the Creative Commons Attribution 4.0 License.
the Creative Commons Attribution 4.0 License.
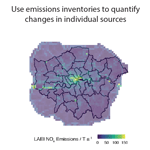
Pandemic restrictions in 2020 highlight the significance of non-road NOx sources in central London
Will Drysdale
James D. Lee
Carole Helfter
Eiko Nemitz
Stefan Metzger
Janet F. Barlow
Fluxes of nitrogen oxides () and carbon dioxide (CO2) were measured using eddy covariance at the British Telecommunications (BT) Tower in central London during the coronavirus pandemic. Comparing fluxes to those measured in 2017 prior to the pandemic restrictions and the introduction of the Ultra-Low Emissions Zone (ULEZ) highlighted a 73 % reduction in NOx emissions between the two periods but only a 20 % reduction in CO2 emissions and a 32 % reduction in traffic load. Use of a footprint model and the London Atmospheric Emissions Inventory (LAEI) identified transport and heat and power generation to be the two dominant sources of NOx and CO2 but with significantly different relative contributions for each species. Application of external constraints on NOx and CO2 emissions allowed the reductions in the different sources to be untangled, identifying that transport NOx emissions had reduced by >73 % since 2017. This was attributed in part to the success of air quality policy in central London but crucially due to the substantial reduction in congestion that resulted from pandemic-reduced mobility. Spatial mapping of the fluxes suggests that central London was dominated by point source heat and power generation emissions during the period of reduced mobility. This will have important implications on future air quality policy for NO2 which, until now, has been primarily focused on the emissions from diesel exhausts.
- Article
(5974 KB) - Full-text XML
- BibTeX
- EndNote
Air pollution is thought to be the world's largest environmental risk to human health, causing an estimated 7 million premature deaths every year (World Health Organisation, 2018). One species of pollutants of particular concern, especially in the UK, is NOx. Formed as a byproduct of high-temperature combustion, NOx is commonly emitted from the tailpipe exhaust of internal combustion engine vehicles and through the use of fossil fuels to generate heat and energy in the residential, commercial and industrial sectors. The major component of NOx is nitrogen dioxide (NO2), direct exposure to which is known to contribute to respiratory infections such as bronchitis and pneumonia (Ciencewicki and Jaspers, 2007). Indirectly, NOx is a key component to the photochemical formation of ozone and fine particulate matter (PM2.5). Exposure to ozone and PM2.5 has additional adverse effects on the respiratory and cardiovascular systems (Zhang et al., 2019). Consequentially, NO2 and PM2.5 were estimated to cost the UK's National Health Service (NHS) and social care GBP 1.6 billion between 2017 and 2025, rising to GBP 5.6 billion if diseases with less robust evidence for an association are included (Public Health England, 2018). This has become particularly relevant since the start of the coronavirus pandemic, whereby long-term exposure to air pollution has been associated with the severity of COVID-19 cases (Imperial College London, 2021).
In 2008, countries in the EU were set legally binding limits for NO2 concentrations in line with the World Health Organisation (WHO) recommendations. These are 40 µg m−3 for the annual mean, with no more than 18 exceedances of the 200 µg m−3 hourly limit every year (European Parliament, 2008). This target was expected to be met by 2010. In 2021, the WHO reduced the recommended annual mean limit by 75 % to 10 µg m−3 (World Health Organisation, 2021).
London is a megacity in the UK with extensive NO2 air quality issues. Almost all roadside locations exceeded the European limit value for NO2 every year between 2010 and 2016 (Font et al., 2019). Being in a highly developed position with significant resources, it has acted as a testing bed for policy intervention to try and curb emissions and to achieve these air quality targets. These have been focused largely on traffic pollution and congestion charging and have the primary goal of reducing NOx concentrations via reduced road transport emissions, either through reduced traffic numbers or through reduced average emission per vehicle per unit distance. Most notable is the introduction of the world's first Ultra-Low Emissions Zone (ULEZ), launched on 8 April 2019 with the zone spatially shown in Fig. 1a. This operates 24 h d−1, 364 d a year (excludes Christmas Day) and requires a daily payment if the vehicle driven inside the zone does not meet the most stringent emissions standards (currently Euro III for motorbikes, Euro IV for petrol cars and Euro VI for diesel cars and larger vehicles) in addition to the congestion-charging payment within the same area. The ULEZ was expanded on 25 October 2021 up to the north and south circular roads in an 18-fold increase in size. In addition to policy, the coronavirus pandemic had significant implications on NOx emissions in the UK through reduced mobility. During 2020 and 2021, the UK staged three lockdowns with “stay-at-home” orders. Full details on the timings and the severity of lockdown restrictions in London can be found in Fig. A1.
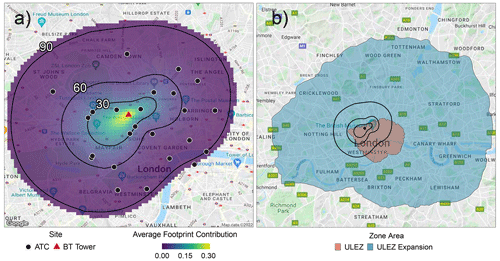
Figure 1(a) The average footprint climatology for the September 2020–September 2021 time period, with the 30 %, 60 % and 90 % contribution contours and the location of the 24 ATC sites and the BT Tower site overlaid. (b) Spatial boundary of the ULEZ (red) and ULEZ expansion (blue) with the same footprint contribution contours presented in (a) overlaid as black lines and the BT Tower site marked as a black dot. Maps produced from Google Maps (© Google Maps 2022), accessed using an API in R.
Assessment of the impact of policy intervention and other external stimuli like the coronavirus pandemic on NOx emissions is crucial for the future design and implementation of air quality policy in the UK. Eddy covariance is a technique used to quantify the surface–atmosphere exchange of an atmospheric pollutant. The calculated flux coupled with a footprint model provides information on surface emissions, allowing for changes to be studied and for direct comparison to the emissions inventories used in policy development. Whilst most frequently used for measuring carbon dioxide exchange with ecosystems from stationary towers (Baldocchi et al., 2001; Griffis et al., 2008; Butterbach-Bahl et al., 2013), the technique has been extended to the urban canopy for both greenhouse gases and air pollutants (Langford et al., 2010a; Lee et al., 2014; Helfter et al., 2016; Karl et al., 2017), as well as to airborne measurements for the assessment of fluxes at a much greater spatial extent (Vaughan et al., 2021; Metzger et al., 2013; Vaughan et al., 2017). Recently, Lamprecht et al. (2021) used long-term air pollutant emissions measurements to understand how COVID-19 restrictions impacted different sources of NOx in the small European city of Innsbruck, Austria. We undertake a similar analysis but for the megacity of London. This offers the perspective of a different location where not only are emissions much higher but where contributions from different sources can vary significantly due to the nature of the activity required to support both greater population size and density. With the number of megacities consistently increasing and being expected to reach 43 in 2030 (up from 31 in 2018), improving our understanding of the air pollutant sources in them is as critical as ever (United Nations, 2019).
Here, we present the first year of data from the long-term NOx flux measurement programme at the BT Tower (London, UK). As the only long-term measurements of NOx emissions from a megacity in the world, this is a highly unique and potentially informative data set. The NOx emissions measurements are combined with additional CO2 emissions measurements, a footprint model and the London Atmospheric Emissions Inventory (LAEI) and are compared to previous measurements in 2017 to source apportion changes in emissions due to the coronavirus pandemic and the ULEZ. Resulting policy implications are inferred.
