the Creative Commons Attribution 4.0 License.
the Creative Commons Attribution 4.0 License.
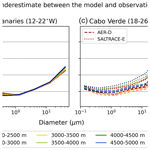
Long-range transport of coarse mineral dust: an evaluation of the Met Office Unified Model against aircraft observations
Natalie G. Ratcliffe
Claire L. Ryder
Nicolas Bellouin
Stephanie Woodward
Anthony Jones
Ben Johnson
Lisa-Maria Wieland
Maximilian Dollner
Josef Gasteiger
Bernadett Weinzierl
Coarse mineral dust particles have been observed much further from the Sahara than expected based on theory. They have impacts different to finer particles on Earth's radiative budget, as well as carbon and hydrological cycles, though they tend to be under-represented in climate models. We use measurements of the full dust size distribution from aircraft campaigns over the Sahara, Canaries, Cabo Verde and Caribbean. We assess the observed and modelled dust size distribution over long-range transport at high vertical resolution using the Met Office Unified Model, which represents dust up to 63.2 µm diameter, greater than most climate models. We show that the model generally replicates the vertical distribution of the total dust mass but transports larger dust particles too low in the atmosphere. Importantly, coarse particles in the model are deposited too quickly, resulting in an underestimation of dust mass that is exacerbated with westwards transport; the 20–63 µm dust mass contribution between 2 and 3.7 km altitude is underestimated by factors of up to 11 in the Sahara, 140 in the Canaries and 240 in Cabo Verde. In the Caribbean, there is negligible modelled contribution of d > 20 µm particles to total mass, compared to 10 % in the observations. This work adds to the growing body of research that demonstrates the need for a process-based evaluation of climate model dust simulations to identify where improvements could be implemented.
- Article
(5254 KB) - Full-text XML
-
Supplement
(638 KB) - BibTeX
- EndNote
Every year, 400–2200 Mt of mineral dust is lifted from Earth's surface and becomes suspended in the atmosphere (Huneeus et al., 2011). This lofted dust can alter the global radiation budget by directly reflecting and absorbing radiation (Kok et al., 2018) and altering cloud properties (Lohmann and Feichter, 2005; Price et al., 2018) and precipitation patterns (Rosenfeld et al., 2008) by activating ice and liquid droplet nucleation. Shao et al. (2011) estimate that 75 % of the uplifted dust is deposited on land, providing important nutrients to locations such as the Amazon rainforest (Prospero et al., 2020) as well as altering the surface albedo upon deposition, for example on snow and ice (Dumont et al., 2020; Painter et al., 2007). The remaining dust supplies valuable nutrients to nutrient-poor oceans, potentially resulting in the formation of phytoplankton blooms (Jickells et al., 2005; Dansie et al., 2022). Lofted dust also negatively impacts aviation (Nickovic et al., 2021), energy production (Piedra et al., 2018) and human health (Kotsyfakis et al., 2019). Many of these processes are sensitive to particle size.
Coarse (2.5 10 µm), super-coarse (10 62.5 µm) and giant (d > 62.5 µm) dust particles (size ranges as reviewed and defined in Adebiyi et al., 2023) have vastly different impacts on the Earth system than fine (d < 2.5 µm) particles. The lifetime of dust in the atmosphere decreases exponentially with increasing particle diameter (Kok et al., 2017). Sedimentation varies strongly with particle size and dominantly affects super-coarse and giant particles (Foret et al., 2006). The larger particles are also more susceptible to wet deposition processes as they are efficient in-cloud nucleators of ice (Hoose and Möhler, 2012; Pruppacher and Klett, 2010; Sassen et al., 2003; Adebiyi et al., 2023) and, after undergoing in-cloud chemical processing, liquid water (Nenes et al., 2014; Karydis et al., 2011). Coarser particles are also more likely to be removed by below-cloud scavenging (Jones et al., 2022). Coarser particles decrease the amount of outgoing longwave radiation at the top of the atmosphere (TOA) and increase shortwave absorption in the atmosphere, both of which cause a net warming effect at the TOA (Kok et al., 2018). Larger particles also contain a greater mass of the nutrients which provide vital sustenance for the biosphere (Barkley et al., 2021; Baker et al., 2006; Dansie et al., 2017). Simulating the lifetime and transport range of differently sized dust particles in models is therefore key to capturing their various effects and impacts.
Recent field campaigns have revealed that coarse, super-coarse and giant particles are transported further across the Atlantic from the Sahara than expected, given their estimated deposition velocity and amount of time in transit (Ryder et al., 2018; Weinzierl et al., 2017; Ryder et al., 2019; van der Does et al., 2016; Denjean et al., 2016). The processes responsible for this unexpected long-range transport are unclear. Additionally, many global climate models (GCMs) do not represent super-coarse or giant particles and fail to represent the mass concentration of coarse particles at any stage of transport (Adebiyi and Kok, 2020; O'Sullivan et al., 2020; Huang et al., 2021; Ansmann et al., 2017). Ryder et al. (2019) estimate that by not representing these particles, dust mass over the Sahara in GCMs is underestimated by up to a factor of 5. The lack of representation of coarser dust particles in GCMs means that they may simulate a direct radiative effect (DRE) forcing that is too small in the longwave (positive DRE) and too negative in the shortwave (negative DRE) (Kok et al., 2017; Adebiyi and Kok, 2020) and therefore are too negative in total forcing (shortwave plus longwave). By representing particles up to 20 µm, Adebiyi and Kok (2020) estimate that the dust DRE at the TOA in AeroCom models (currently in the range of −0.78 to −0.03 W m−2) would be shifted to approximately −0.4 to +0.3 W m−2, meaning that dust could have a net warming or cooling impact on climate.
By comparing observations to model simulations, previous studies have been able to evaluate the representation of dust size distribution at various points throughout the dust life cycle. Ansmann et al. (2017) found that several dust numerical weather prediction (NWP) forecasts were accurate up to 2000 km west of the coast of Africa, but, beyond this, rapid dust removal reduced the quality of the forecast in terms of the total dust mass concentration and 500–550 nm extinction coefficient. Dust-related processes in models are often tuned so that the modelled aerosol optical depth (AOD) matches observed AODs retrieved by satellite instruments. O'Sullivan et al. (2020) show that observations from a campaign obtaining in situ and remote sensing measurements over the eastern Atlantic agreed with an NWP forecast and a reanalysis output in terms of the AOD but struggled to show the correct vertical and horizontal distribution of coarser particles. By tuning models to AOD, a fine bias is often created in the dust size distribution to compensate for the under-represented (or absent) coarser particles.
Some studies have shown that altering certain fixed parameters in the model, such as settling velocity or particle density, can improve model agreement with observations. Drakaki et al. (2022) found that decreasing the settling velocities of dust in the model by 40 %–80 % produced good agreement of the size distribution with in situ aircraft observations over the Sahara and the eastern Atlantic. By reducing the settling velocity (by 13 % in line with suggestions by Huang et al., 2020) and lowering the dust particle density from 2500 to between 125 and 250 kg m−3, Meng et al. (2022) were able to improve model agreement with observations in terms of the super-coarse-particle volume near the Sahara, though the dust volume was still underestimated in dust outflow regions. These significant, order-of-magnitude changes to particle density and settling velocity are not representative of realistic uncertainties in these variables or processes and instead act as a proxy for representing poorly understood processes which can potentially impact particle lifetime, such as electric charging (van der Does et al., 2018; Toth et al., 2020; Renard et al., 2018; Méndez Harper et al., 2022), asphericity (Huang et al., 2021, 2020; Mallios et al., 2020; Saxby et al., 2018; Colarco et al., 2014; Yang et al., 2013), turbulence (Denjean et al., 2016; Rodakoviski et al., 2023), topography (Heisel et al., 2021; Rosenberg et al., 2014) and vertical mixing (Gasteiger et al., 2017; Cornwell et al., 2021). Nowottnick et al. (2010) found that an improvement of wet scavenging processes in a model improved coarse particles' lifetime.
The Fennec (Ryder et al., 2013b, a, 2015), AERosol properties – Dust (AER-D) (Ryder et al., 2018), and Saharan Aerosol Long-range Transport and Aerosol-Cloud-Interaction Experiment (SALTRACE) (Weinzierl et al., 2017) airborne campaigns measured vertically resolved size distributions at four locations between the Sahara and Caribbean and thus represent observations at different stages in the long-range trans-Atlantic transport of Saharan dust. These campaigns measured the full size range of lofted mineral dust particles using open-path wing probes, unlike many previous campaigns which assumed the transport of coarser particles to be minimal. They therefore did not substantially measure into the coarse, super-coarse or giant size range, or measurements of coarser particles were restricted by sampling constraints due to instrument inlets and pipework (Ryder et al., 2019; Rosenberg et al., 2014). This study is the first time that these three campaigns will have been analysed together, in particular taking the vertical distribution of dust size into account. In order to better understand the ability of models to simulate dust transport and deposition, these campaigns will be analysed and compared to a Met Office Unified Model (MetUM) climate simulation (HadGEM3-GA7.1) (Walters et al., 2019). HadGEM3-GA7.1 includes a representation of coarse dust particles up to 63.2 µm in diameter, a notably larger upper size limit than other models which tend to cut off the represented dust size distribution at ∼ 20 µm (Mahowald et al., 2014; Zhao et al., 2022; Huneeus et al., 2011). The HadGEM3-GA7.1 dust simulation has not yet been extensively compared with in situ airborne observations. The campaigns and model have not had their vertically resolved dust size distribution evolution assessed in such detail before and over such a large spatial extent, representing the vertically resolved size distribution evolution over long-range transport. O'Sullivan et al. (2020) suggest that the earlier MetUM NWP GA6.1 configuration (notably different with dust represented by two size bins) often places dust too low in the atmosphere, over the eastern Atlantic, which we investigate in this study.
This study aims to gain a more in-depth insight into the systematic biases between modelled and observed size distributions and how those biases evolve during transport. Such assessments of model performance are crucial in guiding improvements to the model representation of mineral dust transport and deposition.
In Sect. 2, we introduce the aircraft campaigns, the model setup used in this study and our methodology for the analysis. In Sect. 3, we investigate the relationship between the coarser-dust size distribution and the AOD in the aircraft observations. In Sect. 4, we present and discuss our results, analysing the vertical dust structure, size distribution and concentration evolution across the Atlantic in the model and observations. In Sect. 5 we summarise and present conclusions.
2.1 Aircraft observations
The vertically resolved in situ aircraft observations used in this study were taken during scientific flights in the Sahara, the Canary Islands, Cabo Verde and the Caribbean during the Fennec, AER-D and SALTRACE campaigns. Figure 1 shows the location of the observations (flight tracks) used in this study. All aircraft observations are presented at ambient conditions. The Fennec and AER-D campaigns made use of the Facility for Airborne Atmospheric Measurements (FAAM) BAe-146 aircraft and instruments (Ryder et al., 2013b, a, 2018), while the SALTRACE campaign used the Deutsches Zentrum für Luft- und Raumfahrt (DLR) Falcon aircraft and instruments (Weinzierl et al., 2017). The following two sections describe these two different aircraft and instrumentation setups. Henceforth all aerosol sizes will be given in diameters.
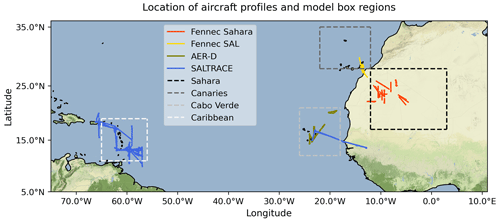
Figure 1Location of the vertical profiles measured during the Fennec and AER-D campaigns, as well as the flight paths followed during the SALTRACE campaign (solid lines), with the regions (boxes) used for the analysis of the model data (dashed lines).
2.1.1 FAAM BAe-146 aircraft setup
The Fennec campaign took place in June 2011, flying over a remote region of the Sahara (Mauritania and Mali), as well as over the Canary Islands (Fig. 1; Fennec Sahara and Fennec SAL, respectively). This campaign therefore provides data at two separate locations: firstly over the desert close to dust sources (Fennec Sahara) (Ryder et al., 2013b) and secondly as the Saharan air layer (SAL) forms over the marine boundary layer (MBL) between the western coast of Africa and the Canary Islands (Fennec SAL) (Ryder et al., 2013a). In total, 41 vertical profiles were conducted during the Fennec campaign: 20 over the Canaries and 21 over the Sahara (Table 1). These profiles are conducted as the aircraft ascends/descends between the minimum safe altitude (around 160 m above ground level depending on visibility) and up to 8 km. The profiles in the Canaries were measured as the aircraft travelled to and from Fuerteventura Airport (28.4° N 13.8° W) and the Sahara, so two profiles were usually measured per flight. The lowest portion of the profile was over the ocean, while the highest altitude of the profile lies just over the continent.
