the Creative Commons Attribution 4.0 License.
the Creative Commons Attribution 4.0 License.
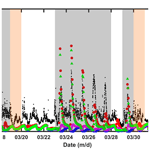
Analysis of new particle formation events and comparisons to simulations of particle number concentrations based on GEOS-Chem–advanced particle microphysics in Beijing, China
Rong Tian
Fangqun Yu
Aerosol particles play important roles in air quality and global climate change. In this study, we analyze the measurements of particle size distribution from 12 March to 6 April 2016 in Beijing to characterize new particle formation (NPF) using the observational data of sulfuric acid, meteorological parameters, solar radiation, and the mass concentration of fine particles (PM2.5, particulate matter with diameters less than 2.5 µm). During this 26 d campaign, 11 new particle formation events are identified with obvious bursts of sub-3 nm particle number concentrations and subsequent growth of these nucleated particles. It is found that sulfuric acid concentration in Beijing does not have a significant difference between NPF event and non-event days. Low relative humidity (RH) and high daily total solar radiation appear to be favorable for the occurrence of NPF events, which is quite obvious in this campaign. The simulations using four nucleation schemes, i.e., H2SO4–H2O binary homogeneous nucleation (BHN), H2SO4–H2O–NH3 ternary homogeneous nucleation (THN), H2SO4–H2O–ion binary ion-mediated nucleation (BIMN), and H2SO4–H2O–NH3–ion ternary ion-mediated nucleation (TIMN), based on a global chemistry transport model (GEOS-Chem) coupled with an advanced particle microphysics (APM) model, are conducted to study the particle number concentrations and new particle formation process. Our comparisons between measurements and simulations indicate that the BHN scheme and BIMN scheme significantly underestimate the observed particle number concentrations, and the THN scheme captures the total particle number concentration on most NPF event days well but fails to capture the noticeable increase in particle number concentrations on 18 March and 1 April. The TIMN scheme has obvious improvement in terms of total and sub-3 nm particle number concentrations and nucleation rates. This study provides a basis for further understanding of the nucleation mechanism in Beijing.
- Article
(6524 KB) - Full-text XML
- BibTeX
- EndNote
New particle formation (NPF) is the process by which gaseous pollutants transform into particles (gas–particle reaction) then grow into particles with larger particle size through further collision and condensation, which is the main source of particle number abundance generated by secondary transformation in the atmosphere (Yu et al., 2008; Merikanto et al., 2009).
With the development of industry and the increase in human activities, air quality is deteriorating day by day, and atmospheric particulate matter has become one of the major sources of air pollutants. Particulate matter not only affects the radiation balance by scattering and absorbing solar radiation but also indirectly modifies the climate by acting as cloud condensation nuclei (CCN) (Merikanto et al., 2009). In addition, new particles can also impact the formation of fog and haze. NPF is capable of increasing PM2.5 mass concentration and haze particle (diameter>100 nm) number concentration (Kulmala et al., 2022). In the context of the decline in pollutant emissions caused by the lockdown during the COVID-19 pandemic, new particles derived from NPF played a significant role in the formation of haze (Tang et al., 2021). Guo et al. (2014) found that after an NPF event, smog–haze pollution continued to occur in Beijing, indicating that aerosol nucleation and growth led to the development of PM2.5. When the concentration of atmospheric particulate matter is high, atmospheric visibility will be reduced in fog and haze weather, and a high concentration of atmospheric fine particulate matter will also harm human health (Kaiser, 2005) as tiny aerosol particles can enter the human body through the respiratory system.
At present, the nucleation and growth of new particles have been widely studied around the world. Sipilä et al. (2010) demonstrated a positive relationship between nucleation rate and sulfuric acid concentration. Kulmala et al. (2013) established a framework of atmospheric nucleation at three different scales below 2 nm based on observations, identifying the participation of sulfuric acid in the nucleation process and emphasizing the important role of organic compounds in atmospheric aerosols. A positive correlation between nucleation rate and sulfuric acid concentration (or H2SO4 proxy) was also found in many NPF studies in China (Xiao et al., 2015; Dai et al., 2017).
Besides sulfuric acid, a number of other nucleating precursors, including atmospheric ions, amines, ammonia, iodine oxides, and organic acids, have been proposed to be involved in the formation of the critical nucleus under different ambient environments (Zhang et al., 2012; Kirkby et al., 2016; Chu et al., 2019). Amines and ammonia are crucial in NPF because of their ability to stabilize sulfuric acid clusters by forming acid–base complexes (Yao et al., 2018). Currently, several major theories have been proposed to explain the phenomenon of nucleation in the atmosphere. H2SO4–H2O binary theory usually predicts the nucleation rates at low temperatures, high relative humidities, small preexisting aerosol concentrations, and high sulfuric acid concentrations (Kulmala et al., 1998, 2000). For NH3 mixing ratios exceeding about 1 ppt, the H2SO4–NH3–H2O ternary nucleation enhances the binary H2SO4–H2O nucleation rate by several orders of magnitude (Korhonen et al., 1999). The ion-mediated nucleation mechanism provides a consistent explanation for a variety of tropospheric observations (Yu and Turco, 2000). Organics-mediated nucleation can explain NPF in some polluted areas (Wang et al., 2015). The HIO3 nucleation mechanism is also found to dominate NPF in the coastal regions (Sipilä et al., 2016).
There are some special features associated with NPF events found in China. For example, the concentrations of nucleating precursors and preexisting aerosol particles both can be quite high in polluted cities, which is different from cleaner environments (Kulmala et al., 2016). The observed nucleation rate of 1.5 nm particles and NPF frequency in urban Beijing usually show an obvious seasonal variation with maxima in winter (Deng et al., 2020). Jayaratne et al. (2017) conducted the observations in Beijing during the winter of 2015 and did not observe any NPF event when the daily mean PM2.5 concentrations were higher than 43 µg m−3. However, in some cases, the condensation sinks (CSs) or the average coagulation sinks are not significantly lower during NPF events compared to non-NPF times, suggesting that other factors, such as the precursor vapor and photochemical activity, may also play an important role in driving NPF (Gong et al., 2010). Yan et al. (2021) found that sulfuric acid and base molecules were responsible for the initial steps of NPF during a wintertime measurement in Beijing. In addition, H2SO4–amine nucleation can explain the observed high new particle nucleation rate under the high coagulation sink, and dimethylamine (DMA) is the key base for H2SO4–base nucleation in highly polluted urban environments (Cai et al., 2021, 2022). Beijing is a representative city in northern China because of its developed industry and commerce and thus quite high concentrations of atmospheric precursors and preexisting aerosol particles. Wu et al. (2007) found that NPF days accounted for 40 % of the observation days from March 2004 to February 2005 in Beijing, which are usually sunny and dry days. Continuous and comprehensive long-term observations will help to understand the mechanism of NPF and answer the key participants and processes of NPF under complex air conditions in China. Besides, laboratory experiments and model simulations will also be very necessary. Chen et al. (2019) investigated the effect of the organics-involved nucleation scheme on NPF in Beijing, but previous model studies and comparisons based on different nucleation schemes in Beijing are still lacking.
Since nucleation is a key process controlling particle properties in the atmosphere, to better understand the formation and evolution mechanisms of air pollution, especially in heavy pollution areas, it is necessary to assess the applicability of nucleation parametrizations currently available. This study aims to evaluate the performance of four currently widely used nucleation schemes and provide some clues on the contribution of different nucleation pathways to aerosol number concentrations. The paper is organized as below. We first analyze the favorable background of new particle formation in Beijing and then conduct the simulations using four nucleation schemes, i.e., H2SO4–H2O binary homogeneous nucleation (BHN) (Yu, 2007, 2008), H2SO4–H2O–NH3 ternary homogeneous nucleation (THN) (Yu, 2006a), H2SO4–H2O–ion binary ion-mediated nucleation (BIMN) (Yu, 2006b), and H2SO4–H2O–NH3–ion ternary ion-mediated nucleation (TIMN) (Yu et al., 2018), based on a global chemistry transport model (GEOS-Chem), and compare these with the observed NPF events from the measurements from 12 March to 6 April 2016 in Beijing to understand the nucleation mechanism.
