements for validating the differencing approach we use to derive NO2.
Global vertical profiles of tropospheric NO2 were obtained for five discrete layers (180–320 hPa, 320–450 hPa, 450–600 hPa, 600–800 hPa, and below 800 hPa) by cloud slicing TROPOMI total columns of NO2 above optically thick clouds. We assessed these against directly measured and calculated (photostationary steady-state) NASA DC-8 aircraft NO2 from 2004 to 2018. We then applied our cloud-sliced NO2 to evaluate the contemporary understanding of climatological tropospheric NOx as simulated by GEOS-Chem. We found that coverage from cloud slicing is greatest in the mid-troposphere (60 %–70 %), where there is an abundance of optically thick clouds, and least in the upper troposphere (8 % coverage; mostly in the tropics). Cloud-sliced NO2 ranges from < 35 pptv throughout the troposphere over remote marine regions to 20-60 pptv in the free troposphere over continents and 160–380 pptv in the boundary layer over source regions in the US, Europe, and Asia. Free-tropospheric NO2 exhibits very little interannual variability, ranging from ∼ 10 pptv over oceans to ∼ 25 pptv over land.
We determined from comparing cloud-sliced NO2 to NASA DC-8 aircraft observations that cloud-sliced NO2 differs from DC-8 NO2 by just 5–15 pptv when sampling in both datasets is abundant and consistent. It was not feasible to assess cloud-sliced NO2 in the boundary layer and in the highest cloud-sliced layer due to a lack of sufficient coincident data in the tropics. The GEOS-Chem model, which represents a contemporary understanding of tropospheric NOx, simulates NO2 concentrations that are typically 10–40 pptv less than cloud-sliced NO2 concentrations in the remote upper troposphere and over remote oceans. This is a substantial improvement over the > 40 pptv model underestimate present before accounting for NOx recycling in the upper troposphere via PPN photolysis and NOx recycling in the middle and lower troposphere via aerosol nitrate photolysis. Differences are greater over source regions influenced by lightning, biomass burning, and evolving anthropogenic emissions that result from rapid development, policies, and events like lockdowns in response to the COVID-19 pandemic. A large positive model bias of 50 pptv over the Northern Hemisphere mid-troposphere in June–August points to an issue with lightning NOx production rates in the model, which are almost double the production rates everywhere else.
The limited coincidence of reliable observations for validating cloud-sliced NO2 remains a challenge, but as we demonstrate, cloud-sliced NO2 holds value for assessing air quality, chemical transport, and Earth system models to identify differences that warrant further investigation, especially given the reliance on these models to understand complex tropospheric chemistry, inform policies, and retrieve trace gas abundances from satellites. Geostationary instruments will further enhance the utility of cloud-sliced NO2 datasets, allowing us to also investigate daytime variability in vertical profiles of tropospheric NOx.
The multiyear seasonal mean NO2 data from cloud slicing TROPOMI and simulating the GEOS-Chem model are publicly available in the University College London (UCL) Research Data Repository (https://doi.org/10.5522/04/25782336, Marais and Horner, 2024). DC-8 aircraft datasets are publicly available from NASA for ARCTAS (https://doi.org/10.5067/SUBORBITAL/ARCTAS2008/DATA001, ARCTAS Science Team, 2011), ATom (https://doi.org/10.3334/ORNLDAAC/1925, ATom Science Team, 2021), INTEX-A (https://doi.org/10.5067/ASDCDAAC/INTEXA/0008, INTEX-A Science Team, 2006), INTEX-B (https://doi.org/10.5067/AIRCRAFT/INTEXB/, INTEX-B Science Team, 2011), and SEAC4RS (https://doi.org/10.5067/AIRCRAFT/SEAC4RS, SEAC4RS Science Team, 2014). TROPOMI PAL and OFFL products are publicly available from the Copernicus Open Access Hub (https://data-portal.s5p-pal.com/, TROPOMI, 2018; https://s5phub.copernicus.eu/dhus/#/homeTS3, TROPOMI, 2021). The GEOS-Chem version 13.3.4 source code is publicly available on Zenodo (https://doi.org/10.5281/zenodo.5764874, The International GEOS-Chem User Community, 2021).
The study concept was developed by EAM and RPH. RPH led the writing and analysis, simulated the GEOS-Chem model, and cloud-sliced TROPOMI NO2 with supervision from EAM. NW provided the NASA DC-8 Python processing code. RGR updated the GEOS-Chem model to include PPN photolysis. VS updated the GEOS-Chem model to include particulate nitrate photolysis. All authors reviewed and edited the paper.
The contact author has declared that none of the authors has any competing interests.
Publisher’s note: Copernicus Publications remains neutral with regard to jurisdictional claims made in the text, published maps, institutional affiliations, or any other geographical representation in this paper. While Copernicus Publications makes every effort to include appropriate place names, the final responsibility lies with the authors.
This research has been supported by the European Research Council under the European Union's Horizon 2020 research and innovation programme (through a Starting Grant awarded to Eloise A. Marais, UpTrop (grant no. 851854)). We are grateful to the NASA DC-8 aircraft campaign teams for providing access to observations; specifically, we thank the NO2 measurement principal investigators Ronald Cohen (INTEX-A and INTEX-B), Andrew Weinheimer (ARCTAS), Thomas B. Ryerson (SEAC4RS), and Chelsea Thompson (ATom).
This research has been supported by the European Research Council through the H2020 research and innovation programme (through a Starting Grant awarded to Eloise A. Marais, UpTrop (grant no. 851854)).
This paper was edited by Bryan N. Duncan and reviewed by two anonymous referees.
Albores, I. S., Buchholz, R. R., Ortega, I., Emmons, L. K., Hannigan, J. W., Lacey, F., Pfister, G., Tang, W., and Worden, H. M.: Continental-scale Atmospheric Impacts of the 2020 Western U.S. Wildfires, Atmos. Environ., 294, 119436, https://doi.org/10.1016/j.atmosenv.2022.119436, 2023.
Allen, D. J., Pickering, K. E., Lamsal, L., Mach, D. M., Quick, M. G., Lapierre, J., Janz, S., Koshak, W., Kowalewski, M., and Blakeslee, R.: Observations of Lightning NOx Production From GOES-R Post Launch Test Field Campaign Flights, J. Geophys. Res.-Atmos., 126, e2020JD033769, https://doi.org/10.1029/2020JD033769, 2021.
Alvarado, M. J., Logan, J. A., Mao, J., Apel, E., Riemer, D., Blake, D., Cohen, R. C., Min, K.-E., Perring, A. E., Browne, E. C., Wooldridge, P. J., Diskin, G. S., Sachse, G. W., Fuelberg, H., Sessions, W. R., Harrigan, D. L., Huey, G., Liao, J., Case-Hanks, A., Jimenez, J. L., Cubison, M. J., Vay, S. A., Weinheimer, A. J., Knapp, D. J., Montzka, D. D., Flocke, F. M., Pollack, I. B., Wennberg, P. O., Kurten, A., Crounse, J., Clair, J. M. St., Wisthaler, A., Mikoviny, T., Yantosca, R. M., Carouge, C. C., and Le Sager, P.: Nitrogen oxides and PAN in plumes from boreal fires during ARCTAS-B and their impact on ozone: an integrated analysis of aircraft and satellite observations, Atmos. Chem. Phys., 10, 9739–9760, https://doi.org/10.5194/acp-10-9739-2010, 2010.