Table 1Details of the Fennec flights used in this study including the date and time of the flights. Time is given to the nearest 30 min. The number of profiles are described by the number taken from the Canaries (C) and the number from northern Mali (Ma) and northern Mauritania (Mu). Data taken from Ryder et al. (2013b, a).
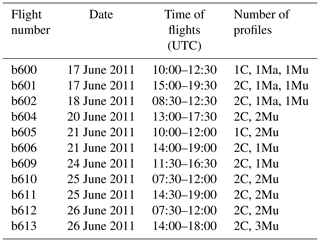
The AER-D campaign took place in August 2015, conducting 26 vertical profiles in the Cabo Verde region. The flights from which these profiles are taken are described in Table 2.
Table 3 shows the instruments operated in each campaign and the size range applied from each instrument, adjusted from geometric to optical diameter (see Ryder et al., 2013a, 2018, for details). Both the Fennec and AER-D campaigns measured particles up to 300 µm diameter. In order to tailor our analysis to the model, only observations corresponding to the model size bins (up to 63.2 µm diameter) are used in this study.
Table 3Size distribution instruments and scattering and absorption instruments used during the Fennec and/or AER-D campaign, where Y (N) indicates the instrument being operational (non-operational). Sizes are given as a geometric diameter. Size ranges correspond to data selected for model intercomparisons (as opposed to the full range measured by the instruments). Additional details are provided in Table S1 in the Supplement. Data taken from Ryder et al. (2013b, 2018, 2015), Walser (2017) and Weinzierl et al. (2017).
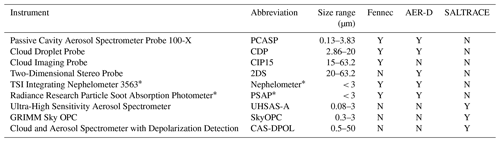
* Instrument is located in the cabin, behind an inlet.
During Fennec, wing-mounted (i.e. with no fuselage inlet) optical particle counter (OPC) probes were operated to measure the accumulation mode and coarse- to super-coarse-mode size distributions (Passive Cavity Aerosol Spectrometer Probe, PCASP; Cloud Droplet Probe, CDP), while measurements from a wing-mounted optical array probe (OAP), the Cloud Imaging Probe (CIP15), are used for the super-coarse and giant modes. The OPCs use light-scattering measurement techniques, and therefore the size bins applied are adjusted for a dust refractive index of 1.53–0.001i, based on scattering and absorption measurements (Ryder et al., 2013a). Errors due to uncertainties and oscillations in the Mie scattering curve for the OPCs, in addition to systematic error for the PCASP and random (counting) errors for all probes, were propagated through to size distribution uncertainties. Full details of Fennec instrument processing are given in Ryder et al. (2013b, a).
During AER-D, the same wing-mounted OPCs were operated (PCASP and CDP), while measurements from the OAP Two-Dimensional Stereo Probe (2DS) are used for the super-coarse to giant mode. As with Fennec, the size bins applied to the OPC data are adjusted for a dust refractive index of 1.53–0.001i based on scattering and absorption measurements (Ryder et al., 2018). Sizing for the 2DS is performed using the mean of the x and y dimensions of each particle image, in order to be consistent with Fennec data processing, and is also curtailed at 300 µm for this reason, though few particles approaching this size were detected during AER-D. We propagate errors in the size and number distribution due to uncertainties and oscillations in the Mie scattering curve for the OPCs, in addition to random errors (from counting and discretisation errors) and systematic errors (from the sample area) for all instruments. Full details of AER-D instrument processing are given in Ryder et al. (2018).
During both Fennec and AER-D, the aircraft measured the scattering coefficient with a TSI Integrating Nephelometer 3563 and absorption coefficient with a Radiance Research Particle Soot Absorption Photometer (PSAP) (Ryder et al., 2015). These instruments are located in the cabin, behind Rosemount inlets, with an estimated 50 % efficiency for diameters below 3 µm, resulting from inlet losses and pipework transmission losses (Ryder et al., 2013b, 2018). The sum of scattering and absorption provides extinction; this has been integrated vertically to provide AOD at 550 nm, representing AOD for d < 3 µm. AOD at the time of observation could therefore be marginally larger than the AODs presented here.
Due to dust-induced visibility reductions impacting the minimum safe altitude for flying, the minimum height of observational data in the Sahara varies by flight, from around 100–500 m above ground. Therefore, we impose a minimum altitude threshold of 500 m here for the Fennec Sahara profile analysis to avoid sampling bias across different flight, weather and dust conditions. Data collected in the MBL may contain contaminated dust and non-dust aerosols, such as sea salt and anthropogenic pollution. Compositional analysis carried out by Ryder et al. (2018) of the aerosols measured during the AER-D campaign showed that particles of d > 0.5 µm were dominated by aluminosilicates and quartz, while, for those between 0.1 and 0.5 µm, the dominant particles were sulfates and salts. As we are most interested in the coarser dust particles in this study, these finely sized contaminants should not impact our analysis. Therefore, profiles over the Canary Islands and during AER-D are analysed at their minimum sampling altitude (∼ 16 m or during landing at Fuerteventura Airport). Finally, filtering of the data removed noise based on a signal-to-noise ratio as a function of diameter.
2.1.2 DLR Falcon aircraft setup
The SALTRACE campaign took place in June and July 2013, conducting flights in the eastern Atlantic in the Cabo Verde region (SALTRACE-E) and in the western Atlantic around the Caribbean (SALTRACE-W) (Fig. 1 and Table 4).
Table 4Details of the SALTRACE flights, including the location and the time (UTC) of the flights. ES: Spain, CV: Cabo Verde, SN: Senegal, PR: Puerto Rico. The number of horizontal segments and vertical profiles measured during each flight are shown; each horizontal segment is measured at over 150 s. Data taken from the supplement of Weinzierl et al. (2017).
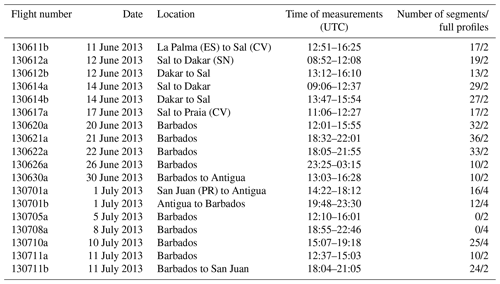
During SALTRACE, the DLR Falcon took measurements using a combination of OPCs: GRIMM Sky OPC (SkyOPC), Ultra-High Sensitivity Aerosol Spectrometer (UHSAS-A), and the Cloud and Aerosol Spectrometer with Depolarization Detection (CAS-DPOL). Some details of these instruments are shown in Table 3. Full details can be found in Walser (2017) and the supplement of Weinzierl et al. (2017).
We use data from both vertical profiles and horizontal segments in our analysis of the SALTRACE data. SALTRACE data from horizontal flight legs are broken down into 330 flight segments, each lasting for 150 s. These have been inverted and represented using lognormal modes in order to consistently propagate measurement uncertainties (e.g. optical particle counter response and properties, correction for refractive index) (Walser, 2017). These horizontal segments provide size distributions at a high resolution in diameter space. Additionally, in order to provide a vertically continuous description of dust mass and size variation with altitude, we use SALTRACE profile observations. The profile data have not undergone such extensive processing as the horizontal segments, and instead adjustments to the instrument bin sizes were applied to account for refractive index. Comparisons between the detailed size distributions from horizontal segments and those from profiles show good agreement (not shown). This allowed 44 size-resolved vertical profiles from SALTRACE to be analysed.
In order to calculate AOD, retrieved mass concentration profiles calculated from size distributions were combined with a mass extinction efficiency determined from an optical model (Gasteiger and Wiegner, 2018). This produced profiles of the extinction coefficient which were vertically integrated to provide AOD at 500 nm. See Wieland et al. (2024) for details. The SALTRACE AODs therefore represent the full size range in contrast to those which use the FAAM data.
Atmospheric concentrations of coarse and super-coarse particles during the airborne measurements of the presented mean vertical mass concentration profiles were often near to or below the detection limit of the CAS-DPOL. Hence, the mean mass concentrations should be considered a lower threshold.
2.1.3 Processing of aircraft data
For all campaigns, profile data were aggregated across instrument size bins to match the broader six size bins of the model (Table 5), assuming homogeneous distributions across instrument size bins. For example, for Fennec Sahara, model size bin 1 is compared against corresponding data at sizes measured by the PCASP (0.0632 0.2 µm), while model size bin 6 (20 63.2 µm) is compared against data from the CIP15. Where model and instrument size bins did not match up perfectly, the number concentration was proportioned across instrumental size bins. For example, for SALTRACE, model size bin 4 (2 6.32 µm) is compared against concentrations measured by the CAS-DPOL over instrumental size bins 11 to 15 as well as half of the number concentration from bin 10 (see the Supplement for full details; Table S1). This provides measured number concentrations corresponding to each model size bin as a function of time for the aircraft data. Assuming the density of dust to be 2.65 g cm−3 (Hess et al., 1998) and that the particles are spherical, we calculate mass concentrations for each of these size bins using standard volumetric and mass equations, based on the instrumental mid-bin diameter. These size- and time-resolved mass concentrations can then be manipulated as follows to provide mass concentration profiles and size distributions.
Table 5Size range and representative diameter (Drep) of the modelled transported mineral dust size bins in the CLASSIC aerosol scheme described in Woodward (2001). Drep is used in calculating the emitted size distribution and the particle settling velocity. Within each size bin, is assumed constant, where V is the particle volume and r is the particle radius.
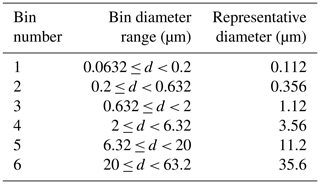
Profiles are measured as either one single “deep” profile or several smaller profile segments combined together. Quasi-vertical profile data are averaged over 50 m intervals for high-resolution analysis and model evaluation, for both FAAM and DLR measurements. For size distribution analysis, FAAM (i.e. Fennec, AER-D) aircraft profiles were averaged over 500 m altitude intervals. DLR (i.e. SALTRACE) size distributions were taken from horizontal flight segments, and measurements performed within 500 m altitude bands were averaged. The data are regionally averaged for each campaign. In some portions of our analysis, we do not analyse data below 1 km or above 6 km in order to avoid the observed data becoming skewed by non-dust particles in the MBL or at the top of/above the SAL.
A caveat of our analysis is that this removes any measured particles outside the model limits (0.063 63.2 µm). Particles larger than 63.2 µm accounted for 10 %–40 % of the total dust mass measured in the Sahara below 5 km, but in the Canaries and Cabo Verde, these particles accounted for less than 10 % of the dust mass and only occurred below 2 km (not shown). Hence, these giant particles were not included in this study as we focus our comparison on the size range transported in the model's atmosphere. Particularly over the Sahara, giant dust particles are likely to be omitted by model simulations, and the extent of this should be addressed in the future but is not in the scope of this study.
