the Creative Commons Attribution 4.0 License.
the Creative Commons Attribution 4.0 License.
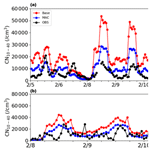
Substantially positive contributions of new particle formation to cloud condensation nuclei under low supersaturation in China based on numerical model improvements
Chupeng Zhang
Shangfei Hai
Shaoqing Zhang
Lifang Sheng
Shuxiao Wang
Jingkun Jiang
Xin Huang
Xiaojing Shen
Junying Sun
Aura Lupascu
Manish Shrivastava
Jerome D. Fast
Wenxuan Cheng
Xiuwen Guo
Ming Chu
Nan Ma
Juan Hong
Qiaoqiao Wang
Xiaohong Yao
Huiwang Gao
New particle formation (NPF) and subsequent particle growth are important sources of condensation nuclei (CN) and cloud condensation nuclei (CCN). While many observations have shown positive contributions of NPF to CCN at low supersaturation, negative NPF contributions were often simulated in polluted environments. Using the observations in a coastal city of Qingdao, Beijing, and Gucheng in north China, we thoroughly evaluate the simulated number concentrations of CN and CCN using an NPF-explicit parameterization embedded in the WRF-Chem model. For CN, the initial simulation shows large biases of particle number concentrations at 10–40 and 40–100 nm. By adjusting the process of gas–particle partitioning, including the mass accommodation coefficient (MAC) of sulfuric acid, the phase changes in primary organic aerosol emissions, and the condensational amount of nitric acid, the improvement of the particle growth process yields substantially reduced overestimation of CN. Regarding CCN, secondary organic aerosol (SOA) formed from the oxidation of semi-volatile and intermediate-volatility organic compounds (S/IVOCs) is called SI-SOA, the yield of which is an important contributor. At default settings, the SI-SOA yield is too high without considering the differences in precursor oxidation rates. Lowering the SI-SOA yield under linear H2SO4 nucleation scheme results in much-improved CCN simulations compared to observations. On the basis of the bias-corrected model, we find substantially positive contributions of NPF to CCN at low supersaturation (∼ 0.2 %) over broad areas of China, primarily due to competing effects of increasing particle hygroscopicity, a result of reductions in SI-SOA amount, surpassing that of particle size decreases. The bias-corrected model is robustly applicable to other schemes, such as the quadratic H2SO4 nucleation scheme, in terms of CN and CCN, though the dependence of CCN on SI-SOA yield is diminished likely due to changes in particle composition. This study highlights potentially much larger NPF contributions to CCN on a regional and even global basis.
- Article
(2074 KB) - Full-text XML
-
Supplement
(3184 KB) - BibTeX
- EndNote
New particle formation (NPF) is a process in which gaseous vapors nucleate and form critical molecular clusters, followed by subsequent growth to larger sizes through condensation and coagulation (Kulmala et al., 2004, 2013; Lee et al., 2019). Newly formed particles could effectively grow into the size of cloud condensation nuclei (CCN) under certain supersaturation (SS), which exerts an impact on the cloud microphysical process and global radiation balance (Merikanto et al., 2007; Kerminen et al., 2018; Ren et al., 2021). In addition, the efficient nucleation and explosive growth of particles may contribute to the formation of haze (Guo et al., 2014), affecting air quality and human health (Yuan et al., 2015; Chu et al., 2019; Kulmala et al., 2021).
The overestimation of condensation nuclei (CN) in numerical models is commonly seen despite the attempt to rectify the bias (Matsui et al., 2013; Arghavani et al., 2022). There is a common way to reduce the nucleation rate which may reduce the particle number concentration in proportion (Matsui et al., 2013). For instance, in the study of NPF in East Asia in the spring of 2009, even after lowering the nucleation rate in a regional model of WRF-Chem applied in their study, the reduced number concentration of particles at 10–130 nm remained overestimated (Matsui et al., 2013). Using the same regional model and a similar method to reduce the nucleation rate, Arghavani et al. (2022) found that particle number concentration at 10–100 nm was still overestimated by nearly 1 order of magnitude, despite the effectiveness of reducing the overestimation of smaller particles, such as those of 2.5–10 nm. In addition to the rate of NPF, the growth process of particles also has a crucial effect on particle number concentration and size distribution. In this process, the condensation of some chemical species such as sulfuric acid, nitrate, and organic gases on particles plays a major role in particle growth (Yao et al., 2018; Lee et al., 2019; Li et al., 2022), and the uncertainty in their condensation amount may lead to the bias of CN simulation.
