the Creative Commons Attribution 4.0 License.
the Creative Commons Attribution 4.0 License.
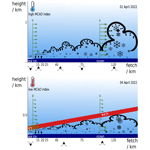
Clouds and precipitation in the initial phase of marine cold-air outbreaks as observed by airborne remote sensing
Imke Schirmacher
Sabrina Schnitt
Marcus Klingebiel
Nina Maherndl
Benjamin Kirbus
André Ehrlich
Mario Mech
Susanne Crewell
Marine cold-air outbreaks (MCAOs) strongly affect the Arctic water cycle and, thus, climate through large-scale air mass transformations. The description of air mass transformations is still challenging, partly because previous observations do not resolve fine scales, particularly for the initial development of an MCAO, and due to a lack of information about the thermodynamical evolution starting over sea ice and continuing over open ocean and associated cloud microphysical properties. Therefore, we focus on the crucial initial development within the first 200 km over open water for two case studies in April 2022 during the HALO-(AC)3 campaign (named after the High Altitude and Long Range Research Aircraft and Transregional Collaborative Research Centre ArctiC Amplification: Climate Relevant Atmospheric and SurfaCe Processes and Feedback Mechanisms (AC)3). The two events, just 3 d apart, belong to a particularly long-lasting MCAO and occurred under relatively similar thermodynamic conditions. Even though both events were stronger than the climatological 75th percentile of that period, the first event was characterized by colder air masses from the central Arctic which led to an MCAO index twice as high compared to that of the second event.
The evolution and structure were assessed by flight legs crossing the Fram Strait multiple times at the same location, sampling perpendicularly to the cloud streets. Airborne remote sensing and in situ measurements were used to build statistical descriptions of the boundary layer, dynamics, clouds, and precipitation. For this purpose, we established a novel approach based solely on radar reflectivity measurements to detect roll circulation that forms cloud streets. The two cases exhibit different properties of clouds, riming, and roll circulations, though the width of the roll circulation is similar. For the stronger event, cloud tops are higher; more liquid-topped clouds exist; the liquid water path, mean radar reflectivity, precipitation rate, and precipitation occurrence have increased; and riming is active. The variability in rime mass has the same horizontal scale as the roll circulation, implying the importance of roll circulation on cloud microphysics and precipitation.
Boundary layer and cloud properties evolve with distance over open water, as seen by, e.g., cloud top height rising. In general, cloud streets form after traveling 15 km over open water. After 20 km, this formation enhances cloud cover to just below 100 %. After around 30 km, precipitation forms, though for the weaker event, the development of precipitation is shifted to larger distances. Within our analysis, we developed statistical descriptions of various parameters (i) within the roll circulation and (ii) as a function of distance over open water. These detailed cloud metrics are particularly well suited for the evaluation of cloud-resolving models close to the sea ice edge to evaluate their representation of dynamics and microphysics.
- Article
(6609 KB) - Full-text XML
-
Supplement
(462 KB) - BibTeX
- EndNote
Marine cold-air outbreaks (MCAOs) are strong air mass transformations. During Arctic MCAOs, cold and dry air flows from the ice-covered central Arctic southward over the open ocean. There, cloud streets form that are clearly visible in satellite images and transform to cellular convection downstream under extreme surface heat fluxes (Brümmer, 1996). Especially over open ocean, cloud streets have important implications for the radiative surface energy budget due to their high albedo induced by liquid cloud tops (Geerts et al., 2022). Moreover, their long lifetimes affect precipitation evolution and characteristics (Abel et al., 2017) and, thus, the Arctic water cycle. Arctic MCAOs can also strongly influence the weather in the mid-latitudes (Turner and Marshall, 2011).
The Arctic is a hot spot with respect to climate change, most pronounced by strong surface temperature increases and sea ice decline (Wendisch et al., 2023). Dahlke et al. (2022) also showed significant shifts in the occurrence of MCAOs, i.e., decreases in early winter and increases in late winter. These shifts are possibly caused by changes in synoptic circulation patterns and feedback mechanisms involving retreating sea ice. How MCAO characteristics will develop in the future will require improved modeling capabilities (Geerts et al., 2022) and a better process understanding of these air mass transformations, including cloud phase changes (Pithan et al., 2018). To resolve the mesoscale cloud organization in MCAOs, large-eddy simulations (LESs) are required. Yet, the transition between organizational states is especially difficult to model as initial conditions, turbulence, cloud microphysics, and large-scale flow interact. Furthermore, small-scale surface heterogeneity in the marginal sea ice zone (MIZ) is important for the formation of rolls (Gryschka et al., 2014), whereby the exposure of air to open water in the MIZ plays an important role (Spensberger and Spengler, 2021). LES studies also highlight the importance of mixed-phase microphysical processes in preconditioning the transition of cloud organization (Abel et al., 2017; Tornow et al., 2021). Model settings like the employed ice microphysical scheme and model resolution affect the timing of transformation that differs between the models, e.g., when the ice phase is permitted (de Roode et al., 2019). Meanwhile, a higher resolution evokes roll convection at smaller distances to the sea ice edge and increases the precipitation amount (Spensberger and Spengler, 2021). So far, no consensus has been reached and progress is delayed by the lack of observations for models.
The first airborne in situ measurements during the Convection and Turbulence (KonTur) experiment (Markson, 1975; Brummer et al., 1982; Brümmer et al., 1985); ARKTIS '88 (Brümmer et al., 1992), 1991, and 1993 (Brümmer, 1999); and Marginal Ice Zone Experiment (MIZEX; Walter and Overland, 1984) investigated the mesoscale roll convection inside the atmospheric boundary layer (ABL) that is strengthened by thermal instability when air flows from the sea ice over the open water (Atkinson and Wu Zhang, 1996). Brümmer (1996) and Müller et al. (1999) showed how the ABL was modified as a function of distance from the sea ice within the first 300 km under the influence of strong surface heat fluxes from open water. While cloud reflectance measurements by satellites have provided important insights into the geometrical appearance of MCAOs since their beginning, recent studies such as Murray-Watson et al. (2023), Wu and Ovchinnikov (2022), and Tornow et al. (2023) quantitatively studied cloud development in a quasi-Lagrangian way. Using back trajectories, they investigated cloud properties derived by the Moderate Resolution Imaging Spectroradiometer (MODIS) as a function of time since the air passed the sea ice edge. The liquid water path (LWP) and cloud top height (CTH) increase within the first 10 h, with the strongest increase in the initial MCAO phase, i.e., the first couple of hours. Further, they demonstrate that MCAO strength affects the development until 30 h after the air left the ice edge. However, due to retrieval limitations, they only considered liquid-dominated clouds, and no information on the vertical structure and precipitation is available. Based on CloudSat observations, Mateling et al. (2023) demonstrate the important role of snowfall from MCAOs, which produce the majority of snowfall in the North Atlantic. Yet, CloudSat sampling is limited by its blind zone of 1 km (Maahn et al., 2014; Schirmacher et al., 2023), and it is unclear whether differences with model precipitation result from model deficits or instrument limitation (von Lerber et al., 2022).
In summary, there is a clear need for high-resolution cloud observations within MCAOs. These are typically only available from ground-based remote sensing measurements at supersites. Therefore, the Cold-Air Outbreaks in the Marine Boundary Layer Experiment (COMBLE) in 2021–2022 (Geerts et al., 2022) established two ground stations at Andenes and Bear Island, Norway, providing important insights into cloud properties (Mages et al., 2023; Lackner et al., 2023) and supporting model evaluation (Geerts et al., 2022). As these stations were located about 1000 km away from the sea ice edge, only open and closed cellular convection was observed, whereas cloud streets were not.
In this study, we exploit detailed cloud observations taken during the initial MCAO phase close to the ice edge, where the rapid development of mixed-phase clouds occurred. We use airborne remote sensing observations that target model evaluation in a statistical sense and suggest suitable metrics for this. The measurements were performed during the HALO-(AC)3 campaign (named after the High Altitude and Long Range Research Aircraft and Transregional Collaborative Research Centre ArctiC Amplification: Climate Relevant Atmospheric and SurfaCe Processes and Feedback Mechanisms (AC)3; Wendisch et al., 2024) that took place over the Fram Strait where MCAOs occur frequently (Dahlke et al., 2022; Mateling et al., 2023; Papritz et al., 2015). We focus on a major MCAO event that remained active for more than 2 weeks (Walbröl et al., 2024). Within this period of northerly flow, two dedicated research flights were performed just 3 d apart, equipped with active and passive remote sensing instrumentation. The environmental conditions during the flights were similar but slightly different, particularly with respect to the cloud properties. Strait flight tracks crossed cloud streets multiple times perpendicularly to their elongated orientation to perform high-resolution measurements of cloud and precipitation properties which are not possible from a satellite (e.g., see LWP observations by MODIS; Fig. 1c, d). The data cover a fetch, i.e., the distance the air traveled over open water prior to the measurement, of up to about 150 km. From these unique measurements, we aim to answer the following questions:
-
What are the differences between the environmental conditions on both flight days, and what are their implications for cloud development?
-
Can we identify characteristic changes in cloud and precipitation properties perpendicular to the cloud street orientation, i.e., within the roll circulation?
-
How do roll circulation, clouds, and precipitation properties evolve with fetch in the initial MCAO phase, e.g., up to travel times of 4 h?
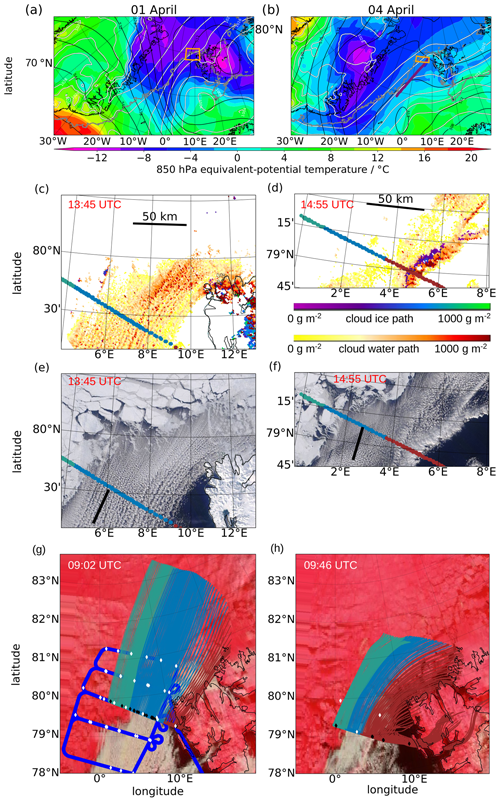
Figure 1Overview of 1 (left) and 4 April 2022 (right). (a, b) Maps of mean sea level pressure (white contours), 500 hPa geopotential height (black contours), and 850 hPa equivalent potential temperature (shading) from ERA5 at 12:00 UTC with 15 % sea ice concentration (gray dots), the flight area (orange) shown in (c)–(h), and the convergence line (purple) on 4 April. (c, d) Total cloud water path (NASA Worldview, 2023a; 1 km resolution) and (e, f) corrected reflectance (NASA Worldview, 2023b; 500 m resolution) of MODIS Terra on 1 April (13:45 UTC) and 4 April (14:15 UTC). Thick colored dots show the P5 track, categorized by the measurement regime (Table 1). Black lines represent the orientation of the cloud streets. (g, h) Near-surface back trajectories for 12 h for the P5 measurement locations (colored lines), dropsonde locations (diamonds) of P5 (black) and HALO (white), and the flight path of HALO on 1 April 2022 (blue line). Background image from the Visible Infrared Imaging Radiometer Suite (VIIRS) (NASA Worldview, 2023c) at 09:02 UTC on 1 April and 09:46 UTC on 4 April.
The paper is organized as follows: first, we introduce the airborne measurements and data (Sect. 2). Second, we describe the methodology that we developed to assign the fetch to each measurement using back-trajectory calculations (Sect. 3.1) and the identification algorithm to detect roll circulations from cloud radar measurements (Sect. 3.2). Section 4 describes the boundary layer and cloud development during the two flights (Sect. 4.1) and characterizes cloud properties within roll circulation (Sect. 4.3) and the development along fetch (Sect. 4.4). Finally, Sect. 5 concludes with the questions raised above and discusses pathways for future model evaluation.
