the Creative Commons Attribution 4.0 License.
the Creative Commons Attribution 4.0 License.
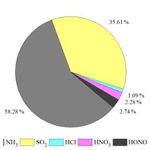
Chloride (HCl ∕ Cl−) dominates inorganic aerosol formation from ammonia in the Indo-Gangetic Plain during winter: modeling and comparison with observations
Pooja V. Pawar
Sachin D. Ghude
Gaurav Govardhan
Prodip Acharja
Rachana Kulkarni
Rajesh Kumar
Baerbel Sinha
Vinayak Sinha
Chinmay Jena
Preeti Gunwani
Tapan Kumar Adhya
Eiko Nemitz
Mark A. Sutton
The Winter Fog Experiment (WiFEX) was an intensive field campaign conducted at Indira Gandhi International Airport (IGIA) Delhi, India, in the Indo-Gangetic Plain (IGP) during the winter of 2017–2018. Here, we report the first comparison in South Asia of high-temporal-resolution simulation of ammonia (NH3) along with ammonium (NH) and total NHx (i.e., NH3+ NH) using the Weather Research and Forecasting model coupled with chemistry (WRF-Chem) and measurements made using the Monitor for AeRosols and Gases in Ambient Air (MARGA) at the WiFEX research site. In the present study, we incorporated the Model for Simulating Aerosol Interactions and Chemistry (MOSAIC) aerosol scheme into WRF-Chem. Despite simulated total NHx values and variability often agreeing well with the observations, the model frequently simulated higher NH3 and lower NH concentrations than the observations. Under the winter conditions of high relative humidity (RH) in Delhi, hydrogen chloride (HCl) was found to promote the increase in the particle fraction of NH (which accounted for 49.5 % of the resolved aerosol in equivalent units), with chloride (Cl−) (29.7 %) as the primary anion. By contrast, the absence of chloride (HCl Cl−) chemistry in the standard WRF-Chem model results in the prediction of sulfate (SO) as the dominant inorganic aerosol anion. To understand the mismatch associated with the fraction of NHx in the particulate phase (NH NHx), we added HCl Cl− to the model and evaluated the influence of its chemistry by conducting three sensitivity experiments using the model: no HCl, base case HCl (using a published waste burning inventory), and 3 × base HCl run. We found that 3 × base HCl increased the simulated average NH by 13.1 µg m−3 and NHx by 9.8 µg m−3 concentration while reducing the average NH3 by 3.2 µg m−3, which is more in accord with the measurements. Thus HCl Cl− chemistry in the model increases total NHx concentration, which was further demonstrated by reducing NH3 emissions by a factor of 3 (−3 × NH3_EMI) in the 3 × base HCl simulation. Reducing NH3 emissions in the 3 × base HCl simulation successfully addressed the discrepancy between measured and modeled total NHx. We conclude that modeling the fate of NH3 in Delhi requires a correct chemistry mechanism accounting for chloride dynamics with accurate inventories of both NH3 and HCl emissions.
- Article
(3866 KB) - Full-text XML
-
Supplement
(1035 KB) - BibTeX
- EndNote
The Indo-Gangetic Plain (IGP) is one of the global hotspots of atmospheric ammonia (NH3) and faces a range of environmental challenges, particularly during the winter season, including adverse air pollution episodes, especially as NH3 plays a substantial role in secondary aerosol formation (Ghude et al., 2020, 2008a, b; Kumar et al., 2021; Saraswati et al., 2019; Sharma et al., 2020; Singh et al., 2021). Atmospheric NH3, along with oxides of nitrogen (NOx), together accounts for the largest source of reactive nitrogen (Nr), which is primarily emitted by agricultural activities, livestock population, industrial activities, and transportation (Ghude et al., 2009, 2010, 2012, 2013; Móring et al., 2021; Pawar et al., 2021). Ammonia in the environment plays a crucial role in atmospheric chemistry and the eutrophication and acidification of ecosystems (Datta et al., 2012; Pawar et al., 2021; Sharma et al., 2008, 2012, 2014b). Control of NH3 becomes a key priority in an emerging international strategy to manage the global nitrogen cycle (Gu et al., 2021; Sutton et al., 2020). Ammonia is one of the important aerosol precursor gases, and ammonium (NH) is a major counter ion for the three anions such as chloride (Cl−), nitrate (NO), and sulfate (SO) contributing to PM2.5 composition (Seinfeld et al., 2016). In addition, as the dominant alkaline gas in the atmosphere, NH3 has attracted the interest of scientific researchers since it has been known to promote new aerosol formation, both in the initial homogeneous nucleation and in the subsequent growth, especially during wintertime (Acharja et al., 2020, 2021; Ali et al., 2019; Duan et al., 2021; Wagh et al., 2021).
In this study, we focus on wintertime since this season is characterized by low-to-dense fog events, lower temperature (T), and variability of relative humidity (RH), which fluctuates from 40 % to 100 % (Ghude et al., 2017; Kumar et al., 2020). Ammonia acts as a neutralization agent for determining the acidity of aerosol particles (Acharja et al., 2020; Ali et al., 2019; Ghude et al., 2017). It also affects PM2.5, the acidity of clouds, and the wet deposition of nitrogen by neutralizing acidic species (Gu et al., 2021; Xu et al., 2020). Increasing NH3 concentration over Delhi compared with the surrounding area leads to an increase in PM2.5 concentrations (Ghude et al., 2022; Sharma et al., 2008, 2012, 2014a), which in turn affects air quality, human health, and climate (Behera et al., 2013; Ghude, 2016; Ghude et al., 2008b; Nivdange et al., 2022; Sutton et al., 2017; Sutton and Howard, 2018).
Satellite observations (Van Damme et al., 2018; Warner et al., 2017), chemical transport models (CTMs) (Clarisse et al., 2009, 2010; T. Wang et al., 2020), and ground-based observations (Pawar et al., 2021) revealed that the IGP is the largest regional hotspot of NH3 concentrations on Earth. Previous studies have identified various sources of NH3, for example, agricultural activities, industrial sectors, motor vehicles, garbage, sewage, and urine from rural populations at the global scale (Behera et al., 2013; Huang et al., 2012; Sutton et al., 2008). However, in Delhi, agricultural activity (including surrounding arable and suburban livestock farming) is estimated to be the dominant source of NH3, along with traffic emissions (Kuttippurath et al., 2020; Móring et al., 2021; Sharma et al., 2020), but its emissions are subject to large uncertainty. Globally, various modeling efforts have investigated the relative effectiveness of reducing NH3 emissions in curtailing PM2.5 formation (Gu et al., 2021; Pinder et al., 2007, 2008; Zhang et al., 2020). However, over India, the impact on reducing PM2.5 might be limited because NH3 emission reductions may be more challenging due to its diverse and area-wide sources. Ianniello et al. (2010) and Lan et al. (2021) have investigated the variation of atmospheric NH3 at an urban and suburban site of Beijing with respect to meteorological factors, where RH was found to be a strong factor in influencing the NH3 mixing ratio. A few studies over Asia have highlighted the gas-to-particle conversion of NH3 in Delhi (Acharja et al., 2021; Saraswati et al., 2019) and China and its subsequent impact on the aerosol formation (Wang et al., 2015; Xu et al., 2020). Furthermore, excess NH3 during fog can also enhance secondary aerosol formation in Delhi during winter (Acharja et al., 2021). However, the wintertime behavior of NH3 in Delhi in CTMs has not yet been investigated and remains poorly understood (Ellis et al., 2011; Metzger et al., 2006). In a recent study, Pawar et al. (2021) highlighted uncertainties associated with gas-to-particle partitioning of NH3 in a global model (Model for Ozone And Related chemical Tracers, MOZART-4) and found a significant overestimation of NH3 in the model compared with the measurements. The overestimation of NH3 in the model led the authors to hypothesize that a source-specific NH3 emission inventory in India, considering agricultural statistics on fertilizer use and animal distribution, was missing. Also, there was a need for a high-resolution regional model with advanced chemistry to resolve the NH3 emissions on the local scale.
The present study utilizes the regional Weather Research and Forecasting model coupled with chemistry (WRF-Chem) interpreted using measurements from the Winter fog Experiment (WiFEX), including NH3, water-soluble ions in PM2.5, other trace gases, and meteorological parameters during December–January 2017–2018. For the first time in South Asia, we discuss and compare the modeled and observed temporal variation in gaseous NH3, particulate NH, and total NHx (i.e., NH3+ NH). Since we found that the total modeled NHx matches well with the observations, we investigate the ability of the model to accurately describe the gas-to-particle partitioning of the measurements (Monitor for AeRosols and Gases in Ambient Air, MARGA) by evaluating the fraction of NHx in the particulate phase (NH NHx). We conducted several sensitivity experiments with and without adding anthropogenic waste burning emissions of hydrochloric acid (HCl) in the model. The updated model with HCl Cl− chemistry was used to analyze and compare the temporal variation of NH3, NH, and total NHx from the WiFEX measurements.
2.1 Observational datasets
2.1.1 Description of MARGA
In the present study, we used the same dataset which was previously published by Acharja et al. (2020, 2021), which described the aerosol time series and chemistry measured with a Monitor for AeRosols and Gases in Ambient Air, model 2S instrument (MARGA). The MARGA system has two channels, one for sampling PM1 and the other for sampling PM2.5 for ground-based observations. The MARGA system (two sampling boxes, analytical box, and connected pumps) was located inside Indira Gandhi International Airport (IGIA), Delhi (28.56∘ N, 77.09∘ E), with the inlet PM1 and PM2.5 impactors fixed on the terrace with 2 m long inlet lines sampling outdoor air at 8 m above ground and 2 m above the rooftop. Measurements covered a winter period (19 December 2017 to 21 January 2018) with frequent moderate to dense fog events. Following intake through the PM1 and PM2.5 impactors, the air was passed through two parallel inlet tubes 2 m long and 14 mm inner diameter polytetrafluoroethylene (PTFE) to the PM1 and PM2.5 sampling channels of the MARGA. The air flow rate in each MARGA sampling box is regulated to a volumetric flow of 1 m3 h−1. The measurements are close to being real-time, as two sets of syringes are employed to collect the samples in which a set of syringes collects the sample, and another set sends the collected samples from the previous hour for analysis. Each MARGA sampling system consists of a steam jet aerosol collector (SJAC) and a wet rotating denuder (WRD) for collecting and measuring water-soluble inorganic particulate species and gases in the ambient air. The continuous coating of the WRD by a thin film of absorption solution (10 ppm hydrogen peroxide (H2O2)) allows for the diffusion of gases into the absorption solution. By contrast, the low diffusion velocity of submicron particles restricts the ability of water-soluble aerosols to diffuse into the absorption solution. The absorption solution is continually changed to replace that abstracted for ion chromatography (IC) analysis of the dissolved gases. The air stream, depleted of gases by the WRD, subsequently enters the SJAC, where the steam enhances water-soluble aerosols to grow, allowing for their mechanical capture in a cyclone. The aqueous solutions deriving from two cyclones (for PM1 and PM2.5, respectively) are then supplied to the IC for chemical analysis (Acharja et al., 2020).
