the Creative Commons Attribution 4.0 License.
the Creative Commons Attribution 4.0 License.
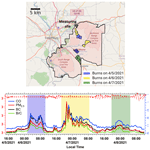
A multi-site passive approach to studying the emissions and evolution of smoke from prescribed fires
Rime El Asmar
Zongrun Li
David J. Tanner
Yongtao Hu
Susan O'Neill
L. Gregory Huey
M. Talat Odman
We conducted a 2-year study utilizing a network of fixed sites with sampling throughout an extended prescribed burning period to characterize the emissions and evolution of smoke from silvicultural prescribed burning at a military base in the southeastern USA. The measurement approach and an assessment of the instrument performance are described. Smoke sources, including those within and off the base, are identified, and plume ages are determined to quantify emissions and study the evolution of smoke PM2.5 (particulate matter with aerodynamic diameters 2.5 µm or smaller) mass, black carbon (BC), and brown carbon (BrC). Over the 2021 and 2022 prescribed burning seasons (nominally January to May), we identified 64 smoke events based on high levels of PM2.5 mass, BC, BrC, and carbon monoxide (CO), of which 61 were linked to a specific burning area. Smoke transport times were estimated in two ways: using the mean wind speed and the distance between the fire and the measurement site, and from Hybrid Single-Particle Lagrangian Integrated Trajectory (HYSPLIT) back-trajectories. PM2.5 emission ratios based on ΔPM2.5 mass ΔCO for fresh smoke (age ≤ 1 h) ranged between 0.04 and 0.18 µg m−3 ppb−1 with a mean of 0.117 µg m−3 ppb−1 (median of 0.121 µg m−3 ppb−1). Both the mean emission ratio and the variability were similar to findings from other prescribed fire studies but were lower than those from wildfires. The mean emission ratios of BC and BrC were 0.014 µg m−3 ppb−1 and 0.442 Mm−1 ppb−1, respectively. Ozone enhancements (ΔO3) were always observed in plumes detected in the afternoon. ΔPM2.5 mass ΔCO was observed to increase with plume age in all of the ozone-enhanced plumes, suggesting photochemical secondary aerosol formation. In contrast, ΔBrC/ΔCO was not found to vary with plume ages less than 8 h during photochemically active periods.
- Article
(8804 KB) - Full-text XML
-
Supplement
(2700 KB) - BibTeX
- EndNote
Large and intense wildfires have been increasing over the past few decades, and their emissions are a critical concern (Singleton et al., 2019; Jaffe et al., 2020). Fire is also an essential ecological process, and prescribed burning, which is the act of starting controlled fires for specific purposes, is an important tool for restoration of ecosystems, land management, and reducing fuel to prevent destructive wildfires (Kelp et al., 2023). Prescribed fires are typically conducted under favorable conditions associated with fuel type and amount, soil moisture, and meteorology. For example, in 2018, the United States Department of Agriculture (USDA) Forest Service indicated a high risk of hazardous wildfires over approximately 234 million acres (∼ 95 million hectares) of forest lands in the USA (Wyden and Manchin, 2020). However, prescribed fires were conducted over approximately 8.5 million forestry and rangeland acres (3.4 million hectares) in 2018 (Melvin, 2020). The southeastern USA has a long history of using prescribed fires (Melvin, 2021). For example, in 2017, 7.6 million acres (3 million hectares) out of the 11.3 million acres (4.6 million hectares) burned nationally were in the southeast (Melvin, 2018). Florida and Georgia each exceeded 1 million acres (0.4 million hectares) burned annually (Melvin, 2018). Recognizing the need to mitigate the size and severity of wildfires, prescribed burning is anticipated to increase in the coming years (USDA, 2022).
While prescribed burning can be performed under favorable weather conditions, it can still contribute to serious local and regional air pollution, as it is a source of primary and secondary air pollutants (Lee et al., 2008). Like other types of biomass burning, prescribed burning releases large amounts of particulate matter, CO, and inorganic and organic compounds (Lee et al., 2005), which have negative effects on health and visibility (Bell, 2004; Huang et al., 2019). Particularly in the southeastern USA, prescribed burning was significantly associated with high PM2.5 (particulate matter with aerodynamic diameters 2.5 µm or smaller) levels (Afrin and Garcia-Menendez, 2020; Larkin et al., 2020). Prescribed fires are often conducted at urban–rural interfaces, creating a buffer zone to prevent the spread of wildfires to the built environment. However, this means that planned fires often occur closer to populated areas and potentially lead to high population exposure due to this proximity. Although prescribed fires generally produce less pollutants by consuming less fuel per area burned than wildfires, the population health costs can be substantially higher for prescribed fires due to burning near higher population densities (Borchers-Arriagada et al., 2021).
Both wildfires and prescribed fires emit a large variety of gases and particulates (X. Liu et al., 2017; Burling et al., 2011; Gkatzelis et al., 2024; Permar et al., 2021; Travis et al., 2023). The gases include nitrogen oxides and volatile organic compounds, which can form ozone and secondary particulate matter. Hazardous air pollutants are also produced but may be less detrimental to exposed populations than particulates (O'Dell et al., 2022). PM2.5 is directly emitted as primary particles and is also formed from condensation of emitted gases and their oxidation products (Liu et al., 2016; May et al., 2014). While secondary organic aerosol (SOA) can be a significant component of aged biomass burning PM2.5, its contribution changes depending on emissions and atmospheric conditions. Additionally, the volatile nature of primary and secondary components of PM2.5 can lead to evaporation and a net loss of mass as the plume ages. PM2.5 exposure has been linked by many epidemiological studies to serious health problems, e.g., respiratory, cardiovascular, and neurological diseases as well as an increased risk of adverse birth outcomes (Liu et al., 2015; Reid et al., 2016; Naeher et al., 2007; Yu et al., 2023; Xi et al., 2020; Garcia et al., 2023). Given their significant impact on the environment and health, satellite, airborne, or ground-based studies of smoke emissions have been conducted extensively.
Detection and characterization of wildland fires is an important step towards assessing their impacts. Remote sensing via satellites can detect wildland fires by thermal anomalies (Kuenzer et al., 2008) or vegetation changes (Mildrexler et al., 2007). While satellite-based approaches offer valuable insights (Martinsson et al., 2022; Ichoku and Kaufman, 2005; Christopher et al., 1998), challenges such as cloud cover, spatial resolution limitations, and the complex nature of fire emissions can hinder accurate detection and quantification of fire impacts, especially for lower-intensity fires like prescribed burns (Liu et al., 2019; Wang et al., 2018; Martin et al., 2018). Therefore, factors like fire radiative power (FRP), burned area estimation, and fuel consumption modeling are often integrated into fire monitoring systems (Li et al., 2020; Nguyen and Wooster, 2020).
Aircraft (fixed-wing and helicopters) and more recently drones have been commonly used in airborne studies of wildland fires (Decker et al., 2021b; Cubison et al., 2011; Aurell and Gullett, 2024) and have been deployed for prescribed burning studies (Yokelson et al., 1999; May et al., 2014; Pratt et al., 2011; Aurell et al., 2021). Airborne studies provide high-spatial-resolution data that are often used to assess the evolution of smoke properties by measurements at various downwind distances. However, this is discontinuous and can miss certain aspects of smoke emissions, such as longer-term smoldering, especially at night (Burling et al., 2011). Employing a combination of airborne and ground-based measurements can be beneficial in providing a comprehensive view of the plume (Burling et al., 2011; Akagi et al., 2014; Yokelson et al., 2013; Strand et al., 2016).
In ground-based studies, mobile labs may capture dynamic air quality patterns and to some extent assess the spatial variability of species in plumes and their changes with plume age (Levy et al., 2014; Fiddler et al., 2024; Lee et al., 2023). However, they are usually limited in space and instrumentation capacity, such as filter samples collected only during stationary measurements (Warneke et al., 2023). Interferences from the power source, vibration, and speed changes during transportation can affect the instrument stability and performance, leading to inaccurate measurements or limiting the types of instruments that can be used. Attempting to track wildland smoke plumes can be challenging due to unpredictable winds and dispersion conditions combined with access limitations. For example, Burling et al. (2011) reported successfully sampling smoke from 2 out of 14 prescribed fires using a battery-powered mobile Fourier transform infrared (FTIR) system.
Fixed ground-based monitoring stations equipped with various instruments provide continuous, localized measurements for short- or long-term monitoring by studies assessing diurnal, seasonal, and long-term trends in air pollution. Multiple sites provide spatial coverage within a region. A variety of highly sensitive instruments can be deployed, ensuring accurate and precise measurements of various pollutants that can be compared with air quality data across different locations for regional assessments (Strand et al., 2016; Warneke et al., 2023). The importance of pre-existing fixed monitoring sites lies in their ability to capture wildfire smoke events that can occur at any time (Selimovic et al., 2019; Jaffe et al., 2022). These sites often include regulatory monitoring stations, which are highly valuable for studying local and regional smoke impacts over both short- and long-term periods. For example, Jaffe et al. (2022) used PM2.5 and CO observations from a regulatory monitoring site in Sparks, Nevada, collected from May to September between 2018 and 2021, as indicators of wildfire smoke in urban areas (Jaffe et al., 2022). Investigating the emissions and evolution of prescribed fires based on fixed sites is not as common, and there are limitations with this approach, but also some advantages.
Here, we present the results from a 2-year study utilizing fixed monitoring stations and continuous sampling in a region of active prescribed burning at Fort Moore in central Georgia, USA. The observations are analyzed to identify smoke plumes and determine their sources, such as those set within the fort or from burning in surrounding areas. We also use these data to estimate the age of the detected smoke to determine emission ratios and changes with plume age in PM2.5 mass, black carbon (BC), brown carbon (BrC), and their variability. Not all smoke from the prescribed fires set within the fort is detected, so the overall impact of all the fires on the regional air quality cannot be determined and is better addressed by a model simulation. Instead, our goal is to sample multiple smoke events so that an analysis of the data will provide a robust characterization of smoke from prescribed burning within the fort and in the region and with sufficient data to evaluate ground-level pollutant concentrations predicted by “smoke” models in prescribed fire simulations. Our concentration data cover measurements over a large range of distances from the burn plots. Fresh plume measurements with ages less than 1 h can be used to evaluate the predictions of local-scale models such as the Wildland Fire Dynamics Simulator (WFDS) urban interface (Mell et al., 2007) and QUIC-Fire (Linn et al., 2020). They can also be used to evaluate the emissions and plume rise parameterizations of larger-scale models like the BlueSky framework (Larkin et al., 2009). Additionally, more aged smoke measurements can be used to test the predictions of downwind concentrations in coupled fire–atmosphere models such as the Weather Research and Forecasting model, coupled with the fire-spread model (WRF-SFIRE) (Mandel et al., 2011) as well as chemical transport models like the Community Multiscale Air Quality (CMAQ) model (Appel et al., 2021), equipped with fire plume parameterizations. In the following sections, we describe the methodology, data analysis approach, and studies of various detected or missed smoke plumes, so that attribution of smoke from fires within the fort can be assessed. Findings on the emission estimates of PM2.5 mass, BC, BrC, and their evolution are compared to other prescribed and wildfire studies. These findings can help to assess the impact of prescribed burns by a specific entity or organization on a variety of public health and policy issues.
2.1 Site description
Prescribed burning at Fort Moore Army Base (formerly Fort Benning), in western central Georgia, United States, was studied from March through May 2021 and February through May 2022. Since 1981, prescribed burning has been used as a land management tool at the 182 000 acres (∼ 74 000 ha) military base, of which 145 000 acres (∼ 59 000 ha) are forested lands. Vegetation is characterized by pine-dominated uplands and hardwood-dominated bottom lands, with the dominant tree species being longleaf pine and white oak, respectively. Small wildfires ignited during military training exercises also occur at the base, and the land managers have been recording data on both prescribed fires and wildfires since the 1980s. Prescribed burning at the fort has been effective: it has reduced the frequency of wildfires from ∼ 300 to 500 wildfires per year in the early 1980s to less than 100 wildfires per year in the mid-1990s. During this period the prescribed fire-burned area changed from ∼ 7500 acres (∼ 3000 ha) in 1981 to ∼ 12 000 acres (∼ 5000 ha) in 1992. Currently, 30 000 woodland acres (∼ 12 000 ha) are burned annually using controlled fires, with future planned burning of 45 000 acres (∼ 18 000 ha) annually. Prescribed burning at the fort is also used for ecological objectives, such as restoring the longleaf pine forest and creating and maintaining the habitat for red-cockaded woodpeckers. Prescribed burning occurs from December through May, when there is sufficient but not excessive rainfall and suitable temperatures and wind conditions to burn deadwood, brush, and low-growing vegetation accumulating on the forest floor. The area of the base is divided into 332 burn units that range in size from 100 to 1800 acres (∼ 40 to 728 ha) and are burned alternately every 2 to 3 years.
2.2 Measuring sites
One instrumented research trailer (7′ W × 18′ L × 6.5′ H) was deployed in the 2021 burning season (18 March to 15 May 2021), and successive trailers (6′ W × 12′ L × 7′ H) were added in 2022 (11 February to 18 May 2022), reaching a total of five trailers located at different sites throughout the fort. In 2021, the one trailer operated at the same location until it was moved on 26 April 2021 to a new site for the remaining season as the expected burning regions at the fort changed. The trailers sampled continuously, except during periods of power loss or technical issues. The locations of the trailers, shown in Fig. 1, were chosen based on power availability, prevailing wind, and burning plans set prior to the burning season.
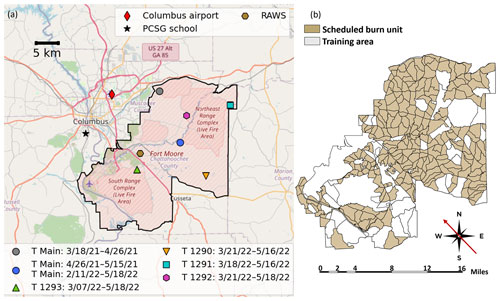
Figure 1Study region overview. The (a) Fort Moore map with the locations of the trailers, the RAWS weather station, and two state-operated sampling sites (Columbus Airport and Phenix City South Girard (PCSG) school) are shown along with the location of the city of Columbus, GA. (b) Fort Moore map showing the planned burn units for the year 2021, sourced from the Fort Moore authorities and natural resource management team, with the prevailing winds in the region.
2.3 Instrumentation
To characterize the prescribed fire smoke, the trailers were equipped with several instruments selected based on factors such as availability, ability for extended standalone operation, and significance to the study. All sampling was done through inlets nominally 4 m above ground level and 1.5 m above the trailer roof. The measurements included carbon monoxide (CO), nitrogen oxides (NO, NO2, NOx), ozone (O3), PM2.5 mass concentration, BC concentration, and BrC light absorption coefficients. Carbon monoxide serves as a standard tracer for combustion sources in atmospheric chemistry studies since it is a relatively long-lived species, with a typical lifetime of ∼ 1 month, emitted during incomplete combustion and used as a tracer of smoke movement and dispersion (Forrister et al., 2015; Liu et al., 2016). Other forms of incomplete combustion emissions (e.g., mobile sources) and oxidation of volatile organic compounds (VOCs) are also CO sources. CO mixing ratios were measured by IR analyzers (Thermo Fisher Scientific Inc, model 48C, Franklin, MA) with a lower detection limit (LOD) of 0.04 ppm at an averaging time of 390 s. The measurements alternated between blank and ambient measurements every 195 s. The blanks were determined with a custom-built CO scrubber made of 0.5 % Pd on an alumina catalyst heated to 180 °C (Parrish et al., 1994), which oxidizes CO to CO2. Calibration of CO analyzers was performed at a 2.2 ppm concentration before and after each field study using a 100 ppm CO in-air standard purchased from nexAir (Memphis, TN).
