the Creative Commons Attribution 4.0 License.
the Creative Commons Attribution 4.0 License.
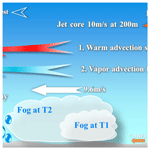
Effect of the boundary layer low-level jet on fast fog spatial propagation
Shuqi Yan
Xiaohui Liu
Fan Zu
The spatiotemporal variation of fog reflects the complex interactions among fog, boundary layer thermodynamics and synoptic systems. Previous studies revealed that fog can present a fast spatial propagation feature and attribute it to the boundary layer low-level jet (BLLJ), but the effect of the BLLJ on fog propagation is not quantitatively understood. Here we analyze a large-scale fog event in Jiangsu, China, from 20 to 21 January 2020. Satellite retrievals show that fog propagates from the southeast coastal area to the northwest inland area with a speed of 9.6 m s−1, which is 3 times larger than the ground wind speeds. The ground meteorologies are insufficient to explain the fast fog propagation, which is further investigated by Weather Research and Forecasting model (WRF) simulations. The fast fog propagation could be attributed to the BLLJ occurring between 50 and 500 m, because the wind speeds (10 m s−1) and directions (southeast) of the BLLJ core are consistent with fog propagation. Through sensitive experiments and process analysis, three possible mechanisms of the BLLJ are revealed: (1) the abundant oceanic moisture is transported inland, increasing the humidity of the boundary layer and promoting condensation; (2) the oceanic warm air is transported inland, enhancing the inversion layer and favoring moisture accumulation; and (3) the moisture advection probably promotes low-stratus formation, and later it subsides to become ground fog by turbulent mixing of fog droplets. The fog propagation speed would decrease notably by 6.4 m s−1 (66 %) in the model if the BLLJ-related moisture and warm advections were turned off.
- Article
(5005 KB) - Full-text XML
- BibTeX
- EndNote
Fog is a kind of low-visibility weather phenomenon that occurs near the surface, causing adverse impacts on traffic transportation. The formation, development and dissipation of fog are the comprehensive results of the interactions among radiation, moisture, microphysics, turbulence, aerosols and other factors (Gultepe et al., 2007; Koračin et al., 2014; Nakanishi, 2000). The relations of fog with meteorological factors are highly variable under different conditions. Therefore, the mechanism of fog evolution needs to be intensively studied.
Under favorable conditions, the fog intensity or its spatial extent can develop extraordinarily fast with time. Field observations conducted at a single site reveal that visibility in fog can deteriorate drastically, from about 1 km to less than 200 m within 30 min (Li et al., 2019). It is referred to as fog burst reinforcement, which was firstly raised by Korb and Zdunkowski (1970) and systematically reviewed by Liu et al. (2012) and Li et al. (2019). Fog burst reinforcement is accompanied by the drastic formation of fog droplets, sudden increase of fog liquid water and broadening of droplet spectrum (D. Liu et al., 2017; Q. Liu et al., 2021). Additionally, fog can develop rather fast in spatial extent, i.e., the fast spatial propagation of fog (Zhu et al., 2022). It is reflected by the successive visibility dropping in space along a certain direction. The influencing factors of fast spatial propagation could be more complex than that of the burst reinforcement at a single site, which has received fewer quantitative studies recently.
Synoptic systems and planetary boundary layer (PBL) thermodynamic structures are key to understanding the cause of fog burst reinforcement and fast propagation. Weak cold air invasion and radiative cooling is an important factor for fog burst reinforcement and fast propagation (Liu et al., 2011; Wang et al., 2020; Zhu et al., 2018). Dhangar et al. (2021) demonstrated that the radiative cooling at the surface and fog top can increase supersaturation and promote fog vertical development. Shen et al. (2022) found that the different cooling rates at two nearby stations lead to a remarkable difference in fog formation time, fog duration and vertical extent. Sufficient water supply is also an important factor. Wobrock et al. (1992) revealed that the role of moisture advection outweighs radiative cooling in large-scale fog events. Pu et al. (2008) found that two layers of moisture advection enhance fog development and maintenance. Under stable synoptic systems, the PBL thermodynamic can also favor fog burst reinforcement and fast fog propagation. The formation of dense fog is usually accompanied by a strong inversion layer, the intensity of which could reach 16 K/100 m (Pu et al., 2008; Liu et al., 2012). Liu et al. (2016) found that upper-level warm advection and low-level cold advection significantly enhance inversion intensity and promote fog development. The vapor advection resulting from southerly winds further increases fog intensity. Appropriate turbulence also facilitates fog formation and enhancement (Ye et al., 2015; Zhou and Ferrier, 2008). Turbulent results in the exchange of heat and moisture within the PBL, e.g., the downward entrainment of vapor and cold air, can promote condensation and droplet formation (Liu et al., 2016; Zhang et al., 2005). Other studies highlight the role of hygroscopic aerosols and aerosol indirect effects in strong fog events (Boutle et al., 2017; Quan et al., 2021; Shao et al., 2023; Wang et al., 2023; Yan et al., 2021).
Previous studies found that the large-scale fog events are accompanied by the boundary layer low-level jet (BLLJ) and tried to attribute the spatial propagation of fog to the BLLJ. The causes of the BLLJ include synoptic systems, the terrain effect and inertial oscillation (Kraus et al., 1985). Tian et al. (2019) demonstrated that the warm-and-wet southerly BLLJ favors water vapor transportation and inversion layer construction, and later the fog is triggered by a weak cold front invasion. Wu et al. (2020) found that the strong northerly BLLJ associated with cold air can destroy the inversion layer and lead to early dissipation of fog, while the weak BLLJ can promote fog maintenance. Li and Fu (2012) revealed that the strengthened turbulence generated by BLLJ wind shear promotes vertical mixing and facilitates fog development. However, the relations between the BLLJ and fog propagation and the key synoptic factors have not been quantitatively addressed. Also, the current horizontal and vertical observations are not sufficient to reveal the mechanism of fog propagation. It requires further investigation by numerical models.
In this work, we study a large-scale fog event with a fast-propagation feature occurring in Jiangsu Province, China from 20 to 21 January 2020. Through the combination of observations and numerical simulations, we aim to quantitatively reveal the BLLJ effect on fast fog propagation and to identify the key impact factors and mechanisms. This work is expected to better understand the complex interactions among synoptic systems, PBL thermodynamics, and fog spatial propagation, as well as provide prediction indicators for operational fog forecast. The study is organized as follows: Sect. 2 describes the data, methods and numerical models of this study. Section 3.1 to 3.4 analyzes the fog propagation feature and PBL characteristics. Section 3.5 quantitatively studies the BLLJ effect on fast fog propagation and identifies key influencing factors. Section 4 concludes the findings of this study.
2.1 Data and study area
This study focuses on the Jiangsu area, China (Fig. 1), where a large-scale fog event occurred from 20 to 21 January 2020. We collected the data from 70 ground automatic weather stations (AWSs) in Jiangsu Province, China. The data are recorded every 10 min, including visibility, temperature, relative humidity (RH), wind direction and wind speed. These data are used to analyze the temporal variation of meteorology, as well as evaluate the model performance on temperature, RH and wind. Additionally, the Sheyang (SY; 33.76∘ N, 120.25∘ E; 3 m) station is a sounding station used for model evaluation in the vertical direction. The sounding observations include temperature, RH, wind direction and wind speed, which are sampled each second. It is conducted twice a day (00:00 and 12:00 UTC).
The geostationary satellite Himawari 8 (https://www.eorc.jaxa.jp/ptree/index.html, last access: 6 November 2023) is used to retrieve nighttime fog area and evaluate the model performance of fog simulation. The high spatiotemporal resolution (2 km in space and 1 h in time) is suitable for detecting the fast evolution of the fog area. This satellite observation includes 16 bands, and the bands at 3.9 and 11.2 µm are used.