Ambient surface concentrations of NH3 along with other trace gases (HCl, nitrous acid (HONO), nitric acid (HNO3), and sulfur dioxide (SO2)) and water-soluble inorganic components of PM1 and PM2.5 (Cl−, nitrate (NO), SO, NH, sodium (Na+), potassium (K+), magnesium (Mg2+), and calcium (Ca2+) were then quantified online by anion and cation chromatography in the analytical box at an hourly resolution. We have only used PM2.5 inorganic water-soluble components and the gaseous measurements (available from both the PM1 and PM2.5 MARGA collection systems). Since NH with the three major anions, Cl−, NO, and SO, constituted 97.3 % of the total measured ions in PM2.5 (Acharja et al., 2020), we consider these four significant ions in our present study. In contrast, the remaining ionic species (i.e., Na+, K+, Mg2+, and Ca2+) contributed only about 3 % of the total measured ions and were neglected as it would not impact our present study significantly (Acharja et al., 2020). Anions are separated in a Metrosep A Supp 10 () column with sodium carbonate (Na2CO3) and sodium bicarbonate (NaHCO3) ( mmol L−1) eluent, whereas for cation separation, a Metrosep C4 () cation column with 3.2 mmol L−1 HNO3 eluent was used (Acharja et al., 2020). To suppress the eluent background conductivity of anion chromatographs, three ion exchange units were used to ensure that the ion exchange unit is regenerated in each analysis. For this purpose, 1 M phosphoric acid (H3PO4) was used. This was performed to improve the signal-to-noise (S N) of the anion chromatographs. Details of the MARGA instrument can be found in Makkonen et al. (2012), Thomas et al. (2009), and Twigg et al. (2015).
2.1.2 Quality assurance and quality control (QA/QC) of MARGA
To ensure the observation's accuracy and check the data's quality, we have followed best practices during the study. The eluents, absorption, and regenerant solutions were prepared with minimum manual intervention. The operational parameters like anion and cation conductivity, SJAC heater temperature, column oven temperature, and airflow were regularly monitored to keep them within the safe limit. In addition to these, before injection of each sample into the anion and cation IC columns, the lithium bromide (LiBr) internal standard solution containing 320 µg L−1 lithium (Li+) and 3680 µg L−1 bromide (Br−) was mixed with each sample to provide calibration of each analysis. This ensures that each analysis is calibrated, and the concentration of gaseous and ionic samples is measured accurately. The PM1 and PM2.5 impactors were typically cleaned fortnightly to remove any material that may have stuck on the surface and inlets of the impactors. The lower detection limits (LODs) of the species monitored by MARGA were mentioned in Acharja et al. (2021). It shows that concentrations of species like Cl−, NO, SO, NH, SO2, and NH3 were always higher than LODs during the winter period. Concentrations of species like Na+, K+, Ca2+, Mg2+, HCl, HONO, and HNO3 were sometimes below LODs, but the fraction of them was less than ∼ 10 % of the total observation period. We have omitted these values and treated them as not available (NA). As the fraction of observational hours is small, and these species contribute much less to the PM1 and PM2.5 mass concentrations, we believe values that are below the LODs would not significantly deviate our results. The quality of the data obtained was then checked using the ion-balance method. As an additional quality check, the ratio of the sum of cations to anions (nano equivalent per cubic meter – neq m−3) was used as an indicator for the viable data. We have checked the cation-to-anion ratio of each hourly sample expressed in units of neq m−3. We only accepted those values near to unity and rejected those not within the 10 % error bar limit. Based on this evaluation method, overall, for the campaign, the ratio was near unity (1.06 for PM1 and 0.96 for PM2.5). Excellent charge balance between anions and cations measured by the system also confirms that there are no significant contamination issues associated with the aerosol measurements. Values in slight excess of unity may indicate the presence of formate and acetate in the aerosol, which MARGA does not measure. Further details on the quality control of MARGA can be found in Acharja et al. (2020).
2.1.3 Other ground-based measurements
Hourly NOx measurements were made by the chemiluminescence method, and hourly ozone (O3) measurements were made by the UV photometric method (CPCB, 2011) at the nearest air quality monitoring station (AQMS) of IGIA, operated by the Central Pollution Control Board (CPCB). CPCB follows the United States Environmental Protection Agency (USEPA)-approved AC32M NOx and 42M O3 analyzer manufactured by Environment S.A. India Private Limited. We used 1 h monitored NOx and O3 values in our study. These air quality monitoring stations' quality control and assurance processes were followed as outlined in CPCB (2014, 2020). For data quality of CPCB, we omitted all those observed values which fell below the LOD of the instrument (2 µg m−3 for NOx and 4 µg m−3 for O3) (Technical specifications for CAAQM station, 2019) and above 500 µg m−3 for NOx and 140 µg m−3 for O3 and treated them as NA at a given site. For the NOx and O3 datasets, only a small fraction of data (2 %) were outside the instrument operating ranges specified. This step aims to remove any short-term local influence that the models cannot capture and retain the regional-scale variability because the nearest sites are located in the urban environment. We removed a single spike represented by a change of more than 100 µg m−3 in just 1 h for all the data in CPCB monitoring stations to filter out random fluctuations in the observations. We removed some very high NOx and O3 values that appeared in the time series right after measurement gaps. Meteorological parameters, including air temperature (T), relative humidity (RH), wind speed, and wind direction, were measured with the automatic weather station (AWS) platform on a 20 m flux tower (Ghude et al., 2017). For detailed information on the measurement site and its meteorological parameters, refer to Ali et al. (2019).
2.2 WRF-Chem v 3.9.1 model
The Weather Research and Forecasting model coupled with chemistry (WRF-Chem v3.9.1) was employed in this study to simulate atmospheric gases and aerosols over Delhi during the peak winter period, starting from 19 December 2017 to 21 January 2018. We recently used a similar model configuration to simulate the air quality over Delhi (Ghude et al., 2020; Kulkarni et al., 2020). This study used the Model for Ozone And Related chemical Tracers (MOZART-4) gas-phase chemical mechanism coupled with the Model for Simulating Aerosol Interactions and Chemistry (MOSAIC) aerosol scheme, which simulates SO, NH, NO, methanesulfonate, Na+, Ca2+, Cl−, carbonate, black carbon, and primary organic mass. Other inert minerals, trace elements, and inorganic species are lumped together as different inorganic masses. MOSAIC allows for gas-to-particle formation, which includes NH3, HCl, sulfuric acid (H2SO4), HNO3, and methane sulfonic acid (MSA) and also includes secondary organic aerosols (SOAs). Aerosol size distributions are represented by a sectional aerosol bin approach with four size bins (Georgiou et al., 2018). MOSAIC incorporates the thermodynamic and gas–particle partitioning module described by Zaveri et al. (2008). To reduce the computational cost, we selected a four-bin MOSAIC mechanism that simulates thermodynamic equilibrium and other aerosol processes such as condensation, coagulation, and nucleation. The same mechanism has been widely used with WRF-Chem for simulations outside India (Bucaram and Bowman, 2021; Sha et al., 2019; Yang et al., 2018), but only a limited number of studies have applied it to the Indian domain to include more detailed chemistry and species (Gupta and Mohan, 2015; Jena et al., 2020; Kumar et al., 2018). The SOA formation in MOSAIC is simulated using the volatility basis set approach (Knote et al., 2015). For consistency with the PM2.5 MARGA measurements, we have chosen three bins according to simulated aerosol size (0.04–0.156, 0.156–0.625, and 0.625–2.5 µm) in accordance with the WRF-Chem aerosol size distribution.
The model domain covers the entire northern region of India, but here model simulations are compared with the observations at IGIA, Delhi (28.56∘ N, 77.09∘ E). The domain was set with a horizontal grid spacing of 10 km in both the latitudinal and longitudinal directions. The model top vertical grid included 47 vertical levels, with the model top set to 10 hPa. The physical parameterization schemes of model configuration are the same as those described by Ghude et al. (2020) and Jena et al. (2021). EDGAR-HTAP (Emission Database for Global Atmospheric Research for Hemispheric Transport of Air Pollution) for the year 2010 at grid resolution was used in this study for anthropogenic emissions of aerosols and trace gases (PM2.5, PM10, organic carbon (OC), black carbon (BC), CO, and NOx, etc.) and is scaled to 2018 as per Jena et al. (2021). Biogenic emissions are calculated online using the Model of Emissions of Gases and Aerosols from Nature version 2.1 (MEGAN2.1) (Guenther et al., 2006), and dust emissions are based on the traditional Goddard Global Ozone Chemistry Aerosol Radiation and Transport (GOCART) dust scheme that works with MOSAIC (Ginoux et al., 2001). Fire INventory from NCAR (FINNv1.5, 2017) was used in this study for daily open biomass burning emissions that are vertically distributed within the model using Freitas et al. (2007). The chemical initial and lateral boundary conditions come from the global model simulations from the Model for Ozone and Related Chemical Tracers (MOZART-4), and the meteorological initial and lateral boundary conditions are provided from the fifth-generation European Centre for Medium-Range Weather Forecasts (ECMWF) atmospheric reanalysis of the global climate (ERA5) with 6-hourly temporal resolution. The simulations were reinitialized every fifth day to limit the growth of meteorological errors in our simulations, but the chemical fields were carried forward from the previous simulation.
3.1 Comparison of temporal variation in NH3, NH, and total NHx using WRF-Chem and MARGA
3.1.1 Diurnal variation
To investigate how well a state-of-the-art chemical transport model performs in capturing the diurnal behavior of NH3 and NH, we compared observed and model-simulated diurnal profiles of NH3 and NH. Figure 1 displays the comparison of diurnal variation (00:00 to 23:00 Indian standard time (IST)) in meteorological parameters (T and RH) at the IGIA site in Delhi (Fig. 1a) along with NH3 and NH averaged over the study period (Fig. 1b) between observations and the model. We adopted diurnal variation in emissions from a recent study by Jena et al. (2021). Note that diurnal variability in the model simulations is primarily controlled by the planetary boundary layer mixing. We first investigated the ability of WRF-Chem to accurately predict the meteorological parameters of RH and T, which are important determinants of the gas-to-aerosol partitioning of (semi-)volatile compounds. As shown in Fig. 1a, simulated T and RH are in reasonable agreement with the observations, with the simulated RH values falling in the range of 50 %–90 %. Overall, it can be seen that the model shows cold and wet bias compared to the observations but shows warm bias (about 2–3 ∘C) and dry bias (about 10 %–12 %) in the afternoon hours. In spite of the small change in the amplitude of the diurnal cycle of RH, the phase characteristics of the diurnal cycle of both T and RH are reasonably well captured by the model. Figure 1b shows that simulated NH3 and NH are very different compared with the MARGA measurements. The model predicts an average NH3 and NH ± 1σ mass loading of 56.7 ± 14.3 and 14.7 ± 4.9 µg m−3, respectively, while MARGA measurements indicate an average NH3 and NH ± 1σ mass loading of 28.2 ± 12.4 and 36.9 ± 15.1 µg m−3, respectively. We find the diurnal variation of gas-phase NH3 is significantly overestimated by the model (normalized mean bias (NMB) = 1.02). Conversely, NH is underestimated by about 60 % (NMB = −0.60). Simulated NH3 concentrations peak between 07:00–09:00 and 22:00–23:00 with bimodal variation, whilst MARGA shows a single peak around 12:00–13:00. Conversely, a nearly flat diurnal profile of NH is predicted by the model, whereas the average MARGA NH concentration maxima and minima were observed during nighttime (16:00–03:00) and daytime (03:00–08:00 and 09:00–16:00), respectively.