2.1 Measurement site
Instruments for measuring fluxes of urban air pollutants and greenhouse gases are situated in a small laboratory atop the BT Tower located in central London, UK (51∘31′17.4′′ N, 0∘8′20.04′′ W). The measurement height is 190 m above street level, with a mean building height of 8.8±3.0 m in the 10 km radius surrounding the tower (Lee et al., 2014). The gas inlet and ultrasonic anemometer are attached to a solid mast that extends 3 m above the top of the tower. Air is pumped down a 45 m Teflon tube ( OD) in a turbulent flow of 20–25 L min−1 to the gas instruments, which are situated in a small air-conditioned room inside the tower on the 35th floor.
2.2 NOx measurements
Long-term measurements of NO and NO2 fluxes began in September 2020 with data presented here up to September 2021. Data are compared to previous measurements made by Drysdale et al. (2022) from March–August 2017. Both chemical species were measured using a dual-channel chemiluminescence analyser (Air Quality Design Inc., Boulder Colorado, USA; 5 Hz), as described previously by Squires et al. (2020); Drysdale et al. (2022). The number of photons measured by the photomultiplier tube was converted into a part-per-trillion (ppt) mixing ratio using a five-point calibration curve produced through dilutions of a 5 ppm NO in N2 calibration standard (BOC Ltd., UK; traceable to the scale of the UK National Physical Laboratory, NPL) into NOx-free air (generated from an external Sofnofil and activated charcoal trap). NO2 was calculated by conversion of NO2 into NO using a photolytic blue-light converter (BLC). Here, both NO and NO2 were measured, from which NO2 can be quantified by subtracting the NO mixing ratio and applying a correction factor for the conversion efficiency of the BLC. The instrument was calibrated every 37 h in addition to an hourly zero measurement to subtract the temperature-dependent background signal of each channel. The uncertainty of the NO measurement is given as ±3 %, resulting from uncertainties in the sample mass flow controller, calibration gas mass flow controller and calibration gas certification. The uncertainty for the NO2 measurement is given as ±4.7 % due to the additional uncertainty in the conversion efficiency calculation, determined in the laboratory via variation in repeated tests. The precision for each channel is calculated as 53 and 184 ppt for NO and NO2 respectively from the standard deviation in all the hourly zeros during the measurement period.
2.3 CO2 measurements
Long-term measurements of CO2 have been ongoing at the BT Tower since 2011 as part of UKCEH's National Capability programme. Dry mass fractions (1σ precision < 300 ppb) were measured initially using a cavity ringdown spectrometer (Model 1301-f, Picarro Inc., Santa Clara, California, USA; 10 Hz) as described by Helfter et al. (2016). Unfortunately, instrumental failure means data are not available between February and June 2021, after which a closed-path infrared gas analyser (Li-7000, LI-COR Environmental, Lincoln, Nebraska, USA; 10 Hz) took over the CO2 flux measurement.
2.4 Meteorological measurements
Meteorological measurements were made at the BT Tower as described by Lane et al. (2013). Wind speed, wind direction and sonic temperature were measured using an ultrasonic anemometer (Gill R3-50, Gill Instruments, Lymington, UK; 20 Hz) along with pressure and relative humidity measurements using a weather station (WXT520, Vaisala Corp. Helsinki, Finland; 1 Hz). For ease of processing, each chemical species is logged separately at its maximum measurement frequency into a file with the sonic-anemometer data averaged to the same measurement frequency.
2.5 Flux calculations
The flux, F, is defined in this context as the vertical transport of a chemical species per unit area per unit time. Hourly fluxes were calculated using eddy covariance theory as described by Eq. (1), where F is equal to the covariance between the instantaneous change in vertical wind speed, w′, and the instantaneous change in species concentration, c′, averaged over the hour.
Eddy covariance calculations were performed using the modular software packages in eddy4R, adopting the same processing settings described in Drysdale et al. (2022), as adapted from Squires et al. (2020). This was to allow a direct comparison to be made to the previous measurements made in 2017. The lag time correction was determined by maximisation of the cross-covariance between the pollutant concentration and the vertical wind component, with an additional application of a high-pass filter which improves the precision of the determined lag time by an order of magnitude (Hartmann et al., 2018; Squires et al., 2020). This resulted in median lag times of 7.2 s for NO, 7.6 s for NO2 and 21 s for CO2. Data were filtered such that the friction velocity (u*) is >0.2 to ensure sufficiently developed turbulence and using eddy4R's quality control flagging scheme. The QA/QC process is described in detail by Smith and Metzger (2013) and Metzger et al. (2022). Data are flagged as either valid or invalid based on the combination of individual flags for input data validation, homogeneity and stationarity, and development of turbulence.
2.6 Flux uncertainties
2.6.1 NOx chemistry
Eddy covariance has traditionally only been used for relatively unreactive greenhouse gases with long atmospheric lifetimes such as CO2. Attempting the calculation of NOx fluxes is potentially problematic due to the greater reactivity and hence shorter lifetime of the species. If the loss rates of the reactive species are of a similar timescale to the vertical transport to the measurement height, the measured flux would be an underestimate and would not be representative of those emitted at the ground. In the case of NOx, the major loss route to the atmosphere is via the reaction between NO2 and OH. The rate constant for this simple association reaction can be calculated for the BT-Tower-specific conditions from Eq. (2) using mean values of temperature (T, 289 K) and pressure (P, 989 hPa) (Jet Propulsion Laboratory, 2020). This is derived from the low-pressure limiting rate constant (k0(T)) and the high-pressure limiting rate constant (k∞(T)) using location-specific total gas concentrations ([M]). n and m are simple exponents for the given reaction, in this case 3 and 0 respectively.
where
Assuming a simple first-order loss rate, the level of NOx loss to the atmosphere can then be estimated from Eq. (6) using the previously determined rate constant (), the concentration of OH ([OH]) and the transport time to the measurement height (t).
Since [OH] is not routinely measured in London, a typical midday summer value of 2×106 is used from London measurements in 2012, which would represent the maximum loss rate observed throughout the year (Lee et al., 2016). Barlow et al. (2011) estimate a typical transport time of <10 min for the BT Tower, although under stable conditions this could increase to 20–50 min. This results in a loss of 2 %, increasing up to 11 % for a 50 min transport time. The 11 % loss represents the maximum loss observed at the BT Tower, since it occurs under the most stable conditions during peak OH concentrations for London in summer. In reality, this level of loss will not be observed in the data, since stable conditions are filtered out in the QA/QC process, and the majority of the OH concentration present throughout the year is less than that used in this calculation. Since it is much more likely to be at or below the 2 % threshold, we consider NOx reactivity to be a minor uncertainty in the flux calculations, and a correction is not applied.
2.6.2 Vertical flux divergence
Another source of uncertainty is the size of the measurement height relative to the boundary layer. At 191 m, the sample inlet and sonic anemometer are often an appreciable portion of the boundary layer and can extend above the constant flux layer. On occasion, this results in concentration enhancements below the measurement height and an underestimation of the surface flux through vertical flux divergence (VFD). The impact of storage and vertical flux divergence at the BT Tower has been discussed previously by Helfter et al. (2016) and Drysdale et al. (2022) and, in the absence of concentrations and wind measurements at different heights up the tower, remains a notable source of uncertainty in the measurement. Helfter et al. (2016) speculate that venting after the onset of turbulence would capture some, if not most, of the material stored below the measurement height. Drysdale et al. (2022) demonstrate a correction for vertical flux divergence as a function of effective measurement height and effective entrainment height. The correction was typically around 20 % for 2017 but is not applied to the data due to uncertainties in the boundary layer height data. Since this work studies relative magnitudes between two periods, the impact of vertical flux divergence will likely cancel out, provided the meteorology is similar. Boundary layer height was on average 8 % lower in 2020–2021 compared to the 2017 measurement period. As such, a comparison between the VFD correction presented in Drysdale et al. (2022) for both periods was conducted. The lower boundary layer height in 2020–2021 meant that the correction was slightly higher at 24 %. A discussion on the impact this had on the results of this paper is given in Sect. 3.