The ERA5 reanalysis data (https://cds.climate.copernicus.eu/cdsapp#!/dataset/reanalysis-era5-pressure-levels, last access: 6 November 2023) are used to analyze synoptic conditions and provide initial and boundary fields for model simulation. The grid resolution is 0.125∘ (about 12.5 km), and the time interval is 6 h. All the times in this study are in local time (UTC + 8).
2.2 Methods
2.2.1 Satellite fog retrieval
Since the ground AWSs are not sufficiently fine in spatial resolution, the high-spatiotemporal-resolution product of Himawari 8 is suitable to study the propagation of fog. Nighttime fog has notably different optical properties at the bands of 3.9 and 11.2 µm, so it can be indicated by the dual-band brightness temperature difference ( minus ) below a threshold (Cermak and Bendix, 2008). In this study, the threshold is determined to be −2 K following the dynamic threshold algorithm proposed by Di Vittorio et al. (2002). Daytime fog after 08:00 is not retrieved because we mainly focus on the formation and development stage of fog before 08:00.
2.2.2 Fog propagation speed calculation
We calculate the propagation speed according to satellite-retrieved fog area. At 22:00 on 20 January 2020, a tiny fog area appeared at the Nantong and Yancheng coastal region with an area smaller than 50 km2 (figure not shown). The center of this fog area is set as point A (32.9∘ N, 120.6∘ E). We draw a line starting from A with an arbitrary direction and find its intersection with the fog boundary area at 07:00 the next day (point B). Then the propagation speed in this direction can be calculated by the distance from A to B divided by 9 h (22:00–07:00). By looping from 0 to 360 with the interval of 1∘, propagation speeds in all directions are calculated, and the maximum speed is defined as the fog propagation speed.
The fog propagation speed is verified by AWS data. We select three representative stations along the fog propagation direction: Dafeng (DF; 33.20∘ N, 120.48∘ E; 14 m), Baoying (BY; 33.23∘ N, 119.30∘ E; 15 m) and Sihong (SH; 33.48∘ N, 118.22∘ E; 13 m) (Fig. 1). According to their distances and the time differences when visibility drops to 200 m, the propagation speed between two adjacent stations is calculated.
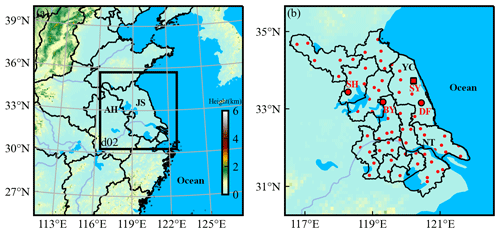
Figure 1The parent and nest model domain. The shaded color represents terrain height. The red points are automatic weather stations in Jiangsu, China. The three larger circle points are Sihong (SH), Baoying (BY), and Dafeng (DF) stations, and the square point is Sheyang (SY) sounding station. The black labels are some province or city names. (JS: Jiangsu Province; AH: Anhui Province; YC: Yancheng; NT: Nantong).
2.2.3 Process analysis on fog
The simulated fog is indicated by fog liquid water content (LWC). Process analysis is used to quantify the contribution of each physical process to LWC variation (Schwenkel and Maronga, 2019; Yan et al., 2020). The variation of LWC is related to the following terms:
where Advc includes horizontal and vertical advection, Vmix is associated with the fog droplet vertical exchange by turbulent mixing, Cond is the vapor condensation (negative means droplets evaporation) and Sedi is fog droplets sedimentation. Other microphysical processes include autoconversion, accretion and cold phase processes. They are much smaller than the previous four processes, so they can be safely ignored.
2.3 Model configuration and experiments
The Weather Research and Forecasting model (WRF) is implemented to study the fast spatial propagation of fog events. Two domains are set up (Fig. 1). The parent domain covers East China, with the grid size of 181 × 181 and grid interval of 9 km. The nested domain covers Jiangsu Province and its coastal area, with the grid size of 199 × 199 and grid interval of 3 km. To simulate the turbulent process more reasonably, the vertical levels are refined to 42 levels, with 25 levels under 1500 m and 9 levels under 100 m (Yang et al., 2019; Yan et al., 2020). The first model level is about 4 m. The model is driven by the initial and boundary field from the ERA5 reanalysis. The simulation starts at 08:00 on 19 January and ends at 08:00 on 21 January 2020, with the first 24 h as a spin-up period. All the times in this study are in local time (UTC + 8).
Fog is hard to simulate or predict well (Zhou et al., 2010, 2012) and is sensitive to the choice of parameterization schemes (Steeneveld et al., 2014; van der Velde et al., 2010). Through massive tests, the QNSE boundary layer scheme (Sukoriansky et al., 2005) and Pleim-Xiu land surface scheme (Pleim and Gilliam, 2009) yield the best simulation performance. Other parameterization schemes are listed in Table 1. The simulated fog is indicated by the liquid water content (LWC) greater than 0.015 g kg−1 under the height of 500 m, which corresponds to a horizontal visibility less than 1 km (Kunkel, 1983).
Apart from the base experiment, three sensitive experiments are performed to elucidate the mechanism of fast fog propagation (Table 1). The experiment “Tadv0” turns off the temperature advection within the PBL during the fog period. The experiments “QvAdv0” and “QcAdv0” are the same as “Tadv0” except for turning off water vapor advection and cloud water advection, respectively. The experiment “NoAdv” turns off all the advections above. Therefore, the differences of the base experiment with Tadv0, QvAdv0 and Qcadv0 represent the effect of temperature advection, moisture advection and cloud water advection, respectively. The reasons and results of the sensitive experiments are discussed in Sect. 3.5.
3.1 Fog overview and synoptic background
The studied fog event occurs during the night of 20 January and dissipates in the daytime of 21 January 2020 (Fig. 2). Figure 3 shows the synoptic situations at 08:00 and 20:00 on 20 January. At 500 hPa, a frontal zone is located north of 38∘ N. The Jiangsu area is dominated by prevailing westerly flows with no obvious troughs. At 850 hPa, a ridge moves eastward and controls the Jiangsu area. The descending motions associated with the ridge can favor the establishment of inversions. The inversions are also favored by the nocturnal radiative cooling at the ground. At ground level, a weak, cold high pressure moves eastward with the central pressure of 1030 hPa. The Jiangsu area is dominated by a uniform pressure field with small wind speeds, which strengthens atmospheric stratification stability and promotes the accumulation of aerosols and moisture. The moisture condition in Jiangsu is additionally favored by the water vapor transportation from the ocean by easterly winds at 20:00. Under this conductive situation, the fog event occurred from the nighttime of 20 January to the daytime of 21 January over Jiangsu Province (Fig. 2).
3.2 Fog and ground meteorology variation
The hourly Himawari 8 satellite image clearly shows the spatial propagation of fog (Fig. 2). The fog begins at 22:00 on 20 January in the Nantong and Yancheng coastal region with an area smaller than 50 km2. Later, this small fog area expands to a large-scale fog. Specifically, the southeast side of the fog area varies relatively little, but the northwest side expands remarkably, indicating a large propagation speed. At 07:00 on 21 January, the front of fog expands to Anhui Province. After 07:00, the fog begins to dissipate, and it fully disappears at 11:00 (figure not shown). Figure 4 quantitatively describes the propagation direction and speed of fog. From the east to south directions (the fourth quadrant), the fog propagation speed is less than 3 m s−1. In the west-northwest and west directions, the fog propagation speed is larger than 6 m s−1, and the maximum propagation speed is 9.6 m s−1, occurring at the 160∘ direction (in the Cartesian coordinate system). The fast propagation of fog was also reported previously in the Jiangsu area (Gao et al., 2023; Zhu et al., 2022), where the fog propagates from the coastal area to the west boundary of Jiangsu within about 10 h.