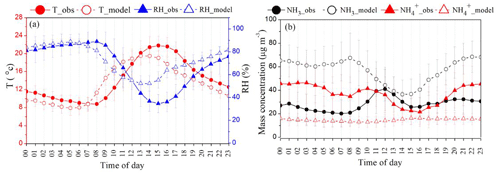
Figure 1Comparison of observed and simulated average diurnal variation in (a) meteorological parameters such as temperature (T in ∘C) and relative humidity (RH in %) and (b) NH3 and NH concentration (µg m−3) during the sampling period (bar indicates mean standard deviation of each hour).
We also looked into the average diurnal profile of NOx and NH3 during dense fog events, and the details can be found in the Supplement (Figs. S1 and S2). It is evident that the observed daytime peak of NH3 did not coincide with NOx peaks, suggesting that traffic emissions do not contribute significantly to the observed NH3 rise. The observed correlation between fog water and enhanced NH3 pulses is consistent with what would also be expected from the evaporation of dew (Sutton et al., 1998; Wentworth et al., 2014, 2016) (Fig. S2 in the Supplement) but is not sufficient to identify whether it is the main cause of the daytime increase of NH3. In the future, measurements of the dew water NH and the accumulation of dew water would be ideal for illuminating the contributing processes. The daytime increase in NH3 concentration could be associated with NH aerosol volatilization driven by an associated sharp change in T and RH (∼ 11:00–12:00) (Sutton et al., 2009a, 2013) off-ground surfaces. The fastest increase in T is 12:00, which is indeed when NH3 was at maximum concentration, indicating that gas-to-particle partitioning may impact the diurnal behavior of NH3 at Delhi during winter (Sutton et al., 2009a, b). However, in the model, because the largest increase in simulated NH3 also precedes the large changes in simulated meteorological parameters, and because the simulated particulate NH is flat compared to observations, simulated meteorology is ruled out as a significant contribution to high bias in simulated NH3. Also, the current model does not include the bidirectional exchange of NH3 with surfaces such as dew and fog water.
3.1.2 Daily mean variation
To assess the validity of the model, the ratio between simulated and observed (model obs) was tested. Figure 2 displays the model obs ratio of daily mean variations in the NH3, NH, and total NHx concentrations. The model shows large differences in NH3 and NH compared with observations. We find a model obs higher than 1 (1.5–4.5) in simulated NH3, indicating the model is biased high (NMB = 1.02), while there is a poor agreement for NH (model obs less than 0.5), indicating the model is biased low (NMB = −0.62). There is good agreement between the modeled total NHx, which is mostly consistent with the observation (model obs close to 1) with a small bias (NMB = 0.08). Despite the adequate ability of the model to reproduce the accurate total NHx, the model is biased low for NH and high for NH3, indicating that the model's representation of the gas-to-particle partitioning is not correct. It is, therefore, necessary to understand missing chemical processes in gas-to-particle partitioning responsible for the overestimation of NH3 and underestimation of NH in the model.
3.2 Gas-to-particle partitioning
We investigated the ability of the model to accurately describe the gas-to-particle partitioning of the measurements (MARGA) by evaluating the fraction of total NHx in the particulate phase (NH NHx) (Ellis et al., 2011; Wang et al., 2015) for which statistical values are summarized in Table 1. The correlation coefficient (r) indicates an inverse relationship of NH NHx with NH3 for both MARGA and the model (, −0.58, respectively). A strong correlation of the MARGA ratio NH NHx with the dominant anion concentration (Cl−: r=0.79) was observed. However, the measurement shows a poor relationship between SO and NH NHx followed by NO, which is probably due to very low concentrations that do not change NH NHx significantly, even when SO and NO are neutralized (see Fig. 6). By contrast, the model shows a strong correlation between NH NHx with SO concentration (r=0.77). MARGA indicates high particulate fractions of NH and Cl−, while the modeled composition is dominated by NH and SO. This mismatch is due to the complete absence of Cl− chemistry in the standard model. The measured NH NHx suggests that anthropogenic HCl may be promoting this increase in particle fraction of NH and Cl− via partitioning into the aerosol, deprotonating in the aerosol water, followed by NH3 partitioning and being protonated by the ionization of the strong electrolyte HCl (Chen et al., 2022; Gunthe et al., 2021).
Table 1Performance statistics of correlation coefficient (r) of NH NHx with NH3 and aerosols (NH, Cl−, SO, and NO).
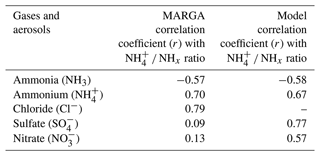
Figure 3 shows the percentage contribution of gases (NH3, SO2, HCl, HNO3, and HONO) and PM2.5 aerosol (NH, SO, NO, and Cl−) during the WiFEX measurements. The pie charts for the gases show that NH3 (accounting for 53.3 % of the measured total gas concentration) dominates the gas phase, followed by sulfur dioxide (SO2) (35.61 %), whereas PM2.5 aerosol shows NH (49.5 %) as a major cation and Cl− (29.7 %) as a significant anion followed by NO (11.7 %) and SO (9 %). There is also a very high amount of SO2 reaching the site from the nearby industrial area, which is not converted to SO very quickly (Acharja et al., 2021). In a normally NH3-rich atmosphere, gas-phase oxidation of SO2 is much slower than the aqueous-phase oxidation by O3, and due to nearby sources, much of the sulfur is present as SO2 (Li et al., 2007). This appears to be because of the slow rate of gas-phase oxidation of SO2. Although the atmosphere is rich in NH3, in principle favoring aqueous-phase oxidation via O3, it appears that O3 concentrations are often insufficient (mean = 36.3, median = 33.8, minimum = 26.5, and maximum = 53.9 µg m−3 respectively) at the IGIA site (Fig. S3 in the Supplement). Hence for many periods during the WiFEX campaign, SO and NO are very low, with the result that the NH NHx ratio does not change appreciably when SO is neutralized (Table 1).
According to thermodynamic equilibrium theory, an aqueous solution maintains charge neutralization initially by balancing NH3 uptake with the uptake of sulfuric acid (H2SO4) before HNO3 and HCl can partition into the aqueous aerosol; hence all SO in the condensed phase will be fully neutralized before any HNO3 or HCl can partition (Behera et al., 2013). Typical Delhi winter conditions of excess NH3, high RH, and low T favor gas-to-particle partitioning of NH3. The principal inorganic chemical reactions that occur in aqueous atmospheric aerosols form pairs of non-volatile NH and acid anions (SO, NO, and Cl−) and are summarized in Reactions (R1) to (R3) (Seinfeld et al., 1998).
NH and Cl− (Reaction R3), which are favored by low T and high RH, form a reversible equilibrium with NH3 and HCl (Ianniello et al., 2011; Seinfeld and Pandis, 2016), which was the case during WiFEX. It is likely that high Cl− in Delhi resulted from gas–to-particle partitioning of HCl into aerosol water in the presence of excess NH3 (Reaction R3), with aqueous-phase Cl− stimulating further water uptake and jointly driving aerosol mass composition and growth through co-condensation (Chen et al., 2022; Gunthe et al., 2021). Hence, to understand the driver of the measured NH and the role of aqueous chemistry, we plotted the fraction of the ratio of HCl to Cl− (HCl Cl−) as a function of NH concentration and RH in Fig. 4. The decrease in the fraction of HCl Cl− is associated with an increase in NH concentration at high RH between 70 %–100 %. The HCl Cl− is highly anticorrelated (r = −0.53) with NH concentration in the presence of high RH (70 %–100 %), further supporting the view that HCl promotes the increase in the particle fraction of NH (49.5 %), with Cl− (29.7 %) the primary anion.
We investigated the directions of local emission sources associated with concentration increases of NH3, NH, Cl−, and NHx through bivariate polar graphs using the OpenAir software (Carslaw and Ropkins, 2012) at the IGIA site. Figure 5 shows the bivariate polar plots of mean NH3 (Fig. 5a), NH (Fig. 5b), Cl− (Fig. 5c), and total NHx (Fig. 5d) concentration for the observation period in relation to wind speed and wind direction. The 270–300∘ sector dominated the wind direction at IGIA (Acharja et al., 2021). Figure 5a shows that the highest NH3 concentration was associated with the winds coming from the east and southeast of the site, where it could have been emitted from dairy farms, including animal houses, yards, and manure storage, as well as by the application to the farmland of urea and other ammoniacal fertilizers, ammoniacal wastes, and ruminant urine located at this region (Hindustan Times, 2021; Leytem et al., 2018; Sherlock et al., 1994). Such sources of NH3 volatilization (Hristov et al., 2011; Laubach et al., 2013) can also explain the higher concentrations of total NH (and, by definition, NHx) for air coming from the southeast of the measurement site (Fig. 5b and d). This enhancement in the southeast region is not only affected by emissions, but also by meteorology and chemistry. Thus higher NH3 concentration may also be due to the lack of turbulent mixing, which restricts the dilution of plumes from local point sources at lower wind speeds (Ianniello et al., 2010). The bivariate polar plots of NH (Fig. 5b) and Cl− (Fig. 5c) concentration point to the west direction as a principal source for thermodynamic partitioning of NH3 and HCl to the condensed phase to form NH and Cl−. Two industrial sources are located in this direction: the site is impacted by a cluster in northwest Delhi of industrial processes, such as steel pickling industries, and others include metal finishing and electroplating, which are known to be vital HCl emitters (Acharja et al., 2021; Jaiprakash et al., 2017). Near the source, abundant quantities of NH3 may drive the partitioning of HCl to the condensed phase, resulting in high concentrations of NH and Cl− towards the west at lower wind speeds. Thus, high NH and Cl− correspond to the lowest NH3 concentration region (inverse relation), which can be observed in Fig. 5a, b, and c, highlighting the importance of nearby HCl industrial sources in driving the particle fraction of NH and Cl−.
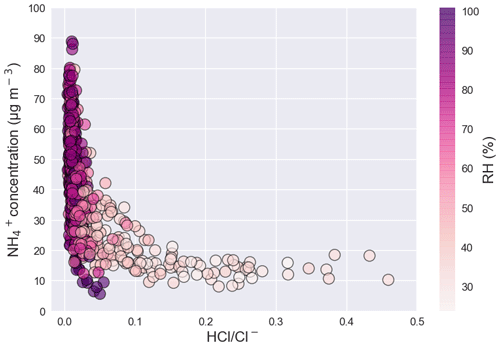
Figure 4Fraction of HCl Cl− ratio as a function of NH concentration (µgm−3) and relative humidity (RH).
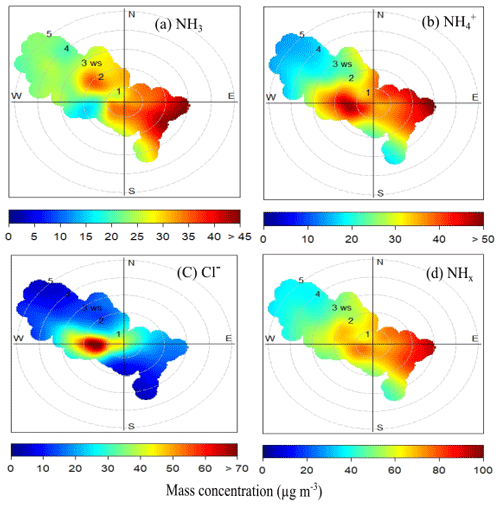
Figure 5Bivariate plots of mean (a) NH3 concentration, (b) NH concentration, (c) Cl− concentration, and (d) total NHx concentration in relation to wind speed (m s−1) and direction.