Airborne measurements from the HALO-(AC)3 campaign (Wendisch et al., 2021) manifest the backbone of this study. During this campaign, the High Altitude and Long Range Research Aircraft (HALO; Ziereis and Gläßer, 2006), the research aircraft Polar 5 (P5), and the research aircraft Polar 6 (P6; Wesche et al., 2016) operated in the North Atlantic sector of the Arctic at altitudes of around 10 km, 3 km, and below 3 km, respectively. This analysis mostly focuses on radar, radiometer, lidar, and dropsonde measurements from P5 that probed MCAO events in their early phase. Dropsonde measurements from HALO and in situ observations from P6 further support the analyses. We limit the analyses to measurements taken over the ocean and restrict the remote sensing measurements to straight flight segments that exceed a flight altitude of 2 km to observe clouds from aloft. The focus is on two P5 flights, namely on 1 April 2022 (Fig. 1a) and 4 April 2022 (Fig. 1b). To investigate roll circulation, the flight paths crossed the cloud streets perpendicularly. P5 probed along the same path going back and forth, yielding six legs on 1 April (09:08–14:20 UTC) and four legs on 4 April (10:06–14:22 UTC).
2.1 Synoptic overview of cases
On 1 and 4 April, the large-scale constellation of a high-pressure system over Greenland and a low-pressure system over Siberia led to advection of cold air from the central Arctic over the open ocean (Fig. 1a, b) and to cloud street formation (Fig. 1e, f). On 1 April, the center of the cold air at 850 hPa was located over Svalbard. A local near-surface low-pressure system southwest of Svalbard resulted in a near-surface northeasterly flow. With height, the flow turned northerly as indicated by the 500 hPa geopotential (Fig. 1a). On 4 April, contrarily, the cold air at 850 hPa height was shifted more to the west, and the flow at all heights aligned parallel to the sea ice edge over the Fram Strait (Fig. 1b). The easterly flow forced air to ascend over Svalbard, thereby causing a lee effect. Therefore, a larger cloud-free region west of the island appeared, and a convergence line parallel to the ice edge at the transition to the cloudy regime formed (Fig. 1f). However, note that the flow within our study area might deviate from the large-scale condition. On both days, the MCAO index was stronger than the 75th percentile of the climatology from 1979 to 2022 (Walbröl et al., 2024). While the synoptic conditions are similar for both cases, differences in flow directions led to different MCAO strengths which in turn precondition the evolution over water.
2.2 Airborne instrumentation
2.2.1 Dropsondes
Vaisala RD94 dropsondes were launched from P5 and HALO. From P5, 18 and 14 sondes were launched on 1 and 4 April, respectively. They provide vertical profiles of potential temperature (θ; accuracy of 0.2 K), relative humidity (2 %), pressure (0.4 hPa), and horizontal wind components derived from GPS recordings (Vaisala, 2010; George et al., 2021).
2.2.2 Airborne Mobile Aerosol Lidar (AMALi)
The AMALi instrument on board P5 measures profiles of backscattered intensities at 532 nm (parallel and perpendicular polarized) and 355 nm (not polarized; Stachlewska et al., 2010). The lidar measurements are processed with a vertical resolution of 7.5 m and temporal resolution of 1 s. Lidar backscatter is highly sensitive to hydrometeors, especially to liquid, which, in our case, is always supercooled. Cloud top height (CTH) is obtained for every profile that has consecutive heights with backscatter coefficients exceeding one of cloud-free sections by a factor of 5. The CTH is the maximum altitude of these consecutive heights. Further details can be found in Mech et al. (2022a) and Schirmacher et al. (2023).
2.2.3 Microwave Radar/radiometer for Arctic Clouds (MiRAC)
The active component of the downward-looking airborne MiRAC (Mech et al., 2019) on board P5 consists of a frequency-modulated continuous-wave (FMCW) radar that operates at 94 GHz. Additionally, a passive channel at 89 GHz accompanies the active measurements. Both measurements are taken at a 25° backward inclination. While the vertically resolved radar measurements are reconstructed to nadir measurements, the passive measurements are taken on a slanted path. MiRAC measures every second, corresponding to a horizontal resolution of the equivalent radar reflectivity (Ze) of about 85 m at the ground in the flight direction for typical cruise altitudes of 3 km height and ground speeds of 80 m s−1. The radar measurements are quality-controlled and corrected for surface clutter and aircraft attitude (Mech et al., 2019). Sensitivity and the vertical resolution of the cloud radar depend on the chirp settings. During HALO-(AC)3, the detection limit for the most distant ranges from P5 of 3 km was around −45 dBZ and the vertical resolution was 4.5 m close to the aircraft and at most 13.5 m (Mech et al., 2022a). The processing interpolated the vertical resolution to 5 m over the whole profile. A blind zone of 150 m above ground is omitted due to ground clutter (Schirmacher et al., 2023). The accuracy of Ze is about 0.5 dBZ. Attenuation by water vapor (<1 dBZ) and clouds (∼ 0.6 dBZ) can potentially reduce this accuracy (Schirmacher et al., 2023).
The cloud top height is also derived from the radar profiles and corresponds to the height of the uppermost radar reflectivity signal above the noise level. Comparing this height with CTH derived from lidar allows us to assess the supercooled liquid layer thickness (LLT). Here, we exploit the fact that the lidar is more sensitive to the particle amount (liquid), whereas the radar is more sensitive to the particle size, i.e., ice particles (Ruiz-Donoso et al., 2020). Due to the limited vertical resolution of the instruments and resulting uncertainties in CTH, the CTH of the lidar has to exceed the CTH of the radar by at least 10 m for a profile to be defined as liquid topped and thus mixed-phase. For the calculation of the hydrometeor depth (D), we take the difference between the lidar CTH and the lowest radar signal within a continuous cloud layer. However, if a minor gap in the cloud profile occurs (i.e., the vertical distance between two layers is smaller than 50 m), we define only one layer from the lower cloud bottom to the upper cloud top.
We define profiles containing a Ze value higher than −5 dBZ (Schirmacher et al., 2023) in the lowest 500 m (Shupe et al., 2008) as precipitating. Using the Ze–S relation for three bullet rosettes (Kulie and Bennartz, 2009), this value corresponds to a snowfall rate (S) of 0.07 mm h−1. This relation is also used to analyze S close to the ground at 150 m. Note that these S estimates are inaccurate since Ze–S relations highly depend on ice habits, which are very variable within cloud streets (Maherndl et al., 2023; Moser et al., 2023).
The passive channel observes the brightness temperature (TB), which is primarily influenced by the emission of liquid clouds and the surface. Differences in TB between clear-sky and cloudy situations are used to retrieve LWP over the ocean via a regression approach (Ruiz-Donoso et al., 2020). Due to the unknown emissivity of sea ice, LWP is only derived over open ocean. Depending on atmospheric conditions, the maximum uncertainty is below 30 g m−2 (Ruiz-Donoso et al., 2020). While radar reflectivities are corrected to nadir profiles, TB and, thus, LWP measurements are measured along a slanted path (Mech et al., 2022a). Due to the strong attenuation of the lidar backscatter close to the cloud top, we assume that most liquid resides in the uppermost few hundred meters of the cloud, which is in accordance with Shupe et al. (2008). Therefore, LWP lags behind the radar observations in time. Based on geometric considerations, we shift the LWP measurements, assuming a daily average CTH for cloud streets. Since this average differs for both days, we shift the LWP measurements by different time periods, i.e., 16 and 19 s on 1 and 4 April, respectively, which results in an estimated maximum error of 4 s. As a result, a good agreement between LWP peaks and profiles of high Ze is observed.
2.2.4 In situ probes
P6 was equipped with three in situ probes, namely the Cloud Droplet Probe (CDP; Lance et al., 2010), Cloud Imaging Probe (CIP; Baumgardner et al., 2011), and Precipitation Imaging Probe (PIP; Baumgardner et al., 2011). The CDP is a forward-scattering optical spectrometer that measures small cloud particles (2.8–50 µm). Larger cloud particles are observed by the CIP (15–960 µm) and PIP (103 µm–6.4 mm), which record shadow images of the cloud particles as particles pass through the sampling area (Moser et al., 2023). Rimed mass is calculated from images of the fractal particle shapes, as well as the continuous particle size distribution derived from combining CDP, CIP, and PIP observations. CIP and PIP data are processed similarly to those from previous campaigns (Mech et al., 2022a).
2.2.5 Collocation
On both days, P5 (remote sensing) and P6 (in situ) were closely collocated. In the riming analyses, we use a data subset during which both aircraft flew on straight paths with a time difference between the collocated measurements of less than 5 min, a spatial distance between both platforms below 5 km, and a flight altitude of P6 between 0.15 and 1.3 km. Thereby, we reduce the error caused by sampling different air masses with P5 and P6 and caused by sampling air masses with varying microphysical properties due to changing P6 locations within the cloud vertical extent. On 1 April, 3971 s of collocated observations covers longitudes between 4.5 and 6.5° E, corresponding to 25–165 km of fetch. On 4 April, only 845 s of observations is collocated, located between 1.5 and 4.5° E and covering fetches between 55 and 165 km and mostly at around 80 km. On 1 April, seven collocated data segments exist with gaps of less than 5 s. These segments cover 39 min at a fetch of 60–140 km, with most measurements concentrated around 7° E.
2.3 Satellite and reanalysis data
For the sea ice concentration (SIC), we use a daily product that merges satellite observations from MODIS and the second Advanced Microwave Scanning Radiometer (AMSR2) at 1 km horizontal resolution (Ludwig et al., 2020). For the analysis, we interpolate the data to a 0.05° × 0.05° latitude–longitude grid. The sea surface temperature (SST) is obtained from the Arctic Ocean – Sea and Ice Surface Temperature product based on observations from the Metop-A Advanced Very High Resolution Radiometer (AVHRR). The daily product (Copernicus Marine Service, 2023) has a spatial resolution of 0.05° and covers surface temperatures of the ocean, sea ice, and the MIZ. Using satellite SST and dropsonde temperature measurements over open water (Fig. 1g, h, black and white dots), we calculate the MCAO index from the difference between the potential temperature (θ) at the sea surface and 850 hPa altitude. Generally, the MCAO index is positive during a MCAO and describes its strength (Papritz et al., 2015; Kolstad, 2017). Over the ocean, we use the Coupled Ocean–Atmosphere Response Experiment (COARE) bulk air–sea flux algorithm (Fairall et al., 2003) to additionally calculate surface heat fluxes from satellite SST data and dropsonde observations at 10 m height.
The European Centre for Medium-Range Weather Forecasts (ECMWF) reanalysis product version 5 (ERA5; Hersbach et al., 2020) is used to analyze the large-scale environmental conditions and to compute back trajectories using Lagranto (Sprenger and Wernli, 2015). ERA5's temporal, horizontal, and vertical resolution is 1 h, 31 km, and 137 model levels from the surface up to the top of the atmosphere, respectively (Kirbus et al., 2024). Note that most HALO dropsondes have been assimilated into ERA5, leading to improved performance for our study cases.
In the following, we describe two approaches we established for analyzing the airborne measurements: first, the quantification of fetch assigned to each measurement (Sect. 3.1) and, second, a novel method to identify roll circulation from airborne radar reflectivities only (Sect. 3.2).
3.1 Trajectory calculations and fetch
During MCAOs, the warm ocean alters thermodynamic ABL conditions of air masses initially formed in the central Arctic through turbulent surface heat and moisture fluxes (e.g., Brümmer, 1996) whenever SIC is below 100 %. We aim to quantify this influence of open water on ABL development. Since it is impossible to calculate integrated surface fluxes along the trajectories with our data, we derive the fetch for each airborne measurement. We follow Spensberger and Spengler (2021) and account for open water over the MIZ as well as leads in the ice. For flows unaffected by land masses, travel time over open water and fetch can be linearly converted and are both valid to study. The correlation coefficient between travel time and fetch is 0.99 for all P5 measurements that are not influenced by land masses and −0.5 for measurements influenced by Svalbard. Thus, the latter data have been removed from the analysis (see below).
We need to know the air masses' previous path to calculate fetch for each measurement. Therefore, we compute near-surface Lagrangian back trajectories using Lagranto (Sprenger and Wernli, 2015) with ERA5 wind fields as input. Specifically, we calculate back trajectories for the previous 12 h for every flight minute and assign them to the observations within each minute. The trajectories originate from the horizontal location of P5 and 1000 hPa height, corresponding to roughly 300 m above the surface. To investigate the influence of the surface on the air masses, we take a near-surface starting point for the trajectories. Similar to Spensberger and Spengler (2021), we calculate the fetch for every back trajectory by integrating the ratio of open water obtained from MODIS–AMSR2 SIC data (Sect. 2.3) along the back-trajectory paths over the previous 12 h until measurement time (0 h):
Note that due to the resolution of ERA, neighboring trajectories are rather similar (Fig. 1g, h). Differences in fetches between two neighboring trajectories mainly come from differences in SIC along the trajectories. The median of the relative change between two adjacent fetches is 9.6 %.
