the Creative Commons Attribution 4.0 License.
the Creative Commons Attribution 4.0 License.
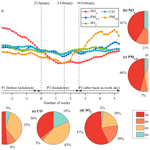
Unbalanced emission reductions of different species and sectors in China during COVID-19 lockdown derived by multi-species surface observation assimilation
Xiao Tang
Jiang Zhu
Zifa Wang
Pingqing Fu
Huangjian Wu
Miaomiao Lu
Qian Wu
Shuyuan Huang
Wenxuan Sui
Jie Li
Xiaole Pan
Lin Wu
Hajime Akimoto
Gregory R. Carmichael
The unprecedented lockdown of human activities during the COVID-19 pandemic has significantly influenced social life in China. However, understanding the impact of this unique event on the emissions of different species is still insufficient, prohibiting the proper assessment of the environmental impacts of COVID-19 restrictions. Here we developed a multi-air-pollutant inversion system to simultaneously estimate the emissions of NOx, SO2, CO, PM2.5 and PM10 in China during COVID-19 restrictions with high temporal (daily) and horizontal (15 km) resolutions. Subsequently, contributions of emission changes versus meteorological variations during the COVID-19 lockdown were separated and quantified. The results demonstrated that the inversion system effectively reproduced the actual emission variations in multi-air pollutants in China during different periods of COVID-19 lockdown, which indicate that the lockdown is largely a nationwide road traffic control measure with NOx emissions decreasing substantially by ∼40 %. However, emissions of other air pollutants were found to only decrease by ∼10 % because power generation and heavy industrial processes were not halted during lockdown, and residential activities may actually have increased due to the stay-at-home orders. Consequently, although obvious reductions of PM2.5 concentrations occurred over the North China Plain (NCP) during the lockdown period, the emission change only accounted for 8.6 % of PM2.5 reductions and even led to substantial increases in O3. The meteorological variation instead dominated the changes in PM2.5 concentrations over the NCP, which contributed 90 % of the PM2.5 reductions over most parts of the NCP region. Meanwhile, our results suggest that the local stagnant meteorological conditions, together with inefficient reductions of PM2.5 emissions, were the main drivers of the unexpected PM2.5 pollution in Beijing during the lockdown period. These results highlighted that traffic control as a separate pollution control measure has limited effects on the coordinated control of O3 and PM2.5 concentrations under current complex air pollution conditions in China. More comprehensive and balanced regulations for multiple precursors from different sectors are required to address O3 and PM2.5 pollution in China.
- Article
(7673 KB) -
Supplement
(4762 KB) - BibTeX
- EndNote
A novel coronavirus disease (COVID-19) broke out in Wuhan at the end of 2019 but quickly spread across the whole of China within a month. To curb the spread of the virus, strict epidemic control measures were implemented by the Chinese government to prevent large gatherings, including strict travel restriction, shutting down non-essential industries, extended holidays, and the closing of schools and entertainment establishments (Cheng et al., 2020). These restrictions have had a significant impact on industrial activities and social lives, as exemplified by the drop in China's industrial output by 15 %–30 % (https://data.stats.gov.cn/, last access: 22 October 2022) and the dramatic decrease in traffic flow by 60 %–90 % in major cities of China during the COVID-19 epidemic (http://jiaotong.baidu.com/, last access: 22 October 2022), which provides us a natural experiment to examine the responses of the emissions and air quality on the changes in human activities.
It has been well documented that the short-term stringent emission controls targeted on power generation and heavy industry enacted by the Chinese government during certain societal events, such as the 2008 Olympics Games, 2014 Asia-Pacific Economic Cooperation conference and 2015 China Victory Day parade, are an effective way to reduce emissions and improve air quality (Okuda et al., 2011; Wang et al., 2014; Tang et al., 2015; Zhang et al., 2016; Wu et al., 2020; Chu et al., 2018). However, different from those stringent emission controls, the COVID-19 restrictions are inclined to affect emissions from sectors more closely to social life whose influence on emissions has still not been well assessed. Previous studies suggest that the COVID-19 restrictions have substantially reduced China's anthropogenic emissions from almost all sectors (Zheng et al., 2021; Huang et al., 2021; Xing et al., 2020). For example, by using a bottom-up method based on near-real-time activity data, Zheng et al. (2021) reported that the emissions of NOx, SO2, CO and primary PM2.5 decreased by 36 %, 27 %, 28 % and 24 % during COVID-19 restrictions, mostly due to the reductions in the industry and transportation sectors. Xing et al. (2020), by using a response model, estimated stronger COVID-19 shutdown effects on emissions over the North China Plain (NCP) with emissions of NOx, SO2 and primary PM2.5 dropping by 51 %, 28 % and 63 %, respectively. Others argue that the COVID-19 restrictions may mainly affect the emissions from transportation, light industry and manufacturing, while they have much smaller effects on the emissions from power generation and heavy industry because of their non-interruptible processes (Chu et al., 2021; Hammer et al., 2021; Le et al., 2020; Zhao et al., 2020). Moreover, the residential emissions may even increase during the COVID-19 lockdown due to the increased demand for space heating and cooking with the stay-at-home orders. Therefore, Le et al. (2020) only considered the NOx reductions during COVID-19 restrictions in their investigation of the severe haze during the COVID-19 lockdown, and similarly, Hammer et al. (2021) only considered the emission reductions in the transportation sector. This indicates that there is large uncertainty in the current understanding of the effects of COVID-19 restrictions on the emissions of different species.
Quantification of the emission changes in different species and different sectors during the COVID-19 lockdown is thus necessary for the comprehensive understanding of the environmental impacts of COVID-19 restrictions. In particular, although observations indeed show decreases in air pollutant concentrations during COVID-19 restrictions (Fan et al., 2020; Wang et al., 2021; He et al., 2020; Shi and Brasseur, 2020), the air quality improvement is much smaller than expected (Shi et al., 2021; Diamond and Wood, 2020; Yan et al., 2022). Moreover, severe haze still occurred in northern China (Sulaymon et al., 2021; Le et al., 2020), and O3 concentrations even showed significant increases (Zhang et al., 2021; Li et al., 2020). A number of studies were conducted to explain this anomalistic air quality change by analyzing the effects of emission changes, meteorological variations and secondary production (Huang et al., 2021; Le et al., 2020; Hammer et al., 2021; Zhao et al., 2020, 2021; Sulaymon et al., 2021; Wang et al., 2020; Li et al., 2021). However, due to the unknown emission changes during COVID-19 restrictions, the emission reduction scenarios used to represent the COVID-19 shutdown effects varied among different studies and did not consider the spatial and temporal heterogeneity of the emission changes, leading to biases in the model simulation (Zhao et al., 2021; Li et al., 2021; Hammer et al., 2021; Zheng et al., 2021) and uncertainty in the quantification of the contributions of different factors.
Pioneer studies by Zheng et al. (2021) and Forster et al. (2020) have derived multi-air-pollutant emissions from social activity data using a bottom-up method, but due to the lack of detailed social activity data, large uncertainties existed in their estimates. The meteorologically and seasonally driven variability in the concentrations of air pollutants also prohibits drawing fully quantitative conclusions on the changes in emissions based on observations alone (Levelt et al., 2022). The emission inversion technique, which takes advantage of the chemical transport model (CTM) and real-time observations, provides an attractive way to estimate the sector-specific and space-based emission changes during COVID-19 restrictions, as shown in Zhang et al. (2020, 2021), Feng et al. (2020), and Hu et al. (2022). However, these studies only inversed the emissions of single species (e.g., NOx and SO2) without insights into multiple species. In view of this discrepancy, in this study we developed a multi-air-pollutant inversion system to simultaneously estimate the multi-air-pollutant emissions in China, including NOx, SO2, CO, PM2.5 and PM10, during the COVID-19 restrictions using an ensemble Kalman filter (EnKF) and surface observations from the China National Environmental Monitoring Centre (CNEMC). Subsequently, the inversed emission inventory was used to quantify the contributions of emission changes versus meteorological variations to the changes in PM2.5 and O3 concentrations over the NCP region during the COVID-19 restrictions.
We developed a high-resolution multi-air-pollutant inversion system to estimate the daily emissions of NOx, SO2, CO, PM2.5 and PM10 in China from 1 January to 29 February 2020 when the COVID-19 pandemic was at its most serious and the effects of the COVID-19 restrictions were most profound in China. This system uses the NAQPMS (Nested Air Quality Prediction Modelling System) model as the forecast model and the EnKF coupled with the state argumentation method as the inversion method. It has the capabilities of the simultaneous inversion of multi-air-pollutant emissions at high temporal (daily) and spatial (15 km) resolutions. An iteration inversion scheme was also developed in this study to address the large biases in the a priori emissions. In order to better characterize the emission changes during the COVID-19 restrictions, the whole time period was divided into three periods according to different control phases of COVID-19 and the timing of the Chinese Lunar New Year: before lockdown (P1, 1–20 January), lockdown (P2, 21 January–9 February) and after back-to-work days (P3, 10–29 February). Emission changes in different regions of China were also analyzed, including the North China Plain (NCP), northeast China (NE), southeast China (SE), southwest China (SW), northwest China (NW) and central regions (defined in Fig. 1), to investigate the responses of emissions to the COVID-19 restrictions in different regions. In the following sections, we briefly introduce each component of the inversion system.
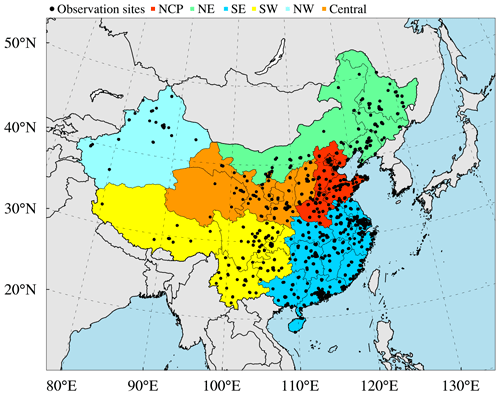
Figure 1Modeling domain of the ensemble simulation overlaying the distributions of observation sites from CNEMC. Different colors denote the different regions in mainland of China, namely the North China Plain (NCP), northeast China (NE), southwest China (SW), southeast China (SE), northwest China (NW) and central.
2.1 Chemical transport model and its configuration
The NAQPMS model was used as the forecast model to represent the atmospheric chemistry in this study, which has been used in previous inversion studies (Tang et al., 2011, 2013; Kong et al., 2019; Wu et al., 2020), where detailed descriptions of NAQPMS are available. The Weather Research and Forecasting Model (WRF) (Skamarock, 2008) is used to provide the meteorological inputs to the NAQPMS model.