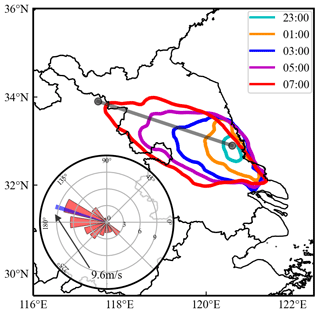
Figure 4The colored curves represent the fog boundaries (satellite retrievals) from 23:00 on 20 January to 07:00 the next day every 2 h. Fog boundaries from small to large represent 23:00, 01:00, 03:00, 05:00 and 07:00, respectively. The gray straight line indicates the fog propagation direction, and the vertical features of meteorologies at this line are analyzed in Figs. 7, 8 and 9. The lower-left polar plot is the fog propagation speed at 16 directions (22.5∘ interval), and the narrow blue bar highlights the maximum propagation speed (9.6 m s−1) occurring at the 160∘ direction (in the Cartesian coordinate system; from southeast to northwest).
Visibilities at three representative stations – Dafeng (DF), Baoying (BY) and Sihong (SH) – are used to verify the fog propagation speed calculated by satellite (Table 2; Fig. 5). At DF, fog forms (visibility less than 1 km) early at 19:45 on 20 January. The visibility drops sharply at 23:15 and reaches the minimum at about 00:15. At BY and SH, fog forms in turn, and their visibilities also have a burst decreasing feature at 03:40 and 07:00, respectively. We calculate the fog propagation speed by the distances among stations and the time differences when visibility drops to 200 m. The propagation speed is 7.6 m s−1 between DF and BY and 8.3 m s−1 between BY and SH. These values correspond to the speed calculated by satellite observation.
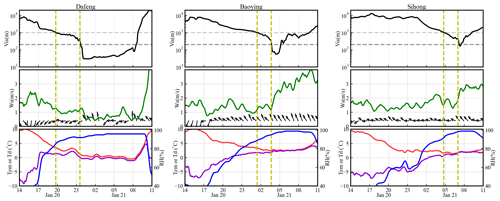
Figure 5The temporal variation of ground visibility (Vis; black line), wind speed (Ws; green line), wind direction (vectors), temperature (Tem; red line), dew point (Td; violet line), and relative humidity (RH; blue line) at Dafeng, Baoying and Sihong stations. The horizontal dashed lines are visibilities of 1000 and 200 m. The vertical dashed lines mark the times of fog formation and visibility burst dropping.
Table 2The times when visibility reaches 1000, 500 and 200 m at three representative stations. (DF: Dafeng; BY: Baoying; SH: Sihong).
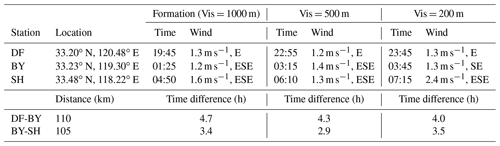
Figure 5 shows the variation of other meteorological fields. We focus on the characteristics from fog formation to the burst visibility dropping (indicated by yellow dashed lines). At DF, the northerly wind decreases to lower than 1.5 m s−1 at fog formation, which causes the weak cold advection and decreasing temperature. The temperature keeps decreasing and favors the burst reduction of visibility at 23:15. The vapor content (indicated by dew point) increases sharply before 17:00 and decreases slightly since then, so the increasing RH after fog formation is caused by the temperature drop. At BY and SH, the wind directions are dominantly southeast, and the speeds are generally less than 2 m s−1 before fog formation. The temperature keeps decreasing and vapor content keeps increasing, leading to the further reduction of visibility. Later, the southeasterly winds are obviously enhanced by about 1 m s−1, which may contribute to the burst visibility dropping due to the intensified vapor advection from the ocean.
The preliminary cause of fog formation and intensification are summarized. As it is located near the ocean, the moisture at DF reaches the maximum prior to fog formation, so the fog formation and intensification are largely caused by radiative cooling and weak cold advection. At BY and SH, the temperature cooling rate is weaker than DF, which is partly due to the weak warm advection by southeasterly winds. The vapor advection by southeasterly winds favors fog development, and the burst decrease in visibility coincides with the increase in wind speed. Therefore, deduced from BY and SH, the vapor transportation associated with southeasterly winds could be an important reason for northwesterly propagation of fog. However, it is obvious that the ground wind speed is rather small compared with fog propagation speed. Statistics on AWSs show that although wind direction (east, southeast and south winds at 70 % of stations) is generally in accordance with fog propagation direction, wind speed is lower than 3 m s−1 at 97 % of stations from 22:00 to 07:00, which is about one-third of the fog propagation speed. Therefore, the ground meteorological field is insufficient to explain the fast propagation of fog. The fog PBL characteristics and the key influencing factors need to be investigated by numerical simulations.
3.3 Model evaluation
Figure 6a evaluates the model performance on temperature, relative humidity (RH) and wind field at the surface. The simulated temperature and RH agree well with observations, with the root mean square error (RMSE) of 1.0 K and 11 %, respectively. The simulation reasonably captures the wind direction transition from north to east, and the RMSE is less than 1 m s−1.
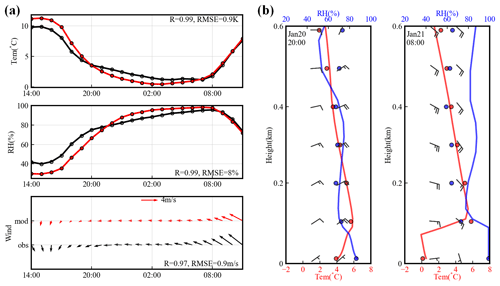
Figure 6(a) The model performance on 2 m temperature (Tem), 2 m relative humidity (RH), and 10 m wind speed and direction. The red color represents the simulation, and the black color represents the observation. The time is from 14:00 on 20 January 2020 to 11:00 the next day. (b) The model performance on temperature (red), RH (blue) and wind (barbs) profiles at Sheyang sounding station. For temperature and RH, the observations are represented by scatters, and simulations are represented by solid lines. For wind barbs, the left column is observations, and the right column is simulations. The scatters and barbs are interpolated onto the heights of 0, 100, 200, 300, 400, 500 and 600 m.
Figure 6b evaluates the model performance on temperature, RH and wind field in the vertical direction at the SY sounding station. The temperature profile is simulated well by the model, with a mean bias of less than 1 K. The RH bias is relatively small below about 200 m, while it is a bit larger above 200 m at 08:00 on 21 January. The simulated wind speed and direction are basically consistent with the observation. The large winds (greater than 6 m s−1) at about 200 m are well reproduced by the model, indicating that the model reasonably simulates the boundary layer low-level jet. Studies on the boundary layer low-level jet are presented in the following sections.
Figure 2 compares the satellite-observed and simulated fog area. The simulation is only evaluated before 07:00, because the dissipation of fog after 08:00 is not the focus in this study. The model reasonably captures the spatiotemporal evolution of fog, with a slight overestimation of 5 %–10 % in the fog area.
Overall, the simulation reasonably captures the temporal variation of meteorology and reproduces the spatial propagation of fog. It establishes the basis for discerning the mechanism of fog propagation.