Andersen, S. T., Carpenter, L. J., Reed, C., Lee, J. D., Chance, R., Sherwen, T., Vaughan, A. R., Stewart, J., Edwards, P. M., Bloss, W. J., Sommariva, R., Crilley, L. R., Nott, G. J., Neves, L., Read, K., Heard, D. E., Seakins, P. W., Whalley, L. K., Boustead, G. A., Fleming, L. T., Stone, D., and Fomba, K. W.: Extensive field evidence for the release of HONO from the photolysis of nitrate aerosols, Sci. Adv., 9, 3, https://doi.org/10.1126/sciadv.add6266, 2023.
Andreae, M. O., Artaxo, P., Fischer, H., Freitas, S. R., Grégoire, J.-M., Hansel, A., Hoor, P., Kormann, R., Krejci, R., Lange, L., Lelieveld, J., Lindinger, W., Longo, K., Peters, W., De Reus, M., Scheeren, B., Silva Dias, M. A. F., Ström, J., Van Velthoven, P. F. J., and Williams, J.: Transport of biomass burning smoke to the upper troposphere by deep convection in the equatorial region, Geophys. Res. Lett., 28, 951–954, https://doi.org/10.1029/2000GL012391, 2001.
ARCTAS Science Team: Arctic Research of the Composition of the Troposphere from Aircraft and Satellites (ARCTAS) NASA Airborne Mission Overview, NASA [data set], https://doi.org/10.5067/SUBORBITAL/ARCTAS2008/DATA001, 2011
Atkinson, R.: Atmospheric chemistry of VOCs and NOx, Atmos. Environ., 34, 2063–2101, https://doi.org/10.1016/S1352-2310(99)00460-4, 2000.
ATom Science Team: Atmospheric Tomography Mission (ATom): Merged Atmospheric Chemistry, Trace Gases, and Aerosols, Version 2, NASA [data set], https://doi.org/10.3334/ORNLDAAC/1925, 2021
Barth, M. C., Cantrell, C. A., Brune, W. H., Rutledge, S. A., Crawford, J. H., Huntrieser, H., Carey, L. D., MacGorman, D., Weisman, M., Pickering, K. E., Bruning, E., Anderson, B., Apel, E., Biggerstaff, M., Campos, T., Campuzano-Jost, P., Cohen, R., Crounse, J., Day, D. A., Diskin, G., Flocke, F., Fried, A., Garland, C., Heikes, B., Honomichl, S., Hornbrook, R., Huey, L. G., Jimenez, J. L., Lang, T., Lichtenstern, M., Mikoviny, T., Nault, B., O'Sullivan, D., Pan, L. L., Peischl, J., Pollack, I., Richter, D., Riemer, D., Ryerson, T., Schlager, H., St. Clair, J., Walega, J., Weibring, P., Weinheimer, A., Wennberg, P., Wisthaler, A., Wooldridge, P. J., and Ziegler, C.: The Deep Convective Clouds and Chemistry (DC3) Field Campaign, Bull. Am. Meteorol. Soc., 96, 1281–1309, https://doi.org/10.1175/BAMS-D-13-00290.1, 2015.
Beirle, S., Borger, C., Dörner, S., Li, A., Hu, Z., Liu, F., Wang, Y., and Wagner, T.: Pinpointing nitrogen oxide emissions from space, Sci. Adv., 5, eaax9800, https://doi.org/10.1126/sciadv.aax9800, 2019.
Belmonte Rivas, M., Veefkind, P., Eskes, H., and Levelt, P.: OMI tropospheric NO2 profiles from cloud slicing: constraints on surface emissions, convective transport and lightning NOx, Atmos. Chem. Phys., 15, 13519–13553, https://doi.org/10.5194/acp-15-13519-2015, 2015.
Bertram, T. H., Perring, A. E., Wooldridge, P. J., Crounse, J. D., Kwan, A. J., Wennberg, P. O., Scheuer, E., Dibb, J., Avery, M., Sachse, G., Vay, S. A., Crawford, J. H., McNaughton, C. S., Clarke, A., Pickering, K. E., Fuelberg, H., Huey, G., Blake, D. R., Singh, H. B., Hall, S. R., Shetter, R. E., Fried, A., Heikes, B. G., and Cohen, R. C.: Direct Measurements of the Convective Recycling of the Upper Troposphere, Science, 315, 816–820, https://doi.org/10.1126/science.1134548, 2007.
Bian, H., Colarco, P. R., Chin, M., Chen, G., Rodriguez, J. M., Liang, Q., Blake, D., Chu, D. A., da Silva, A., Darmenov, A. S., Diskin, G., Fuelberg, H. E., Huey, G., Kondo, Y., Nielsen, J. E., Pan, X., and Wisthaler, A.: Source attributions of pollution to the Western Arctic during the NASA ARCTAS field campaign, Atmos. Chem. Phys., 13, 4707–4721, https://doi.org/10.5194/acp-13-4707-2013, 2013.
Bloss, W. J., Evans, M. J., Lee, J. D., Sommariva, R., Heard, D. E., and Pilling, M. J.: The oxidative capacity of the troposphere: Coupling of field measurements of OH and a global chemistry transport model, Faraday Discuss., 130, 425, https://doi.org/10.1039/b419090d, 2005.
Boersma, K. F., Eskes, H. J., and Brinksma, E. J.: Error analysis for tropospheric NO2 retrieval from space, J. Geophys. Res.-Atmos., 109, D4, https://doi.org/10.1029/2003JD003962, 2004.
Bradshaw, J., Davis, D., Grodzinsky, G., Smyth, S., Newell, R., Sandholm, S., and Liu, S.: Observed distributions of nitrogen oxides in the remote free troposphere from the Nasa Global Tropospheric Experiment Programs, Rev. Geophys., 38, 61–116, https://doi.org/10.1029/1999RG900015, 2000.
Brenninkmeijer, C. A. M., Crutzen, P. J., Fischer, H., Güsten, H., Hans, W., Heinrich, G., Heintzenberg, J., Hermann, M., Immelmann, T., Kersting, D., Maiss, M., Nolle, M., Pitscheider, A., Pohlkamp, H., Scharffe, D., Specht, K., and Wiedensohler, A.: CARIBIC – Civil Aircraft for Global Measurement of Trace Gases and Aerosols in the Tropopause Region, J. Atmospheric Ocean. Technol., 16, 1373–1383, https://doi.org/10.1175/1520-0426(1999)016<1373:CCAFGM>2.0.CO;2, 1999.
Browne, E. C., Perring, A. E., Wooldridge, P. J., Apel, E., Hall, S. R., Huey, L. G., Mao, J., Spencer, K. M., Clair, J. M. St., Weinheimer, A. J., Wisthaler, A., and Cohen, R. C.: Global and regional effects of the photochemistry of CH3O2NO2: evidence from ARCTAS, Atmos. Chem. Phys., 11, 4209–4219, https://doi.org/10.5194/acp-11-4209-2011, 2011.