Figure 1 shows the modeling domain of this study with a high horizontal resolution of 15 km. The a priori emission inventory used in this study includes monthly anthropogenic emissions from the HTAP_v2.2 emission inventory for the base year of 2010 (Janssens-Maenhout et al., 2015), biomass burning emissions from the Global Fire Emissions Database (GFED) version 4 (Randerson et al., 2017; Van Der Werf et al., 2010), biogenic volatile organic compound (BVOC) emissions from MEGAN-MACC (Sindelarova et al., 2014), marine volatile organic compound emissions from the POET database (Granier et al., 2005), soil NOx emissions from the regional emission inventory in Asia (Yan et al., 2003) and lightning NOx emissions from Price et al. (1997). Chemical top and boundary conditions were provided by the global CTM MOZART (Model for Ozone and Related Chemical Tracers) (Brasseur et al., 1998; Hauglustaine et al., 1998). We assumed no monthly variations in the a priori emission inventory and used January's emission inventory for the whole simulation period so that the emission variation was solely derived from the surface observations. A 2-week free run of NAQPMS was conducted as a spin-up time. For each day's meteorological simulation, a 36 h free run of WRF was conducted, of which the first 12 h simulation was a spin-up run and the next 24 h simulation provided the meteorological inputs to NAQPMS. Initial and boundary conditions for the meteorological simulation were provided by the reanalysis data of the National Center for Atmospheric Research and National Centers for Environment Prediction (NCAR/NCEP). Evaluation results for the WRF simulation are available in Text S1 in the Supplement.
2.2 Surface observations
The hourly concentrations of NO2, SO2, CO, PM2.5 and PM10 from CNEMC were used in this study to estimate the emissions during COVID-19. The spatial distributions of these observation sites are shown in Fig. 1, which contains 1436 observation sites covering most regions of China. Before assimilation, outliers of observations were first filtered out using the automatic outlier detection method developed by Wu et al. (2018) to prevent the adverse effects of the outliers on data assimilation. Then, the hourly concentrations were averaged to the daily values for the inversions of daily emissions.
The observation error is one of the key inputs to the data assimilation, which together with the background error determines the relative weights of the observation and background values on the analysis. The observation error includes measurement error and representativeness error. The measurement error of each species was designated according to the officially released documents of the Chinese Ministry of Ecology and Environmental Protection (HJ 193-2013 and HJ 654-2013, available at http://www.cnemc.cn/jcgf/dqhj/, last access: 22 October 2022), which is 5 % for PM2.5 and PM10 and 2 % for SO2, NO2 and CO. A representativeness error arises from the different spatial scales that the discrete observation data and model simulation represent, which was estimated based on the previous study by Li et al. (2019) and Kong et al. (2021). It should be noted that the NO2 measurements from CNEMC are made with a chemiluminescent analyzer with a molybdenum converter. Due to the interference of HNO3, PAN (peroxyacetyl nitrate) and alkyl nitrates (ANs), the NO2 concentrations can be overestimated (Dunlea et al., 2007; Lamsal et al., 2008), which may lead to spurious decreases in NOx emissions during the lockdown period. Previous studies usually use a chemical transport model to simulate NOx, HNO3, PAN and AN to produce correction factors (CFs) for the NO2 measurements (Cooper et al., 2020; He et al., 2022) using the following relationship proposed by Lamsal et al. (2008):
but the calculation of CF could be affected by the simulation errors in the model caused by uncertainties in the emission inventory or other error sources which may contaminate the observations. Therefore, similar to Feng et al. (2020), we did not correct the NO2 measurements in our inversion of NOx emissions since there were large uncertainties in the NOx emissions during the COVID-19 pandemic that possibly led to erroneous CFs. Since the EnKF considered the errors in observations through the use of an observation error covariance matrix, the chemiluminescence monitor interference to the NO2 measurements was treated as the observation error during the assimilation. A sensitivity inversion experiment was also conducted based on the corrected NO2 measurements using CFs, which suggests that the chemiluminescence monitor interference only has small impacts on the inversed NOx emissions in terms of magnitude and its variation during the COVID-19 pandemic. Detailed results of the sensitivity experiment are available in Text S2.
2.3 Inversion estimation scheme
The EnKF coupled with the state augmentation method was used in this study to constrain the emissions of multiple species. EnKF is an advanced data assimilation method proposed by Evensen (1994) that features the representation of the uncertainties of the model state by a stochastic ensemble of model realizations. Different from the mass balance method used in Zhang et al. (2020, 2021) that has difficulties in accounting for nonlinear relationships between emissions and concentrations and is more suitable for short-lived species (e.g. NOx) under relatively coarse () resolutions (Streets et al., 2013), the EnKF can consider the indirect relationship between emissions and concentrations caused by complex physical and chemical processes in the atmosphere through the use of flow-dependent background error covariance produced by ensemble CTM forecasts (Evensen, 2009; Miyazaki et al., 2012). Compared with the four-dimensional variational assimilation method used in Hu et al. (2022), the EnKF method has comparable computational cost (Skachko et al., 2014) but is more easily implemented without the need to develop complicated adjoint models for complex CTMs. The state augmentation method is a commonly used parameter estimation method (Tandeo et al., 2020), in which the emissions of multiple species are treated as a state variable and are simultaneously updated according to the relationship between the emissions and concentrations of related species. Due to the chemical reactions in the atmosphere, the concentrations of different species are interrelated with each other. For example, the ambient PM2.5 is not only primarily emitted but also formed secondarily through reactions with several gaseous precursors, such as NO2 and SO2. This means that the estimations of PM2.5 emissions by the single inversed estimation method could be biased if the errors in NO2 and SO2 emissions were not corrected synchronously. Therefore, it is beneficial to do the multi-species inversion estimation which can provide more constraints on the atmospheric chemical system and lead to more reasonable inversion results. Meanwhile, the use of the EnKF method coupled with the state augmentation method allows the estimations of multi-species emissions almost without additional computational cost.
The appropriate estimation of the uncertainty in emissions and chemical concentrations is important for the performance of the inversion estimation using EnKF. Since the source emission data over mainland China in the HTAP_v2.2 inventory are obtained from the MIX inventory (Li et al., 2017b), the uncertainties of emissions of different species, including PMF (fine-mode unspeciated aerosol), PMC (coarse-mode unspeciated aerosol), BC (black carbon), OC (organic carbon), NOx, CO, SO2, NH3 and NMVOCs (non-methane volatile organic compounds), were obtained from Li et al. (2017b) and Streets et al. (2003), which were represented by an ensemble of perturbed emissions generated by multiplying the a priori emissions with a perturbation factor βi,s:
where Ei,s represents the vector of the ith member of perturbed emissions for species s, represents the a priori emissions for this species, ∘ denotes the Schur product and Nens denotes the ensemble size. In this way, the adjustment of emissions is equivalent to the adjustment of perturbation factors.
In terms of the uncertainty in chemical concentrations, considering that emission uncertainty is the major contributor to the uncertainties in air quality modeling, especially during the COVID-19 period when emissions changed rapidly, uncertainties in chemical variables were obtained through ensemble simulations driven by perturbed emissions. The ensemble size was chosen to be 50 to maintain the balance between the filter performance and computational cost. After the ensemble simulations, emissions of multiple species were updated using a deterministic form of EnKF (DEnKF) proposed by Sakov and Oke (2008), which is formulated by
where x denotes the state variables, b the background state (a priori), a the analysis state (posteriori), the ensemble-estimated background error covariance matrix and N the ensemble size. yo represents the vector of observations with an error covariance matrix of R. H is the linear observational operator that maps the m-dimensional state vector x to a p-dimensional (number of observations) observational vector (). The state variables were defined as follows according to the state augmentation method during the assimilation:
where xi represents the ith member of the assimilated state variable, which consists of the fields of chemical variables ci and emission perturbation factors βi. Detailed descriptions of the model state variables are summarized in Table 1. The use of PM10−2.5 (PM10 minus PM2.5) values was aimed at avoiding the potential cross-correlations between PM2.5 and PM10 (Peng et al., 2018; Ma et al., 2019). Moreover, to prevent spurious correlations between non- or weakly related variables, similar to Ma et al. (2019) and Miyazaki et al. (2012), state variable localization was used during assimilation, with observations of one particular species only used in the updates of the same species' emission rate. The corresponding relationship between the chemical observations and adjusted emissions is summarized in Table 1. The PM2.5 observations were one exception and were used to update the emissions of PMF, BC and OC since the observations of speciated PM2.5 were not available in this study. The lack of speciated PM2.5 observations may lead to uncertainties in the estimated emissions of PMF, BC and OC. Therefore, we only analyzed the emissions of PM2.5, which were the sum of the emissions of these three species. Similarly, only PM10 emissions were analyzed in this study, which includes the emissions of PM2.5 and PMC.
Due to the strict control measures implemented during the last decades, the emissions in China decreased dramatically from 2010 to 2020, especially for SO2. Thus, there are large biases in the a priori estimates of emissions in China (Zheng et al., 2018), which could lead to incomplete adjustments of the a priori emissions and degrade the performance of the assimilation. Therefore, an iteration inversion scheme was developed in this study to address the large biases of SO2 emissions. As illustrated in Fig. 2, the main idea of the iteration inversion scheme is to update the ensemble mean of the state variable using the inversion results of the kth iteration and corresponding simulations. The state variable used in the (k+1)th inversions is written as follows:
where ck represents the simulation results using the inversed emissions of the kth iteration, represents the ith member of ensemble simulations with an ensemble mean of , βk represents the perturbation factors of the kth iteration, and represents the ith member of the ensemble of perturbation factors with a mean value of .
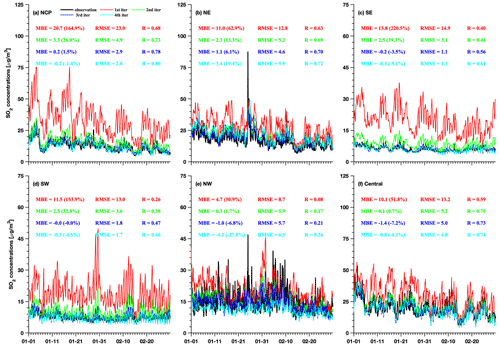
Figure 3Comparisons of the observed and simulated mean SO2 concentrations using emissions of different iteration time at validation sites over (a) the NCP region, (b) NE region, (c) SE region, (d) SW region, (e) NW region and (f) central region.
Using this method, the problems of large biases in the a priori emissions were well addressed as exemplified in Fig. 3 for SO2 emissions. It can be clearly seen that due to the large positive biases in the a priori SO2 emissions, the model still has large positive biases (normalized mean bias: NMB =30.9 %–220.5 %) and errors (RMSE =8.7–23.0 µg m−3) in simulated SO2 concentration over all regions of China even after assimilation (first iteration). However, the biases and errors continued to decrease with the increase in iteration times till the fourth iteration in which there was no significant improvement in SO2 simulations compared to those in the third iteration. These results suggested that the iteration inversion method used in this study can constrain well the a priori emissions with large biases and that, in this application, conducting three iterations is enough for constraining the emissions. Besides SO2 emissions, the iteration inversion scheme was also applied to the emissions of other species. Meanwhile, to reduce the influences of random model errors (e.g., errors in meteorological inputs) on the estimation of the variation in emissions, a 15 d running average was performed on our daily inversion results after the inversion estimation.