To gain insight into the role of NH in the neutralization of anions (SO, NO, and Cl−), the aerosol neutralization ratio (ANR) was calculated using the observed data. The ANR is defined as the equivalent ratio of NH to the sum of SO, NO, and Cl− because these species represent the dominant cations and anions in PM2.5, respectively. Figure 6 demonstrates, on average, how well the charge balance works between Cl−, NO, and SO (in µeq m−3) as the anions and NH as the major cation (ANR close to unity), with Cl− as the most significant anion followed by NO and SO. The mean ± 1σ ANR value for PM2.5 during the observed period was 0.96 ± 0.14. It ranges from a minimum of 0.35 ± 0.04 to a maximum of 2.31 ± 0.08. Higher values than unity may indicate the presence of organic acids in the aerosol, which MARGA does not measure (Acharja et al., 2020). Also, high standard error in Fig. 6 indicates the possibility of uncertainties associated with the breakthrough of NH3 spikes on the denuder at high concentration (∼ 1 %) (Stieger et al., 2019). However, the good charge balance indicates this was not a major issue. There also were certain periods where low concentrations were observed of Cl− and NO (3–6 January 2018 and 16–17 January 2018) in Fig.6. Comparing the model obs for NH3, NH, and total NHx during these periods provides some degree of validation of the model where sulfur chemistry dominates the reaction with NH3. Figure S4 (in the Supplement) shows that model obs indicates substantial variability, which appears to be overestimating NH3 (model obs >1) while underestimating total NH (model obs <1) on average in the model.
3.3 Influence of HCl Cl− chemistry in WRF-Chem
We further conducted three scenario simulations for the period 7–16 January 2018 (10 d) to explore the potential impacts of the addition of anthropogenic chloride (HCl Cl−) emissions in the concentrations of NH3, NH, and total NHx. We employ the HCl emissions from trash-burning activities in Delhi, as predicted by Sharma et al. (2019) in our model setup. We tested the three sensitivity experiments, named no HCl (0 mol km−2 h−1), base case HCl (3× Sharma et al., 2019; 24.8 mol km−2 h−1), and 3 × base HCl (74 mol km−2 h−1) scenarios, reflecting adjustments which are consistent with the more recent upward adjustments in the amount of waste burned in landfills by Chaudhary et al. (2021) and also reflect additional industrial HCl sources not accounted for in the inventory. Figure 7 presents the box-and-whisker plots for secondary inorganic aerosols and trace gases from the observations (MARGA) and those simulated by the model for the three sensitivity experiments. Daily mean ± 1σ values are summarized in Table 2 for three different model scenarios. As can be observed from Fig. 7a–c, increasing the HCl emissions (Fig. 7g) in the model partitions more NH3 to the condensed phase due to its high concentrations, reaching maximum mass loadings of NH and Cl− of 70 and 110 µg m−3, respectively, in the 3 × base HCl scenario while increasing the total mean NHx concentration by 15 µg m−3 compared to the no HCl run, presumably reflecting the longer residence time of NH for near-surface air measurements.
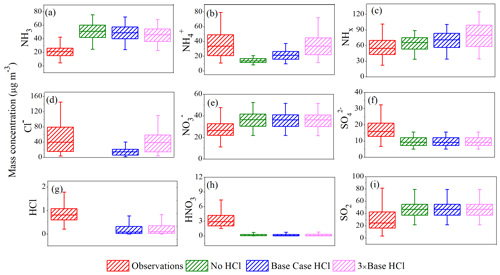
Figure 7Box-and-whisker plot for trace gases and secondary inorganic aerosols from the observations (MARGA) and simulated in sensitivity test with changes in HCl emissions in no HCl (0 mol km−2 h−1), base case HCl (24.8 mol km−2 h−1), and 3 × base HCl (74 mol km−2 h−1) runs at IGIA, Delhi.
Table 2Daily mean ± 1σ in gases and inorganic aerosol concentration observed (MARGA) and simulated in sensitivity test with changes in total HCl emissions in no HCl (0 mol km−2 h−1), base case HCl (24.8 mol km−2 h−1), and 3 × base HCl (74 mol km−2 h−1).
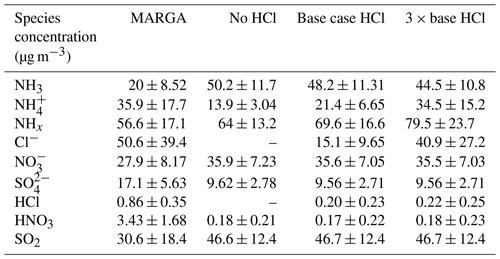
The simulated NO concentration (Fig. 7e) generally exceeds the measurements in all three experiments; since the main neutralizing species for NO is NH, it is controlled via the equilibrium between NO, HNO3, and NH3, but also the competition with HCl for free NH3. Simulated HNO3 is significantly underestimated (by ∼ 3 µg m−3) (Fig. 7h) by the model compared to the observations. As a consequence, the model suggests that NO formation from gaseous NH3 and HNO3 cannot occur. The gas fraction of observed HNO3 will be determined by aerosol pH and liquid water content based on NH3 and NO availability (Nenes et al., 2020). The over-prediction of NH3 concentration in the model compared with the observations generates more NO (and simultaneously reduces HNO3), with the total fraction of HNO3+ NO (THNO3) concentration in the model also exceeding the observed THNO3, which is more strongly affected by reducing the NH3 emissions in the model (Fig. S5 in the Supplement). On average, THNO3 reduced by only 0.38 µg m−3 in 3 × base HCl compared to the no HCl run. But reducing NH3 emissions by a factor of 3 (−3 × NH3_EMI) in the 3 × base HCl scenario reduced mean THNO3 by a further 4.71 µg m−3. The extent of partitioning and accumulation of NH4NO3 depends on T, aerosol water, and pH, as well as NH3 availability (Nenes et al., 2020). Our model simulations find that the presence of HCl Cl− does not significantly alter THNO3 but that the excess NH3 with missing chloride chemistry is a major contributor and will lead to mismatches in the model between measured simulated gas and particulate matter concentrations.
The simulated SO concentration (Fig. 7f) was underestimated (by ∼ 7.5 µg m−3), while gas-phase SO2 (Fig. 7i) was found to be overestimated by about 16 µg m−3 in all three experiments compared with the observations. This may be caused by the fact that the drivers for typical sulfate production via OH or aqueous H2O2 oxidation pathway are likely to be wrong in the model. The missing chemistry may underlie this mismatch and requires further sensitivity studies considering different SO2 oxidation pathways. This requires further study, such as scenario evaluation of altered SO2 emissions in the model, to examine the main pathway(s) for SO2 to SO conversion. Measurements of OH and other radicals in Delhi are currently lacking, making it difficult to constrain the associated chemical schemes. To investigate the further impact of 3 × base HCl in the model, uptake of gaseous NH3 to form NH and Cl− was analyzed via a strong correlation coefficient values of r = 0.84 for NH NHx with Cl− concentration, indicating that a fraction of gas-to-particle conversion in the model correlates well with the Cl− concentration and was reasonably well simulated in the 3 × base HCl run.
3.4 Comparison of the temporal variation in NH3, NH, and NHx using WRF-Chem (HCl Cl−) and MARGA
3.4.1 Diurnal variation
Here, diurnal variations of monitored aerosol compounds and gases were analyzed to investigate the gas-to-particle conversion of NH3 in the model. We analyzed the simulation results of the 3 × base HCl run. The diurnal variations for NH3 and NH are controlled mainly by thermodynamic gas-to-particle partitioning, boundary layer mixing, and emission and deposition processes, along with vertical and horizontal advection (Meng et al., 2018). Figure 8 (top) presents the diurnal variations of NH3 and NH (in µg m−3), along with particulate NH, Cl−, NO, SO, SO2, HCl, and HNO3 concentrations (in µeq m−3) measured (Fig. 8a (top)) and modeled (Fig. 8b (top)), along with meteorological parameters such as T and RH (Fig. 8 (bottom)). We adopted diurnal variation in emissions from Jena et al. (2021) based on boundary layer mixing. It can be seen in Fig. 8a (top and bottom) that a much bigger peak in NH3 concentration is observed in the daytime than is modeled (despite turbulence differences), indeed suggesting a much stronger NH3 in the middle of the day (11:00–13:00). As evaporation proceeds mainly in the morning (08:00–12:00), getting warmer, the peak is near midday (11:00–13:00), rather than in the afternoon (13:00–14:00), when warmest, similar to what was also observed in Sutton et al. (1998). Indeed, the decreasing NH and Cl− during the late morning (10:00) correspond to the increasing NH3 peak, which reflects the fact that warming promotes the shift of aerosols to the gas phase. Ammonium decreases more than NH3 during the day, as this also evaporates to form NH3. Similarly, Cl− evaporates during the day since the HCl concentration increases. However, it can be seen that NO and SO are slightly changed diurnally, inferring longer-range transport perhaps, whereas HCl and Cl−are from more local sources. The diurnal variability in gases and aerosols in 3 × base HCl simulations in Fig. 8b (top) is primarily controlled by the planetary boundary layer mixing, meteorology/dispersion, environment (T and RH in Fig. 8b (bottom)), and transport. So presumably, maximum NH3 at 08:00 is due to limited turbulence/boundary layer, with dilution by mixing after 08:00. However, the model is able to represent the diurnal variation of NH and Cl− well, both in terms of amount and pattern, which was not the case in the no HCl run, where NH was observed to be flat in Sect. 1. During the hours of 09:00 and 11:00, when measured NH3 rises, the model predicts a large decrease in NH3, while during 19:00–23:00, when measured NH3 decreases, the model predicts a large increase. Furthermore, the modeled HCl and HNO3 are very low compared to the measurements, whereas SO2 concentration matches well with the observations. It can be seen that NO and SO are flat in the model. This highlights the need to develop accurate diurnal variability in NH3 emissions over this region.
Figure 9 presents the differences in diurnal variation of mean NH3 (Fig. 9a), NH (Fig. 9b), and total NHx (Fig. 9c) concentration for the three sensitivity experiments. While the simulated NH3 concentrations decrease in the 3 × base HCl compared to the no HCl and base case HCl run (Table 2), none of the model experiments capture the diurnal cycle of NH3. Higher levels of observed NH3 during daytime and modeled NH3 during nighttime highlight the need to improve diurnal variability in NH3 emissions over this region based on the nature and strength of the actual sources. Between the no HCl and the 3 × base HCl run, the NMB for NH3 reduced from 1.38 to 1.13, and NMB for NH systematically improved from −0.61 to −0.03. In contrast, NMB for total NHx increased from 0.12 to 0.39. Table 3 summarizes the statistical indicators for the three sensitivity experiments. An increase in HCl emissions in the 3 × base HCl leads to a higher mass concentration of NH and Cl−, which also increases total mean NHx concentration by 22.4 µg m−3, presumably reflecting the longer atmospheric lifetime of NH compared with NH3. We find consistent high bias in all the simulations for NH3, which is highest during the early morning and at nighttime. In order to better understand the relationship between NH3, NH, and NHx concentrations in the diurnal profile of model, one sensitivity study is conducted in the best case HCl experiment to simulate the response of NHx concentrations by changing NH3 emissions. In these simulations, only NH3 emissions were reduced further by a factor of 3 (−3 × NH3_EMI) in the 3 × base HCl experiment, while all other processes and chemical schemes were unchanged. Figure S6 in the Supplement shows the diel profile of model obs ratio for NH3 (Fig. S6a), NH (Fig. S6b), and total NHx (Fig. S6c) concentration simulated with the 3 × base HCl and −3 × NH3_EMI scenario. Reducing NH3 emissions in the model (−3 × NH3_EMI) significantly improves model–measurement agreement for NH3 (mean model obs = 1.9), NH (mean model obs = 0.9), and total NHx concentration(mean model obs = 1.2) compared to the 3 × base HCl run, further suggesting that the longer lifetime of NH may be the controlling driver for the total NHx concentration in the model.