To focus on cloud street characteristics during MCAO conditions, we limit the analysis to air masses that did not pass Svalbard at any time and that are not affected by the convergence line on 4 April (Table 1; Fig. 1c–h, non-red dots). The remaining measurements are classified as either “cloud streets” (Fig. 1c–h, blue) if radar reflectivities appear regularly or else as “prior to cloud streets” (Fig. 1c–h, green). The latter category includes samples taken over and close to sea ice that have fetches less than 15 km on 1 April and longitudes smaller than 1.7° E on 4 April (fetches of about 17 km; Table 1). On 4 April, longitude instead of fetch is used for classification as cloud streets over open ocean and cloud-free conditions over sea ice both have fetches of 17 km. Note that fetch includes contributions from the MIZ (80 % < SIC < 100 %) and open water.
3.2 Roll circulation identification
To identify roll circulation from radar measurements, we must rely on indirect information. Previous studies applied spectral analyses to observations of the three wind components, temperature, mixing ratio, and radiative fluxes (Brümmer et al., 1985, 1992; Brümmer, 1999; Walter and Overland, 1984). While vertical velocity cannot be extracted from the airborne Doppler measurements (Mech et al., 2022a), we exploit the fact that cloud particles form to the largest extent at the location of the strongest updraft due to vertical motion. Here, frequent saturation with respect to ice occurs, facilitating the formation of cloud droplets and growth of both liquid and ice particles (Korolev and Field, 2008). In order to account for potential influences by dry entrainment at the cloud top (Klingebiel et al., 2015) or occurrence of precipitation (Morrison et al., 2012), we only consider measurements at the height of 0.7 of the hydrometeor depth (D; Sect. 2.2) for the identification of roll circulation. By using the radar reflectivity at this height (), we target the largest ice particles within the profile and minimize the influence of dry-air entrainment and supercooled liquid water droplets at the cloud top as well as precipitation at the bottom of D. We refer the reader to Appendix A for further discussion on the height selection, including a sensitivity analysis.
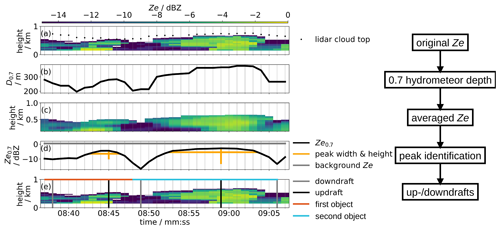
Figure 2Visualization of roll cloud circulation identification. Time series (30 s) of the equivalent radar reflectivity Ze profiles measured by MiRAC starting at 10:08:37 UTC on 1 April (a), 0.7 of the hydrometeor depth (D0.7, b), Ze smoothed in space and time (c), and smoothed Ze at D0.7 (d, black) with its peak width and height (d, orange) and the background Ze (d, gray). The original Ze observations (same as in a), detected up- (black) and downdrafts (gray), and circulation objects (blue, red) are shown in (e). For comparison, cloud top height observed by the AMALi lidar is displayed (a, black dots). The shown time period covers fetches from 73 to 80 km and corresponds to a flight distance of 7 km.
serves as a proxy for vertical velocity. We assume that maxima in represent updraft regions, while minima in represent downdraft regions of the roll circulation. For cloud-free areas, we define the downdraft in the area's center. The workflow of the circulation detection is summarized in Fig. 2. Appendix A further summarizes details, including Table A1 for a sensitivity analysis. Among different configurations, we selected the detection algorithm with the best ratio between determining peaks and ignoring noise. This automated peak detection depends only on the large-scale condition and, thus, might not determine every maximum of considered by the human eye.
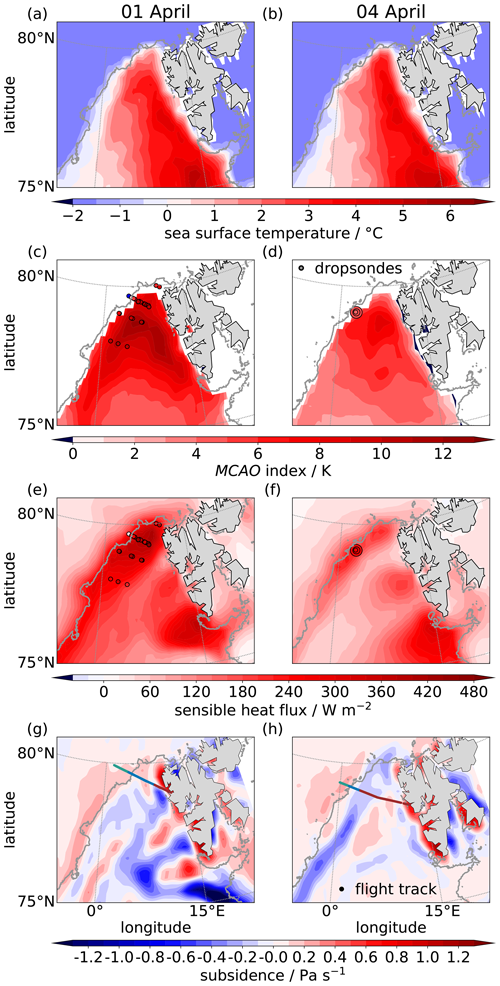
Figure 3Overview of environmental conditions on 1 April (left) and 4 April (right). Maps of sea surface temperature (a, b), the MCAO index (c, d), sensible heat fluxes (e, f), and subsidence at 925 (g) and 975 hPa (h) from ERA5 reanalysis data. Positive subsidence values indicate downward motion. MCAO indices (c, d) and sensible heat fluxes (e, f) from dropsonde observations are shown as circles. Flight tracks in (g) and (h) are color-coded according to the measurement categorization (Table 1). The gray lines indicate the 15 % sea ice concentration from ERA5.
Table 2Conditions during 1 and 4 April. BLH and CTH stand for atmospheric boundary layer height, i.e., the inversion height of potential temperature, and cloud top height, respectively.
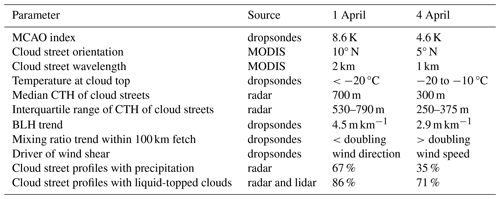
We only apply the detection algorithm to the cloud street regime as roll convection is invisible to the radar as long as no significant number of hydrometeors is present. According to our definition, the maximum updraft (maximum ) is not necessarily centered between the two detected edges of our roll circulation object. The wavelength of the circulation λ is the distance between two identified adjacent downdrafts. The mesoscale circulation is described by the aspect ratio (AR), which is the ratio between λ and the CTH at the updraft position. In total, we identified 356 and 112 cloud circulation objects in the cloud street regime on 1 and 4 April, respectively.
In the following, we first investigate the boundary layer conditions (Sect. 4.1) and the preconditioning by riming (Sect. 4.2). We then statistically analyze cloud morphological and microphysical properties within the roll circulation object within the cloud street regime (Sect. 4.3), followed by an assessment of cloud and precipitation properties as a function of fetch within the first 170 km (4 h) of the MCAO development (Sect. 4.4).
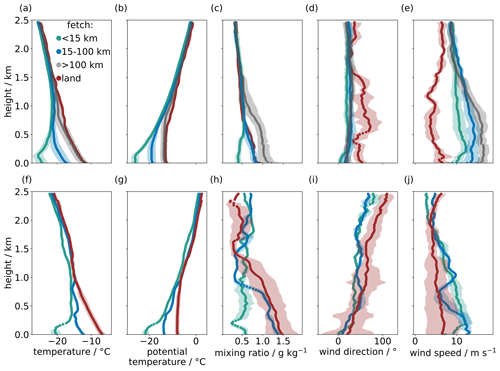
Figure 4Averaged dropsonde profiles from HALO and P5 of temperature (a, f), potential temperature (b, g), mixing ratio (c, h), wind direction (d, i), and speed (e, j) binned by fetch on 1 April (first row) and 4 April 2022 (second row). The shaded areas represent the standard deviation of each category. The color coding follows the categorization shown in Table 1. On 1 April, the number of dropsondes per category is 27 (<15 km fetch; green), 14 (15–100 km and >100 km fetch; dark and light blue), and 3 (land; red). On 4 April, the number is 3, 4, and 9, respectively.
4.1 ABL conditioning
First, we investigate how much the ABL conditions differ between the cases, including possible drivers. The influence of the ocean on the ABL through surface sensible and latent heat fluxes is more pronounced on 1 than 4 April (Fig. 3e, f). Dropsondes show maxima of 450 (225) W m−2 for sensible (latent) heat fluxes, respectively. Over the MIZ, fluxes decrease, and the ratio changes to a higher contribution of the sensible heat flux due to less evaporation over sea ice as also found by Li et al. (2020). Along the whole flight track, ERA5 shows that the sensible heat flux decreases much faster with distance from the sea ice edge on 4 than 1 April (Fig. 3e, f), even though oceanic conditions here represented by SST are similar (Fig. 3a, b). This indicates that the atmospheric conditions differ between the cases, which is especially confirmed for temperature by differences in MCAO fields (Fig. 3c, d). The MCAO index averaged over all dropsondes launched from P5 is stronger on 1 than 4 April (8.6 K versus 4.6 K, Table 2). Note that although dropsondes were launched further away from sea ice on 1 April, ERA5 fields show roughly the same differences of a factor of 2 over both flight tracks. In accordance with Seethala et al. (2021), fluxes and MCAO indices from ERA5 generally correspond to dropsonde estimates, except over sea ice, where ERA5 seems to overestimate the fluxes. Finer spatial structures in both parameters are resolved in the dropsondes.
Cloud conditions on 1 April are characterized by cloud streets oriented by about 10° to the north (Fig. 1e, black line) and a wavelength of about 2 km with shorter distances between the separated streets close to sea ice. Note that this information is retrieved from MODIS sensors (bands 1, 3, and 4) that have a spatial resolution of at least 500 m. At the height of the median CTH, here 925 hPa, air subsides within the regimes of prior to cloud streets and cloud streets, respectively (Fig. 3g, green and blue track). Over the ocean, subsidence is generally reduced compared to over sea ice. The area of fetches between 75 and 120 km around 7° E is characterized by strong subsidence (Fig. 3a, c) throughout the entire atmospheric column (not shown) despite increasing SST and MCAO indices. This wave-like pattern is likely induced by wave effects originating from the Svalbard archipelago (Shestakova et al., 2022).
The thermodynamic state of the ABL is described by mean profiles of dropsondes released from P5 and HALO over sea ice and open water. On 1 April, temperatures are lower than −20 °C throughout all altitudes over sea ice (fetch of <15 km) and for parts over open water (Fig. 4a). Over sea ice, surface layers generally develop an inversion as air is cooled from the ground and warmed by subsidence from above. Profiles that were sampled by HALO dropsondes over sea ice exhibit a thin (<250 m deep) ABL. Close to the sea ice edge, the depth of the ABL is similar to conditions over closed sea ice (Fig. 4b). The ABL is capped by a low-level jet at 250 m height (Fig. 4e) and has a low water vapor mixing ratio (0.5 g kg−1; Fig. 4c). The mixing ratio indicates the low background vapor concentration of the polar air mass. With 28°, wind direction is constant with height, while the near-surface wind originates from 0° N regardless of surface properties (Fig. 4d). Over open water, temperatures and, thus, wind speeds within the neutrally stratified ABL increase with fetch. The boundary layer height (BLH), which is the inversion height of the potential temperature θ, doubles within the first 100 km (Fig. 4b). Mixing ratio increases with fetch due to strong surface heat fluxes and turbulent mixing of near-surface air.
On 4 April, the MODIS image shows cloud streets with an orientation of 5° to the north (Fig. 1f, black line) and a wavelength of about 1 km. Compared to conditions on 1 April, the air mass at CTH (975 hPa) ascends for fetches larger than 60 km (Fig. 3h). A wave effect is notable within the region affected by the lee effect but not for the analyzed data west of the convergence line. Warmer temperatures on 4 April reveal a difference in air mass between both days: all temperatures below 2 km height range within −20 to −10 °C (Fig. 4f). Free-tropospheric θ is higher by about 5 K on average compared to 1 April (Fig. 4b, g). Furthermore, mixing ratio of the polar air mass is slightly higher at all heights (Fig. 4c, h). Flow conditions differ compared to 1 April, even though a low-level jet also exists at 200 m over sea ice (Fig. 4j). A directional shear from northerly winds at the surface to westerly winds occurs at all heights and is strongest at the BLH (Fig. 4i). Although HALO dropsondes cannot detect a BLH in the central Arctic (81.3–87.0° N) as it is likely too shallow (not shown), the capping inversion is stronger over the sea ice close to its edge. On 4 April, this inversion weakens less with fetch compared to 1 April due to a layer of warm air above the BLH. Together with surface fluxes about half as high as those of ERA5 on 4 April (Fig. 3e, f), this results in a much weaker BLH increase rate averaged over all fetches (Table 2) and a reduction in wind speeds by 5 m s−1.