2.4 Quantification of the effects of emission changes and meteorological variations
In previous studies, the meteorological-induced (MI) changes were usually determined by the CTM with a fixed emission input setting and a varying meteorological input. Then, the difference between the MI changes and total changes in air pollutant concentrations is defined as emission-induced (EI) changes. Another approach to estimate EI changes is to perform simulations with a fixed meteorological input setting and varying emission inputs. Then, the MI changes are defined as the difference between EI changes and total changes in air pollutant concentrations. Due to the nonlinear effects of atmospheric chemical systems, these two methods yield different results. Thus, both methods were used in this study to account for the nonlinear effects. The averaged results of these two methods are used to represent the impacts of emission changes and meteorological variation on the air quality changes during the COVID-19 restrictions. In total, three scenario experiments were designed based on our inversion results (Table 2). The first scenario simulation used the varying meteorological and emission inputs from the P1 to P2 period, which represents the real-world scenario and is used to estimate the total changes in air pollutant concentrations induced by emissions and meteorological changes from the P1 to P2 period (BASE scenario). The second scenario experiment used the varying meteorological inputs but replaced the emissions during the P2 period with those during the P1 period, which was used to estimate the MI changes using the first method (MET change scenario). The third scenario experiment used the varying emission input and replaced the meteorological input during the P2 period with that during the P1 period, which was used to estimate the EI changes using the second method (EMIS change scenario). Based on the first method, the MI and EI changes can be estimated as follows:
where MIMET change scenario represents the MI changes estimated based on the results from the MET change scenario; concp1, MET change scenario and concp2, MET change scenario represent the averaged concentrations of air pollutants during the P1 and P2 periods under the MET change scenario; EIMET change scenario represents the EI changes estimated based on the results from the MET change scenario; and concp1, BASE scenario and concp2, BASE scenario, respectively, represent the averaged concentrations of air pollutants during the P1 and P2 periods under the BASE scenario. Similarly, the MI and EI changes estimated based on the second method are formulated as follows:
Then, the estimations from these two methods are averaged to estimate the contributions of meteorological change and emission change to the changes in PM2.5 and O3 concentrations during the COVID-19 lockdown:
where contrimet and contriemis represent the relative contributions (%) of the meteorological variations and emission changes to the changes in air pollutant concentrations. A detailed definition of each notation used in the calculation of MI and EI changes is given in Table 3.
3.1 Validation of the inversion results
We firstly validate our inversion system by using a cross-validation method, in which 20 % of observation sites were withheld from the emission inversion and used as the validation datasets. Figures S1–S6 in the Supplement show the concentrations of different air pollutants in China from 1 January to 29 February 2020 obtained from observations at validation sites and simulations using a priori and a posteriori emissions. Commonly used statistical evaluation indices, including correlation coefficient (R), mean bias error (MBE), NMB and root mean square error (RMSE), are summarized in Table S1 in the Supplement. The validation results suggest that the a posteriori simulation agreed well with the observed concentrations for all species. The large biases in the a priori simulation of PM2.5, PM10, SO2 and CO were almost completely removed in the a posteriori simulation with NMBs of about −3.9 %–15.7 % for PM2.5, −3.1 %–11.6 % for PM10, −12.6 %–5.3 % for NO2, −9.5 %–6.2 % for SO2 and −10 %–7.6 % for CO (Table S1). RMSE values were also significantly reduced in the a posteriori simulation, which were 9.1–32.2 µg m−3 for PM2.5, 12.6–42.4 µg m−3 for PM10, 5.1–12.3 µg m−3 for NO2, 1.2–5.6 µg m−3 for SO2 and 0.10–0.46 mg m−3 for CO. Moreover, the inversion emissions considerably improved the fit to the observed time evolution of air pollutants' concentrations. The R values were improved for all species in the a posteriori simulation, which were up to 0.74–0.94 for PM2.5, 0.63–0.92 for PM10, 0.76–0.94 for NO2, 0.23–0.79 for SO2 and 0.63–0.92 for CO. These results suggest that our inversion results have excellent performance in representing the magnitude and variation in these species' emissions in China during COVID-19 restrictions. Model performance in simulating O3 concentrations is relatively poor compared to other species although improvement was remarkable in the NCP, NE and SW regions. This would be due to the use of an outdated emission inventory for the base year of 2010 and that the emissions of non-mental volatile organic compounds (NMVOCs), another important precursor for O3, were not constrained in this study. As shown in Fig. S7, the NMVOC emissions for the base year of 2010 were generally lower than those for 2018 except over the SW regions. Considering the increasing trend of NMVOC emissions in China (Li et al., 2019), the underestimates of NMVOC emissions for the base year of 2020 could be larger. This is in line with the negative biases in the simulated O3 concentrations over these regions.
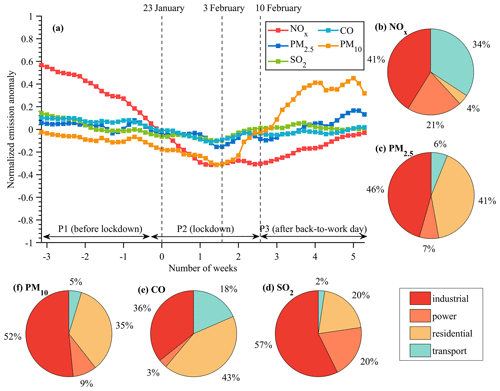
Figure 4(a) Time series of normalized emission anomalies estimated by inversion results for different species in China from 1 January to 29 February 2020 and (b–f) relative contributions of different sectors to the total anthropogenic emissions of NOx, PM2.5, PM10, CO and SO2 obtained from Zheng et al. (2018). The normalized emission anomaly is calculated by the emission anomaly divided by the average emissions during the whole period.
3.2 Emission changes in multiple species during COVID-19 restrictions
3.2.1 Unbalanced emission changes between NOx and other species
The control of COVID-19 began on 23 January 2020 when the Chinese government declared the first level of national responses to public health emergencies, 1 d before Chinese New Year's Eve. Figure 4 shows the time evolution of the normalized emission anomaly for different species in China from 1 January to 29 February. The temporal variation in the emissions varied largely between NOx and other species. Due to the combined effects of the Spring Festival and COVID-19 lockdown, NOx emissions decreased continuously at the beginning of January until approximately 1 week after the implementation of the COVID-19 lockdown, with estimated decreases in NOx emissions of up to 42.5 % from the P1 to P2 period (Table 4). Subsequently, the NOx emissions stabilized with small fluctuations until the official back-to-work day when the NOx emissions began to increase due to the easing of the control measures and the resumption of business. According to inversion estimation, NOx emissions recovered by 3.9 % during the P3 period. These results indicate that the temporal variation in our estimated NOx emissions agreed well with the timing of the Spring Festival and different control stages of COVID-19. However, for other species (i.e., PM2.5, PM10, SO2 and CO), although their emissions generally decreased from 1 January to the end of the 2020 Spring Festival holiday, they showed much smaller reductions than the NOx emissions. The emission reduction for these species was only approximately 7.9 %–12.1 % (Table 4). This is consistent with the inversion results of Hu et al. (2022), who found that SO2 emissions in China decreased only by 9.2 % during the COVID-19 lockdown. In addition, the emissions of these species quickly rebounded to their normal level just 1 week after the end of the Spring Festival holiday. As estimated by our inversion results, the SO2 emissions recovered by 7.2 % during the P3 period, which was only 2.5 % lower than that during the P1 period. The PM2.5 and PM10 emissions during the P3 period were 3.3 % and 43.6 % higher, respectively, than those during the P1 period.
Table 4Inversion estimated emissions of different air pollutants in China and their changes between different periods during COVID-19.
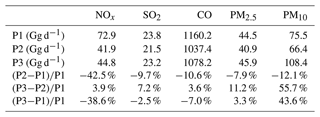
Table 5Inversion estimated emission changes of different air pollutants over different regions in China between different periods during COVID-19 restrictions.
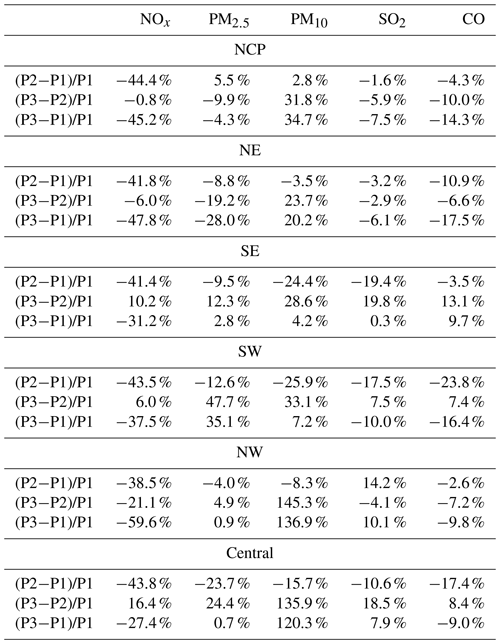
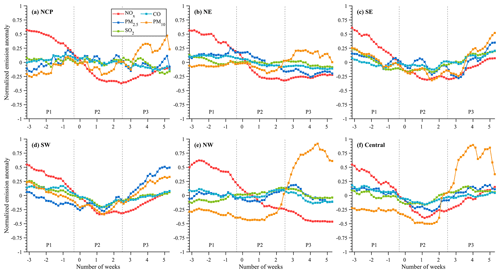
Figure 5Time series of normalized emission anomalies estimated by inversion results for different species over (a) the NCP region, (b) NE region, (c) SE region, (d) SW region, (e) NW region and (f) central region from 1 January to 29 February 2020.
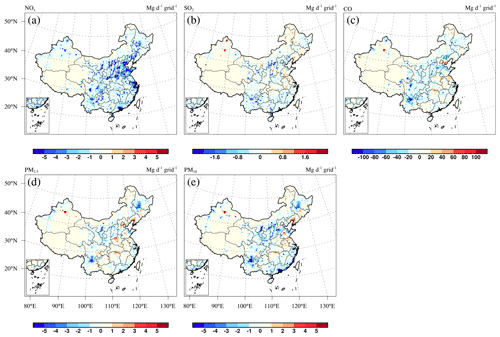
Figure 6The inversion estimated emission changes in (a) NOx, (b) SO2, (c) CO, (d) PM2.5 and (e) PM10 in China from P1 to P2 period.
Table 6Calculated MI and EI changes in PM2.5 concentrations during the COVID-19 pandemic by previous studies.