O3 was measured using an ultraviolet (UV) photometric analyzer (Thermo Fisher Scientific Inc, model 49C, Franklin, MA) zeroed through an O3 scrubber in the instrument, with an LOD of 1.0 ppb and an averaging time of 20 s. The analyzer was calibrated before and after each field deployment using an O3 calibrator (Thermo Fisher Scientific Inc, model 49C, Franklin, MA). We note that O3 may be overestimated due to interferences from VOCs emitted by the fire (Long et al., 2021), but the instrument used has been found to be in agreement with a federal reference method (Gao and Jaffe, 2017). NOx species were measured using a chemiluminescence NO–NO2–NOx analyzer (Thermo Fisher Scientific Inc, model 42i, Franklin, MA). The NOx analyzer was calibrated automatically every 6 h using NO and NO2 calibration standards purchased from Airgas (Radnor, PA) and has an LOD of 0.40 ppb.
The PM2.5 mass concentration was determined using a Tapered Element Oscillating Microbalance (TEOM) series 1400a ambient particulate monitor (Thermo Fisher Scientific, Franklin, MA) with data recorded at an averaging time of 60 s and a typical detection limit of 5.58 µg m−3 determined by 3 standard deviations of blank (filtered ambient air) measurements. These data were subsequently averaged to time intervals of 20 and 60 min to mitigate noise, especially when sampling under background conditions. The TEOM series 1400a developed originally by Rupprecht and Patashnick is a US EPA approved instrument for measuring mass concentrations of ambient PM2.5 and PM10 and could be used for Federal Equivalent Method (FEM) regulatory measurements (D. Liu et al., 2017; Patashnick and Rupprecht, 1991). It is a gravimetric measurement that determines the mass accumulated on a microbalance over a specified time interval at a monitored sample air flow rate. The sample air is preconditioned to a temperature of 50 °C to remove liquid water interferences (Patashnick and Rupprecht, 1991), which may lead to the evaporation of highly volatile PM2.5 components, potentially underestimating the total mass concentration. The mass concentration over an averaging period is calculated from the difference recorded between successive intervals. Due to random fluctuations in the instrument operation when concentrations are low, this can lead to negative numbers, illustrated by the frequency distribution of the high-time-resolution data recorded by one TEOM shown in Fig. S1 in the Supplement. When determining the average background concentration, we include the negative mass concentrations since converting negative concentrations to one-half of the LOD or ignoring them will produce an average that is biased high. In 2021, PM10 TEOMs were also deployed, but this was found to be highly influenced by pollen, which can be high in the springtime, and so the measurement was discontinued. Regional hourly PM2.5 mass was reported at two Environmental Protection Division (EPD) sites. In the following analysis we compare the PM2.5 measured within the fort to the EPD measurements at Columbus Airport and Phenix City South Girard (PCSG) school shown on the map in Fig. 1a. At Columbus Airport, the Teledyne T640, which is based on broadline spectroscopy, is used, while the Met One BAM-1022 mass monitor is used in Phenix City, utilizing a beta attenuation technique.
The PM2.5 BC mass concentration was measured using aethalometers. A range of multi- and single-wavelength instruments was deployed. Two were seven-wavelength instruments (Magee Scientific, model AE33 and model AE31, Berkeley, CA) with detection ranges of 0.1–100 µg m−3 and averaging times of 60 and 120 s, respectively. One was a two-wavelength aethalometer (Magee Scientific, model AE22, Berkeley, CA) with a 0.1 µg m−3 detection limit and a 60 s averaging time. Two were single-wavelength particle soot absorption photometers (PSAPs) (Radiance Research, Seattle, WA) with sensitivity > 0.1 µg m−3 and a 60 s averaging time. For the multi-wavelength aethalometers, BC was determined from the light absorption at 880 nm using the manufacturer's specified mass absorption cross section (MAC) of 7.77 m2 g−1, whereas for the single-wavelength PSAPs BC was determined from the optical absorption coefficient at 565 nm assuming a specific mass absorption cross section of 10 m2 g−1 following the manufacturer's specifications. Two spot samplings of the AE33 model were corrected for mass loading errors. This was not done in the other instruments, and so the data of the aethalometers (AE31 and AE22) were corrected for loading interference using the method of Virkkula et al. (2007). PSAP measurements were not corrected due to the unavailability of the scattering coefficients needed for correcting filter-based PSAP measurements (Bond et al., 1999; Virkkula et al., 2005), which may lead to 10 %–20 % underestimation of BC at sites where PSAPs were installed.
BrC was calculated from the seven-wavelength aethalometer measurements. It is largely produced from biomass burning (Hecobian et al., 2010; Laskin et al., 2015; Yan et al., 2018; Fleming et al., 2020), and in the following analysis it is used as a unique indicator of biomass burning smoke. While a small amount of BrC can be produced from mobile sources and other sources of incomplete combustion, in the USA its predominant source is biomass burning (Jo et al., 2016; Hecobian et al., 2010). We calculate the light absorption of BrC at 365 nm as a marker for BrC levels. Using the aethalometer data, the absorption coefficient, which corresponds to BC + BrC, was inferred by multiplying the mass concentration at each wavelength by the corresponding MAC value provided by the manufacturer (Magee Scientific, Berkeley, CA). The absorption coefficient at 365 nm was determined by extrapolating the linear regression of the log absorption coefficient and log wavelength since the lowest wavelength at which the aethalometer operates is 370 nm. The slope of the linear relationship represents the negative absorption Ångström exponent (AAE), a parameter used to study the optical properties of the aerosol. BrC at 365 nm was then calculated by removing the estimated contribution of BC at 365 nm, assuming that BrC does not absorb at 880 nm and that the AAE of pure BC is 1. The BrC absorption at shorter wavelengths is the difference of the aethalometer-measured total absorption and the extrapolated BC absorption (Lack and Langridge, 2013). All the data of the light absorption of BrC discussed in this work correspond to the absorption calculated at 365 nm. Both AAEtotal and AAEBrC were calculated as the negative slopes of the log absorption coefficient of the total (BC + BrC) and BrC, respectively, as a function of log wavelengths. For AAEtotal, the fit included the wavelengths from 370 to 880 nm (i.e., 370, 470, 520, 590, 660, and 880), whereas for AAEBrC the wavelengths ranged from 370 to 660 nm (i.e., 370, 470, 520, 590, and 660).
In our analysis, we used meteorological and fuel moisture data from the Remote Automated Weather Stations (RAWS) available online (https://raws.dri.edu/index.html, last access: 1 May 2024). The RAWS closest to all of the sites is Fort Benning, Georgia (Fig. 1a). At each trailer, all of the instruments were connected to a laptop computer with remote access to reduce the personnel time spent at the sites. The sites were generally visited every 1 to 2 weeks, during which regular instrument checks and maintenance were performed, such as restoring power, changing filters (for TEOMs and PSAPs), and measuring and recording flow rates and other instrument performance parameters.
2.4 Tools and analysis methods
2.4.1 Normalized excess mixing ratios
To account for dilution of species of interest in a smoke plume, normalized excess mixing ratios (NEMRs) are used. An NEMR is the ratio of enhancement of a studied species above the local background concentrations to the enhancement of a long-lived component co-emitted from a biomass burning event. CO is often used as the reference species. For example, the NEMR of species X is , where Y is CO measured in the same sample as X. To determine the NEMR of X and the contribution of smoke to X from an identified burning region, the background concentration of X (i.e., the concentration in the case of no smoke emissions) is subtracted from the measurement. In our study we used the average of the measurements before and after the smoke event as the background since sampling was not performed upwind of the fire. This method is supported by the observation from multiple sampling sites of spatially uniform background concentrations and, in most cases, very low background concentrations relative to those recorded in smoke. However, there is more uncertainty when calculating O3 NEMRs due to significant levels and diurnal changes in background concentrations. NEMRs can also be determined from the slopes of linear regressions. Here, we determine NEMRs for each smoke event for PM2.5 mass, BC, and BrC normalized by CO by first removing background concentrations for data recorded during the event and then calculating the slope by linear regressions (i.e., the slope of PM2.5 mass concentration, BC concentration, or BrC absorption at 365 nm vs. CO concentrations to determine the respective NEMRs).
2.4.2 Determining smoke sources and plume age
To match specific fires to observed smoke at monitoring sites, several methods were used. Data from the Fire Information for Resource Management System (FIRMS) provided active fire data based on thermal anomalies. These are based on measurements from the Moderate Resolution Imaging Spectroradiometer (MODIS), carried by the Aqua and Terra satellites, and the Visible Infrared Imaging Radiometer Suite (VIIRS), carried by the Suomi National Polar-orbiting Partnership (Suomi NPP) and NOAA-20 satellites. FIRMS provides live and historical fire maps and data that can be accessed online (https://firms.modaps.eosdis.nasa.gov/, last access: 1 May 2024). This platform can be used to pinpoint specific locations and obtain distances between points, which is useful for identifying possible fires where smoke was transported to the sampling site and the time for smoke transport when combined with wind speed and direction data. Although the FIRMS fire map is updated every 5 min, the polar-orbiting satellites only pass over the location twice per day, meaning that some fires starting and ending between satellite observations are not detected (Schroeder and Giglio, 2018; Giglio et al., 2021). Also, small or relatively cool fires may not be detected, especially when there is significant cloud coverage, thick smoke, or a continuous thick forest canopy, which can block satellite detection of prescribed understory burns in forests. Cloud coverage data are available online (https://worldview.earthdata.nasa.gov, last access: 5 May 2024), and satellite data, including MODIS/VIIRS overpass times, the number of active fire detections per pass, and the FRP for all fires that impacted the monitoring sites, can be downloaded from the abovementioned FIRMS website. Burn data provided by Fort Moore were used with the FIRMS data to minimize the limitations of each method in identifying sources of observed smoke. For each of the 64 smoke events studied in the paper, burn data are added to the Supplement (Table S1). Additionally, the temperature, relative humidity, and fuel moisture data used can be accessed online through the RAWS USA Climate Archive (https://raws.dri.edu/index.html, last access: 1 May 2024) at the weather station that is closest to all the measurement sites (Fort Benning, Georgia).
The Hybrid Single-Particle Lagrangian Integrated Trajectory (HYSPLIT) model (Stein et al., 2015) was used to calculate back-trajectories from monitoring sites. This trajectory analysis was based on meteorological data derived from the WRF model (Shamarock et al., 2019) and enhanced with grid nudging and observational nudging (Deng et al., 2009; Liu et al., 2005) using a 20 min time step. The WRF domain settings are shown in Fig. S2 in the Supplement. The winds used in the trajectory analysis are from the 1 km grid resolution domain. Each analysis covered a total of 10 trajectories, all below the planetary boundary layer (PBL). HYSPLIT was run with 10 min time steps, and the locations of the fires were determined based on FIRMS data and the Fort Moore fire management records.
3.1 Assessment of PM2.5 monitors and background concentrations
The focus of this analysis is on PM2.5 mass concentrations from the prescribed fires. Aerosol particle mass concentration measurements are difficult, especially under background conditions, when concentrations are low. Calibrating instruments with known mass standards is also problematic. We performed intercomparisons between monitors, including direct comparisons for two pairs (side by side) and intercomparison of background PM2.5 mass concentrations measured by the study TEOMs to the values reported at state monitoring sites. For example, two TEOMs (used in the main and T1293 trailers) collocated at Eglin Air Force Base from 19 March 2023 at 08:00 local time till 20 March 2023 at 10:00 had an orthogonal regression slope of 0.98 ± 0.09, an intercept of 0.45 ± 0.37 µg m−3, and an r2 of 0.84 (see Fig. S3 in the Supplement). The main trailer TEOM was also compared with the TEOM used on T1291 when they were collocated at the Georgia Institute of Technology from 22 September 2023 at 19:00 till 7 October 2023 at 14:00. Although measurements during that period were close to the background levels, the comparison resulted in an orthogonal regression slope of 0.88 ± 0.03, an intercept of 3.75 ± 0.09 µg m−3, and an r2 of 0.76 (see Fig. S4 in the Supplement). The frequency distributions used to determine the mean values and the mean background values of the data recorded at the main trailer and the EPD sites in 2022 are shown in Fig. S5 in the Supplement. The mean concentrations in 2022 were 7.02, 9.47, 9.01, 9.26, and 7.11 µg m−3 at the main trailer, T1293, T1292, T1921, and T1290 and 10.33 and 10.67 µg m−3 at the Columbus Airport and PCSG school EPD sites, respectively. Background air PM2.5 mass concentrations were also determined by excluding smoke events (discussed below). The monthly backgrounds of PM2.5 mass concentrations are shown in Table S2 in the Supplement. Background concentrations were in the range of approximately 3–7 µg m−3 for monitors at the fort and between 7 and 9 µg m−3 at the state monitoring sites (Table S3 in the Supplement). The higher background PM2.5 mass concentrations at the state sites are likely due to local anthropogenic (urban) influence. These comparisons provide confidence in the mass measurements that cannot be calibrated in a manner similar to gas monitors.
Background concentrations of CO and BC are also given in Table S2. The background CO ranged from ∼ 150 to 200 ppb, and the background BC ranged from 0.14 to 0.57 µg m−3. In terms of the spatial variation at Fort Moore, the background levels of the measured species were slightly lower at sites located far from the main roads and training areas, such as measurements at the main trailer during May 2021 and the entire 2022 season. No significant temporal variation is observed, although fires within the base and in its vicinity increase during the transition from winter to spring, indicating that smoke was efficiently dispersed on timescales of approximately 1 d. Frequent smoke events where concentrations of the various measured species were substantially above these background levels were observed during the 2021 and 2022 field deployments.
3.2 Study of fires at Fort Moore during 2021 and 2022
We first present an overview of the measurements at Fort Moore during two burning seasons. In the 2021 season, only one research (main) trailer was deployed. In the following year, four more were deployed for a total of five sites.
On 18 March 2021, a fully equipped trailer was deployed at the northern boundary of Fort Moore, and we sampled at that location until 26 April 2021. It was then moved to the center of the fort for sampling from 26 April to 15 May 2021 (see Fig. 1a). During this period, peaks of measured species were observed, as shown in the time series of PM2.5 mass in Fig. 2b. A peak of a measured species is defined as the highest value observed within its data points, spanning from an initial rise until a return to background levels. The maximum PM2.5 mass concentrations reached 2000 µg m−3 for 20 min averaged data and 1400 µg m−3 for hourly averaged data (Table S4 in the Supplement). A total of 11 PM2.5 peaks with mass concentrations greater than 35 µg m−3 were recorded. In 2022, over the course of the entire burning season, 32 d recorded a total of 53 PM2.5 mass concentration peaks greater than 35 µg m−3 across the five measuring sites, as shown in Fig. 2c with similar high concentrations, reaching 841 µg m−3 for 20 min averaged data and 513 µg m−3 for hourly averaged data (Tables S5 to S8 in the Supplement).