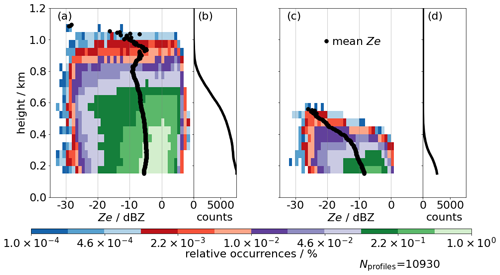
Figure 5Contoured frequency by altitude diagram (a, c) and absolute counts per altitude (b, d) for all radar reflectivities (Ze) obtained by MiRAC in the cloud street regime on 1 April (a, b) and 4 April (c, d). Moreover, each averaged Ze profile (black dots) and the total number of profiles (Nprofiles) are displayed.
On both days, the radar profiles in the cloud street regime frequently (93 %) exhibit clouds. The diagrams of contoured frequency by altitude (Fig. 5) reveal the different cloud and precipitation characteristics of cloud streets between the days. CTH is twice as high on 1 as on 4 April, respectively. Furthermore, mean Ze (black dots) is higher at all heights. On 1 April, values larger than −5 dBZ associated with the onset of snowfall occur at all heights. The shorter the fetch on 1 April, the stronger the decrease in the mean Ze profile close to the surface (not shown). Thus, near-surface ice particles might experience stronger sublimation on 1 April when the mixing ratio is comparably small, and the relative humidity with respect to ice is below 100 % (not shown). On 4 April, Ze rarely exceeds −5 dBZ even below 500 m, reducing the frequency of precipitation compared to 1 April (Table 2). Moreover, mean Ze increases towards the surface, indicating the ongoing growth process of ice particles. On both days, most cloud streets are liquid topped (Table 2). Liquid-topped cloud streets are mainly characterized by a higher CTH compared to non-liquid-topped clouds (not shown).
In summary, the MCAO case on 1 April is stronger by a factor of 2 than that on 4 April due to colder and drier air masses (Table 2). Wind shear occurs during wind speed changes on 1 April and directional changes on 4 April, respectively. Contradicting the MCAO index, air subsides close to the surface in the cloud street regime at fetches around 100 km on 1 April due to a wave effect caused by Svalbard.
4.2 Preconditioning by riming
Ice growth affects the boundary layer evolution during MCAOs in several ways, e.g., by reducing cloud liquid water and triggering early and light precipitation, which then in turn cools and moistens the air below the cloud (Tornow et al., 2021). We evaluate the strength and variability in riming to investigate whether riming preconditions cloud microphysics and whether it impacts precipitation characteristics already in the initial state of MCAO evolution. We use a subset of in situ and remote sensing data during which P5 and P6 were collocated within the cloud street regime (Sect. 2). To determine the degree of riming, we calculate the normalized rime mass (M) defined as the rime mass divided by the mass of the size-equivalent spherical graupel particle. Following Maherndl et al. (2024), two methods are applied. The combined method uses the closure of in situ particle size distributions and Ze simulations obtained from running averages of in situ particle size distributions over 30 s. The in situ method relates M to in situ particle shape measurements only. The results of both retrievals are comparable. However, since the collocation of P5 and P6 measurements might be inaccurate, we only show results from the in situ method for which no matching is necessary. Note that our definition of updrafts might bias the following findings.
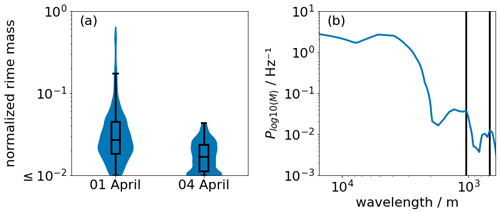
Figure 6(a) Violin and box plot of the normalized rime mass (M) obtained by the in situ method for collocated cloud street flight data on 1 and 4 April. (b) Corresponding power spectrum of M on 1 April with black lines marking important local maxima of the spectrum.
Considering particles with as rimed, more rimed particles exist on 1 April (97 %) than on 4 April (80 %), respectively. The median M of 10−1.6 on 1 April and 10−1.8 on 4 April (Fig. 6a) clearly reveals that riming is only significantly active in cloud streets on 1 April. In particular, normalized rime masses of only exist on 1 April. On this day, cloud top temperatures are colder than or at the low end of temperatures within the dendritic growth zone (DGZ; −20 to −10 °C). Hence, conditions are too cold for aggregation to be dominant (Chellini et al., 2022). On 4 April, contrarily, riming is not significant because cloud top temperatures lie within the DGZ, fostering aggregation.
The spatial variability in riming is investigated by linearly detrended and mean-centered power spectra of M obtained during seven collocated segments (Sect. 2). Edge effects are minimized by applying a Hann window for smoothing. Due to the units of variance, the power spectrum increases automatically for smaller wavelengths. The averaged power spectrum of M peaks at about 0.7 and 1.1 km (Fig. 6b). These values roughly correspond to the wavelength λ of cloud streets as seen in the MODIS images (Fig. 1; also see Sect. 4.4 below) and as derived from the roll circulation analyses below. For 1 April, we, hence, infer that riming is mainly present within the updraft regions of cloud streets. A more detailed comparison with λ of the roll circulation detected by the remote sensing measurements is performed in Sect. 4.4. We further analyze the effect of active riming, present on 1 April, on cloud microphysics in the following sections.
4.3 Impact of roll circulation on cloud and precipitation properties
Our measurement strategy across cloud streets allows us to detect individual roll circulation objects (Sect. 3.2). For the following statistical assessment, we refer to “clouds” as objects that have at least five successive radar measurements, resulting in 344 and 109 objects on 1 and 4 April, respectively. First, we investigate the location of their updraft center () within the cloud. As explained before, these objects are not necessarily symmetric. However, most clouds form centered around the updraft of the circulation. Around 50 % of the time, maxima of occur within the central tercile of the cloud, and only rarely are they within the tercile closest to the lateral cloud boundary (7 %).
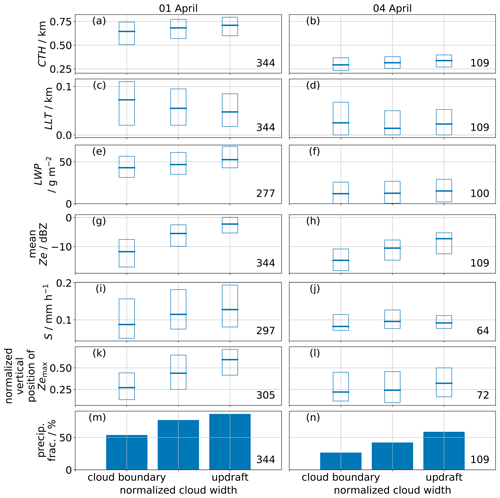
Figure 7Average composites of cloud and precipitation properties within the normalized distance between the lateral cloud boundary and updraft (max ) for circulation objects within the cloud street regime on 1 April (left column) and 4 April (right column): cloud top height (CTH; a, b), supercooled liquid layer thickness (LLT; c, d), liquid water path (LWP; e, f), mean radar reflectivity (Ze) over each profile (g, h), snowfall rate at 150 m (S; i, j), vertical position of the maximum Ze for each profile normalized by the hydrometeor depth (k, l), and fraction of precipitating profiles (precip. frac.; m, n). The median (horizontal line) and lower and upper quartile (box edges) are displayed at the boundary of the clouds, the updraft position, and in between. The total number of objects is given for every parameter separately.
To characterize how dynamics within roll circulations affect cloud and precipitation properties, circulation objects are composited for their relative distance to (Fig. 7). More precisely, we group cloud properties into three regions according to their distance from the maximum updraft region (): the central updraft region, the region close to a cloud boundary, and the region in between.
On 1 April, the medians of several parameters show consistent behavior, although the variability, expressed by the interquartile distance, is high: CTH increases by 9 % from the cloud boundary to the location of the maximum updraft (Fig. 7a). In line with liquid formation within updrafts, LWP increases by 22 % (Fig. 7e). The mean of Ze over each profile (82 %; Fig. 7g) and S (42 %; Fig. 7i) increases, supporting the assumption of enhanced ice production in updrafts. In contrast, a decrease in LLT (32 %; Fig. 7c) of 20 m can be seen, which exceeds the uncertainty of 10 m. We speculate that, here, updrafts carry ice particles to higher cloud regions. If so, the mixed-phase region would expand at the expense of the liquid layer and would enhance riming (Fig. 4.2). Potential riming occurrence would increase ice particle size, Ze, and S in updrafts. The observed slight LWP increase in updrafts (Fig. 7e, f) could indicate that, in our study, condensation is more favored than the depletion of liquid.
Strong riming events might explain the frequently high extremes of S. Precipitation events not only intensify at updraft locations but also occur more frequently than at the cloud boundary (87 % compared to 55 % of the profiles, Fig. 7). On 1 April, we expect that most ice occurs at 0.6 of the hydrometeor depth for updraft positions as indicated by (Fig. 7k). Large-rimed particles close to the cloud top might lift the height compared to the cloud boundary, where most ice is located within the lowest third of the hydrometeor depth.
On 4 April, the absolute increase in CTH (Fig. 7b) and mean Ze (Fig. 7h) within the clouds is about half of that on 1 April. Moreover, S (Fig. 7j) and LLT (Fig. 7d) stay constant within the clouds. In all three categories, the normalized height at which ice occurrence is highest is similar to cloud boundary conditions on 1 April (Fig. 7l). The smaller MCAO strength on 4 April seems to weaken the updraft motion and might, thus, suppress the rise in CTH and the lifting of ice into the liquid layer in updrafts. In updrafts, this might prevent riming, likely hampering an increase in S and mean Ze as well as a lifting of the height level with the highest ice occurrence. While the absolute precipitation fraction is lower than on 1 April, e.g., reduced by 50 % at the cloud boundary, the relative increase in precipitation fraction from the object center to the boundary is similar (30 percentage points).
4.4 Development along fetch
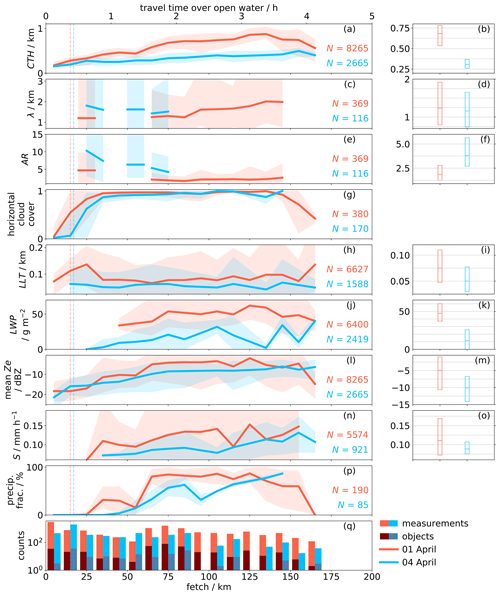
Figure 8The left column shows the development of circulation, cloud, and precipitation characteristics with fetch on 1 (red) and 4 April (blue): cloud top height (CTH; a, b), wavelength of the circulation (λ; c, d), aspect ratio of the circulation (AR; e, f), horizontal cloud cover (g), liquid layer thickness (LLT; h, i), liquid water path (LWP; j, k), mean radar reflectivity over each profile (mean Ze; l, m), and snowfall rate at 150 m height (S; n, o), with precipitation fraction (precip. frac.; p) and number of measured profiles (light color) and identified cloud circulations (dark color) per fetch bin (q). LLT, LWP, and S statistics are only calculated when a cloud/precipitation occurs. Lines and shades represent mean values and the 5th and 95th percentile, respectively. The vertical dashed lines indicate the regime change from prior to cloud streets to cloud streets (Sect. 3.1). On the right box plots show each distribution's median and interquartile range within the cloud street regime. The total number of measurements (N) is given for each parameter and day.
To investigate how open water affects roll circulation and cloud properties, we analyze their evolution over all observed fetches (Fig. 8). The most prominent characteristic of an MCAO event is the rise in BLH driven by the strong heat fluxes as air flows over the relatively warm ocean. Already in the MIZ, evaporation and convection lead to the appearance of initial, still unorganized clouds in the regime of prior to cloud streets, although horizontal cloud cover is low (Fig. 8g). Within the cloud street regime, cloud cover increases rapidly and exceeds 90 % for fetches beyond 30 km. The comparison of BLH, derived from dropsondes, and closely located airborne measurements shows that CTH is generally only 8.5 m lower than BLH, which indicates that we can use CTH as a proxy for BLH. On both days, CTH increases steadily with fetch (Fig. 8a). Both the median CTH and CTH growth rate are reduced by more than half on 4 April compared to conditions on 1 April. A potential reason for this reduction might be a reduced buoyancy in the ABL, with warm air being advected above the boundary layer. Future modeling experiments could test this hypothesis, including lee effects on air mass development caused by the Svalbard archipelago. Interestingly, on 1 April, the linear increase in CTH levels at around 100 km fetch (2.5 h travel time). This area of fetch corresponds to an area of increased subsidence (see Fig. 3), capping cloud development. On 4 April, the CTH, cloud cover, and precipitation fraction decrease rapidly beyond fetches of 140 km. Corresponding trajectories are geographically close to trajectories excluded from the analysis due to land mass influences. We speculate that the Svalbard lee effect gains importance and increasingly suppresses cloud and precipitation formation despite coarse ERA5 subsidence values indicating rising air masses.