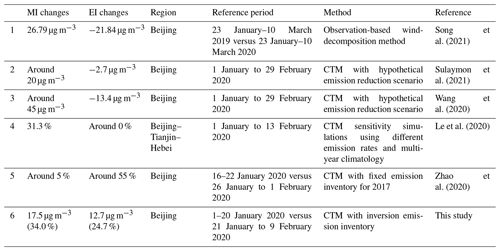
Similar results were found in different regions of China (Fig. 5 and Table 5), where the NOx emissions decreased much more than other species. In addition, unlike the uniform decreases in NOx emissions in different regions of China (∼40 %), there was apparent spatial heterogeneity in the emission changes of PM2.5, PM10, SO2 and CO (Table 5 and Fig. 6). For example, from the P1 to P2 period, the PM2.5 emissions decreased by over 20 % in the central region but only by 8.8 % in the NE region. The PM2.5 emissions even increased by 5.5 % in the NCP region. This may be due to the increased emissions from industry and fireworks according to the field measurements conducted by previous studies (Li et al., 2022; Ma et al., 2022; Zuo et al., 2022; Dai et al., 2020). Based on the measurement of stable Cu and Si isotopic signatures and distinctive metal ratios in Beijing and Hebei, Zuo et al. (2022) analyzed the variations in the PM2.5 sources during the COVID-19 pandemic, who reported that the primary PM2.5 emissions did not decrease in Beijing and Hebei and that the PM-associated industrial emissions may have actually increased during the lockdown period. The increased industrial heat sources detected by Li et al. (2022) based on VIIRS (Visible Infrared. Imaging Radiometer Suite) active fire data also supported the increased industrial emissions over the NCP region during the lockdown period. Meanwhile, consistent with the field measurements in Beijing and Tianjin conducted by Ma et al. (2022) and Dai et al. (2020), substantially high levels of potassium (K+) and magnesium (Mg2+) ions were found over the NCP region during the Spring Festival according to the aerosol chemical composition measurements obtained from CNEMC (Fig. S8). Since K+ and Mg2+ are two important fingerprints of the firework emissions, the high levels of K+ and Mg2+ suggest that the emissions from fireworks during the Spring Festival were also a potential contributor to the increase in PM2.5 emissions over the NCP region. In contrast, the SW and central regions exhibited relatively larger emission reductions for these species (Fig. 5 and Table 5) by 12.6 %–25.9 % and 10.6 %–23.7 %, respectively. The emission rebound during the P3 period was more prominent in the SE, central and SW regions (Figs. 5 and 7), where emissions recovered by 6.0 %–16.4 % for NOx, 7.5 %–19.8 % for SO2, 7.4 %–13.1 % for CO, 12.3 %–47.7 % for PM2.5 and 28.6 %–135.9 % for PM10 (Table 5). This result is consistent with the earlier degradation of the response level to the COVID-19 virus (from the first level to the second or third level) over these regions (Table S2). In contrast, there were decreases in emissions in the NCP, NE and NW regions. PM2.5 emissions were reduced by 9.9 % in the NCP region and by 19.2 % in the NE region from the P2 to P3 period (Table 5). Moreover, we found that the PM10 emissions surged in the NW and central regions, where the PM10 emissions during the P3 period were almost 2 times higher than those during the P2 period (Table 5). However, this finding may be related to the enhanced dust emissions over these two regions rather than the effects of returning to work according to the decreased PM2.5 PM10 ratios during the P3 period. According to Fig.S9, the PM2.5 PM10 ratio was relatively stable during the P1 and P2 period, but it decreased substantially during the P3 period, from 0.81 to 0.48 over the NW region and from 0.77 to 0.53 over the central region. A lower PM2.5 PM10 ratio commonly suggests that the PM10 is more likely to be attributed to natural sources such as dust (Wang et al., 2015; Fan et al., 2021). Moreover, the NW and central region are typical source areas of dust in China, therefore the increasing PM10 emissions over the NW and central regions may be mainly related to the enhanced dust emissions. This demonstrates the necessity of considering changes in natural emissions during COVID-19 restrictions. Thus, to reduce the effects of natural emissions on our findings, the same analysis was performed for the emissions over southeast China (Fig. S10) where emissions were dominated by anthropogenic sources, which shows consistent results with the findings above (Fig. S11 and Table S3).
3.2.2 Explanations for the emission changes during COVID-19 restrictions
Two explanations may help clarify the unbalanced emission changes between NOx and other species. First, the COVID-19 lockdown policy has led to dramatic decreases in transportation activities throughout China; however, as shown in Fig. 4, the relative contributions of the transportation sector to the emissions of SO2 (2.4 %), CO (18.5 %), PM2.5 (6.1 %) and PM10 (4.7 %) are much smaller than those for NOx emissions (34.3 %) (Zheng et al., 2018; Li et al., 2017a). Thus, the reduction in traffic activities can only substantially decrease NOx emissions. Reductions of CO emissions (−10.6 %) were relatively larger than those for SO2 (−9.7 %) and PM2.5 (−7.9 %) emissions, which is consistent with the relatively larger contributions of the transportation sector to CO emissions. However, the differences in the percentage decreases in emissions of CO, SO2 and PM2.5 are not as significant as the differences in their transportation share (18 % versus 2 % and 6 %). This may be, on the one hand, due to the uncertainty in the estimated relative contributions of different sectors to the total emissions of CO, SO2 and PM2.5, but, on the other hand, they were possibly due to the uncertainty in the emission inversions, especially considering that the decreasing trend of CO, SO2 and PM2.5 was not significant. Also, other factors beyond transportation may have influenced the reductions of anthropogenic emissions during the P2 period. For example, the PM10 emissions showed the largest reductions among these four species, which is related in part to the reduced dust emissions due to shutting down of construction sites during the lockdown period (Li et al., 2020). Second, as shown in Fig. 4, the industrial and residential sectors are the major contributors to the anthropogenic emissions of SO2, CO, PM2.5 and PM10 in China, together contributing 77.6 %, 78.3 %, 86.5 % and 86.3 %, respectively, to their total emissions. The much smaller reductions of these species' emissions were thus in line with the fact that there were no intentional restrictions on heavy industry during the COVID-19 restrictions. A large number of non-interruptible processes, such as steel, glass, coke, refractory, petrochemical, electric power, and especially heating, could not be stopped during the COVID-19 lockdown. According to statistical data from the National Bureau of Statistics of China (Fig. S12), the industrial and power sectors did not show similar reductions of their activity levels as those seen in the transportation sector. Power generation and steel production even showed increases in many provinces, which corresponds well with the emission increases over these regions. In addition, since people were required to stay at home, residential emissions were likely increased due to the increased energy consumption for heating or cooking. Therefore, our inversion results supported the views that the emissions of species related to industrial and residential activities did not decline much during the lockdown period and that the COVID-19 lockdown policy was largely a traffic control measure with small influences on other sectors.
3.3 Investigation of air quality change over the NCP region during COVID-19 restrictions
Using the inversion results, we reassessed the environmental impacts of the COVID-19 restrictions on the air pollution over the NCP region. The NCP region was chosen because it is the key target region of air pollution control in China and where unexpected severe haze occurred. A major caveat in previous studies that explored the impacts of COVID-19 lockdowns on air quality is the uncertainty in the emission changes during COVID-19 restrictions. The inversion results enable us to give a more reliable assessment of the environmental impacts of COVID-19 restrictions. Figure 8 shows the observed changes in PM2.5 and O3 concentrations over the NCP region from the P1 to P2 period. The observations showed consistent reductions of PM2.5 concentrations over the NCP region (by 13.6 µg m−3). However, substantial increases in PM2.5 concentrations were observed in the Beijing area (by 31.2 µg m−3). In contrast to the widespread reductions of PM2.5 concentrations, the O3 concentrations significantly increased over the whole NCP region (by 28.3 µg m−3) and the Beijing area (by 16.8 µg m−3). The simulations based on our inversion results reproduced the observed changes in PM2.5 and O3 concentrations over the NCP region well, although the O3 concentrations were underestimated in all regions (Fig. S6) and the changes in PM2.5 and O3 concentrations were slightly overestimated by 1.6 and 2.6 µg m−3 in the simulation (Fig. 8).
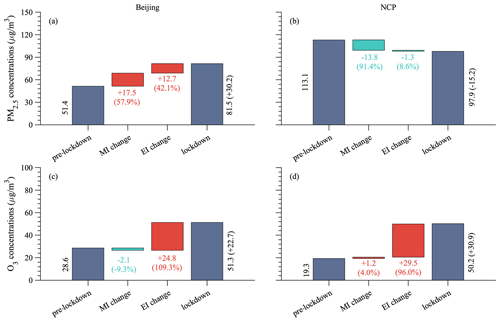
Figure 9Contributions of the meteorological variations and emission changes to the changes in (a, b) PM2.5 and (c, d) O3 concentrations over Beijing and the NCP region from the pre-lockdown period (P1) to the lockdown period (P2).
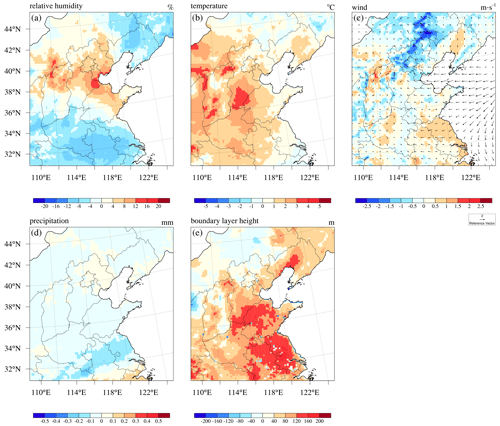
Figure 10Changes in the (a) relative humidity, (b) temperature, (c) wind speed, (d) precipitation and (e) boundary layer height over the NCP region from P1 to P2 period obtained from WRF simulations.
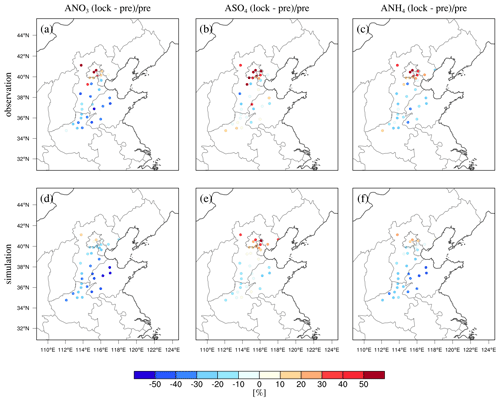
Figure 11Relative changes in the simulated and observed concentrations of (a, d) ANO3, (b, e) ASO4, (c, f) ANH4 over the NCP region from P1 to P2 period.
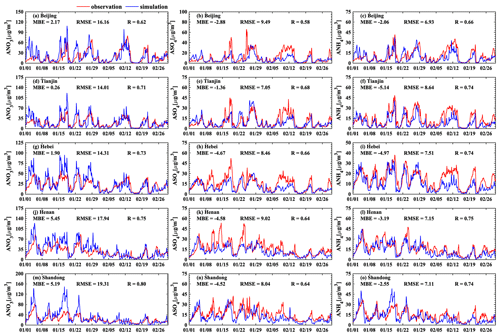
Figure 12Time series of observed and simulated concentrations of ANO3, ASO4 and ANH4 in (a–c) Beijing, (b–f) Tianjin, and (g–i) Heibei, (j–l) Henan and (m–o) Shangdong provinces from 1 January to 29 February 2020.