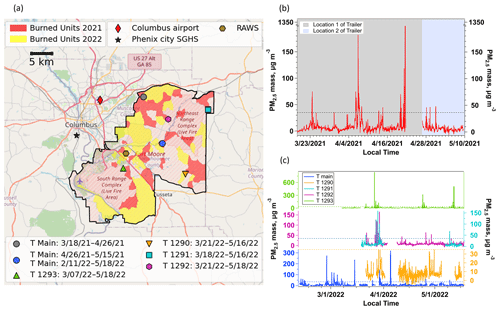
Figure 2PM2.5 mass measurements over 2 burning years. (a) Map of the burned areas in the years 2021 and 2022 and locations of the monitoring sites. (b) Time series of 20 min average PM2.5 mass concentrations measured at the main trailer during the burning seasons of 2021 and (c) 2022 across the different sites. The dotted lines represent a PM2.5 mass concentration of 35 µg m−3 above which peaks were selected for detailed analysis.
We focus on smoke plumes with higher PM2.5 mass concentrations to identify their sources and estimate the emissions and evolution of the PM2.5 mass, because the burning areas are readily identified (e.g., detected remotely by satellites) and the plume can be easily delineated from the background. An increase in the measured species is considered a peak, or event, when the 20 min average PM2.5 mass is greater than 35 µg m−3 and the 40 min average PM2.5 mass concentration (average of two consecutive measurements) is higher than 30 µg m−3. This excludes shorter transient events, which include a passing vehicle, that can occur at measuring sites near training areas.
The high peaks in PM2.5 mass are always accompanied by an increase in CO, BC, and BrC. Figure 3 shows the scatterplots of the 20 min averaged data collected in 2021 and 2022. The linear relation between PM2.5, CO, BC, and BrC during the events resulted in r2 values of 0.85, 0.68, and 0.71, respectively. On the other hand, for the non-event data, which include all the observations during the entirety of the measurement period, r2 drops to 0.12, 0.33, and 0.17 for PM2.5 mass vs. CO, BC, and BrC, respectively. These correlations suggest that the identified events correspond to periods of measuring smoke from biomass burning sources. However, it is important to note that variability still exists in slopes among different events, which will be explored and discussed in the later sections.
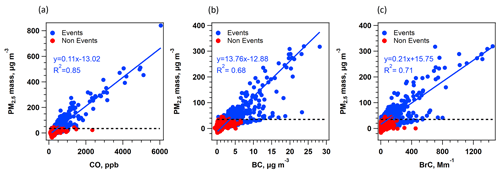
Figure 3Correlations between the PM2.5 mass concentration and CO, PM2.5 BC, and PM2.5 BrC for measurements from the main trailer in 2021 and 2022 and T1291 and T1293 in 2022. The blue data points are characterized as PM2.5 events when the concentration is more than 35 µg m−3 averaged over a 20 min period. In the plot, all data associated with an identified event are shown in blue (this includes event data down to the background levels before and after the peak). All other data (non-events) are shown in red. The slope is from the orthogonal distance regression (ODR) of the 20 min averaged data during the event periods.
3.3 Determining smoke sources
To study the emission and evolution of smoke plumes and make our measurements useful for evaluating smoke transport and dispersion models, we aim to link identified smoke plumes to specific burn areas and determine their transport time. Attribution of the smoke to specific fires is also useful for assessing the impacts of a specific prescribed burning program, such as the one at Fort Moore. Identifying the locations of prescribed fires was complicated by several factors. In this study, we had limited prior information on the timing and location of planned burns from the burn managers. Moreover, smoke from other sources, such as prescribed and wildfires in the region but not within the fort, as well as uncertainty and variability in wind patterns at the time of burning, led us to utilize multiple methods to determine the source of each identified smoke episode.
Our analysis started by using satellite data from FIRMS to identify the locations of the fires (when the satellites passed overhead). After the end of the study, these locations were verified by cross-referencing with the Fort Moore fire management reports, which provided the locations and acreage of the prescribed burns and ongoing wildfires exclusively within the fort for each day. Afterwards, we pinpointed the smoke source that reached the monitors by averaging the wind vectors at and before the peaks using the meteorological data from RAWS. This provided the expected general upwind region the smoke likely came from. We also used the HYSPLIT model to conduct back-trajectory analysis from the measurement trailer for 8 h prior to ascertain whether the air mass containing the measured smoke had passed the satellite-identified hotspot or the units reported as burned by the fort's fire management. HYSPLIT's initial altitudes were determined by the PBL height, where the trajectories for 10 equally distributed altitudes between 10 m above the surface and the top of the PBL were generated for each simulation. For example, if the PBL height was 100 m, the trajectories were calculated at 10, 20, 30, 40, 50, 60, 70, 80, 90, and 100 m.
Through the systematic combination of these methods, we attempted to identify specific fire sources associated with each observed smoke event and the time of transport of the smoke from the fire to the measurement site (referred to as the smoke age). This procedure was successful for 61 of the 64 identified smoke events. We failed to identify three events that had no apparent source, in agreement with the studied wind patterns. Moreover, of the 61 identified smoke events, 7 were matched to different sources using the observed wind vector method vs. using the HYSPLIT trajectories, 7 were matched to sources using HYSPLIT only, and 5 were matched to sources using the wind vector method only.
The variability of the smoke sources determined in some cases is attributed to the difference between the wind direction used by HYSPLIT and that recorded by RAWS used for the wind vector calculation. In HYSPLIT, wind data are derived from the three-dimensional wind fields predicted by the application of the WRF model. Figure 4 shows a comparison between modeled and observed wind directions during the events identified in 2021 and 2022 at the main trailer. A closer alignment in wind direction is observed under higher-speed wind conditions.
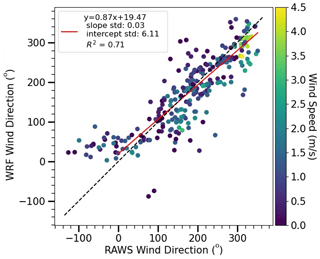
Figure 4Comparison between the wind direction modeled by WRF and that recorded by the RAWS located at Fort Moore. The slope is from the ODR.
As an example of source determination, Fig. 5a shows the time series of CO, PM2.5 mass, BC, and BrC during three smoke episodes recorded on 6, 7, and 8 April 2021, which are indicated by blue, yellow, and green shading, respectively. Along the top of the graph are the hourly averaged wind vectors based on data from RAWS. Note the high correlation between PM2.5 mass, CO concentration, and BrC absorption coefficient, indicating that the PM2.5 peaks were due to smoke. During the period of 3 d, the three events were measured during the late evening, nighttime, and early morning periods. In each case, there is a time delay between when the burning occurred and when the plume was measured, which is due to the transport time. In all three cases, burning regions at the fort were identified as the source. Consider the first smoke event detected at the trailer between 01:00 and 11:00 on 6 April 2021 (blue-shaded region in Fig. 5a). Figure 5b shows the map of the fort and FIRMS satellite data on the day before (5 April 2021), indicating two hotspots on the base that were later verified in the fire report as burning of two units and four sections of a third unit. Both burns were to the south and south-southeast of the trailer, and the winds were from the west during the daytime on 5 April 2021. By midnight, the wind direction had shifted, with air flowing from the south and southeast, transporting smoke to the trailer's location and leading to elevated concentrations of species on monitors. Wind speeds were very low at night. At about 08:00, the wind speed increased, its direction changed, and the concentrations of the species all dropped.
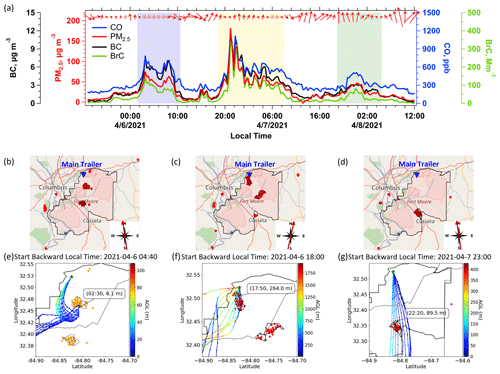
Figure 5Three case studies illustrating the application of our method in determining the sources of smoke events. (a) Time series of species measured at the main trailer. The time resolution is 20 min for CO, PM2.5 mass, BC, and BrC. The wind vectors depict hourly data obtained from RAWS, with the direction of the arrow indicating the wind direction and the length of the arrow representing the wind speed. (b, c, d) Maps of the fort showing historical satellite data from the FIRMS website observed for 5, 6, and 7 April 2022. The red dots represent fires detected by the satellite. (e, f, g) HYSPLIT back-trajectories during the occurrence of each of the three peaks. The date and time of the back-trajectory are indicated on top of each map. The time and height at which the trajectory crosses the trailer are shown in the box inside each map. The red dots are fires detected by FIRMS on the same day as the back-trajectory. The orange dots are fires detected by FIRMS 1 d before the back-trajectory. The colors of the traces in the back-trajectories indicate the height above ground level. The green star marks the location of the main trailer. The satellite overpass times are shown in Table S9 in the Supplement.
Burning of the other units took place on 6 April 2021 at distances of 1.3, 3.4, 4.0, 10, and 12 km from the trailer. The level of the measured smoke products started increasing in the evening after the winds became southwesterly and stayed high until the morning of the next day (7 April 2021) (yellow-shaded region in Fig. 5a). Later on the night of 7 April (green-shaded region in Fig. 5a), the concentration levels increased slightly after the burning of two connected units to the south of the base during the daytime of 7 April 2021 at a distance ranging from 17 to 20 km, as indicated by the fort's fire management and seen on FIRMS. HYSPLIT back-trajectory analysis, shown in Fig. 5e, f, and g, was conducted to assess our conclusion about the sources, especially in the cases of wind variation and/or multiple fires such as for the peaks monitored on 6 April (blue-shaded region in Fig. 5a) and 7 April 2021 (yellow-shaded region in Fig. 5a). Since there are multiple fires on the fort, all in the same southerly direction relative to the trailer, the exact source cannot be determined solely based on wind vectors from RAWS data. In these cases, HYSPLIT back-trajectories help to pinpoint the exact fire or fires contributing to the observed smoke event. In both cases on 6 April (blue-shaded region in Fig. 5a) and 7 April 2021 (yellow-shaded region in Fig. 5a), the closer fire was the smoke source as shown in Fig. 5e and f.
3.4 Determining smoke age
An estimate of the smoke age is needed to separate fresh and aged smoke to estimate emissions of various species (i.e., in fresh smoke) and the changes in their concentrations with plume age. The physical age of smoke is the time it takes the smoke to be transported from the source to the monitoring sites. Following the concept presented for source identification, the transport time of smoke is estimated by averaging wind speed over the period it takes for the smoke to travel from the fire to the measurement sites and is determined by iteration (mean wind speed recalculated with a new transport time until convergence). When the average wind speed in the hour leading up to the peak does not result in a smoke age of 1 h or less, we begin iterative steps by calculating the average wind vector for additional increments 1 h at a time. A detailed example of using an average wind vector in estimating the physical age of smoke is provided in Supplement Sect. S1. It is important to note the uncertainty in the estimated smoke age using this method for smoke monitored before and after the peak (maximum concentration), particularly when the smoke event duration (from the start to the end of smoke monitoring) is prolonged and when wind conditions are highly variable. The age was also determined from the HYSPLIT back-trajectories as the time when the lowest trajectory intersects the smoke source that was identified. The back-trajectory is initiated from the start time of the smoke event. Due to uncertainties in the WRF-simulated winds, particularly at night when wind speeds are low, the back-trajectory occasionally missed the source. Therefore, a series of HYSPLIT simulations was conducted with 20 min intervals from the event start time until the smoke source could be identified. The 20 min interval was chosen based on the temporal resolution of the WRF data.
For the three events discussed in Fig. 5, the physical ages estimated using the wind vector averaged from the observed RAWS wind data are 75 min for 6 April (blue shading), 14 min for 7 April (yellow shading), and 162 min for 8 April (green shading). For the same events, and using HYSPLIT trajectories closest to the surface and passing through the identified sources, the ages were estimated as 130 min for 6 April (blue shading), 10 min for 7 April (yellow shading), and 40 min for 8 April (green shading). Based on our analysis, 6 April (blue shading) stands out as the only case where the HYSPLIT age exceeds that estimated using the mean wind vector for the same fire source. The difference between the modeled and observed wind for these three instances was investigated further by comparison with the observed wind at Columbus Airport. As shown in Fig. S6 in the Supplement, the wind direction observed at the airport aligns more closely with that observed at the RAWS site at Fort Moore (though with faster winds at the airport, likely due to the forest canopy effect on wind flow) than with the WRF-modeled winds at both sites. However, it is difficult to determine which method is more reliable for studying any specific smoke event. For all the smoke plumes identified, the age of smoke estimated based on HYSPLIT back-trajectories ranged from 10 min (single time step of the trajectory) to 6 h (36 time steps) and from a few minutes to 8 h based on the average wind vector method (Table S10 in the Supplement). A comparison summary between wind speeds observed by the RAWS and those modeled by WRF during all the events identified in 2021 and 2022 at the main trailer is shown in Fig. 6a. The observed weak correlation (r2=0.29) could be due to several factors. For the wind vector analysis, observed winds are measured at one location and 2 m above ground level with a single monitor, which may not accurately represent the wind patterns along the entire smoke transport path, especially in forested areas where the canopy can affect the wind flow (Mallia et al., 2020). On the other hand, WRF simulates winds for 34 layers at different altitudes from 10 m (the lowest) to levels higher than the PBL. HYSPLIT applies bilinear interpolation to the data from WRF for the 10 trajectories that it calculates, introducing additional uncertainty into the wind patterns used in the simulations. Although the comparison between ages estimated based on the two different methods resulted in a reasonable correlation (r2=0.59), the slope clearly indicates a significantly higher estimation of age when using the wind vector method, particularly for more aged smoke events as shown in Fig. 6b, where ages from the two methods show stronger agreement for fresh smoke. This can be attributed, in many cases, to the uncertainty in observed winds under low-speed wind conditions, the measurement being far from where winds are observed (RAWS), and most importantly to the fact that the RAWS measures winds at 2 m above ground level, whereas smoke transport happens at higher altitudes with stronger winds. There are additional discrepancies resulting from wind variation at each altitude at which HYSPLIT is running.
3.5 Limitations of the fixed-site method
The goal of this project is to study the emissions and evolution of smoke from prescribed fires and provide data to test model simulations and assessments of prescribed burning impacts. Some limitations and challenges are associated with our approach of collecting data from a network of fixed sites.
3.5.1 Identification of burning regions
First, due to the limitations of satellite fire detection, some fires were not seen in FIRMS satellite detection data but were subsequently identified from the fire management report, such as the prescribed fires on 23 March 2021 shown in Fig. S7a in the Supplement. The 20 min averaged PM2.5 mass concentration at the trailer increased to 74.8 µg m−3 and to a 47.8 µg m−3 hourly average at the EPD site located off-base at Columbus Airport on the afternoon of 23 March 2021, as shown in the time series of Fig. S7b in the Supplement. This increase was accompanied by an elevation in the levels of CO, PM2.5 mass, BC, and BrC measured at the trailer. This is an example of burning on the fort likely affecting the nearby urban population. The prevailing winds were from the southeast at the time of the smoke event, as can be seen from the wind vectors presented in the same time series in Fig. S7b. However, FIRMS satellite data showed no hotspots on the fort during the entire day. After checking the fire management report for 2021, prescribed burns were identified for three units located in the eastern central part of the fort at distances ranging from 13 to 24 km from the trailer. Looking at either the wind vector at the time of the peak or the HYSPLIT back-trajectories, the source of the smoke event identified on 23 March 2021 matches the closer prescribed burn conducted on the fort.