2.2 Model setup
The GA7.1 atmosphere-only version of the Hadley Centre Global Environment Model 3 (HadGEM3-GA7.1) (Walters et al., 2019) configuration of the MetUM is used to model, among other variables, global mineral dust concentrations and aerosol optical depths. This setup is identical to those used in the HadGEM3 CMIP6 (Coupled Model Intercomparison Project Phase 6) AMIP (Atmospheric Model Intercomparison Project) simulations and configured to use observed sea surface temperatures (SSTs) and CMIP6 historical inventories (Eyring et al., 2016). The model has a horizontal grid resolution of 1.875° × 1.25° (N96) and 85 height levels, 50 of which are concentrated below 18 km. The finest vertical resolution is the lowest layer, with a depth (dZ) of 36 m. dZ increases with altitude so that at ∼ 500 m altitude, dZ is 120 m; at ∼ 2 km altitude, dZ is 226 m; and at ∼ 5 km altitude, dZ is 373 m. The relatively high vertical resolution suggests that sensitivity to vertical numerical diffusion is unlikely to be important, though this may have a small effect (Zhuang et al., 2018). Mineral dust is represented by the Coupled Large-scale Aerosol Simulator for Studies in Climate (CLASSIC) scheme, described in Woodward et al. (2022) and Woodward (2001). The CLASSIC dust emission scheme calculates horizontal flux in nine size bins of between 0.0632 and 2000 µm diameter and uses this to derive vertical flux in six size bins up to 63.2 µm. Dust emissions are calculated interactively at each time step from modelled fields of friction velocity, soil moisture and the soil particle size distribution together with the model's land surface and vegetation data. A fraction of the coarsest particles are re-deposited to the surface within the same time step as they are emitted, and these never enter the model atmosphere. The remaining particles are lofted into the atmosphere and are transported as independent tracers corresponding to the six size bins shown in Table 5. The dust scheme is called at every model time step, using the driving fields calculated directly from HadGEM3-GA7.1 and the Joint UK Land Environment Simulator (JULES) (Woodward et al., 2022). The dust is mixed externally with other aerosols, which are simulated by the United Kingdom Chemistry and Aerosols (UKCA) GLobal Model of Aerosol Processes (GLOMAP-mode) scheme (Bellouin et al., 2013). The dust cannot act as cloud condensation nuclei or ice-nucleating particles or chemically interact with the model. The dust interacts with the rest of the model through radiative interactions with the atmosphere and ocean biogeochemistry via the Model of Ecosystem Dynamics, nutrient Utilisation, Sequestration and Acidification (MEDUSA). The dust particles are also assumed to be spherical.
The dust emission scheme is described in detail in Woodward et al. (2022). The method of calculating horizontal (G) and vertical flux (F) is derived from the work of Marticorena and Bergametti (1995), using dry threshold friction velocities () from Bagnold (1941) with a correction for soil moisture, based on the method of Fécan et al. (1999), and the clay fraction (Fc). Measurements from Gillette (1979) are used to relate G and F by assuming a clay content of less than 20 %. G is calculated in each of the nine size bins i, representing the horizontal flux:
where ρ is the air density, B is the bare soil fraction in the grid box, U* is the surface layer friction velocity, Mi is the ratio of dust mass in the size division i to the total mass, C is a constant of proportionality, D is a dimensionless tunable parameter and g is the acceleration due to gravity. The ratio of U* to and M combine to calculate the emitted size distribution, with being dependent on particle size using Drep values from Table 5. M is calculated from the soil clay, silt and sand fractions. The total vertical flux (F) is represented with six size bins. The mass in each is related to the total horizontal flux across all nine size bins (Woodward et al., 2022) according to
The particles are then transported as six independent tracers and are subject to deposition by below-cloud scavenging, gravitational settling and turbulent mixing in the boundary layer (BL). The impact of gravitational settling on the distribution of dust mass is calculated by computing the flux of dust out of a given layer and down to up to two model levels below (determined partly by the vertical spacing of the model levels), in proportion to the Stokes velocity and the length of the time step. The sensitivity of model results to the precise numerics has not been tested. Dry deposition in the BL is calculated using a resistance analogue method where the particle deposition velocity is treated as an inverse resistance based on gravitational settling and turbulent mixing (Seinfeld, 1986).
The model dust emissions are tuned to improve agreement between the simulation and observations of AOD, near-surface concentrations and deposition rates. To do this, three dimensionless parameters are altered: a global emission multiplier, a friction velocity multiplier and a soil moisture multiplier. The purpose of tuning is to correct for the effects of processes not included in the model, such as the gustiness of wind at the source and the relationship of soil moisture in the model's top level and at the soil surface (Woodward et al., 2022). The dust was not specifically tuned for this study, and an improved dust simulation would almost certainly be achievable if tuning were undertaken. However, we chose to use this configuration of settings as it is the same as those used in the HadGEM3 CMIP6 AMIP simulations (Eyring et al., 2016) and has been widely used.
The model is free-running but uses observed SSTs to simulate 5 June months, 2010–2014, outputting vertically resolved daily mean dust mass mixing ratios for each size bin. The averaged 5 June months provide a “June climatology” which is used to compare with our campaign averages. As the model is free-running, it does not represent specific meteorology and dust events, and therefore we cannot compare the specific dates on which the measurements were taken. We found minimal variability in Moderate Resolution Imaging Spectroradiometer (MODIS) Terra AOD in the two adjacent 5-year periods (2005–2009 and 2015–2019), suggesting that this 5-year period captures relatively average conditions and is of a sufficient length for this study. The data are averaged over boxes representative of the campaign locations (Fig. 1). Careful consideration was taken to make sure that the boxes were suitably located so as to represent the locations measured during the observations. The Sahara, Canaries and Cabo Verde boxes do not overlap with the African coast as this was found to alter the distribution and magnitude of the vertical dust profile.
The daily mean dust mixing ratio, temperature and pressure at model levels are used to calculate the air density and the mass, number, volume and surface area concentration per size bin. The calculations of the size distributions and normalisations were carried out in the same way as with the aircraft data. The model data are not averaged in the vertical.
As shown in Tables 1, 2 and 4, the aircraft campaigns cover limited periods of time, often only taking measurements for 2 to 3 weeks. The data collected during these campaigns can be biased towards certain types of events; for example, an effort may be made to schedule and direct flights through forecasted high-concentration dust events. Assuming that there may have been a scheduling bias towards high-concentration dust events during the campaigns, it is important to understand to what extent the dust size distribution, especially at the coarser size range, is dependent on the AOD, which we are using to represent the magnitude of the dust event. In this section, we show that any bias in data collection is unlikely to impact the findings from this study.
3.1 Spatial AOD comparisons
In order to ascertain whether the dust conditions measured during the campaigns are representative of average conditions, combined MODIS Dark Target and Deep Blue AOD retrievals for land and ocean (Levy et al., 2013; Hsu et al., 2013) from the Terra satellite are used. A monthly mean AOD at 550 nm during the campaigns (June 2011 for Fennec, June 2013 for SALTRACE and August 2015 for AER-D) at the campaign locations (i.e. regional boxes shown in Fig. 1) was compared to a 5-year (2010–2014) and 20-year (2000–2019) average of AOD in June (or August for AER-D). During the Fennec campaign in June 2011, the variability in the AOD in the Sahara was comparable to the longer-term June averages, whereas in the Canaries, the AOD during the Fennec campaign in June 2011 was greater (AOD between 0.4 and 0.6) than the 5- and 20-year averages (0.2–0.4), seemingly due to a slightly more northwestwards transport of dust during June 2011. In Cabo Verde during the AER-D campaign, the mean August AOD was comparable to the longer-term August averages. However, in June 2013, during the SALTRACE campaign, the AOD in Cabo Verde and the Caribbean was greater (0.5–1.0 and 0.3–0.6, respectively) than the longer averaging periods (0.5–0.6 and 0.3–0.4, respectively). This suggests that the campaigns observed conditions similar to (Fennec Sahara and AER-D) or dustier than average (Fennec SAL, SALTRACE-E and SALTRACE-W). Next, we analyse whether greater AOD impacts the shape of the measured coarse size distribution.
3.2 Relationship between AOD and size distribution
As AOD is the vertical integral of extinction caused by aerosols, which partially depends on the number concentration, as well as size-varying optical properties, we expect a greater concentration of dust to coincide with a higher AOD value. We aim to test this hypothesis with our observational data, and, additionally, we want to understand the dependence of coarse-particle size distribution on AOD: do high-AOD events contain a different proportion of particles from the coarser size bins 5 and 6 (6.32–63.2 µm) than low-AOD events?
Here, we show the impact of AOD on the size distribution by splitting campaign flights into low-, medium- and high-magnitude AOD events based on in situ AOD measurements taken during the Fennec (Ryder et al., 2015) AER-D and SALTRACE campaigns. The minimum, maximum and mean AOD from the AER-D campaign profiles was 0.06, 0.92 and 0.42, respectively. The AOD thresholds used to split up each campaign is given in the Table 6 caption; these thresholds were chosen as they approximately split the number of profiles from each campaign into thirds and are different for each campaign. We use Student's t test to test the statistical significance of our proposed hypotheses. The smaller the returned p value, the greater the statistical significance of the observed difference. We propose that the null hypothesis states that there is no difference between the total dust mass concentration profile in low- (L), medium- (M) and high-AOD (H) events. We found a statistically significant difference (at the 95 % confidence interval) between the total dust mass concentration and the AOD measured in the Sahara, the Canaries and Cabo Verde during L, M and H events (Table 6 indicated by small p values) in most cases; hence, we reject our null hypothesis. Thus, low-AOD events measured during the two campaigns, for example, had a significantly different concentration profile for medium- or high-AOD events.
Table 6The p values resulting from Student's t test to test the null hypothesis: there is no difference between the total mass concentration profile in low- (L), medium- (M) and high-AOD (H) events. Bold values are significant to a 95 % confidence interval. This is tested for the Fennec (Sahara and Canaries) and the AER-D (Cabo Verde) campaign data. Each set of aircraft profiles from each location was split into thirds based on AOD at 550 nm measurements from the aircraft. The thresholds separating categories of low to medium and medium to high AOD in the Sahara, Canaries and Cabo Verde are 0.75 and 1.5, 0.5 and 0.75, and 0.4 and 0.6, respectively.
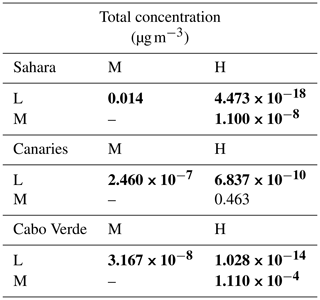
Next, we look at the relationship between the AOD and the relative mass contribution of coarse particles to the total mass concentration at each location. It is difficult to determine the relationship between AOD and the size distribution in observations because these measurements often characterise a different subset of the full dust size range, but even qualitative insights are worthwhile. Figure 2 shows AOD as a function of the mass contribution of size bin 6 for the Fennec, AER-D and SALTRACE campaigns (see the Supplement for the equivalent figure of size bin 5). AOD is calculated differently between the Fennec and AER-D and SALTRACE campaigns due to different instrumentation, but no campaign individually shows a strong correlation between the coarse mass contribution and AOD. Combining campaign data, there is a suggestion of a correlation between the AOD and coarse mass contribution, whereby coarse contribution may increase with AOD, which is to be expected in some cases as a result of different transport distances for each campaign region. So, a model bias in AOD is unlikely to be a dominant cause for simulating too few or too many coarse particles. The next section investigates the difference in the size distribution further to identify additional causes.
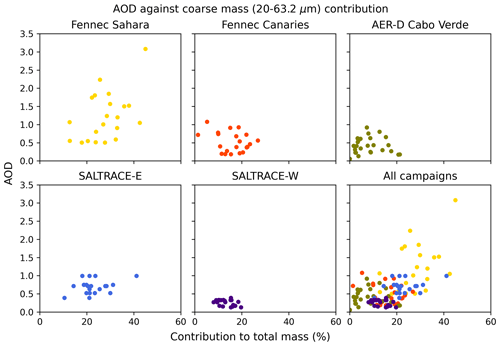
Figure 2AOD against coarse mass (20–63.2 µm; size bin 6) contribution to total mass in each campaign. For Fennec and AER-D, AODs represent particles with diameters below 3 µm and mass contribution was averaged over profiles between 1–5 km in the Sahara, 0–5.5 km in the Canaries and 0–5 km in Cabo Verde. For SALTRACE, AOD represents the full size range and mass contribution is taken from horizontal segments.
In this section, the observations at the four observed locations (Sahara, Canaries, Cabo Verde and Caribbean) will be compared to the model simulation. Initially, this comparison will investigate the specifics of the vertical structure of the dust layer before focusing on the evolution of the observed and modelled size distributions over long-range transport.
4.1 Vertical structure
In terms of the absolute values, we have analysed the mean total mass concentration profile from each location for between 0.063 and 63.2 µm diameter to match the modelled size range and between 1 and 6 km altitude to avoid contamination from the MBL or above the SAL. The mean mass concentration from observations between 1 and 6 km from each set of profiles has been calculated: 341 µg m−3 in the Sahara, 162 µg m−3 in the Canaries, 161 and 1680 µg m−3 in Cabo Verde (AER-D and SALTRACE-E, respectively), and 340 µg m−3 in the Caribbean. Despite the expectation that the highest mean concentration would be measured in the Sahara, the SALTRACE-E mean is almost 5 times larger, while the SALTRACE-W mean is nearly as large as that measured in the Sahara. This suggests that the events measured during the SALTRACE campaign were significantly larger than those measured during Fennec and AER-D. Despite these campaigns covering a range of magnitudes, the model tends to underestimate the mean total dust mass by a factor of between 4 and 44 (not shown), with the largest underestimations occurring with the comparison to the SALTRACE-E data. It is likely that this underestimation is partly due to a bias in the model size distribution towards smaller particles which constitute less mass. This underestimation is also likely a consequence of the tuning which has been applied to the model emissions as well as the different temporal scales which we are comparing. Due to the large magnitude of difference between the model and campaigns, the vertical mass profiles have been normalised. In order to compare the vertical distribution of dust, the profiles have been normalised by the mean dust mass concentration between 1 and 6 km altitude.