2.6.3 High- and low-frequency loss
Due to the height of the measurement and the large eddy size above the urban roughness layer, the high-frequency contributions to the fluxes are expected to be small. Drysdale et al. (2022) calculated high-frequency loss for NO and NO2 at the BT Tower via co-spectra relative to temperature measured at 20 Hz. Correction factors above 1 Hz were shown to be of the order of 2 %–3 %. Losses due to low frequency can occur due to an insufficient length of averaging period. Previous studies at the BT Tower for 30 min flux averaging periods have calculated losses due to high-pass filtering to be <5 % (Helfter et al., 2011; Langford et al., 2010b). Since a 60 min averaging period is used here, loss will be even lower. Both of these errors are considered minor, and as a result, no correction has been applied.
2.7 Footprint modelling
A parameterised version of the backwards Lagrangian stochastic particle dispersion model implemented in eddy4R was used to estimate the footprint for each hourly flux measurement at the BT Tower. The model is described by Kljun et al. (2004) and has been parameterised for a range of meteorological conditions and receptor heights to reduce the computational expense of running it. The original model aims to produce a cross-wind-integrated footprint function as a function of its along-wind distance, which has now been further extended into two dimensions using a Gaussian distribution driven by the standard deviation in the cross-wind component (Metzger et al., 2012; Kljun et al., 2015). Meteorology statistics from the eddy covariance calculations are used in combination with modelled boundary layer height from ERA5 (CDS, 2021) and a surface roughness length of 1.1 m to produce a weighted matrix of 100×100 m grid cells. Each output-weighted matrix was then scaled and aligned to an appropriate coordinate reference system to allow each matrix to be plotted onto a map.
2.8 Traffic data
Hourly traffic loads surrounding the BT Tower were calculated by summing the traffic load from each of the 24 automatic traffic counters (ATCs) within the flux footprint, as shown in Fig. 1. This gave an indication of the magnitude of traffic load for both measurement periods and allowed relative changes to be studied between the two years. In addition, daily vehicle length breakdown was examined, from which vehicles were separated into three length classes: <5.2 m, indicating the number of passenger cars; 5.2–12 m, indicating the number of vans and rigid lorries; and >12 m, indicating the number of buses and articulated lorries. As the LAEI estimates that almost all lorry emissions in central London are due to the rigid class, the >12 m class is assumed to solely be made up of buses. Data were provided by the Operational Analysis Department, Transport for London (TFL) via a freedom-of-information request (Transport for London, 2021).
2.9 Emissions inventories
The London Atmospheric Emissions Inventory (LAEI) is a spatially disaggregated 1 km2 gridded map of the annual emissions of various air pollutants for the London area up to the M25 motorway ring road (Greater London Authority, 2016). Annual emissions are estimated using emission factors and activity factors for the different sources. For example, emissions from domestic combustion in tonnes per year would be calculated as gas consumption (GW h per year) × emission factor (tonnes of pollutant per GW h). The inventory is produced roughly every 3 years by TFL and the Greater London Authority (GLA). At the time of writing, there were no inventory estimates for the pandemic-affected years of 2020 and 2021. We therefore use the most recent version produced in 2016, which relates to a “normal” year unaffected by lockdowns, to understand how emissions have changed since the 2017 measurements.
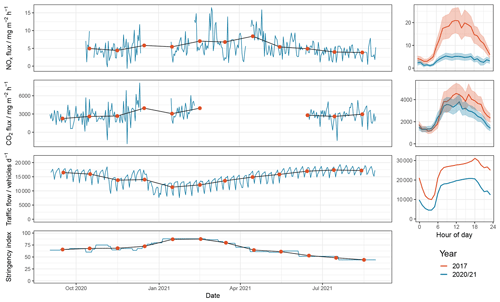
Figure 2Time series from September 2020 to September 2021 for daily time-averaged NOx flux, CO2 flux, traffic load around the tower and stringency index in the UK. Also shown are monthly averages for each variable as red dots centred around the centre of each month. Average median diurnal profiles with error bars (calculated as the combination of random and systematic errors in the flux calculations, as described by Mann and Lenschow (1994)) for the data are shown to the right in blue for 2020–2021 in comparison to those generated from the 2017 data in red.
Of the 8760 h in the year, 7034 h of NOx fluxes were calculated. Data loss was largely due to instrument or sample pump failure. Of these 7034 h, a further 3621 h were removed by the quality control flagging to leave 3413 h of NOx fluxes to be analysed. These data are displayed in Fig. 2 along with measured CO2 flux, traffic load around the tower and the UK's restrictions stringency index as calculated by the Oxford COVID-19 Government Response Tracker (Hale et al., 2021). Traffic load around the tower was strongly anti-correlated with stringency index, as expected. However, there was no obvious correlation of NOx flux with traffic load. In fact, NOx flux displays an anti-correlation with traffic flow and stringency index from April to August. This is likely due to a reduction in heat and power generation emissions due to the warmer weather, which is a first indication that traffic may not be the dominant source of NOx flux during this period. Traffic loads had not recovered to pre-pandemic levels either (Fig. A3) despite the fact that all lockdown restrictions were fully removed on 21 June 2021, hinting at a more long-term change in behaviour. This is not unexpected, as the stringency index remained at 40 %, mainly due to self-isolation requirements and international travel restrictions, which were still present at this stage. In the absence of a long-term time series from which a number of studies have used boosted regression models to predict normal emission scenarios, we compare data to that previously measured from March–August 2017 by Drysdale et al. (2022). This is an ideal time period for comparison, since the measurement footprint was very similar (see Fig. A2), and meteorological conditions meant minimal bias was expected between the years (see Sect. 2.6.2). Average diurnal NOx fluxes were down by 73 % (3.45 mg m−2 h−1 vs. 12.88 mg m−2 h−1). However, only a corresponding 20 % reduction in CO2 flux (2455 mg m−2 h−1 vs. 3062 mg m−2 h−1) and a 32 % reduction in traffic load (16 540 vehicles per day vs. 24 405 vehicles per day) around the measurement site were observed. These changes can be clearly seen in the diurnal profiles in Fig. 2. These % changes were calculated after application of the different vertical flux divergence corrections discussed in Sect. 2.6.2, and they exhibited a negligible variation of <1 %.
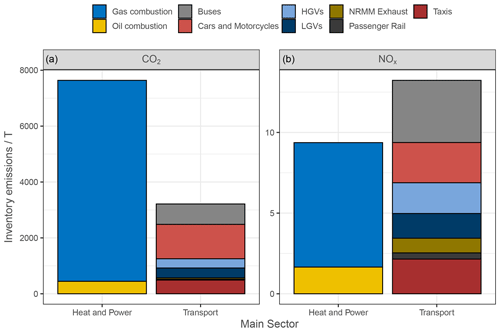
Figure 3Inventory-estimated breakdown of emissions for CO2 (a) and NOx (b) in tonnes for March through August of 2017, as determined from the inventory emissions extraction for our 1-hourly measurement footprints. LGV = light-goods vehicles; HGV = heavy-goods vehicles; NRMM = non-road mobile machinery.