Another issue with this approach is that relying only on data from the burning authorities at Fort Moore can, in some cases, be insufficient due to the lack of information about fires taking place off-base by landowners, such as the off-base fire seen on FIRMS during three overpasses of satellites at 12:38, 13:54, and 14:42 (Fig. S8a). On 9 May 2022 at 16:30, the monitored species increased at the main trailer and the 20 min average of the PM2.5 mass reached 52.3 µg m−3 (Fig. S8b). The fort's fire management reported no prescribed fires and one wildfire in the southern part of the base, with an indication of zero probability of smoke from that fire reaching the trailer based on wind patterns. Based on both wind vectors and HYSPLIT simulations, the source of the event was identified as an off-base fire detected to the northeast of Fort Moore. The same smoke event was also observed at multiple trailers operating at the time and will be discussed more in the following section.
3.5.2 Identifying a specific fire impacting the site when multiple burns are occurring
When multiple fires take place simultaneously under varying wind conditions, it can be difficult to identify the specific fire impacting the site, which can lead to uncertainty in the smoke age. This occurred in smoke detected around midnight on 14 March 2022 (see Fig. S9a in the Supplement). Relying on wind data, the smoke source is likely one or more of the fires on the eastern and/or southeastern sides of the base, with a zero probability of it being one of the fires in the northern part of the base. HYSPLIT may help in narrowing down the possibilities of the smoke source (Fig. S9b), but there is still uncertainty in linking a specific fire to an observed event.
When several burning units are in close proximity and near the measurement site, identifying the specific source and smoke age can also be difficult (for example, see Fig. S10 in the Supplement). In this case burning in three units indicated by the fort's fire management occurred at the same time close to each other and the trailer (distances of 0.97, 2.3, and 3.5 km from the trailer). The HYSPLIT trajectory at the lowest altitude passed near (to the east) but not over the prescribed fires. Wind direction at the time of the event suggests the influence of a minor portion from the northern part of the fire. It is important to note that, in such cases, transport near the surface may be heavily influenced by fire–atmosphere interactions, making it difficult to rely on data from RAWS or WRF simulations as accurate indicators of atmospheric flows close to an active fire.
We note that there is no direct correlation between the amount of smoke reaching the trailer, i.e., measured species concentrations, and the distance of the fire from the monitoring site. The relation depends on the smoke transport and dispersion that may allow smoke to either directly hit the measuring site, partially reach the measuring site, or pass above the trailer with little or no smoke detection by the monitors. To illustrate this, we compare three case studies. Looking again at the smoke event of 11 February 2022 shown in Fig. S10, smoke reaching the trailer from 0.97 to 3.5 km fires resulted in a 20 min maximum PM2.5 mass of 62.8 µg m−3 and a CO concentration of 1.3 ppm at 13:30. On 12 February 2022 (Fig. S11a and b in the Supplement), smoke from burns of units at distances 6.9 to 7.4 km from the trailer caused increases in the 20 min PM2.5 mass concentration to 60 µg m−3 and CO to 0.9 ppm at 13:50, whereas, on the night of 4 April 2022 until the morning of 5 April 2022 (Fig. S11c and d in the Supplement), smoke from fires 6.1 and 6.3 km from the trailer caused increases in the 20 min PM2.5 mass concentration to 319 µg m−3 and CO to 3.0 ppm at 01:10 over a longer smoke-monitoring period. The much higher PM2.5 mass concentrations measured on 4 April 2022 suggest that the trailer received a more direct smoke hit on that day than on 11 or 12 February 2022, despite the fire being closer on 11 February and having a very similar distance to the one detected on 12 February. This can also be attributed to the much lower nighttime PBL on 4 April, which was 9.8 m and caused all HYSPLIT trajectories to overlap as shown in Fig. 11d. Emissions from a smoldering fire with very little buoyant energy were most likely trapped in this shallow layer, leading to high concentration measurements. During the daytime on 11 and 12 February, higher PBLs of 1645 and 1305 m, respectively, favored more vertical dispersion of smoke.
3.5.3 Smoke not detected although regions of burning identified
On certain days, based on the wind data and the information presented in the fire management report, it appears likely that smoke from the fires at the base would reach specific monitoring sites. However, in these instances, such as the situation on 15 February 2022 shown in Fig. 7, no significant smoke peaks were detected. To explain this outcome, two HYSPLIT forward-trajectory simulations were run. The simulations showed that, if the fire starts at 10:00, the smoke will not intercept the monitor, but if it starts at 11:00, the smoke at higher altitudes has a slight chance of reaching the monitor. Overall, regardless of the wind direction favoring smoke transport to the monitors, other factors like dispersion and smoke plume behavior such as lofting play a significant role in the transport process.
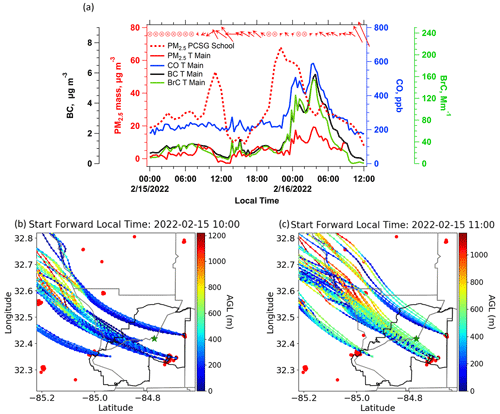
Figure 7Case study of missing smoke at the monitoring site despite expectations according to wind direction. (a) Time series of species measured at the main trailer. The time resolution is 20 min for CO, PM2.5 mass, BC, and BrC. The wind vectors depict hourly data sourced from the RAWS, with the direction of the arrow indicating wind direction and the length of the arrow indicating wind speed. The data from the PCSG school are hourly averages. (b, c) HYSPLIT forward trajectories starting from the two prescribed fires on the base on 15 February 2022 at 10:00 and 11:00, respectively. The red dots are fires detected by FIRMS on the same day (satellite overpasses occurred on 15 February 2022 at 12:54, 13:49, 14:32, and 14:36).
3.6 Using multiple monitoring sites to increase chances of measuring smoke and studying smoke evolution
There are distinct advantages to setting up multiple measuring sites and studying smoke over an extended period. First, this helps to capture more smoke events, as seen during the 2022 study in comparison with that in 2021, when a single trailer was used. It minimizes issues with predicting downwind locations and is not affected by uncertainty in planned burning locations and times. Second, it reduces the labor and time required to relocate a single trailer and set it up several times throughout a prescribed burning period when burning occurs over different regions. Third, it provides a high spatial resolution, and occasionally smoke from the same fire is detected at several sites, which can be useful for studying smoke chemical evolution with higher certainty than studies of multiple plumes of varying ages measured on different days.
An example of the same fire detected at several sites is shown in Fig. 8. On 9 May 2022, all of T1291, T1292, Main Trailer, and T1293 detected an off-base fire taking place approximately 18 km north-northeast of the base, as shown in Fig. 8a. T1291, the trailer closest to the fire, measured PM2.5 mass and CO peaks at 15:10. The time series of species measured in the various trailers is shown in Fig. 8. Subsequent peaks in PM2.5 mass, CO, BC, and O3 concentrations were recorded at T1292 at 15:50, then at Main Trailer at 16:30, and finally at T1293, the trailer furthest from the fire, at 18:10 local time. For O3, note the O3 enhancement (ΔO3) superimposed onto the diurnal O3 trend. The ages of the smoke detected based on wind vector analysis were 266, 296, 330, and 480 min for the various trailers. The difference in smoke age is close to the difference in peak arrival times, with maximum PM2.5 mass concentrations observed at 15:02, 15:53, 16:25, and 18:16 at T1291, T1292, T1293, and T Main, respectively. The differences in peak concentrations can be due to a number of factors, including changes in fire emissions with time, the extent of plume dilutions with distance from the fire, and changes in which portions of the plume were measured due to changes in winds. Wind vectors are shown at the top of the plots in Fig. 8. Wind direction and speed varied during the period when the plumes were recorded; the wind direction was between 52 and 86° from 11:00 till 14:00, and the speeds were between 1.3 and 3.1 m s−1 on 9 May 2022. A shift in wind direction to 348° at a speed of 1.8 m s−1 happened at 15:00. Then, the wind direction fluctuated between 11 and 44° before the wind speed decreased to 0 m s−1 at 20:00 and remained calm until the morning of 10 May 2022. Normalizing these plume data by a stable smoke tracer, such as CO, can account for some of these factors when comparing the emissions and evolution of various plume properties.
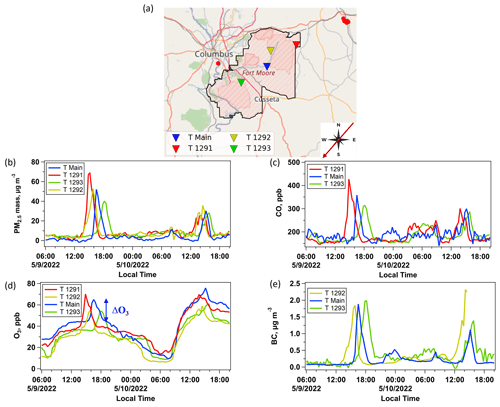
Figure 8Case study of sequential smoke detection at four monitoring trailers. (a) Map of the fort showing historical satellite data from the FIRMS website observed for 9 May 2022 (satellite overpasses occurred on 9 May 2022 at 12:38, 13:54, and 14:42 local time) and the average wind vector from 13:00 to 16:00 local time. Time series showing 20 min data on the (b) PM2.5 mass concentration, (c) CO concentration, (d) O3 concentration, and (e) BC concentration for the main trailer, T1291, T1292, and T1293. Note that no CO instrument operated at T1292 and that there were no BC data for T1291.
3.7 Interpretation of measurements to characterize smoke emission and evolution
3.7.1 PM2.5 emissions
We used the NEMRs to study the emissions of PM2.5 species and their evolution in the various measured smoke plumes. The NEMRs determined from the linear regression slopes of PM2.5 species (mass concentration, BC concentration, BrC absorption, and CO, with their backgrounds subtracted) and the correlation values (r2) for all of the smoke events are summarized in Table 1. PM2.5 mass-concentration NEMRs from other studies are summarized in Table S11 in the Supplement.
Table 1The PM2.5 mass, BC, and BrC NEMRs relative to CO (based on regression slopes) and the coefficients of determination (r2) in the column to the right of each NEMR for the smoke events identified in this study*.
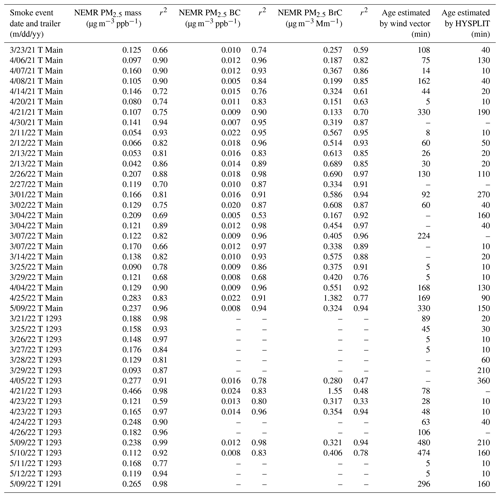
* The table lists all events where both PM2.5 mass and CO concentration were both available. In some cases BC and BrC data was not available and left as blank values (–).
The NEMR of fresh smoke near a fire is interpreted as an emission ratio (ER), assuming that the smoke has undergone limited chemical and/or physical changes. ERs based on NEMRs are used widely (Liu et al., 2017b; Collier et al., 2016; Burling et al., 2011; Gkatzelis et al., 2024). They are compiled in reviews and emission inventories for ambient (Andreae, 2019; Prichard et al., 2020) and laboratory fire studies (Yokelson et al., 2013) and for evaluating or making model predictions (Xiu et al., 2022; Jaffe et al., 2022).
By focusing on fresh smoke (age less than 1 h), the emission ratios (ERs) of the prescribed fires can be estimated and compared to those from other studies. The PM2.5 mass concentration ER ranged between 0.04 and 0.18 µg m−3 ppb−1 and is shown in Fig. 9. These ERs are comparable to other prescribed fires measured at both ground level (Alves et al., 2010; Desservettaz et al., 2017; Korontzi et al., 2003; Balachandran et al., 2013) and aloft in airborne studies (Sinha et al., 2003; May et al., 2014; Gkatzelis et al., 2024; Travis et al., 2023) that span a large range of burning conditions and fuels (details are provided in Table S11). The mean PM2.5 mass concentration ER for our data is 0.117 ± 0.045 µg m−3 ppb−1, and those of these other prescribed fire studies are 0.098 ± 0.034 µg m−3 ppb−1 for ground-based measurements and 0.188 ± 0.154 µg m−3 ppb−1 for airborne measurements. There is substantial and similar variability in the ground-based measurements of prescribed fire ERs in this study relative to other studies. More recent airborne-measured prescribed fires have reported substantially higher ERs (Fig. 9). Smoke transported for 10 min from the Blackwater river state forest prescribed fire reported by Gkatzelis et al. (2024) had an ER of 0.462 µg m−3 ppb−1 (Gkatzelis et al., 2024), and Travis et al. (2023) reported a range of 0.188–0.433 µg m−3 ppb−1 for 22 prescribed fires studied and grouped into four categories based on fuel type (Travis et al., 2023). Figure 9 also shows comparisons with wildfires reported in other studies (Liu et al., 2017b; Collier et al., 2016; Palm et al., 2020; Gkatzelis et al., 2024). Wildfire PM2.5 mass ERs are significantly higher than the ERs for prescribed fires in this work, with ER ranges between 0.04 and 0.43 µg m−3 ppb−1 and a mean of 0.264 ± 0.091 µg m−3 ppb−1 for wildfires, and the difference is statistically significant (the two-tailed p value is < 0.0001). Lower PM2.5 mass ERs from smaller prescribed fires have been noted in other studies (Liu et al., 2017b) and support the utilization of prescribed burning as a land management tool to limit wildfires. However, differences in altitude at which the measurements were made may have some effect on the ERs. Selimovic et al. (2019) noted that the PM2.5 CO in ground-level smoke was about half of that observed from aloft, which was apparently due to a reduction in aerosol mass from evaporation of semivolatile aerosol particle components resulting from higher surface temperatures compared to aloft. Pagonis et al. (2023) also found airborne OA NEMRs to be a factor of 2 higher than ground-based NEMRs, giving the same interpretation (Pagonis et al., 2023). When comparing ERs of prescribed fires in ground and airborne studies of prescribed fires, shown in Fig. 9, the mean of airborne studies is a factor of ∼ 1.9 higher than ground-based studies, and the difference is statistically significant (the p value is 0.025).