Bucsela, E. J., Pickering, K. E., Allen, D. J., Holzworth, R. H., and Krotkov, N. A.: Midlatitude Lightning NO x Production Efficiency Inferred From OMI and WWLLN Data, J. Geophys. Res.-Atmos., 124, 13475–13497, https://doi.org/10.1029/2019JD030561, 2019.
Burkholder, J. B., Sander, S. P., Abbatt, J. P. D., Barker, J. R., Cappa, C., Crounse, J. D., Dibble, T. S., Huie, R. E., Kolb, C. E., Kurylo, M. J., Orkin, V. L., Percival, C. J., Wilmouth, D. M., and Wine, P. H.: Chemical Kinetics and Photochemical Data for Use in Atmospheric Studies, Evaluation No. 19, https://jpldataeval.jpl.nasa.gov/ (last access: 30 August 2023), 2020.
Castellanos, P., Boersma, K. F., and van der Werf, G. R.: Satellite observations indicate substantial spatiotemporal variability in biomass burning NOx emission factors for South America, Atmos. Chem. Phys., 14, 3929–3943, https://doi.org/10.5194/acp-14-3929-2014, 2014.
Chatfield, R. B.: Anomalous HNO3/NOx ratio of remote tropospheric air: Conversion of nitric acid to formic acid and NOx?, Geophys. Res. Lett., 21, 2705–2708, https://doi.org/10.1029/94GL02659, 1994.
Chen, Y., Morton, D. C., Jin, Y., Collatz, G. J., Kasibhatla, P. S., Van Der Werf, G. R., DeFries, R. S., and Randerson, J. T.: Long-term trends and interannual variability of forest, savanna and agricultural fires in South America, Carbon Manag., 4, 617–638, https://doi.org/10.4155/cmt.13.61, 2013.
Choi, S., Joiner, J., Choi, Y., Duncan, B. N., Vasilkov, A., Krotkov, N., and Bucsela, E.: First estimates of global free-tropospheric NO2 abundances derived using a cloud-slicing technique applied to satellite observations from the Aura Ozone Monitoring Instrument (OMI), Atmos. Chem. Phys., 14, 10565–10588, https://doi.org/10.5194/acp-14-10565-2014, 2014.
Christian, H. J., Blakeslee, R. J., Boccippio, D. J., Boeck, W. L., Buechler, D. E., Driscoll, K. T., Goodman, S. J., Hall, J. M., Koshak, W. J., Mach, D. M., and Stewart, M. F.: Global frequency and distribution of lightning as observed from space by the Optical Transient Detector, J. Geophys. Res.-Atmos., 108, D1, https://doi.org/10.1029/2002JD002347, 2003.
Clappier, A., Thunis, P., Beekmann, M., Putaud, J. P., and De Meij, A.: Impact of SOx, NOx and NH3 emission reductions on PM2.5 concentrations across Europe: Hints for future measure development, Environ. Int., 156, 106699, https://doi.org/10.1016/j.envint.2021.106699, 2021.
Crawford, J., Davis, D., Chen, G., Bradshaw, J., Sandholm, S., Gregory, G., Sachse, G., Anderson, B., Collins, J., Blake, D., Singh, H., Heikes, B., Talbot, R., and Rodriguez, J.: Photostationary state analysis of the NO2-NO system based on airborne observations from the western and central North Pacific, J. Geophys. Res.-Atmos., 101, 2053–2072, https://doi.org/10.1029/95JD02201, 1996.
Crutzen, P. J. and Andreae, M. O.: Biomass Burning in the Tropics: Impact on Atmospheric Chemistry and Biogeochemical Cycles, Science, 250, 1669–1678, https://doi.org/10.1126/science.250.4988.1669, 1990.
Davis, D. D., Chen, G., Chameides, W., Bradshaw, J., Sandholm, S., Rodgers, M., Schendal, J., Madronich, S., Sachse, G., Gregory, G., Anderson, B., Barrick, J., Shipham, M., Collins, J., Wade, L., and Blake, D.: A photostationary state analysis of the NO2-NO system based on airborne observations from the subtropical/tropical North and South Atlantic, J. Geophys. Res., 98, 23501, https://doi.org/10.1029/93JD02412, 1993.
Di Carlo, P., Aruffo, E., Busilacchio, M., Giammaria, F., Dari-Salisburgo, C., Biancofiore, F., Visconti, G., Lee, J., Moller, S., Reeves, C. E., Bauguitte, S., Forster, G., Jones, R. L., and Ouyang, B.: Aircraft based four-channel thermal dissociation laser induced fluorescence instrument for simultaneous measurements of NO2, total peroxy nitrate, total alkyl nitrate, and HNO3, Atmos. Meas. Tech., 6, 971–980, https://doi.org/10.5194/amt-6-971-2013, 2013.
Dickerson, R. R., Stedman, D. H., and Delany, A. C.: Direct measurements of ozone and nitrogen dioxide photolysis rates in the troposphere, J. Geophys. Res.-Oceans, 87, 4933–4946, https://doi.org/10.1029/JC087iC07p04933, 1982.
Dignon, J.: NOx and SOx emissions from fossil fuels: A global distribution, Atmospheric Environ. Part Gen. Top., 26, 1157–1163, https://doi.org/10.1016/0960-1686(92)90047-O, 1992.
Duncan, B. N., Martin, R. V., Staudt, A. C., Yevich, R., and Logan, J. A.: Interannual and seasonal variability of biomass burning emissions constrained by satellite observations, J. Geophys. Res., 108, 4100, https://doi.org/10.1029/2002JD002378, 2003.
Ehhalt, D. H., Rohrer, F., and Wahner, A.: Sources and distribution of NOx in the upper troposphere at northern mid-latitudes, J. Geophys. Res., 97, 3725, https://doi.org/10.1029/91JD03081, 1992.
Emmons, L. K., Hauglustaine, D. A., Müller, J., Carroll, M. A., Brasseur, G. P., Brunner, D., Staehelin, J., Thouret, V., and Marenco, A.: Data composites of airborne observations of tropospheric ozone and its precursors, J. Geophys. Res.-Atmos., 105, 20497–20538, https://doi.org/10.1029/2000JD900232, 2000.
Eskes, H. J. and Eichmann, K.-U.: S5P Mission Performance Centre Nitrogen Dioxide [L2_NO2_] Readme, https://sentinels.copernicus.eu/documents/247904/3541451/Sentinel-5P-Nitrogen-Dioxide-Level-2-Product-Readme-File (last access: 7 July 2022), 2023.
Fuelberg, H. E., Hannan, J. R., Van Velthoven, P. F. J., Browell, E. V., Bieberbach, G., Knabb, R. D., Gregory, G. L., Pickering, K. E., and Selkirk, H. B.: A meteorological overview of the Subsonic Assessment Ozone and Nitrogen Oxide Experiment (SONEX) period, J. Geophys. Res.-Atmos., 105, 3633–3651, https://doi.org/10.1029/1999JD900917, 2000.
Ghude, S. D., Kulkarni, S. H., Jena, C., Pfister, G. G., Beig, G., Fadnavis, S., and Van Der A, R. J.: Application of satellite observations for identifying regions of dominant sources of nitrogen oxides over the Indian Subcontinent, J. Geophys. Res.-Atmos., 118, 1075–1089, https://doi.org/10.1029/2012JD017811, 2013.