3.4 Characteristics of fog and PBL structure
The thermodynamic variation of PBL is crucial for understanding the propagation of fog. Figure 7a shows the temporal variation of horizontal winds in vertical directions. The simulated wind speed is consistently smaller than 4 m s−1 under about 30 m, while it remarkably increases with height. At 18:00 on 20 January, a large wind speed zone (> 6 m s−1) forms at a height between 50 and 500 m to the east of 120∘ E. Since then, the large wind zone moves westward quickly, accompanied by increasing wind speed. During the fog period, the average wind speed exceeds 6 m s−1 at a height between 50 and 500 m (Fig. 7b), which is commonly larger than the wind speed in most fog events. Here, we refer to this large wind speed zone as the boundary layer low-level jet (BLLJ). The existence of the BLLJ is supported by ERA5 reanalysis on 1000 and 975 hPa levels (Fig. 7b).
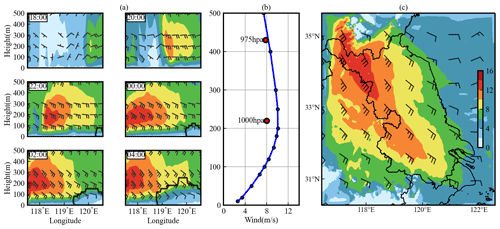
Figure 7(a) The height–longitude variation of horizontal wind direction (vectors) and wind speed (shaded colors) at the crossing line in Fig. 4. The lower-right black polygons represent the fog area. The times are from 18:00 on 20 January to 04:00 the next day. (b) The averaged wind speed profile at the crossing line during 23:00–07:00. The two red points are the wind speed calculated from the ERA5 reanalysis. (c) The averaged wind direction (vectors) and wind speed (shaded colors) at 1000 hPa during 23:00–07:00.
The formation of the BLLJ is likely caused by the easterly movement of a high pressure at 1000 hPa over East China. The central pressure gets enhanced, which strengthens the pressure gradient over the Jiangsu area and favors increasing wind speed (figure not shown). The jet core (maximum wind speed) occurs at about 1000 hPa (200 m), with a time-averaged speed of 10 m s−1 (Fig. 7b). At that level, the dominant wind direction is southeast and the wind speed over the fog area is 8–16 m s−1 (Fig. 7c), which can fit the propagation direction and speed of fog. Also, the expansion speed of the vertical fog zone is comparable to the movement speed of the jet core (Fig. 7a). Therefore, we hypothesize that the southeasterly BLLJ could account for the fast propagation of fog.
Previous studies reveal that the southerly BLLJ can transport abundant water vapor to China inland and thus promote fog formation (Liu et al., 2016; Tian et al., 2019). Figure 8 shows the temporal variation of water vapor mixing ratio (Qv) profiles. Since the vapor content over the ocean is higher, it is transported to inland areas by the southeasterly BLLJ. The BLLJ can further increase the Qv in the PBL by wind speed horizontal convergency and vertical shear. The larger wind speed in the BLLJ zone and lower wind speed outside the BLLJ zone cause wind speed convergence, which favors the increase in PBL moisture. Additionally, the turbulence generated by vertical shear of wind speed can promote vapor turbulent mixing, leading to the higher Qv above the surface being entrained downward and increasing the ground Qv (Gao et al., 2007). The Qv under 300 m is generally higher than 3 g kg−1 under the effect of the BLLJ. Wu et al. (2020) also found that the BLLJ continuously transports water vapor to the fog layer, resulting in a surface Qv higher than 3 g kg−1. It is notable that the expansion of the vertical fog area coincides with the movement of the zone of Qv > 4 g kg−1. Therefore, moisture advection by the BLLJ could be an important reason for fast fog propagation.
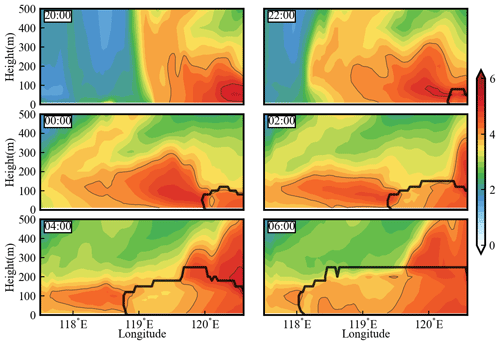
Figure 8The height–longitude distribution of the water vapor mixing ratio (g kg−1) at the crossing line in Fig. 4. The deep black polygons represent the fog area. The light black lines are the region of water vapor mixing ratio larger than 4 g kg−1. The times are from 20:00 on 20 January to 06:00 the next day.
The BLLJ has previously been reported to result in warm advection and to deepen the inversion layer (Tian et al., 2019), and the inversion layer is an important reason for fog burst reinforcement in most fog cases (e.g., Li et al., 2019; Liu et al., 2012; Jiao et al., 2016). Figure 9 shows the temporal variation of the temperature profile and inversion layer. The inversion layer here refers to the height above ground where temperature monotonically decreases with height. Since 20:00 on 20 January, the ground temperature keeps decreasing due to radiative cooling. Within the fog area, the temperature drop is more significant, which is due to the longwave radiative cooling by fog droplets (Bott, 1991; Jia et al., 2018). Approximately above the fog top, there is an obvious warm air mass transported from the ocean to inland areas. The BLLJ-induced warm advection increases the vertical temperature gradient and strengthens atmospheric stability. Accordingly, the inversion height over non-fog areas basically keeps increasing. The approximate inversion layer height is about 100–300 m, which is consistent with previous studies (Dorman et al., 2021; Li et al., 2019). The maximum inversion intensity is 15 K/100 m, which is also reported in a dense fog event (16 K/100 m) by Pu et al. (2008). It favors the accumulation of vapor and condensation nuclei, which is also a possible reason for fog formation in the downstream area.
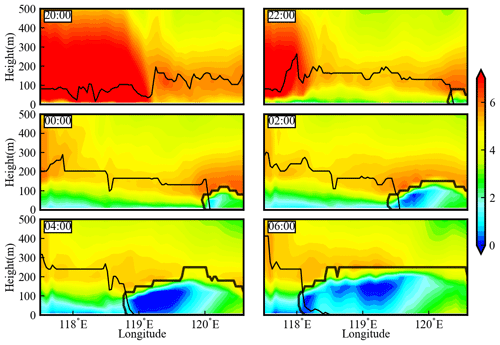
Figure 9Same as the previous figure but for the temperature. The bold black polygons represent the fog area. The thin black lines are the top of inversion layer.
Additionally seen from Fig. 9, the west boundary of the vertical fog region below about 100 m has a negative slope; i.e., fog forms at the upper level ahead of forming at the ground level. The upper-level fog with no ground contact is referred to as low stratus. The height at which fog/low stratus firstly forms is shown in Fig. 10. An initial fog area forms at ground level before 00:00 on 21 January. Since then, low stratus forms at the upper level (about 10–66 m) over the downstream area, while the ground fog in the downstream area forms about 0–20 min later than low stratus. The formation of low stratus may also be caused by the BLLJ-induced moisture advection. In addition, the cloud water advection (Sect. 2.2.3) to the downstream area by the BLLJ could also be a potential reason. We hypothesize that the formation of ground fog is partly favored by the stratus lowering, which has been reported by previous studies (e.g., Haeffelin et al., 2010; Liu et al., 2012); the base height of stratus can be smaller than 100 m before fog formation (Dupont et al., 2012; Fathalli et al., 2022), which is basically close to our results (10–66 m in Fig. 10), while in this event the stratus-lowering phenomenon remains to be verified by additional high-spatiotemporal-resolution vertical observations.