3.1 Calculation of inventory-estimated emissions
Estimated emissions from the London Atmospheric Emissions Inventory (LAEI) for the measurement footprint were calculated to aid understanding of these observations. The hourly-footprint-weighted matrix output from eddy4R was used to select the relevant areas of the LAEI. The theoretical contribution to the flux was extracted from each footprint grid cell and scaled for hour of day, day of week and month of year for each emissions sector using a set of anthropogenic scaling factors described by Drysdale et al. (2022). An excellent agreement between the diurnal profiles and measurement footprint (shown in Fig. A2) for the 2017 and 2020–2021 measurement periods was seen. This gave us confidence that any changes in emissions were not to do with sampling in different times of year or sampling in different areas of central London. The source breakdown of the 2017 inventory-generated time series for both CO2 and NOx is shown in Fig. 3. Emissions of both species are almost entirely made up from combustion of fossil fuels to generate heat and power in the domestic, commercial and industrial sectors and the transport sector, which is dominated by various forms of road transport. However, each species has a significantly different relative contribution from each sector. A total of 75 % of CO2 emissions are estimated to arise from heat and power generation, but only 42 % of NOx emissions are estimated to arise from the same source.
3.2 Source apportionment of emissions reduction
The inventory breakdown for each species and the different percentage reductions in measured emissions since 2017 were used to disentangle changes in emissions of each sector. This was done simultaneously using a number of assumptions.
Helfter et al. (2016) highlight the excellent agreement of CO2 emissions measured at the BT Tower with those estimated by the LAEI, and thus, the source contributions of 75 % from heat and power generation and 25 % from transport for CO2 are taken as accurate here. On the other hand, previous observations have shown a significant underestimation of NOx emissions in central London (Vaughan et al., 2021; Drysdale et al., 2022). This is most likely due to an underrepresentation of road transport NOx emissions, in line with a poor representation of diesel vehicle emissions and/or congestion. Therefore, rather than using the inventory-predicted 42:58 split for heat and power generation:transport, the relative contributions were varied. Labelled as α:β, different scenarios between 50:50 and 0:100 (where %) were chosen to represent all possible levels of NOx underestimation. The percentage reduction in heat and power generation emissions for both species is labelled as x with the assumption that any reduction in this sector's emissions would have the same reduction in measured flux for both species. With minimal legislation for the sector introduced between 2017 and 2020–2021 and a failure to address NOx emissions from boilers in the UK's Clean Air Strategy, this assumption is considered reasonable (DEFRA, 2019). However, this is likely to be untrue for transport. Policy implemented between the two measurement periods specifically targeted NOx emissions, and NOx emissions are disproportionately higher in higher traffic loads due to the ineffectiveness of exhaust treatment systems in that environment. Additionally, the modernisation of the vehicle fleet will have introduced more vehicles with lower emission ratios. Therefore, the relative change in the emissions of NOx and CO2 from traffic sources may not have been the same, and different values are given here as y and y′. This information is all summarised in Table 1, with the two independent constraints displayed in Eq. (7) for CO2 and Eq. (8) for NOx:
The different scenarios are visualised in Fig. 4 to aid understanding of the possible solutions. Two bounding conditions drawn as dashed lines are applied to constrain the solutions. These are as follows: (1) a reduction in transport emissions greater than 100 % is not possible, and (2) CO2 emissions from transport must have decreased by at least 32 % in line with the 32 % reduction in traffic load. In reality, CO2 emissions will have decreased by more than 32 % as a result of the fleet modernisation which has lead to a decrease in the average CO2 emissions per new vehicle registration (European Environment Agency, 2022).
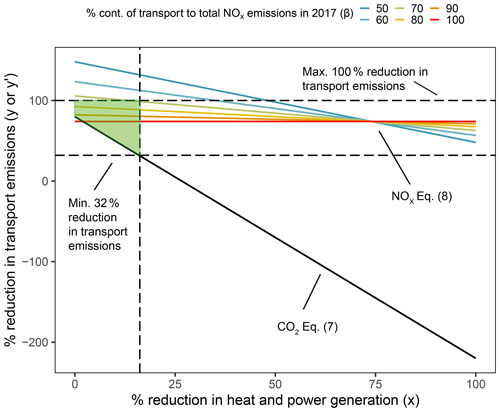
Figure 4A plot showing the external constraints on NOx and CO2 emissions. Equation (7) is shown as the solid black line, and Eq. (8) is shown as the coloured solid lines, with each colour reflecting a different value of β. The two horizontal dashed lines represent the two discussed constraints, and the vertical dashed line shows the constraint that the minimum reduction of 32 % in traffic CO2 emissions places on the NOx scenarios. All possible solutions when the constraints are applied are highlighted by the shaded green area.
Highlighted in green are all the resulting possible solutions where, crucially, to achieve the observed reductions in NOx flux, there must have been a 73 %–100 % reduction in transport NOx emissions, with transport contributing >70 % to total NOx emissions. This transport contribution percentage demonstrates the underestimation in the inventory of transport emissions, in agreement with Karl et al. (2017) and Drysdale et al. (2022). However, the most interesting observation is that a 73 %–100 % decrease in transport NOx emissions is seen for only a 32 % decrease in traffic load since 2017.
When compared to concentrations, we found that NOx concentrations at Marylebone Road, a kerbside monitoring site within the flux footprint, had declined by 62 % between the two periods (248 µg m−3 vs. 95 µg m−3). This is increased to 69 % (214 µg m−3 vs. 67 µg m−3) when looking at the roadside increment concentration (roadside – urban background) as determined from Marylebone Road and London North Kensington monitoring sites. Whilst this was slightly lower in magnitude than the measured change in flux, the concentration data will have been heavily influenced by meteorology, and so some disagreement was expected. There are a number of plausible explanations for the large decrease in NOx fluxes. The introduction of the ULEZ is thought to have resulted in a pre-pandemic 31 % reduction in NOx emissions from road transport in central London (Greater London Authority, 2020); this is likely to be an upper estimate for our measurements due to the fact of a significant proportion of our flux footprint being situated outside of the ULEZ zone. With average traffic loads between April and November 2019 after the ULEZ was introduced only being down 1.8 % on 2018 levels for the same period, the vast majority of the reduction is due to a clean-up of the fleet, which reduces the emissions per vehicle per unit distance. The remaining emissions are further reduced by 32 % due to there being 32 % less vehicles on the roads surrounding the BT tower during the pandemic. This leaves ∼20 %–45 % of unaccounted-for emissions reduction. A small portion of this unaccounted-for reduction may be due to a 40 % reduction in the number of buses on the roads surrounding the BT Tower during 2020–2021. The >12 m class of the vehicle length breakdown in Fig. A4 represents the bus classification. With buses making up 17 % of the total NOx emissions from road transport, the decrease in relative proportion between 2017 and 2020–2021 could result in a maximum of 7 % extra reduction in NOx emissions. It is likely to be less than 7 % due to the small increase in the relative proportion of the 5.2–12 m class.
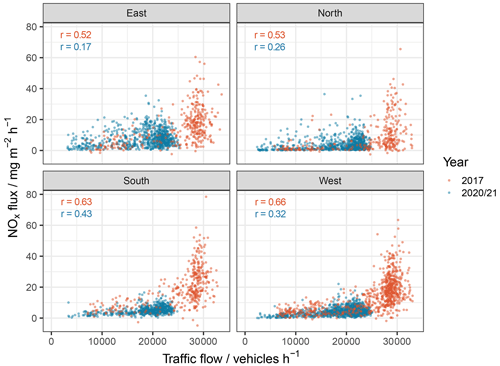
Figure 5Comparison of the measured NOx flux with hourly traffic load (sum of the 24 surrounding ATCs) for March through August 2017 (red) and September 2020–September 2021 (blue). The data are split by wind direction: north (315–45∘), east (45–135∘), south (135–225∘) and west (225–315∘). In the top left of each facet is the Spearman correlation coefficient for each year for the corresponding wind direction.