2.1 Observations
The observational data used in this paper include particle size distributions, temperature (T), relative humidity (RH), and sulfuric acid concentration, which were provided by Cai et al. (2017b). The measurement field was located on the top floor of a four-story building in the center of the campus of Tsinghua University in Beijing, and all observations were collected during the period from 12 March to 6 April 2016. A homemade diethylene glycol scanning mobility particle spectrometer (DEG-SMPS) was used to measure 1–5 nm particle size distributions, and the particle size distributions from 3 nm to 10 µm (in aerodynamic diameter; Liu et al., 2016) were measured by a particle size distribution system (including a TSI aerodynamic particle sizer and two parallel SMPSs, equipped with a TSI nano-DMA and a TSI long-DMA, respectively) with a resolution of 5 min. A specially designed miniature cylindrical differential mobility analyzer (mini-cyDMA) for effective classification of 1–3 nm (sub-3 nm) aerosol was equipped with the DEG-SMPS (Cai et al., 2017a). Sulfuric acid was measured by a modified high-resolution time-of-flight chemical ionization mass spectrometer (HR-ToF-CIMS; Aerodyne Research Inc.) with a resolution of 5 min. Further details of the measurement methods can be found in Cai et al. (2017b).
In addition, the daily PM2.5 and PM10 mass concentrations provided by the China National Environmental Monitoring Center (http://www.cnemc.cn/, last access: 20 November 2022) and solar radiation datasets, including daily maximum solar radiation flux density and the daily total solar radiation, measured by the National Meteorological Information Center of the China Meteorological Administration (http://data.cma.cn/, last access: 20 November 2022), are also employed in this study.
2.2 Model description
The GEOS-Chem model is a global three-dimensional chemical transport model driven by assimilated meteorological observations from the Goddard Earth Observing System (GEOS) of the NASA Global Modeling Assimilation Office (GMAO). The GEOS-Chem model includes a detailed simulation of tropospheric O3–NOx–hydrocarbon chemistry as well as aerosols and their precursors (Park et al., 2004). An advanced particle microphysics (APM) model has been coupled with GEOS-Chem to study detailed particle formation and growth processes in the global atmosphere (Yu and Luo, 2009). The APM model is an advanced multi-type, multi-component, size-resolved microphysics code developed for explaining atmospheric particle observations (e.g., Yu, 2006b; Yu and Luo, 2009; Yu and Turco, 2011). The basic microphysical processes in APM include nucleation, coagulation, condensation/evaporation, thermodynamic equilibrium with local humidity, and dry deposition. In GEOS-Chem–APM, sulfate (or secondary) particles are represented by 40 sectional bins with dry diameters ranging from 1.2 nm to 12 µm, including 30 bins for the 1.2–120 nm range and another 10 bins for 0.12–12 µm. In the model, new particle formation is parameterized by ion-mediated nucleation (IMN), which is based on physics and constrained by laboratory data (Yu, 2006b) and can finely predict global nucleation distributions with a reasonable consistency (Yu et al., 2008). In addition, the IMN takes into account the complex interactions among small air ions, neutral and charged clusters of various sizes, precursor vapor molecules, and preexisting aerosols. The growth of nucleated particles through the condensation of sulfuric acid vapor and equilibrium uptake of nitrate, ammonium, and secondary organic aerosol (SOA) is explicitly simulated, along with the scavenging of secondary particles by primary particles (dust, black carbon, organic carbon, and sea salt) (Yu and Luo, 2009). Yu (2011) has further developed the APM module to explicitly calculate the co-condensation of H2SO4 and low-volatility secondary organic gases (LV-SOGs) or low-volatility organic compounds (LVOCs) on secondary particles and primary particles. The aerosol simulation considered the successive oxidation aging of the oxidation products of various volatile organic compounds (VOCs) (Yu, 2011). New particle formation and particle number concentrations predicted by the GEOS-Chem–APM model have been extensively evaluated against a wide range of aircraft-, land-, and ship-based field data (Yu and Luo, 2009, 2010; Yu et al., 2010). In recent years, the APM model has also been coupled with other numerical models such as the Nested Air Quality Prediction Modeling System (NAQPMS) and the Weather Research and Forecast model coupled with Chemistry (WRF-Chem) to study new particle formation (Luo and Yu, 2011; Chen et al., 2019).
In this study, we use a version of GEOS-Chem (v12.6.0) driven by the GEOS Forward Processing (GEOS-FP) assimilated meteorological field, with a spatial resolution of and 47 vertical levels during the period of 1 March to 7 April 2016. We focus on four nucleation schemes to compare simulations with particle number concentration measurements from 12 March to 7 April in this study.
3.1 Occurrence of new particle formation events
NPF generally includes the following two steps: (1) condensable vapors are produced via chemical reactions, then gaseous vapors form critical clusters through a gas–particle reaction. (2) The critical clusters continue to grow to a larger size (1–2 nm) (Kulmala et al., 2013) and compete with preexisting particles to survive in the collision and removal processes; at the same time, in this quasi-steady-state process, new particles can only be formed when growth is dominant.
Currently, there is no unique mathematical criterion or definition for NPF events. Dal Maso et al. (2005) proposed a criterion to justify NPF events; i.e., a new mode of particles start in the nucleation-mode size range and prevail within a few hours and show signs of growth. The days without the particles in the nucleation-mode are called non-event days. It is noted that some days are not easily classified as either NPF days or non-event days, so these days are usually classified undefined days. The criteria to determine NPF in this study are as follows: (1) high concentrations of sub-3 nm clusters/particles appear over a time of hours at the onset of the event, and (2) there is subsequent growth to larger sizes for a few hours.
Figure 1 shows the particle size distributions observed during 12 March–6 April 2016 in Beijing. According to the criteria of NPF events defined above, 11 typical event days and 13 non-event days are identified during the 26 d campaign. The other 2 d, i.e., 19 and 30 March, when the sub-3 nm particle number concentration is relatively low and the evolution of particle size distributions is not continuous, are identified as undefined days. The NPF events are observed with a high frequency (42 %) in spring in this campaign, which is similar to the previous study in Beijing (Wu et al., 2007) in which it was found that spring is usually the season with the highest frequency of NPF events in northern China. One explanation is that stronger wind from the north removes preexisting particles to create a clean regime, which further leads to the occurrence of NPF events (Wu et al., 2008; Cai et al., 2017b; Chu et al., 2019; Wu et al., 2020).
3.2 Analysis based on observations
3.2.1 The sulfuric acid concentration on NPF days and non-event days
Since the number concentration of new particulate matter in the atmosphere is strongly dependent on the concentration of sulfuric acid, sulfuric acid is a key precursor species for atmospheric nucleation and has an important contribution to NPF events (Sipilä et al., 2010). However, it was not easy to measure the concentration of sulfuric acid a few years ago. With the development of technology, chemical ionization mass spectrometry (CIMS) has been used to detect gaseous sulfuric acid in the atmosphere (Zheng et al., 2011, 2015).
The gaseous sulfuric acid in the atmosphere is mainly produced by the oxidation reaction of sulfur dioxide and OH. Stockwell and Calvert (1983) demonstrated the reaction as follows:
Figure 2 shows the average diurnal concentration of sulfuric acid on the NPF event days and non-event days. The gaseous H2SO4 concentrations exhibit significant diurnal variation, and sulfuric acid concentration closely follows the diurnal solar cycle because of its short atmospheric lifetime (less than 1 min) (Zheng et al., 2011). It is known that NPF events usually occur within a few hours after sunrise and end in the afternoon. During the start period, the average sulfuric acid concentration on NPF days is close to that on non-event days, and compared to NPF days, the average sulfuric acid concentration on non-event days is not significantly low during the whole time period. The average sulfuric acid concentration during non-event periods, cm−3, is obviously higher than that between 06:00 and 18:00 LT on NPF days, cm−3.
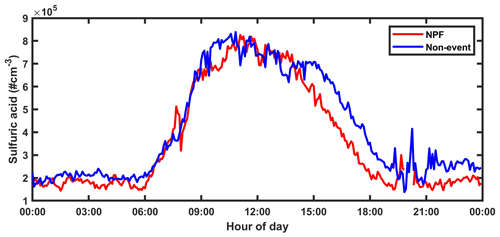
Figure 2Averaged diurnal cycles of sulfuric acid concentrations for NPF days and non-event days during the 26 d measurement period.