As detailed in the Sect. 2.4, the simulated changes in air pollutant concentrations before and after lockdown were decomposed into meteorological-induced (MI) changes and emission-induced (EI) changes through two different scenarios to account for the nonlinearity of the atmospheric chemical system. According to Fig. S13, the differences in calculated MI and EI changes based on different scenarios were small for PM2.5 concentrations, which were about 2 µg m−3 in this application, while they were relatively larger for O3, which were around 5 µg m−3 over the Beijing and NCP region (Fig. S14). In addition, the sign of calculated MI changes using different scenarios was opposite, although both suggested weak contributions of meteorological variation to the changes in O3 concentrations. This suggests that the calculated MI and EI changes in O3 concentrations could be more sensitive to the scenarios used, which may be associated with the stronger chemical nonlinearity of the O3 concentrations. Figure 9 shows the mean results of the calculated MI and EI changes using the two different scenarios. It shows that the meteorological variation dominated the changes in PM2.5 concentrations over the NCP region, which contributed 90 % of the PM2.5 reductions over most parts of the NCP region. Moreover, this variation made significant contributions (57.9 %) to the increases in PM2.5 concentrations over the Beijing area. This finding suggested that meteorological variations played an irreplaceable role in the occurrence of the unexpected PM2.5 pollution around the Beijing area. Compared with the meteorological conditions before lockdown (Fig. 10), there were increases in relative humidity over northern China, which facilitated the reactions for aerosol formation and growth. Wind speed also decreased over the Beijing area accompanied by an anomalous south wind, which facilitated aerosol accumulation and the transportation of air pollutants from the polluted industrial regions of Hebei Province to Beijing. The increases in boundary layer height from the P1 to P2 period were also much smaller in the Beijing area than in other areas of the NCP. Thus, the Beijing area has exhibited distinct meteorological variations from other areas of the NCP region, which correspond well to the different changes in PM2.5 concentrations over the Beijing area.
The emission changes contributed slightly to the PM2.5 reductions over the NCP region (8.6 %). This is because, on the one hand, the large reductions of NOx emissions (by 44.4 %) only reduced nitrate by approximately 10 %–30 % due to the nonlinear effects of chemical reactions (Fig. 11), and, on the other hand, the emissions of primary PM2.5 and its precursors from other sectors changed little during the COVID-19 restrictions (Table 5). The emission changes contributed more to the increased PM2.5 concentrations over the Beijing area (42.1 %). This is mainly associated with the increases in primary PM2.5 emissions around the Beijing area, as seen in Fig. 6, possibly due to the increased emissions from the different industries as we mentioned before (Zuo et al., 2022) and the increased firework emissions during the Spring Festival as shown by the rapid increases in concentrations of K+ and Mg2+ measured by CNEMC (Fig. S15). Therefore, our results suggested that the unexpected PM2.5 pollution during the lockdown period was mainly driven by unfavorable meteorological conditions, together with small changes or even increases in primary PM2.5 emissions. This finding is in line with previous results of Le et al. (2020) but different from those of Huang et al. (2021), who suggested that enhanced secondary aerosol formation was the main driver of severe haze during the COVID-19 restrictions. To investigate it, we further analyzed the changes in the concentrations of secondary inorganic aerosols (SIAs). First, we evaluated our model results against the observed SIA concentrations, which showed that the model results using our inversion emissions reproduced well the observed concentrations of SIAs over the NCP region (Fig. 12) with mean bias (MB) ranging from −5.14 to 5.45 µg m−3 and correlation coefficient (R) ranging from 0.59 to 0.80. The observed increases in SIA concentrations over the Beijing area, especially for sulfate concentrations, were also captured in our simulations (Fig. 11), although underestimation occurred due to the uncertainty in simulating SIA concentrations. Through sensitivity experiments, we found that the increases in SIA concentrations were still driven by meteorological variations (Fig. 13). In fact, the emission reductions only led to a 10 % decrease in SIA concentrations over the NCP region. This finding suggests that the enhanced secondary aerosol formation was likely mainly driven by the unfavorable meteorological conditions associated with higher temperature and relative humidity instead of the emission reductions during the lockdown period. This is in line with the observation evidence from Ma et al. (2022), who emphasized that the increased temperature and relative humidity promoted the formation of secondary pollutants during the COVID-19 restrictions.
In terms of O3 concentrations, the emission changes subsequently became the dominant contributor to the O3 increases by more than 100 % in the Beijing area and by 96.0 % over the NCP region. This result is mainly because the lockdown period occurred in midwinter when photochemical O3 formation was minimal; thus, the large increase in O3 is expected solely from the effect of the reduced titration reaction associated with the large reductions of NOx emissions. Although the higher temperature and slower wind speed during the lockdown period were favorable for the increases in O3 concentrations, their contributions were much smaller than those of emission changes (Fig. 9). These results suggested that control measures, such as COVID-19 restrictions, were inefficient for air pollution mitigation in China considering the high economic cost of the COVID-19 restrictions.
We also compared our results with previous studies that differentiated between the contributions of meteorology and emissions to the PM2.5 and O3 concentrations. Before comparisons, it should be noted that it is difficult to directly compare our results with previous studies due to the altered definition of the meteorological contribution, different reference period that was used to quantify the meteorological contributions and different targeted region. For example, in Song et al. (2021), the reference period used to determine the meteorological contribution is the corresponding period of the COVID-19 pandemic in 2019. Le et al. (2020) used the multiyear climatology as the reference period. In Wang et al. (2020) and Sulaymon et al. (2021), the MI changes in PM2.5 concentrations were defined as the difference between the modeled concentrations on high-pollution days and those on low-pollution days in hypothetical emission reduction scenarios. Zhao et al. (2020) used a similar reference period to ours to determine the MI changes, but they used the outdated emission inventory. Table 6 summarized the results from the selected studies over Beijing and the Beijing–Tianjin–Hebei region. Note that some studies only provided the relative changes in the modeled PM2.5 concentrations. It shows that due to the uncertainties in emission changes during the COVID-19 pandemic, the EI changes estimated by Zhao et al. (2020) were possibly overestimated compared to our studies (55 % versus 24.7 %). Both Sulaymon et al. (2021) and Wang et al. (2020) suggested negative EI changes during the COVID-19 period in Beijing. This because they presumed that the emissions were largely reduced during the COVID-19 lockdown which may deviate from the real changes in emissions according to our inversion results. Meanwhile, although they used the same method and reference period, their results differed largely (−2.7 versus −13.4 µg m−3) due to the different emission reduction scenario they assumed. Le et al. (2020) only considered the emission reductions of NOx in their sensitivity simulations without considerations of other species; therefore their calculated EI changes may be underestimated compared to our results (almost 0 % versus 24.7 %). However, the calculated MI changes were consistent between our study and Le et al. (2020). In terms of O3, the calculated EI changes by our study were also higher than those calculated by Zhao et al. (2020) in Beijing (85.7 % versus 70 %). These results suggested that the EI and MI changes calculated by our study could be more reasonable, considering that the emissions of different species were well constrained, which could better represent the temporal variation and spatial heterogeneity of emission changes during COVID-19.
The COVID-19 pandemic is an unprecedented event that significantly influenced social activity and the associated emissions of air pollutants. Our results provide a quantitative assessment of the influences of COVID-19 restrictions on multi-air-pollutant emissions in China. Otherwise, understanding the relationship between air quality and human activities may be biased. The inversion results provide important evidence that the COVID-19 lockdown policy was largely a traffic control measure with substantially reduced impacts on NOx emissions but much smaller influences on the emissions of other species and other sectors. Traffic control has widely been considered to be the normal protocol in implementing regulations in many cities of China, but its effectiveness for air pollution control is still disputed (Han and Naeher, 2006; Zhang et al., 2007; Chen et al., 2021; Cai and Xie, 2011; Chowdhury et al., 2017; X. Li et al., 2017). Thus, the COVID-19 restrictions provided us with a real nationwide traffic control scenario to investigate the effectiveness of traffic control on the mitigation of air pollution in China. The results suggested that traffic control as a separate pollution control measure has limited effects on the coordinated control of high concentrations of O3 and PM2.5 under the current air pollution conditions in China. In this case, the PM2.5 concentrations were slightly reduced, while there were substantial increases in O3 concentrations. Severe haze was also not avoided during the COVID-19 restrictions due to unbalanced emission changes from other sectors and unfavorable meteorological conditions. China is now facing major challenges in both controlling PM2.5 and controlling emerging O3 pollution. The tragic COVID-19 pandemic has revealed the limitation of the road traffic control measures in the coordinated control of PM2.5 and O3. More comprehensive regulations for multiple precursors from different sectors are required in the future to address O3 and PM2.5 pollution in China.
Finally, there are certain limitations that we should be aware of in our inversion work. Firstly, the COVID-19 restrictions were initiated during the Spring Festival of China which would also influence the air pollutant emissions in China. However, the inversion method used in this study did not differentiate between the contributions of the Spring Festival from the COVID-19 restrictions. Similarly, the effects of natural emission changes were not differentiated in this study, which could lead to uncertainty in quantifying the effects of the COVID-19 restrictions on air pollutant emissions. Secondly, the overestimations of NO2 measurements induced by chemiluminescence monitor interference were not directly corrected in our study due to the lack of synchronous observations of HNO3, PAN and AN; thus the estimated NOx emissions could be slightly overestimated according to the sensitivity run with corrected NO2 measurements using CFs (Figs. S16–S18). Meanwhile, the sensitivity results suggest that the inversed NOx emissions may even drop faster if the NO2 measurements were corrected over the SE and SW regions (Fig. S19). Thirdly, the use of an outdated emission inventory as the a priori emissions would also be a potential limitation in our work, although the iteration inversion method was used. A sensitivity inversion run was thus conducted based on the a priori emissions for a more recent year of 2018 to test the influence of the a priori emission inventory. This new emission inventory is comprised of the anthropogenic emissions obtained from HTAPv3 (Crippa et al., 2023); the biogenic, soil and oceanic emissions obtained from the CAMS global emission inventory (https://ads.atmosphere.copernicus.eu/cdsapp#!/dataset/cams-global-emission-inventories?tab=overview, last access: 15 March 2023); and the biomass burning emissions obtained from the Global Fire Assimilation System (GFAS) (Kaiser et al., 2012). Detailed steps of the new inversion estimation were the same as those elucidated in Sect. 2. The results suggest that the inversion results based on the 2010 and 2018 inventory were broadly close to each other, while the inversion results based on the 2018 inventory were relatively higher than those based on the 2010 inventory, reflecting the uncertainty in our inversion results caused by the choice of a priori emission inventory (Figs. S20–S22). However, the sensitivity run consistently showed that the NOx emissions decreased much more than other species (Figs. S23–S24). This suggests that the choice of a priori emission inventory may not obviously influence the main conclusion of our study but can lead to uncertainty in the magnitude of the inversion results, which potential readers should be aware of.
The inversion estimated emissions of multi-air pollutants in China during the COVID-19 lockdown period and the NAQPMS simulation results are available from the corresponding authors on request.
The supplement related to this article is available online at: https://doi.org/10.5194/acp-23-6217-2023-supplement.