Next, we examine the dependency of circulation characteristics within the cloud street regime as function of fetch, specifically wavelength (λ) and aspect ratio (AR). Note that bins of fetch with less than 10 roll circulation objects, e.g., at fetches around 50 km, are removed as outliers from all following analyses of λ and AR (Fig. 8c, e). On 1 April, λ increases from roughly 1 to about 2 km at a fetch of 150 km (Fig. 8c), approaching the width of the cloud streets seen by MODIS (2 km; Sect. 4.1). For fetches larger than 60 km, AR is around 2 and remains rather constant with fetch. As discussed in Sect. 4.2 and Fig. 6b, the normalized rimed mass M follows a multi-modal power spectrum. Local maxima of 0.7 and 1.1 km, respectively, align with λ, suggesting that riming is enhanced in updraft regions of roll circulation objects. On 4 April, the measurements only revealed reliable circulation information for fetches smaller than 80 km. There, λ does not increase substantially with fetch and aligns with the cloud street width of the MODIS images (1 km; Sect. 4.1). Yet, the spread in λ values is high as roll convection is comparatively weaker at low fetches. This spread propagates to the derived AR distribution, which is characterized by a large interquartile range from 2.7 to 5.6. Beyond fetches of 60 km, where convection is stronger, variability is lower and AR slightly reduces with fetch. There, observed AR nearly doubles compared to conditions on 1 April. Brown (1972) relates larger AR to reduced available energy for convection. Heat fluxes observed at fetches of 60 km and beyond are indeed reduced on 4 April (Fig. 3e, f). In summary, median λ is similar on both days (around 1.2 km; Fig. 8d), while CTH on 1 April is increased by a factor of 2 compared to 4 April (Fig. 8b). Consequently, median AR on 1 April (Fig. 8f) is smaller than on 4 April (1.8 and 3.9, respectively).
We further analyze liquid layer thickness (LLT; Fig. 8h), LWP (Fig. 8j), mean Ze (Fig. 8l), and S (Fig. 8n) to investigate how the exposure to open water influences cloud microphysics. All parameters except for LLT show a slight increase with fetch. Median LWP conditions differ substantially on both days as the median LWP reduces from 50 g m−2 on 1 April to 10 g m−2 on 4 April, respectively. While LWP ranges within the uncertainty limit of 30 g m−2 (see Sect. 2.2) for small fetches below 100 km, it steadily increases with fetch and exceeds the uncertainty limit for fetches larger 100 km. The median LLT of 75 (50) m on 1 (4) April points to the dominant presence of liquid-topped mixed-phase clouds. This finding is in agreement with the overall high occurrence of liquid layers of 86 % (71 %) for the respective days (see Table 2). We attribute the constant LLT with fetch to the fact that both liquid (LWP) and ice (approximated by mean Ze) increase simultaneously, in turn keeping the LLT constant.
While the evolution of cloud microphysics with fetch is similar on both days, thermodynamic conditions modify the intensity of the parameters. On 4 April, characterized by overall warmer temperatures, clouds are more shallow. On this day, 90 % of the profiles containing liquid-topped cloud streets have an LLT of smaller than 100 m, which is more than on 1 April (70 %). Less supercooled liquid may reduce the number of liquid-topped cloud profiles (Table 2), LWP (Fig. 8k), and LLT (Fig. 8i). A potential mechanism could be that the warmer temperature, low amount of supercooled liquid, and weak MCAO index prevent riming, reducing snowfall rate and mean Ze. This could potentially explain why snowfall occurs less frequently on 4 April. Moreover, the lack of riming in updrafts would reduce the variability in snowfall rate within each fetch bin. Lacking preconditioning by riming might delay the precipitation onset on 4 April by more than 10 km (Fig. 8p), which starts forming at fetches of 26 and 39 km on 1 and 4 April, respectively.
Our study investigates the evolution of thermodynamics, cloud and circulation morphology, cloud microphysics, and precipitation within air masses developing the first 170 km fetch (about 4 h of travel time) in a long-lasting cold-air outbreak in the Fram Strait. Airborne remote sensing and in situ observations were performed as part of the HALO-(AC)3 campaign within two research flights just 3 d apart. A unique sampling strategy oriented perpendicularly to cloud streets provided the opportunity to statistically quantify finely resolved macro- and microphysical cloud and circulation properties in the initial MCAO phase. Specifically, two metrics were developed for this purpose. First, we make use of a novel approach to detect roll circulation from vertical radar profiles only, which allows for the analysis of cloud and precipitation parameters as a function of their position within the roll circulation. Second, we use back trajectories to analyze cloud and circulation development with fetch which allows us to draw a consistent picture of the MCAO development on 1 and 4 April 2022 (Fig. 9). Our findings answer the research questions posed in Sect. 1:
-
What are the differences between the environmental conditions on both flight days, and what are their implications for cloud development?
Both MCAO events feature northerly winds advecting dry and cold air masses to the Fram Strait. The event on 1 April is characterized by colder air temperatures, leading to a doubling of the MCAO index and stronger heat fluxes compared to the 4 April case. The more active convection on 1 April deepens the boundary layer which causes higher cloud top heights that are aligned well with the boundary layer height. A rough scaling factor of 2 is observed in fluxes, the MCAO index, CTH, and LWP between both days. The Svalbard archipelago influenced the flow on both days, provoking a wave effect in the lee of Svalbard on 1 April, with subsiding air masses leading to reduced cloud top heights at 75 to 100 km fetch. On 4 April, lee effects caused an even stronger cloud-free zone west of the island, which led to a convergence zone and was not considered in the analysis.The difference in cloud top height between lidar and radar is used to detect the presence and derive the thickness of a supercooled liquid layer at the cloud top. Supercooled-liquid-topped mixed-phase clouds occurred 86 % and 71 % of the time on 1 and 4 April, respectively. With respect to cloud microphysics, the most prominent difference between both days is that riming is only significantly active during the cold and strong MCAO case on 1 April. This day also features a higher amount of supercooled liquid water with a median LWP of roughly 50 g m−2. Riming influences LLT, radar reflectivities, precipitation onset, and strength by producing larger ice particles. Regarding median properties, the cloud liquid layer thickness, liquid water path, and snowfall rate are again roughly lower by a factor of 2 for the weaker MCAO on 4 April.
-
Can we identify characteristic changes in cloud and precipitation properties perpendicular to the cloud street orientation, i.e., within the roll circulation?
Yes, several hundred roll circulation objects were identified using cloud radar measurements performed on multiple legs perpendicular to the cloud street orientation. A composite analysis of these objects reveals that, on 1 April, several parameters show consistent trends from the updraft region towards cloud boundaries, while, on 4 April, only radar reflectivity and cloud top height increase slightly in the respective updraft region. On 1 April, our data reveal the same frequency of the normalized rimed mass and updrafts within clouds. Thus, we speculate that the presence of significant riming on 1 April leads to the observed increases in snowfall rate, increases the height with most ice towards the updraft center, and impacts the observed LLT decrease towards the updraft as ice particles might be lifted into the pure liquid layer. Our statistical analysis of median cloud characteristics within the roll circulation and their variability (Fig. 7) could be used to test the performance of cloud parameterizations and better understand riming effects. -
How do roll circulation, clouds, and precipitation properties evolve with fetch in the initial MCAO phase, e.g., up to travel times of 4 h?
The analysis of our measurements as a function of fetch shows increasing the cloud top height, liquid water path, radar reflectivity, near-surface precipitation rate, horizontal cloud cover, and fraction of precipitating profiles with increasing fetch. Cloud streets form at around 15 km fetch and start precipitating at 25 to 40 km. We suspect that the later onset of precipitation on 4 April is attributed to the lack of riming in air masses with shorter fetches. The wavelength of the cloud streets λ slightly increases with fetch on 1 April, but the variability is rather high. The detected wavelength of around 1 km within the first 100 km of fetch aligns with the local maxima found in the spectral analysis of riming. Even though the airborne-derived results here generally coincide with MODIS-derived wavelengths, our analyses highlight the importance of high-resolution airborne measurements to evaluate satellite-derived products.
To answer the two last research questions, we established composite approaches to characterize the roll circulation (Fig. 7) and fetch (Fig. 8). These metrics can also be generated from cloud-resolving model output and be used to evaluate the model performance with respect to the representation of microphysics and dynamics in the initial phase of an MCAO. By considering the two cases with similar large-scale synoptic settings but differences with respect to microphysics, e.g., in LWP and riming, microphysical parameterization schemes can be evaluated. It will be particularly interesting to analyze whether these models successfully reproduce the observed factor of 2 in scaling found for several parameters between the two cases.
To study the impact of the sharpness of the MIZ and flow divergence on cloud evolution, more observations at a constant fetch over open water and at a variable fetch over the MIZ near the sea ice edge should be obtained in the future. Moreover, since the observed cloud top temperatures lie within the dendritic growth zone, aggregation is an important process to study, e.g., by dual-frequency radar observations (Chellini et al., 2022), in order to understand dominant precipitation-forming microphysical processes.
The Clouds over cOMPlEX environment (COMPEX) campaign planned for spring 2026 northwest of Svalbard will present the opportunity to better characterize the impact of the marginal sea ice zone (MIZ) on the air mass transformation. Flights within the MIZ and along the ice edge could increase the number of samples. Further, by enhancing our measurement suite with an airborne G-band radar, more information on cloud microphysics can be deduced.
To detect up- and downdrafts, we solely use , which is the radar reflectivity at the height of 0.7 of the hydrometeor depth (D; Sect. 2.2). Figure A1 explains the choice of this height (red line). To understand the applied height and Ze thresholds, one has to keep in mind that Ze values larger −5 dBZ (Schirmacher et al., 2023) and below 500 m (Shupe et al., 2008) height are defined as precipitation. At 0.7 of the hydrometeor depth, most largest cloud particles occur, i.e., most maximum Ze values per profile exceeding −10 dBZ among all non-precipitating values (Fig. A1a, gray line). Most maximum Ze values per profile among non-precipitating radar bins occur at the cloud bottom (Fig. A1a, black line). Nevertheless, this bottom near maximum is induced by very small Ze values below −10 dBZ. Since we aim to detect updrafts at the selected height, we take the height where large particles ( dBZ) occur most frequently. Moreover, with 98 %, most precipitation occurs below 0.7 of the hydrometeor depth (Fig. A1b, dashed black line). This finding is not sensitive to the −5 dBZ threshold for precipitation (Fig. A1b, dashed gray line). Dry entrainment and liquid droplets seem rare at 0.7 of the hydrometeor depth since 91 % of the lowest Ze values per profile lie above this altitude (Fig. A1b, solid black line). In conclusion, we take Ze at the height of 0.7 of the hydrometeor depth to consider the largest ice particles within the profiles and minimize the influence of dry-air entrainment and supercooled liquid water droplets at the cloud top and precipitation at the bottom of the hydrometeor depth.
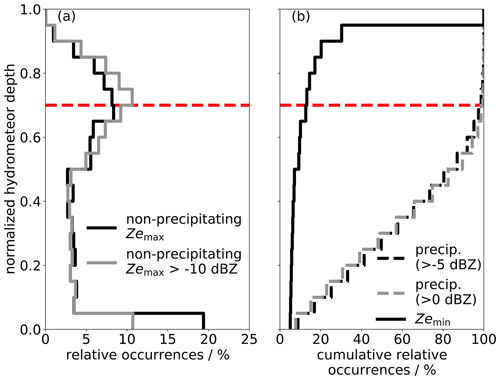
Figure A1Relative occurrences of among non-precipitating hydrometeors (a, solid black line) and of non-precipitating that exceed −10 dBZ (a, solid gray line). Relative occurrence of precipitation defined by Ze values larger than −5 dBZ (b, dashed black line) and larger than 0 dBZ (b, dashed gray line) and minimum Ze of each radar profile (b, solid black line). The y axis is the normalized hydrometeor depth (0 = base, 1 = top). The height that is used to identify roll circulations is indicated by the red line.
Table A1Sensitivity of the steps of the algorithm applied to identify roll circulation objects. Relative changes in the number of objects in total; number of objects inside the cloud street regime; cloud top height (CTH) of cloud streets; and aspect ratio (AR) of the roll circulation to the results obtained by the applied configuration after adjusting, i.e., mostly doubling, parameters.