3.3 Flux correlations with traffic load
Examining how the NOx flux correlated with traffic load for both measurement time periods gives further insight into the unaccounted emissions reduction. Figure 5 generally shows significantly enhanced NOx emissions in 2017 above 25 000 vehicles per hour. With road transport being the dominant source during these measurements, it is highlighting what is thought to be the effect of congestion on NOx emissions.
During periods of high congestion, increased emissions are expected due to increased length in journey time, a greater number of accelerations in the stop–start nature of traffic and the reduced effectiveness of exhaust gas NOx treatment systems in diesel vehicles at low engine temperatures (Carslaw and Rhys-Tyler, 2013). The effect of congestion on NOx emissions is highly dependent on several variables, including the fleet composition, type of exhaust treatment system and the actual level of congestion (Ko et al., 2019). It is thought that, for individual roads, excess emissions from congestion can be anything up to 75 % greater than those from non-congested roads (Gately et al., 2017). Therefore, it is thought that reducing the peak-traffic load below 25 000 vehicles per hour has had a large impact on traffic NOx emissions, more than accounting for the remaining emissions reduction.
3.4 Spatial mapping
This change in emissions is clearly seen in the spatial mapping of the NOx fluxes in Fig. 6. Drysdale et al. (2022) assigned the heightened emissions to the northeast of the BT Tower in Fig. 6a to Euston station, including not only the train station but also the large Euston bus station, taxi ranks and busy roads feeding the station. In addition, the high fluxes measured to the southwest are assigned to highly congested streets such as Oxford Street, Regent Street and Piccadilly. Both areas are associated with high traffic volumes and congestion and support the notion that road transport emissions dominated in central London in 2017. However, these areas have almost an order of magnitude smaller emissions and are barely visible for 2020–2021 when shown on the same scale. This adds additional support to the conclusion that reduced traffic load and thus reduced congestion in 2020–2021 have been a major cause of the reduced NOx flux.
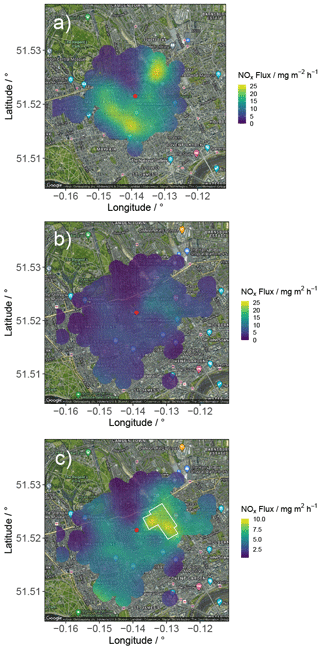
Figure 6NOx flux surfaces as a function of along-wind distance to the footprint maximum contribution and wind direction, as derived by Drysdale et al. (2022), for (a) March through August 2017 and (b) September 2020 to September 2021, displayed on the same scale for ease of visual comparison. Also shown in (c): September 2020 to September 2021 on its own scale with the border for the University of London overlaid in white. The location of the BT Tower site is displayed as a red dot in each spatial map. Maps are produced using Google Maps (© Google Maps 2022), accessed using an API in R.
The spatial map for 2020–2021 in Fig. 6c) on its own scale identified a shift in the dominant NOx emissions source between 2017 and 2020–2021. Whilst Euston station and the previously congested southwesterly area are still noticeable, the major stand-out area is to the east. Depicted as a white box is the outline of the University of London point source as documented by the National Atmospheric Emissions Inventory, a similar inventory to the LAEI but for the whole of the UK (Defra and BEIS, 2020). The University of London is the largest university in the UK, and its Bloomsbury Campus appears directly under the heightened emissions area. This site is made up of much of the University College London (UCL) central administration, the UCL hospital and Bloomsbury Heat and Power, a number of combined heat and power (CHP) sites to power the university. CHP systems simultaneously generate heat and electrical power from a single source of energy. By capturing and utilising the heat that is generated as a byproduct of the electricity generation process, efficiency is increased, which can reduce carbon emissions by up to 30 % compared to conventional separate generation (Department for Business, Energy and Industrial Strategy, 2021). However, the requirement for CHP to be in urban areas risks an increase in air pollution. Indeed, it has been shown that CHP can “substantially” impact air quality due to NOx, the highest criteria judged by Environment Protection UK and the Institute of Air Quality Management (Fuller et al., 2018). Here, the heat and power generation source stands out and dominates over transport but is only seen due to the drastic reduction in transport NOx emissions during the period of pandemic-reduced mobility. The Spearman correlation coefficients presented in Fig. 5 give further evidence that the dominant source between the two periods has changed. Correlations between NOx flux and traffic load are reduced in 2020–2021, particularly in the easterly direction. Here, the lowest correlation is observed, and high NOx fluxes are seen even at low traffic loads. These observations are in agreement with the spatial-mapping interpretation in that heat and power generation is the dominant source from this direction.
Eddy covariance emissions measurements at the unique BT Tower site in central London provide an opportunity to study the evolution of air pollutant emissions in a megacity and the part that policy and other external stimuli play in improving air quality. Here, the direct emissions measurements have shown that reducing congestion could be an even more effective way of reducing NOx emissions from road transport than the ULEZ. However, this is not the direction in which the UK is heading. With much cheaper mileage, the continued uptake of electric vehicles is predicted to increase congestion. Reducing the number of vehicles on the road by improving infrastructure for other greener methods of travel such as cycling would not only achieve reduced congestion but give additional benefits to health, further reducing costs of treatment at health services (Fishman et al., 2015). A more targeted approach to simultaneously reduce congestion, as well as emissions per vehicle per unit distance, is therefore recommended to other cities looking to implement policies to tackle high traffic NOx emissions.
The observation that NOx emissions in central London during this continuing period of reduced mobility were thought to be dominated by heat and power generation is an important one. This is a transition which was expected to occur in the coming years but was brought forward in time by the pandemic, providing a glimpse into future air quality. As of 2020, there were 2659 CHP sites in the UK, with additional widespread usage in Europe (Department for Business, Energy and Industrial Strategy, 2021). Due to their increased efficiency and the push towards net-zero economies, they are expected to increase in popularity. Despite this period of drastically reduced transport emissions, all air quality monitoring sites (urban background, urban traffic and kerbside) in London far exceeded the new WHO NO2 air quality target. To achieve these targets, it is therefore clear that legislation is required to reduce NOx emissions from heat and power generation. The heat and power generation source has been somewhat neglected due to the prominence of issues with diesel vehicle emissions. But with the planned use of hydrogen combustion in decarbonisation, which currently has major uncertainties due to a lack of experimental data, now is the critical time to start thinking about policy intervention for this sector (Lewis, 2021). This makes the lack of acknowledgement for gas combustion in boilers in the UK's Clean Air Strategy highly disappointing. This is the first indication from a megacity which shows that heat and power emissions will need to be regulated to achieve the new air quality NOx targets. As more and more of the world's population is expected to live in urban areas, it is essential that compliance with WHO targets is achieved to minimise health and economic impacts. The conclusions derived from this work will therefore be of interest to other nations, especially with air quality improvements being increasingly sought in the developing world.