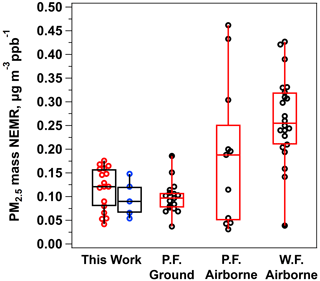
Figure 9Boxplot of PM2.5 mass NEMRs of smoke events of estimated age ≤ 1 h in this study in comparison to other studies. The blue symbols are smoke plumes with observed O3 enhancements. The horizontal line inside the box represents the median of the data. The top line of the box represents the third quartile (Q3), and the bottom line represents the first quartile (Q1). The colored circles represent data outliers. “P.F.” is prescribed fires, and “W.F.” is wildfires. Some of the emission ratios reported in the literature and included in the plot correspond to ΔOA/ΔCO since OA tends to dominate ΔPM2.5 mass concentrations (see Table S10).
This analysis assumes no significant changes in PM2.5 mass for smoke less than 1 h old. We have seen that smoke detected in the afternoon can have enhanced O3 concentrations, which may also lead to secondary aerosol formation. Smoke plumes with enhanced O3 are identified in the ERs shown in Fig. 9 and indicate no bias within the range of ERs recorded, suggesting that possible secondary aerosol formation within the first hour following emissions does not contribute to ER variability. We did not find evidence either of ERs depending on the time of day. No difference was seen between ERs for fires that started on the same day of measurement (i.e., all detected after 09:00 and before 17:00) and those detected at night after 17:00 or early in the morning, corresponding to fires that started on the day before the measurement but were still estimated to correspond to smoke less than 1 h old.
We also determined the ERs for BC and BrC. The BC ERs were in the range 0.008–0.022 µg m−3 ppb−1 with a mean value of 0.014 ± 0.004 µg m−3 ppb−1 and are within the range of NEMRs reported in other studies; 0.006 µg m−3 ppb−1 for prescribed burns in southern African savanna forests (Sinha et al., 2003), 0.020 µg m−3 ppb−1 for rBC (refractory BC) for prescribed burns of California chaparral forests (Akagi et al., 2012), 0.022 µg m−3 ppb−1 for chaparral forests (May et al., 2014), 0.006 µg m−3 ppb−1 for fires in montane ecosystems (May et al., 2014), 0.018 for coastal plain ecosystems in South Carolina (May et al., 2014), and 0.004 µg m−3 ppb−1 for large wildfires over the western USA measured during the Fire Influence on Regional to Global Environments Experiment (FIREX) campaign (Gkatzelis et al., 2024).
The BrC ERs of fresh smoke events ranged from 0.151 to 0.689 Mm−1 ppb−1, with a mean ± standard deviation of 0.442 ± 0.157 Mm−1 ppb−1. There are limited published data on BrC ERs and NEMRs from prescribed fires, and the measurement techniques of BrC vary between the studies. Liu et al. (2016) reported aircraft measurements of BrC at 365 nm inferred from PSAP absorption coefficients measured at two wavelengths (470 and 532 nm), with an ER of 0.223 ± 0.053 Mm−1 ppb−1 for fresh agricultural fires in the southeastern USA, which is lower than our mean but falls within the range of values we observed. For large wildfires measured over the western USA, Zeng et al. (2022) found for photoacoustic spectroscopy (PAS) measurements of BrC at a wavelength of 405 nm, the ER was 0.131 ± 0.001 Mm−1 ppbv−1 in plumes < 2 h old. These values are in the range we recorded, but the BrC ERs for the prescribed fires of this study are more variable.
3.7.2 NEMRs of all smoke events and their change with smoke age
Here we assess the overall variability in NEMRs for PM2.5 mass concentrations, BC mass concentrations, BrC absorption coefficients, and AAEs from all the smoke events (including ages less than 1 h) and assess possible trends with smoke plume age. In this analysis, the observed changes with age are a combination of variability in the emissions and evolution of aerosol since this is not a Lagrangian experiment, meaning that we are not continuously tracking a specific air mass containing smoke particles over time. PM2.5 mass-concentration NEMRs varied between 0.04 and 0.47 µg m−3 ppb−1 for all the reported events, with a mean ± standard deviation of 0.155 ± 0.076 µg m−3 ppb−1 (median 0.138 µg m−3 ppb−1). BC NEMRs ranged from 0.005 to 0.024 µg m−3 ppb−1 with a mean value of 0.013 ± 0.005 µg m−3 ppb−1. BrC NEMRs (ΔBrC ΔCO) ranged from 0.133 to 1.550 Mm−1 ppb−1. (Note that the data collected on 21 April 2022 at trailer 1293 are an outlier with exceptionally high ERs for the PM2.5 mass concentration and BrC absorption coefficient. The ER for BC mass concentration, while elevated, falls within the observed range. This event corresponds to smoke from an identified prescribed fire at the fort and has a relatively low ΔCO of 66.1 ppb, which is unexpected given the burn's proximity and the wind speed on that day, causing ERs to be significantly higher. The HYSPLIT back-trajectory from the measuring site does not intersect with the fire but passes close to it. Although the FRP reported by FIRMS does not differ from that of other fires and there is no significant difference in vegetation type or fuel moisture, the most likely explanation for this event is that the smoke passing through the measurement site was not a direct hit but was from the diluted boundary of the plume, which may have undergone photochemical processing, leading to higher PM2.5, BrC, and O3 NEMRs.) The NEMRs are given in Table 1 for all smoke event data and are plotted in Fig. 10 as functions of the estimated smoke age determined from the wind vector and HYSPLIT analysis. From these plots we assess whether there is any systematic evolution of the PM2.5 mass, BC, and BrC.
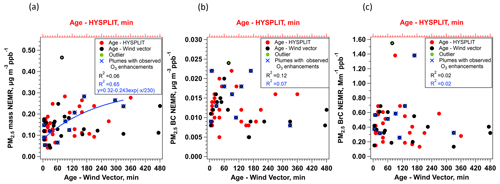
Figure 10(a) PM2.5 mass, (b) BC, and (c) BrC NEMRs of all the studied smoke events as a function of age estimated using average wind vector and HYSPLIT analysis. Smoke plumes with observed O3 enhancements are identified. Linear regression coefficients of determination (r2) for all data and for just O3 enhancement periods are identified. The exponential fit equation for PM2.5 mass NEMRs for O3 enhancement periods is shown in panel (a).
Changes in PM2.5 mass-concentration NEMRs with smoke age: Fig. 10a shows a substantial variability of PM2.5 mass NEMRs at all ages, with no significant statistical difference or clear trend. However, NEMRs tend to be lower for fresh smoke events (≤ 1 h old) than more aged plumes, which is possibly due to secondary aerosol formation. Considering only smoke plumes in which O3 enhancements were observed (i.e., smoke measured between 12:00 and 18:00), PM2.5 mass-concentration NEMRs consistently increase with physical age (r2=0.65), which is possible evidence of secondary aerosol formation driven by photochemistry.
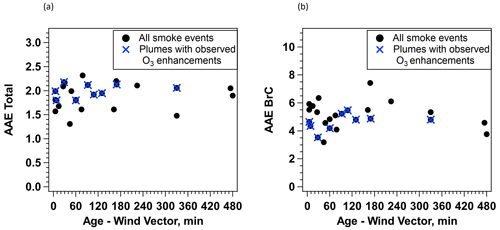
Figure 11Average AAE values for (a) total light absorption (BC + BrC) and (b) BrC species for all smoke events for which aethalometer data are available. Smoke plumes with observed O3 enhancements are identified.
A range of results for changes in PM2.5 mass-concentration NEMRs in wildland fires has been observed in other studies, including systematic increases, little change, or decreases with smoke age. To the best of our knowledge, no ground-based studies have been conducted on the evolution of smoke from prescribed fires, but frequent airborne studies have investigated prescribed and wildland smoke aging because of the ability to spatially characterize a single plume. While studying two prescribed fires in South Carolina, May et al. (2015) observed no statistically significant net change in OA NEMRs near the source and downwind for smoke transported for ≤ 1.5 h. One of the two fires was studied for longer, and the results showed downwind OA NEMRs over 2 to 5 h of transport that were significantly lower than the NEMRs at the source, suggesting a net loss of emitted OA. For wildfires, Collier et al. (2016) found increases, little change, and decreases with smoke age in different wildfire plumes measured in Oregon. For the selected large wildfires in the western USA in summer, Palm et al. (2020) reported that the OA NEMRs remained almost constant at a value of ∼ 0.25 µg m−3 ppb−1 as the plume aged from 20–50 min to 6 h. In their analysis of the data of wildland fires studied during the FIREX-AQ campaign in 2019, Pagonis et al. (2023) reported that the OA NEMRs increased from 0.2 to 0.3 g g−1 in 3 h (Pagonis et al., 2023). While Garofalo et al. (2019) found no significant change in NEMRs between 0.5 and 8 h transport of smoke from 20 western wildfires, they concluded that there was secondary OA formation through oxidation-driven condensation, but this was balanced by dilution-driven evaporation. Gkatzelis et al. (2024) reported NEMRs of some plumes that were more than 1 h old and that are shown in Table S11 with their corresponding physical ages. For the same fire (William's Flat), the NEMR was 0.331 µg m−3 ppb−1 at a physical age of 15 min, which increased to 0.524 µg m−3 ppb−1 at 102 min (Gkatzelis et al., 2024). A similar increase for the Castle fire was seen when the reported NEMRs were 0.204, 0.244, and 0.463 µg m−3 ppb−1 at 25, 27, and 153 min, respectively. For another fire (Horsefly), the NEMR was 0.398 µg m−3 ppb−1 at a physical age of 65 min and remained at a similar value of 0.391 µg m−3 ppb−1 at 104 min. On average, the mean NEMR for plumes of a physical age of less than 1 h, as reported in their study, was 0.218 ± 0.110. This value is lower than that of plumes older than 1 h, which had a mean value of 0.391 ± 0.131 (Gkatzelis et al., 2024). Overall, we find no trends in our data when considering all the detected smoke plumes, but for periods of expected photochemical activity we observe consistent evidence of aerosol formation with plume age, which might be attributed to the optically thin smoke that allows photochemistry throughout the plume compared to large optically thick wildfires and that leads to more complex photochemistry within the plume (Decker et al., 2021a).
We examined other factors that may contribute to the variability of PM2.5 mass NEMRs. No significant difference was observed between on-base and off-base sources of smoke. The mean PM2.5 mass NEMR of smoke originating from outside the base is 0.208 (range 0.112–0.277 µg m−3 ppb−1) compared to 0.147 µg m−3 ppb−1 (range 0.042–0.466 µg m−3 ppb−1) for on-base burning, which is not statistically different (the two-tailed p value is 0.076). A preliminary assessment using Google Earth satellite imagery and the Landscape Fire and Resources Management Planning Tool (LANDFIRE, https://www.landfire.gov/, last access: 5 May 2024) does not show any visible differences in vegetation between the forested areas burned on and off the base. Additionally, no further information regarding the fuel types in the off-base lands could be obtained. Just like no detected differences being observed between day and night PM2.5 mass concentration ERs, there was no significant difference (the p value is 0.169) between smoke plumes of all ages measured during the day corresponding to fires occurring within a few hours of starting the burn (after 09:00 and before 17:00) (mean NEMR = 0.178 µg m−3 ppb−1) and those monitored at night and early in the morning corresponding to fires starting the day before (after 17:00) (mean NEMR = 0.137 µg m−3 ppb−1), in contrast to an observed trend of PM2.5 mass NEMRs with age for smoke with O3 enhancement. This may suggest little nighttime secondary aerosol formation (Brown et al., 2013), but a more focused analysis is needed to better assess possible evidence of secondary aerosol formation. No correlation was observed between PM2.5 mass NEMRs and relative humidity (r2=0.08) or fuel moisture data (r2=0.04) for the smoke events in this study (Fig. S13 in the Supplement). A weak positive correlation between air temperature and PM2.5 mass NEMRs was observed, with an r2 of 0.14 for all smoke events and an r2 of 0.44 for fresh smoke events. Many factors could cause variability in PM2.5 mass NEMRs, but no single factor could be identified when all the data from this study are grouped together.
Changes in BC and BrC NEMRs with smoke age: BC and BrC NEMRs and age are shown in Fig. 10b and c with periods of O3 enhancements identified. No trend in BC NEMRs with age is observed, as expected, since BC is primarily emitted and largely nonvolatile. The lack of a trend supports this analysis approach, and all the BC measured in events largely reflects the BC variability in emissions relative to CO. BrC NEMRs are also highly variable and have no trend with age for all the data or just the periods of O3 enhancements. Since BrC can be both primary and secondary, is semivolatile, and undergoes photo-bleaching, a range of results on BrC evolution has been observed in past studies (Zhong and Jang, 2014; Saleh et al., 2013; Liu et al., 2016). Like BC, a similar large variability, with no trend, in BrC NEMRs with ages up to 8 h has been observed for wildfires in the western USA (Zeng et al., 2022; Sullivan et al., 2022; Palm et al., 2020), whereas in some cases consistent loss (bleaching) of BrC has been reported (Forrister et al., 2015). Optical properties of absorptive aerosol spectral properties characterized by the AAE are shown in Fig. 11 as a function of age. Total absorption AAE values from the two trailers with seven-wavelength aethalometers (i.e., BC + BrC measured by aethalometers) varied between 1.31 and 3.32 (mean ± SD of 1.89 ± 0.23) and between 3.19 and 7.43 (mean = 5.00 ± 0.89) for BrC only. AAEs have no trend with age for either fresh smoke plumes or periods of O3 enhancement. While our total AAE values are similar (Zeng et al., 2022; Strand et al., 2016; Marsavin et al., 2023) or sometimes lower (Liu et al., 2016; Forrister et al., 2015) than those in other biomass burning studies, it is indicative of the presence of BrC in the smoke plumes studied. As for the BrC AAEs, our reported values are significantly higher than those reported for western wildfires, where BrC determined from the PAS had an AAE of 2.07 ± 1.01 (Zeng et al., 2022), indicating differences in the BrC optical properties or with instrumentation, which needs further investigation. Selimovic et al. (2019) showed that duff has the highest AAE of 7.13 (calculated from absorption data at 401 and 870 nm) when burned, and it is typically consumed more in wildfires than in prescribed fires. However, the variability in optical properties is influenced more by the differential consumption of individual components than by the dominant tree species in the ecosystem (Selimovic et al., 2019).
We describe a ground-based observational study for characterizing smoke from prescribed fires based on continuous monitoring at multiple sites for an extended period in a regularly burned region. We focus on burning within a large military fort in the southeastern USA and identify the sources of the smoke to determine whether it was within or outside the fort and study emissions and evolution of the smoke species. The method was successful in capturing a significant number of smoke events (64) monitored on 42 d and linked to 45 fires across two burning seasons. The source and age for each smoke plume detected were estimated. This allowed us to match 95 % of the identified events to their corresponding source and to calculate the estimated transport time of smoke from the source to the monitors. These data were used to characterize the emissions and evolution of key smoke parameters through calculation of normalized excess mixing ratios (NEMRs), with CO as the conserved co-emitted species. Overall, PM2.5 mass-concentration NEMRs (ΔPM2.5 mass ΔCO) ranged between 0.04 and 0.47 µg m−3 ppb−1, with a study mean of 0.155 ± 0.076 µg m−3 ppb−1 (median 0.138 µg m−3 ppb−1). For plumes less than 1 h old, the PM2.5 mass-concentration NEMRs were interpreted as a characteristic of the fire emissions. The emission ratios for the fires of this study ranged between 0.042 and 0.176 µg m−3 ppb−1, with a mean of 0.117 ± 0.045 µg m−3 ppb−1 (median 0.121 µg m−3 ppb−1). These emission estimates are in the range reported in other ground-based studies for a range of fires and fuels but are lower than what has been reported for wildfire smoke measured from aircraft at higher altitudes. BC and BrC NEMRs and emission ratios are also reported. An analysis of PM2.5 mass and BrC NEMRs changes with smoke age showed no consistent trends for all the combined smoke plumes. However, the PM2.5 mass NEMRs did increase with age for smoke detected in the afternoon in plumes where O3 enhancements were observed, indicating the formation of O3 and secondary aerosol. This was not observed for the BrC NEMRs. This data set will be used to assess models predicting the impact of prescribed fires on air quality to enhance the use of prescribed burning in land management practices by minimizing impacts on populations.