In addition to CN, there are large discrepancies in the predicted CCN between the numerical models and observational results. Furthermore, as an important source of CCN (Merikanto et al., 2009), the contribution of nucleation to CCN quantified by numerical models is also highly uncertain. For example, in terms of predicting CCN, Fanourgakis et al. (2019) evaluated the CCN concentrations simulated by 16 global aerosol–climate and chemistry transport models with observations at nine sites in Europe and Japan from 2011 to 2015 and found that all models underestimated CCN concentrations, with a mean normalized mean bias of −36 % at low supersaturation (SS = 0.2 %). WRF-Chem models also tend to underestimate the contribution of NPF to CCN, especially at low supersaturation. The continuous observation of CCN concentrations throughout the year (July 2008–June 2009) carried out in Hyytiälä, Finland, showed that under low SS, nucleation enhanced the CCN by 106 % and 110 % at SS = 0.1 % and 0.2 %, respectively (Sihto et al., 2011). Observations acquired in Beijing from 12 July to 25 September 2008 also suggested that nucleation significantly increases CCN at all supersaturations, even when supersaturation is low (i.e., 0.07 % and 0.26 %). Thus, the occurrence of NPF enhanced CCN by a factor of 1.7 and 2.2, respectively (Yue et al., 2011).
However, previous numerical experiments behave oppositely. For instance, Matsui et al. (2011) quantified the contribution of nucleation to CCN using WRF-Chem in Beijing in August and September 2006 and found reduced CCN under low SS, e.g., when SS = 0.02 %, the concentration of CCN is reduced by up to ∼ 50 %. They attributed this to the fact that the small particles produced by nucleation may inhibit the growth of the pre-existing particles (Matsui et al., 2011). Similarly, Dong et al. (2019) conducted NPF simulations with the WRF-Chem for the summer of 2008 focusing on the Midwest of the United States and found that the nucleation resulted in decreased CCN at low supersaturation (SS = 0.1 %). In addition, a study carried out for East Asia in 2009 also indicated that at low supersaturation (e.g., SS = 0.1 %), nucleation has little impact on CCN (Matsui et al., 2013). The contrasting effects of nucleation on CCN at low supersaturations in model and observations are not explained in these previous studies.
At the stage of particle growth, secondary organic aerosol (SOA) formed by atmospheric oxidation of organic vapors is a major contributor to particle growth to CCN-related sizes (Liu and Matsui, 2022; Qiao et al., 2021). SOA formed by multi-generational gas-phase oxidation of semi-volatile and intermediate-volatility organic compounds (S/IVOCs) is called SI-SOA (Jimenez et al., 2009; Zhang et al., 2007). Zhao et al. (2016) made a comprehensive assessment of the roles of various SOA precursors in SOA formation in real atmosphere in China in 2010, and the results demonstrated that evaporated POA and IVOC (i.e., S/IVOC) made a significant contribution to SOA, contributing up to 82 % of the average SOA concentration in eastern China. However, the effect of SI-SOA on CCN has not been fully studied.
In this paper, WRF-Chem was applied to simulate the effect of the NPF on CCN in China in February 2017. The simulated results from the WRF-Chem model are firstly compared with observations in Qingdao, Beijing, and Gucheng, exhibiting large biases in CN. This is followed by an improvement through a few processes. At the end, the impact of SI-SOA yield and nucleation on CCN is investigated.
2.1 Observations
The measurements used in this study were carried out over the sampling site from 5 to 24 February 2017 at the campus of Ocean University of China (36∘09′37′′ N, 120∘29′44′′ E) in Qingdao, which is surrounded by residential buildings and is situated about 10 km away from the city center. A fast mobility particle sizer (FMPS, TSI Model 3091) was applied to measure the aerosol particle size distribution for the size range of 5.6 to 560 nm (Liu et al., 2014b). The bulk CCN concentration is measured by a cloud condensation nuclei counter at three different supersaturations (0.2 %, 0.4 %, and 0.6 %) and each supersaturation lasts for 20 min. More information about the CCN measurement can be found in Li et al. (2015). The urban site in Beijing is located on the roof of the building of the Chinese Academy of Meteorological Sciences (CAMS, 39∘95′ N, 116∘33′ E) on the campus of the China Meteorological Administration, close to the main road with heavy traffic. The rural site is Gucheng (GC, 39∘08′ N, 115∘40′ E) in Hebei Province, surrounded by farmland and a representative station of the severity of air pollution in the Beijing–Tianjin–Hebei region. The particle number size distribution of these two sites in the range of 4–850 nm is measured by a tandem scanning mobility particle sizer (TSMPS), and more information about the instruments can be found in Shen et al. (2018).