Figure 3 shows the normalised observed and modelled vertical profiles of the total dust mass concentration at each location from each campaign. Firstly, in terms of the observations, in the Sahara (Fig. 3a), dust mass is highest near to the surface, likely due to the high quantity of coarse and super-coarse particles which are lofted and settle relatively close to the source. The mass concentration gradually decreases to nearly zero at 5.5 km, marking the top of the Saharan atmospheric boundary layer (SABL) – a well-mixed, dry layer over the Sahara extending from the surface, often up to ∼ 6 km over the Sahara (Cuesta et al., 2009). In the Canaries (Fig. 3b), the observations start to show the formation of the SAL – the dry, dusty air layer formed when the SABL rises isentropically over the Atlantic Ocean's MBL (Carlson, 2016), residing between ∼ 1 and 6 km – with higher concentrations of dust between 2.5 and 3.5 km altitude, though the profile has relatively high concentrations up to 5.5 km where it is capped at the top of the SAL. With more time and distance from the Sahara, profiles in Cabo Verde (Fig. 3c) represent a more mature version of the SAL; the AER-D profile has a more well-defined base and cap to the SAL with a more concentrated centre between 2 and 4 km. Though not as dramatic as the AER-D profile, the SALTRACE-E profile still peaks between 2 and 4.5 km and tails off both at the top and bottom ends of the profile. Finally, in the Caribbean (Fig. 3d), the dust plume has lowered, bringing the dust mass closer to the surface and lowering the SAL cap to below 5 km.
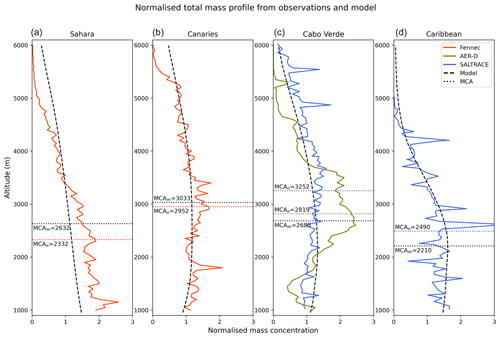
Figure 3Normalised observed (coloured solid line) and modelled (dashed black line) total dust mass concentration profile and dust mass centroid altitude (MCA; dotted horizontal lines in metres) between 1 and 6 km. MCAo and MCAm, respectively, represent the observed and modelled MCA values. Plots show all four observed locations: Sahara (a), Canaries (b), Cabo Verde (c; AER-D and SALTRACE-E) and Caribbean (d; SALTRACE-W) from the Fennec (orange), AER-D (green) and SALTRACE (blue) campaigns. Data have been normalised by the mean profile concentration between 1 and 6 km altitude.
Generally, the shape of the modelled vertical profile resembles the observed profile. However, the model has struggled to represent the rate of change in concentration with height, failing to capture the relative magnitude of the maximum and minimum values measured during Fennec and AER-D (Fig. 3a, b and c). In the Sahara, the model represents a more well-mixed profile whereby the concentration decreases more gradually with altitude than in the observations. The model does not have the same sharp cap at the top of the SABL that we see in the observations. Although the model does not represent the greater mid-SAL concentrations measured in AER-D well in Cabo Verde, its vertical distribution lies fairly close to that from SALTRACE-E (Fig. 3c).
The model appears to represent the top of the SAL most effectively in the Caribbean as the only location where the modelled concentration drops close to zero at the observed SAL top. The model failing to capture this sharp decrease could be in part due to our temporal averaging of the model data, suggesting that the top of the modelled SAL could vary significantly and can occur above 6 km altitude, except for in the Caribbean. The smooth profiles could also be a consequence of limited spatial resolution and numerical diffusion in the model.
The model represents the shape of the observed profiles very well despite the campaigns measuring fairly different total mass concentrations. However, although the AER-D campaign measured mean mass concentrations in Cabo Verde similar to those in the Canaries during Fennec, the AER-D profile is the least well-fitted to the model profiles, as well as appearing fairly different in structure to the SALTRACE-E profile. This difference could be caused by variation in the location of dust emission, which may alter the dust size distribution and distance transported before measurement. The difference could also be a consequence of the different time of year in which the AER-D campaign took place; Fennec and SALTRACE both occurred in June, whereas AER-D happened during August. The time of year impacts the location of the Inter-Tropical Convergence Zone (ITCZ) and the strength of the Saharan heat low (SHL), which work together as the main cause of intense dust uplift in the early summer (Marsham et al., 2008). The difference in meteorology could be why we see a different profile structure measured during the AER-D campaign.
The dust mass centroid altitude (MCA) between 1 and 6 km – the altitude at which 50 % of the mass is below and 50 % is above (Lu et al., 2023) – is shown in Fig. 3. We have not included particles in the lowest 1 km of the atmosphere in our calculations of the MCA due to potential interference from non-dust particles measured in the observations which may lower the MCA. Hence, this value is not a total column mass but is representative of the dust mass between 1 and 6 km at each location. At every location, the modelled MCA is at an altitude similar to the observed MCA, suggesting that the model distributes the total dust mass well in the SAL when compared to observations, in terms of the vertical distribution.
Moving away from the Sahara where the observed MCA is 2332 m, the MCA rises as the dust mass travels to the Canaries and Cabo Verde in the observations. The formation of the MBL aids in the removal of dust mass from the base of the SAL, causing the MCA to rise: 2952 m in the Canaries and 2819 and 3252 m in Cabo Verde. Though, as the plume sinks over the western Atlantic, the MCA reduces to 2490 m in the Caribbean. This raising and lowering of the MCA across the Atlantic is exactly what we would expect to see in our observations (e.g. Carlson, 2016). The model succeeds in representing vertical change in the MCA across the Atlantic. We have shown that the model represents the total dust mass vertical distribution fairly well. O'Sullivan et al. (2020) previously found that an NWP GA6.1 configuration of the MetUM placed dust 0.5–2.5 km too low in the atmosphere when compared with observations. Our analysis of these profiles suggests that this MetUM climate configuration may transport the dust at altitudes and distributions similar to the observations, at least in terms of the total mass across the whole size distribution.
In order to analyse the size distribution that makes up the vertical structure at these locations, we have broken the profiles (shown in their normalised form in Fig. 3) down into the six size bins used by the CLASSIC scheme in HadGEM3-GA7.1. We analyse the percentage contribution of mass to the total mass as a function of size. Figure 4 shows the contribution by size bin and the mean total mass concentration from each campaign for both the model and observations. Table 7 contains the mean percentage mass contribution to the total mass between 2 and 3.7 km altitude from the three coarsest size bins (2–6.32, 6.32–20 and 20–63.2 µm; green, blue and purple in Fig. 4) at each location from the observations and model.
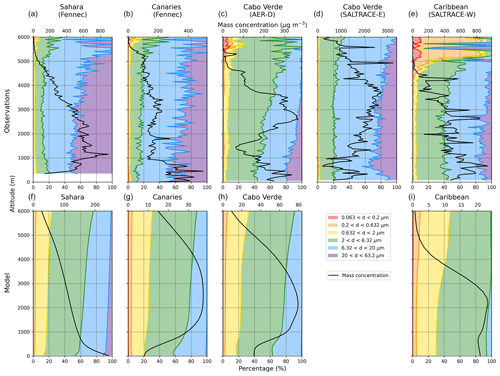
Figure 4Dust mass concentration profiles, showing the total dust mass concentration (in µg m−3; black line) and the percentage contribution of dust mass in the six model size bins (coloured areas). Plots include the mean profiles from the observations (a, b, c, d, e) and from the model (f, g, h, i) in the Sahara (Fennec; a, f), the Canaries (Fennec; b, g), Cabo Verde (AER-D and SALTRACE-E; c, d, h) and the Caribbean (SALTRACE-W; e, i). Note that the mass concentration (black line) scales differ between panels.
Table 7Mean percentage mass contribution to total mass between 2 and 3.7 km altitude from the three largest model size bins (2–6.32, 6.32–20 and 20–63.2 µm; green, blue and purple in Fig. 4) in the Sahara, the Canaries, Cabo Verde (AER-D, A; SALTRACE-E, S) and the Caribbean in the observations (Obs) and model. Data relate to Fig. 4.
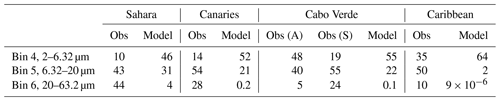
In the Sahara, up to 90 % of the observed dust mass up to 5 km comes from particles 6.32–63.2 µm in diameter (size bins 5 and 6; blue and purple in Fig. 4a). As the dust moves westwards over the Atlantic, the contribution of these coarsest particles decreases as they are deposited from the dust plume. Between 2 and 3.7 km, the 6.32–63.2 µm contribution decreases from ∼ 87 % in the Sahara to ∼ 82 % in the Canaries, ∼ 45 %–79 % in Cabo Verde and ∼ 60 % in the Caribbean (Table 7). As the coarser contribution decreases, the contribution of 2–6.32 µm particles (size bin 4; green) increases, while the contribution of the finest particles (0.063–2 µm; size bins 1–3; red, orange and yellow) remains low up to the top of the SAL, at less than 5 % at all locations except for Cabo Verde during AER-D (Fig. 4c). The coarse and super-coarse particles (6.32–63.2 µm; blue and purple) show a higher dependence on altitude in the AER-D data, whereby their mass contribution is highest in the lowest 1 km at up to 60 % and decreases with altitude to half this contribution at 5 km. Fewer coarser particles were measured during the AER-D campaign, resulting in a higher contribution of 2–6.32 µm particles (size bin 4; green) compared to the other campaigns. The SALTRACE-E profile (Fig. 4d) shows a structure similar to the Fennec observations, suggesting that the AER-D campaign is the more anomalous of the two datasets.
In general, the model overestimates the mass contribution from 0.063–6.32 µm dust particles (size bins 1–4; red, orange, yellow and green) and underestimates the 6.32–63.2 µm particle (size bins 5 and 6; blue and purple) contribution at all locations. In the Sahara, the modelled dust mass between 6.32 and 63.2 µm between 2 and 3.7 km accounts for 35 % of total mass, less than half of the observed contribution of ∼ 87 % (Fig. 4a and f). In the model, less than 15 % of the contribution at the surface is made up of the coarsest particles (20–63.2 µm; size bin 6; purple), decreasing to ∼ 4 % between 2 and 3.7 km altitude, which is 11 times less than the observed contribution. The mass contribution of 20–63.2 µm particles (size bin 6; purple) in the model decreases quickly beyond the Sahara to become negligible. In the Canaries and Cabo Verde, the vast majority of 20–63.2 µm particles have been removed between 2 and 3.7 km, leaving a contribution of less than 0.1 %–0.2 % from this size bin, 2 orders of magnitude less than the observed contribution measured during Fennec and SALTRACE (Fig. 4b, c, d, g and h; Table 7). Upon reaching the Caribbean, only a very small fraction of the mass comes from the 20–63.2 µm particles (size bin 6; purple), and the 6.32–20 µm (size bin 5; blue) contribution below 1 km is less than 10 % and only 2 % between 2 and 3.7 km. The rate at which the model loses coarse and super-coarse particles results in an increasing bias of particles smaller than 6 µm and thus an underestimation of the total dust mass remaining after long-range transport.
From the Sahara, the modelled contribution of particles smaller than 2 µm (size bins 1–3; red, orange and yellow) is overestimated by a factor of 10, with an overestimation of up to 13, 3–12 and 9 in the Canaries, Cabo Verde and the Caribbean, respectively. This overestimation of the fine-particle mass confirms that the model shows a bias towards fine particles over coarser particles.