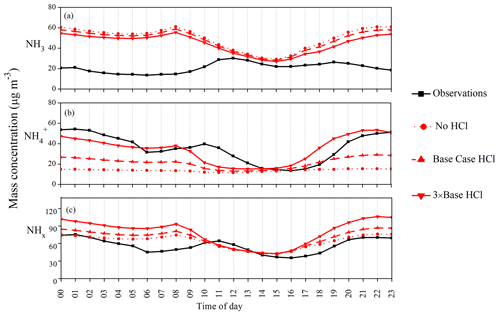
Figure 9Diurnal variation in the mean (a) NH3 concentration (b) NH concentration and (c) total NHx concentration observed (black), simulated in no HCl (dotted red), base case HCl (dashed red), and 3 × base HCl (solid red) runs.
3.4.2 Variation of daily means
Figure S7 in the Supplement illustrates a time-series graph that compares daily mean NH3 (Fig. S7a), NH (Fig. S7b), and total NHx concentrations (Fig. S7c) for the three sensitivity experiments, and Table 2 shows the mean ± 1σ of these variables. The results show that compared to the no HCl run, NH3 mean concentrations decreased by 2 µg m−3 in the base case HCl and decreased by a further 3.2 µg m−3 in the 3 × base HCl run. Conversely, NH mean concentration increases in the base case HCl by 7.5 µg m−3 and further increases by 13.1 µg m−3 (3 × base HCl). This decrease in NH3 is associated with the enhanced gas-to-particle conversion of NH3 to NH. Associated with these changes, total mean NHx also increased by 5.5 and 9.8 µg m−3 in the base case HCl and 3 × base HCl, respectively, compared to the no HCl. This is likely due to associated increases in the atmospheric lifetime of NHx with respect to deposition as the partitioning shifted from the faster depositing gas phase to the aerosol phase. The lifetime of NH3 is very short, a few hours, while that of NH is 1 to 15 d (Aneja et al., 1998; Nair and Yu, 2020; Pawar et al., 2021; Q. Wang et al., 2020).
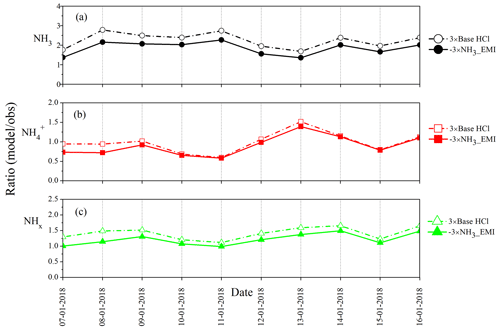
Figure 10Comparison of ratio of model obs in the daily mean (a) NH3 concentration, (b) NH concentration, and (c) total NHx concentration in the 3 × base HCl and -3 × NH3_EMI scenarios.
To understand the overestimation of total NHx in the daily mean variation by the model further, we compared the 3 × base HCl and −3 × NH3_EMI sensitivity experiment. Figure 10 shows the ratio of model obs for NH3 (Fig. 10a), NH (Fig. 10b), and total NHx (Fig. 10c) concentration. It can be seen that the model–measurement agreement improves significantly (model obs closer to 1) after reducing NH3 emissions for all three metrics. The −3 × NH3_EMI run would reduce the mean NH3, NH, and total NHx concentration by ∼ 8.1, 3.2, and 11.3 µg m−3, respectively, compared to the 3 × base HCl run. Even though NH3 emissions are reduced, they are still sufficient to react rapidly with the varying HCl in the sensitivity experiments, contributing to an increase in NH. As can be seen in Fig. 10b, initially, NH is somewhat lower, but it increases later and matches the 3 × base HCl run. This suggests that NH formation in the model is more sensitive to changes in HCl than changes in NH3 emission, while total NHx agrees well by reducing the NH3 emissions. In general, CTMs have higher NH3 concentration than observations, further supporting models having too much NH3. A few factors might contribute to the model discrepancies for NH3: there are uncertainties in the emission inventory of the bottom-up approach of NH3, and the model does not currently include the bidirectional exchange of NH3 with surfaces, such as dew and fog water. Also, the model does not have accurate industrial sources of HCl emission. Diurnal emission profiles are uncertain for both NH3 and HCl. Furthermore, gas-to-particle partitioning associated with SO2 oxidation pathways in the model is not correct at present.
In this study, for the first time in South Asia we have evaluated the performance of a chemical transport model (WRF-Chem) in modeling NH3, NH, and total NHx, by comparing them against the WiFEX measurements (MARGA). In daily means, we find NH3 is significantly overestimated by the model, and NH was underestimated, while simulated total NHx agreed well with the measurement, indicating that incorrect gas-to-particle partitioning, along with missing chemical processes, may impact this mismatch in the model. The ability of the model to accurately describe the gas-to-particle partitioning of the MARGA was evaluated by the fraction of total NHx (i.e., NH3+ NH) in the particulate phase (NH NHx). A strong relation of MARGA NH NHx was observed with the dominant anion (Cl−) (r = 0.79), whereas the standard model showed a strong correlation between NH NHx with the dominant anion (SO) (r = 0.77), pointing to the missing chloride (HCl Cl−) chemistry in the model.
We incorporated HCl Cl− emissions in the model and conducted three sensitivity experiments of varying HCl emissions, named the no HCl (0 mol km−2 h−1), base case HCl (3× Sharma et al., 2019; 24.8 mol km−2 h−1), and 3 × base HCl (74 mol km−2 h−1) run. The revised model shows that by adding HCl emissions, more NHx was partitioned to the condensed phase, improving agreement with the observations. The 3 × base HCl run was able to represent the diurnal variation of NH and Cl− well, both in terms of amount and pattern with improved NMB for NH3. Additional sensitivity tests in changing NH3 emissions (reduction by a factor of 3) in the 3 × base HCl also improved NH3, NH, and NHx concentrations. We find excess NH3 along with longer lifetime of NH may act as a controlling driver for NHx overestimation in the model. These results highlight the need to include correct industrial sources of HCl emissions, along with appropriate emissions of NH3, to reduce biases in NHx. Appropriate NH3 emissions are developed using country-specific emission inventories, which are currently under development as part of the Global Challenges Research Fund (GCRF), South Asian Nitrogen Hub (SANH). Also, there is potential to develop top-down constraints on NH3 emissions by taking inference from the satellite-, model-, and ground-based observations. Challenges remain in simulating NH3 as a contributor to particulate matter due to temporal factors in ammonia peaks, including the role of fog and dew, where more work is needed. This work also suggests that model improvements to SO2 oxidation pathways could improve NHx partitioning.
The emission grid maps can be downloaded from the EDGAR website at https://edgar.jrc.ec.europa.eu/htap_v2/index.php?SECURE=_123 and HCl emissions available at https://doi.org/10.17632/546t9249bv.1 (Sinha et al., 2019). The model data are available on the AADITYA supercomputer at the Indian Institute of Tropical Meteorology (IITM) and can be provided upon request to the corresponding author. The observational and meteorological data of WiFEX are available by contacting the corresponding author.
The supplement related to this article is available online at: https://doi.org/10.5194/acp-23-41-2023-supplement.
SDG designed the research; PVP performed the WRF-Chem model simulations and led the analysis; PA and RK contributed to data collection and its quality control and assurance; GG, RK, and PG helped with the model setup; and PVP and SDG wrote the paper with contributions from all co-authors.
The contact author has declared that none of the authors has any competing interests.
Publisher's note: Copernicus Publications remains neutral with regard to jurisdictional claims in published maps and institutional affiliations.
We thank the Director at IITM for his continuous support and encouragement. IITM is funded by the Ministry of Earth Sciences (MoES), Government of India. We wish to thank the MoES for supporting the WiFEX campaign. The lead author's fellowship was supported by the National Supercomputing Mission (NSM) program grant at C-DAC, and PhD fees are covered by the Natural Environment Research Council (NERC) of the UK Research and Innovation (UKRI) Global Challenges Research Fund (GCRF), South Asian Nitrogen Hub (SANH), and we are grateful to the Executive Director and the Director General of C-DAC and the SANH Director and Chair of the Executive Board. We acknowledge the availability of CPCB-NOx, NO2, and O3 data from the CPCB web portal (https://app.cpcbccr.com/ccr, last access: 1 December 2021). We wish to acknowledge that the National Center for Atmospheric Research is sponsored by the National Science Foundation.
This paper was edited by Eleanor Browne and reviewed by two anonymous referees.
Acharja, P., Ali, K., Trivedi, D. K., Safai, P. D., Ghude, S., Prabhakaran, T., and Rajeevan, M.: Characterization of atmospheric trace gases and water soluble inorganic chemical ions of PM1 and PM2.5 at Indira Gandhi International Airport, New Delhi during 2017–18 winter, Sci. Total Environ., 729, 138800, https://doi.org/10.1016/j.scitotenv.2020.138800, 2020.
Acharja, P., Ali, K., Ghude, S. D., Sinha, V., Sinha, B., Kulkarni, R., Gultepe, I., and Nair, M.: Chemosphere Enhanced secondary aerosol formation driven by excess ammonia during fog episodes, Chemosphere, 289, 133155, https://doi.org/10.1016/j.chemosphere.2021.133155, 2021.
Ali, K., Acharja, P., Trivedi, D. K., Kulkarni, R., Pithani, P., Safai, P. D., Chate, D. M., Ghude, S., Jenamani, R. K., and Rajeevan, M.: Characterization and source identification of PM2.5 and its chemical and carbonaceous constituents during Winter Fog Experiment 2015–16 at Indira Gandhi International Airport, Delhi, Sci. Total Environ., 662, 687–696, https://doi.org/10.1016/j.scitotenv.2019.01.285, 2019.
Aneja, V. P., Murray, G. C., and Southerland, J.: Atmospheric nitrogen compounds: Emissions, transport, transformation, deposition, and assessment, EM Air Waste Manag. Assoc. Mag. Environ. Manag., 22–25, 1998.
Behera, S. N., Sharma, M., Aneja, V. P., and Balasubramanian, R.: Ammonia in the atmosphere: a review on emission sources, atmospheric chemistry and deposition on terrestrial bodies, Environ. Sci. Pollut. Res., 20, 8092–8131, https://doi.org/10.1007/s11356-013-2051-9, 2013.
Bucaram, C. J. and Bowman, F. M.: Wrf-chem modeling of summertime air pollution in the northern great plains: Chemistry and aerosol mechanism intercomparison, Atmosphere, 12, 1121, https://doi.org/10.3390/atmos12091121, 2021.
Carslaw, D. C. and Ropkins, K.: Openair – An r package for air quality data analysis, Environ. Model. Softw., 27–28, 52–61, https://doi.org/10.1016/j.envsoft.2011.09.008, 2012.
CPCB: Guidelines for Real Time Sampling & Analyses, available at: http://www.indiaenvironmentportal.org.in/files/NAAQSManualVolumeII.pdf (last access: 24 November 2022), 2011.
Chaudhary, P., Garg, S., George, T., Shabin, M., Saha, S., Subodh, S., and Sinha, B.: Underreporting and open burning – the two largest challenges for sustainable waste management in India, Resour. Conserv. Recycl., 175, 105865, https://doi.org/10.1016/j.resconrec.2021.105865, 2021.