2.2 Model configurations
WRF-Chem version 3.9 is used to simulate NPF events, with the main physical and chemical parameterization settings summarized in Table 1. The spatial resolution is 36 × 36 km, with 35 vertical layers and a model top at 50 hPa. The regional model simulations at a higher spatial resolution may be desirable in future when urban pollution is focused. A continuous run from 1 to 25 February 2017 was conducted, with the first 5-day results as the spin-up and discarded in the analysis.
The meteorological initial and boundary conditions are driven by Climate Forecast System model version 2 (CFSv2; Saha et al., 2014) reanalysis developed by the National Centers for Environmental Prediction (NCEP). The initial and boundary chemical conditions of WRF-Chem are provided by the Community Atmosphere Model with Chemistry (CAM-Chem; Buchholz et al., 2019). Anthropogenic emissions for the year of 2017 are obtained from the Multiresolution Emission Inventory for China (MEIC, http://www.meicmodel.org/, last access: 4 October 2022) emission dataset (Li et al., 2017; Zheng et al., 2018).
The Model for Simulating Aerosol Interactions and Chemistry (MOSAIC) was used to delineate dynamic gas–particle mass transfer to represent the condensation growth of aerosol (Zaveri et al., 2008). The gas–particle partitioning of gas species on particles is regulated by the mass transfer rate, which is related to the mass accommodation coefficient (MAC, α), a parameter involved in the model representing the probability of gas molecules entering the bulk liquid phase (Pöschl et al., 1998). The original setting of α for all condensing species for all size bins in MOSAIC is 0.1 (Zaveri et al., 2008). In the default release of WRF-Chem, MOSAIC was implemented in the sectional framework with aerosol size distributions divided into four or eight size bins spanning 39 nm to 10 µm in diameter. To explicitly express the nucleation and the growth of newly formed particles, the aerosol size range in the MOSAIC module was extended from 1 nm to 10 µm, with the number of aerosol size bins increased to 20 (Matsui et al., 2011, 2013; Lupascu et al., 2015; Lai et al., 2022). The calculation method of CCN concentration in the WRF-Chem model is taken from the study of Matsui et al. (2011). Based on Köhler theory, CCN concentrations under the three given supersaturations of 0.2 %, 0.4 %, and 0.6 % were calculated. The critical supersaturation (Sc) of each size bin in the WRF-Chem model was calculated by the following formula:
where α (m) is the coefficient of the Kelvin effect, κ is the volume-averaged hygroscopicity, calculated using these values in Table 1, r (m) is the dry diameter, σ is droplet surface tension over water (0.076 N m−1), Rv is the gas constant for water vapor (461.6 J K−1 kg−1), T (K) is the air temperature, and ρω is the density of water (1000 kg m−3).
The chemical aging process of organic aerosols (OAs) is modeled by the volatility basis set (VBS) approach, which was widely used in air quality models to represent complex mixtures of thousands of organic species (Donahue et al., 2006; Shrivastava et al., 2011; Chrit et al., 2018). The VBS method classifies compounds according to the effective saturation concentration (c*), which represents the proportion of the component in the gas phase to the particle phase (Donahue et al., 2006), and species with higher c* values are more volatile. The oxidation of highly volatile precursors to form relatively low-volatility components represents the aging process of OA. OA consists of directly emitted primary organic aerosols and photochemically produced secondary organic aerosols (SOA; Shrivastava et al., 2011). In this study, the simplified two-species VBS mechanism was applied to the simulation of SOA, during which primary organic aerosol was represented by two species based on volatility with effective saturation concentration c* values (at 298 K and 1 atm) of 10−2 and 105 µg m−3 (Shrivastava et al., 2011). Primary organic aerosols with c* of 105 µg m−3 refers to S/IVOC, which is in the gas phase under most atmospheric conditions due to its high volatility, while primary organic matter with c* of 10−2 µg m−3 is treated as gas phase as well in the original model. The SOA formed by photochemical oxidation of S/IVOC precursors is called SI-SOA, and the SOA formed by oxidation of VOC precursors is named V-SOA. In the simplified two-species VBS mechanism, SI-SOA (c* of 10−2 µg m−3) is formed by the oxidation reaction of S/IVOC precursors (c* of 105 µg m−3) and OH with an oxidation rate constant of cm3 molec−1 s−1. The equations for controlling the oxidation of S/IVOC precursors are as follows:
where POA(g) denotes primary organic aerosols with c* of 105 µg m−3, which reacts with OH to form SI-SOA(g) with c* of 10−2 µg m−3. Subscripts c and o represent the non-oxygen and oxygen parts respectively of given species and e is either the biomass or the anthropogenic emission sector. In addition, SVOC and IVOC emissions corresponding to both anthropogenic and biomass burning emissions are derived based on constant emission ratio of S/IVOC to POA (Shrivastava et al., 2011). A detailed description of the two-species VBS mechanism can be found in Shrivastava et al. (2011).