The daily maximum sulfuric acid concentrations measured in this study (>106 cm−3) are lower than those in the summer of 2017 in Beijing (>107 cm−3) (Wu et al., 2020) and close to those in the winter of 2019 in Beijing (>106 cm−3) (Foreback et al., 2022). This might be caused by the relatively weak solar radiation intensity in springtime and wintertime measurements compared with summertime observation. In this study, H2SO4 in Beijing during the campaign period is sufficiently high for nucleation to occur, and NPF events appear to be governed by the aerosol Fuchs surface area, which is a representative parameter of coagulation scavenging (Cai et al., 2017b). Yan et al. (2021) also found that observed H2SO4 concentrations were higher on non-NPF days than on NPF days in the winter of 2018. Therefore, it is shown that the sulfuric acid concentration in Beijing is sufficient to lead to NPF, which is consistent with some earlier studies in Nanjing (An et al., 2015; Qi et al., 2015) and Shanghai (Xiao et al., 2015). There is usually enough SO2 for NPF to occur under heavily polluted conditions (Herrmann et al., 2014). The observed SO2 concentrations during October 2015 showed that the most polluted area was located in northern China (Hu et al., 2022). The surfaces of atmospheric aerosols correspond to the major sink for gas-phase sulfuric acid, but an increase in atmospheric sulfuric acid does not always result in more frequent NPF events (Zhang et al., 2012). So far, the presence of gaseous sulfuric acid in concentrations exceeding 105 molecules cm−3 has been shown as a necessary condition for NPF occurrence in the atmosphere (Weber et al., 1999; Nieminen et al., 2009). Overall, the previous results seem to indicate that adequate sulfuric acid concentration was not a limiting factor for NPF in Chinese megacities even if it was necessary.
3.2.2 Solar radiation and meteorological conditions for NPF
Air pollutants and meteorological conditions are usually studied together with nanoparticles and their precursors. By comparing the pollution characteristics between NPF event and other non-event days, we can obtain some clues on key factors affecting NPF events. Figure 3 shows the scatter plots of the daily total solar radiation and relative humidity, color-coded with PM2.5 mass concentration. The daily values were averaged between 08:00 and 16:00 LT because NPF events usually occur during the daytime. It is found that the favorable conditions on NPF days were similar, which were significantly different from the conditions on non-event days.
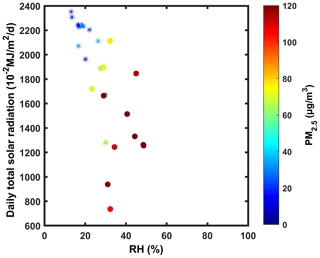
Figure 3Scatter plot of NPF days (asterisks), non-event days (circles), and undefined days (triangles) between daily total radiation and RH, color-coded with PM2.5 mass concentration.
The statistics indicate that the average RH for the 11 NPF days and 13 non-event days was 16 % and 37 %, respectively, implying that NPF events in Beijing likely occur on days with low relative humidity. The explanation is possibly that at high RH, coagulation scavenging of sub-3 nm clusters was enhanced, while reduced solar radiation led to gas-phase oxidation chemistry being diminished, and also the condensation sink of condensable gases was increased due to hygroscopic growth of the preexisting particles (Hamed et al., 2011). Meanwhile, the variation of solar radiation could influence new particle formation via photochemical processes (Shen et al., 2021). Strong solar radiation favors OH formation by a photochemical reaction, then OH is involved in processes of sulfate formation and VOC oxidation and produces sulfuric acid and vapors with low volatility in the atmosphere. Finally, these vapors with low volatility and sulfuric acid molecules can condense together to form new clusters and lead to NPF (Zhang et al., 2012). Laboratory studies and field observations have indicated that organic vapors participate in the particle nucleation besides sulfuric acid (Metzger et al., 2010; Yao et al., 2018). Qiao et al. (2021) found that oxygenated organic molecules significantly promoted the growth of 3–25 nm particles. Kulmala et al. (2022) found that the variability of growth rates of particles originating from NPF depends only weakly on H2SO4 concentrations. In addition, low-volatility organic compounds were proposed to contribute to the particle growth rate (Tröstl et al., 2016). Sulfuric acid was enough to explain the observed growth for particles smaller than 3 nm but was insufficient to explain the observed growth rates of large particles (Xiao et al., 2015; Yao et al., 2018). Therefore, when solar radiation was high, the low-volatility organic vapors generated by VOC oxidation may promote particle growth. Besides, it is proposed that solar radiation may create a turbulence flow and then strengthen the source during the nucleation process and reduce the sink in the growth process (Wu et al., 2020).
Since the meteorological factors have systematical influences on NPF, it is difficult to isolate the effect of a single factor on NPF. For example, higher solar radiation intensity usually increases the temperature, but the role of temperature in NPF was inconsistent in many studies. Qi et al. (2015) found that NPF was accompanied by a higher temperature in the spring, summer, and autumn of 2011–2013 in Nanjing, but Sun et al. (2021) found that temperature had no significant role in NPF in the summertime and wintertime in the coastal area of Qingdao, China. Yan et al. (2021) found that mean temperature in NPF event and non-event days was almost identical during the winter of 2018 in Beijing. It seems that the temperature associated with NPF events in various cities/regions has different characteristics. Besides, Xiao et al. (2021) demonstrated that NPF in polluted urban environments was largely driven by H2SO4–base clusters, stabilized by the presence of amines, high ammonia concentrations, and lower temperatures. A negative correlation between PM2.5 mass concentration and the occurrence of NPF events shown in Fig. 3 indicates that NPF likely occurs when PM2.5 concentration and gas pollutant concentrations are both low (Wu et al., 2007). A previous study found that high PM2.5 concentrations suppress NPF by increasing the sinks of vapor responsible for nucleation and growth of clusters and nucleation-mode particles (Zhou et al., 2020); new particle formation will be typically suppressed when the aerosol surface area exceeds 100 µm2 cm−3 (Aalto et al., 2001). Wu et al. (2020) found that unstable atmospheric turbulence will effectively reduce the CS by diluting preexisting aerosol particles, so as to promote nucleation. Therefore, more measurements of meteorological parameters and gaseous precursors can help improve our understanding of NPF in the future.
3.3 GEOS-Chem–APM model simulation
In order to understand the particle nucleation mechanism in polluted areas such as Beijing and reduce uncertainties in model simulations and predictions, we conduct the simulations from four nucleation schemes based on GEOS-Chem–APM. To ensure the input meteorological parameters are reasonably good and thus reduce the impact on the simulated nucleation, we firstly compare the temperature (at 10 m above the displacement height) and RH (at the first layer, about 70 m above the surface) of GEOS-FP input to GEOS-Chem–APM with measurements taken near surface (Fig. 4). It is shown that the temporal variations of the temperature and humidity input to the model are quite consistent with those of the observations, though the magnitude of the temperature input to the model is slightly lower than the observed value.
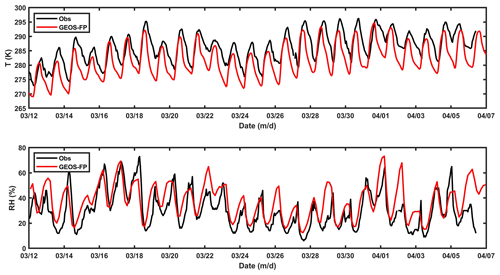
Figure 4Time series of temperature (at 10 m above the displacement height) and RH (at the first layer, about 70 m above the surface) input to the model and time series of measurements at a height of about 10 m during the 26 d campaign.
Figures 5 and 6 present the total and sub-3 nm particle number concentrations from the GEOS-Chem–APM simulations of four nucleation schemes, as well as the observed particle number concentrations, respectively. We found that the simulations with BHN and BIMN schemes significantly underestimate the observed total particle number concentrations and thus do not show significant number concentration fluctuation to distinguish NPF event and non-event days. The simulation with the THN scheme shows the total number concentration fluctuation on most NPF event days but fails to capture the noticeable increase in particle number concentrations on 18 March and 1 April. The simulation with the TIMN scheme reproduces the increase in particle number concentration on all NPF event days quite well, including continuous NPF events on 23 to 27 March and every discontinuous single NPF event day, but underestimates the observed daily maximum particle number concentration on 26 and 27 March and 1 April.
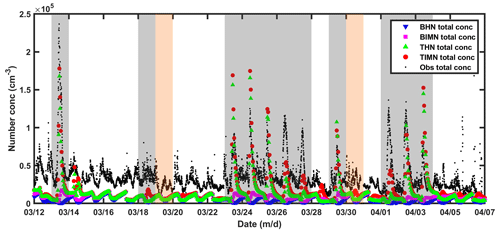
Figure 5Comparison of observed total particle number concentrations with those simulated on the basis of BHN, BIMN, THN, and TIMN schemes during the measurement period. The identified NPF days and undefined days are shaded with a grey and orange background, respectively.