Chen, Y., Wang, Y., Nenes, A., Wild, O., Song, S., Hu, D., Liu, D., He, J., Hildebrandt Ruiz, L., Apte, J. S., Gunthe, S. S., and Liu, P.: Ammonium Chloride Associated Aerosol Liquid Water Enhances Haze in Delhi, India, Environ. Sci. Technol., 56, 7163–7173, https://doi.org/10.1021/acs.est.2c00650, 2022.
Clarisse, L., Clerbaux, C., Dentener, F., Hurtmans, D., and Coheur, P. F.: Global ammonia distribution derived from infrared satellite observations, Nat. Geosci., 2, 479–483, https://doi.org/10.1038/ngeo551, 2009.
Clarisse, L., Shephard, M. W., Dentener, F., Hurtmans, D., Cady-Pereira, K., Karagulian, F., Van Damme, M., Clerbaux, C., and Coheur, P. F.: Satellite monitoring of ammonia: A case study of the San Joaquin Valley, J. Geophys. Res-Atmos., 115, 1–15, https://doi.org/10.1029/2009JD013291, 2010.
CPCB: Annual Report 2014–15, Central Pollution Control Board (CPCB), Delhi, India, available at: http://cpcbenvis.nic.in/annual_report/AnnualReport_55_Annual_Report_2014-15.pdf (last access: 20 April 2021), 2014.
CPCB: Annual Report 2019–20, Central Pollution Control Board (CPCB), Delhi, India, available at: https://cpcb.nic.in/annual-report.php/ (last access: 16 April 2021), 2020.
Datta, A., Sharma, S. K., Harit, R. C., Kumar, V., Mandal, T. K., and Pathak, H.: Ammonia emission from subtropical crop land area in india, Asia-Pacific J. Atmos. Sci., 48, 275–281, https://doi.org/10.1007/s13143-012-0027-1, 2012.
Duan, X., Yan, Y., Peng, L., Xie, K., Hu, D., Li, R., and Wang, C.: Role of ammonia in secondary inorganic aerosols formation at an ammonia-rich city in winter in north China: A comparative study among industry, urban, and rural sites, Environ. Pollut., 291, 118151, https://doi.org/10.1016/j.envpol.2021.118151, 2021.
Ellis, R. A., Murphy, J. G., Markovic, M. Z., Vandenboer, T. C., Makar, P. A., Brook, J., and Mihele, C.: The influence of gas-particle partitioning and surface-atmosphere exchange on ammonia during BAQS-Met, Atmos. Chem. Phys., 11, 133–145, https://doi.org/10.5194/acp-11-133-2011, 2011.
FINNv1.5: FINN Data, https://www.acom.ucar.edu/Data/fire/ (last access: 15 April 2019), 2017.
Freitas, S. R., Longo, K. M., Chatfield, R., Latham, D., Silva Dias, M. A. F., Andreae, M. O., Prins, E., Santos, J. C., Gielow, R., and Carvalho Jr., J. A.: Including the sub-grid scale plume rise of vegetation fires in low resolution atmospheric transport models, Atmos. Chem. Phys., 7, 3385–3398, https://doi.org/10.5194/acp-7-3385-2007, 2007.
Georgiou, G. K., Christoudias, T., Proestos, Y., Kushta, J., Hadjinicolaou, P., and Lelieveld, J.: Air quality modelling in the summer over the eastern Mediterranean using WRF-Chem: chemistry and aerosol mechanism intercomparison, Atmos. Chem. Phys., 18, 1555–1571, https://doi.org/10.5194/acp-18-1555-2018, 2018.
Ghude, S., Kumar, R., Jena, C., Debnath, S., Kulkarni, R., Alessandrini, S., Biswas, M., Kulkrani, S., Pithani, P., Kelkar, S., Sajjan, V., Chate, D., Soni, V., Singh, S., Nanjundiah, R., and Rajeevan, M.: Evaluation of PM2.5 Forecast using Chemical Data Assimilation in the WRF-Chem Model: A Novel Initiative Under the Ministry of Earth Sciences Air Quality Early Warning System for Delhi, India, Curr. Sci., 118, 11, https://doi.org/10.18520/cs/v118/i11/1803-1815, 2020.
Ghude, S. D.: Premature mortality in India due to PM2.5 and ozone exposure, Geophys. Res. Lett., 43, 4650–4658, https://doi.org/10.1002/2016GL068949, 2016.
Ghude, S. D., Fadnavis, S., Beig, G., Polade, S. D., and van der A, R. J.: Detection of surface emission hot spots, trends, and seasonal cycle from satellite-retrieved NO 2 over India, J. Geophys. Res., 113, D20305, https://doi.org/10.1029/2007JD009615, 2008a.
Ghude, S. D., Jain, S. L., Arya, B. C., Beig, G., Ahammed, Y. N., Kumar, A., and Tyagi, B.: Ozone in ambient air at a tropical megacity, Delhi: Characteristics, trends and cumulative ozone exposure indices, J. Atmos. Chem., 60, 237–252, https://doi.org/10.1007/s10874-009-9119-4, 2008b.
Ghude, S. D., Van der A, R. J., Beig, G., Fadnavis, S., and Polade, S. D.: Satellite derived trends in NO2 over the major global hotspot regions during the past decade and their inter-comparison, Environ. Pollut., 157, 1873–1878, https://doi.org/10.1016/j.envpol.2009.01.013, 2009.
Ghude, S. D., Lal, D. M., Beig, G., van der A, R., and Sable, D.: Rain-Induced Soil NO x Emission From India During the Onset of the Summer Monsoon: A Satellite Perspective, J. Geophys. Res., 115, D16304, https://doi.org/10.1029/2009JD013367, 2010.
Ghude, S. D., Pfister, G. G., Jena, C. K., Emmons, L. K., Kumar, R., and van der A, R. J.: Satellite constraints of Nitrogen Oxide (NOX) emissions from India based on OMI observations and WRF-Chem simulations, Geophys. Res. Lett., 40, 423–428, https://doi.org/10.1029/2012gl053926, 2012.
Ghude, S. D., Kulkarni, S. H., Jena, C., Pfister, G. G., Beig, G., Fadnavis, S., and Van Der, R. J.: Application of satellite observations for identifying regions of dominant sources of nitrogen oxides over the indian subcontinent, J. Geophys. Res.-Atmos., 118, 1075–1089, https://doi.org/10.1029/2012JD017811, 2013.
Ghude, S. D., Bhat, G. S., Prabhakaran, T., Jenamani, R. K., Chate, D. M., Safai, P. D., Karipot, A. K., Konwar, M., Pithani, P., Sinha, V., Rao, P. S. P., Dixit, S. A., Tiwari, S., Todekar, K., Varpe, S., Srivastava, A. K., Bisht, D. S., Murugavel, P., Ali, K., Mina, U., Dharua, M., Rao, Y. J., Padmakumari, B., Hazra, A., Nigam, N., Shende, U., Lal, D. M., Chandra, B. P., Mishra, A. K., Kumar, A., Hakkim, H., Pawar, H., Acharja, P., Kulkarni, R., Subharthi, C., Balaji, B., Varghese, M., Bera, S., and Rajeevan, M.: Winter fog experiment over the Indo-Gangetic plains of India, Curr. Sci., 112, 4, https://doi.org/10.18520/cs/v112/i04/767-784, 2017.
Ghude, S. D., Kumar, R., Govardhan, G., Jena, C., Nanjundiah, R. S., and Rajeevan, M.: New Delhi: air-quality warning system cuts peak pollution, Nature, 602, 211, https://doi.org/10.1038/D41586-022-00332-Y, 2022.
Ginoux, P., Chin, M., Tegen, I., Goddard, T., and In, G.: Sources and distribution of dust aerosols simulated with the GOCART model, J. Geophys. Res., 106, 20255–20273, https://doi.org/10.1029/2000JD000053, 2001.
Gu, B., Zhang, L., Dingenen, R. Van, Vieno, M., Grinsven, H. J. Van, Zhang, X., Zhang, S., Chen, Y., Wang, S., Ren, C., Rao, S., Holland, M., Winiwarter, W., Chen, D., Xu, J., and Sutton, M. A.: Abating ammonia is more cost-effective than nitrogen oxides for mitigating PM2.5 air pollution, Science, 374, 758–762, https://doi.org/10.1126/science.abf8623, 2021.
Guenther, A., Karl, T., Harley, P., Wiedinmyer, C., Palmer, P. I., and Geron, C.: Estimates of global terrestrial isoprene emissions using MEGAN (Model of Emissions of Gases and Aerosols from Nature), Atmos. Chem. Phys., 6, 3181–3210, https://doi.org/10.5194/acp-6-3181-2006, 2006.
Gunthe, S. S., Liu, P., Panda, U., Raj, S. S., Sharma, A., Darbyshire, E., Reyes-Villegas, E., Allan, J., Chen, Y., Wang, X., Song, S., Pöhlker, M. L., Shi, L., Wang, Y., Kommula, S. M., Liu, T., Ravikrishna, R., McFiggans, G., Mickley, L. J., Martin, S. T., Pöschl, U., Andreae, M. O., and Coe, H.: Enhanced aerosol particle growth sustained by high continental chlorine emission in India, Nat. Geosci., 14, 77–84, https://doi.org/10.1038/s41561-020-00677-x, 2021.
Gupta, M. and Mohan, M.: Validation of WRF/Chem model and sensitivity of chemical mechanisms to ozone simulation over megacity Delhi, Atmos. Environ., 122, 220–229, https://doi.org/10.1016/j.atmosenv.2015.09.039, 2015.
Hindustan Times: 66 dairies, six dyeing units shut down in east Delhi, Hindustan Times, 6 July, https://www.hindustantimes.com/cities/others/66-dairies-six-dyeing-units-shut-down-in-east-delhi-101625596156203.html (last access: 24 November 2020), 2021.
Hristov, A. N., Hanigan, M., Cole, A., Todd, R., McAllister, T. A., Ndegwa, P. M., and Rotz, A.: Review: Ammonia emissions from dairy farms and beef feedlots, Can. J. Anim. Sci., 91, 1–35, https://doi.org/10.4141/CJAS10034, 2011.
Huang, X., Song, Y., Li, M., Li, J., Huo, Q., Cai, X., Zhu, T., Hu, M., and Zhang, H.: A high-resolution ammonia emission inventory in China, Global Biogeochem. Cy., 26, 1–14, https://doi.org/10.1029/2011GB004161, 2012.
Ianniello, A., Spataro, F., Esposito, G., Allegrini, I., Rantica, E., Ancora, M. P., Hu, M., and Zhu, T.: Occurrence of gas phase ammonia in the area of Beijing (China), Atmos. Chem. Phys., 10, 9487–9503, https://doi.org/10.5194/acp-10-9487-2010, 2010.
Ianniello, A., Spataro, F., Esposito, G., Allegrini, I., Hu, M., and Zhu, T.: Chemical characteristics of inorganic ammonium salts in PM2.5 in the atmosphere of Beijing (China), Atmos. Chem. Phys., 11, 10803–10822, https://doi.org/10.5194/acp-11-10803-2011, 2011.
Jaiprakash, Singhai, A., Habib, G., Raman, R. S., and Gupta, T.: Chemical characterization of PM1.0 aerosol in Delhi and source apportionment using positive matrix factorization, Environ. Sci. Pollut. Res., 24, 445–462, https://doi.org/10.1007/s11356-016-7708-8, 2017.