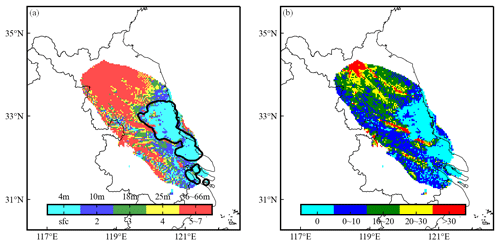
Figure 10(a) The height (shaded color) at which fog/low stratus firstly forms. The black contours are the ground fog areas at 00:00 on 21 January 2020. The color bar represents the model level and the corresponding height above the surface. For example, the cyan colors indicate that fog firstly forms at the surface level with the corresponding height of about 4 m. The red colors indicate that low stratus firstly forms at the 5th to 7th model level with the corresponding height of about 36–66 m. (b) The time differences between ground fog formation and low-stratus formation. For example, the cyan colors indicate that fog firstly forms at ground. The blue colors indicate that the ground fog forms 0–10 min later than the low-stratus formation.
According to the above results, three potential factors for fog propagation are raised: the BLLJ-related temperature advection, moisture advection and cloud water advection. These advections possibly promote low-stratus formation within 100 m above the surface, and subsequently the low stratus could subside to become ground fog by the turbulent mixing or sedimentation of cloud droplets. Currently, their contributions to fog propagation have not been quantitatively revealed. Therefore, it will be addressed in the next section.
3.5 Quantitative reasons for fast fog propagation
Four sensitive experiments – Tadv0, QvAdv0, QcAdv0 and NoAdv0 (Sect. 2.3) – are conducted to quantify the respective contributions of temperature advection, moisture advection, cloud water advection and all these advections to fog propagation (Fig. 11). Under the condition with no advections (Fig. 11a–d), there is an 80 % decrease in fog area and a 6.4 m s−1 (66 %) decrease in propagation speed, which highlights the role of the BLLJ-related advections. When turning off temperature advection (Tadv0) (Fig. 11e–h), the original fog area in the base experiment shrinks 50 % in size and breaks into separate fog patches, and the propagation speed decreases by about 5.2 m s−1 (54 %). When turning off moisture advection (QvAdv0) (Fig. 11i–l), the fog area shrinks by 62 % in size, and the propagation speed decreases by about 4.6 m s−1 (48 %). When turning off cloud water advection (QcAdv0) (Fig. 11m–p), the fog area remains nearly unchanged during 00:00–04:00 and decreases moderately in size (about 25 %) at 06:00. The propagation speed decreases moderately by 2.4 m s−1 (25 %). Deduced from the changes in fog area and propagation speed under various experiments, we can infer that the BLLJ-related warm and moisture advection, especially moisture advection, could be the major cause of fast spatial propagation, while cloud water advection has a minor contribution.
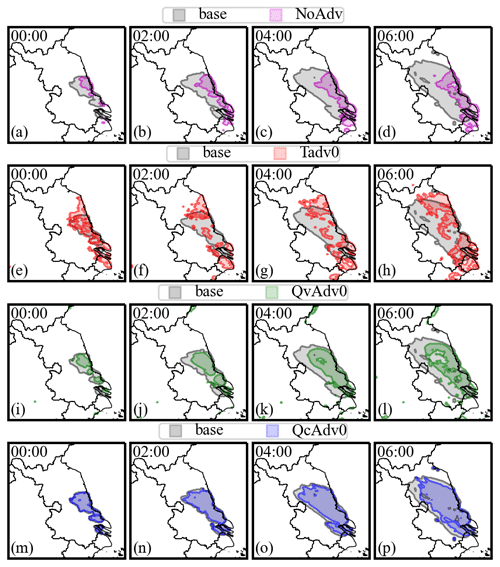
Figure 11The temporal variation of ground fog area under different experiments from 00:00 to 06:00 on 21 January. The black color represents the base experiment. Tadv0 (red), QvAdv0 (green) and QcAdv0 (blue) are the experiments turning off temperature advection, moisture advection and cloud water advection, respectively. NoAdv (pink) is the experiment turning off all of the above advections.
We further perform process analysis on LWC (Sect. 2.2.3) to illustrate the mechanism of fog propagation (Fig. 12). The horizontal and vertical values of Advc and Sedi are at least 1 order of magnitude smaller than that of Cond and Sedi, indicating that cloud water transportation to downstream areas and droplet sedimentation to ground are not the causes of fog propagation. At 00:00 on the ground level, Cond is positive over the newly formed fog area (blue and cyan colors surrounding the fog area), indicating that fog firstly forms at the ground level by radiative cooling before 00:00. After 02:00, Cond is almost negative over the entire fog area, indicating that fog does not firstly form at the ground level (otherwise Cond would have positive values). The formation of ground fog could be caused by the turbulent entrainment of LWC from the upper level to the ground level, which is supported by the significant positive values of Vmix after 02:00. In the vertical direction, Vmix and Cond are still two dominant physical processes (Fig. 12b), and their signs show opposite patterns. At the lower level (0–30 m), Cond is negative and Vmix is positive, which is the same as their ground characteristics. At the upper level (30–200 m), Cond is positive and Vmix is negative instead, indicating that cloud water is produced by vapor condensation at the upper level and is then entrained to ground. The significant positive Cond supports the fact that the BLLJ-related moisture advection promotes vapor condensation and low-stratus formation above the surface, and the significant positive Vmix may indicate that the low stratus favors ground fog formation by turbulent exchange of LWC.
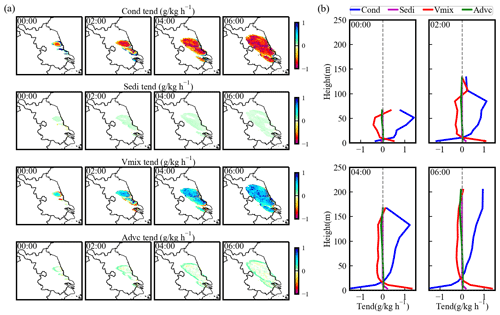
Figure 12(a) The spatial distribution of the four process tendencies contributing to LWC variation at ground level. (b) The vertical profiles of the process tendencies averaged in the fog area. The times are from 00:00 to 06:00 on 21 January (Cond: condensation or evaporation; Sedi: sedimentation; Vmix: turbulent exchange; Advc: horizontal and vertical advection).
Previous studies have elucidated the qualitative reasons for fog propagation. In this study, we describe the features of fast fog propagation and identify its key impact factors more quantitatively. Figure 13 summarizes the mechanism of fog propagation. During the nighttime, a southerly BLLJ controls the study region, and the jet core intensity is about 10 m s−1, which occurs at about 200 m. The ground fog propagates northwestward with a speed of 9.6 m s−1. The BLLJ favors the fast fog propagation by three possible mechanisms: (1) the BLLJ transports sufficient vapor from the ocean to inland area. The turbulence strengthened by wind speed shear further moistens the PBL and promotes vapor condensation. This could be the dominant mechanism. (2) The BLLJ transports warmer air from the ocean to inland area and deepens the inversion layer. The strengthened inversion favors the accumulation of vapor and condensation nuclei. (3) The strong moisture advection could promote the low-stratus formation in the downstream area, and later it subsides to become ground fog by turbulent exchange of cloud droplets. The stratus-lowering phenomenon needs to be verified by additional observations.