Giglio, L., Randerson, J. T., van der Werf, G. R., Kasibhatla, P. S., Collatz, G. J., Morton, D. C., and DeFries, R. S.: Assessing variability and long-term trends in burned area by merging multiple satellite fire products, Biogeosciences, 7, 1171–1186, https://doi.org/10.5194/bg-7-1171-2010, 2010.
Grewe, V., Brunner, D., Dameris, M., Grenfell, J. L., Hein, R., Shindell, D., and Staehelin, J.: Origin and variability of upper tropospheric nitrogen oxides and ozone at northern mid-latitudes, Atmos. Environ., 35, 3421–3433, https://doi.org/10.1016/S1352-2310(01)00134-0, 2001.
Guo, H., Flynn, C. M., Prather, M. J., Strode, S. A., Steenrod, S. D., Emmons, L., Lacey, F., Lamarque, J.-F., Fiore, A. M., Correa, G., Murray, L. T., Wolfe, G. M., St. Clair, J. M., Kim, M., Crounse, J., Diskin, G., DiGangi, J., Daube, B. C., Commane, R., McKain, K., Peischl, J., Ryerson, T. B., Thompson, C., Hanisco, T. F., Blake, D., Blake, N. J., Apel, E. C., Hornbrook, R. S., Elkins, J. W., Hintsa, E. J., Moore, F. L., and Wofsy, S. C.: Heterogeneity and chemical reactivity of the remote troposphere defined by aircraft measurements – corrected, Atmos. Chem. Phys., 23, 99–117, https://doi.org/10.5194/acp-23-99-2023, 2023.
Harwood, M. H., Roberts, J. M., Frost, G. J., Ravishankara, A. R., and Burkholder, J. B.: Photochemical Studies of CH3C(O)OONO2 (PAN) and CH3CH2C(O)OONO2 (PPN): NO3 Quantum Yields, J. Phys. Chem. A, 107, 1148–1154, https://doi.org/10.1021/jp0264230, 2003.
Hudman, R. C., Jacob, D. J., Turquety, S., Leibensperger, E. M., Murray, L. T., Wu, S., Gilliland, A. B., Avery, M., Bertram, T. H., Brune, W., Cohen, R. C., Dibb, J. E., Flocke, F. M., Fried, A., Holloway, J., Neuman, J. A., Orville, R., Perring, A., Ren, X., Sachse, G. W., Singh, H. B., Swanson, A., and Wooldridge, P. J.: Surface and lightning sources of nitrogen oxides over the United States: Magnitudes, chemical evolution, and outflow, J. Geophys. Res.-Atmos., 112, 2006JD007912, https://doi.org/10.1029/2006JD007912, 2007.
INTEX-A Science Team: INTEX-A Aircraft Data, NASA [data set], https://doi.org/10.5067/ASDCDAAC/INTEXA/0008, 2006.
INTEX-B Science Team: INTEX-B Aircraft Data, NASA [data set], https://doi.org/10.5067/AIRCRAFT/INTEXB/AEROSOL-TRACEGAS, 2011.
Jacob, D. J., Crawford, J. H., Maring, H., Clarke, A. D., Dibb, J. E., Emmons, L. K., Ferrare, R. A., Hostetler, C. A., Russell, P. B., Singh, H. B., Thompson, A. M., Shaw, G. E., McCauley, E., Pederson, J. R., and Fisher, J. A.: The Arctic Research of the Composition of the Troposphere from Aircraft and Satellites (ARCTAS) mission: design, execution, and first results, Atmos. Chem. Phys., 10, 5191–5212, https://doi.org/10.5194/acp-10-5191-2010, 2010.
Jaeglé, L., Jacob, D. J., Wang, Y., Weinheimer, A. J., Ridley, B. A., Campos, T. L., Sachse, G. W., and Hagen, D. E.: Sources and chemistry of NOx in the upper troposphere over the United States, Geophys. Res. Lett., 25, 1705–1708, https://doi.org/10.1029/97GL03591, 1998.
Jain, A. K., Tao, Z., Yang, X., and Gillespie, C.: Estimates of global biomass burning emissions for reactive greenhouse gases (CO, NMHCs, and NOx) and CO2, J. Geophys. Res., 111, D06304, https://doi.org/10.1029/2005JD006237, 2006.
Jin, X., Zhu, Q., and Cohen, R. C.: Direct estimates of biomass burning NOx emissions and lifetimes using daily observations from TROPOMI, Atmos. Chem. Phys., 21, 15569–15587, https://doi.org/10.5194/acp-21-15569-2021, 2021.
Kang, Y., Tang, G., Li, Q., Liu, B., Cao, J., Hu, Q., and Wang, Y.: Evaluation and Evolution of MAX-DOAS-observed Vertical NO2 Profiles in Urban Beijing, Adv. Atmos. Sci., 38, 1188–1196, https://doi.org/10.1007/s00376-021-0370-1, 2021.
Karl, T. G., Christian, T. J., Yokelson, R. J., Artaxo, P., Hao, W. M., and Guenther, A.: The Tropical Forest and Fire Emissions Experiment: method evaluation of volatile organic compound emissions measured by PTR-MS, FTIR, and GC from tropical biomass burning, Atmos. Chem. Phys., 7, 5883–5897, https://doi.org/10.5194/acp-7-5883-2007, 2007.
Kasibhatla, P., Sherwen, T., Evans, M. J., Carpenter, L. J., Reed, C., Alexander, B., Chen, Q., Sulprizio, M. P., Lee, J. D., Read, K. A., Bloss, W., Crilley, L. R., Keene, W. C., Pszenny, A. A. P., and Hodzic, A.: Global impact of nitrate photolysis in sea-salt aerosol on NOx, OH, and O3 in the marine boundary layer, Atmos. Chem. Phys., 18, 11185–11203, https://doi.org/10.5194/acp-18-11185-2018, 2018.
Kawakami, S., Kondo, Y., Koike, M., Nakajima, H., Gregory, G. L., Sachse, G. W., Newell, R. E., Browell, E. V., Blake, D. R., Rodriguez, J. M., and Merrill, J. T.: Impact of lightning and convection on reactive nitrogen in the tropical free troposphere, J. Geophys. Res.-Atmos., 102, 28367–28384, https://doi.org/10.1029/97JD02073, 1997.
Keita, S., Liousse, C., Assamoi, E.-M., Doumbia, T., N'Datchoh, E. T., Gnamien, S., Elguindi, N., Granier, C., and Yoboué, V.: African anthropogenic emissions inventory for gases and particles from 1990 to 2015, Earth Syst. Sci. Data, 13, 3691–3705, https://doi.org/10.5194/essd-13-3691-2021, 2021.
Kenagy, H. S., Sparks, T. L., Ebben, C. J., Wooldrige, P. J., Lopez-Hilfiker, F. D., Lee, B. H., Thornton, J. A., McDuffie, E. E., Fibiger, D. L., Brown, S. S., Montzka, D. D., Weinheimer, A. J., Schroder, J. C., Campuzano-Jost, P., Day, D. A., Jimenez, J. L., Dibb, J. E., Campos, T., Shah, V., Jaeglé, L., and Cohen, R. C.: NOx Lifetime and NOy Partitioning During WINTER, J. Geophys. Res.-Atmos., 123, 9813–9827, https://doi.org/10.1029/2018JD028736, 2018.