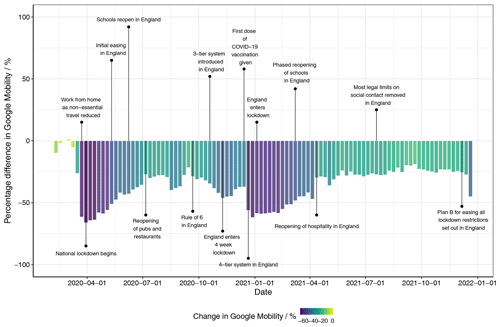
Figure A1A timeline of COVID restrictions in England from the start of the COVID-19 pandemic until January 2022. Coloured bars represent the weekly change in Google mobility across the UK.
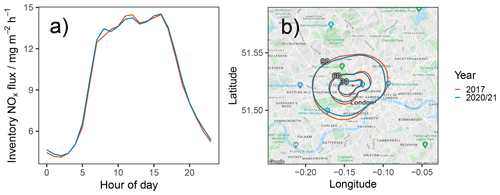
Figure A2Comparison of (a) the diurnal profile of the inventory-generated time series and (b) the 30 %, 60 % and 90 % footprint contribution contours for the 2017 data set in black and the 2020/2021 data set in red. Map in (b) produced from Google Maps (© Google Maps 2021), accessed using an API in R.
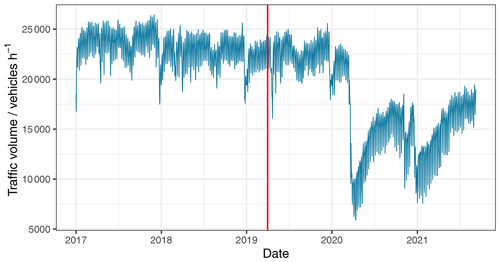
Figure A3Daily average traffic load from 1 January 2017–1 September 2021. The date of the introduction of the ULEZ is marked as a vertical red line.
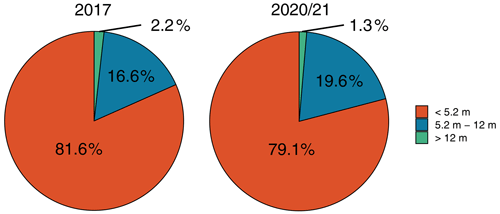
Figure A4Pie chart for the 2017 and 2020–2021 measurement periods displaying the distribution of vehicle length classes measured by the 24 ATCs surrounding the BT Tower.
The eddy4R v.0.2.0 software framework used to generate eddy covariance flux estimates can be freely accessed at https://github.com/NEONScience/eddy4R (Metzger et al., 2017). The eddy4R turbulence v0.0.16 software module was accessed under the terms of use for this study (https://www.eol.ucar.edu/content/cheesehead-code-policy-appendix; EOL, 2023) and are available upon request.
Automatic Urban and Rural Network data used in the analysis were obtained from https://uk-air.defra.gov.uk/networks/network-info?view=aurn (DEFRA, 2021) under “Open Government Licence v3.0”. The London Atmospheric Emissions Inventory is available at https://data.london.gov.uk/dataset/london-atmospheric-emissions-inventory--laei--2016 (Greater London Authority, 2016) (© Crown 2022 copyright Defra & BEIS, licensed under the Open Government Licence – OGL). For the measurement data in this paper, the calculated fluxes are not available in any repository due to the intensity of the processing and interpretation required. We are happy to make this available upon request; 15 min aggregated concentrations are available on the Centre for Environmental Data Analysis database, but these were not directly used here. The ERA5 boundary layer height data can be accessed at http://cds.climate.copernicus.eu/cdsapp#!/home (CDS, 2021). The traffic count data used in this article were provided by Transport for London (2021) (Automatic Traffic Counter data; original source data provided by Operational Analysis department, Transport for London).
SJC made the NOx measurements, calculated the fluxes and performed the analyses. SJC wrote the paper and produced the figures with input from the co-authors. WD provided support with the measurements, flux calculations and interpretation of the data. JDL provided support for the measurements and interpretation of the data. CH and EN provided supporting measurements from the site and aided in interpretation of the data. SM provided training on the eddy4R software and aided in interpretation of the data. JFB provided meteorological data for the site.
The contact author has declared that none of the authors has any competing interests.
Publisher's note: Copernicus Publications remains neutral with regard to jurisdictional claims in published maps and institutional affiliations.
The authors thank the National Ecological Observatory Network, a programme sponsored by the National Science Foundation and operated under cooperative agreement by Battelle. This material is based in part upon work supported by the National Science Foundation through the NEON Programme. The authors also thank Neil Mullinger and Karen Yeung (UK Centre for Ecology and Hydrology) for instrument and sample line maintenance; Ally Lewis and Rhianna Evans for help in paper preparation; and British Telecom (BT) for granting use of the tall tower for research purposes, particularly Karen Ahern and Guille Parada for arranging work permits and facilitating access to the site.
This research has been supported by the UK Natural Environment Research Council and the Integrated Research Observation System for Clean Air project (grant nos. NE/T001917/1, NE/T001798/2) as well as through UKCEH's UK-SCAPE programme delivering National Capability (grant no. NE/R016429/1). Samuel Cliff was supported by the Panorama Natural Environment Research Council (NERC) Doctoral Training Partnership (DTP) (grant no. NE/S007458/1).
This paper was edited by Thomas Karl and reviewed by two anonymous referees.