In the two largest size bins, the model shows a decreasing percentage mass contribution with altitude (Fig. 4). In the Canaries, for example, the model 6.32–20 µm mass contribution (size bin 5; blue) drops from ∼ 30 % at 1 km to ∼ 15 % at 5 km, whereas, in the observations, only the coarsest size bin shows this altitude dependence, whereby the 20–63.2 µm particle contribution (size bin 6; purple) decreases with altitude from ∼ 50 % at 1 km to ∼ 30 % at 5 km in the Sahara and from ∼ 25 % at 1 km to ∼ 10 % at 4 km in the Caribbean. Alternatively, the 6.32–20 µm contribution (size bin 5; blue) remains more consistent with altitude in the observations or shows an increasing relative contribution due to the decreased contribution of the 20–63.2 µm contribution (size bin 6; purple). Thus, where the model shows an altitude dependence in the percentage mass contribution of the coarse and super-coarse dust, the observations show this dependence only visibly affects the super-coarse mass contribution (i.e. 20–63.2 µm; purple).
The model represents the relative mass contribution of coarse and super-coarse particles as relatively height dependent, decreasing with altitude. However, the observations show little variation in coarse dust with height and a decreasing super-coarse-dust contribution with height. The model fails to retain the super-coarse dust during trans-Atlantic transport and incorrectly represents the vertical distribution of coarse dust, with a bias towards lower altitudes.
4.2 Size distribution evolution
The height-resolved modelled and observed volume size distributions have been normalised by total volume (Fig. 5). This highlights the peak of the size distribution and the difference in shape between the model and observations when the total concentrations are different. There are two things which are clear amongst all campaigns. Firstly, this is the shape of the distributions from the smallest size bin to the peak in volume; the model displays a broader shape, while the observations show a more steeply curved, peaking shape. Secondly, the model underestimates volume in the largest size bin at all locations. Beginning in the Sahara, the difference is around 1 order of magnitude. Moving downwind, the difference between the model and observations continues to grow by orders of magnitude such that the model volume distribution drops much more sharply to around 5 orders of magnitude less than the observations in size bin 6 (20–63.2 µm) by the Caribbean. At all locations (except for Cabo Verde during AER-D) the observed volume in the 2–6.32 µm range is very similar in magnitude to the volume in the 20–63.2 µm range (i.e. size bins 4 and 6), whereas, in the model, there is a notable drop from the fourth to the sixth size bin. The increasing difference between the model and observations at the coarsest range is an indication of the rapid deposition of the coarser particles in the model. Not only do we see a growing difference with distance from the Sahara, but also the underestimation of coarser-dust volume in the Sahara suggests there may be an issue with the model emissions and/or vertical transport, whereby not enough coarse and super-coarse particles are transported through the SABL. This underestimation is exacerbated through long-range transport by the overly swift deposition of the coarser particles.
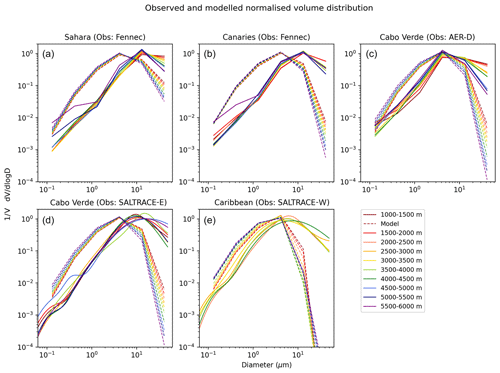
Figure 5Vertically resolved modelled (dashed lines) and observed (solid lines) normalised volume distribution in the Sahara, the Canaries (Fennec), Cabo Verde (AER-D and SALTRACE-E) and the Caribbean (SALTRACE-W) at different altitudes. The volume distributions have been normalised by the total particle volume.
The model tends to peak in volume in the 2–6.32 µm bin, whereas the observations measured during the Fennec and SALTRACE campaigns peak in the next size bin up (6.32–20 µm). Contrary to the other campaigns, the volume distribution from the AER-D campaign in Cabo Verde peaks in the 2–6.32 µm bin. As mentioned previously when observing the different vertical structure, this difference could be a consequence of the different time of year in which this campaign occurred. Despite the differences between the data collected from the AER-D campaign and the Fennec and SALTRACE campaigns, the AER-D data remain consistent with the other campaigns, showing that the model underestimates coarser-dust-particle mass and transport.
When normalised, there is no particular pattern in relation to the altitude except for at the coarsest size ranges, where dust volume tends to decrease with altitude in the model and observations. Otherwise, the shape of the distribution remains consistent with altitude. The only other exception is at high altitudes in the smallest size bin in the observations in the Sahara, the Canaries and Cabo Verde (Fig. 5a, b and d). The volume distribution of fine 0.063–0.632 µm particles is greater above 5 km than at lower altitudes. This could be a signal of non-dust particles.
Figures 4 and 5 show that the model under-represents the coarser size distribution over the Sahara, as well as further downwind during transport. In this study, we focus on the impact of transport processes on the size distribution, rather than examining emission processes. The model emitted size distribution is dominated by size bin 6, although the atmospheric dust size distribution in the lowest model level is already dominated by size bin 4 (see Fig. S1). This suggests additional challenges in representing initial dust transport from emission into the very low atmosphere, which should be an area for future study. The aggregated Saharan observations presented here are from 500 m upwards, which prevents a detailed analysis of the near-surface emission size distribution.
In order to illustrate how the model represents the evolution of the dust size distribution during trans-Atlantic transport and the discrepancies between the model and observations over the Sahara, Fig. 6 shows the vertically resolved fractional model underestimate of the volume size distribution between the observations and the model in the Sahara, the Canaries, Cabo Verde and the Caribbean (i.e. observations model of ). This figure shows that at all locations, the model underestimates the coarse fractions by greater orders of magnitude than the fine fraction. Additionally and most significantly, the magnitude of the coarse-fraction underestimate grows with transport from the Sahara; the fractional underestimate of size bin 6 increases from around a factor of 10 over the Sahara to over 1 000 000 in the Caribbean. Thus, we demonstrate that although there is an underestimation of the volume distribution at the source, this is significantly exacerbated by several orders of magnitude with westwards transport.
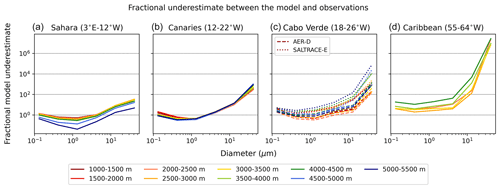
Figure 6Fractional underestimate of the volume size distribution between the observations and model. The vertically resolved difference is shown in the Sahara (a) and Canaries (b), using Fennec observational data; in Cabo Verde (c), using AER-D (dashed lines) and SALTRACE-E (dotted lines) data; and in the Caribbean (d), using SALTRACE-W data.
We have postulated previously that the model struggles to raise the coarse dust high enough, showing more altitudinal dependence than the observations. In Fig. 6c and d in Cabo Verde and the Caribbean, the model underestimate becomes worse at higher altitudes. In Cabo Verde in the SALTRACE-E comparison, there is an order-of-magnitude difference of the underestimate of size bin 6 between 1–1.5 and 5–5.5 km altitude.
While no observations of vertically resolved, size-resolved dust concentration over the mid-Atlantic exist, we are able to look at how the model simulates the concentration evolution across the Atlantic. Figure 7 shows the evolution of mass concentration in each size bin from the Sahara to the Caribbean. Figure 7a shows the modelled mean June AOD as well as stippling, which represents the 65th percentile of AOD between 1° S and 47° N at each degree longitude, which has been used to identify the mean latitudinal plume extent. The 65th percentile of AOD was chosen so as to cover an area including all the observed locations. Figure 7b shows the modelled mass concentration in each of the six size bins in the defined dust plume location and between the 2 and 3.7 km altitude range. The 2–3.7 km altitude range has been selected to analyse the dust plume to minimise interference from the MBL and free troposphere above the SAL, across the entire Atlantic. Figure 7b shows that the 2–6.32 µm particles (green) are the dominant contributors to dust mass across the Atlantic in the model, as in Fig. 4. Although the mass of 6.32–20 µm particles (blue) is double that of the 0.632–2 µm particles (red, orange and yellow) in the Sahara, these larger particles are removed more swiftly and have less mass than the finer particles west of Cabo Verde (∼ 25° W).
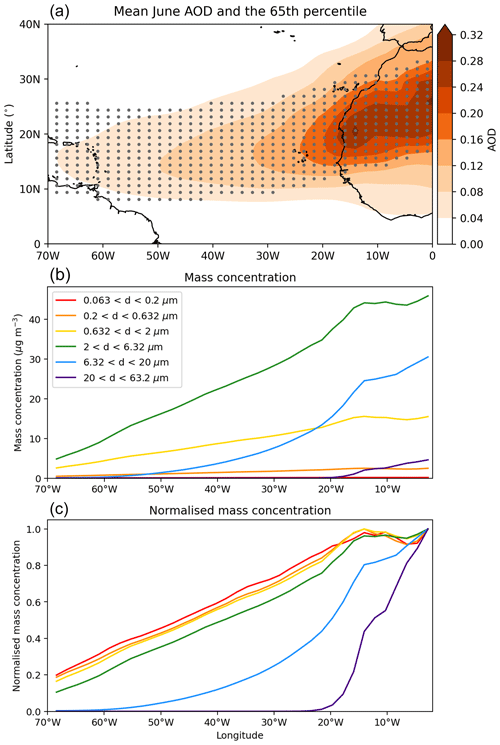
Figure 7The 65th percentile (stippling) of mean June 2010–2014 AOD at 550 nm (orange shading) at each longitude has been used to locate the dust plume (a). The mean modelled dust mass concentration between 2.0 and 3.7 km altitude by longitude in the six CLASSIC size bins from the Sahara to the Caribbean (b). The binned concentrations have been normalised by the mass concentration in each bin at 3° W (c).
Figure 7c shows the normalised mass concentration transect in the six size bins. These have been normalised by their value in the Sahara (∼ 3° W) to allow for a direct comparison of the rate of change in mass concentration between each size bin. All size bins experience change in their mass concentration in two distinct regions, one over the entire African continent (15–3° W) and over the Atlantic, where each size bin loses mass at a size-dependent rate. These two distinct areas hint at the different processes which alter dust transport over land and ocean. The rate of loss of the four finest size bins (0.063–6.32 µm; red–green) appears fairly linear; each size bin loses 70 %–80 % of its mass between the western African coast and the Caribbean. The rate of loss of the coarsest size bins (6.32–63.2 µm; blue and purple) is sharper. These much faster rates of loss result in a negligible mass of 20–63.2 µm particles remaining just west of Cabo Verde and of 6.32–20 µm particles remaining near the Caribbean.
Vertically resolved, in situ observations from three aircraft campaigns, Fennec, AER-D and SALTRACE, in the Sahara, the Canary Islands, Cabo Verde and the Caribbean are analysed together to understand the evolution of dust particle size distribution over long-range transport, with a particular focus on the coarser particles and their vertical distribution. The observations from these campaigns are used to evaluate the Met Office Unified Model (MetUM) HadGEM3-GA7.1 climate model representation of the dust size distribution across the Atlantic. This work presents the first time that all three of these campaigns have been used together and analysed at such a high vertical resolution in order to understand the size distribution evolution from the Sahara to the Caribbean, as well as being the most extensive evaluation of the MetUM HadGEM3-GA7.1 model representation of long-range dust size distribution evolution.
Aircraft observations from the Fennec, AER-D and SALTRACE campaigns show that coarser particles are further transported in the real world than in the model, in which coarser dust particles (6.32–63.2 µm) are underestimated in both mass and volume size distribution at all stages of long-range transport. In the Sahara, the model underestimates the normalised volume size distribution of the largest particles (20–63.2 µm) by more than 1 order of magnitude. The contribution of 20–63.2 µm particle mass to the total mass is only 4 % in the model and 44 % on average in the observations between 2 and 3.7 km over the Sahara, resulting in a model underestimation by a factor of 11. The particle mass contribution of size bin 5 is ∼ 43 % in the observations and only 31 % in the model. This results in a stark overestimation of the 2–6.32 µm mass contribution by 36 %. These underestimations of the coarser particles suggest a challenge in representing the immediate transport upwards through the atmosphere after emission.