The data are available in a publicly accessible repository on Zenodo at https://doi.org/10.5281/zenodo.11222295 (El Asmar, 2024).
The supplement related to this article is available online at: https://doi.org/10.5194/acp-24-12749-2024-supplement.
REA and RJW wrote the paper. RJW, LGH, DJT, and MTO designed the experiment. REA and DJT collected the data. REA, ZL, DJT, and RJW analyzed the data. REA and ZL worked on the HYSPLIT analysis. All the authors reviewed and provided comments on the paper.
The contact author has declared that none of the authors has any competing interests.
Publisher’s note: Copernicus Publications remains neutral with regard to jurisdictional claims made in the text, published maps, institutional affiliations, or any other geographical representation in this paper. While Copernicus Publications makes every effort to include appropriate place names, the final responsibility lies with the authors.
We thank the Fort Moore authorities for hosting the field study and the members of the Natural Resources Management Branch for sharing information about the burns.
This research has been supported by the United States Army Corps of Engineers (contract no. W912HQ-20-C-0019) and the Strategic Environmental Research and Development Program (project no. RC20-1047).
This paper was edited by Sergey A. Nizkorodov and reviewed by two anonymous referees.
Afrin, S. and Garcia-Menendez, F.: The Influence of Prescribed Fire on Fine Particulate Matter Pollution in the Southeastern United States, Geophys. Res. Lett., 47, e2020GL088988, https://doi.org/10.1029/2020GL088988, 2020.
Akagi, S. K., Craven, J. S., Taylor, J. W., McMeeking, G. R., Yokelson, R. J., Burling, I. R., Urbanski, S. P., Wold, C. E., Seinfeld, J. H., Coe, H., Alvarado, M. J., and Weise, D. R.: Evolution of trace gases and particles emitted by a chaparral fire in California, Atmos. Chem. Phys., 12, 1397–1421, https://doi.org/10.5194/acp-12-1397-2012, 2012.
Akagi, S. K., Burling, I. R., Mendoza, A., Johnson, T. J., Cameron, M., Griffith, D. W. T., Paton-Walsh, C., Weise, D. R., Reardon, J., and Yokelson, R. J.: Field measurements of trace gases emitted by prescribed fires in southeastern US pine forests using an open-path FTIR system, Atmos. Chem. Phys., 14, 199–215, https://doi.org/10.5194/acp-14-199-2014, 2014.
Alves, C. A., Gonçalves, C., Pio, C. A., Mirante, F., Caseiro, A., Tarelho, L., Freitas, M. C., and Viegas, D. X.: Smoke emissions from biomass burning in a Mediterranean shrubland, Atmos. Environ., 44, 3024–3033, https://doi.org/10.1016/j.atmosenv.2010.05.010, 2010.
Andreae, M. O.: Emission of trace gases and aerosols from biomass burning – an updated assessment, Atmos. Chem. Phys., 19, 8523–8546, https://doi.org/10.5194/acp-19-8523-2019, 2019.
Appel, K. W., Bash, J. O., Fahey, K. M., Foley, K. M., Gilliam, R. C., Hogrefe, C., Hutzell, W. T., Kang, D., Mathur, R., Murphy, B. N., Napelenok, S. L., Nolte, C. G., Pleim, J. E., Pouliot, G. A., Pye, H. O. T., Ran, L., Roselle, S. J., Sarwar, G., Schwede, D. B., Sidi, F. I., Spero, T. L., and Wong, D. C.: The Community Multiscale Air Quality (CMAQ) model versions 5.3 and 5.3.1: system updates and evaluation, Geosci. Model Dev., 14, 2867–2897, https://doi.org/10.5194/gmd-14-2867-2021, 2021.
Aurell, J. and Gullett, B. K.: Effects of UAS Rotor Wash on Air Quality Measurements, Drones, 8, 73, https://doi.org/10.3390/drones8030073, 2024.
Aurell, J., Gullett, B., Holder, A., Kiros, F., Mitchell, W., Watts, A., and Ottmar, R.: Wildland fire emission sampling at Fishlake National Forest, Utah using an unmanned aircraft system, Atmos. Environ., 247, 118193, https://doi.org/10.1016/j.atmosenv.2021.118193, 2021.
Balachandran, S., Pachon, J. E., Lee, S., Oakes, M. M., Rastogi, N., Shi, W., Tagaris, E., Yan, B., Davis, A., Zhang, X., Weber, R. J., Mulholland, J. A., Bergin, M. H., Zheng, M., and Russell, A. G.: Particulate and gas sampling of prescribed fires in South Georgia, USA, Atmos. Environ., 81, 125–135, https://doi.org/10.1016/j.atmosenv.2013.08.014, 2013.
Bell, M. L.: Ozone and Short-term Mortality in 95 US Urban Communities, 1987–2000, JAMA, 292, 2372, https://doi.org/10.1001/jama.292.19.2372, 2004.
Bond, T. C., Anderson, T. L., and Campbell, D.: Calibration and Intercomparison of Filter-Based Measurements of Visible Light Absorption by Aerosols, Aerosol Sci. Technol., 30, 582–600, https://doi.org/10.1080/027868299304435, 1999.
Borchers-Arriagada, N., Bowman, D. M. J. S., Price, O., Palmer, A. J., Samson, S., Clarke, H., Sepulveda, G., and Johnston, F. H.: Smoke health costs and the calculus for wildfires fuel management: a modelling study, Lancet Planet. Heal., 5, e608–e619, https://doi.org/10.1016/S2542-5196(21)00198-4, 2021.
Brown, S. S., Dubé, W. P., Bahreini, R., Middlebrook, A. M., Brock, C. A., Warneke, C., de Gouw, J. A., Washenfelder, R. A., Atlas, E., Peischl, J., Ryerson, T. B., Holloway, J. S., Schwarz, J. P., Spackman, R., Trainer, M., Parrish, D. D., Fehshenfeld, F. C., and Ravishankara, A. R.: Biogenic VOC oxidation and organic aerosol formation in an urban nocturnal boundary layer: aircraft vertical profiles in Houston, TX, Atmos. Chem. Phys., 13, 11317–11337, https://doi.org/10.5194/acp-13-11317-2013, 2013.
Burling, I. R., Yokelson, R. J., Akagi, S. K., Urbanski, S. P., Wold, C. E., Griffith, D. W. T., Johnson, T. J., Reardon, J., and Weise, D. R.: Airborne and ground-based measurements of the trace gases and particles emitted by prescribed fires in the United States, Atmos. Chem. Phys., 11, 12197–12216, https://doi.org/10.5194/acp-11-12197-2011, 2011.
Christopher, S. A., Chou, J., Welch, R. M., Kliche, D. V., and Connors, V. S.: Satellite investigations of fire, smoke, and Carbon Monoxide during April 1994 MAPS mission: Case studies over tropical Asia, J. Geophys. Res.-Atmos., 103, 19327–19336, https://doi.org/10.1029/97JD01813, 1998.
Collier, S., Zhou, S., Onasch, T. B., Jaffe, D. A., Kleinman, L., Sedlacek, A. J., Briggs, N. L., Hee, J., Fortner, E., Shilling, J. E., Worsnop, D., Yokelson, R. J., Parworth, C., Ge, X., Xu, J., Butterfield, Z., Chand, D., Dubey, M. K., Pekour, M. S., Springston, S., and Zhang, Q.: Regional Influence of Aerosol Emissions from Wildfires Driven by Combustion Efficiency: Insights from the BBOP Campaign, Environ. Sci. Technol., 50, 8613–8622, https://doi.org/10.1021/acs.est.6b01617, 2016.
Cubison, M. J., Ortega, A. M., Hayes, P. L., Farmer, D. K., Day, D., Lechner, M. J., Brune, W. H., Apel, E., Diskin, G. S., Fisher, J. A., Fuelberg, H. E., Hecobian, A., Knapp, D. J., Mikoviny, T., Riemer, D., Sachse, G. W., Sessions, W., Weber, R. J., Weinheimer, A. J., Wisthaler, A., and Jimenez, J. L.: Effects of aging on organic aerosol from open biomass burning smoke in aircraft and laboratory studies, Atmos. Chem. Phys., 11, 12049–12064, https://doi.org/10.5194/acp-11-12049-2011, 2011.
Decker, Z. C. J., Robinson, M. A., Barsanti, K. C., Bourgeois, I., Coggon, M. M., DiGangi, J. P., Diskin, G. S., Flocke, F. M., Franchin, A., Fredrickson, C. D., Gkatzelis, G. I., Hall, S. R., Halliday, H., Holmes, C. D., Huey, L. G., Lee, Y. R., Lindaas, J., Middlebrook, A. M., Montzka, D. D., Moore, R., Neuman, J. A., Nowak, J. B., Palm, B. B., Peischl, J., Piel, F., Rickly, P. S., Rollins, A. W., Ryerson, T. B., Schwantes, R. H., Sekimoto, K., Thornhill, L., Thornton, J. A., Tyndall, G. S., Ullmann, K., Van Rooy, P., Veres, P. R., Warneke, C., Washenfelder, R. A., Weinheimer, A. J., Wiggins, E., Winstead, E., Wisthaler, A., Womack, C., and Brown, S. S.: Nighttime and daytime dark oxidation chemistry in wildfire plumes: an observation and model analysis of FIREX-AQ aircraft data, Atmos. Chem. Phys., 21, 16293–16317, https://doi.org/10.5194/acp-21-16293-2021, 2021a.
Decker, Z. C. J., Wang, S., Bourgeois, I., Campuzano Jost, P., Coggon, M. M., DiGangi, J. P., Diskin, G. S., Flocke, F. M., Franchin, A., Fredrickson, C. D., Gkatzelis, G. I., Hall, S. R., Halliday, H., Hayden, K., Holmes, C. D., Huey, L. G., Jimenez, J. L., Lee, Y. R., Lindaas, J., Middlebrook, A. M., Montzka, D. D., Neuman, J. A., Nowak, J. B., Pagonis, D., Palm, B. B., Peischl, J., Piel, F., Rickly, P. S., Robinson, M. A., Rollins, A. W., Ryerson, T. B., Sekimoto, K., Thornton, J. A., Tyndall, G. S., Ullmann, K., Veres, P. R., Warneke, C., Washenfelder, R. A., Weinheimer, A. J., Wisthaler, A., Womack, C., and Brown, S. S.: Novel Analysis to Quantify Plume Crosswind Heterogeneity Applied to Biomass Burning Smoke, Environ. Sci. Technol., 55, 15646–15657, https://doi.org/10.1021/acs.est.1c03803, 2021b.
Deng, A., Stauffer, D., Gaudet, B., Dudhia, J., Hacker, J., Bruyere, C., Wu, W., Vandenberghe, F., Liu, Y., and Bourgeois, A.: Update on WRF-ARW end-to-end multi-scale FDDA system, 10th Annual WRF Users’ Workshop, 23 June, Boulder, CO, 2009.
Desservettaz, M., Paton-Walsh, C., Griffith, D. W. T., Kettlewell, G., Keywood, M. D., Vanderschoot, M. V., Ward, J., Mallet, M. D., Milic, A., Miljevic, B., Ristovski, Z. D., Howard, D., Edwards, G. C., and Atkinson, B.: Emission factors of trace gases and particles from tropical savanna fires in Australia, J. Geophys. Res.-Atmos., 122, 6059–6074, https://doi.org/10.1002/2016JD025925, 2017.
El Asmar, R.: A Multi-site Passive Approach for Studying the Emissions and Evolution of Smoke from Prescribed Fires, Zenodo [data set], https://doi.org/10.5281/zenodo.11222295, 2024.
Fiddler, M. N., Thompson, C., Pokhrel, R. P., Majluf, F., Canagaratna, M., Fortner, E. C., Daube, C., Roscioli, J. R., Yacovitch, T. I., Herndon, S. C., and Bililign, S.: Emission Factors From Wildfires in the Western US: An Investigation of Burning State, Ground Versus Air, and Diurnal Dependencies During the FIREX-AQ 2019 Campaign, J. Geophys. Res.-Atmos., 129, e2022JD038460, https://doi.org/10.1029/2022JD038460, 2024.
Fleming, L. T., Lin, P., Roberts, J. M., Selimovic, V., Yokelson, R., Laskin, J., Laskin, A., and Nizkorodov, S. A.: Molecular composition and photochemical lifetimes of brown carbon chromophores in biomass burning organic aerosol, Atmos. Chem. Phys., 20, 1105–1129, https://doi.org/10.5194/acp-20-1105-2020, 2020.
Forrister, H., Liu, J., Scheuer, E., Dibb, J., Ziemba, L., Thornhill, K. L., Anderson, B., Diskin, G., Perring, A. E., Schwarz, J. P., Campuzano-Jost, P., Day, D. A., Palm, B. B., Jimenez, J. L., Nenes, A., and Weber, R. J.: Evolution of brown carbon in wildfire plumes, Geophys. Res. Lett., 42, 4623–4630, https://doi.org/10.1002/2015GL063897, 2015.
Gao, H. and Jaffe, D. A.: Comparison of ultraviolet absorbance and NO-chemiluminescence for ozone measurement in wildfire plumes at the Mount Bachelor Observatory, Atmos. Environ., 166, 224–233, https://doi.org/10.1016/j.atmosenv.2017.07.007, 2017.
Garcia, A., Santa-Helena, E., De Falco, A., de Paula Ribeiro, J., Gioda, A., and Gioda, C. R.: Toxicological Effects of Fine Particulate Matter (PM2.5): Health Risks and Associated Systemic Injuries – Systematic Review, Water, Air, Soil Pollut., 234, 346, https://doi.org/10.1007/s11270-023-06278-9, 2023.
Garofalo, L. A., Pothier, M. A., Levin, E. J. T., Campos, T., Kreidenweis, S. M., and Farmer, D. K.: Emission and Evolution of Submicron Organic Aerosol in Smoke from Wildfires in the Western United States, ACS Earth Sp. Chem., 3, 1237–1247, https://doi.org/10.1021/acsearthspacechem.9b00125, 2019.
Giglio, L., Schroeder, W., Hall, J., and Justice, C.: MODIS Collection 6 and Collection 6.1 Active Fire Product User's Guide, Nasa, Version 1., 64, 2021.