Kotamarthi, V. R., Gaffney, J. S., Marley, N. A., and Doskey, P. V.: Heterogeneous NOx chemistry in the polluted PBL, Atmos. Environ., 35, 4489–4498, https://doi.org/10.1016/S1352-2310(01)00221-7, 2001.
Liu, S., Valks, P., Pinardi, G., Xu, J., Chan, K. L., Argyrouli, A., Lutz, R., Beirle, S., Khorsandi, E., Baier, F., Huijnen, V., Bais, A., Donner, S., Dörner, S., Gratsea, M., Hendrick, F., Karagkiozidis, D., Lange, K., Piters, A. J. M., Remmers, J., Richter, A., Van Roozendael, M., Wagner, T., Wenig, M., and Loyola, D. G.: An improved TROPOMI tropospheric NO2 research product over Europe, Atmos. Meas. Tech., 14, 7297–7327, https://doi.org/10.5194/amt-14-7297-2021, 2021.
Lloret, J. and Valiela, I.: Unprecedented decrease in deposition of nitrogen oxides over North America: the relative effects of emission controls and prevailing air-mass trajectories, Biogeochemistry, 129, 165–180, https://doi.org/10.1007/s10533-016-0225-5, 2016.
Loyola, D. G., Gimeno García, S., Lutz, R., Argyrouli, A., Romahn, F., Spurr, R. J. D., Pedergnana, M., Doicu, A., Molina García, V., and Schüssler, O.: The operational cloud retrieval algorithms from TROPOMI on board Sentinel-5 Precursor, Atmos. Meas. Tech., 11, 409–427, https://doi.org/10.5194/amt-11-409-2018, 2018.
Lu, G., Marais, E. A., Vohra, K., Horner, R. P., Zhang, D., Martin, R. V., and Guttikunda, S. K.: Near-Automated Estimate of City Nitrogen Oxides Emissions Applied to South and Southeast Asia, ESS Open Archive, https://doi.org/10.22541/essoar.171033213.36792308/v1, 13 March 2024.
Marais, E. and Horner, R.: Vertical profiles of global seasonal mean nitrogen dioxide in five distinct layers in the troposphere, University College London [data set], https://doi.org/10.5522/04/25782336, 2024.
Marais, E. A. and Wiedinmyer, C.: Air Quality Impact of Diffuse and Inefficient Combustion Emissions in Africa (DICE-Africa), Environ. Sci. Technol., 50, 10739–10745, https://doi.org/10.1021/acs.est.6b02602, 2016.
Marais, E. A., Jacob, D. J., Jimenez, J. L., Campuzano-Jost, P., Day, D. A., Hu, W., Krechmer, J., Zhu, L., Kim, P. S., Miller, C. C., Fisher, J. A., Travis, K., Yu, K., Hanisco, T. F., Wolfe, G. M., Arkinson, H. L., Pye, H. O. T., Froyd, K. D., Liao, J., and McNeill, V. F.: Aqueous-phase mechanism for secondary organic aerosol formation from isoprene: application to the southeast United States and co-benefit of SO2 emission controls, Atmos. Chem. Phys., 16, 1603–1618, https://doi.org/10.5194/acp-16-1603-2016, 2016.
Marais, E. A., Jacob, D. J., Choi, S., Joiner, J., Belmonte-Rivas, M., Cohen, R. C., Beirle, S., Murray, L. T., Schiferl, L. D., Shah, V., and Jaeglé, L.: Nitrogen oxides in the global upper troposphere: interpreting cloud-sliced NO2 observations from the OMI satellite instrument, Atmos. Chem. Phys., 18, 17017–17027, https://doi.org/10.5194/acp-18-17017-2018, 2018.
Marais, E. A., Roberts, J. F., Ryan, R. G., Eskes, H., Boersma, K. F., Choi, S., Joiner, J., Abuhassan, N., Redondas, A., Grutter, M., Cede, A., Gomez, L., and Navarro-Comas, M.: New observations of NO2 in the upper troposphere from TROPOMI, Atmos. Meas. Tech., 14, 2389–2408, https://doi.org/10.5194/amt-14-2389-2021, 2021.
McDuffie, E. E., Smith, S. J., O'Rourke, P., Tibrewal, K., Venkataraman, C., Marais, E. A., Zheng, B., Crippa, M., Brauer, M., and Martin, R. V.: A global anthropogenic emission inventory of atmospheric pollutants from sector- and fuel-specific sources (1970–2017): an application of the Community Emissions Data System (CEDS), Earth Syst. Sci. Data, 12, 3413–3442, https://doi.org/10.5194/essd-12-3413-2020, 2020.
Meng, J., Martin, R. V., Ginoux, P., Hammer, M., Sulprizio, M. P., Ridley, D. A., and van Donkelaar, A.: Grid-independent high-resolution dust emissions (v1.0) for chemical transport models: application to GEOS-Chem (12.5.0), Geosci. Model Dev., 14, 4249–4260, https://doi.org/10.5194/gmd-14-4249-2021, 2021.
Moxim, W. J., Levy, H., and Kasibhatla, P. S.: Simulated global tropospheric PAN: Its transport and impact on NOx, J. Geophys. Res.-Atmos., 101, 12621–12638, https://doi.org/10.1029/96JD00338, 1996.
Murphy, J. G., Thornton, J. A., Wooldridge, P. J., Day, D. A., Rosen, R. S., Cantrell, C., Shetter, R. E., Lefer, B., and Cohen, R. C.: Measurements of the sum of HO2NO2 and CH3O2NO2 in the remote troposphere, Atmos. Chem. Phys., 4, 377–384, https://doi.org/10.5194/acp-4-377-2004, 2004.
Murray, L. T., Jacob, D. J., Logan, J. A., Hudman, R. C., and Koshak, W. J.: Optimized regional and interannual variability of lightning in a global chemical transport model constrained by LIS/OTD satellite data, J. Geophys. Res.-Atmos., 117, D20, https://doi.org/10.1029/2012JD017934, 2012.
Nault, B. A., Garland, C., Pusede, S. E., Wooldridge, P. J., Ullmann, K., Hall, S. R., and Cohen, R. C.: Measurements of CH3O2NO2 in the upper troposphere, Atmos. Meas. Tech., 8, 987–997, https://doi.org/10.5194/amt-8-987-2015, 2015.
Nault, Benjamin. A., Garland, C., Wooldridge, P. J., Brune, W. H., Campuzano-Jost, P., Crounse, J. D., Day, D. A., Dibb, J., Hall, S. R., Huey, L. G., Jimenez, J. L., Liu, X., Mao, J., Mikoviny, T., Peischl, J., Pollack, I. B., Ren, X., Ryerson, T. B., Scheuer, E., Ullmann, K., Wennberg, P. O., Wisthaler, A., Zhang, L., and Cohen, R. C.: Observational Constraints on the Oxidation of NOx in the Upper Troposphere, J. Phys. Chem. A, 120, 1468–1478, https://doi.org/10.1021/acs.jpca.5b07824, 2016.