Observations suggest that the contribution of coarse-particle mass to the total mass is not strongly correlated with AOD, at least within a given campaign. The use of campaign periods with slightly higher-than-average AOD could therefore contribute to the poor representation of coarse particles in the model but is not a dominant driver. The model underestimation of coarse-particle concentration is so large that AOD variations within a campaign alone are not sufficient to explain the differences between the model and observations. We find that the model underestimates the coarser-particle volume distribution by increasing orders of magnitude with distance from the Sahara. The normalised volume size distribution in the largest model size bin (20–63.2 µm) is underestimated by 1 order of magnitude over the Sahara, up to 3 orders of magnitude in the Canaries, 5 orders of magnitude in Cabo Verde and 7 orders of magnitude in the Caribbean. This increasing disparity between the model and observations is a consequence of the overly swift removal of coarse and super-coarse particles from the modelled atmosphere, which is marked over the Sahara and is exacerbated during long-range transport. The majority of 20–63.2 µm particles have been removed from the Saharan air layer (SAL) just west of Cabo Verde, contributing only 0.1 % of the total mass, where the observations show this size bin contributing up to 25 % of the total mass between 2 and 3.7 km. The model's fifth size bin (6.32–20 µm) shows a slightly slower rate of removal from the model; however this still leaves a negligible concentration in the Caribbean, where the mass contributed by this size bin to the total dust mass is underestimated by a factor of 25 between 2 and 3.7 km. We suggest that the model is simulating far too swift a deposition of particles sized larger than 6.32 µm during the full course of long-range transport, leading to an increasing underestimation of dust mass with distance from the Sahara.
We have shown that the model generally agrees with the vertical distribution of total dust mass in the observations. We show that the mass centroid altitude (MCA) in the model is consistently within range of the observations. However, we find an underestimation in the super-coarse volume size distribution which increases with altitude, showing that the model increasingly struggles with coarser-particle representation over long-range horizontal and vertical transport and, despite representing the MCA of total dust mass, transports coarser dust particles too low in the atmosphere.
Our results are subject to some limitations. Firstly, it is noted that the aircraft observations used in this study cannot be fully representative of climatic conditions due to limitations in the temporal and spatial coverage of the observations. This makes our comparisons to the model more complex as the model provides daily mean data, covering each full 24 h period. Additionally, we find that the AER-D data have a slightly different vertical distribution of dust compared to SALTRACE-E in Cabo Verde, as well as a finer size distribution in comparison to the Fennec and SALTRACE campaigns, despite having instrumentation consistent with that of the Fennec campaign. It is not exactly clear what causes this disparity, but it could be a consequence of the measurements being taken in August compared to the other campaigns which were conducted in June. Additionally, we note that we excluded observations for which d > 63.2 µm since this is the maximum size represented by the model which was significant in the Sahara observations. Finally, we must consider that any biases in the model's representation of the dust vertical and horizontal distribution, as well as the size distribution, could be due to either the dust scheme or biases in the modelled climate. There is the potential for future research into the sensitivity of coarser-particle transport in the model regarding the numerical schemes which could provide additional valuable information for this research topic.
We have shown that the model has difficulty with representing the coarse-dust size distribution from the Sahara to the western Atlantic. This is consistent with other studies which have evaluated a range of models on more restricted spatial and vertical scales (Adebiyi and Kok, 2020; Ansmann et al., 2017; O'Sullivan et al., 2020). Incorrect representations of dust size distributions in climate models will result in erroneous dust radiative effects, impacts on clouds, and deposition of nutrients within dust to the ocean and land surfaces (Adebiyi et al., 2023; Kok et al., 2017; Dansie et al., 2022). It is therefore important to understand and improve modelled dust size distributions. The discrepancy in the size distribution could be due to over-active processes affecting the dust deposition, such as sedimentation, wet deposition, and convective or turbulent mixing. It could also be a consequence of the dust not absorbing enough shortwave radiation (Colarco et al., 2014; Balkanski et al., 2021) and potentially affecting heating and therefore dust lofting after emission or plume height during transport. Alternatively, this long-range transport could be due to processes not considered in the model and not yet fully understood in practice, such as electric charging (van der Does et al., 2018; Toth et al., 2020), asphericity (Huang et al., 2020, 2021; Saxby et al., 2018), turbulence (Denjean et al., 2016; Cornwell et al., 2021) and vertical mixing (Gasteiger et al., 2017). There is a need to better understand and observe dust size distributions during emission and in the lowest layers of the atmosphere over source regions. Whilst model dust concentration and size distribution near sources could be improved by re-tuning the emission scheme, this is unlikely to affect the evolution of the size distribution with transport, where additional processes are necessary to retain coarser particles, and should be investigated in further research along with size-resolved dust emissions.
This study presents an in-depth analysis of the evolution of the vertically resolved dust size distribution from the Sahara to the Caribbean from aircraft observations and the Met Office Unified Model. We show that the model underestimates super-coarse particles over the Sahara compared to observations, a difference which is exacerbated by up to 5 orders of magnitude during trans-Atlantic transport. As the presence and relative fraction of coarse particles is important for, among other processes, Earth's radiative budget and ice nucleation, it is imperative for the scientific community to obtain a better understanding of the physical processes which could be better understood and/or improved for models to improve simulations of super-coarse-dust transport. The work presented here demonstrates the need for a thorough analysis of processes affecting dust transport and deposition across the Atlantic in both observations and modelling in order to fully constrain models and to accurately simulate dust size changes during long-range transport and the diverse impacts on weather, climate and socio-economics dependent on this.
Aircraft data for the Fennec and AER-D campaigns are available at the Centre for Environmental Data Archive on https://doi.org/10.5285/1f4555d2589841a8a4bbbf1fe42f54c8 (Facility for Airborne Atmospheric Measurements et al., 2018) and http://catalogue.ceda.ac.uk/uuid/d7e02c75191a4515a28a208c8a069e70 (Facility for Airborne Atmospheric Measurements and Blyth, 2015), respectively. Aircraft data for the SALTRACE campaigns are currently in the process having a DOI created. Data from the model simulation are available on https://doi.org/10.5281/zenodo.10722717 (Ratcliffe et al., 2024).
The supplement related to this article is available online at: https://doi.org/10.5194/acp-24-12161-2024-supplement.
NGR, CLR and NB designed the research. NGR carried out the analysis and wrote the paper. All authors discussed the methodology and results. BW, LMW, JG and MD provided the SALTRACE data. All authors read and commented on the paper.
The contact author has declared that none of the authors has any competing interests.
Publisher's note: Copernicus Publications remains neutral with regard to jurisdictional claims made in the text, published maps, institutional affiliations, or any other geographical representation in this paper. While Copernicus Publications makes every effort to include appropriate place names, the final responsibility lies with the authors.
Natalie G. Ratcliffe would like to acknowledge the funding and support given by CASE funding from the UK Met Office. Further detailed analysis of the SALTRACE data forms part of Lisa-Maria Wieland's PhD thesis. Lisa-Maria Wieland, Maximilian Dollner and Bernadett Weinzierl acknowledge funding through the University of Vienna and the Gottfried and Vera Weiss Award and the Austrian Science Fund (FWF) in the context of the PlasticSphere project (no. AP3641721). Natalie G. Ratcliffe acknowledges the use of the MONSooN system, a collaborative facility supplied under the Joint Weather and Climate Research Programme, a strategic partnership between the Met Office and the Natural Environment Research Council. The MODIS data in this study were acquired as part of NASA's Earth Science Enterprise. The algorithms were developed by the MODIS Science Team, and the data were processed by the MODIS Adaptive Processing System (MODAPS) and Goddard Distributed Active Archive Center (DAAC); they are archived and distributed by the Goddard DAAC.
This research has been supported by the Natural Environment Research Council (grant nos. NE/S007261/1 and NE/M018288/1).
This paper was edited by Annika Oertel and reviewed by two anonymous referees.
Adebiyi, A., Kok, J. F., Murray, B. J., Ryder, C. L., Stuut, J.-B. W., Kahn, R. A., Knippertz, P., Formenti, P., Mahowald, N. M., García-Pando, C. P., Klose, M., Ansmann, A., Samset, B. H., Ito, A., Balkanski, Y., Di Biagio, C., Romanias, M. N., Huang, Y., and Meng, J.: A review of coarse mineral dust in the Earth system, Aeolian Res., 60, 100849, https://doi.org/10.1016/j.aeolia.2022.100849, 2023. a, b, c
Adebiyi, A. A. and Kok, J. F.: Climate models miss most of the coarse dust in the atmosphere, Science Advances, 6, eaaz9507, https://doi.org/10.1126/sciadv.aaz9507, 2020. a, b, c, d
Ansmann, A., Rittmeister, F., Engelmann, R., Basart, S., Jorba, O., Spyrou, C., Remy, S., Skupin, A., Baars, H., Seifert, P., Senf, F., and Kanitz, T.: Profiling of Saharan dust from the Caribbean to western Africa – Part 2: Shipborne lidar measurements versus forecasts, Atmos. Chem. Phys., 17, 14987–15006, https://doi.org/10.5194/acp-17-14987-2017, 2017. a, b, c
Bagnold, R. A.: The Physics of Blown Sand and Desert Dunes, Metheun, New York, ISBN 978-94-009-5682-7, 1941. a
Baker, A. R., Jickells, T. D., Witt, M., and Linge, K. L.: Trends in the solubility of iron, aluminium, manganese and phosphorus in aerosol collected over the Atlantic Ocean, Mar. Chem., 98, 43–58, https://doi.org/10.1016/J.MARCHEM.2005.06.004, 2006. a