XT, JZ and ZW conceived and designed the project; HW, LK, XT and LW established the data assimilation system; and ML, QW, SH and WS contributed to interpreting the data. LK conducted the inversion estimate, drew figures and wrote the paper with comments provided by JL, XP, MG, PF, YS, HA and GRC.
The contact author has declared that none of the authors has any competing interests.
Publisher’s note: Copernicus Publications remains neutral with regard to jurisdictional claims in published maps and institutional affiliations.
We acknowledge the use of surface air quality observation data from CNEMC and the support from the National Key Scientific and Technological Infrastructure project “Earth System Science Numerical Simulator Facility” (EarthLab). We would also like to thank the two reviewers and the editor who helped us to improve the article.
This research has been supported by the National Natural Science Foundation of China (grant nos. 42175132, 41875164, 92044303, 42205119) and the CAS Strategic Priority Research Program (grant no. XDA19040201).
This paper was edited by Chul Han Song and reviewed by two anonymous referees.
Brasseur, G. P., Hauglustaine, D. A., Walters, S., Rasch, P. J., Muller, J. F., Granier, C., and Tie, X. X.: MOZART, a global chemical transport model for ozone and related chemical tracers 1. Model description, J. Geophys. Res.-Atmos., 103, 28265–28289, https://doi.org/10.1029/98jd02397, 1998.
Cai, H. and Xie, S.: Traffic-related air pollution modeling during the 2008 Beijing Olympic Games: The effects of an odd-even day traffic restriction scheme, Sci. Total Environ., 409, 1935–1948, https://doi.org/10.1016/j.scitotenv.2011.01.025, 2011.
Chen, Z., Hao, X., Zhang, X., and Chen, F.: Have traffic restrictions improved air quality? A shock from COVID-19, J. Clean Prod., 279, 123622, https://doi.org/10.1016/j.jclepro.2020.123622, 2021.
Cheng, C., Barceló, J., Hartnett, A. S., Kubinec, R., and Messerschmidt, L.: COVID-19 Government Response Event Dataset (CoronaNet v.1.0), Nat. Hum. Behav., 4, 756–768, https://doi.org/10.1038/s41562-020-0909-7, 2020.
Chowdhury, S., Dey, S., Tripathi, S. N., Beig, G., Mishra, A. K., and Sharma, S.: “Traffic intervention” policy fails to mitigate air pollution in megacity Delhi, Environ. Sci. Policy, 74, 8–13, https://doi.org/10.1016/j.envsci.2017.04.018, 2017.
Chu, B., Zhang, S., Liu, J., Ma, Q., and He, H.: Significant concurrent decrease in PM2.5 and NO2 concentrations in China during COVID-19 epidemic, J. Environ. Sci., 99, 346–353, https://doi.org/10.1016/j.jes.2020.06.031, 2021.
Chu, K. K., Peng, Z., Liu, Z. Q., Lei, L. L., Kou, X. X., Zhang, Y., Bo, X., and Tian, J.: Evaluating the Impact of Emissions Regulations on the Emissions Reduction During the 2015 China Victory Day Parade With an Ensemble Square Root Filter, J. Geophys. Res.-Atmos., 123, 4122–4134, https://doi.org/10.1002/2017jd027631, 2018.
Cooper, M. J., Martin, R. V., McLinden, C. A., and Brook, J. R.: Inferring ground-level nitrogen dioxide concentrations at fine spatial resolution applied to the TROPOMI satellite instrument, Environ. Res. Lett., 15, 104013, https://doi.org/10.1088/1748-9326/aba3a5, 2020.
Crippa, M., Guizzardi, D., Butler, T., Keating, T., Wu, R., Kaminski, J., Kuenen, J., Kurokawa, J., Chatani, S., Morikawa, T., Pouliot, G., Racine, J., Moran, M. D., Klimont, Z., Manseau, P. M., Mashayekhi, R., Henderson, B. H., Smith, S. J., Suchyta, H., Muntean, M., Solazzo, E., Banja, M., Schaaf, E., Pagani, F., Woo, J.-H., Kim, J., Monforti-Ferrario, F., Pisoni, E., Zhang, J., Niemi, D., Sassi, M., Ansari, T., and Foley, K.: HTAP_v3 emission mosaic: a global effort to tackle air quality issues by quantifying global anthropogenic air pollutant sources, Earth Syst. Sci. Data Discuss. [preprint], https://doi.org/10.5194/essd-2022-442, in review, 2023.
Dai, Q., Liu, B., Bi, X., Wu, J., Liang, D., Zhang, Y., Feng, Y., and Hopke, P. K.: Dispersion Normalized PMF Provides Insights into the Significant Changes in Source Contributions to PM2.5 after the COVID-19 Outbreak, Environ. Sci. Technol., 54, 9917–9927, https://doi.org/10.1021/acs.est.0c02776, 2020.
Diamond, M. S. and Wood, R.: Limited Regional Aerosol and Cloud Microphysical Changes Despite Unprecedented Decline in Nitrogen Oxide Pollution During the February 2020 COVID-19 Shutdown in China, Geophys. Res. Lett., 47, e2020GL088913, https://doi.org/10.1029/2020gl088913, 2020.
Dunlea, E. J., Herndon, S. C., Nelson, D. D., Volkamer, R. M., San Martini, F., Sheehy, P. M., Zahniser, M. S., Shorter, J. H., Wormhoudt, J. C., Lamb, B. K., Allwine, E. J., Gaffney, J. S., Marley, N. A., Grutter, M., Marquez, C., Blanco, S., Cardenas, B., Retama, A., Ramos Villegas, C. R., Kolb, C. E., Molina, L. T., and Molina, M. J.: Evaluation of nitrogen dioxide chemiluminescence monitors in a polluted urban environment, Atmos. Chem. Phys., 7, 2691–2704, https://doi.org/10.5194/acp-7-2691-2007, 2007.
Evensen, G.: Sequential data assimilation with a nonlinear quasi-geostrophic model using Monte Carlo methods to forecast error statistics, J. Geophys. Res.-Oceans, 99, 10143–10162, https://doi.org/10.1029/94JC00572, 1994.
Fan, C., Li, Y., Guang, J., Li, Z. Q., Elnashar, A., Allam, M., and de Leeuw, G.: The Impact of the Control Measures during the COVID-19 Outbreak on Air Pollution in China, Remote Sens., 12, 1613, https://doi.org/10.3390/rs12101613, 2020.
Fan, H., Zhao, C., Yang, Y., and Yang, X.: Spatio-Temporal Variations of the PM2.5/PM10 Ratios and Its Application to Air Pollution Type Classification in China, Front. Environ. Sci., 9, 692440, https://doi.org/10.3389/fenvs.2021.692440, 2021.
Feng, S., Jiang, F., Wang, H., Wang, H., Ju, W., Shen, Y., Zheng, Y., Wu, Z., and Ding, A.: NOx Emission Changes Over China During the COVID-19 Epidemic Inferred From Surface NO2 Observations, Geophys. Res. Lett., 47, e2020GL090080, https://doi.org/10.1029/2020gl090080, 2020.
Forster, P. M., Forster, H. I., Evans, M. J., Gidden, M. J., Jones, C. D., Keller, C. A., Lamboll, R. D., Le Quere, C., Rogelj, J., Rosen, D., Schleussner, C. F., Richardson, T. B., Smith, C. J., and Turnock, S. T.: Current and future global climate impacts resulting from COVID-19, Nat. Clim. Change, 10, 971–971, https://doi.org/10.1038/s41558-020-0883-0, 2020.
Granier, C., Lamarque, J., Mieville, A., Muller, J., Olivier, J., Orlando, J., Peters, J., Petron, G., Tyndall, G., and Wallens, S.: POET, a database of surface emissions of ozone precursors, http://www.aero.jussieu.fr/projet/ACCENT/POET.php (last access: 3 June 2023), 2005.
Hammer, M. S., van Donkelaar, A., Martin, R. V., McDuffie, E. E., Lyapustin, A., Sayer, A. M., Hsu, N. C., Levy, R. C., Garay, M. J., Kalashnikova, O. V., and Kahn, R. A.: Effects of COVID-19 lockdowns on fine particulate matter concentrations, Sci. Adv., 7, eabg7670, https://doi.org/10.1126/sciadv.abg7670, 2021.
Han, X. L. and Naeher, L. P.: A review of traffic-related air pollution exposure assessment studies in the developing world, Environ. Int., 32, 106–120, https://doi.org/10.1016/j.envint.2005.05.020, 2006.
Hauglustaine, D. A., Brasseur, G. P., Walters, S., Rasch, P. J., Muller, J. F., Emmons, L. K., and Carroll, C. A.: MOZART, a global chemical transport model for ozone and related chemical tracers 2. Model results and evaluation, J. Geophys. Res.-Atmos., 103, 28291–28335, https://doi.org/10.1029/98jd02398, 1998.
He, G. J., Pan, Y. H., and Tanaka, T.: The short-term impacts of COVID-19 lockdown on urban air pollution in China, Nat. Sustain, 3, 1005–1011, https://doi.org/10.1038/s41893-020-0581-y, 2020.
He, T.-L., Jones, D. B. A., Miyazaki, K., Bowman, K. W., Jiang, Z., Chen, X., Li, R., Zhang, Y., and Li, K.: Inverse modelling of Chinese NOx emissions using deep learning: integrating in situ observations with a satellite-based chemical reanalysis, Atmos. Chem. Phys., 22, 14059–14074, https://doi.org/10.5194/acp-22-14059-2022, 2022.
Hu, Y., Zang, Z., Ma, X., Li, Y., Liang, Y., You, W., Pan, X., and Li, Z.: Four-dimensional variational assimilation for SO2 emission and its application around the COVID-19 lockdown in the spring 2020 over China, Atmos. Chem. Phys., 22, 13183–13200, https://doi.org/10.5194/acp-22-13183-2022, 2022.
Huang, X., Ding, A., Gao, J., Zheng, B., Zhou, D., Qi, X., Tang, R., Wang, J., Ren, C., Nie, W., Chi, X., Xu, Z., Chen, L., Li, Y., Che, F., Pang, N., Wang, H., Tong, D., Qin, W., Cheng, W., Liu, W., Fu, Q., Liu, B., Chai, F., Davis, S. J., Zhang, Q., and He, K.: Enhanced secondary pollution offset reduction of primary emissions during COVID-19 lockdown in China, Natl. Sci. Rev., 8, nwaa137, https://doi.org/10.1093/nsr/nwaa137, 2021.
Janssens-Maenhout, G., Crippa, M., Guizzardi, D., Dentener, F., Muntean, M., Pouliot, G., Keating, T., Zhang, Q., Kurokawa, J., Wankmüller, R., Denier van der Gon, H., Kuenen, J. J. P., Klimont, Z., Frost, G., Darras, S., Koffi, B., and Li, M.: HTAP_v2.2: a mosaic of regional and global emission grid maps for 2008 and 2010 to study hemispheric transport of air pollution, Atmos. Chem. Phys., 15, 11411–11432, https://doi.org/10.5194/acp-15-11411-2015, 2015.