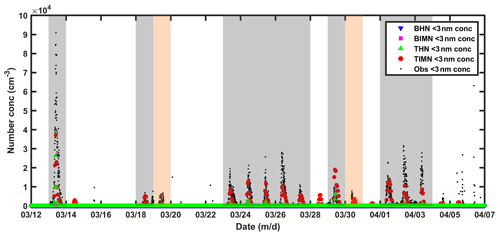
Figure 6Comparison of observed sub-3 nm particle number concentrations with those simulated on the basis of BHN, BIMN, THN, and TIMN schemes during the measurement period. The identified NPF days and undefined days are shaded with a grey and orange background, respectively.
The sub-3 nm particle number concentrations (Fig. 6) and nucleation rates (Fig. 7) from the simulations with BHN and BIMN are quite close to zero and not sensitive to daily variations. Such low nucleation rates lead to low particle number concentrations in the consequent growth process. The nucleation rates of different schemes in this study were calculated using lookup tables, which captured the absolute values of nucleation rates and their dependence on key controlling parameters well, as observed during the well-controlled Cosmics Leaving OUtdoor Droplets (CLOUD) experiments (Yu et al., 2020). Some uncertainties may exist in nucleation schemes as a result of uncertainties in the thermodynamics data used in the nucleation model. There are six parameters controlling nucleation rates for TIMN (JTIMN), including sulfuric acid vapor concentration ([H2SO4]), ammonia gas concentration ([NH3]), temperature (T), relative humidity (RH), ionization rate (Q), and surface area of preexisting particles (S). Therefore, on the ground, the ion nucleation rates could be limited by ion production rates and would not produce nucleation rates higher than Q. Depending on the definition, THN may be treated as a part of TIMN in the ternary nucleation system (Yu et al., 2020). Compared to JTIMN, there is one fewer controlling parameter for nucleation rates for THN (JTHN) (no Q dependence) and BIMN (JBIMN) (no [NH3] dependence), while the nucleation rate for BHN (JBHN) only depends on four parameters ([H2SO4], T, RH, and S). The uncertainties in the values of these parameters simulated by the model, as a result of uncertainties in the emissions, chemistry, and meteorology, will affect the simulation results. In addition, the real nucleation mechanisms in the atmosphere are complex and may involve additional parameters. Besides, the comparison between simulation results based on large grids and measurements also created uncertainties. Yu et al. (2020) found that nucleation rates with TIMN agreed with the CLOUD measurements within the uncertainties under nearly all conditions. Besides, the comparisons of horizontal spatial distributions of annual mean nucleation rates in the lower boundary layer (0–0.4 km) simulated with six different nucleation schemes indicated that the annual mean nucleation rate based on the BHN scheme significantly underestimated particle number concentrations (Yu et al., 2010). In contrast, the nucleation rates based on the TIMN scheme were high not only on NPF days but also on some non-event days. Not all these days with high nucleation rates have high particle number concentrations as well. Even so, the eventual simulated particle number concentrations show good agreement with observations. Nevertheless, the simulated nucleation rates in Fig. 7 were lower than the measured particle nucleation rates of 1.5 nm particles (Max J1.5) reported in Cai et al. (2017b). Besides, nucleation rates measured in urban areas can be higher than 10 (Yao et al., 2018; Yan et al., 2021). The relatively coarse spatial resolution in a global model implies that the model produces a regional mean nucleation rate compared to observation. Thus, it is difficult to perfectly reproduce the nucleation rate characteristics over urban areas. Nevertheless, we acknowledge that it is possible other nucleation mechanisms such as H2SO4–amine and H2SO4–organics nucleation may also simultaneously contribute to nucleation in polluted urban areas, which needs further study in the future.
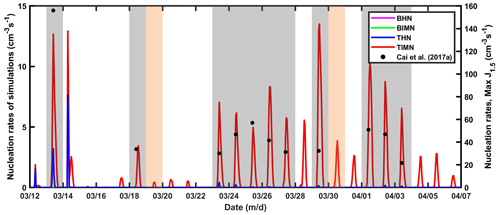
Figure 7Time series of nucleation rates simulated on the basis of BHN, BIMN, THN, and TIMN schemes during the measurement period and the measured particle nucleation rates of 1.5 nm particles (Max J1.5) reported in Cai et al. (2017b). The identified NPF days and undefined days are shaded with a grey and orange background, respectively.
Recent measurements in urban Shanghai found that DMA is perhaps the dominating base to stabilize H2SO4 clusters (Yao et al., 2018). It is good to address the roles of amines in the simulation. However, there is probably a long way to go before using the model to address the role of amines in nucleation for the following reasons. Firstly, the parameterization of the sulfuric acid–amine nucleation scheme is not yet mature enough, and a lot of validations against observations need to be done. Secondly, there is quite limited information on amine sources, and thus all current emission inventories, to our knowledge, do not contain the inventories for amines. Therefore, it is not possible currently to carry out such simulations.
Table 1 shows the correlation coefficients between the simulated particle number concentrations and the observed ones during the entire study period and NPF event days. The simulation with BHN and the observation is negatively correlated, indicating that there are obvious differences between the simulated results of BHN and the observed total particle number concentrations. According to the correlation coefficients, the BIMN scheme also does not perform well. In contrast, the particle number concentrations with THN and TIMN are positively correlated with the observations, and the correlation coefficients between observations and simulations with the TIMN scheme are higher than those with THN, indicating that TIMN has a better performance in simulating NPF events. The correlation coefficients between sub-3 nm particle number concentrations and simulation results based on the TIMN scheme (about 0.79) during two periods are significantly higher than those between the total particle number concentrations and simulations (about 0.65).
Table 1Correlation coefficient of observation total and sub-3 nm particle number concentrations with the BHN, BIMN, THN, and TIMN scheme, respectively.
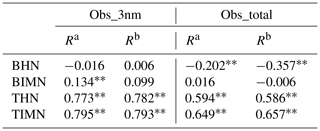
a During the measurement period. b During NPF days. The correlation coefficient passes the statistical significant test (p<0.01).
Figure 8 shows the temporal evolution of simulated particle number size distribution based on the TIMN scheme during 26 d in Beijing. According to the same criterion, all NPF days can be identified, which is consistent with observations. On most NPF days, the simulated number concentrations and particle number size distributions are close to observations. However, the simulated particle number size distributions on non-event days are obviously different from Fig. 1 because the background number concentration is difficult to simulate. Thus, the simulated number concentrations on most non-event days in Fig. 5 are indeed significantly lower than observations. One NPF event on 28 March predicted by the model is not observed with measurements, and the simulated particle number concentrations on some NPF days (e.g., 27 March and 1 April) are obviously underestimated. This may suggest that the model would not be able to capture effects at a given site during certain periods when the measurements are affected by sub-grid-scale processes (emissions, plumes, etc.). It may be helpful to compare high-resolution simulations with observations in order to address the issue.
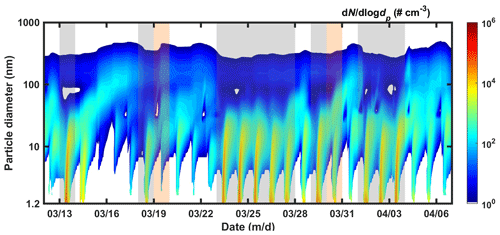
Figure 8The simulated particle size distributions based on the TIMN scheme. The identified NPF days and undefined days are shaded with a grey and orange background, respectively.
The time series of the simulated PM2.5 and PM10 mass concentrations are compared against the measurements during the study period (Fig. 9). It is shown that APM generally reproduces the temporal variations of PM2.5 and PM10 mass concentrations but tends to underestimate high values of PM2.5 and PM10 mass concentrations, possibly due to relatively low spatial resolution and the emission inventory uncertainty. Nevertheless, the correlation coefficients between simulations and observation PM2.5 and PM10 mass concentrations can reach 0.619 and 0.496, respectively, indicating that the simulation performance of APM is effective. In the atmosphere, the surface area of preexisting particles not only serves as a coagulation sink but also as a condensation sink for precursor gases. The APM model takes into account the effect of surface area of preexisting particles (Yu et al., 2020). In the model, the precursor gases that are not involved in the growth of nucleated particles (for cases with very small nucleation rates) condense on preexisting particles instead, so PM2.5 and PM10 mass concentrations are close for all four schemes.