Balkanski, Y., Bonnet, R., Boucher, O., Checa-Garcia, R., and Servonnat, J.: Better representation of dust can improve climate models with too weak an African monsoon, Atmos. Chem. Phys., 21, 11423–11435, https://doi.org/10.5194/acp-21-11423-2021, 2021. a
Barkley, A. E., Olson, N. E., Prospero, J. M., Gatineau, A., Panechou, K., Maynard, N. G., Blackwelder, P., China, S., Ault, A. P., and Gaston, C. J.: Atmospheric Transport of North African Dust-Bearing Supermicron Freshwater Diatoms to South America: Implications for Iron Transport to the Equatorial North Atlantic Ocean, Geophys. Res. Lett., 48, e2020GL090476, https://doi.org/10.1029/2020GL090476, 2021. a
Bellouin, N., Mann, G. W., Woodhouse, M. T., Johnson, C., Carslaw, K. S., and Dalvi, M.: Impact of the modal aerosol scheme GLOMAP-mode on aerosol forcing in the Hadley Centre Global Environmental Model, Atmos. Chem. Phys., 13, 3027–3044, https://doi.org/10.5194/acp-13-3027-2013, 2013. a
Carlson, T. N.: The Saharan Elevated Mixed Layer and its Aerosol Optical Depth, The Open Atmospheric Science Journal, 10, 26–38, https://doi.org/10.2174/1874282301610010026, 2016. a, b
Colarco, P. R., Nowottnick, E. P., Randles, C. A., Yi, B., Yang, P., Kim, K. M., Smith, J. A., and Bardeen, C. G.: Impact of radiatively interactive dust aerosols in the NASA GEOS-5 climate model: Sensitivity to dust particle shape and refractive index, J. Geophys. Res., 119, 753–786, https://doi.org/10.1002/2013JD020046, 2014. a, b
Cornwell, G. C., Xiao, H., Berg, L. K., and Burrows, S. M.: Simulated dust transport in the convective boundary layer, J. Geophys. Res.-Atmos., 126, e2020JD033429, https://doi.org/10.1029/2020JD033429, 2021. a, b
Cuesta, J., Marsham, J. H., Parker, D. J., and Flamant, C.: Dynamical mechanisms controlling the vertical redistribution of dust and the thermodynamic structure of the West Saharan atmospheric boundary layer during summer, Atmos. Sci. Lett., 10, 34–42, https://doi.org/10.1002/asl.207, 2009. a
Dansie, A., Wiggs, G., and Thomas, D.: Iron and nutrient content of wind-erodible sediment in the ephemeral river valleys of Namibia, Geomorphology, 290, 335–346, https://doi.org/10.1016/j.geomorph.2017.03.016, 2017. a
Dansie, A., Thomas, D., Wiggs, G., Baddock, M., and Ashpole, I.: Plumes and blooms – Locally-sourced Fe-rich aeolian mineral dust drives phytoplankton growth off southwest Africa, Sci. Total Environ., 829, 154562, https://doi.org/10.1016/j.scitotenv.2022.154562, 2022. a, b
Denjean, C., Cassola, F., Mazzino, A., Triquet, S., Chevaillier, S., Grand, N., Bourrianne, T., Momboisse, G., Sellegri, K., Schwarzenbock, A., Freney, E., Mallet, M., and Formenti, P.: Size distribution and optical properties of mineral dust aerosols transported in the western Mediterranean, Atmos. Chem. Phys., 16, 1081–1104, https://doi.org/10.5194/acp-16-1081-2016, 2016. a, b, c
Drakaki, E., Amiridis, V., Tsekeri, A., Gkikas, A., Proestakis, E., Mallios, S., Solomos, S., Spyrou, C., Marinou, E., Ryder, C. L., Bouris, D., and Katsafados, P.: Modeling coarse and giant desert dust particles, Atmos. Chem. Phys., 22, 12727–12748, https://doi.org/10.5194/acp-22-12727-2022, 2022. a
Dumont, M., Tuzet, F., Gascoin, S., Picard, G., Kutuzov, S., Lafaysse, M., Cluzet, B., Nheili, R., and Painter, T. H.: Accelerated Snow Melt in the Russian Caucasus Mountains After the Saharan Dust Outbreak in March 2018, J. Geophys. Res.-Earth, 125, e2020JF005641, https://doi.org/10.1029/2020JF005641, 2020. a
Eyring, V., Bony, S., Meehl, G. A., Senior, C. A., Stevens, B., Stouffer, R. J., and Taylor, K. E.: Overview of the Coupled Model Intercomparison Project Phase 6 (CMIP6) experimental design and organization, Geosci. Model Dev., 9, 1937–1958, https://doi.org/10.5194/gmd-9-1937-2016, 2016. a, b
Facility for Airborne Atmospheric Measurements and Blyth, A.: UK ICE-D: atmospheric measurements dataset collection, Centre for Environmental Data Analysis [data set], http://catalogue.ceda.ac.uk/uuid/d7e02c75191a4515a28a208c8a069e70/, 2015. a
Facility for Airborne Atmospheric Measurements, Natural Environment Research Council, and Met Office: FAAM B606 FENNEC, LADUNEX and EUFAR flight: Airborne atmospheric measurements from core and non-core instrument suites on board the BAE-146 aircraft, NCAS British Atmospheric Data Centre [data set], https://doi.org/10.5285/1f4555d2589841a8a4bbbf1fe42f54c8, 2018. a
Fécan, F., Marticorena, B., and Bergametti, G.: Parametrization of the increase of the aeolian erosion threshold wind friction velocity due to soil moisture for arid and semi-arid areas, Ann. Geophys., 17, 149–157, https://doi.org/10.1007/s00585-999-0149-7, 1999. a
Foret, G., Bergametti, G., Dulac, F., and Menut, L.: An optimized particle size bin scheme for modeling mineral dust aerosol, J. Geophys. Res.-Atmos., 111, D17310, https://doi.org/10.1029/2005JD006797, 2006. a
Gasteiger, J. and Wiegner, M.: MOPSMAP v1.0: a versatile tool for the modeling of aerosol optical properties, Geosci. Model Dev., 11, 2739–2762, https://doi.org/10.5194/gmd-11-2739-2018, 2018. a
Gasteiger, J., Groß, S., Sauer, D., Haarig, M., Ansmann, A., and Weinzierl, B.: Particle settling and vertical mixing in the Saharan Air Layer as seen from an integrated model, lidar, and in situ perspective, Atmos. Chem. Phys., 17, 297–311, https://doi.org/10.5194/acp-17-297-2017, 2017. a, b
Gillette, D. A.: Environmental factors affecting dust emission by wind erosion, in: Saharan Dust, John Wiley, New York, ISBN 9780471996804, 1979. a
Heisel, M., Chen, B., Kok, J. F., and Chamecki, M.: Gentle Topography Increases Vertical Transport of Coarse Dust by Orders of Magnitude, J. Geophys. Res.-Atmos., 126, e2021JD034564, https://doi.org/10.1029/2021JD034564, 2021. a
Hess, M., Koepke, P., and Schult, I.: Optical Properties of Aerosols and Clouds: The Software Package OPAC, B. Am. Meteorol. Soc., 79, 831–844, https://doi.org/10.1175/1520-0477(1998)079<0831:OPOAAC>2.0.CO;2, 1998. a
Hoose, C. and Möhler, O.: Heterogeneous ice nucleation on atmospheric aerosols: a review of results from laboratory experiments, Atmos. Chem. Phys., 12, 9817–9854, https://doi.org/10.5194/acp-12-9817-2012, 2012. a
Hsu, N. C., Jeong, M. J., Bettenhausen, C., Sayer, A. M., Hansell, R., Seftor, C. S., Huang, J., and Tsay, S. C.: Enhanced Deep Blue aerosol retrieval algorithm: The second generation, J. Geophys. Res.-Atmos., 118, 9296–9315, https://doi.org/10.1002/jgrd.50712, 2013. a
Huang, Y., Kok, J. F., Kandler, K., Lindqvist, H., Nousianinen, T., Sakai, T., Adebiyi, A., and Jokinen, O.: Climate models and remote sensing retrievals neglect substantial desert dust asphericity, Geophys. Res. Lett., 47, e2019GL086592, https://doi.org/10.1029/2019GL086592, 2020. a, b, c
Huang, Y., Adebiyi, A. A., Formenti, P., and Kok, J. F.: Linking the Different Diameter Types of Aspherical Desert Dust Indicates That Models Underestimate Coarse Dust Emission, Geophys. Res. Lett., 48, e2020GL092054, https://doi.org/10.1029/2020GL092054, 2021. a, b, c
Huneeus, N., Schulz, M., Balkanski, Y., Griesfeller, J., Prospero, J., Kinne, S., Bauer, S., Boucher, O., Chin, M., Dentener, F., Diehl, T., Easter, R., Fillmore, D., Ghan, S., Ginoux, P., Grini, A., Horowitz, L., Koch, D., Krol, M. C., Landing, W., Liu, X., Mahowald, N., Miller, R., Morcrette, J.-J., Myhre, G., Penner, J., Perlwitz, J., Stier, P., Takemura, T., and Zender, C. S.: Global dust model intercomparison in AeroCom phase I, Atmos. Chem. Phys., 11, 7781–7816, https://doi.org/10.5194/acp-11-7781-2011, 2011. a, b
Jickells, T. D., An, Z. S., Andersen, K. K., Baker, A. R., Bergametti, G., Brooks, N., Cao, J. J., Boyd, P. W., Duce, R. A., Hunter, K. A., Kawahata, H., Kubilay, N., laRoche, J., Liss, P. S., Mahowald, N., Prospero, J. M., Ridgwell, A. J., Tegen, I., and Torres, R.: Global Iron Connections Between Desert Dust, Ocean Biogeochemistry, and Climate, Science, 308, 67–71, https://doi.org/10.1126/science.1105959, 2005. a
Jones, A. C., Hill, A., Hemmings, J., Lemaitre, P., Quérel, A., Ryder, C. L., and Woodward, S.: Below-cloud scavenging of aerosol by rain: a review of numerical modelling approaches and sensitivity simulations with mineral dust in the Met Office's Unified Model, Atmos. Chem. Phys., 22, 11381–11407, https://doi.org/10.5194/acp-22-11381-2022, 2022. a
Karydis, V. A., Kumar, P., Barahona, D., Sokolik, I. N., and Nenes, A.: On the effect of dust particles on global cloud condensation nuclei and cloud droplet number, J. Geophys. Res.-Atmos., 116, D23204, https://doi.org/10.1029/2011JD016283, 2011. a
Kok, J. F., Ridley, D. A., Miller, R. L., Zhao, C., Heald, C. L., Ward, D. S., Albani, S., and Haustein, K.: Smaller desert dust cooling effect estimated from analysis of dust size and abundance, Nat. Geosci., 10, 274–278, https://doi.org/10.1038/ngeo2912, 2017. a, b, c
Kok, J. F., Ward, D. S., Mahowald, N. M., and Evan, A. T.: Global and regional importance of the direct dust-climate feedback, Nat. Commun., 9, 241, https://doi.org/10.1038/s41467-017-02620-y, 2018. a, b
Kotsyfakis, M., Zarogiannis, S. G., and Patelarou, E.: The Health Impact of Saharan Dust Exposure, Int. J. Occup. Med. Env., 32, 749–760, https://doi.org/10.13075/ijomeh.1896.01466, 2019. a
Levy, R. C., Mattoo, S., Munchak, L. A., Remer, L. A., Sayer, A. M., Patadia, F., and Hsu, N. C.: The Collection 6 MODIS aerosol products over land and ocean, Atmos. Meas. Tech., 6, 2989–3034, https://doi.org/10.5194/amt-6-2989-2013, 2013. a
Lohmann, U. and Feichter, J.: Global indirect aerosol effects: a review, Atmos. Chem. Phys., 5, 715–737, https://doi.org/10.5194/acp-5-715-2005, 2005. a
Lu, Z., Wang, J., Chen, X., Zeng, J., Wang, Y., Xu, X., Christian, K. E., Yorks, J. E., Nowottnick, E. P., Reid, J. S., and Xian, P.: First Mapping of Monthly and Diurnal Climatology of Saharan Dust Layer Height Over the Atlantic Ocean From EPIC/DSCOVR in Deep Space, Geophys. Res. Lett., 50, e2022GL102552, https://doi.org/10.1029/2022GL102552, 2023. a
Mahowald, N., Albani, S., Kok, J. F., Engelstaeder, S., Scanza, R., Ward, D. S., and Flanner, M. G.: The size distribution of desert dust aerosols and its impact on the Earth system, Aeolian Res., 15, 53–71, https://doi.org/10.1016/j.aeolia.2013.09.002., 2014. a
Mallios, S. A., Drakaki, E., and Amiridis, V.: Effects of dust particle sphericity and orientation on their gravitational settling in the earth's atmosphere, J. Aerosol Sci., 150, 105634, https://doi.org/10.1016/j.jaerosci.2020.105634, 2020. a
Marsham, J. H., Parker, D. J., Grams, C. M., Taylor, C. M., and Haywood, J. M.: Uplift of Saharan dust south of the intertropical discontinuity, J. Geophys. Res.-Atmos., 113, D21102, https://doi.org/10.1029/2008JD009844, 2008. a
Marticorena, B. and Bergametti, G.: Modeling the atmospheric dust cycle: 1. Design of a soil-derived dust emission scheme, J. Geophys. Res., 100, 415–431, https://doi.org/10.1029/95JD00690, 1995. a
Meng, J., Huang, Y., Leung, D. M., Li, L., Adebiyi, A. A., Ryder, C. L., Mahowald, N. M., and Kok, J. F.: Improved Parameterization for the Size Distribution of Emitted Dust Aerosols Reduces Model Underestimation of Super Coarse Dust, Geophys. Res. Lett., 49, e2021GL097287, https://doi.org/10.1029/2021GL097287, 2022. a