Kaiser, J. W., Heil, A., Andreae, M. O., Benedetti, A., Chubarova, N., Jones, L., Morcrette, J.-J., Razinger, M., Schultz, M. G., Suttie, M., and van der Werf, G. R.: Biomass burning emissions estimated with a global fire assimilation system based on observed fire radiative power, Biogeosciences, 9, 527–554, https://doi.org/10.5194/bg-9-527-2012, 2012.
Kong, L., Tang, X., Zhu, J., Wang, Z., Pan, Y., Wu, H., Wu, L., Wu, Q., He, Y., Tian, S., Xie, Y., Liu, Z., Sui, W., Han, L., and Carmichael, G.: Improved Inversion of Monthly Ammonia Emissions in China Based on the Chinese Ammonia Monitoring Network and Ensemble Kalman Filter, Environ. Sci. Technol., 53, 12529–12538, https://doi.org/10.1021/acs.est.9b02701, 2019.
Kong, L., Tang, X., Zhu, J., Wang, Z., Li, J., Wu, H., Wu, Q., Chen, H., Zhu, L., Wang, W., Liu, B., Wang, Q., Chen, D., Pan, Y., Song, T., Li, F., Zheng, H., Jia, G., Lu, M., Wu, L., and Carmichael, G. R.: A 6-year-long (2013–2018) high-resolution air quality reanalysis dataset in China based on the assimilation of surface observations from CNEMC, Earth Syst. Sci. Data, 13, 529–570, https://doi.org/10.5194/essd-13-529-2021, 2021.
Lamsal, L. N., Martin, R. V., van Donkelaar, A., Steinbacher, M., Celarier, E. A., Bucsela, E., Dunlea, E. J., and Pinto, J. P.: Ground-level nitrogen dioxide concentrations inferred from the satellite-borne Ozone Monitoring Instrument, J. Geophys. Res.-Atmos., 113, D16308, https://doi.org/10.1029/2007JD009235, 2008.
Le, T. H., Wang, Y., Liu, L., Yang, J. N., Yung, Y. L., Li, G. H., and Seinfeld, J. H.: Unexpected air pollution with marked emission reductions during the COVID-19 outbreak in China, Science, 369, 702–706, https://doi.org/10.1126/science.abb7431, 2020.
Levelt, P. F., Stein Zweers, D. C., Aben, I., Bauwens, M., Borsdorff, T., De Smedt, I., Eskes, H. J., Lerot, C., Loyola, D. G., Romahn, F., Stavrakou, T., Theys, N., Van Roozendael, M., Veefkind, J. P., and Verhoelst, T.: Air quality impacts of COVID-19 lockdown measures detected from space using high spatial resolution observations of multiple trace gases from Sentinel-5P/TROPOMI, Atmos. Chem. Phys., 22, 10319–10351, https://doi.org/10.5194/acp-22-10319-2022, 2022.
Li, B., Fan, J., Han, L., Sun, G., Zhang, D., and Zhang, P.: An Industrial Heat Source Extraction Method: BP Neural Network Using Temperature Feature Template, Journal of Geo-Information Science, 24, 533–545, 2022 (in Chinese with English abstract).
Li, F., Tang, X., Wang, Z., Zhu, L., Wang, X., Wu, H., Lu, M., Li, J., and Zhu, J.: Estimation of Representative Errors of Surface Observations of Air Pollutant Concentrations Based on High-Density Observation Network over Beijing-Tianjin-Hebei Region, Chinese Journal of Atmospheric Sciences, 43, 277–284, 2019.
Li, L., Li, Q., Huang, L., Wang, Q., Zhu, A., Xu, J., Liu, Z., Li, H., Shi, L., Li, R., Azari, M., Wang, Y., Zhang, X., Liu, Z., Zhu, Y., Zhang, K., Xue, S., Ooi, M. C. G., Zhang, D., and Chan, A.: Air quality changes during the COVID-19 lockdown over the Yangtze River Delta Region: An insight into the impact of human activity pattern changes on air pollution variation, Sci. Total Environ., 732, 139282, https://doi.org/10.1016/j.scitotenv.2020.139282, 2020.
Li, M., Liu, H., Geng, G. N., Hong, C. P., Liu, F., Song, Y., Tong, D., Zheng, B., Cui, H. Y., Man, H. Y., Zhang, Q., and He, K. B.: Anthropogenic emission inventories in China: a review, Natl. Sci. Rev., 4, 834–866, https://doi.org/10.1093/nsr/nwx150, 2017a.
Li, M., Zhang, Q., Kurokawa, J.-I., Woo, J.-H., He, K., Lu, Z., Ohara, T., Song, Y., Streets, D. G., Carmichael, G. R., Cheng, Y., Hong, C., Huo, H., Jiang, X., Kang, S., Liu, F., Su, H., and Zheng, B.: MIX: a mosaic Asian anthropogenic emission inventory under the international collaboration framework of the MICS-Asia and HTAP, Atmos. Chem. Phys., 17, 935–963, https://doi.org/10.5194/acp-17-935-2017, 2017b.
Li, M., Zhang, Q., Zheng, B., Tong, D., Lei, Y., Liu, F., Hong, C., Kang, S., Yan, L., Zhang, Y., Bo, Y., Su, H., Cheng, Y., and He, K.: Persistent growth of anthropogenic non-methane volatile organic compound (NMVOC) emissions in China during 1990–2017: drivers, speciation and ozone formation potential, Atmos. Chem. Phys., 19, 8897–8913, https://doi.org/10.5194/acp-19-8897-2019, 2019.
Li, M., Wang, T., Xie, M., Li, S., Zhuang, B., Fu, Q., Zhao, M., Wu, H., Liu, J., Saikawa, E., and Liao, K.: Drivers for the poor air quality conditions in North China Plain during the COVID-19 outbreak, Atmos. Environ., 246, 118103, https://doi.org/10.1016/j.atmosenv.2020.118103, 2021.
Li, X., Zhang, Q., Zhang, Y., Zhang, L., Wang, Y. X., Zhang, Q. Q., Li, M., Zheng, Y. X., Geng, G. N., Wallington, T. J., Han, W. J., Shen, W., and He, K. B.: Attribution of PM2.5 exposure in Beijing-Tianjin-Hebei region to emissions: implication to control strategies, Sci. Bull., 62, 957–964, https://doi.org/10.1016/j.scib.2017.06.005, 2017.
Ma, C. Q., Wang, T. J., Mizzi, A. P., Anderson, J. L., Zhuang, B. L., Xie, M., and Wu, R. S.: Multiconstituent Data Assimilation With WRF-Chem/DART: Potential for Adjusting Anthropogenic Emissions and Improving Air Quality Forecasts Over Eastern China, J. Geophys. Res.-Atmos., 124, 7393–7412, https://doi.org/10.1029/2019jd030421, 2019.
Ma, T., Duan, F. K., Ma, Y. L., Zhang, Q. Q., Xu, Y. Z., Li, W. G., Zhu, L. D., and He, K. B.: Unbalanced emission reductions and adverse meteorological conditions facilitate the formation of secondary pollutants during the COVID-19 lockdown in Beijing, Sci. Total Environ., 838, 155970, https://doi.org/10.1016/j.scitotenv.2022.155970, 2022.
Miyazaki, K., Eskes, H. J., Sudo, K., Takigawa, M., van Weele, M., and Boersma, K. F.: Simultaneous assimilation of satellite NO2, O3, CO, and HNO3 data for the analysis of tropospheric chemical composition and emissions, Atmos. Chem. Phys., 12, 9545–9579, https://doi.org/10.5194/acp-12-9545-2012, 2012.
Okuda, T., Matsuura, S., Yamaguchi, D., Umemura, T., Hanada, E., Orihara, H., Tanaka, S., He, K., Ma, Y., Cheng, Y., and Liang, L.: The impact of the pollution control measures for the 2008 Beijing Olympic Games on the chemical composition of aerosols, Atmos. Environ., 45, 2789–2794, https://doi.org/10.1016/j.atmosenv.2011.01.053, 2011.
Peng, Z., Lei, L., Liu, Z., Sun, J., Ding, A., Ban, J., Chen, D., Kou, X., and Chu, K.: The impact of multi-species surface chemical observation assimilation on air quality forecasts in China, Atmos. Chem. Phys., 18, 17387–17404, https://doi.org/10.5194/acp-18-17387-2018, 2018.
Price, C., Penner, J., and Prather, M.: NOx from lightning .1. Global distribution based on lightning physics, J. Geophys. Res.-Atmos., 102, 5929–5941, https://doi.org/10.1029/96jd03504, 1997.
Randerson, J. T., Van Der Werf, G. R., Giglio, L., Collatz, G. J., and Kasibhatla, P. S.: Global Fire Emissions Database, Version 4.1 (GFEDv4), Oak Ridge, Tennessee, USA, ORNL DAAC [data set], https://doi.org/10.3334/ORNLDAAC/1293, 2017.
Sakov, P. and Oke, P. R.: A deterministic formulation of the ensemble Kalman filter: an alternative to ensemble square root filters, Tellus A, 60, 361–371, https://doi.org/10.1111/j.1600-0870.2007.00299.x, 2008.
Skachko, S., Errera, Q., Ménard, R., Christophe, Y., and Chabrillat, S.: Comparison of the ensemble Kalman filter and 4D-Var assimilation methods using a stratospheric tracer transport model, Geosci. Model Dev., 7, 1451–1465, https://doi.org/10.5194/gmd-7-1451-2014, 2014.
Shi, X. and Brasseur, G. P.: The Response in Air Quality to the Reduction of Chinese Economic Activities During the COVID-19 Outbreak, Geophys. Res. Lett., 47, e2020GL088070, https://doi.org/10.1029/2020gl088070, 2020.
Shi, Z., Song, C., Liu, B., Lu, G., Xu, J., Van Vu, T., Elliott, R. J. R., Li, W., Bloss, W. J., and Harrison, R. M.: Abrupt but smaller than expected changes in surface air quality attributable to COVID-19 lockdowns, Sci. Adv., 7, eabd6696, https://doi.org/10.1126/sciadv.abd6696, 2021.
Sindelarova, K., Granier, C., Bouarar, I., Guenther, A., Tilmes, S., Stavrakou, T., Müller, J.-F., Kuhn, U., Stefani, P., and Knorr, W.: Global data set of biogenic VOC emissions calculated by the MEGAN model over the last 30 years, Atmos. Chem. Phys., 14, 9317–9341, https://doi.org/10.5194/acp-14-9317-2014, 2014.
Skamarock, W. C.: A description of the advanced research WRF version 3, Ncar Technical, 113, 7–25, 2008.
Song, Y. S., Lin, C. Q., Li, Y., Lau, A. K. H., Fung, J. C. H., Lu, X. C., Guo, C., Ma, J., and Lao, X. Q.: An improved decomposition method to differentiate meteorological and anthropogenic effects on air pollution: A national study in China during the COVID-19 lockdown period, Atmos. Environ., 250, 118270, https://doi.org/10.1016/j.atmosenv.2021.118270, 2021.