Gkatzelis, G. I., Coggon, M. M., Stockwell, C. E., Hornbrook, R. S., Allen, H., Apel, E. C., Bela, M. M., Blake, D. R., Bourgeois, I., Brown, S. S., Campuzano-Jost, P., St. Clair, J. M., Crawford, J. H., Crounse, J. D., Day, D. A., DiGangi, J. P., Diskin, G. S., Fried, A., Gilman, J. B., Guo, H., Hair, J. W., Halliday, H. S., Hanisco, T. F., Hannun, R., Hills, A., Huey, L. G., Jimenez, J. L., Katich, J. M., Lamplugh, A., Lee, Y. R., Liao, J., Lindaas, J., McKeen, S. A., Mikoviny, T., Nault, B. A., Neuman, J. A., Nowak, J. B., Pagonis, D., Peischl, J., Perring, A. E., Piel, F., Rickly, P. S., Robinson, M. A., Rollins, A. W., Ryerson, T. B., Schueneman, M. K., Schwantes, R. H., Schwarz, J. P., Sekimoto, K., Selimovic, V., Shingler, T., Tanner, D. J., Tomsche, L., Vasquez, K. T., Veres, P. R., Washenfelder, R., Weibring, P., Wennberg, P. O., Wisthaler, A., Wolfe, G. M., Womack, C. C., Xu, L., Ball, K., Yokelson, R. J., and Warneke, C.: Parameterizations of US wildfire and prescribed fire emission ratios and emission factors based on FIREX-AQ aircraft measurements, Atmos. Chem. Phys., 24, 929–956, https://doi.org/10.5194/acp-24-929-2024, 2024.
Hecobian, A., Zhang, X., Zheng, M., Frank, N., Edgerton, E. S., and Weber, R. J.: Water-Soluble Organic Aerosol material and the light-absorption characteristics of aqueous extracts measured over the Southeastern United States, Atmos. Chem. Phys., 10, 5965–5977, https://doi.org/10.5194/acp-10-5965-2010, 2010.
Huang, R., Hu, Y., Russell, A. G., Mulholland, J. A., and Odman, M. T.: The Impacts of Prescribed Fire on PM2.5 Air Quality and Human Health: Application to Asthma-Related Emergency Room Visits in Georgia, USA, Int. J. Environ. Res. Public Heal., 16, 2312, https://doi.org/10.3390/ijerph16132312, 2019.
Ichoku, C. and Kaufman, Y. J.: A method to derive smoke emission rates from MODIS fire radiative energy measurements, IEEE Trans. Geosci. Remote Sens., 43, 2636–2649, https://doi.org/10.1109/TGRS.2005.857328, 2005.
Jaffe, D. A., O'Neill, S. M., Larkin, N. K., Holder, A. L., Peterson, D. L., Halofsky, J. E., and Rappold, A. G.: Wildfire and prescribed burning impacts on air quality in the United States, J. Air Waste Manage. Assoc., 70, 583–615, https://doi.org/10.1080/10962247.2020.1749731, 2020.
Jaffe, D. A., Schnieder, B., and Inouye, D.: Technical note: Use of PM2.5 to CO ratio as an indicator of wildfire smoke in urban areas, Atmos. Chem. Phys., 22, 12695–12704, https://doi.org/10.5194/acp-22-12695-2022, 2022.
Jo, D. S., Park, R. J., Lee, S., Kim, S.-W., and Zhang, X.: A global simulation of brown carbon: implications for photochemistry and direct radiative effect, Atmos. Chem. Phys., 16, 3413–3432, https://doi.org/10.5194/acp-16-3413-2016, 2016.
Kelp, M. M., Carroll, M. C., Liu, T., Yantosca, R. M., Hockenberry, H. E., and Mickley, L. J.: Prescribed Burns as a Tool to Mitigate Future Wildfire Smoke Exposure: Lessons for States and Rural Environmental Justice Communities, Earth's Futur., 11, e2022EF003468, https://doi.org/10.1029/2022EF003468, 2023.
Korontzi, S., Ward, D. E., Susott, R. A., Yokelson, R. J., Justice, C. O., Hobbs, P. V., Smithwick, E. A. H., and Hao, W. M.: Seasonal variation and ecosystem dependence of emission factors for selected trace gases and PM 2.5 for southern African savanna fires, J. Geophys. Res.-Atmos., 108, 4758, https://doi.org/10.1029/2003JD003730, 2003.
Kuenzer, C., Hecker, C., Zhang, J., Wessling, S., and Wagner, W.: The potential of multidiurnal MODIS thermal band data for coal fire detection, Int. J. Remote Sens., 29, 923–944, https://doi.org/10.1080/01431160701352147, 2008.
Lack, D. A. and Langridge, J. M.: On the attribution of black and brown carbon light absorption using the Ångström exponent, Atmos. Chem. Phys., 13, 10535–10543, https://doi.org/10.5194/acp-13-10535-2013, 2013.
Larkin, N. K., O'Neill, S. M., Solomon, R., Raffuse, S., Strand, T., Sullivan, D. C., Krull, C., Rorig, M., Peterson, J., and Ferguson, S. A.: The BlueSky smoke modeling framework, Int. J. Wildl. Fire, 18, 906–920, https://doi.org/10.1071/WF07086, 2009.
Larkin, N. K., Raffuse, S. M., Huang, S. M., Pavlovic, N., Lahm, P., and Rao, V.: The Comprehensive Fire Information Reconciled Emissions (CFIRE) inventory: Wildland fire emissions developed for the 2011 and 2014 U.S. National Emissions Inventory, J. Air Waste Manag. Assoc., 70, 1165–1185, https://doi.org/10.1080/10962247.2020.1802365, 2020.
Laskin, A., Laskin, J., and Nizkorodov, S. A.: Chemistry of Atmospheric Brown Carbon, Chem. Rev., 115, 4335–4382, https://doi.org/10.1021/cr5006167, 2015.
Lee, J. Y., Daube, C., Fortner, E., Ellsworth, N., May, N. W., Tallant, J., Herndon, S., and Pratt, K. A.: Chemical characterization of prescribed burn emissions from a mixed forest in Northern Michigan, Environ. Sci. Atmos., 3, 35–48, https://doi.org/10.1039/D2EA00069E, 2023.
Lee, S., Baumann, K., Schauer, J. J., Sheesley, R. J., Naeher, L. P., Meinardi, S., Blake, D. R., Edgerton, E. S., Russell, A. G., and Clements, M.: Gaseous and Particulate Emissions from Prescribed Burning in Georgia, Environ. Sci. Technol., 39, 9049–9056, https://doi.org/10.1021/es051583l, 2005.
Lee, S., Kim, H. K., Yan, B., Cobb, C. E., Hennigan, C., Nichols, S., Chamber, M., Edgerton, E. S., Jansen, J. J., Hu, Y., Zheng, M., Weber, R. J., and Russell, A. G.: Diagnosis of Aged Prescribed Burning Plumes Impacting an Urban Area, Environ. Sci. Technol., 42, 1438–1444, https://doi.org/10.1021/es7023059, 2008.
Levy, I., Mihele, C., Lu, G., Narayan, J., Hilker, N., and Brook, J. R.: Elucidating multipollutant exposure across a complex metropolitan area by systematic deployment of a mobile laboratory, Atmos. Chem. Phys., 14, 7173–7193, https://doi.org/10.5194/acp-14-7173-2014, 2014.
Li, F., Zhang, X., Kondragunta, S., and Lu, X.: An evaluation of advanced baseline imager fire radiative power based wildfire emissions using carbon monoxide observed by the Tropospheric Monitoring Instrument across the conterminous United States, Environ. Res. Lett., 15, 094049, https://doi.org/10.1088/1748-9326/ab9d3a, 2020.
Linn, R. R., Goodrick, S. L., Brambilla, S., Brown, M. J., Middleton, R. S., O'Brien, J. J., and Hiers, J. K.: QUIC-fire: A fast-running simulation tool for prescribed fire planning, Environ. Model. Softw., 125, 104616, https://doi.org/10.1016/j.envsoft.2019.104616, 2020.
Liu, D., Zhang, Q., Jiang, J., and Chen, D. R.: Performance calibration of low-cost and portable particular matter (PM) sensors, J. Aerosol Sci., 112, 1–10, https://doi.org/10.1016/j.jaerosci.2017.05.011, 2017.
Liu, J. C., Pereira, G., Uhl, S. A., Bravo, M. A., and Bell, M. L.: A systematic review of the physical health impacts from non-occupational exposure to wildfire smoke, Environ. Res., 136, 120–132, https://doi.org/10.1016/j.envres.2014.10.015, 2015.
Liu, T., Marlier, M. E., Karambelas, A., Jain, M., Singh, S., Singh, M. K., Gautam, R., and Defries, R. S.: Missing emissions from post-monsoon agricultural fires in northwestern India: Regional limitations of modis burned area and active fire products, Environ. Res. Commun., 1, 011007, https://doi.org/10.1088/2515-7620/ab056c, 2019.
Liu, X., Zhang, Y., Huey, L. G., Yokelson, R. J., Wang, Y., Jimenez, J. L., Campuzano-Jost, P., Beyersdorf, A. J., Blake, D. R., Choi, Y., St. Clair, J. M., Crounse, J. D., Day, D. A., Diskin, G. S., Fried, A., Hall, S. R., Hanisco, T. F., King, L. E., Meinardi, S., Mikoviny, T., Palm, B. B., Peischl, J., Perring, A. E., Pollack, I. B., Ryerson, T. B., Sachse, G., Schwarz, J. P., Simpson, I. J., Tanner, D. J., Thornhill, K. L., Ullmann, K., Weber, R. J., Wennberg, P. O., Wisthaler, A., Wolfe, G. M., and Ziemba, L. D.: Agricultural fires in the southeastern U.S. during SEAC4RS: Emissions of trace gases and particles and evolution of ozone, reactive nitrogen, and organic aerosol, J. Geophys. Res.-Atmos., 121, 7383–7414, https://doi.org/10.1002/2016JD025040, 2016.
Liu, X., Huey, L. G., Yokelson, R. J., Selimovic, V., Simpson, I. J., Müller, M., Jimenez, J. L., Campuzano-Jost, P., Beyersdorf, A. J., Blake, D. R., Butterfield, Z., Choi, Y., Crounse, J. D., Day, D. A., Diskin, G. S., Dubey, M. K., Fortner, E., Hanisco, T. F., Hu, W., King, L. E., Kleinman, L., Meinardi, S., Mikoviny, T., Onasch, T. B., Palm, B. B., Peischl, J., Pollack, I. B., Ryerson, T. B., Sachse, G. W., Sedlacek, A. J., Shilling, J. E., Springston, S., St. Clair, J. M., Tanner, D. J., Teng, A. P., Wennberg, P. O., Wisthaler, A., and Wolfe, G. M.: Airborne measurements of western U.S. wildfire emissions: Comparison with prescribed burning and air quality implications, J. Geophys. Res.-Atmos., 122, 6108–6129, https://doi.org/10.1002/2016JD026315, 2017.
Liu, Y., Bourgeois, A., Warner, T., Swerdlin, S., and Hacker, J.: Implementation of observation-nudging based FDDA into WRF for supporting ATEC test operations, WRF/MM5 Users' Workshop, NCAR, Boulder, Colorado, USA, June 2005, 27–30, 2005.
Long, R. W., Whitehill, A., Habel, A., Urbanski, S., Halliday, H., Colón, M., Kaushik, S., and Landis, M. S.: Comparison of ozone measurement methods in biomass burning smoke: an evaluation under field and laboratory conditions, Atmos. Meas. Tech., 14, 1783–1800, https://doi.org/10.5194/amt-14-1783-2021, 2021.
Mallia, D. V., Kochanski, A. K., Urbanski, S. P., Mandel, J., Farguell, A., and Krueger, S. K.: Incorporating a Canopy Parameterization within a Coupled Fire-Atmosphere Model to Improve a Smoke Simulation for a Prescribed Burn, Atmosphere, 11, 832, https://doi.org/10.3390/atmos11080832, 2020.
Mandel, J., Beezley, J. D., and Kochanski, A. K.: Coupled atmosphere-wildland fire modeling with WRF 3.3 and SFIRE 2011, Geosci. Model Dev., 4, 591–610, https://doi.org/10.5194/gmd-4-591-2011, 2011.
Marsavin, A., van Gageldonk, R., Bernays, N., May, N. W., Jaffe, D. A., and Fry, J. L.: Optical properties of biomass burning aerosol during the 2021 Oregon fire season: comparison between wild and prescribed fires, Environ. Sci. Atmos., 3, 608–626, https://doi.org/10.1039/D2EA00118G, 2023.
Martin, M. V., Kahn, R. A., and Tosca, M. G.: A global analysis of wildfire smoke injection heights derived from space-based multi-angle imaging, Remote Sens., 10, 1609, https://doi.org/10.3390/rs10101609, 2018.
Martinsson, B. G., Friberg, J., Sandvik, O. S., and Sporre, M. K.: Five-satellite-sensor study of the rapid decline of wildfire smoke in the stratosphere, Atmos. Chem. Phys., 22, 3967–3984, https://doi.org/10.5194/acp-22-3967-2022, 2022.
May, A. A., McMeeking, G. R., Lee, T., Taylor, J. W., Craven, J. S., Burling, I., Sullivan, A. P., Akagi, S., Collett, J. L., Flynn, M., Coe, H., Urbanski, S. P., Seinfeld, J. H., Yokelson, R. J., and Kreidenweis, S. M.: Aerosol emissions from prescribed fires in the United States: A synthesis of laboratory and aircraft measurements, J. Geophys. Res.-Atmos., 119, 11826–11849, https://doi.org/10.1002/2014JD021848, 2014.
May, A. A., Lee, T., McMeeking, G. R., Akagi, S., Sullivan, A. P., Urbanski, S., Yokelson, R. J., and Kreidenweis, S. M.: Observations and analysis of organic aerosol evolution in some prescribed fire smoke plumes, Atmos. Chem. Phys., 15, 6323–6335, https://doi.org/10.5194/acp-15-6323-2015, 2015.
Mell, W., Jenkins, M. A., Gould, J., and Cheney, P.: A physics-based approach to modelling grassland fires, Int. J. Wildl. Fire, 16, 1–22, https://doi.org/10.1071/WF06002, 2007.
Melvin, M. A.: National Prescribed Fire Use Survey Report, Coalition of Prescribed Fire Councils and the National Associations of State Foresters, https://www.stateforesters.org/newsroom-category/publications/ (last access: 15 July 2024), 2018.
Melvin, M. A.: National Prescribed Fire Use Survey Report, Coalition of Prescribed Fire Councils and the National Associations of State Foresters, https://www.stateforesters.org/newsroom-category/publications/ (last access: 15 July 2024), 2020.
Melvin, M. A.: National Prescribed Fire Use Survey Report, Coalition of Prescribed Fire Councils and the National Associations of State Foresters, https://www.stateforesters.org/newsroom-category/publications/ (last access: 15 July 2024), 2021.
Mildrexler, D. J., Zhao, M., Heinsch, F. A., and Running, S. W.: A new satellite-based methodology for continental-scale disturbance detection, Ecol. Appl., 17, 235–250, https://doi.org/10.1890/1051-0761(2007)017[0235:ANSMFC]2.0.CO;2, 2007.
Naeher, L. P., Brauer, M., Lipsett, M., Zelikoff, J. T., Simpson, C. D., Koenig, J. Q., and Smith, K. R.: Woodsmoke Health Effects: A Review, Inhal. Toxicol., 19, 67–106, https://doi.org/10.1080/08958370600985875, 2007.