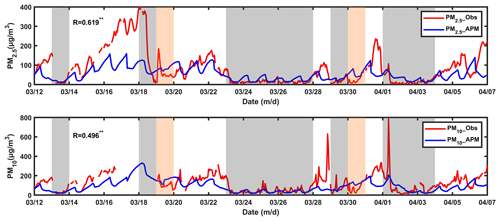
Figure 9Comparison of observation PM2.5 and PM10 mass concentrations with those in the simulation based on the APM model during the measurement period. The identified NPF days and undefined days are shaded with a grey and orange background, respectively. The correlation coefficient passes the statistical significant test (p<0.01).
In summary, in order to evaluate the ability of GEOS-Chem–APM and improve our understanding of nucleation mechanisms, four nucleation schemes are chosen for the model. The BHN scheme and BIMN scheme underestimate the observed particle number concentrations and fail to show significant number concentration fluctuation to distinguish NPF event and non-event days. The THN scheme simulates the particle number concentrations well on most NPF days except 18 March and 1 April. The TIMN scheme can simulate the total and sub-3 nm particle number concentrations and nucleation rates in Beijing well overall, which provides a basis for discussing the nucleation mechanism in Beijing. The real nucleation mechanisms in the atmosphere are complex and may involve additional parameters. Besides, further understanding of the nucleation mechanism in Beijing requires more meteorological and precursor gas concentration data in other seasons and model evaluation based on other schemes. The effect of meteorological conditions and precursors on nucleation can be further explored in sensitivity tests focusing on nucleation.
During 12 March to 6 April 2016 in Beijing, there are 11 typical NPF event days, 13 non-event days, and 2 undefined days. The sulfuric acid concentration in Beijing is sufficient to lead to NPF. The meteorological factors have systematical influences on NPF. Our study confirms that low RH is indeed favorable to the occurrence of an NPF event, as found by previous studies. Low RH and PM2.5 mass concentrations reduce the CS of condensable gases and the coagulation scavenging of new clusters. In contrast, high PM2.5 concentrations and RH will increase the sinks of vapor responsible for nucleation and growth of clusters.
To understand the applicability of different nucleation schemes in polluted areas such as Beijing and improve our understanding of nucleation mechanisms, the simulations based on the GEOS-Chem–APM model are conducted during this observation period. It is found that the simulations with BHN and BIMN schemes systematically underestimate both number concentrations and nucleation rates. The TIMN scheme overall has a better performance than the THN scheme in terms of the simulations of the total and sub-3 nm particle number concentrations and nucleation rates. APM also reproduces the temporal variations of particle matter concentrations, indicating that the simulation performance of APM is effective. H2SO4–amine and H2SO4–organics nucleation may also simultaneously contribute to nucleation in polluted urban areas. Some problems should to be solved before using the model to address the role of amines in nucleation, which needs further study in the future.
It is acknowledged that more observations on NPF are required in order to further understand nucleation mechanisms in Beijing, especially long-term observational data, which is very important to examine the favorable conditions for nucleation events. More detailed and comprehensive comparisons between model predictions and relevant data obtained in various field measurements will help to further improve the understanding of nucleation mechanisms, explain observed nucleation events, and accurately predict air quality.
Details of the data used in this work and how to access them are given in Sect. 2.1 and the acknowledgements.
KW and XM developed the project idea. KW, XM, and RT designed and conducted the model experiments. KW and XM analyzed the result and wrote the paper. XM and FY proposed scientific suggestions and revised the paper. All coauthors read and commented on the manuscript.
The contact author has declared that none of the authors has any competing interests.
Publisher's note: Copernicus Publications remains neutral with regard to jurisdictional claims in published maps and institutional affiliations.
We are thankful to Jiang Jingkun from Tsinghua University for kindly providing new particle formation measurements. We are also grateful to the GEOS-Chem Support Team for their management and maintenance of the GEOS-Chem model.
This research has been supported by the National Key Research and Development Program of China (grant no. 2019YFA0606802), the National Natural Science Foundation of China (grant no. 42061134009), and the National Natural Science Foundation of China (grant no. 41975002).
This paper was edited by Veli-Matti Kerminen and reviewed by two anonymous referees.
Aalto, P., Hämeri, K., Becker, E., Weber, R., Salm, J., Mäkelä, J., Hoell, C., O'dowd, C., Hansson, H., Väkevä, M., Koponen, I., Buzorius, G., and Kulmala, M.: Physical characterization of aerosol particles during nucleation events, Tellus B, 53, 344–358, https://doi.org/10.3402/tellusb.v53i4.17127, 2001.
An, J., Wang, H., Shen, L., Zhu, B., Zou, J., Gao, J., and Kang, H.: Characteristics of new particle formation events in Nanjing, China: Effect of water-soluble ions, Atmos. Environ., 108, 32–40, https://doi.org/10.1016/j.atmosenv.2015.01.038, 2015.
Cai, R., Chen, D.-R., Hao, J., and Jiang, J.: A Miniature Cylindrical Differential Mobility Analyzer for sub-3 nm Particle Sizing, J. Aerosol Sci., 106, 111–119, https://doi.org/10.1016/j.jaerosci.2017.01.004, 2017a.
Cai, R., Yang, D., Fu, Y., Wang, X., Li, X., Ma, Y., Hao, J., Zheng, J., and Jiang, J.: Aerosol surface area concentration: a governing factor in new particle formation in Beijing, Atmos. Chem. Phys., 17, 12327–12340, https://doi.org/10.5194/acp-17-12327-2017, 2017b.
Cai, R., Yan, C., Yang, D., Yin, R., Lu, Y., Deng, C., Fu, Y., Ruan, J., Li, X., Kontkanen, J., Zhang, Q., Kangasluoma, J., Ma, Y., Hao, J., Worsnop, D. R., Bianchi, F., Paasonen, P., Kerminen, V.-M., Liu, Y., Wang, L., Zheng, J., Kulmala, M., and Jiang, J.: Sulfuric acid–amine nucleation in urban Beijing, Atmos. Chem. Phys., 21, 2457–2468, https://doi.org/10.5194/acp-21-2457-2021, 2021.
Cai, R., Yin, R., Yan, C., Yang, D., Deng, C., Dada, L., Kangasluoma, J., Kontkanen, J., Halonen, R., Ma, Y., Zhang, X., Paasonen, P., Petäjä, T., Kerminen, V.-M., Liu, Y., Bianchi, F., Zheng, J., Wang, L., Hao, J., Smith, J. N., Donahue, N. M., Kulmala, M., Worsnop, D. R., and Jiang, J.: The missing base molecules in atmospheric acid–base nucleation, Natl. Sci. Rev., 9, nwac137, https://doi.org/10.1093/nsr/nwac137, 2022.
Chen, X., Yang, W., Wang, Z., Li, J., Hu, M., An, J., Wu, Q., Wang, Z., Chen, H., Wei, Y., Du, H., and Wang, D.: Improving new particle formation simulation by coupling a volatility-basis set (VBS) organic aerosol module in NAQPMS+APM, Atmos. Environ., 204, 1–11, https://doi.org/10.1016/j.atmosenv.2019.01.053, 2019.
Chu, B., Kerminen, V.-M., Bianchi, F., Yan, C., Petäjä, T., and Kulmala, M.: Atmospheric new particle formation in China, Atmos. Chem. Phys., 19, 115–138, https://doi.org/10.5194/acp-19-115-2019, 2019.
Dai, L., Wang, H., Zhou, L., An, J., Tang, L., Lu, C., Yan, W., Liu, R., Kong, S., Chen, M., Lee, S., and Yu, H.: Regional and local new particle formation events observed in the Yangtze River Delta region, China, J. Geophys. Res., 122, 2389–2402, https://doi.org/10.1002/2016JD026030, 2017.
Dal Maso, M., Kulmala, M., Riipinen, I., and Wagner, R.: Formation and growth of fresh atmospheric aerosols: Eight years of aerosol size distribution data from SMEAR II, Hyytiälä, Finland, Boreal Environ. Res., 10, 323–336, 2005.
Deng, C., Fu, Y., Dada, L., Yan, C., Cai, R., Yang, D., Zhou, Y., Yin, R., Lu, Y., Li, X., Qiao, X., Fan, X., Nie, W., Kontkanen, J., Kangasluoma, J., Chu, B., Ding, A., Kerminen, V., Paasonen, P., Worsnop, R. D., Bianchi, F., Liu, Y., Zheng, J., Wang, L., Kulmala, M., and Jiang, J.: Seasonal characteristics of new particle formation and growth in urban Beijing, Environ. Sci. Technol., 54, 8547–8557, https://doi.org/10.1021/acs.est.0c00808, 2020.