Ossohou, M., Galy-Lacaux, C., Yoboué, V., Hickman, J. E., Gardrat, E., Adon, M., Darras, S., Laouali, D., Akpo, A., Ouafo, M., Diop, B., and Opepa, C.: Trends and seasonal variability of atmospheric NO2 and HNO3 concentrations across three major African biomes inferred from long-term series of ground-based and satellite measurements, Atmos. Environ., 207, 148–166, https://doi.org/10.1016/j.atmosenv.2019.03.027, 2019.
Petzold, A., Thouret, V., Gerbig, C., Zahn, A., Brenninkmeijer, C. A. M., Gallagher, M., Hermann, M., Pontaud, M., Ziereis, H., Boulanger, D., Marshall, J., Nédélec, P., Smit, H. G. J., Friess, U., Flaud, J.-M., Wahner, A., Cammas, J.-P., Volz-Thomas, A., and Team, I.: Global-scale atmosphere monitoring by in-service aircraft – current achievements and future prospects of the European Research Infrastructure IAGOS, Tellus B, 67, 28452, https://doi.org/10.3402/tellusb.v67.28452, 2015.
Pickering, K. E., Wang, Y., Tao, W.-K., Price, C., and Müller, J.-F.: Vertical distributions of lightning NOx for use in regional and global chemical transport models, J. Geophys. Res.-Atmos,, 103, 31203–31216, https://doi.org/10.1029/98JD02651, 1998.
Pinardi, G., Van Roozendael, M., Hendrick, F., Theys, N., Abuhassan, N., Bais, A., Boersma, F., Cede, A., Chong, J., Donner, S., Drosoglou, T., Dzhola, A., Eskes, H., Frieß, U., Granville, J., Herman, J. R., Holla, R., Hovila, J., Irie, H., Kanaya, Y., Karagkiozidis, D., Kouremeti, N., Lambert, J.-C., Ma, J., Peters, E., Piters, A., Postylyakov, O., Richter, A., Remmers, J., Takashima, H., Tiefengraber, M., Valks, P., Vlemmix, T., Wagner, T., and Wittrock, F.: Validation of tropospheric NO2 column measurements of GOME-2A and OMI using MAX-DOAS and direct sun network observations, Atmos. Meas. Tech., 13, 6141–6174, https://doi.org/10.5194/amt-13-6141-2020, 2020.
Poulida, O., Dickerson, R. R., and Heymsfield, A.: Stratosphere-troposphere exchange in a midlatitude mesoscale convective complex: 1. Observations, J. Geophys. Res.-Atmos., 101, 6823–6836, https://doi.org/10.1029/95JD03523, 1996.
Reed, C., Evans, M. J., Di Carlo, P., Lee, J. D., and Carpenter, L. J.: Interferences in photolytic NO2 measurements: explanation for an apparent missing oxidant?, Atmos. Chem. Phys., 16, 4707–4724, https://doi.org/10.5194/acp-16-4707-2016, 2016.
Romer, P. S., Wooldridge, P. J., Crounse, J. D., Kim, M. J., Wennberg, P. O., Dibb, J. E., Scheuer, E., Blake, D. R., Meinardi, S., Brosius, A. L., Thames, A. B., Miller, D. O., Brune, W. H., Hall, S. R., Ryerson, T. B., and Cohen, R. C.: Constraints on Aerosol Nitrate Photolysis as a Potential Source of HONO and NOx, Environ. Sci. Technol., 52, 13738–13746, https://doi.org/10.1021/acs.est.8b03861, 2018.
Ryan, R. G., Marais, E. A., Gershenson-Smith, E., Ramsay, R., Muller, J.-P., Tirpitz, J.-L., and Frieß, U.: Measurement report: MAX-DOAS measurements characterise Central London ozone pollution episodes during 2022 heatwaves, Atmos. Chem. Phys., 23, 7121–7139, https://doi.org/10.5194/acp-23-7121-2023, 2023.
Ryerson, T. B., Williams, E. J., and Fehsenfeld, F. C.: An efficient photolysis system for fast-response NO2 measurements, J. Geophys. Res.-Atmos., 105, 26447–26461, https://doi.org/10.1029/2000JD900389, 2000.
Sahu, L. K. and Sheel, V.: Spatio-temporal variation of biomass burning sources over South and Southeast Asia, J. Atmos. Chem., 71, 1–19, https://doi.org/10.1007/s10874-013-9275-4, 2014.
Scharko, N. K., Berke, A. E., and Raff, J. D.: Release of Nitrous Acid and Nitrogen Dioxide from Nitrate Photolysis in Acidic Aqueous Solutions, Environ. Sci. Technol., 48, 11991–12001, https://doi.org/10.1021/es503088x, 2014.
Schreier, S. F., Peters, E., Richter, A., Lampel, J., Wittrock, F., and Burrows, J. P.: Ship-based MAX-DOAS measurements of tropospheric NO2 and SO2 in the South China and Sulu Sea, Atmos. Environ., 102, 331–343, https://doi.org/10.1016/j.atmosenv.2014.12.015, 2015.
SEAC4RS Science Team: SEAC4RS Field Campaign Data, NASA [data set], https://doi.org/10.5067/AIRCRAFT/SEAC4RS/AEROSOL-TRACEGAS-CLOUD, 2014.
Sen, P. K.: Estimates of the Regression Coefficient Based on Kendall's Tau, J. Am. Stat. Assoc., 63, 1379–1389, https://doi.org/10.1080/01621459.1968.10480934, 1968.
Shah, V., Jacob, D. J., Dang, R., Lamsal, L. N., Strode, S. A., Steenrod, S. D., Boersma, K. F., Eastham, S. D., Fritz, T. M., Thompson, C., Peischl, J., Bourgeois, I., Pollack, I. B., Nault, B. A., Cohen, R. C., Campuzano-Jost, P., Jimenez, J. L., Andersen, S. T., Carpenter, L. J., Sherwen, T., and Evans, M. J.: Nitrogen oxides in the free troposphere: implications for tropospheric oxidants and the interpretation of satellite NO2 measurements, Atmos. Chem. Phys., 23, 1227–1257, https://doi.org/10.5194/acp-23-1227-2023, 2023.
Silvern, R. F., Jacob, D. J., Travis, K. R., Sherwen, T., Evans, M. J., Cohen, R. C., Laughner, J. L., Hall, S. R., Ullmann, K., Crounse, J. D., Wennberg, P. O., Peischl, J., and Pollack, I. B.: Observed NO/NO2 Ratios in the Upper Troposphere Imply Errors in NO-NO2-O3 Cycling Kinetics or an Unaccounted NOx Reservoir, Geophys. Res. Lett., 45, 4466–4474, https://doi.org/10.1029/2018GL077728, 2018.
Singh, H. B., Thompson, A. M., and Schlager, H.: SONEX airborne mission and coordinated POLINAT-2 activity: Overview and accomplishments, Geophys. Res. Lett., 26, 3053–3056, https://doi.org/10.1029/1999GL900588, 1999.
Singh, H. B., Brune, W. H., Crawford, J. H., Jacob, D. J., and Russell, P. B.: Overview of the summer 2004 Intercontinental Chemical Transport Experiment–North America (INTEX-A), J. Geophys. Res., 111, D24S01, https://doi.org/10.1029/2006JD007905, 2006.