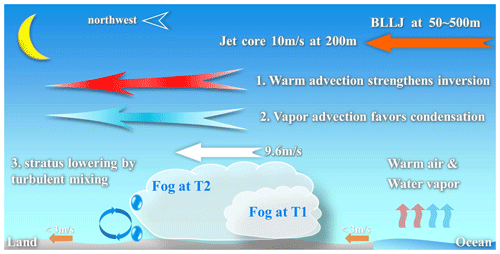
Figure 13The concept diagram of fog propagation. The ground wind speed (short orange arrows) is generally less than 3 m s−1. A southeasterly BLLJ exists at the height from 50 to 500 m, and the jet core intensity is 10 m s−1 at 200 m (the long orange arrow). The updraft arrows represent the warm and wet air from the ocean. The two cloud shapes are fog areas at two adjacent times, and the white arrow indicates the fog propagation speed (9.6 m s−1). The fog propagation is probably caused by three approaches: (1) moisture advection from the ocean promotes vapor condensation in the downstream area, which could be the dominant cause (the fancy blue arrow); (2) warm advection from the ocean deepens the inversion layer and additionally promotes vapor accumulation within the PBL (the fancy red arrow); (3) the moisture advection probably results in the low-stratus formation, and later it subsides to ground by turbulent mixing of cloud droplets (the blue water drops and circular arrows). Note that warm and moisture advections occur at nearly all heights below 500 m, not merely at the height indicated by arrows.
The results could facilitate the understanding of cloud formation and development. Clouds, such as convective clouds, can develop and expand extraordinarily fast under strong synoptic forcing or unstable conditions. Fog can be viewed as a kind of near-surface stratus cloud, which usually forms under stable conditions with weak synoptic forcings. However, as revealed in this study, it can also develop and propagate fast under the effect of the BLLJ. The quantitative relations between the BLLJ and fast fog propagation may have implications on the cloud formation and development mechanism under stable synoptic conditions.
Previous studies have found that the spatial propagation of fog could be rather fast under favorable conditions, and the boundary layer low-level jet (BLLJ) could be a potential reason. In this study, we analyze the fast spatial propagation feature of a large-scale fog event in Jiangsu Province, China, by high-spatiotemporal-resolution ground and satellite observations. The key impact factors and mechanisms of the BLLJ effect on fast spatial propagation are quantitatively revealed by WRF model simulations. Results show the following.
The fog begins at 22:00 on 20 January 2020 over the Jiangsu coastal area, and it reaches the west boundary of Jiangsu at 07:00 the next day. Satellite retrievals show that the southeast side of the fog area varies slightly, but the northwest side expands fast, with a maximum propagation speed of 9.6 m s−1. During the fog period, the ground wind direction is consistent with fog propagation, which favors the vapor transportation from the ocean and promotes fog formation. However, the wind speed (< 3 m s−1) is at least one-third less than the fog propagation speed. Therefore, the ground meteorologies are insufficient to explain the fast propagation of fog. The influencing factors and mechanisms need to be investigated by exploring the PBL characteristics through numerical simulations.
The WRF model simulates the temporal variation of meteorologies well and reproduces the spatiotemporal evolution of the fog area. A BLLJ (> 6 m s−1) exists at a height between 50 and 500 m. The jet core occurs at 1000 hPa (200 m) with the southeasterly winds of 10 m s−1, which can fit the propagation direction and speed of fog. Therefore, the southeasterly BLLJ is hypothesized to be the cause of fast propagation. The BLLJ creates favorable PBL conditions by transporting moisture and warm air from the ocean. The moisture advection and the vapor turbulent mixing generated by wind speed shear increase the humidity within the PBL, and the propagation of the fog area coincides with the movement of the high-humidity zone (vapor mixing ratio > 4 g kg−1). The warm advection from the ocean deepens the inversion layer and additionally favors the accumulation of moisture and condensation nuclei. Additionally, it is found that low stratus could form above the surface and subsides to become ground fog within 0–20 min. The moisture advection is also responsible for the formation of low stratus.
Sensitive experiments quantitatively reveal the contributions of moisture advection and temperature advection to fog propagation. When moisture (temperature) advection is turned off, the fog area decreases by 62 % (50 %) and the propagation speed decreases by about 4.6 m s−1 (5.2 m s−1). Process analysis on fog liquid water content (LWC) further illustrates the mechanism of fog propagation. Condensation (Cond) and LWC turbulent exchange (Vmix) are two important physical processes. At the upper level (30–200 m), Cond is positive and Vmix is negative. It indicates that the BLLJ-related moisture advection significantly promotes condensation and probably favors low-stratus formation. At the ground and lower level (0–30 m), Cond is basically negative and Vmix is positive. It indicates that cloud droplets at the upper level are entrained downward by turbulent mixing, leading to the subsequent formation of ground fog. The stratus-lowering phenomenon needs to be verified by additional observations.
In this study, through the combination of observations and simulations, we have revealed the effect of the southeasterly BLLJ on fog propagation and quantified the contributions of the BLLJ-related moisture advection and temperature advection to fog propagation. Three possible mechanisms are revealed: (1) moisture advection from the ocean promotes vapor condensation in the downstream area, which could be the dominant cause; (2) warm advection from the ocean deepens the inversion layer and additionally promotes vapor accumulation within the PBL; (3) the moisture advection probably promotes low-stratus formation first, and later it subsides to become ground fog by turbulent mixing of cloud droplets. The coexistence of fast fog propagation and the BLLJ is not a common phenomenon, so finding more cases requires additional work. It should be addressed in future studies in order to deeply understand the relationships between fog propagation and the BLLJ under different regions and synoptic conditions. Their quantitative relationships could facilitate the understanding of cloud formation and development under stable synoptic conditions, since fog can be viewed as a near-surface stratus cloud that can potentially propagate fast under stable conditions.
The ground automatic weather station data and Sheyang sounding data in Jiangsu Province are from http://www.nmic.cn/dataService/cdcindex/datacode/A.0012.0001/show_value/normal.html (China Meteorological Data Service Centre, 2005). The ERA5 reanalysis data are from https://doi.org/10.24381/cds.bd0915c6 (Hersbach et al., 2018). The geostationary satellite Himawari 8 data are from https://www.eorc.jaxa.jp/ptree/ (Bessho et al., 2016). The WRF source code is from https://www2.mmm.ucar.edu/wrf/users/download/get_source.html (Skamarock et al., 2008). The other data, model outputs and codes can be accessed by contacting Duanyang Liu at liuduanyang2001@126.com.
SY performed the model simulation, data analysis and manuscript writing. HW and DL proposed the idea, supervised this work and revised the manuscript. XL helped the revision of the manuscript. FZ provided and analyzed the observation data.
The contact author has declared that none of the authors has any competing interests.
Publisher’s note: Copernicus Publications remains neutral with regard to jurisdictional claims made in the text, published maps, institutional affiliations, or any other geographical representation in this paper. While Copernicus Publications makes every effort to include appropriate place names, the final responsibility lies with the authors.
We thank the two anonymous reviewers and Binbin Zhou for their constructive comments and suggestions.
This research has been supported by the Special Project of Innovative Development of CMA (grant no. CXFZ2023J022), the Open Research Foundation of Jiangsu Marine Meteorology (HYQX2022), the Beijige Foundation (grant no. BJG202307), the Research Foundation of Jiangsu Meteorology Bureau (grant no. KM202307), and the Basic Research Fund of CAMS (grant no. 2022Y025).
This paper was edited by Paulo Ceppi and reviewed by two anonymous referees.
Bessho, K., Date, K., Masahiro, H., Ikeda, A., Imai, T., Inoue, H., Kumagai, Y., Miyakawa, T., Murata, H., Ohno, T., Okuyama, A., Oyama, R., Sasaki, Y., Shimazu, Y., Shimoji, K., Sumida, Y., Suzuki, M., Taniguchi, H., Tsuchiyama, H., Uesawa, D., Yokota, H., and Yoshida, R.: An Introduction to Himawari-8/9 – Japan's New-Generation Geostationary Meteorological Satellites, J. Meteorol. Soc. Jpn., 94, 151–183, https://doi.org/10.2151/jmsj.2016-009, 2016 (data available at: https://www.eorc.jaxa.jp/ptree/.
Bott, A.: On the influence of the physico-chemical properties of aerosols on the life cycle of radiation fogs, J. Aerosol Sci., 21, 1–31, https://doi.org/10.1007/BF00119960, 1991.