Foreback, B., Dada, L., Daellenbach, K. R., Yan, C., Wang, L., Chu, B., Zhou, Y., Kokkonen, T. V., Kurppa, M., Pileci, R. E., Wang, Y., Chan, T., Kangasluoma, J., Zhuohui, L., Guo, Y., Li, C., Baalbaki, R., Kujansuu, J., Fan, X., Feng, Z., Rantala, P., Gani, S., Bianchi, F., Kerminen, V.-M., Petäjä, T., Kulmala, M., Liu, Y., and Paasonen, P.: Measurement report: A multi-year study on the impacts of Chinese New Year celebrations on air quality in Beijing, China, Atmos. Chem. Phys., 22, 11089–11104, https://doi.org/10.5194/acp-22-11089-2022, 2022.
Gong, Y., Hu, M., Cheng, Y., Su, H., Yue, D., Liu, F., Wiedensohler, A., Wang, Z., Kalesse, H., and Liu, S.: Competition of coagulation sink and source rate: New particle formation in the Pearl River Delta of China, Atmos. Environ., 44, 3278–3285, https://doi.org/10.1016/j.atmosenv.2010.05.049, 2010.
Guo, S., Hu, M., Zamora, M., Peng, J., Shang, D., Zheng, J., Du, Z., Wu, Z., Shao, M., Zeng, L., Molina, M., and Zhang, R.: Elucidating severe urban haze formation in China, P. Natl. Acad. Sci. USA, 111, 17373–17378, https://doi.org/10.1073/pnas.1419604111, 2014.
Hamed, A., Korhonen, H., Sihto, S., Joutsensaari, J., Järvinen, H., Petäjä, T., Arnold, F., Nieminen, T., Kulmala, M., Smith, J., Lehtinen, K., and Laaksonen, A.: The role of relative humidity in continental new particle formation, J. Geophys. Res., 116, D03202, https://doi.org/10.1029/2010jd014186, 2011.
Herrmann, E., Ding, A. J., Kerminen, V.-M., Petäjä, T., Yang, X. Q., Sun, J. N., Qi, X. M., Manninen, H., Hakala, J., Nieminen, T., Aalto, P. P., Kulmala, M., and Fu, C. B.: Aerosols and nucleation in eastern China: first insights from the new SORPES-NJU station, Atmos. Chem. Phys., 14, 2169–2183, https://doi.org/10.5194/acp-14-2169-2014, 2014.
Hu, Y., Zang, Z., Chen, D., Ma, X., Liang, Y., You, W., Pan, X., Wang, L., Wang, D., and Zhang, Z.: Optimization and Evaluation of SO2 Emissions Based on WRF-Chem and 3DVAR Data Assimilation, Remote Sens.-Basel, 14, 220, https://doi.org/10.3390/rs14010220, 2022.
Jayaratne, R., Pushpawela, B., He, C., Li, H., Gao, J., Chai, F., and Morawska, L.: Observations of particles at their formation sizes in Beijing, China, Atmos. Chem. Phys., 17, 8825–8835, https://doi.org/10.5194/acp-17-8825-2017, 2017.
Kaiser, J.: How dirty air hurts the heart, Science, 307, 1858–1859, https://doi.org/10.1126/science.307.5717.1858b, 2005.
Kirkby, J., Duplissy, J., Sengupta, K., Frege, C., Gordon, H., Williamson, C., Heinritzi, M., Simon, M., Yan, C., Almeida, J., Troestl, J., Nieminen, T., Ortega, I. K., Wagner, R., Adamov, A., Amorim, A., Bernhammer, A.-K., Bianchi, F., Breitenlechner, M., Brilke, S., Chen, X., Craven, J., Dias, A., Ehrhart, S., Flagan, R. C., Franchin, A., Fuchs, C., Guida, R., Hakala, J., Hoyle, C. R., Jokinen, T., Junninen, H., Kangasluoma, J., Kim, J., Krapf, M., Kuerten, A., Laaksonen, A., Lehtipalo, K., Makhmutov, V., Mathot, S., Molteni, U., Onnela, A., Peraekylae, O., Piel, F., Petaejae, T., Praplan, A. P., Pringle, K., Rap, A., Richards, N. A. D., Riipinen, I., Rissanen, M. P ., Rondo, L., Sarnela, N., Schobesberger, S., Scott, C. E., Seinfeld, J. H., Sipilae, M., Steiner, G., Stozhkov, Y., Stratmann, F., Tome, A., Virtanen, A., Vogel, A. L., Wagner, A. C., Wagner, P. E., Weingartner, E., Wimmer, D., Winkler, P. M., Ye, P., Zhang, X., Hansel, A., Dommen, J., Donahue, N. M., Worsnop, D. R., Baltensperger, U., Kulmala, M., Carslaw, K. S., and Curtius, J.: Ion-induced nucleation of pure biogenic particles, Nature, 533, 521–526, https://doi.org/10.1038/nature17953, 2016.
Korhonen, P., Kulmala, M., Laaksonen, A., Viisanen, Y., McGraw, R., and Seinfeld, J.: Ternary nucleation of H2SO4, NH3, and H2O in the atmosphere, J. Geophys. Res., 104, 26349–26353, https://doi.org/10.1029/1999jd900784, 1999.
Kulmala, M., Laaksonen, A., and Pirjola, L.: Parameterizations for sulfuric acid/water nucleation rates, J. Geophys. Res., 103, 8301–8307, https://doi.org/10.1029/97jd03718, 1998.
Kulmala, M., Pirjola, L., and Mäkelä, J.: Stable sulphate clusters as a source of new atmospheric particles, Nature, 404, 66–69, https://doi.org/10.1038/35003550, 2000.
Kulmala, M., Kontkanen, J., Junninen, H., Lehtipalo, K., Manninen, H. E., Nieminen, T., Petaja, T., Sipila, M., Schobesberger, S., Rantala, P., Franchin, A., Jokinen, T., Jarvinen, E., Aijala, M., Kangasluoma, J., Hakala, J., Aalto, P. P., Paasonen, P., Mikkila, J., Vanhanen, J., Aalto, J., Hakola, H., Makkonen, U., Ruuskanen, T., Mauldin III, R. L., Duplissy, J., Vehkamaki, H., Back, J., Kortelainen, A., Riipinen, I., Kurten, T., Johnston, M. V., Smith, J. N., Ehn, M., Mentel, T. F., Lehtinen, K. E. J., Laaksonen, A., Kerminen, V.-M., and Worsnop, D. R.: Direct observations of atmospheric aerosol nucleation, Science, 339, 943–946, https://doi.org/10.1126/science.1227385, 2013.
Kulmala, M., Petaja, T., Kerminen, V. M., Kujansuu, J., Ruuskanen, T., Ding, A. J., Nie, W., Hu, M., Wang, Z. B., Wu, Z. J., Wang, L., and Worsnop, D. R.: On secondary new particle formation in China, Front. Env. Sci. Eng., 10, 8, https://doi.org/10.1007/s11783-016-0850-1, 2016.
Kulmala, M., Cai, R., Stolzenburg, D., Zhou, Y., Dada, L., Guo, Y., Yan, C., Petäjä, T., Jiang, J., and Kerminen, V.-M.: The contribution of new particle formation and subsequent growth to haze formation, Environ. Sci.-Atmos., 2, 352–361, https://doi.org/10.1039/D1EA00096A, 2022.
Liu, J., Jiang, J., Zhang, Q., Deng, J., and Hao, J.: A spectrometer for measuring particle size distributions in the range of 3 nm to 10 µm, Front. Env. Sci. Eng., 10, 63–72, https://doi.org/10.1007/s11783-014-0754-x, 2016.
Luo, G. and Yu, F.: Simulation of particle formation and number concentration over the Eastern United States with the WRF-Chem + APM model, Atmos. Chem. Phys., 11, 11521–11533, https://doi.org/10.5194/acp-11-11521-2011, 2011.
Merikanto, J., Spracklen, D. V., Mann, G. W., Pickering, S. J., and Carslaw, K. S.: Impact of nucleation on global CCN, Atmos. Chem. Phys., 9, 8601–8616, https://doi.org/10.5194/acp-9-8601-2009, 2009.
Metzger, A., Verheggen, B., Dommen, J., Duplissy, J., Prevot, A. S., Weingartner, E., Riipinen, I., Kulmala, M., Spracklen, D. V., Carslaw, K. S., and Baltensperger, U.: Evidence for the role of organics in aerosol particle formation under atmospheric conditions, P. Natl. Acad. Sci. USA, 107, 6646–6651, https://doi.org/10.1073/pnas.0911330107, 2010.