Singh, H. B., Brune, W. H., Crawford, J. H., Flocke, F., and Jacob, D. J.: Chemistry and transport of pollution over the Gulf of Mexico and the Pacific: spring 2006 INTEX-B campaign overview and first results, Atmos. Chem. Phys., 9, 2301–2318, https://doi.org/10.5194/acp-9-2301-2009, 2009.
Stettler, M. E. J., Eastham, S., and Barrett, S. R. H.: Air quality and public health impacts of UK airports. Part I: Emissions, Atmos. Environ., 45, 5415–5424, https://doi.org/10.1016/j.atmosenv.2011.07.012, 2011.
Stratmann, G., Ziereis, H., Stock, P., Brenninkmeijer, C. A. M., Zahn, A., Rauthe-Schöch, A., Velthoven, P. V., Schlager, H., and Volz-Thomas, A.: NO and NOy in the upper troposphere: Nine years of CARIBIC measurements onboard a passenger aircraft, Atmos. Environ., 133, 93–111, https://doi.org/10.1016/j.atmosenv.2016.02.035, 2016.
Theil H.: A Rank-Invariant Method of Linear and Polynomial Regression Analysis, Part I, Proceedings of the Royal Netherlands Academy Science, 53, 386–292, 1950.
The International GEOS-Chem User Community: GEOS-Chem Version 13.3.4, Zenodo [code], https://doi.org/10.5281/zenodo.5764874, 2021.
Thompson, C. R., Wofsy, S. C., Prather, M. J., Newman, P. A., Hanisco, T. F., Ryerson, T. B., Fahey, D. W., Apel, E. C., Brock, C. A., Brune, W. H., Froyd, K., Katich, J. M., Nicely, J. M., Peischl, J., Ray, E., Veres, P. R., Wang, S., Allen, H. M., Asher, E., Bian, H., Blake, D., Bourgeois, I., Budney, J., Bui, T. P., Butler, A., Campuzano-Jost, P., Chang, C., Chin, M., Commane, R., Correa, G., Crounse, J. D., Daube, B., Dibb, J. E., DiGangi, J. P., Diskin, G. S., Dollner, M., Elkins, J. W., Fiore, A. M., Flynn, C. M., Guo, H., Hall, S. R., Hannun, R. A., Hills, A., Hintsa, E. J., Hodzic, A., Hornbrook, R. S., Huey, L. G., Jimenez, J. L., Keeling, R. F., Kim, M. J., Kupc, A., Lacey, F., Lait, L. R., Lamarque, J.-F., Liu, J., McKain, K., Meinardi, S., Miller, D. O., Montzka, S. A., Moore, F. L., Morgan, E. J., Murphy, D. M., Murray, L. T., Nault, B. A., Neuman, J. A., Nguyen, L., Gonzalez, Y., Rollins, A., Rosenlof, K., Sargent, M., Schill, G., Schwarz, J. P., Clair, J. M. St., Steenrod, S. D., Stephens, B. B., Strahan, S. E., Strode, S. A., Sweeney, C., Thames, A. B., Ullmann, K., Wagner, N., Weber, R., Weinzierl, B., Wennberg, P. O., Williamson, C. J., Wolfe, G. M., and Zeng, L.: The NASA Atmospheric Tomography (ATom) Mission: Imaging the Chemistry of the Global Atmosphere, B. Am. Meteorol. Soc., 103, E761–E790, https://doi.org/10.1175/BAMS-D-20-0315.1, 2022.
Tirpitz, J.-L.: Enhancing MAX-DOAS atmospheric remote sensing by multispectral polarimetry, PhD thesis, University of Heidelberg, Heidelberg, https://doi.org/10.11588/heidok.00030159, 241 pp., 2021.
Toon, O. B., Maring, H., Dibb, J., Ferrare, R., Jacob, D. J., Jensen, E. J., Luo, Z. J., Mace, G. G., Pan, L. L., Pfister, L., Rosenlof, K. H., Redemann, J., Reid, J. S., Singh, H. B., Thompson, A. M., Yokelson, R., Minnis, P., Chen, G., Jucks, K. W., and Pszenny, A.: Planning, implementation, and scientific goals of the Studies of Emissions and Atmospheric Composition, Clouds and Climate Coupling by Regional Surveys (SEAC4RS) field mission, J. Geophys. Res.-Atmos., 121, 4967–5009, https://doi.org/10.1002/2015JD024297, 2016.
Torres, O., Bhartia, P. K., Jethva, H., and Ahn, C.: Impact of the ozone monitoring instrument row anomaly on the long-term record of aerosol products, Atmos. Meas. Tech., 11, 2701–2715, https://doi.org/10.5194/amt-11-2701-2018, 2018.
Travis, K. R., Jacob, D. J., Fisher, J. A., Kim, P. S., Marais, E. A., Zhu, L., Yu, K., Miller, C. C., Yantosca, R. M., Sulprizio, M. P., Thompson, A. M., Wennberg, P. O., Crounse, J. D., St. Clair, J. M., Cohen, R. C., Laughner, J. L., Dibb, J. E., Hall, S. R., Ullmann, K., Wolfe, G. M., Pollack, I. B., Peischl, J., Neuman, J. A., and Zhou, X.: Why do models overestimate surface ozone in the Southeast United States?, Atmos. Chem. Phys., 16, 13561–13577, https://doi.org/10.5194/acp-16-13561-2016, 2016.
Travis, K. R., Heald, C. L., Allen, H. M., Apel, E. C., Arnold, S. R., Blake, D. R., Brune, W. H., Chen, X., Commane, R., Crounse, J. D., Daube, B. C., Diskin, G. S., Elkins, J. W., Evans, M. J., Hall, S. R., Hintsa, E. J., Hornbrook, R. S., Kasibhatla, P. S., Kim, M. J., Luo, G., McKain, K., Millet, D. B., Moore, F. L., Peischl, J., Ryerson, T. B., Sherwen, T., Thames, A. B., Ullmann, K., Wang, X., Wennberg, P. O., Wolfe, G. M., and Yu, F.: Constraining remote oxidation capacity with ATom observations, Atmos. Chem. Phys., 20, 7753–7781, https://doi.org/10.5194/acp-20-7753-2020, 2020.
TROPOMI: Nitrogen Dioxide (NO2) Level 2 data product (PAL), Copernicus Open Access Hub [data set], https://data-portal.s5p-pal.com/ (last access: 17 February 2022), 2018.