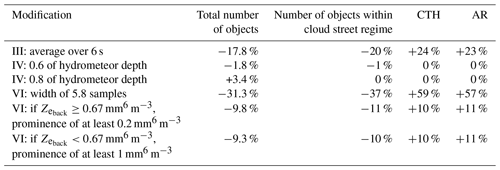
To find up- and downdraft regions using , we use the following method:
-
Determine 0.7 of D for every profile (Fig. 2b).
-
Average Ze over 100 m in the vertical to reduce noise.
-
Smooth Ze by averaging over 3 s to minimize noise detection (Fig. 2c).
-
Extract the smoothed at 0.7 of D for each profile, which is the average between 0.65 and 0.75 times D (Fig. 2d, black line).
-
Derive the large-scale background by averaging over 500 s (∼ 40 km; Fig. 2d, gray line).
-
Determine peaks in using the Python package scipy.signal.find_peaks (Virtanen et al., 2020).
If ≥0.67 mm6 m−3, find peaks for with a prominence of at least 0.5 mm6 m−3 (difference between the height of the peak and its lowest contour line; Fig. 2d, vertical orange line) and a width of at least 2.9 samples (about 230 m horizontal distance; Fig. 2d, horizontal orange line). Note that Fig. 2 shows Ze in logarithmic space, while peaks are detected in linear space.
If <0.67 mm6 m−3, find peaks for with a prominence of at least 0.1 mm6 m−3 and a width of at least 2.9 samples.
The detected peaks are defined as updrafts (Fig. 2e, vertical black lines). Here, we apply two different thresholds depending on since the magnitude of the averaged Ze and its peaks generally increase with fetch. -
Find the minimum between every two maxima (Fig. 2e, vertical gray line). If conditions between two cloud streets are cloud-free, we consider the downdraft location at the center of the cloud-free distance.
Processed radar, in situ, and dropsonde observations obtained during the HALO-(AC)3 campaign are published by Ehrlich et al. (2024). The retrieved LWP data are currently being prepared for publication on PANGAEA. All airborne data are accessed via the ac3airborne module (https://doi.org/10.5281/zenodo.7305585, Mech et al., 2022b). The merged MODIS–AMSR2 sea ice concentration data are provided by the Institute of Environmental Physics at the University of Bremen (https://data.seaice.uni-bremen.de/modis_amsr2/, Ludwig and Spreen, 2023). Raw in situ data are stored at the German Aerospace Center and available on request. Back trajectories are calculated from ERA5 reanalysis data (https://doi.org/10.24381/cds.143582cf, Hersbach et al., 2017, 2020). ERA5 is available for pressure levels (https://doi.org/10.24381/cds.bd0915c6, Hersbach et al., 2023a) and single levels (https://doi.org/10.24381/cds.adbb2d47, Hersbach et al., 2023b). A Python implementation of the COARE 3.5 bulk air–sea flux algorithm is available in https://doi.org/10.5281/zenodo.5110991 (Ludovic et al., 2021). MODIS observations of the total water path can be found in NASA Worldview (2023a) (https://go.nasa.gov/46vE70B) and of corrected reflectance in NASA Worldview (2023b) (https://go.nasa.gov/46o4aXO). The corrected reflectance observed by VIIRS is available in NASA Worldview (2023c) (https://go.nasa.gov/47mKJjr).
The supplement related to this article is available online at: https://doi.org/10.5194/acp-24-12823-2024-supplement.
IS analyzed and visualized the data, wrote the paper, and developed the methodology, and together with SaS, MK, AE, and SC, the paper was conceptualized and results were discussed. MM and AE developed the flight strategy for the cloud street investigation. BK calculated the back trajectories. NM collocated the P5 and P6 measurements and computed the rime mass fraction. All authors contributed to manuscript revisions.
The contact author has declared that none of the authors has any competing interests.
Publisher's note: Copernicus Publications remains neutral with regard to jurisdictional claims made in the text, published maps, institutional affiliations, or any other geographical representation in this paper. While Copernicus Publications makes every effort to include appropriate place names, the final responsibility lies with the authors.
This article is part of the special issue “HALO-(AC)3 – an airborne campaign to study air mass transformations during warm-air intrusions and cold-air outbreaks”. It is not associated with a conference.
We gratefully acknowledge the funding from the Deutsche Forschungsgemeinschaft (DFG, German Research Foundation; project no. 268020496) through the Transregional Collaborative Research Centre ArctiC Amplification: Climate Relevant Atmospheric and SurfaCe Processes and Feedback Mechanisms (AC)3 (TR 172) (sub-project B03). We are grateful for the support from the Alfred Wegener Institute, Deutsches Zentrum für Luft- und Raumfahrt (DLR, German Aerospace Center), and aircraft crews during the HALO-(AC)3 campaign. Moreover, we acknowledge the use of imagery from the NASA Worldview application (NASA Worldview, 2023a, b, c), part of the NASA Earth Observing System Data and Information System (EOSDIS). Furthermore, we thank the Institute of Environmental Physics at the University of Bremen for providing the merged MODIS–AMSR2 sea ice concentration dataset (Ludwig and Spreen, 2023). Many thanks go to the principal investigators (PIs): Stephan Borrmann, Johannes Schneider, and Veronika Pörtge. We are also grateful to Bjorn Stevens for discussing the flight strategy and cloud street investigation, Matt Shupe for discussing the roll circulation identification, and Vera Schemann for discussing future model evaluation efforts.
This research has been supported by the Deutsche Forschungsgemeinschaft (grant no. 268020496, TR 172).
This open-access publication was funded by Universität zu Köln.
This paper was edited by Markus Petters and reviewed by three anonymous referees.
Abel, S. J., Boutle, I. A., Waite, K., Fox, S., Brown, P. R. A., Cotton, R., Lloyd, G., Choularton, T. W., and Bower, K. N.: The Role of Precipitation in Controlling the Transition from Stratocumulus to Cumulus Clouds in a Northern Hemisphere Cold-Air Outbreak, J. Atmos. Sci., 74, 2293–2314, https://doi.org/10.1175/JAS-D-16-0362.1, 2017. a, b
Atkinson, B. W. and Wu Zhang, J.: Mesoscale shallow convection in the atmosphere, Rev. Geophys., 34, 403–431, https://doi.org/10.1029/96RG02623, 1996. a
Baumgardner, D., Brenguier, J. L., Bucholtz, A., Coe, H., DeMott, P., Garrett, T. J., Gayet, J. F., Hermann, M., Heymsfield, A., Korolev, A., Krämer, M., Petzold, A., Strapp, W., Pilewskie, P., Taylor, J., Twohy, C., Wendisch, M., Bachalo, W., and Chuang, P.: Airborne instruments to measure atmospheric aerosol particles, clouds and radiation: A cook's tour of mature and emerging technology, Atmos. Res., 102, 10–29, https://doi.org/10.1016/j.atmosres.2011.06.021, 2011. a, b
Brown, R. A.: On the Inflection Point Instability of a Stratified Ekman Boundary Layer, J. Atmos. Sci., 29, 850–859, https://doi.org/10.1175/1520-0469(1972)029<0850:OTIPIO>2.0.CO;2, 1972. a
Brummer, B., Schlunzen, H., and Bogel, W.: Cloud streets during Kontur, 63–77 pp., Hamburger Geophysikalische Einzelschriften, 1982. a
Brümmer, B.: Boundary-layer modification in wintertime cold-air outbreaks from the Arctic sea ice, Bound.-Lay. Meteorol., 80, 109–125, https://doi.org/10.1007/BF00119014, 1996. a, b, c
Brümmer, B.: Roll and Cell Convection in Wintertime Arctic Cold-Air Outbreaks, J. Atmos. Sci., 56, 2613–2636, https://doi.org/10.1175/1520-0469(1999)056<2613:RACCIW>2.0.CO;2, 1999. a, b
Brümmer, B., Bakan, S., and Hinzpeter, H.: Kontur: Observations of cloud streets and open cellular structures, Dynam. Atmos. Ocean., 9, 281–296, https://doi.org/10.1016/0377-0265(85)90024-7, 1985. a, b
Brümmer, B., Rump, B., and Kruspe, G.: A cold air outbreak near Spitsbergen in springtime – Boundary-layer modification and cloud development, Bound.-Lay. Meteorol., 61, 13–46, https://doi.org/10.1007/BF02033993, 1992. a, b
Chellini, G., Gierens, R., and Kneifel, S.: Ice Aggregation in Low‐Level Mixed‐Phase Clouds at a High Arctic Site: Enhanced by Dendritic Growth and Absent Close to the Melting Level, J. Geophys. Res.-Atmos., 127, 16, https://doi.org/10.1029/2022JD036860, 2022. a, b
Copernicus Marine Service: Arctic Ocean – Sea and Ice Surface Temperature, Copernicus [data], https://doi.org/10.48670/moi-00130, 2023. a
Dahlke, S., Solbès, A., and Maturilli, M.: Cold Air Outbreaks in Fram Strait: Climatology, Trends, and Observations During an Extreme Season in 2020, J. Geophys. Res.-Atmos., 127, e2021JD035741, https://doi.org/10.1029/2021JD035741, 2022. a, b
de Roode, S. R., Frederikse, T., Siebesma, A. P., Ackerman, A. S., Chylik, J., Field, P. R., Fricke, J., Gryschka, M., Hill, A., Honnert, R., Krueger, S. K., Lac, C., Lesage, A. T., and Tomassini, L.: Turbulent Transport in the Gray Zone: A Large Eddy Model Intercomparison Study of the CONSTRAIN Cold Air Outbreak Case, J. Adv. Model. Earth Syst., 11, 597–623, https://doi.org/10.1029/2018MS001443, 2019. a
Ehrlich, A., Crewell, S., Herber, A., Klingebiel, M., Lüpkes, C., Mech, M., Becker, S., Borrmann, S., Bozem, H., Buschmann, M., Clemen, H.-C., De La Torre Castro, E., Dorff, H., Dupuy, R., Eppers, O., Ewald, F., George, G., Giez, A., Grawe, S., Gourbeyre, C., Hartmann, J., Jäkel, E., Joppe, P., Jourdan, O., Jurányi, Z., Kirbus, B., Lucke, J., Luebke, A. E., Maahn, M., Maherndl, N., Mallaun, C., Mayer, J., Mertes, S., Mioche, G., Moser, M., Müller, H., Pörtge, V., Risse, N., Roberts, G., Rosenburg, S., Röttenbacher, J., Schäfer, M., Schaefer, J., Schäfler, A., Schirmacher, I., Schneider, J., Schnitt, S., Stratmann, F., Tatzelt, C., Voigt, C., Walbröl, A., Weber, A., Wetzel, B., Wirth, M., and Wendisch, M.: A comprehensive in-situ and remote sensing data set collected during the HALO–(AC)3 aircraft campaign, Earth Syst. Sci. Data Discuss. [preprint], https://doi.org/10.5194/essd-2024-281, in review, 2024. a
Fairall, C. W., Bradley, E. F., Hare, J. E., Grachev, A. A., and Edson, J. B.: Bulk Parameterization of Air–Sea Fluxes: Updates and Verification for the COARE Algorithm, J. Climate, 16, 571–591, https://doi.org/10.1175/1520-0442(2003)016<0571:BPOASF>2.0.CO;2, 2003. a