Jena, C., Ghude, S. D., Kulkarni, R., Debnath, S., Kumar, R., Soni, V. K., Acharja, P., Kulkarni, S. H., Khare, M., Kaginalkar, A. J., Chate, D. M., Ali, K., Nanjundiah, R. S., and Rajeevan, M. N.: Evaluating the sensitivity of fine particulate matter (PM2.5) simulations to chemical mechanism in Delhi, Atmos. Chem. Phys. Discuss. [preprint], https://doi.org/10.5194/acp-2020-673, 2020.
Jena, C., Ghude, S. D., Kumar, R., Debnath, S., Govardhan, G., Soni, V. K., Kulkarni, S. H., Beig, G., Nanjundiah, R. S., and Rajeevan, M.: Performance of high resolution (400 m) PM2.5 forecast over Delhi, Sci. Rep., 11, 1–9, https://doi.org/10.1038/s41598-021-83467-8, 2021.
Knote, C., Hodzic, A., and Jimenez, J. L.: The effect of dry and wet deposition of condensable vapors on secondary organic aerosols concentrations over the continental US, Atmos. Chem. Phys., 15, 1–18, https://doi.org/10.5194/acp-15-1-2015, 2015.
Kulkarni, S. H., Ghude, S. D., Jena, C., Karumuri, R. K., Sinha, B., Sinha, V., Kumar, R., Soni, V. K., and Khare, M.: How Much Does Large-Scale Crop Residue Burning Affect the Air Quality in Delhi?, Environ. Sci. Technol., 54, 4790–4799, https://doi.org/10.1021/acs.est.0c00329, 2020.
Kumar, A., Hakkim, H., Ghude, S. D., and Sinha, V.: Probing wintertime air pollution sources in the Indo-Gangetic Plain through 52 hydrocarbons measured rarely at Delhi and Mohali, Sci. Total Environ., 801, 149711, https://doi.org/10.1016/j.scitotenv.2021.149711, 2021.
Kumar, R., Barth, M. C., Pfister, G. G., Delle Monache, L., Lamarque, J. F., Archer-Nicholls, S., Tilmes, S., Ghude, S. D., Wiedinmyer, C., Naja, M., and Walters, S.: How Will Air Quality Change in South Asia by 2050?, J. Geophys. Res.-Atmos., 123, 1840–1864, https://doi.org/10.1002/2017JD027357, 2018.
Kumar, R., Ghude, S. D., Biswas, M., Jena, C., Alessandrini, S., Debnath, S., Kulkarni, S., Sperati, S., Soni, V. K., Nanjundiah, R. S., and Rajeevan, M.: Enhancing Accuracy of Air Quality and Temperature Forecasts During Paddy Crop Residue Burning Season in Delhi Via Chemical Data Assimilation, J. Geophys. Res.-Atmos., 125, 1–16, https://doi.org/10.1029/2020JD033019, 2020.
Kuttippurath, J., Singh, A., Dash, S. P., Mallick, N., Clerbaux, C., Van Damme, M., Clarisse, L., Coheur, P. F., Raj, S., Abbhishek, K., and Varikoden, H.: Record high levels of atmospheric ammonia over India: Spatial and temporal analyses, Sci. Total Environ., 740, 139986, https://doi.org/10.1016/j.scitotenv.2020.139986, 2020.
Lan, Z., Lin, W., Pu, W., and Ma, Z.: Measurement report: Exploring NH3behavior in urban and suburban Beijing: Comparison and implications, Atmos. Chem. Phys., 21, 4561–4573, https://doi.org/10.5194/acp-21-4561-2021, 2021.
Laubach, J., Taghizadeh-Toosi, A., Gibbs, S. J., Sherlock, R. R., Kelliher, F. M., and Grover, S. P. P.: Ammonia emissions from cattle urine and dung excreted on pasture, Biogeosciences, 10, 327–338, https://doi.org/10.5194/bg-10-327-2013, 2013.
Leytem, A. B., Bjorneberg, D. L., Rotz, C. A., Moraes, L. E., Kebreab, E., and Dungan, R. S.: Ammonia emissions from dairy lagoons in the western U.S., Trans. ASABE, 61, 1001–1015, https://doi.org/10.13031/trans.12646, 2018.
Li, L., Chen, Z. M., Zhang, Y. H., Zhu, T., Li, S., Li, H. J., Zhu, L. H., and Xu, B. Y.: Heterogeneous oxidation of sulfur dioxide by ozone on the surface of sodium chloride and its mixtures with other components, J. Geophys. Res.-Atmos., 112, 1–13, https://doi.org/10.1029/2006JD008207, 2007.
Makkonen, U., Virkkula, A., Mäntykenttä, J., Hakola, H., Keronen, P., Vakkari, V., and Aalto, P. P.: Semi-continuous gas and inorganic aerosol measurements at a Finnish urban site: comparisons with filters, nitrogen in aerosol and gas phases, and aerosol acidity, Atmos. Chem. Phys., 12, 5617–5631, https://doi.org/10.5194/acp-12-5617-2012, 2012.
Meng, Z., Xu, X., Lin, W., Ge, B., Xie, Y., Song, B., Jia, S., Zhang, R., Peng, W., Wang, Y., Cheng, H., Yang, W., and Zhao, H.: Role of ambient ammonia in particulate ammonium formation at a rural site in the North China Plain, Atmos. Chem. Phys., 18, 167–184, https://doi.org/10.5194/acp-18-167-2018, 2018.
Metzger, S., Mihalopoulos, N., and Lelieveld, J.: Importance of mineral cations and organics in gas-aerosol partitioning of reactive nitrogen compounds: Case study based on MINOS results, Atmos. Chem. Phys., 6, 2549–2567, https://doi.org/10.5194/acp-6-2549-2006, 2006.
Móring, A., Hooda, S., Raghuram, N., Adhya, T. K., Ahmad, A., Bandyopadhyay, S. K., Barsby, T., Beig, G., Bentley, A. R., Bhatia, A., Dragosits, U., Drewer, J., Foulkes, J., Ghude, S. D., Gupta, R., Jain, N., Kumar, D., Kumar, R. M., Ladha, J. K., Mandal, P. K., Neeraja, C. N., Pandey, R., Pathak, H., Pawar, P., Pellny, T. K., Poole, P., Price, A., Rao, D. L. N., Reay, D. S., Singh, N. K., Sinha, S. K., Srivastava, R. K., Shewry, P., Smith, J., Steadman, C. E., Subrahmanyam, D., Surekha, K., Venkatesh, K., Varinderpal-Singh, Uwizeye, A., Vieno, M., and Sutton, M. A.: Nitrogen Challenges and Opportunities for Agricultural and Environmental Science in India, Front. Sustain. Food Syst., 5, 505347, https://doi.org/10.3389/fsufs.2021.505347, 2021.
Nair, A. A. and Yu, F.: Quantification of atmospheric ammonia concentrations: A review of its measurement and modeling, Atmosphere, 11, atmos11101092, https://doi.org/10.3390/atmos11101092, 2020.
Nenes, A., Pandis, S. N., Weber, R. J., and Russell, A.: Aerosol pH and liquid water content determine when particulate matter is sensitive to ammonia and nitrate availability, Atmos. Chem. Phys., 20, 3249–3258, https://doi.org/10.5194/acp-20-3249-2020, 2020.
Nivdange, S., Jena, C., and Pawar, P.: Nationwide CoViD-19 lockdown impact on air quality in India, Mausam, 73, 115–128, https://doi.org/10.54302/mausam.v73i1.1475, 2022.
Pawar, P. V., Ghude, S. D., Jena, C., Móring, A., Sutton, M. A., Kulkarni, S., Lal, D. M., Surendran, D., Van Damme, M., Clarisse, L., Coheur, P.-F., Liu, X., Govardhan, G., Xu, W., Jiang, J., and Adhya, T. K.: Analysis of atmospheric ammonia over South and East Asia based on the MOZART-4 model and its comparison with satellite and surface observations, Atmos. Chem. Phys., 21, 6389–6409, https://doi.org/10.5194/acp-21-6389-2021, 2021.
Pinder, R. W., Adams, P. J., and Pandis, S. N.: Ammonia Emission Controls as a Cost-Effective Strategy for Reducing Atmospheric Particulate Matter in the Eastern United States, Environ. Sci. Technol., 41, 380–386, https://doi.org/10.1021/es060379a, 2007.
Pinder, R. W., Gilliland, A. B., and Dennis, R. L.: Environmental impact of atmospheric NH3 emissions under present and future conditions in the eastern United States, Geophys. Res. Lett., 35, L12808, https://doi.org/10.1029/2008GL033732, 2008.
Saraswati, George, M. P., Sharma, S. K., Mandal, T. K., and Kotnala, R. K.: Simultaneous Measurements of Ambient NH3 and Its Relationship with Other Trace Gases, PM2.5 and Meteorological Parameters over Delhi, India, Mapan – J. Metrol. Soc. India, 34, 55–69, https://doi.org/10.1007/s12647-018-0286-0, 2019.
Seinfeld, J. H. and Pandis, S. N.: Atmospheric chemistry and physics: from air pollution to climate change, Phys. Today, 51, 88–90, https://doi.org/10.1063/1.882420, 1998.
Seinfeld, J. H., Bretherton, C., Carslaw, K. S., Coe, H., DeMott, P. J., Dunlea, E. J., Feingold, G., Ghan, S., Guenther, A. B., Kahn, R., Kraucunas, I., Kreidenweis, S. M., Molina, M. J., Nenes, A., Penner, J. E., Prather, K. A., Ramanathan, V., Ramaswamy, V., Rasch, P. J., Ravishankara, A. R., Rosenfeld, D., Stephens, G., and Wood, R.: Improving our fundamental understanding of the role of aerosol–cloud interactions in the climate system, P. Natl. Acad. Sci. USA, 113, 5781, https://doi.org/10.1073/pnas.1514043113, 2016.
Sha, T., Ma, X., Jia, H., Tian, R., Chang, Y., Cao, F., and Zhang, Y.: Aerosol chemical component: Simulations with WRF-Chem and comparison with observations in Nanjing, Atmos. Environ., 218, 116982, https://doi.org/10.1016/j.atmosenv.2019.116982, 2019.
Sharma, C., Tiwari, M. K., and Pathak, H.: Estimates of emission and deposition of reactive nitrogenous species for India, Curr. Sci., 94, 1439–1446, 2008.
Sharma, G., Sinha, B., Pallavi, Hakkim, H., Chandra, B. P., Kumar, A., and Sinha, V.: Gridded Emissions of CO, NOx, SO2, CO2, NH3, HCl, CH4, PM2.5, PM10, BC, and NMVOC from Open Municipal Waste Burning in India, Environ. Sci. Technol., 53, 4765–4774, https://doi.org/10.1021/acs.est.8b07076, 2019.
Sharma, S. K., Saxena, M., Saud, T., Korpole, S., and Mandal, T. K.: Measurement of NH3, NO, NO2 and related particulates at urban sites of indo gangetic plain (IGP) of India, J. Sci. Ind. Res., 71, 360–362, 2012.
Sharma, S. K., Harit, R. C., Kumar, V., Mandal, T. K., and Pathak, H.: Ammonia Emission from Rice-Wheat Cropping System in Subtropical Soil of India, Agric. Res., 3, 175–180, https://doi.org/10.1007/s40003-014-0107-9, 2014a.