Nieminen, T., Manninen, H. E., Sihto, S. L., Yli-Juuti, T., Mauldin, I. R. L., Petaja, T., Riipinen, I., Kerminen, V. M., and Kulmala, M.: Connection of sulfuric acid to atmospheric nucleation in boreal forest, Environ. Sci. Technol., 43, 4715–4721, https://doi.org/10.1021/es803152j, 2009.
Park, R. J., Jacob, D. J., Field, B. D., Yantosca, R. M., and Chin, M.: Natural and transboundary pollution influences on sulfate-nitrate-ammonium aerosols in the United States: Implications for policy, J. Geophys. Res., 109, D15204, https://doi.org/10.1029/2003jd004473, 2004.
Qi, X. M., Ding, A. J., Nie, W., Petäjä, T., Kerminen, V.-M., Herrmann, E., Xie, Y. N., Zheng, L. F., Manninen, H., Aalto, P., Sun, J. N., Xu, Z. N., Chi, X. G., Huang, X., Boy, M., Virkkula, A., Yang, X.-Q., Fu, C. B., and Kulmala, M.: Aerosol size distribution and new particle formation in the western Yangtze River Delta of China: 2 years of measurements at the SORPES station, Atmos. Chem. Phys., 15, 12445–12464, https://doi.org/10.5194/acp-15-12445-2015, 2015.
Qiao, X., Yan, C., Li, X., Guo, Y., Yin, R., Deng, C., Li, C., Nie, W., Wang, M., Cai, R., Huang, D., Wang, Z., Yao, L., Worsnop, D., Bianchi, F., Liu, Y., Donahue, N., Kulmala, M., and Jiang, J.: Contribution of Atmospheric Oxygenated Organic Compounds to Particle Growth in an Urban Environment, Environ. Sci. Technol., 55, 13646–13656, https://doi.org/10.1021/acs.est.1c02095, 2021.
Shen, X., Sun, J., Yu, F., Wang, Y., Zhong, J., Zhang, Y., Hu, X., Xia, C., Zhang, S., and Zhang, X.: Enhancement of nanoparticle formation and growth during the COVID-19 lockdown period in urban Beijing, Atmos. Chem. Phys., 21, 7039–7052, https://doi.org/10.5194/acp-21-7039-2021, 2021.
Sipilä, M., Berndt, T., Petäjä, T., Brus, D., Vanhanen, J., Stratmann, F., Patokoski, J., Mauldin, R., Hyvärinen, A., Lihavainen, H., and Kulmala, M.: The role of sulfuric acid in atmospheric nucleation, Science, 327, 1243–1246, https://doi.org/10.1126/science.1180315, 2010.
Sipilä, M., Sarnela, N., Jokinen, T., Henschel, H., Junninen, H., Kontkanen, J., Richters, S., Kangasluoma, J., Franchin, A., Peräkylä, O., Rissanen, M. P., Ehn, M., Vehkamäki, H., Kurten, T., Berndt, T., Petäjä, T., Worsnop, D., Ceburnis, D., Kerminen, V. M., Kulmala, M., and O'Dowd, C.: Molecular-scale evidence of aerosol particle formation via sequential addition of HIO3, Nature, 537, 532–534, https://doi.org/10.1038/nature19314, 2016.
Stockwell, W. and Calvert, J.: The mechanism of the HO-SO2 reaction, Atmos. Environ., 17, 2231–2235, https://doi.org/10.1016/0004-6981(83)90220-2, 1983.
Sun, Y., Zhu, Y., Meng, H., Liu, B., Liu, Y., Dong, C., Yao, X., Wang, W., and Xue, L.: New particle formation events in summer and winter in the coastal atmosphere in Qingdao, China, Environm. Sci., 42, 2133–2142, https://doi.org/10.13227/j.hjkx.202007230, 2021.
Tang, L., Shang, D., Fang, X., Wu, Z., Qiu, Y., Chen, S., Li, X., Zeng, L., Guo, S., and Hu, M.: More significant impacts from new particle formation on haze formation during COVID-19 lockdown, Geophys. Res. Lett., 48, e2020GL091591, https://doi.org/10.1029/2020gl091591, 2021.
Tröstl, J., Chuang, W. K., Gordon, H., Heinritzi, M., Yan, C., Molteni, U., Ahlm, L., Frege, C., Bianchi, F., Wagner, R., Simon, M., Lehtipalo, K., Williamson, C., Craven, J. S., Duplissy, J., Adamov, A., Almeida, J., Bernhammer, A.-K., Breitenlechner, M., Brilke, S., Dias, A., Ehrhart, S., Flagan, R. C., Franchin, A., Fuchs, C., Guida, R., Gysel, M., Hansel, A., Hoyle, C. R., Jokinen, T., Junninen, H., Kangasluoma, J., Keskinen, H., Kim, J., Krapf, M., Kuerten, A., Laaksonen, A., Lawler, M., Leiminger, M., Mathot, S., Moehler, O., Nieminen, T., Onnela, A., Petaejae, T., Piel, F. M., Miettinen, P., Rissanen, M. P., Rondo, L., Sarnela, N., Schobesberger, S., Sengupta, K., Sipila, M., Smith, J. N., Steiner, G., Tome, A., Virtanen, A., Wagner, A. C., Weingartner, E., Wimmer, D., Winkler, P. M., Ye, P., Carslaw, K. S., Curtius, J., Dommen, J., Kirkby, J., Kulmala, M., Riipinen, I., Worsnop, D. R., Donahue, N. M., and Baltensperger, U.: The role of low-volatility organic compounds in initial particle growth in the atmosphere, Nature, 533, 527–531, https://doi.org/10.1038/nature18271, 2016.
Wang, Z., Hu, M., Pei, X., Zhang, R., Paasonen, P., Zheng, J., Yue, D., Wu, Z., Boy, M., and Wiedensohler, A.: Connection of organics to atmospheric new particle formation and growth at an urban site of Beijing, Atmos. Environ., 103, 7–17, https://doi.org/10.1016/j.atmosenv.2014.11.069, 2015.
Weber, R. J., McMurry, P. H., Mauldin, R. L., Tanner, D. J., Eisele, F. L., Clarke, A. D., and Kapustin, V. N.: New particle formation in the remote troposphere: a comparison of observations at various sites, Geophys. Res. Lett., 26, 307–310, https://doi.org/10.1029/1998gl900308, 1999.
Wu, H., Li, Z., Li, H., Luo, K., Wang, Y., Yan, P., Hu, F., Zhang, F., Sun, Y., Shang, D., Liang, C., Zhang, D., Wei, J., Wu, T., Jin, X., Fan, X., Cribb, M., Fischer, M., Kulmala, M., and Petäjä, T.: The impact of the atmospheric turbulence-development tendency on new particle formation: a common finding on three continents, Natl. Sci. Rev., 8, 140–150, https://doi.org/10.1093/nsr/nwaa157, 2020.
Wu, Z., Hu, M., Liu, S., Wehner, B., Bauer, S., Ma ßling, A., Wiedensohler, A., Petäjä, T., Dal Maso, M., and Kulmala, M.: New particle formation in Beijing, China: Statistical analysis of a 1 year data set, J. Geophys. Res., 112, D09209, https://doi.org/10.1029/2006jd007406, 2007.
Wu, Z., Hu, M., Lin, P., Liu, S., Wehner, B., and Wiedensohler, A.: Particle number size distribution in the urban atmosphere of Beijing, China, Atmos. Environ., 42, 7967–7980, https://doi.org/10.1016/j.atmosenv.2008.06.022, 2008.