Nguyen, H. M. and Wooster, M. J.: Advances in the estimation of high Spatio-temporal resolution pan-African top-down biomass burning emissions made using geostationary fire radiative power (FRP) and MAIAC aerosol optical depth (AOD) data, Remote Sens. Environ., 248, 111971, https://doi.org/10.1016/j.rse.2020.111971, 2020.
O'Dell, K., Hornbrook, R. S., Permar, W., Levin, E. J. T., Garofalo, L. A., Apel, E. C., Blake, N. J., Jarnot, A., Pothier, M. A., Farmer, D. K., Hu, L., Campos, T., Ford, B., Pierce, J. R., and Fischer, E. V.: Correction to Hazardous Air Pollutants in Fresh and Aged Western US Wildfire Smoke and Implications for Long-Term Exposure, Environ. Sci. Technol., 56, 3304–3304, https://doi.org/10.1021/acs.est.2c01008, 2022.
Pagonis, D., Selimovic, V., Campuzano-Jost, P., Guo, H., Day, D. A., Schueneman, M. K., Nault, B. A., Coggon, M. M., DiGangi, J. P., Diskin, G. S., Fortner, E. C., Gargulinski, E. M., Gkatzelis, G. I., Hair, J. W., Herndon, S. C., Holmes, C. D., Katich, J. M., Nowak, J. B., Perring, A. E., Saide, P., Shingler, T. J., Soja, A. J., Thapa, L. H., Warneke, C., Wiggins, E. B., Wisthaler, A., Yacovitch, T. I., Yokelson, R. J., and Jimenez, J. L.: Impact of Biomass Burning Organic Aerosol Volatility on Smoke Concentrations Downwind of Fires, Environ. Sci. Technol., 57, 17011–17021, https://doi.org/10.1021/acs.est.3c05017, 2023.
Palm, B. B., Peng, Q., Fredrickson, C. D., Lee, B. H., Garofalo, L. A., Pothier, M. A., Kreidenweis, S. M., Farmer, D. K., Pokhrel, R. P., Shen, Y., Murphy, S. M., Permar, W., Hu, L., Campos, T. L., Hall, S. R., Ullmann, K., Zhang, X., Flocke, F., Fischer, E. V., and Thornton, J. A.: Quantification of organic aerosol and brown carbon evolution in fresh wildfire plumes, P. Natl. Acad. Sci. USA, 117, 29469–29477, https://doi.org/10.1073/pnas.2012218117, 2020.
Parrish, D. D., Holloway, J. S., and Fehsenfeld, F. C.: Routine, Continuous Measurement of Carbon Monoxide with Parts per Billion Precision, Environ. Sci. Technol., 28, 1615–1618, https://doi.org/10.1021/es00058a013, 1994.
Patashnick, H. and Rupprecht, E. G.: Continuous PM-10 Measurements Using the Tapered Element Oscillating Microbalance, J. Air Waste Manag. Assoc., 41, 1079–1083, https://doi.org/10.1080/10473289.1991.10466903, 1991.
Permar, W., Wang, Q., Selimovic, V., Wielgasz, C., Yokelson, R. J., Hornbrook, R. S., Hills, A. J., Apel, E. C., Ku, I., Zhou, Y., Sive, B. C., Sullivan, A. P., Collett, J. L., Campos, T. L., Palm, B. B., Peng, Q., Thornton, J. A., Garofalo, L. A., Farmer, D. K., Kreidenweis, S. M., Levin, E. J. T., DeMott, P. J., Flocke, F., Fischer, E. V., and Hu, L.: Emissions of Trace Organic Gases From Western U.S. Wildfires Based on WE-CAN Aircraft Measurements, J. Geophys. Res.-Atmos., 126, e2020JD033838, https://doi.org/10.1029/2020JD033838, 2021.
Pratt, K. A., Murphy, S. M., Subramanian, R., DeMott, P. J., Kok, G. L., Campos, T., Rogers, D. C., Prenni, A. J., Heymsfield, A. J., Seinfeld, J. H., and Prather, K. A.: Flight-based chemical characterization of biomass burning aerosols within two prescribed burn smoke plumes, Atmos. Chem. Phys., 11, 12549–12565, https://doi.org/10.5194/acp-11-12549-2011, 2011.
Prichard, S. J., O'Neill, S. M., Eagle, P., Andreu, A. G., Drye, B., Dubowy, J., Urbanski, S., and Strand, T. M.: Wildland fire emission factors in North America: synthesis of existing data, measurement needs and management applications, Int. J. Wildl. Fire, 29, 132–147, https://doi.org/10.1071/WF19066, 2020.
Reid, C. E., Brauer, M., Johnston, F. H., Jerrett, M., Balmes, J. R., and Elliott, C. T.: Critical Review of Health Impacts of Wildfire Smoke Exposure, Environ. Health Perspect., 124, 1334–1343, https://doi.org/10.1289/ehp.1409277, 2016.
Saleh, R., Hennigan, C. J., McMeeking, G. R., Chuang, W. K., Robinson, E. S., Coe, H., Donahue, N. M., and Robinson, A. L.: Absorptivity of brown carbon in fresh and photo-chemically aged biomass-burning emissions, Atmos. Chem. Phys., 13, 7683–7693, https://doi.org/10.5194/acp-13-7683-2013, 2013.
Schroeder, W. and Giglio, L.: NASA VIIRS Land Science Investigator Processing System (SIPS) Visible Infrared Imaging Radiometer Suite (VIIRS) 375 m and 750 m Active Fire Products: Product User's Guide, Nasa, 1.4, 2–23, 2018.
Selimovic, V., Yokelson, R. J., McMeeking, G. R., and Coefield, S.: In situ measurements of trace gases, PM, and aerosol optical properties during the 2017 NW US wildfire smoke event, Atmos. Chem. Phys., 19, 3905–3926, https://doi.org/10.5194/acp-19-3905-2019, 2019.
Shamarock, W. C., Klemp, J. B., Dudhia, J., Gill, D. O., Liu, Z., Berner, J., Wang, W., Powers, J. G., Duda, M. G., Barker, D. M., and Huang, X.-Y.: A Description of the Advanced Research WRF Model Version 4, https://doi.org/10.5065/1DFH-6P97, 2019.
Singleton, M. P., Thode, A. E., Sánchez Meador, A. J., and Iniguez, J. M.: Increasing trends in high-severity fire in the southwestern USA from 1984 to 2015, Forest Ecol. Manag., 433, 709–719, https://doi.org/10.1016/j.foreco.2018.11.039, 2019.
Sinha, P., Hobbs, P. V., Yokelson, R. J., Bertschi, I. T., Blake, D. R., Simpson, I. J., Gao, S., Kirchstetter, T. W., and Novakov, T.: Emissions of trace gases and particles from savanna fires in southern Africa, J. Geophys. Res.-Atmos., 108, 8487, https://doi.org/10.1029/2002JD002325, 2003.
Stein, A. F., Draxler, R. R., Rolph, G. D., Stunder, B. J. B., Cohen, M. D., and Ngan, F.: NOAA's HYSPLIT Atmospheric Transport and Dispersion Modeling System, Bull. Am. Meteorol. Soc., 96, 2059–2077, https://doi.org/10.1175/BAMS-D-14-00110.1, 2015.
Strand, T., Gullett, B., Urbanski, S., O'Neill, S., Potter, B., Aurell, J., Holder, A., Larkin, N., Moore, M., and Rorig, M.: Grassland and forest understorey biomass emissions from prescribed fires in the south-eastern United States – RxCADRE 2012, Int. J. Wildl. Fire, 25, 102–113, https://doi.org/10.1071/WF14166, 2016.
Sullivan, A. P., Pokhrel, R. P., Shen, Y., Murphy, S. M., Toohey, D. W., Campos, T., Lindaas, J., Fischer, E. V., and Collett Jr., J. L.: Examination of brown carbon absorption from wildfires in the western US during the WE-CAN study, Atmos. Chem. Phys., 22, 13389–13406, https://doi.org/10.5194/acp-22-13389-2022, 2022.
Travis, K. R., Crawford, J. H., Soja, A. J., Gargulinski, E. M., Moore, R. H., Wiggins, E. B., Diskin, G. S., DiGangi, J. P., Nowak, J. B., Halliday, H., Yokelson, R. J., McCarty, J. L., Simpson, I. J., Blake, D. R., Meinardi, S., Hornbrook, R. S., Apel, E. C., Hills, A. J., Warneke, C., Coggon, M. M., Rollins, A. W., Gilman, J. B., Womack, C. C., Robinson, M. A., Katich, J. M., Peischl, J., Gkatzelis, G. I., Bourgeois, I., Rickly, P. S., Lamplugh, A., Dibb, J. E., Jimenez, J. L., Campuzano-Jost, P., Day, D. A., Guo, H., Pagonis, D., Wennberg, P. O., Crounse, J. D., Xu, L., Hanisco, T. F., Wolfe, G. M., Liao, J., St. Clair, J. M., Nault, B. A., Fried, A., and Perring, A. E.: Emission Factors for Crop Residue and Prescribed Fires in the Eastern US During FIREX-AQ, J. Geophys. Res.-Atmos., 128, e2023JD039309, https://doi.org/10.1029/2023JD039309, 2023.
USDA: Wildfire crisis strategy implementation plan: A 10-year implementation plan, https://www.fs.usda.gov/sites/default/files/Wildfire-Crisis-Implementation-Plan.pdf/ (last aaccess: 18 July 2024), 2022.
Virkkula, A., Ahlquist, N. C., Covert, D. S., Arnott, W. P., Sheridan, P. J., Quinn, P. K., and Coffman, D. J.: Modification, calibration and a field test of an instrument for measuring light absorption by particles, Aerosol Sci. Technol., 39, 68–83, https://doi.org/10.1080/027868290901963, 2005.
Virkkula, A., Mäkelä, T., Hillamo, R., Yli-Tuomi, T., Hirsikko, A., Hämeri, K., and Koponen, I. K.: A Simple Procedure for Correcting Loading Effects of Aethalometer Data, J. Air Waste Manage. Assoc., 57, 1214–1222, https://doi.org/10.3155/1047-3289.57.10.1214, 2007.
Wang, J., Yue, Y., Wang, Y., Ichoku, C., Ellison, L., and Zeng, J.: Mitigating Satellite-Based Fire Sampling Limitations in Deriving Biomass Burning Emission Rates: Application to WRF-Chem Model Over the Northern sub-Saharan African Region, J. Geophys. Res.-Atmos., 123, 507–528, https://doi.org/10.1002/2017JD026840, 2018.
Warneke, C., Schwarz, J. P., Dibb, J., Kalashnikova, O., Frost, G., Al-Saad, J., Brown, S. S., Brewer, W. A., Soja, A., Seidel, F. C., Washenfelder, R. A., Wiggins, E. B., Moore, R. H., Anderson, B. E., Jordan, C., Yacovitch, T. I., Herndon, S. C., Liu, S., Kuwayama, T., Jaffe, D., Johnston, N., Selimovic, V., Yokelson, R., Giles, D. M., Holben, B. N., Goloub, P., Popovici, I., Trainer, M., Kumar, A., Pierce, R. B., Fahey, D., Roberts, J., Gargulinski, E. M., Peterson, D. A., Ye, X., Thapa, L. H., Saide, P. E., Fite, C. H., Holmes, C. D., Wang, S., Coggon, M. M., Decker, Z. C. J., Stockwell, C. E., Xu, L., Gkatzelis, G., Aikin, K., Lefer, B., Kaspari, J., Griffin, D., Zeng, L., Weber, R., Hastings, M., Chai, J., Wolfe, G. M., Hanisco, T. F., Liao, J., Campuzano Jost, P., Guo, H., Jimenez, J. L., and Crawford, J.: Fire Influence on Regional to Global Environments and Air Quality (FIREX-AQ), J. Geophys. Res.-Atmos., 128, e2022JD037758, https://doi.org/10.1029/2022JD037758, 2023.
Wyden, R. and Manchin, J.: National Prescribed Fire Act of 2020, 116th Congress, 2nd Session, U.S. Government Publishing Office, https://www.govtrack.us/congress/bills/116/s4625/ (last access: 10 April 2024) 2020.
Xi, Y., Kshirsagar, A. V., Wade, T. J., Richardson, D. B., Brookhart, M. A., Wyatt, L., and Rappold, A. G.: Mortality in US Hemodialysis Patients Following Exposure to Wildfire Smoke, J. Am. Soc. Nephrol., 31, 1824–1835, https://doi.org/10.1681/ASN.2019101066, 2020.
Xiu, M., Jayaratne, R., Thai, P., Christensen, B., Zing, I., Liu, X., and Morawska, L.: Evaluating the applicability of the ratio of PM2.5 and carbon monoxide as source signatures, Environ. Pollut., 306, 119278, https://doi.org/10.1016/j.envpol.2022.119278, 2022.
Yan, J., Wang, X., Gong, P., Wang, C., and Cong, Z.: Review of brown carbon aerosols: Recent progress and perspectives, Sci. Total Environ., 634, 1475–1485, https://doi.org/10.1016/j.scitotenv.2018.04.083, 2018.
Yokelson, R. J., Goode, J. G., Ward, D. E., Susott, R. A., Babbitt, R. E., Wade, D. D., Bertschi, I., Griffith, D. W. T., and Hao, W. M.: Emissions of formaldehyde, acetic acid, methanol, and other trace gases from biomass fires in North Carolina measured by airborne Fourier transform infrared spectroscopy, J. Geophys. Res.-Atmos., 104, 30109–30125, https://doi.org/10.1029/1999JD900817, 1999.
Yokelson, R. J., Burling, I. R., Gilman, J. B., Warneke, C., Stockwell, C. E., de Gouw, J., Akagi, S. K., Urbanski, S. P., Veres, P., Roberts, J. M., Kuster, W. C., Reardon, J., Griffith, D. W. T., Johnson, T. J., Hosseini, S., Miller, J. W., Cocker III, D. R., Jung, H., and Weise, D. R.: Coupling field and laboratory measurements to estimate the emission factors of identified and unidentified trace gases for prescribed fires, Atmos. Chem. Phys., 13, 89–116, https://doi.org/10.5194/acp-13-89-2013, 2013.
Yu, Y., Zou, W., Jerrett, M., and Meng, Y.-Y.: Acute health impact of wildfire-related and conventional PM2.5 in the United States: A narrative review, Environ. Adv., 12, 100179, https://doi.org/10.1016/j.envadv.2022.100179, 2023.
Zeng, L., Dibb, J., Scheuer, E., Katich, J. M., Schwarz, J. P., Bourgeois, I., Peischl, J., Ryerson, T., Warneke, C., Perring, A. E., Diskin, G. S., DiGangi, J. P., Nowak, J. B., Moore, R. H., Wiggins, E. B., Pagonis, D., Guo, H., Campuzano-Jost, P., Jimenez, J. L., Xu, L., and Weber, R. J.: Characteristics and evolution of brown carbon in western United States wildfires, Atmos. Chem. Phys., 22, 8009–8036, https://doi.org/10.5194/acp-22-8009-2022, 2022.
Zhong, M. and Jang, M.: Dynamic light absorption of biomass-burning organic carbon photochemically aged under natural sunlight, Atmos. Chem. Phys., 14, 1517–1525, https://doi.org/10.5194/acp-14-1517-2014, 2014.