Boutle, I., Price, J., Kudzotsa, I., Kokkola, H., and Romakkaniemi, S.: Aerosol–fog interaction and the transition to well-mixed radiation fog, Atmos. Chem. Phys., 18, 7827–7840, https://doi.org/10.5194/acp-18-7827-2018, 2018.
Cermak, J. and Bendix, J.: A novel approach to fog/low stratus detection using Meteosat 8 data, Atmos. Res., 87, 279–292, https://doi.org/10.1016/j.atmosres.2007.11.009, 2008.
China Meteorological Data Service Centre: National Ground Meteorology Observation Data, China National Meteorological Information Centre [data set], http://www.nmic.cn/dataService/cdcindex/datacode/A.0012.0001/show_value/normal.html (last access: 6 November 2023), 2005.
Dhangar, N. G., Lal, D. M., Ghude, S. D., Kulkarni, R., and Rajeevan, M.: On the conditions for onset and development of fog over new delhi: an observational study from the wifex, Pure Appl. Geophys., 673, 1–20, https://doi.org/10.1007/s00024-021-02800-4, 2021.
Dorman, C. E., Hoch, S. W., Gultepe, I., Wang, Q., Yamaguchi, R., Fernando, H., and Krishnamurthy, R.: Large-Scale Synoptic Systems and Fog During the C-FOG Field Experiment, Bound.-Lay. Meteorol., 181, 171–202, https://doi.org/10.1007/s10546-021-00641-1, 2021.
Dupont, J., Haeffelin, M., Protat, A., Bouniol, D., Boyouk, N., and Morille, Y.: Stratus–Fog Formation and Dissipation: A 6-Day Case Study, Bound.-Lay. Meteorol., 143, 207–225, https://doi.org/10.1007/s10546-012-9699-4, 2012.
Di Vittorio, A. V. and Emery, W. J.: An automated, dynamic threshold cloud-masking algorithm for daytime AVHRR images over land, IEEE T. Geosci., 40, 1682–1694, https://doi.org/10.1109/TGRS.2002.802455, 2002.
Fathalli, M., Lac, C., Burneta, F., and Vié, B.: Fog due to stratus lowering: Experimental and modelling case study, Q. J. Roy. Meteor. Soc., 148, 2299–2324, https://doi.org/10.1002/qj.4304, 2022.
Gao, S., Lin, H., Shen, B., and Fu, G.: A heavy sea fog event over the Yellow Sea in March 2005: Analysis and numerical modeling, Adv. Atmos. Sci., 24, 65–81, https://doi.org/10.1007/s00376-007-0065-2, 2007.
Gao, Y., Liu, D., Yan, S., Zhou, W., Wang, H., Zu, F., Mei, Q., Yi, C., and Sheng, Y.: Influence of sea-land breeze on formation and dissipation of severe dense fog and its explosive enhancement in the Yellow Sea Coastal Area, Sci. China Earth Sci., accepted, 2023.
Gultepe, I., Tardif, R., Michaelides, S. C., Cermak, J., Bott, A., Bendix, J., Muller, M. D., Pagowski, M., Hansen, B., Ellrod, G., Jacobs, W., Toth, G., and Cober, S. G.: Fog research: a review of past achievements and future perspectives, Pure Appl. Geophys., 164, 1121–1159, https://doi.org/10.1007/s00024-007-0211-x, 2007.
Haeffelin, M., Bergot, T., Elias, T., Tardif, R., Carrer, D., Chazette, P., and Zhang, X.: PARISFOG: Shedding new light on fog physical processes, B. Am. Meteorol. Soc., 91, 767–783, https://doi.org/10.1175/2009bams2671.1, 2010.
Hersbach, H., Bell, B., Berrisford, P., Biavati, G., Horányi, A., Muñoz Sabater, J., Nicolas, J., Peubey, C., Radu, R., Rozum, I., Schepers, D., Simmons, A., Soci, C., Dee, D., and Thépaut, J.-N.: ERA5 hourly data on pres-sure levels from 1940 to present, Copernicus Climate Change Service (C3S) Climate Data Store (CDS) 3 [data set], https://doi.org/10.24381/cds.bd0915c6, 2018.
Jia, X., Quan, J., Zheng, Z., Liu, X., Liu, Q., He, H., and Liu, Y.: Impacts of anthropogenic aerosols on fog in North China Plain, J. Geophys. Res.-Atmos., 124, 252–265, https://doi.org/10.1029/2018jd029437, 2018.
Jiao, S., Zhu, C., Zhu, Y., Yuan, C., Zu, F., and Sun, K.: A discussion on the reason for a rare persistent heavy fog event in Jiangsu Province, Acta Meteorol. Sin., 74, 200–212, https://doi.org/10.11676/qxxb2016.015, 2016.
Koračin, D., Dorman, C. E., Lewis, J. M., Hudson, J. G., Wilcox, E. M., and Torregrosa, A.: Marine fog: A review, Atmos. Res., 143, 142–175, https://doi.org/10.1016/j.atmosres.2013.12.012, 2014.
Korb, G. and Zdunkowski, W.: Distribution of radiative energy in ground fog, Tellus, 22, 298–320, https://doi.org/10.3402/tellusa.v22i3.10223, 1970.
Kraus, H., Malcher, J., and Schaller, E.: A nocturnal low level jet during PUKK, Bound.-Lay. Meteorol., 31, 187–195, https://doi.org/10.1007/BF00121177, 1985.
Kunkel, B. A.: Parameterization of Droplet Terminal Velocity and Extinction Coefficient in Fog Models, J. Appl. Meteorol, 23, 34–41, https://doi.org/10.1175/1520-0450(1984)023<0034:PODTVA>2.0.CO;2, 1983.
Li, P. and Fu, G.: The Formation Mechanism of a Spring Sea Fog Event over the Yellow Sea Associated with a Low-Level Jet, Weather Forecast., 27, 1538–1553, https://doi.org/10.1175/WAF-D-11-00152.1, 2012.
Li, Z., Liu, D., Yan, W., Wang, H., Zhu, C., Zhu, Y., and Zu, F.: Dense fog burst reinforcement over Eastern China: A review, Atmos. Res., 230, 104639, https://doi.org/10.1016/j.atmosres.2019.104639, 2019.
Liu, D., Yang, J., Niu, S., and Li, Z.: On the Evolution and Structure of a Radiation Fog Event in Nanjing, Adv. Atmos. Sci., 28, 223–237, https://doi.org/10.1007/s00376-010-0017-0, 2011.
Liu, D., Yan, W., Yang, J., Pu, M., Niu, S., and Li, Z.: A Study of the Physical Processes of an Advection Fog Boundary Layer, Bound.-Lay. Meteorol., 158, 125–138, https://doi.org/10.1007/s10546-015-0076-y, 2016.
Liu, D., Li, Z., Yan, W., and Li, Y.:. Advances in fog microphysics research in China, Asia Pac. J. Atmos. Sci., 53, 131–148, https://doi.org/10.1007/s13143-016-0028-6, 2017.
Liu, D. Y., Niu, S. J., Yang, J., Zhao L., Lv, J., and Lu, C.: Summary of a 4-year fog field study in Northern Nanjing, part 1: fog boundary layer, Pure Appl. Geophys., 169, 809–819, https://doi.org/10.1007/s00024-011-0343-x, 2012.
Liu, Q., Wang, Z. Y., Wu, B. G., Liu, J. L., and Gultepe, I.: Microphysics of fog bursting in polluted urban air, Atmos. Environ., 10, 118357, https://doi.org/10.1016/j.atmosenv.2021.118357, 2021.