Baldocchi, D., Falge, E., Gu, L., Olson, R., Hollinger, D., Running, S., Anthoni, P., Bernhofer, C., Davis, K., Evans, R., Fuentes, J., Goldstein, A., Katul, G., Law, B., Lee, X., Malhi, Y., Meyers, T., Munger, W., Oechel, W., Paw, U. K. T., Pilegaard, K., Schmid, H. P., Valentini, R., Verma, S., Vesala, T., Wilson, K., and Wofsy, S.: FLUXNET: A new tool to study the temporal and spatial variability of ecosystem-scale carbon dioxide, water vapor, and energy flux densities, B. Am. Meteorol. Soc., 82, 2415–2434, https://doi.org/10.1175/1520-0477(2001)082<2415:FANTTS>2.3.CO;2, 2001. a
Barlow, J. F., Dunbar, T. M., Nemitz, E. G., Wood, C. R., Gallagher, M. W., Davies, F., O'Connor, E., and Harrison, R. M.: Boundary layer dynamics over London, UK, as observed using Doppler lidar during REPARTEE-II, Atmos. Chem. Phys., 11, 2111–2125, https://doi.org/10.5194/acp-11-2111-2011, 2011. a
Butterbach-Bahl, K., Baggs, E. M., Dannenmann, M., Kiese, R., and Zechmeister-Boltenstern, S.: Nitrous oxide emissions from soils: how well do we understand the processes and their controls?, Philos. T. Roy. Soc. B, 368, 20130122, https://doi.org/10.1098/rstb.2013.0122, 2013. a
Carslaw, D. C. and Rhys-Tyler, G.: New insights from comprehensive on-road measurements of NOx, NO2 and NH3 from vehicle emission remote sensing in London, UK, Atmos. Environ., 81, 339–347, https://doi.org/10.1016/j.atmosenv.2013.09.026, 2013. a
CDS – Copernicus Climate Change Service Climate Data Store: Copernicus Climate Change Service (C3S): ERA5: Fifth generation of ECMWF atmospheric reanalyses of the global climate, https://cds.climate.copernicus.eu/cdsapp#!/home, last access: October 2021. a, b
Ciencewicki, J. and Jaspers, I.: Air Pollution and Respiratory Viral Infection, Inhalat. Toxicol. 19, 1135–1146, https://doi.org/10.1080/08958370701665434, 2007. a
DEFRA – Department for Environment, Food and Rural Affairs: Clean Air Strategy 2019, https://assets.publishing.service.gov.uk/government/uploads/system/uploads/attachment_data/file/770715/clean-air-strategy-2019.pdf (last access: 15 February 2023), 2019. a
DEFRA – Department for Environment, Food and Rural Affairs: UK AIR Air Information Resource – Automatic Urban and Rural Network (AURN), https://uk-air.defra.gov.uk/networks/network-info?view=aurn (last access: 15 February 2023), 2021. a
Defra and BEIS: National Atmospheric Emissions Inventory, licenced under the Open Government Licence (OGL), Crown Copyright, https://naei.beis.gov.uk/ (last access: 15 February 2023), 2020. a
Department for Business, Energy and Industrial Strategy (BEIS): Digest of UK Energy Statistics, https://www.gov.uk/government/statistics/digest-of-uk-energy-statistics-dukes-2021 (last access: 15 February 2023), 2021. a, b
Drysdale, W. S., Vaughan, A. R., Squires, F. A., Cliff, S. J., Metzger, S., Durden, D., Pingintha-Durden, N., Helfter, C., Nemitz, E., Grimmond, C. S. B., Barlow, J., Beevers, S., Stewart, G., Dajnak, D., Purvis, R. M., and Lee, J. D.: Eddy covariance measurements highlight sources of nitrogen oxide emissions missing from inventories for central London, Atmos. Chem. Phys., 22, 9413–9433, https://doi.org/10.5194/acp-22-9413-2022, 2022. a, b, c, d, e, f, g, h, i, j, k, l, m
EOL: CHEESEHEAD Code Policy Appendix, https://www.eol.ucar.edu/content/cheesehead-code-policy-appendix, last access: 15 February 2023. a
European Environment Agency: CO2 performance of new passenger cars in Europe, https://www.eea.europa.eu/ims/co2-performance-of-new-passenger#ref-DNi82, last access: December 2022. a
European Parliament: Directive 2008/50/EC of the European Parliament and of the council of 21 May 2008 on ambient air quality and cleaner air for Europe, https://eur-lex.europa.eu/legal-content/EN/TXT/?uri=CELEX:02008L0050-20150918 (last access: October 2022), 2008. a
Fishman, E., Schepers, P., and Kamphuis, C. B. M.: Dutch cycling: Quantifying the health and related economic benefits, Am. J. Publ. Health, 105, e13–e15, https://doi.org/10.2105/AJPH.2015.302724, 2015. a
Font, A., Guiseppin, L., Blangiardo, M., Ghersi, V., and Fuller, G. W.: A tale of two cities: is air pollution improving in Paris and London?, Environ. Pollut., 249, 1–12, https://doi.org/10.1016/j.envpol.2019.01.040, 2019. a
Fuller, G., Font, A., Baker T., and Johnson, P.: Urban air pollution from combined heat and power plants A measurement-based investigation, King's College London, https://www.london.gov.uk/sites/default/files/urban_air_pollution_from_combined_heat_and_power_plants.pdf (last access: 15 February 2023), 2018. a
Gately, C. K., Hutyra, L. R., Peterson, S., and Sue Wing, I.: Urban emissions hotspots: Quantifying vehicle congestion and air pollution using mobile phone GPS data, Environ. Pollut., 229, 496–504, https://doi.org/10.1016/j.envpol.2017.05.091, 2017. a
Greater London Authority: London Atmospheric Emissions Inventory (LAEI) 2016, Greater London Authority [data set], https://data.london.gov.uk/dataset/london-atmospheric-emissions-inventory--laei--2016 (last access: March 2022), 2016. a, b
Greater London Authority: Central London ultra low emission zone – ten month report, https://www.london.gov.uk/sites/default/files/ulez_ten_month_evaluation_report_23_april_2020.pdf (last access: 15 February 2023), 2020. a
Griffis, T. J., Sargent, S. D., Baker, J. M., Lee, X., Tanner, B. D., Greene, J., Swiatek, E., and Billmark, K.: Direct measurement of biosphere-atmosphere isotopic CO2 exchange using the eddy covariance technique, J. Geophys. Res.-Atmos., 113, D08304, https://doi.org/10.1029/2007JD009297, 2008. a
Hale, T., Angrist, N., Goldszmidt, R., Kira, B., Petherick, A., Phillips, T., Webster, S., Cameron-Blake, E., Hallas, L., Majumdar, S., and Tatlow, H.: A global panel database of pandemic policies (Oxford COVID-19 Government Response Tracker), Nat. Hum. Behav., 5, 529–538, https://doi.org/10.1038/s41562-021-01079-8, 2021. a
Hartmann, J., Gehrmann, M., Kohnert, K., Metzger, S., and Sachs, T.: New calibration procedures for airborne turbulence measurements and accuracy of the methane fluxes during the AirMeth campaigns, Atmos. Meas. Tech., 11, 4567–4581, https://doi.org/10.5194/amt-11-4567-2018, 2018. a
Helfter, C., Famulari, D., Phillips, G. J., Barlow, J. F., Wood, C. R., Grimmond, C. S. B., and Nemitz, E.: Controls of carbon dioxide concentrations and fluxes above central London, Atmos. Chem. Phys., 11, 1913–1928, https://doi.org/10.5194/acp-11-1913-2011, 2011. a
Helfter, C., Tremper, A. H., Halios, C. H., Kotthaus, S., Bjorkegren, A., Grimmond, C. S. B., Barlow, J. F., and Nemitz, E.: Spatial and temporal variability of urban fluxes of methane, carbon monoxide and carbon dioxide above London, UK, Atmos. Chem. Phys., 16, 10543–10557, https://doi.org/10.5194/acp-16-10543-2016, 2016. a, b, c, d, e
Imperial College London: Investigating links between air pollution, COVID-19 and lower respiratory infectious diseases, https://www.imperial.ac.uk/media/imperial-college/medicine/sph/environmental-research-group/ReportfinalAPCOVID19_v10.pdf (last access: 15 February 2023), 2021. a
Jet Propulsion Laboratory: Chemical Kinetics and Photochemical Data for Use in Atmospheric Studies, Evaluation No. 19, https://jpldataeval.jpl.nasa.gov/ (last access: January 2022), 2020. a