Streets, D. G., Bond, T. C., Carmichael, G. R., Fernandes, S. D., Fu, Q., He, D., Klimont, Z., Nelson, S. M., Tsai, N. Y., Wang, M. Q., Woo, J. H., and Yarber, K. F.: An inventory of gaseous and primary aerosol emissions in Asia in the year 2000, J. Geophys. Res.-Atmos., 108, 8809, https://doi.org/10.1029/2002JD003093, 2003.
Streets, D. G., Canty, T., Carmichael, G. R., de Foy, B., Dickerson, R. R., Duncan, B. N., Edwards, D. P., Haynes, J. A., Henze, D. K., Houyoux, M. R., Jacob, D. J., Krotkov, N. A., Lamsal, L. N., Liu, Y., Lu, Z., Martin, R. V., Pfister, G. G., Pinder, R. W., Salawitch, R. J., and Wecht, K. J.: Emissions estimation from satellite retrievals: A review of current capability, Atmos. Environ., 77, 1011–1042, https://doi.org/10.1016/j.atmosenv.2013.05.051, 2013.
Sulaymon, I. D., Zhang, Y., Hopke, P. K., Hu, J., Zhang, Y., Li, L., Mei, X., Gong, K., Shi, Z., Zhao, B., and Zhao, F.: Persistent high PM2.5 pollution driven by unfavorable meteorological conditions during the COVID-19 lockdown period in the Beijing-Tianjin-Hebei region, China, Environ. Res., 198, 111186, https://doi.org/10.1016/j.envres.2021.111186, 2021.
Tandeo, P., Ailliot, P., Bocquet, M., Carrassi, A., Miyoshi, T., Pulido, M., and Zhen, Y. C.: A Review of Innovation-Based Methods to Jointly Estimate Model and Observation Error Covariance Matrices in Ensemble Data Assimilation, Mon. Weather Rev., 148, 3973–3994, https://doi.org/10.1175/mwr-d-19-0240.1, 2020.
Tang, G., Zhu, X., Hu, B., Xin, J., Wang, L., Münkel, C., Mao, G., and Wang, Y.: Impact of emission controls on air quality in Beijing during APEC 2014: lidar ceilometer observations, Atmos. Chem. Phys., 15, 12667–12680, https://doi.org/10.5194/acp-15-12667-2015, 2015.
Tang, X., Zhu, J., Wang, Z. F., and Gbaguidi, A.: Improvement of ozone forecast over Beijing based on ensemble Kalman filter with simultaneous adjustment of initial conditions and emissions, Atmos. Chem. Phys., 11, 12901–12916, https://doi.org/10.5194/acp-11-12901-2011, 2011.
Tang, X., Zhu, J., Wang, Z. F., Wang, M., Gbaguidi, A., Li, J., Shao, M., Tang, G. Q., and Ji, D. S.: Inversion of CO emissions over Beijing and its surrounding areas with ensemble Kalman filter, Atmos. Environ., 81, 676–686, https://doi.org/10.1016/j.atmosenv.2013.08.051, 2013.
van der Werf, G. R., Randerson, J. T., Giglio, L., Collatz, G. J., Mu, M., Kasibhatla, P. S., Morton, D. C., DeFries, R. S., Jin, Y., and van Leeuwen, T. T.: Global fire emissions and the contribution of deforestation, savanna, forest, agricultural, and peat fires (1997–2009), Atmos. Chem. Phys., 10, 11707–11735, https://doi.org/10.5194/acp-10-11707-2010, 2010.
Wang, P., Chen, K., Zhu, S., Wang, P., and Zhang, H.: Severe air pollution events not avoided by reduced anthropogenic activities during COVID-19 outbreak, Resour. Conserv. Recy., 158, 104814, https://doi.org/10.1016/j.resconrec.2020.104814, 2020.
Wang, S., Gao, J., Zhang, Y., Zhang, J., Cha, F., Wang, T., Ren, C., and Wang, W.: Impact of emission control on regional air quality: An observational study of air pollutants before, during and after the Beijing Olympic Games, J. Environ. Sci., 26, 175–180, https://doi.org/10.1016/S1001-0742(13)60395-2, 2014.
Wang, Y. Q., Zhang, X. Y., Sun, J. Y., Zhang, X. C., Che, H. Z., and Li, Y.: Spatial and temporal variations of the concentrations of PM10, PM2.5 and PM1 in China, Atmos. Chem. Phys., 15, 13585–13598, https://doi.org/10.5194/acp-15-13585-2015, 2015.
Wang, Z., Uno, I., Yumimoto, K., Itahashi, S., Chen, X., Yang, W., and Wang, Z.: Impacts of COVID-19 lockdown, Spring Festival and meteorology on the NO2 variations in early 2020 over China based on in-situ observations, satellite retrievals and model simulations, Atmos. Environ., 244, 117972, https://doi.org/10.1016/j.atmosenv.2020.117972, 2021.
Wu, H., Tang, X., Wang, Z., Wu, L., Li, J., Wang, W., Yang, W., and Zhu, J.: High-spatiotemporal-resolution inverse estimation of CO and NOx emission reductions during emission control periods with a modified ensemble Kalman filter, Atmos. Environ., 236, 117631, https://doi.org/10.1016/j.atmosenv.2020.117631, 2020.
Wu, H. J., Tang, X., Wang, Z. F., Wu, L., Lu, M. M., Wei, L. F., and Zhu, J.: Probabilistic Automatic Outlier Detection for Surface Air Quality Measurements from the China National Environmental Monitoring Network, Adv. Atmos. Sci., 35, 1522–1532, https://doi.org/10.1007/s00376-018-8067-9, 2018.
Xing, J., Li, S., Jiang, Y., Wang, S., Ding, D., Dong, Z., Zhu, Y., and Hao, J.: Quantifying the emission changes and associated air quality impacts during the COVID-19 pandemic on the North China Plain: a response modeling study, Atmos. Chem. Phys., 20, 14347–14359, https://doi.org/10.5194/acp-20-14347-2020, 2020.
Yan, C., Shen, Y., Stolzenburg, D., Dada, L., Qi, X., Hakala, S., Sundström, A.-M., Guo, Y., Lipponen, A., Kokkonen, T. V., Kontkanen, J., Cai, R., Cai, J., Chan, T., Chen, L., Chu, B., Deng, C., Du, W., Fan, X., He, X.-C., Kangasluoma, J., Kujansuu, J., Kurppa, M., Li, C., Li, Y., Lin, Z., Liu, Y., Liu, Y., Lu, Y., Nie, W., Pulliainen, J., Qiao, X., Wang, Y., Wen, Y., Wu, Y., Yang, G., Yao, L., Yin, R., Zhang, G., Zhang, S., Zheng, F., Zhou, Y., Arola, A., Tamminen, J., Paasonen, P., Sun, Y., Wang, L., Donahue, N. M., Liu, Y., Bianchi, F., Daellenbach, K. R., Worsnop, D. R., Kerminen, V.-M., Petäjä, T., Ding, A., Jiang, J., and Kulmala, M.: The effect of COVID-19 restrictions on atmospheric new particle formation in Beijing, Atmos. Chem. Phys., 22, 12207–12220, https://doi.org/10.5194/acp-22-12207-2022, 2022.
Yan, X. Y., Akimoto, H., and Ohara, T.: Estimation of nitrous oxide, nitric oxide and ammonia emissions from croplands in East, Southeast and South Asia, Glob. Change Biol., 9, 1080–1096, https://doi.org/10.1046/j.1365-2486.2003.00649.x, 2003.
Zhang, L., Shao, J., Lu, X., Zhao, Y., Hu, Y., Henze, D. K., Liao, H., Gong, S., and Zhang, Q.: Sources and Processes Affecting Fine Particulate Matter Pollution over North China: An Adjoint Analysis of the Beijing APEC Period, Environ. Sci. Technol., 50, 8731–8740, https://doi.org/10.1021/acs.est.6b03010, 2016.
Zhang, Q., Pan, Y., He, Y., Walters, W. W., Ni, Q., Liu, X., Xu, G., Shao, J., and Jiang, C.: Substantial nitrogen oxides emission reduction from China due to COVID-19 and its impact on surface ozone and aerosol pollution, Sci. Total Environ., 753, 142238, https://doi.org/10.1016/j.scitotenv.2020.142238, 2021.
Zhang, R. X., Zhang, Y. Z., Lin, H. P., Feng, X., Fu, T. M., and Wang, Y. H.: NOx Emission Reduction and Recovery during COVID-19 in East China, Atmosphere, 11, 433, https://doi.org/10.3390/atmos11040433, 2020.
Zhang, W., Guo, J. H., Sun, Y. L., Yuan, H., Zhuang, G. S., Zhuang, Y. H., and Hao, Z. P.: Source apportionment for urban PM10 and PM2.5 in the Beijing area, Chin. Sci. Bull., 52, 608–615, https://doi.org/10.1007/s11434-007-0076-5, 2007.
Zhao, X., Wang, G., Wang, S., Zhao, N., Zhang, M., and Yue, W.: Impacts of COVID-19 on air quality in mid-eastern China: An insight into meteorology and emissions, Atmos. Environ., 266, 118750, https://doi.org/10.1016/j.atmosenv.2021.118750, 2021.
Zhao, Y. B., Zhang, K., Xu, X. T., Shen, H. Z., Zhu, X., Zhang, Y. X., Hu, Y. T., and Shen, G. F.: Substantial Changes in Nitrogen Dioxide and Ozone after Excluding Meteorological Impacts during the COVID-19 Outbreak in Mainland China, Environ. Sci. Tech. Lett., 7, 402–408, https://doi.org/10.1021/acs.estlett.0c00304, 2020.
Zheng, B., Zhang, Q., Geng, G., Chen, C., Shi, Q., Cui, M., Lei, Y., and He, K.: Changes in China's anthropogenic emissions and air quality during the COVID-19 pandemic in 2020, Earth Syst. Sci. Data, 13, 2895–2907, https://doi.org/10.5194/essd-13-2895-2021, 2021.
Zheng, B., Tong, D., Li, M., Liu, F., Hong, C., Geng, G., Li, H., Li, X., Peng, L., Qi, J., Yan, L., Zhang, Y., Zhao, H., Zheng, Y., He, K., and Zhang, Q.: Trends in China's anthropogenic emissions since 2010 as the consequence of clean air actions, Atmos. Chem. Phys., 18, 14095–14111, https://doi.org/10.5194/acp-18-14095-2018, 2018.
Zuo, P. J., Zong, Z., Zheng, B., Bi, J. Z., Zhang, Q. H., Li, W., Zhang, J. W., Yang, X. Z., Chen, Z. G., Yang, H., Lu, D. W., Zhang, Q. H., Liu, Q., and Jiang, G. B.: New Insights into Unexpected Severe PM2.5 Pollution during the SARS and COVID-19 Pandemic Periods in Beijing, Environ. Sci. Technol., 56, 155–164, https://doi.org/10.1021/acs.est.1c05383, 2022.