Nakanishi, M.: Large-eddy simulation of radiation fog, Bound.-Lay. Meteorol., 94, 461–493, https://doi.org/10.1023/A:1002490423389, 2000.
Pleim, J. E. and Gilliam, R.: An indirect data assimilation scheme for deep soil temperature in the Pleim-Xiu land surface model, J. Appl. Meteorol., 48, 1362–1376, https://doi.org/10.1175/2009JAMC2053.1, 2009.
Pu, M. J., Zhang, G. Z., Yan, W. L., and Li, Z. H.: Features of a rare advection-radiation fog event, Sci. China Earth Sci., 51, 1044–1052, https://doi.org/10.1007/s11430-008-0071-y, 2008.
Quan, J., Liu, Y., Jia, X., Liu, L., Dou, Y., Xin, J., and Seinfeld, J. H.: Anthropogenic aerosols prolong fog lifetime in China, Environ. Res. Lett., 16, 044048, https://doi.org/10.1088/1748-9326/abef32, 2021.
Schwenkel, J. and Maronga, B.: Large-eddy simulation of radiation fog with comprehensive two-moment bulk microphysics: impact of different aerosol activation and condensation parameterizations, Atmos. Chem. Phys., 19, 7165–7181, https://doi.org/10.5194/acp-19-7165-2019, 2019.
Shao, N., Lu, C., Jia, X., Wang, Y., Li, Y., Yin, Y., Zhu, B., Zhao, T., Liu, D., Niu, S., Fan, S., Yan, S., and Lv, J.: Radiation fog properties in two consecutive events under polluted and clean conditions in the Yangtze River Delta, China: a simulation study, Atmos. Chem. Phys., 23, 9873–9890, https://doi.org/10.5194/acp-23-9873-2023, 2023.
Shen, P., Liu, D., Gultep, I., Lin, H., Cai, N., and Cao, S.: Boundary layer features of one winter fog in the Yangtze River Delta, China, Pure Appl. Geophys., 179, 3463–3480, https://doi.org/10.1007/s00024-022-03119-4, 2022.
Skamarock, W. C., Klemp, J. B., Dudhia, J., Gill, D. O., Barker, D. M., Duda, M. G., Huang, X., Wang, W., and Powers, J. G.: A description of the advanced research WRF Version 3 (NCAR Technical Note NCAR/TN-475+STR), National Center for Atmospheric Research: Boulder, CO, USA, https://doi.org/10.5065/D68S4MVH, 2008 (data available at: https://www2.mmm.ucar.edu/wrf/users/download/get_source.html, last access: 6 November 2023).
Steeneveld, G. J., Ronda, R. J., and Holtslag, A. A. M.: The Challenge of Forecasting the Onset and Development of Radiation Fog Using Mesoscale Atmospheric Models, Bound.-Lay. Meteorol., 154, 265–289, https://doi.org/10.1007/s10546-014-9973-8, 2014.
Sukoriansky, S., Galperin, B., and Perov, V.: Application of a new spectral model of stratified turbulence to the atmospheric boundary layer over sea ice, Bound.-Lay. Meteorol., 117, 231–257, https://doi.org/10.1007/s10546-004-6848-4, 2005.
Tian, M., Wu, B., Huang, H., Zhang, H., Zhang, W., and Wang, Z.: Impact of water vapor transfer on a Circum-Bohai-Sea heavy fog Observation and numerical simulation, Atmos. Res., 229, 1–22, https://doi.org/10.1016/j.atmosres.2019.06.008, 2019.
van der Velde, I. R., Steeneveld, G. J., Wichers Schreur, B. G. J., and Holtslag, A. A. M.: Modeling and Forecasting the Onset and Duration of Severe Radiation Fog under Frost Conditions, Mon. Weather Rev., 138, 4237–4253, https://doi.org/10.1175/2010mwr3427.1, 2010.
Wang, H., Zhang, Z., Liu, D., Zhu, Y., Zhang, X., and Yuan, C.: Study on a Large-Scale Persistent Strong Dense Fog Event in Central and Eastern China, Adv. Meteorol., 4, 1–15, https://doi.org/10.1155/2020/8872334, 2020.
Wang, Y., Lu, C., Niu, S., Lv, J., Jia, X., Xu, X., Xue, Y., Zhu, L., and Yan, S.: Diverse dispersion effects and parameterization of relative dispersion in urban fog in eastern China, J. Geophys. Res.-Atmos., 128, e2022JD037514, https://doi.org/10.1029/2022JD037514, 2023.
Wobrock, W., Schell, D., Maser, R., Kessel, M., Jaeschke, W., Fuzzi, S., and Bendix, J.: Meteorological characteristics of the Po Valley fog, Tellus B, 44, 469–488, https://doi.org/10.3402/tellusb.v44i5.15562, 1992.
Wu, B., Li, Z., Ju, T., and Zhang, H.: Characteristics of Low-level jets during 2015–2016 and the effect on fog in Tianjin, Atmos. Res., 245, 105102, https://doi.org/10.1016/j.atmosres.2020.105102, 2020.
Yan, S., Zhu, B., Huang, Y., Zhu, J., Kang, H., Lu, C., and Zhu, T.: To what extents do urbanization and air pollution affect fog?, Atmos. Chem. Phys., 20, 5559–5572, https://doi.org/10.5194/acp-20-5559-2020, 2020
Yan, S., Zhu, B., Zhu, T., Shi, C., Liu, D., Kang, H., Lu, W., and Lu, C.: The effect of aerosols on fog lifetime: observational evidence and model simulations, Geophys. Res. Lett, 48, e2020GL61803, https://doi.org/10.1029/2020GL091156, 2021.
Yang, Y., Hu, X.-M., Gao, S., and Wang, Y.: Sensitivity of WRF simulations with the YSU PBL scheme to the lowest model level height for a sea fog event over the Yellow Sea, Atmos. Res., 215, 253–267, https://doi.org/10.1016/j.atmosres.2018.09.004, 2019.
Ye, X., Wu, B., and Zhang, H.: The turbulent structure and transport in fog layers observed over the Tianjin area, Atmos. Res., 153, 217–234, https://doi.org/10.1016/j.atmosres.2014.08.003, 2015.
Zhang, G., Bian, L., Wang, J., Yang, Y., Yao, W., and Xu, X.: The boundary layer characteristics in the heavy fog formation process over beijing and its adjacent areas, Sci. China Earth Sci., 48, 88–101, 2005.
Zhou, B. and Du, J.: Fog prediction from a multimodel mesoscale ensemble prediction system, Weather Forecast., 25, 303–322, https://doi.org/10.1175/2009WAF2222289.1, 2010.
Zhou, B. and Ferrier, B.: Asymptotic Analysis of Equilibrium in Radiation Fog, J. Appl. Meteorol. Clim., 47, 1704–1722, https://doi.org/10.1175/2007JAMC1685.1, 2008.
Zhou, B., Du, J., Gultepe, I., and Dimego, G.: Forecast of low visibility and fog from NCEP: Current status and efforts, Pure Appl. Geophys., 169, 895–909, https://doi.org/10.1007/s00024-011-0327-x, 2012.
Zhu, Y., Zhu, C., Zu, F., Wang, H., Liu Q., Qi, M., and Wang, Y.: A persistent fog event involving heavy pollutants in Yancheng area of Jiangsu Province, Adv. Meteorol., 2018, 2512138, https://doi.org/10.1155/2018/2512138, 2018.
Zhu, Y., Li, Z., Zu, F., Wang, H., Liu, Q., Qi, M., and Wang, Y.: The propagation of fog and its related pollutants in the Central and Eastern China in winter, Atmos. Res., 265, 105914, https://doi.org/10.1016/j.atmosres.2021.105914, 2022.