Geerts, B., Giangrande, S. E., McFarquhar, G. M., Xue, L., Abel, S. J., Comstock, J. M., Crewell, S., DeMott, P. J., Ebell, K., Field, P., Hill, T. C. J., Hunzinger, A., Jensen, M. P., Johnson, K. L., Juliano, T. W., Kollias, P., Kosovic, B., Lackner, C., Luke, E., Lüpkes, C., Matthews, A. A., Neggers, R., Ovchinnikov, M., Powers, H., Shupe, M. D., Spengler, T., Swanson, B. E., Tjernström, M., Theisen, A. K., Wales, N. A., Wang, Y., Wendisch, M., and Wu, P.: The COMBLE Campaign: A Study of Marine Boundary Layer Clouds in Arctic Cold-Air Outbreaks, B. Am. Meteorol. Soc., 103, E1371–E1389, https://doi.org/10.1175/BAMS-D-21-0044.1, 2022. a, b, c, d
George, G., Stevens, B., Bony, S., Pincus, R., Fairall, C., Schulz, H., Kölling, T., Kalen, Q. T., Klingebiel, M., Konow, H., Lundry, A., Prange, M., and Radtke, J.: JOANNE: Joint dropsonde Observations of the Atmosphere in tropical North atlaNtic meso-scale Environments, Earth Syst. Sci. Data, 13, 5253–5272, https://doi.org/10.5194/essd-13-5253-2021, 2021. a
Gryschka, M., Fricke, J., and Raasch, S.: On the impact of forced roll convection on vertical turbulent transport in cold air outbreaks, J. Geophys. Res.-Atmos., 119, 12–513, 2014. a
Hersbach, H., Bell, B., Berrisford, P., Hirahara, S., Horányi, A., Muñoz-Sabater, J., Nicolas, J., Peubey, C., Radu, R., Schepers, D., Simmons, A., Soci, C., Abdalla, S., Abellan, X., Balsamo, G., Bechtold, P., Biavati, G., Bidlot, J., Bonavita, M., De Chiara, G., Dahlgren, P., Dee, D., Diamantakis, M., Dragani, R., Flemming, J., Forbes, R., Fuentes, M., Geer, A., Haimberger, L., Healy, S., Hogan, R. J., Hólm, E., Janisková, M., Keeley, S., Laloyaux, P., Lopez, P., Lupu, C., Radnoti, G., de Rosnay, P., Rozum, I., Vamborg, F., Villaume, S., and Thépaut, J.-N.: Complete ERA5 from 1940: Fifth generation of ECMWF atmospheric reanalyses of the global climate, Copernicus Climate Change Service (C3S) Data Store (CDS), Copernicus [data set], https://doi.org/10.24381/cds.143582cf, 2017. a
Hersbach, H., Bell, B., Berrisford, P., Hirahara, S., Horányi, A., Muñoz-Sabater, J., Nicolas, J., Peubey, C., Radu, R., Schepers, D., Simmons, A., Soci, C., Abdalla, S., Abellan, X., Balsamo, G., Bechtold, P., Biavati, G., Bidlot, J., Bonavita, M., De Chiara, G., Dahlgren, P., Dee, D., Diamantakis, M., Dragani, R., Flemming, J., Forbes, R., Fuentes, M., Geer, A., Haimberger, L., Healy, S., Hogan, R. J., Hólm, E., Janisková, M., Keeley, S., Laloyaux, P., Lopez, P., Lupu, C., Radnoti, G., de Rosnay, P., Rozum, I., Vamborg, F., Villaume, S., and Thépaut, J.-N.: The ERA5 global reanalysis, Q. J. Roy. Meteorol. Soc., 146, 1999–2049, https://doi.org/10.1002/qj.3803, 2020. a, b
Hersbach, H., Bell, B., Berrisford, P., Biavati, G., Horányi, A., Muñoz Sabater, J., Nicolas, J., Peubey, C., Radu, R., Rozum, I., Schepers, D., Simmons, A., Soci, C., Dee, D., and Thépaut, J.-N.: ERA5 hourly data on pressure levels from 1940 to present, Copernicus Climate Change Service (C3S) Climate Data Store (CDS), Copernicus [data set], https://doi.org/10.24381/cds.bd0915c6, 2023a. a
Hersbach, H., Bell, B., Berrisford, P., Biavati, G., Horányi, A., Muñoz Sabater, J., Nicolas, J., Peubey, C., Radu, R., Rozum, I., Schepers, D., Simmons, A., Soci, C., Dee, D., and Thépaut, J.-N.: ERA5 hourly data on single levels from 1940 to present, Copernicus Climate Change Service (C3S) Climate Data Store (CDS), Copernicus [data set], https://doi.org/10.24381/cds.adbb2d47, 2023b. a
Kirbus, B., Schirmacher, I., Klingebiel, M., Schäfer, M., Ehrlich, A., Slättberg, N., Lucke, J., Moser, M., Müller, H., and Wendisch, M.: Thermodynamic and cloud evolution in a cold-air outbreak during HALO-(AC)3: quasi-Lagrangian observations compared to the ERA5 and CARRA reanalyses, Atmos. Chem. Phys., 24, 3883–3904, https://doi.org/10.5194/acp-24-3883-2024, 2024. a
Klingebiel, M., de Lozar, A., Molleker, S., Weigel, R., Roth, A., Schmidt, L., Meyer, J., Ehrlich, A., Neuber, R., Wendisch, M., and Borrmann, S.: Arctic low-level boundary layer clouds: in situ measurements and simulations of mono- and bimodal supercooled droplet size distributions at the top layer of liquid phase clouds, Atmos. Chem. Phys., 15, 617–631, https://doi.org/10.5194/acp-15-617-2015, 2015. a
Kolstad, E. W.: Higher ocean wind speeds during marine cold air outbreaks, Q. J. Roy. Meteorol. Soc., 143, 2084–2092, https://doi.org/10.1002/qj.3068, 2017. a
Korolev, A. and Field, P. R.: The effect of dynamics on mixed-phase clouds: Theoretical considerations, J. Atmos. Sci., 65, 66–86, 2008. a
Kulie, M. S. and Bennartz, R.: Utilizing Spaceborne Radars to Retrieve Dry Snowfall, J. Appl. Meteorol. Climatol., 48, 2564–2580, https://doi.org/10.1175/2009JAMC2193.1, 2009. a
Lackner, C. P., Geerts, B., Juliano, T. W., Xue, L., and Kosovic, B.: Vertical structure of clouds and precipitation during Arctic cold-air outbreaks and warm-air intrusions: Observations from COMBLE, J. Geophys. Res.-Atmos., 128, e2022JD038403, https://doi.org/10.1029/2022JD038403, 2023. a
Lance, S., Brock, C. A., Rogers, D., and Gordon, J. A.: Water droplet calibration of the Cloud Droplet Probe (CDP) and in-flight performance in liquid, ice and mixed-phase clouds during ARCPAC, Atmos. Meas. Tech., 3, 1683–1706, https://doi.org/10.5194/amt-3-1683-2010, 2010. a
Li, X., Krueger, S. K., Strong, C., Mace, G. G., and Benson, S.: Midwinter Arctic leads form and dissipate low clouds, Nat. Commun., 11, 206, https://doi.org/10.1038/s41467-019-14074-5, 2020. a
Ludovic, B., Byron, B., Christopher, F., Elizabeth, T., Jim, E., and Robert, P.: Python implementation of the COARE 3.5 Bulk Air-Sea Flux algorithm, Zenodo [code], https://doi.org/10.5281/zenodo.5110991, 2021. a
Ludwig, V. and Spreen, G.: modis_amsr2, University Bremen [data], https://data.seaice.uni-bremen.de/modis_amsr2/ (last access: 20 October 2023), 2023. a, b
Ludwig, V., Spreen, G., and Pedersen, L. T.: Evaluation of a New Merged Sea-Ice Concentration Dataset at 1 km Resolution from Thermal Infrared and Passive Microwave Satellite Data in the Arctic, Remote Sens., 12, 3183, https://doi.org/10.3390/rs12193183, 2020. a
Maahn, M., Burgard, C., Crewell, S., Gorodetskaya, I. V., Kneifel, S., Lhermitte, S., Van Tricht, K., and van Lipzig, N. P. M.: How does the spaceborne radar blind zone affect derived surface snowfall statistics in polar regions?, J. Geophys. Res.-Atmos., 119, 13604–13620, https://doi.org/10.1002/2014JD022079, 2014. a
Mages, Z., Kollias, P., Zhu, Z., and Luke, E. P.: Surface-based observations of cold-air outbreak clouds during the COMBLE field campaign, Atmos. Chem. Phys., 23, 3561–3574, https://doi.org/10.5194/acp-23-3561-2023, 2023. a
Maherndl, N., Maahn, M., Tridon, F., Leinonen, J., Ori, D., and Kneifel, S.: A riming-dependent parameterization of scattering by snowflakes using the self-similar Rayleigh–Gans approximation, Q. J. Roy. Meteorol. Soc., 149, 1–20, https://doi.org/10.1002/qj.4573, 2023. a
Maherndl, N., Moser, M., Lucke, J., Mech, M., Risse, N., Schirmacher, I., and Maahn, M.: Quantifying riming from airborne data during the HALO-(AC)3 campaign, Atmos. Meas. Tech., 17, 1475–1495, https://doi.org/10.5194/amt-17-1475-2024, 2024. a
Markson, R.: Atmospheric Electrical Detection of Organized Convection, Am. Assoc. Adv. Sci., 188, 1171–1177, https://doi.org/10.1126/science.188.4194.1171, 1975. a
Mateling, M. E., Pettersen, C., Kulie, M. S., and L’Ecuyer, T. S.: Marine Cold‐Air Outbreak Snowfall in the North Atlantic: A CloudSat Perspective, J. Geophys. Res.-Atmos., 128, e2022JD038053, https://doi.org/10.1029/2022JD038053, 2023. a, b
Mech, M., Kliesch, L.-L., Anhäuser, A., Rose, T., Kollias, P., and Crewell, S.: Microwave Radar/radiometer for Arctic Clouds (MiRAC): first insights from the ACLOUD campaign, Atmos. Meas. Tech., 12, 5019–5037, https://doi.org/10.5194/amt-12-5019-2019, 2019. a, b
Mech, M., Ehrlich, A., Herber, A., Lüpkes, C., Wendisch, M., Becker, S., Boose, Y., Chechin, D., Crewell, S., Dupuy, R., Gourbeyre, C., Hartmann, J., Jäkel, E., Jourdan, O., Kliesch, L.-L., Klingebiel, M., Kulla, B. S., Mioche, G., Moser, M., Risse, N., Ruiz-Donoso, E., Schäfer, M., Stapf, J., and Voigt, C.: MOSAiC-ACA and AFLUX – Arctic airborne campaigns characterizing the exit area of MOSAiC, Sci. Data, 9, 790, https://doi.org/10.1038/s41597-022-01900-7, 2022a. a, b, c, d, e
Mech, M., Risse, N., Marrollo, G., and Paul, D.: Ac3airborne, Zenodo [code], https://doi.org/10.5281/zenodo.7305585, 2022b. a
Morrison, H., de Boer, G., Feingold, G., Harrington, J., Shupe, M. D., and Sulia, K.: Resilience of persistent Arctic mixed-phase clouds, Nat. Geosci., 5, 11–17, https://doi.org/10.1038/ngeo1332, 2012. a
Moser, M., Voigt, C., Jurkat-Witschas, T., Hahn, V., Mioche, G., Jourdan, O., Dupuy, R., Gourbeyre, C., Schwarzenboeck, A., Lucke, J., Boose, Y., Mech, M., Borrmann, S., Ehrlich, A., Herber, A., Lüpkes, C., and Wendisch, M.: Microphysical and thermodynamic phase analyses of Arctic low-level clouds measured above the sea ice and the open ocean in spring and summer, Atmos. Chem. Phys., 23, 7257–7280, https://doi.org/10.5194/acp-23-7257-2023, 2023. a, b
Müller, G., Brümmer, B., and Alpers, W.: Roll Convection within an Arctic Cold-Air Outbreak: Interpretation of In Situ Aircraft Measurements and Spaceborne SAR Imagery by a Three-Dimensional Atmospheric Model, Mon. Weather Rev., 127, 363–380, https://doi.org/10.1175/1520-0493(1999)127<0363:RCWAAC>2.0.CO;2, 1999. a
Murray-Watson, R. J., Gryspeerdt, E., and Goren, T.: Investigating the development of clouds within marine cold-air outbreaks, Atmos. Chem. Phys., 23, 9365–9383, https://doi.org/10.5194/acp-23-9365-2023, 2023. a
NASA Worldview: Cloud Water Path Terra/MODIS [data set], https://go.nasa.gov/46vE70B (last access: 21 November 2023), 2023a. a, b, c
NASA Worldview: Corrected Reflectance (True Color) Terra/MODIS [data set], https://go.nasa.gov/46o4aXO (last access: 21 November 2023), 2023b. a, b, c
NASA Worldview: Corrected Reflectance (Bands M3-I3-M11) Suomi NPP/VIIRS [data set], https://go.nasa.gov/47mKJjr (last access: 21 November 2023), 2023c. a, b, c
Papritz, L., Pfahl, S., Sodemann, H., and Wernli, H.: A Climatology of Cold Air Outbreaks and Their Impact on Air-Sea Heat Fluxes in the High-Latitude South Pacific, J. Climate, 28, 342–364, https://doi.org/10.1175/JCLI-D-14-00482.1, 2015. a, b