TROPOMI: Nitrogen Dioxide (NO2) Level 2 data product (OFFL), Copernicus Open Access Hub [data set], https://s5phub.copernicus.eu/dhus/#/homeTS3 (last access: 7 July 2022); now available at: https://dataspace.copernicus.eu/browser/, 2021.
van der Velde, I. R., van der Werf, G. R., Houweling, S., Eskes, H. J., Veefkind, J. P., Borsdorff, T., and Aben, I.: Biomass burning combustion efficiency observed from space using measurements of CO and NO2 by the TROPOspheric Monitoring Instrument (TROPOMI), Atmos. Chem. Phys., 21, 597–616, https://doi.org/10.5194/acp-21-597-2021, 2021.
van der Werf, G. R., Randerson, J. T., Giglio, L., Collatz, G. J., Kasibhatla, P. S., and Arellano Jr., A. F.: Interannual variability in global biomass burning emissions from 1997 to 2004, Atmos. Chem. Phys., 6, 3423–3441, https://doi.org/10.5194/acp-6-3423-2006, 2006.
van Geffen, J., Eskes, H., Compernolle, S., Pinardi, G., Verhoelst, T., Lambert, J.-C., Sneep, M., ter Linden, M., Ludewig, A., Boersma, K. F., and Veefkind, J. P.: Sentinel-5P TROPOMI NO2 retrieval: impact of version v2.2 improvements and comparisons with OMI and ground-based data, Atmos. Meas. Tech., 15, 2037–2060, https://doi.org/10.5194/amt-15-2037-2022, 2022.
Verhoelst, T., Compernolle, S., Pinardi, G., Lambert, J.-C., Eskes, H. J., Eichmann, K.-U., Fjæraa, A. M., Granville, J., Niemeijer, S., Cede, A., Tiefengraber, M., Hendrick, F., Pazmiño, A., Bais, A., Bazureau, A., Boersma, K. F., Bognar, K., Dehn, A., Donner, S., Elokhov, A., Gebetsberger, M., Goutail, F., Grutter de la Mora, M., Gruzdev, A., Gratsea, M., Hansen, G. H., Irie, H., Jepsen, N., Kanaya, Y., Karagkiozidis, D., Kivi, R., Kreher, K., Levelt, P. F., Liu, C., Müller, M., Navarro Comas, M., Piters, A. J. M., Pommereau, J.-P., Portafaix, T., Prados-Roman, C., Puentedura, O., Querel, R., Remmers, J., Richter, A., Rimmer, J., Rivera Cárdenas, C., Saavedra de Miguel, L., Sinyakov, V. P., Stremme, W., Strong, K., Van Roozendael, M., Veefkind, J. P., Wagner, T., Wittrock, F., Yela González, M., and Zehner, C.: Ground-based validation of the Copernicus Sentinel-5P TROPOMI NO2 measurements with the NDACC ZSL-DOAS, MAX-DOAS and Pandonia global networks, Atmos. Meas. Tech., 14, 481–510, https://doi.org/10.5194/amt-14-481-2021, 2021.
Vinken, G. C. M., Boersma, K. F., van Donkelaar, A., and Zhang, L.: Constraints on ship NOx emissions in Europe using GEOS-Chem and OMI satellite NO2 observations, Atmos. Chem. Phys., 14, 1353–1369, https://doi.org/10.5194/acp-14-1353-2014, 2014.
Vohra, K., Marais, E. A., Bloss, W. J., Schwartz, J., Mickley, L. J., Van Damme, M., Clarisse, L., and Coheur, P.-F.: Rapid rise in premature mortality due to anthropogenic air pollution in fast-growing tropical cities from 2005 to 2018, Sci. Adv., 8, eabm4435, https://doi.org/10.1126/sciadv.abm4435, 2022.
Wang, P., Stammes, P., van der A, R., Pinardi, G., and van Roozendael, M.: FRESCO+: an improved O2 A-band cloud retrieval algorithm for tropospheric trace gas retrievals, Atmos. Chem. Phys., 8, 6565–6576, https://doi.org/10.5194/acp-8-6565-2008, 2008.
Wang, P.-H., Minnis, P., McCormick, M. P., Kent, G. S., and Skeens, K. M.: A 6-year climatology of cloud occurrence frequency from Stratospheric Aerosol and Gas Experiment II observations (1985–1990), J. Geophys. Res.-Atmos., 101, 29407–29429, https://doi.org/10.1029/96JD01780, 1996.
Wang, Y., Dörner, S., Donner, S., Böhnke, S., De Smedt, I., Dickerson, R. R., Dong, Z., He, H., Li, Z., Li, Z., Li, D., Liu, D., Ren, X., Theys, N., Wang, Y., Wang, Y., Wang, Z., Xu, H., Xu, J., and Wagner, T.: Vertical profiles of NO2, SO2, HONO, HCHO, CHOCHO and aerosols derived from MAX-DOAS measurements at a rural site in the central western North China Plain and their relation to emission sources and effects of regional transport, Atmos. Chem. Phys., 19, 5417–5449, https://doi.org/10.5194/acp-19-5417-2019, 2019.
Weng, H., Lin, J., Martin, R., Millet, D. B., Jaeglé, L., Ridley, D., Keller, C., Li, C., Du, M., and Meng, J.: Global high-resolution emissions of soil NOx, sea salt aerosols, and biogenic volatile organic compounds, Sci. Data, 7, 148, https://doi.org/10.1038/s41597-020-0488-5, 2020.
van der Werf, G. R., Randerson, J. T., Giglio, L., van Leeuwen, T. T., Chen, Y., Rogers, B. M., Mu, M., van Marle, M. J. E., Morton, D. C., Collatz, G. J., Yokelson, R. J., and Kasibhatla, P. S.: Global fire emissions estimates during 1997–2016, Earth Syst. Sci. Data, 9, 697–720, https://doi.org/10.5194/essd-9-697-2017, 2017.
Wild, O., Prather, M. J., and Akimoto, H.: Indirect long-term global radiative cooling from NOx Emissions, Geophys. Res. Lett., 28, 1719–1722, https://doi.org/10.1029/2000GL012573, 2001.
Yang, L. H., Jacob, D. J., Colombi, N. K., Zhai, S., Bates, K. H., Shah, V., Beaudry, E., Yantosca, R. M., Lin, H., Brewer, J. F., Chong, H., Travis, K. R., Crawford, J. H., Lamsal, L. N., Koo, J.-H., and Kim, J.: Tropospheric NO2 vertical profiles over South Korea and their relation to oxidant chemistry: implications for geostationary satellite retrievals and the observation of NO2 diurnal variation from space, Atmos. Chem. Phys., 23, 2465–2481, https://doi.org/10.5194/acp-23-2465-2023, 2023.
Ye, C., Zhang, N., Gao, H., and Zhou, X.: Photolysis of Particulate Nitrate as a Source of HONO and NOx, Environ. Sci. Technol., 51, 6849–6856, https://doi.org/10.1021/acs.est.7b00387, 2017.
Zhao, B., Wang, S. X., Liu, H., Xu, J. Y., Fu, K., Klimont, Z., Hao, J. M., He, K. B., Cofala, J., and Amann, M.: NOx emissions in China: historical trends and future perspectives, Atmos. Chem. Phys., 13, 9869–9897, https://doi.org/10.5194/acp-13-9869-2013, 2013.
Ziemke, J. R., Chandra, S., and Bhartia, P. K.: “Cloud slicing”: A new technique to derive upper tropospheric ozone from satellite measurements, J. Geophys. Res.-Atmos., 106, 9853–9867, https://doi.org/10.1029/2000JD900768, 2001.
Zien, A. W., Richter, A., Hilboll, A., Blechschmidt, A.-M., and Burrows, J. P.: Systematic analysis of tropospheric NO2 long-range transport events detected in GOME-2 satellite data, Atmos. Chem. Phys., 14, 7367–7396, https://doi.org/10.5194/acp-14-7367-2014, 2014.