Sharma, S. K., Kumar, M., Rohtash, Gupta, N. C., Saraswati, Saxena, M., and Mandal, T. K.: Characteristics of ambient ammonia over Delhi, India, Meteorol. Atmos. Phys., 124, 67–82, https://doi.org/10.1007/s00703-013-0299-8, 2014b.
Sharma, S. K., Kotnala, G., and Mandal, T. K.: Spatial Variability and Sources of Atmospheric Ammonia in India: A Review, Aerosol Sci. Eng., 4, 1–8, https://doi.org/10.1007/s41810-019-00052-3, 2020.
Sherlock, R. R., Freney, J. R., Bacon, P. E., and van der Weerden, T. J.: Estimating ammonia volatilization from unsaturated urea fertilized and urine affected soils by an indirect method, Fertil. Res., 40, 197–205, https://doi.org/10.1007/BF00750466, 1994.
Singh, G. K., Rajeev, P., Paul, D., and Gupta, T.: Chemical characterization and stable nitrogen isotope composition of nitrogenous component of ambient aerosols from Kanpur in the Indo-Gangetic Plains., Sci. Total Environ., 763, 143032, https://doi.org/10.1016/j.scitotenv.2020.143032, 2021.
Sinha, B., Sharma, G., Jangra, P., Hakim, H., Chandra, B. P., Sharma, A. K., and Sinha, V.: Gridded emissions of CO, NOx, SO2, CO2, NH3, HCl, CH4, PM2.5, PM10, BC and NMVOCs emissions from open municipal waste burning in India, Mendeley Data [data set], V1, https://doi.org/10.17632/546t9249bv.1, 2019.
Stieger, B., Spindler, G., van Pinxteren, D., Grüner, A., Wallasch, M., and Herrmann, H.: Development of an online-coupled MARGA upgrade for the 2\,h interval quantification of low-molecular-weight organic acids in the gas and particle phases, Atmos. Meas. Tech., 12, 281–298, https://doi.org/10.5194/amt-12-281-2019, 2019.
Sutton, M. A. and Howard, C. M.: Ammonia maps make history, Nature, 564, 49–50, 2018.
Sutton, M. A., Burkhardt, J. K., Guerin, D., Nemitz, E., and Fowler, D.: Development of resistance models to describe measurements of bi-directional ammonia surface-atmosphere exchange, Atmos. Environ., 32, 473–480, https://doi.org/10.1016/S1352-2310(97)00164-7, 1998.
Sutton, M. A., Erisman, J. W., Dentener, F., and Möller, D.: Ammonia in the environment: From ancient times to the present, Environ. Pollut., 156, 583–604, https://doi.org/10.1016/j.envpol.2008.03.013, 2008.
Sutton, M. A., Reis, S. ,and Baker, S. M. H.: Atmospheric Ammonia: Detecting emission changes and environmental impacts, Results of an Expert Workshop under the Convention on Long-range Transboundary Air Pollution, Springer, thw Netherlands, ISBNs: 1402091206, 9781402091209, 464 pp., 2009a.
Sutton, M. A., Nemitz, E., Milford, C., Campbell, C., Erisman, J. W., Hensen, A., Cellier, P., David, M., Loubet, B., Personne, E., Schjoerring, J. K., Mattsson, M., Dorsey, J. R., Gallagher, M. W., Horvath, L., Weidinger, T., Meszaros, R., Dämmgen, U., Neftel, A., Herrmann, B., Lehman, B. E., Flechard, C., and Burkhardt, J.: Dynamics of ammonia exchange with cut grassland: synthesis of results and conclusions of the GRAMINAE Integrated Experiment, Biogeosciences, 6, 2907–2934, https://doi.org/10.5194/bg-6-2907-2009, 2009b.
Sutton, M. A., Reis, S., Riddick, S. N., Dragosits, U., Nemitz, E., Theobald, M. R., Tang, Y. S., Braban, C. F., Vieno, M., Dore, A. J., Mitchell, R. F., Wanless, S., Daunt, F., Fowler, D., Blackall, T. D., Milford, C., Flechard, C. R., Loubet, B., Massad, R., Cellier, P., Personne, E., Coheur, P. F., Clarisse, L., Van Damme, M., Ngadi, Y., Clerbaux, C., Skjøth, C. A., Geels, C., Hertel, O., Kruit, R. J. W., Pinder, R. W., Bash, J. O., Walker, J. T., Simpson, D., Horváth, L., Misselbrook, T. H., Bleeker, A., Dentener, F., and de Vries, W.: Towards a climate-dependent paradigm of ammonia emission and deposition, Philos. Trans. R. Soc. B, 368, 20130166–20130166, https://doi.org/10.1098/rstb.2013.0166, 2013.
Sutton, M. A., Drewer, J., Moring, A., Adhya, T. K., Ahmed, A., Bhatia, A., Brownlie, W., Dragosits, U., Ghude, S. D., Hillier, J., Hooda, S., Howard, C. M., Jain, N., Kumar, D., Kumar, R. M., Nayak, D. R., Neeraja, C. N., Prasanna, R., Price, A., Ramakrishnan, B., Reay, D. S., Singh, R., Skiba, U., Smith, J. U., Sohi, S., Subrahmanyan, D., Surekha, K., van Grinsven, H. J. M., Vieno, M., Voleti, S. R., Pathak, H., and Raghuram, N.: 2 – The Indian Nitrogen Challenge in a Global Perspective, in: The Indian Nitrogen Assessment, edited by: Abrol, Y. P., Adhya, T. K., Aneja, V. P., Raghuram, N., Pathak, H., Kulshrestha, U., Sharma, C., and Singh, B., 9–28, Elsevier, ISBN: 978-0-12-811836-8, 2017.
Sutton, M. A., Van Dijk, N., Levy, P. E., Jones, M. R., Leith, I. D., Sheppard, L. J., Leeson, S., Sim Tang, Y., Stephens, A., Braban, C. F., Dragosits, U., Howard, C. M., Vieno, M., Fowler, D., Corbett, P., Naikoo, M. I., Munzi, S., Ellis, C. J., Chatterjee, S., Steadman, C. E., Móring, A., and Wolseley, P. A.: Alkaline air: changing perspectives on nitrogen and air pollution in an ammonia-rich world: Alkaline Air, Philos. Trans. R. Soc. A, 378, 20190315, https://doi.org/10.1098/rsta.2019.0315, 2020.
Technical specifications for CAAQM station: Technical specifications for CAAQM station: Technical Specifications for CAAQM station: Real time, Central Pollution Control Board, East Arjun Nagar, Shahdara, India, available at: https://erc.mp.gov.in/Documents/doc/Guidelines/CAAQMS_Specs_new.pdf (last access: 10 May 2021), 2019.
Thomas, R. M., Trebs, I., Otjes, R., Jongejan, P. A. C., Brink, H. ten, Phillips, G., Kortner, M., Meixner, F. X., and Nemitz, E.: An Automated Analyzer to Measure Surface-Atmosphere Exchange Fluxes of Water Soluble Inorganic Aerosol Compounds and Reactive Trace Gases, Environ. Sci. Technol., 43, 1412–1418, https://doi.org/10.1021/es8019403, 2009.
Twigg, M. M., Di Marco, C. F., Leeson, S., van Dijk, N., Jones, M. R., Leith, I. D., Morrison, E., Coyle, M., Proost, R., Peeters, A. N. M., Lemon, E., Frelink, T., Braban, C. F., Nemitz, E., and Cape, J. N.: Water soluble aerosols and gases at a UK background site – Part 1: Controls of PM2.5 and PM10 aerosol composition, Atmos. Chem. Phys., 15, 8131–8145, https://doi.org/10.5194/acp-15-8131-2015, 2015.
Van Damme, M., Clarisse, L., Whitburn, S., Hadji-Lazaro, J., Hurtmans, D., Clerbaux, C., and Coheur, P. F.: Industrial and agricultural ammonia point sources exposed, Nature, 564, 99–103, https://doi.org/10.1038/s41586-018-0747-1, 2018.
Wagh, S., Singh, P., Ghude, S. D., Safai, P., Prabhakaran, T., and Kumar, P. P.: Study of ice nucleating particles in fog-haze weather at New Delhi, India: A case of polluted environment, Atmos. Res., 259, 105693, https://doi.org/10.1016/j.atmosres.2021.105693, 2021.
Wang, Q., Miao, Y., and Wang, L.: Regional transport increases ammonia concentration in Beijing, China, Atmosphere, 11, atmos11060563, https://doi.org/10.3390/ATMOS11060563, 2020.
Wang, S., Nan, J., Shi, C., Fu, Q., Gao, S., Wang, D., Cui, H., Saiz-Lopez, A., and Zhou, B.: Atmospheric ammonia and its impacts on regional air quality over the megacity of Shanghai, China, Sci. Rep., 5, 1–13, https://doi.org/10.1038/srep15842, 2015.
Wang, T., Song, Y., Xu, Z., Liu, M., Xu, T., Liao, W., Yin, L., Cai, X., Kang, L., Zhang, H., and Zhu, T.: Why is the Indo-Gangetic Plain the region with the largest NH3 column in the globe during pre-monsoon and monsoon seasons?, Atmos. Chem. Phys., 20, 8727–8736, https://doi.org/10.5194/acp-20-8727-2020, 2020.
Warner, J. X., Dickerson, R. R., Wei, Z., Strow, L. L., Wang, Y., and Liang, Q.: Increased atmospheric ammonia over the world's major agricultural areas detected from space, Geophys. Res. Lett., 44, 2875–2884, https://doi.org/10.1002/2016GL072305, 2017.
Wentworth, G. R., Murphy, J. G., Gregoire, P. K., Cheyne, C. A. L., Tevlin, A. G., and Hems, R.: Soil-atmosphere exchange of ammonia in a non-fertilized grassland: Measured emission potentials and inferred fluxes, Biogeosciences, 11, 5675–5686, https://doi.org/10.5194/bg-11-5675-2014, 2014.
Wentworth, G. R., Murphy, J. G., Benedict, K. B., Bangs, E. J., and Collett, J. L.: The role of dew as a night-time reservoir and morning source for atmospheric ammonia, Atmos. Chem. Phys., 16, 7435–7449, https://doi.org/10.5194/acp-16-7435-2016, 2016.
Xu, J., Chen, J., Huo, J., Lin, Y., Fu, Q., Guo, H., and Lee, S. H.: Importance of gas-particle partitioning of ammonia in haze formation in the rural agricultural environment, Atmos. Chem. Phys., 20, 7259–7269, https://doi.org/10.5194/acp-20-7259-2020, 2020.
Yang, J., Kang, S., and Ji, Z.: Sensitivity analysis of chemical mechanisms in the WRF-chem model in reconstructing aerosol concentrations and optical properties in the Tibetan Plateau, Aerosol Air Qual. Res., 18, 505–521, https://doi.org/10.4209/aaqr.2017.05.0156, 2018.
Zaveri, R. A., Easter, R. C., Fast, J. D., and Peters, L. K.: Model for Simulating Aerosol Interactions and Chemistry (MOSAIC), J. Geophys. Res.-Atmos., 113, 1–29, https://doi.org/10.1029/2007JD008782, 2008.
Zhang, X., Liu, J., Han, H., Zhang, Y., Jiang, Z., Wang, H., Meng, L., Li, Y. C., and Liu, Y.: Satellite-Observed Variations and Trends in Carbon Monoxide over Asia and Their Sensitivities to Biomass Burning, Remote Sens., 12, 1–26, https://doi.org/10.3390/rs12050830, 2020.