Xiao, M., Hoyle, C. R., Dada, L., Stolzenburg, D., Kürten, A., Wang, M., Lamkaddam, H., Garmash, O., Mentler, B., Molteni, U., Baccarini, A., Simon, M., He, X.-C., Lehtipalo, K., Ahonen, L. R., Baalbaki, R., Bauer, P. S., Beck, L., Bell, D., Bianchi, F., Brilke, S., Chen, D., Chiu, R., Dias, A., Duplissy, J., Finkenzeller, H., Gordon, H., Hofbauer, V., Kim, C., Koenig, T. K., Lampilahti, J., Lee, C. P., Li, Z., Mai, H., Makhmutov, V., Manninen, H. E., Marten, R., Mathot, S., Mauldin, R. L., Nie, W., Onnela, A., Partoll, E., Petäjä, T., Pfeifer, J., Pospisilova, V., Quéléver, L. L. J., Rissanen, M., Schobesberger, S., Schuchmann, S., Stozhkov, Y., Tauber, C., Tham, Y. J., Tomé, A., Vazquez-Pufleau, M., Wagner, A. C., Wagner, R., Wang, Y., Weitz, L., Wimmer, D., Wu, Y., Yan, C., Ye, P., Ye, Q., Zha, Q., Zhou, X., Amorim, A., Carslaw, K., Curtius, J., Hansel, A., Volkamer, R., Winkler, P. M., Flagan, R. C., Kulmala, M., Worsnop, D. R., Kirkby, J., Donahue, N. M., Baltensperger, U., El Haddad, I., and Dommen, J.: The driving factors of new particle formation and growth in the polluted boundary layer, Atmos. Chem. Phys., 21, 14275–14291, https://doi.org/10.5194/acp-21-14275-2021, 2021.
Xiao, S., Wang, M. Y., Yao, L., Kulmala, M., Zhou, B., Yang, X., Chen, J. M., Wang, D. F., Fu, Q. Y., Worsnop, D. R., and Wang, L.: Strong atmospheric new particle formation in winter in urban Shanghai, China, Atmos. Chem. Phys., 15, 1769–1781, https://doi.org/10.5194/acp-15-1769-2015, 2015.
Yan, C., Yin, R., Lu, Y., Dada, L., Yang, D., Fu, Y., Kontkanen, J., Deng, C., Garmash, O., Ruan, J., Baalbaki, R., Schervish, M., Cai, R., Bloss, M., Chan, T., Chen, T., Chen, Q., Chen, X., Chen, Y., Chu, B., Dällenbach, K., Foreback, B., He, X., Heikkinen, L., Jokinen, T., Junninen, H., Kangasluoma, J., Kokkonen, T., Kurppa, M., Lehtipalo, K., Li, H., Li, H., Li, X., Liu, Y., Ma, Q., Paasonen, P., Rantala, P., Pileci, R. E., Rusanen, A., Sarnela, N., Simonen, P., Wang, S., Wang, W., Wang, Y., Xue, M., Yang, G., Yao, L., Zhou, Y., Kujansuu, J., Petäjä, T., Nie, W., Ma, Y., Ge, M., He, H., Donahue, N. M., Worsnop, D. R., Veli-Matti, K., Wang, L., Liu, Y., Zheng, J., Kulmala, M., Jiang, J., and Bianchi, F.: The Synergistic Role of Sulfuric Acid, Bases, and Oxidized Organics Governing New-Particle Formation in Beijing, Geophys. Res. Lett., 48, e2020GL091944, https://doi.org/10.1029/2020gl091944, 2021.
Yao, L., Garmash, O., Bianchi, F., Zheng, J., Yan, C., Kontkanen, J., Junninen, H., Mazon, S., Ehn, M., Paasonen, P., Sipilä, M., Wang, M., Wang, X., Xiao, S., Chen, H., Lu, Y., Zhang, B., Wang, D., Fu, Q., Geng, F., Li, L., Wang, H., Qiao, L., Yang, X., Chen, J., Kerminen, V., Petäjä, T., Worsnop, D., Kulmala, M., and Wang, L.: Atmospheric new particle formation from sulfuric acid and amines in a Chinese megacity, Science, 361, 278–281, https://doi.org/10.1126/science.aao4839, 2018.
Yu, F.: Effect of ammonia on new particle formation: A kinetic H2SO4–H2O–NH3 nucleation model constrained by laboratory measurements, J. Geophys. Res., 111, D01204, https://doi.org/10.1029/2005JD005968, 2006a.
Yu, F.: From molecular clusters to nanoparticles: second-generation ion-mediated nucleation model, Atmos. Chem. Phys., 6, 5193–5211, https://doi.org/10.5194/acp-6-5193-2006, 2006b.
Yu, F.: Improved quasi-unary nucleation model for binary H2SO4–H2O homogeneous nucleation, J. Chem. Phys., 127, 054301, https://doi.org/10.1063/1.2752171, 2007.
Yu, F.: Updated H2SO4–H2O binary homogeneous nucleation look-up tables, J. Geophys. Res., 113, D24201, https://doi.org/10.1029/2008jd010527, 2008.
Yu, F.: A secondary organic aerosol formation model considering successive oxidation aging and kinetic condensation of organic compounds: global scale implications, Atmos. Chem. Phys., 11, 1083–1099, https://doi.org/10.5194/acp-11-1083-2011, 2011.
Yu, F. and Luo, G.: Simulation of particle size distribution with a global aerosol model: contribution of nucleation to aerosol and CCN number concentrations, Atmos. Chem. Phys., 9, 7691–7710, https://doi.org/10.5194/acp-9-7691-2009, 2009.
Yu, F. and Luo, G.: Oceanic dimethyl sulfide emission and new particle formation around the coast of Antarctica: a modeling study of seasonal variations and comparison with measurements, Atmosphere, 1, 34–50, https://doi.org/10.3390/atmos1010034, 2010.
Yu, F. and Turco, R.: Ultrafine aerosol formation via ion-mediated nucleation, Geophys. Res. Lett., 27, 883–886, https://doi.org/10.1029/1999gl011151, 2000.
Yu, F. and Turco, R. P.: The size-dependent charge fraction of sub-3 nm particles as a key diagnostic of competitive nucleation mechanisms under atmospheric conditions, Atmos. Chem. Phys., 11, 9451–9463, https://doi.org/10.5194/acp-11-9451-2011, 2011.
Yu, F., Wang, Z., Luo, G., and Turco, R.: Ion-mediated nucleation as an important global source of tropospheric aerosols, Atmos. Chem. Phys., 8, 2537–2554, https://doi.org/10.5194/acp-8-2537-2008, 2008.
Yu, F., Luo, G., Bates, T., Anderson, B., Clarke, A., Kapustin, V., Yantosca, R., Wang, Y., and Wu, S.: Spatial distributions of particle number concentrations in the global troposphere: simulations, observations, and implications for nucleation mechanisms, J. Geophys. Res., 115, D17205, https://doi.org/10.1029/2009jd013473, 2010.
Yu, F., Nadykto, A. B., Herb, J., Luo, G., Nazarenko, K. M., and Uvarova, L. A.: H2SO4– ternary ion-mediated nucleation (TIMN): kinetic-based model and comparison with CLOUD measurements, Atmos. Chem. Phys., 18, 17451–17474, https://doi.org/10.5194/acp-18-17451-2018, 2018.
Yu, F., Nadykto, A. B., Luo, G., and Herb, J.: H2SO4–H2O binary and H2SO4–H2O–NH3 ternary homogeneous and ion-mediated nucleation: lookup tables version 1.0 for 3-D modeling application, Geosci. Model Dev., 13, 2663–2670, https://doi.org/10.5194/gmd-13-2663-2020, 2020.
Zhang, R., Khalizov, A., Wang, L., Hu, M., and Xu, W.: Nucleation and growth of nanoparticles in the atmosphere, Chem. Rev., 112, 1957–2011, https://doi.org/10.1021/cr2001756, 2012.
Zheng, J., Hu, M., Zhang, R., Yue, D., Wang, Z., Guo, S., Li, X., Bohn, B., Shao, M., He, L., Huang, X., Wiedensohler, A., and Zhu, T.: Measurements of gaseous H2SO4 by AP-ID-CIMS during CAREBeijing 2008 Campaign, Atmos. Chem. Phys., 11, 7755–7765, https://doi.org/10.5194/acp-11-7755-2011, 2011.
Zheng, J., Yang, D., Ma, Y., Chen, M., Cheng, J., Li, S., and Wang, M.: Development of a new corona discharge based ion source for high resolution time-of-flight chemical ionization mass spectrometer to measure gaseous H2SO4 and aerosol sulfate, Atmos. Environ., 119, 167–173, https://doi.org/10.1016/j.atmosenv.2015.08.028, 2015.
Zhou, Y., Dada, L., Liu, Y., Fu, Y., Kangasluoma, J., Chan, T., Yan, C., Chu, B., Daellenbach, K. R., Bianchi, F., Kokkonen, T. V., Liu, Y., Kujansuu, J., Kerminen, V.-M., Petäjä, T., Wang, L., Jiang, J., and Kulmala, M.: Variation of size-segregated particle number concentrations in wintertime Beijing, Atmos. Chem. Phys., 20, 1201–1216, https://doi.org/10.5194/acp-20-1201-2020, 2020.