Karl, T., Graus, M., Striednig, M., Lamprecht, C., Hammerle, A., Wohlfahrt, G., Held, A., von der Heyden, L., Deventer, M. J., Krismer, A., Haun, C., Feichter, R., and Lee, J.: Urban eddy covariance measurements reveal significant missing NO_x emissions in central Europe, Sci. Rep., 7, 2536–2545, https://doi.org/10.1038/s41598-017-02699-9, 2017. a, b
Kljun, N., Calanca, P., and Rotach, M. W.: A Simple Parameterisation for Flux Footprint Predictions, Bound.-Lay. Meteorol., 112, 503–523, https://doi.org/10.1023/B:BOUN.0000030653.71031.96, 2004. a
Kljun, N., Calanca, P., Rotach, M. W., and Schmid, H. P.: A simple two-dimensional parameterisation for Flux Footprint Prediction (FFP), Geosci. Model Dev., 8, 3695–3713, https://doi.org/10.5194/gmd-8-3695-2015, 2015. a
Ko, J., Myung, C.-L., and Park, S.: Impacts of ambient temperature, DPF regeneration, and traffic congestion on NOx emissions from a Euro 6-compliant diesel vehicle equipped with an LNT under real-world driving conditions, Atmos. Environ., 200, 1–14, https://doi.org/10.1016/j.atmosenv.2018.11.029, 2019. a
Lamprecht, C., Graus, M., Striednig, M., Stichaner, M., and Karl, T.: Decoupling of urban CO2 and air pollutant emission reductions during the European SARS-CoV-2 lockdown, Atmos. Chem. Phys., 21, 3091–3102, https://doi.org/10.5194/acp-21-3091-2021, 2021. a
Lane, S., Barlow, J., and Wood, C.: An assessment of a three-beam Doppler lidar wind profiling method for use in urban areas, Environ. Sci. Technol., 49, 53–59, https://doi.org/10.1016/j.jweia.2013.05.010, 2013. a
Langford, B., Nemitz, E., House, E., Phillips, G. J., Famulari, D., Davison, B., Hopkins, J. R., Lewis, A. C., and Hewitt, C. N.: Fluxes and concentrations of volatile organic compounds above central London, UK, Atmos. Chem. Phys., 10, 627–645, https://doi.org/10.5194/acp-10-627-2010, 2010a. a
Langford, B., Nemitz, E., House, E., Phillips, G. J., Famulari, D., Davison, B., Hopkins, J. R., Lewis, A. C., and Hewitt, C. N.: Fluxes and concentrations of volatile organic compounds above central London, UK, Atmos. Chem. Phys., 10, 627–645, https://doi.org/10.5194/acp-10-627-2010, 2010b. a
Lee, J. D., Helfter, C., Purvis, R. M., Beevers, S., Carslaw, D. C., Lewis, A. C., Moller, S. J., Tremper, A., Vaughan, A., and Nemitz, E. G.: Measurement of NOx fluxes from a tall tower in central London, UK and comparison with emissions inventories, J. Wind Eng. Indust. Aerodynam., 49, 1025–1034, https://doi.org/10.1021/es5049072, 2014. a, b
Lee, J. D., Whalley, L. K., Heard, D. E., Stone, D., Dunmore, R. E., Hamilton, J. F., Young, D. E., Allan, J. D., Laufs, S., and Kleffmann, J.: Detailed budget analysis of HONO in central London reveals a missing daytime source, Atmos. Chem. Phys., 16, 2747–2764, https://doi.org/10.5194/acp-16-2747-2016, 2016. a
Lewis, A. C.: Optimising air quality co-benefits in a hydrogen economy: a case for hydrogen-specific standards for NOx emissions, Environ. Sci.: Atmos., 1, 201–207, https://doi.org/10.1039/D1EA00037C, 2021. a
Mann, J. and Lenschow, D. H.: Errors in airborne flux measurements, J. Geophys. Res.-Atmos., 99, 14519–14526, https://doi.org/10.1029/94JD00737, 1994. a
Metzger, S., Junkermann, W., Mauder, M., Beyrich, F., Butterbach-Bahl, K., Schmid, H. P., and Foken, T.: Eddy-covariance flux measurements with a weight-shift microlight aircraft, Atmos. Meas. Tech., 5, 1699–1717, https://doi.org/10.5194/amt-5-1699-2012, 2012. a
Metzger, S., Junkermann, W., Mauder, M., Butterbach-Bahl, K., Trancón y Widemann, B., Neidl, F., Schäfer, K., Wieneke, S., Zheng, X. H., Schmid, H. P., and Foken, T.: Spatially explicit regionalization of airborne flux measurements using environmental response functions, Biogeosciences, 10, 2193–2217, https://doi.org/10.5194/bg-10-2193-2013, 2013. a
Metzger, S., Durden, D., Xu, K., Natchaya, P.-D., Hongyan, L., and Florian, C.: NEON Algorithm Theoretical Basis Document (ATBD): Eddy-Covariance Data Products Bundle, https://data.neonscience.org/api/v0/documents/NEON.DOC.004571vF (last access: January 2023), 2022. a
Metzger, S., Durden, D., Sturtevant, C., Luo, H., Pingintha-Durden, N., Sachs, T., Serafimovich, A., Hartmann, J., Li, J., Xu, K., and Desai, A. R.: eddy4R 0.2.0: a DevOps model for community extensible processing and analysis of eddy-covariance data based on R, Git, Docker, and HDF5, Geosci. Model Dev., 10, 31893206, https://doi.org/0.5194/gmd-10-3189-2017 (code available at: https://github.com/NEONScience/eddy4R, last access: 15 February 2023), 2017. a
Public Health England: Estimation of costs to the NHS and social care due to the health impacts of air pollution: summary report, https://assets.publishing.service.gov.uk/government/uploads/system/uploads/attachment_data/file/708855/Estimation_of_costs_to_the_NHS_and_social_care_due_to_the_health_impacts_of_air_pollution_-_summary_report.pdf (last access: 15 February 2023), 2018. a
Smith, D. and Metzger, S.: Algorithm Theoretical Basis Document: Quality Flags and Quality Metrics for TIS Data Products, https://data.neonscience.org/api/v0/documents/NEON.DOC.001113vA (last access: June 2022), 2013. a
Squires, F. A., Nemitz, E., Langford, B., Wild, O., Drysdale, W. S., Acton, W. J. F., Fu, P., Grimmond, C. S. B., Hamilton, J. F., Hewitt, C. N., Hollaway, M., Kotthaus, S., Lee, J., Metzger, S., Pingintha-Durden, N., Shaw, M., Vaughan, A. R., Wang, X., Wu, R., Zhang, Q., and Zhang, Y.: Measurements of traffic-dominated pollutant emissions in a Chinese megacity, Atmos. Chem. Phys., 20, 8737–8761, https://doi.org/10.5194/acp-20-8737-2020, 2020. a, b, c
Transport for London: Transport for London: original source data provided by Operational Analysis department, 2021. a
United Nations: World Urbanisation Prospects The 2018 Revision, https://population.un.org/wup/publications/Files/WUP2018-Report.pdf (last access: 15 February 2023), 2019. a
Vaughan, A. R., Lee, J. D., Shaw, M. D., Misztal, P. K., Metzger, S., Vieno, M., Davison, B., Karl, T. G., Carpenter, L. J., Lewis, A. C., Purvis, R. M., Goldstein, A. H., and Hewitt, C. N.: VOC emission rates over London and South East England obtained by airborne eddy covariance, Faraday Discuss., 200, 599–620, https://doi.org/10.1039/C7FD00002B, 2017. a
Vaughan, A. R., Lee, J. D., Metzger, S., Durden, D., Lewis, A. C., Shaw, M. D., Drysdale, W. S., Purvis, R. M., Davison, B., and Hewitt, C. N.: Spatially and temporally resolved measurements of NOx fluxes by airborne eddy covariance over Greater London, Atmos. Chem. Phys., 21, 15283–15298, https://doi.org/10.5194/acp-21-15283-2021, 2021. a, b
World Health Organisation: Global health estimates 2016: deaths by cause, age, sex, by country and by region, 2000–2016, https://www.who.int/health-topics/air-pollution#tab=tab_1 (last access: October 2021), 2018. a
World Health Organisation: WHO global air quality guidelines: particulate matter (PM2.5 and PM10), ozone, nitrogen dioxide, sulfur dioxide and carbon monoxide, https://apps.who.int/iris/handle/10665/345329 (last access: December 2022), 2021. a
Zhang, J., Wei, Y., and Fang, Z.: Ozone pollution: A major health hazard worldwide, Front. Immunol., 10, 2518, https://doi.org/10.3389/fimmu.2019.02518, 2019. a