Pithan, F., Svensson, G., Caballero, R., Chechin, D., Cronin, T. W., Ekman, A. M. L., Neggers, R., Shupe, M. D., Solomon, A., Tjernström, M., and Wendisch, M.: Role of air-mass transformations in exchange between the Arctic and mid-latitudes, Nat. Geosci., 11, 805–812, https://doi.org/10.1038/s41561-018-0234-1, 2018. a
Ruiz-Donoso, E., Ehrlich, A., Schäfer, M., Jäkel, E., Schemann, V., Crewell, S., Mech, M., Kulla, B. S., Kliesch, L.-L., Neuber, R., and Wendisch, M.: Small-scale structure of thermodynamic phase in Arctic mixed-phase clouds observed by airborne remote sensing during a cold air outbreak and a warm air advection event, Atmos. Chem. Phys., 20, 5487–5511, https://doi.org/10.5194/acp-20-5487-2020, 2020. a, b, c
Schirmacher, I., Kollias, P., Lamer, K., Mech, M., Pfitzenmaier, L., Wendisch, M., and Crewell, S.: Assessing Arctic low-level clouds and precipitation from above – a radar perspective, Atmos. Meas. Tech., 16, 4081–4100, https://doi.org/10.5194/amt-16-4081-2023, 2023. a, b, c, d, e, f
Seethala, C., Zuidema, P., Edson, J., Brunke, M., Chen, G., Li, X.-Y., Painemal, D., Robinson, C., Shingler, T., Shook, M., Sorooshian, A., Thornhill, L., Tornow, F., Wang, H., Zeng, X., and Ziemba, L.: On Assessing ERA5 and MERRA2 Representations of Cold-Air Outbreaks Across the Gulf Stream, Geophys. Res. Lett., 48, e2021GL094364, https://doi.org/10.1029/2021GL094364, 2021. a
Shestakova, A. A., Chechin, D. G., Lüpkes, C., Hartmann, J., and Maturilli, M.: The foehn effect during easterly flow over Svalbard, Atmos. Chem. Phys., 22, 1529–1548, https://doi.org/10.5194/acp-22-1529-2022, 2022. a
Shupe, M. D., Kollias, P., Persson, P. O. G., and McFarquhar, G. M.: Vertical Motions in Arctic Mixed-Phase Stratiform Clouds, J. Atmos. Sci., 65, 1304–1322, https://doi.org/10.1175/2007JAS2479.1, 2008. a, b, c
Spensberger, C. and Spengler, T.: Sensitivity of Air-Sea Heat Exchange in Cold-Air Outbreaks to Model Resolution and Sea-Ice Distribution, J. Geophys. Res.-Atmos., 126, e2020JD033610, https://doi.org/10.1029/2020JD033610, 2021. a, b, c, d
Sprenger, M. and Wernli, H.: The LAGRANTO Lagrangian analysis tool – version 2.0, Geosci. Model Dev., 8, 2569–2586, https://doi.org/10.5194/gmd-8-2569-2015, 2015. a, b
Stachlewska, I. S., Neuber, R., Lampert, A., Ritter, C., and Wehrle, G.: AMALi – the Airborne Mobile Aerosol Lidar for Arctic research, Atmos. Chem. Phys., 10, 2947–2963, https://doi.org/10.5194/acp-10-2947-2010, 2010. a
Tornow, F., Ackerman, A. S., and Fridlind, A. M.: Preconditioning of overcast-to-broken cloud transitions by riming in marine cold air outbreaks, Atmos. Chem. Phys., 21, 12049–12067, https://doi.org/10.5194/acp-21-12049-2021, 2021. a, b
Tornow, F., Ackerman, A. S., Fridlind, A. M., Tselioudis, G., Cairns, B., Painemal, D., and Elsaesser, G.: On the Impact of a Dry Intrusion Driving Cloud-Regime Transitions in a Midlatitude Cold-Air Outbreak, J. Atmos. Sci., 80, 2881–2896, https://doi.org/10.1175/JAS-D-23-0040.1, 2023. a
Turner, J. and Marshall, G. J.: Climate change in the polar regions, Cambridge University Press, ISBN 9780521850100, https://books.google.de/books?id=klTlaWWWxNoC (last access: 23 August 2024), 2011. a
Vaisala: Vaisala Dropsonde RD94, https://www.vaisala.com/sites/default/files/documents/RD94-Dropsonde-Datasheet-B210936EN-A-LoRes.pdf (last access: 31 October 2023), 2010. a
Virtanen, P., Gommers, R., Oliphant, T. E., Haberland, M., Reddy, T., Cournapeau, D., Burovski, E., Peterson, P., Weckesser, W., Bright, J., van der Walt, S. J., Brett, M., Wilson, J., Millman, K. J., Mayorov, N., Nelson, A. R. J., Jones, E., Kern, R., Larson, E., Carey, C. J., Polat, İ., Feng, Y., Moore, E. W., VanderPlas, J., Laxalde, D., Perktold, J., Cimrman, R., Henriksen, I., Quintero, E. A., Harris, C. R., Archibald, A. M., Ribeiro, A. H., Pedregosa, F., van Mulbregt, P., and SciPy 1.0 Contributors: SciPy 1.0: Fundamental Algorithms for Scientific Computing in Python, Nat. Methods, 17, 261–272, https://doi.org/10.1038/s41592-019-0686-2, 2020. a
von Lerber, A., Mech, M., Rinke, A., Zhang, D., Lauer, M., Radovan, A., Gorodetskaya, I., and Crewell, S.: Evaluating seasonal and regional distribution of snowfall in regional climate model simulations in the Arctic, Atmos. Chem. Phys., 22, 7287–7317, https://doi.org/10.5194/acp-22-7287-2022, 2022. a
Walbröl, A., Michaelis, J., Becker, S., Dorff, H., Ebell, K., Gorodetskaya, I., Heinold, B., Kirbus, B., Lauer, M., Maherndl, N., Maturilli, M., Mayer, J., Müller, H., Neggers, R. A. J., Paulus, F. M., Röttenbacher, J., Rückert, J. E., Schirmacher, I., Slättberg, N., Ehrlich, A., Wendisch, M., and Crewell, S.: Contrasting extremely warm and long-lasting cold air anomalies in the North Atlantic sector of the Arctic during the HALO-(𝒜𝒞)3 campaign, Atmos. Chem. Phys., 24, 8007–8029, https://doi.org/10.5194/acp-24-8007-2024, 2024. a, b
Walter, B. A. and Overland, J. E.: Observations of Longitudinal Rolls in a Near Neutral Atmosphere, Mon. Weather Rev., 112, 200–208, https://doi.org/10.1175/1520-0493(1984)112<0200:OOLRIA>2.0.CO;2, 1984. a, b
Wendisch, M., Handorf, D., Tegen, I., Neggers, R., and Spreen, G.: Glimpsing the Ins and Outs of the Arctic Atmospheric Cauldron, Eos, 102, https://doi.org/10.1029/2021EO155959, 2021. a
Wendisch, M., Brückner, M., Crewell, S., Ehrlich, A., Notholt, J., Lüpkes, C., Macke, A., Burrows, J. P., Rinke, A., Quaas, J., Maturilli, M., Schemann, V., Shupe, M. D., Akansu, E. F., Barrientos-Velasco, C., Bärfuss, K., Blechschmidt, A.-M., Block, K., Bougoudis, I., Bozem, H., Böckmann, C., Bracher, A., Bresson, H., Bretschneider, L., Buschmann, M., Chechin, D. G., Chylik, J., Dahlke, S., Deneke, H., Dethloff, K., Donth, T., Dorn, W., Dupuy, R., Ebell, K., Egerer, U., Engelmann, R., Eppers, O., Gerdes, R., Gierens, R., Gorodetskaya, I. V., Gottschalk, M., Griesche, H., Gryanik, V. M., Handorf, D., Harm-Altstädter, B., Hartmann, J., Hartmann, M., Heinold, B., Herber, A., Herrmann, H., Heygster, G., Höschel, I., Hofmann, Z., Hölemann, J., Hünerbein, A., Jafariserajehlou, S., Jäkel, E., Jacobi, C., Janout, M., Jansen, F., Jourdan, O., Jurányi, Z., Kalesse-Los, H., Kanzow, T., Käthner, R., Kliesch, L. L., Klingebiel, M., Knudsen, E. M., Kovács, T., Körtke, W., Krampe, D., Kretzschmar, J., Kreyling, D., Kulla, B., Kunkel, D., Lampert, A., Lauer, M., Lelli, L., Lerber, A. v., Linke, O., Löhnert, U., Lonardi, M., Losa, S. N., Losch, M., Maahn, M., Mech, M., Mei, L., Mertes, S., Metzner, E., Mewes, D., Michaelis, J., Mioche, G., Moser, M., Nakoudi, K., Neggers, R., Neuber, R., Nomokonova, T., Oelker, J., Papakonstantinou-Presvelou, I., Pätzold, F., Pefanis, V., Pohl, C., Pinxteren, M. v., Radovan, A., Rhein, M., Rex, M., Richter, A., Risse, N., Ritter, C., Rostosky, P., Rozanov, V. V., Donoso, E. R., Garfias, P. S., Salzmann, M., Schacht, J., Schäfer, M., Schneider, J., Schnierstein, N., Seifert, P., Seo, S., Siebert, H., Soppa, M. A., Spreen, G., Stachlewska, I. S., Stapf, J., Stratmann, F., Tegen, I., Viceto, C., Voigt, C., Vountas, M., Walbröl, A., Walter, M., Wehner, B., Wex, H., Willmes, S., Zanatta, M., and Zeppenfeld, S.: Atmospheric and Surface Processes, and Feedback Mechanisms Determining Arctic Amplification: A Review of First Results and Prospects of the (AC)3 Project, B. Am. Meteorol. Soc., 104, E208–E242, https://doi.org/10.1175/BAMS-D-21-0218.1, 2023. a
Wendisch, M., Crewell, S., Ehrlich, A., Herber, A., Kirbus, B., Lüpkes, C., Mech, M., Abel, S. J., Akansu, E. F., Ament, F., Aubry, C., Becker, S., Borrmann, S., Bozem, H., Brückner, M., Clemen, H.-C., Dahlke, S., Dekoutsidis, G., Delanoë, J., De La Torre Castro, E., Dorff, H., Dupuy, R., Eppers, O., Ewald, F., George, G., Gorodetskaya, I. V., Grawe, S., Groß, S., Hartmann, J., Henning, S., Hirsch, L., Jäkel, E., Joppe, P., Jourdan, O., Jurányi, Z., Karalis, M., Kellermann, M., Klingebiel, M., Lonardi, M., Lucke, J., Luebke, A. E., Maahn, M., Maherndl, N., Maturilli, M., Mayer, B., Mayer, J., Mertes, S., Michaelis, J., Michalkov, M., Mioche, G., Moser, M., Müller, H., Neggers, R., Ori, D., Paul, D., Paulus, F. M., Pilz, C., Pithan, F., Pöhlker, M., Pörtge, V., Ringel, M., Risse, N., Roberts, G. C., Rosenburg, S., Röttenbacher, J., Rückert, J., Schäfer, M., Schaefer, J., Schemann, V., Schirmacher, I., Schmidt, J., Schmidt, S., Schneider, J., Schnitt, S., Schwarz, A., Siebert, H., Sodemann, H., Sperzel, T., Spreen, G., Stevens, B., Stratmann, F., Svensson, G., Tatzelt, C., Tuch, T., Vihma, T., Voigt, C., Volkmer, L., Walbröl, A., Weber, A., Wehner, B., Wetzel, B., Wirth, M., and Zinner, T.: Overview: quasi-Lagrangian observations of Arctic air mass transformations – introduction and initial results of the HALO-(𝒜𝒞)3 aircraft campaign, Atmos. Chem. Phys., 24, 8865–8892, https://doi.org/10.5194/acp-24-8865-2024, 2024. a
Wesche, C., Steinhage, D., and Nixdorf, U.: Polar aircraft Polar5 and Polar6 operated by the Alfred Wegener Institute, Journal of large-scale research facilities JLSRF, 2, A87–A87, https://doi.org/10.17815/jlsrf-2-153, 2016. a
Wu, P. and Ovchinnikov, M.: Cloud Morphology Evolution in Arctic Cold-Air Outbreak: Two Cases During COMBLE Period, J. Geophys. Res.-Atmos., 127, e2021JD035966, https://doi.org/10.1029/2021JD035966, 2022. a
Ziereis, H. and Gläßer, M.: HALO – Global Player für die Atmosphärenforschung, DLR Nachrichten, 32–36 pp., https://www.dlr.de/de/medien/publikationen/sonstige-publikationen/2011/global-player-fuer-die-atmosphaerenforschung-halo_86/@@download/file (last access: 23 August 2024), 2006. a
- Abstract
- Introduction
- Data
- Analysis and identification approaches
- Variability in thermodynamic conditions and cloud street properties
- Conclusions
- Appendix A: Peak detection algorithm and sensitivity
- Data availability
- Author contributions
- Competing interests
- Disclaimer
- Special issue statement
- Acknowledgements
- Financial support
- Review statement
- References
- Supplement
- Abstract
- Introduction
- Data
- Analysis and identification approaches
- Variability in thermodynamic conditions and cloud street properties
- Conclusions
- Appendix A: Peak detection algorithm and sensitivity
- Data availability
- Author contributions
- Competing interests
- Disclaimer
- Special issue statement
- Acknowledgements
- Financial support
- Review statement
- References
- Supplement