Méndez Harper, J., Harvey, D., Huang, T., McGrath, J., Meer, D., and Burton, J. C.: The lifetime of charged dust in the atmosphere, PNAS Nexus, 1, pgac220, https://doi.org/10.1093/pnasnexus/pgac220, 2022. a
Nenes, A., Murray, B., and Bougiatioti, A.: Mineral Dust and its Microphysical Interactions with Clouds, in: Mineral Dust: A Key Player in the Earth System, Springer, Dordrecht, https://doi.org/10.1007/978-94-017-8978-3, 2014. a
Nickovic, S., Cvetkovic, B., Petković, S., Amiridis, V., Pejanović, G., Solomos, S., Marinou, E., and Nikolic, J.: Cloud icing by mineral dust and impacts to aviation safety, Scientific Reports, 11, 6411, https://doi.org/10.1038/s41598-021-85566-y, 2021. a
Nowottnick, E., Colarco, P., Ferrare, R., Chen, G., Ismail, S., Anderson, B., and Browell, E.: Online simulations of mineral dust aerosol distributions: Comparisons to NAMMA observations and sensitivity to dust emission parameterization, J. Geophys. Res.-Atmos., 115, D03202, https://doi.org/10.1029/2009JD012692, 2010. a
O'Sullivan, D., Marenco, F., Ryder, C. L., Pradhan, Y., Kipling, Z., Johnson, B., Benedetti, A., Brooks, M., McGill, M., Yorks, J., and Selmer, P.: Models transport Saharan dust too low in the atmosphere: a comparison of the MetUM and CAMS forecasts with observations, Atmos. Chem. Phys., 20, 12955–12982, https://doi.org/10.5194/acp-20-12955-2020, 2020. a, b, c, d, e
Painter, T. H., Barrett, A. P., Landry, C. C., Neff, J. C., Cassidy, M. P., Lawrence, C. R., McBride, K. E., and Farmer, G. L.: Impact of disturbed desert soils on duration of mountain snow cover, Geophys. Res. Lett., 34, L12502, https://doi.org/10.1029/2007GL030284, 2007. a
Piedra, P. G., Llanza, L. R., and Moosmüller, H.: Optical losses of photovoltaic modules due to mineral dust deposition: Experimental measurements and theoretical modeling, Sol. Energy, 164, 160–173, https://doi.org/10.1016/j.solener.2018.02.030, 2018. a
Price, H. C., Baustian, K. J., McQuaid, J. B., Blyth, A., Bower, K. N., Choularton, T., Cotton, R. J., Cui, Z., Field, P. R., Gallagher, M., Hawker, R., Merrington, A., Miltenberger, A., Neely III, R. R., Parker, S. T., Rosenberg, P. D., Taylor, J. W., Trembath, J., Vergara-Temprado, J., Whale, T. F., Wilson, T. W., Young, G., and Murray, B. J.: Atmospheric Ice-Nucleating Particles in the Dusty Tropical Atlantic, J. Geophys. Res.-Atmos., 123, 2175–2193, https://doi.org/10.1002/2017JD027560, 2018. a
Prospero, J. M., Barkley, A. E., Gaston, C. J., Gatineau, A., y Sansano, A. C., and Panechou, K.: Characterizing and Quantifying African Dust Transport and Deposition to South America: Implications for the Phosphorus Budget in the Amazon Basin, Global Biogeochem. Cy., 34, e2020GB006536, https://doi.org/10.1029/2020GB006536, 2020. a
Pruppacher, H. and Klett, J.: Microphysics of Clouds and Precipitation, Springer, Dordrecht, Netherlands, ISBN 978-0-7923-4211-3, 2010. a
Ratcliffe, N. G., Ryder, C. L., Bellouin, N., Woodward, S., Jones, A., and Johnson, B.: Mean modelled dust mass concentration vertical profile (Junes 2010-2014) at Sahara, Canaries, Cape Verde and Caribbean (HadGEM3A-GA7.1), Zenodo [data set], https://doi.org/10.5281/zenodo.10722717, 2024. a
Renard, J.-B., Dulac, F., Durand, P., Bourgeois, Q., Denjean, C., Vignelles, D., Couté, B., Jeannot, M., Verdier, N., and Mallet, M.: In situ measurements of desert dust particles above the western Mediterranean Sea with the balloon-borne Light Optical Aerosol Counter/sizer (LOAC) during the ChArMEx campaign of summer 2013, Atmos. Chem. Phys., 18, 3677–3699, https://doi.org/10.5194/acp-18-3677-2018, 2018. a
Rodakoviski, R., Kok, J., and Chamecki, M.: Dust Settling From Turbulent Layers in the Free Troposphere: Implications for the Saharan Air Layer, J. Geophys. Res.-Atmos., 128, e2022JD037724, https://doi.org/10.1029/2022JD037724, 2023. a
Rosenberg, P. D., Parker, D. J., Ryder, C. L., Marsham, J. H., Garcia-Carreras, L., Dorsey, J. R., Brooks, I. M., Dean, A. R., Crosier, J., McQuaid, J. B., and Washington, R.: Quantifying particle size and turbulent scale dependence of dust flux in the Sahara using aircraft measurements, J. Geophys. Res.-Atmos., 119, 7577–7598, https://doi.org/10.1002/2013JD021255, 2014. a, b
Rosenfeld, D., Lohmann, U., Raga, G. B., O'Dowd, C. D., Kulmala, M., Fuzzi, S., Reissell, A., and Andreae, M. O.: Flood or Drought: How Do Aerosols Affect Precipitation?, Science, 321, 1309–1313, https://doi.org/10.1126/science.1160606, 2008. a
Ryder, C. L., Marenco, F., Brooke, J. K., Estelles, V., Cotton, R., Formenti, P., McQuaid, J. B., Price, H. C., Liu, D., Ausset, P., Rosenberg, P. D., Taylor, J. W., Choularton, T., Bower, K., Coe, H., Gallagher, M., Crosier, J., Lloyd, G., Highwood, E. J., and Murray, B. J.: Coarse-mode mineral dust size distributions, composition and optical properties from AER-D aircraft measurements over the tropical eastern Atlantic, Atmos. Chem. Phys., 18, 17225–17257, https://doi.org/10.5194/acp-18-17225-2018, 2018. a, b, c, d, e, f, g, h, i
Ryder, C. L., Highwood, E. J., Lai, T. M., Sodemann, H., and Marsham, J. H.: Impact of atmospheric transport on the evolution of microphysical and optical properties of Saharan dust, Geophys. Res. Lett., 40, 2433–2438, https://doi.org/10.1002/grl.50482, 2013a. a, b, c, d, e, f, g
Ryder, C. L., Highwood, E. J., Rosenberg, P. D., Trembath, J., Brooke, J. K., Bart, M., Dean, A., Crosier, J., Dorsey, J., Brindley, H., Banks, J., Marsham, J. H., McQuaid, J. B., Sodemann, H., and Washington, R.: Optical properties of Saharan dust aerosol and contribution from the coarse mode as measured during the Fennec 2011 aircraft campaign, Atmos. Chem. Phys., 13, 303–325, https://doi.org/10.5194/acp-13-303-2013, 2013b. a, b, c, d, e, f, g
Ryder, C. L., McQuaid, J. B., Flamant, C., Rosenberg, P. D., Washington, R., Brindley, H. E., Highwood, E. J., Marsham, J. H., Parker, D. J., Todd, M. C., Banks, J. R., Brooke, J. K., Engelstaedter, S., Estelles, V., Formenti, P., Garcia-Carreras, L., Kocha, C., Marenco, F., Sodemann, H., Allen, C. J. T., Bourdon, A., Bart, M., Cavazos-Guerra, C., Chevaillier, S., Crosier, J., Darbyshire, E., Dean, A. R., Dorsey, J. R., Kent, J., O'Sullivan, D., Schepanski, K., Szpek, K., Trembath, J., and Woolley, A.: Advances in understanding mineral dust and boundary layer processes over the Sahara from Fennec aircraft observations, Atmos. Chem. Phys., 15, 8479–8520, https://doi.org/10.5194/acp-15-8479-2015, 2015. a, b, c, d
Ryder, C. L., Highwood, E. J., Walser, A., Seibert, P., Philipp, A., and Weinzierl, B.: Coarse and giant particles are ubiquitous in Saharan dust export regions and are radiatively significant over the Sahara, Atmos. Chem. Phys., 19, 15353–15376, https://doi.org/10.5194/acp-19-15353-2019, 2019. a, b, c
Sassen, K., DeMott, P. J., Prospero, J. M., and Poellot, M. R.: Saharan dust storms and indirect aerosol effects on clouds: CRYSTAL-FACE results, Geophys. Res. Lett., 30, 1633, https://doi.org/10.1029/2003GL017371, 2003. a
Saxby, J., Beckett, F., Cashman, K., Rust, A., and Tennant, E.: The impact of particle shape on fall velocity: Implications for volcanic ash dispersion modelling, J. Volcanol. Geoth. Res., 362, 32–48, https://doi.org/10.1016/J.JVOLGEORES.2018.08.006, 2018. a, b
Seinfeld, J. H.: Atmospheric Chemistry and Physics of Air Pollution, John Wiley, New York, ISBN 9780471828570, 1986. a
Shao, Y., Wyrwoll, K.-H., Chappell, A., Huang, J., Lin, Z., McTainsh, G. H., Mikami, M., Tanaka, T. Y., Wang, X., and Yoon, S.: Dust cycle: An emerging core theme in Earth system science, Aeolian Res., 2, 181–204, https://doi.org/10.1016/j.aeolia.2011.02.001, 2011. a
Toth III, J. R., Rajupet, S., Squire, H., Volbers, B., Zhou, J., Xie, L., Sankaran, R. M., and Lacks, D. J.: Electrostatic forces alter particle size distributions in atmospheric dust, Atmos. Chem. Phys., 20, 3181–3190, https://doi.org/10.5194/acp-20-3181-2020, 2020. a, b
van der Does, M., Korte, L. F., Munday, C. I., Brummer, G.-J. A., and Stuut, J.-B. W.: Particle size traces modern Saharan dust transport and deposition across the equatorial North Atlantic, Atmos. Chem. Phys., 16, 13697–13710, https://doi.org/10.5194/acp-16-13697-2016, 2016. a
van der Does, M., Knippertz, P., Zschenderlein, P., Harrison, R. G., and Stuut, J. B. W.: The mysterious long-range transport of giant mineral dust particles, Science Advances, 4, eaau2768, https://doi.org/10.1126/sciadv.aau2768, 2018. a, b
Walser, A.: On the Saharan Air Layer Aerosol and its Role as a Reservoir of Cloud Condensation Nuclei, PhD thesis, Ludwig Maximillians Universitat Munchen, https://doi.org/10.5282/edoc.21664, 2017. a, b, c
Walters, D., Baran, A. J., Boutle, I., Brooks, M., Earnshaw, P., Edwards, J., Furtado, K., Hill, P., Lock, A., Manners, J., Morcrette, C., Mulcahy, J., Sanchez, C., Smith, C., Stratton, R., Tennant, W., Tomassini, L., Van Weverberg, K., Vosper, S., Willett, M., Browse, J., Bushell, A., Carslaw, K., Dalvi, M., Essery, R., Gedney, N., Hardiman, S., Johnson, B., Johnson, C., Jones, A., Jones, C., Mann, G., Milton, S., Rumbold, H., Sellar, A., Ujiie, M., Whitall, M., Williams, K., and Zerroukat, M.: The Met Office Unified Model Global Atmosphere 7.0/7.1 and JULES Global Land 7.0 configurations, Geosci. Model Dev., 12, 1909–1963, https://doi.org/10.5194/gmd-12-1909-2019, 2019. a, b
Weinzierl, B., Ansmann, A., Prospero, J. M., Althausen, D., Benker, N., Chouza, F., Dollner, M., Farrell, D., Fomba, W. K., Freudenthaler, V., Gasteiger, J., Groß, S., Haarig, M., Heinold, B., Kandler, K., Kristensen, T. B., Mayol-Bracero, O. L., Müller, T., Reitebuch, O., Sauer, D., Schäfler, A., Schepanski, K., Spanu, A., Tegen, I., Toledano, C., and Walser, A.: The Saharan aerosol long-range transport and aerosol-cloud-interaction experiment: Overview and selected highlights, B. Am. Meteorol. Soc., 98, 1427–1451, https://doi.org/10.1175/BAMS-D-15-00142.1, 2017. a, b, c, d, e, f
Wieland, L.-M., Dollner, M., Gasteiger, J., Ryder, C. L., Tegen, I., and Weinzierl, B.: Constraining AOD-dependence of size-resolved dust radiative effects during Saharan mineral dust transport, in preparation, 2024. a
Woodward, S.: Modelling the atmospheric life cycle and radiative impact of mineral dust in the Hadley Centre climate model, J. Geophys. Res., 106, 18155–18166, https://doi.org/10.1029/2000JD900795, 2001. a, b
Woodward, S., Sellar, A. A., Tang, Y., Stringer, M., Yool, A., Robertson, E., and Wiltshire, A.: The simulation of mineral dust in the United Kingdom Earth System Model UKESM1, Atmos. Chem. Phys., 22, 14503–14528, https://doi.org/10.5194/acp-22-14503-2022, 2022. a, b, c, d, e
Yang, W., Marshak, A., Kostinski, A. B., and Várnai, T.: Shape-induced gravitational sorting of Saharan dust during transatlantic voyage: Evidence from CALIOP lidar depolarization measurements, Geophys. Res. Lett., 40, 3281–3286, https://doi.org/10.1002/grl.50603, 2013. a
Zhao, A., Ryder, C. L., and Wilcox, L. J.: How well do the CMIP6 models simulate dust aerosols?, Atmos. Chem. Phys., 22, 2095–2119, https://doi.org/10.5194/acp-22-2095-2022, 2022. a
Zhuang, J., Jacob, D. J., and Eastham, S. D.: The importance of vertical resolution in the free troposphere for modeling intercontinental plumes, Atmos. Chem. Phys., 18, 6039–6055, https://doi.org/10.5194/acp-18-6039-2018, 2018. a