2.3 Model sensitivity formulations
Three sets of sensitivity tests are designed and listed in Table 3. The purposes of the three sets of experiments are as follows: (1) adjust the condensation growth process of ultrafine particles in WRF-Chem model (Base, MAC, PEP, NOCD, RACD, with details in Table 3); (2) explore the effect of SI-SOA yield on CCN (Low_Yield and High_Yield); and (3) study the effect of nucleation process on CCN under the change in SI-SOA yield (Low_Yield and High_Yield and their corresponding cases without nucleation parameterization, i.e., Low_nucoff and High_nucoff). Each scenario will be explained in conjunction with the results.
3.1 Observational analysis
Based on the criteria (Dal Maso et al., 2005; Kulmala et al., 2012), NPF is defined as an event with the emergence of a nucleation mode with particle diameters smaller than 25 nm, lasting for 2 h or more, followed in general by continuous particle growth. Six NPF events were identified in February 2017 in Qingdao, on days 6, 9, 10, 17, 20, and 23 (Fig. 1a), yielding a frequency of ∼ 30 % and displaying a typical banana-shaped growth of particles in the particle number size distribution. Compared to a few other studies on NPF frequency in Qingdao, the results in this study are to a large extent consistent with that in the fall of 2012–2013 (30 %; Zhu et al., 2019), slightly higher than that in summer 2016 (22 %; Zhu et al., 2019), and lower than that in spring of 2010 (41 %; Liu et al., 2014b). The higher frequency in spring in Qingdao is consistent with the observational results at different stations in the Northern Hemisphere in Nieminen et al. (2018).
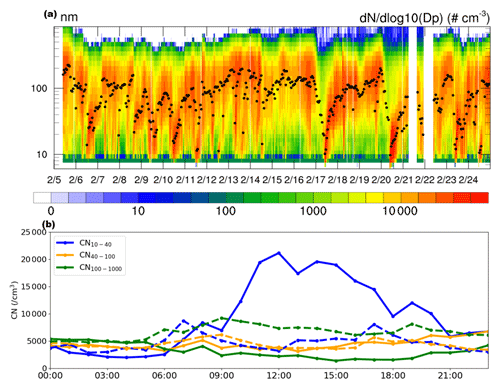
Figure 1Distribution of particle number concentration. (a) Temporal evolution of particle size distributions (colored shading) and geometric median diameter (GMD; dots in black) in Qingdao on 5–24 February 2017. (b) The mean diurnal variation in CN10−40 (blue), CN40−100 (orange), and CN100−1000 (green) composited during the NPF (solid lines) and non-NPF (dashed lines) days on 5–24 February 2017. All times are local (LT).
During the six NPF events identified in February in Qingdao, the mean diurnal cycle of CN10−40 (10–40 nm) particles exhibits triple peaks (solid blue in Fig. 1b), in the morning (08:00 LT), noon (12:00–14:00 LT), and evening (19:00 LT), respectively. A comparable three-peak feature was also observed in earlier years during 2016–2018 in Qingdao (Zhu et al., 2021). The morning and evening peaks of CN10−40, with values of ∼ 5300 and ∼ 12 000 cm−3, respectively, are likely caused by the primary emissions from traffic and cooking activities (Wu et al., 2021a; Wang et al., 2022; Cai et al., 2020). The occurrence of NPF starts approximately at 09:00 LT, accompanied by a substantial increase in CN10−40 compared with non-NPF days (solid vs. dashed lines, in blue), yielding a peak around noon (20 000 cm−3 during 12:00–14:00 LT). In addition, larger particles (e.g., CN40−100 and CN100−1000) displayed a slow or no increase in the afternoon.
3.2 Model improvement in particle number concentration simulations
Particle number concentrations, primarily in the ranges of 10–40 and 40–100 nm, are commonly simulated with large biases. In the smaller size range (10–40 nm), the particle number concentration is associated with NPF and particle growth. During NPF, despite differences among the formation mechanisms, H2SO4 is considered the common species (Yu, 2005; Lovejoy et al., 2004), which often suffer from large biases (Cai et al., 2016; Matsui et al., 2011). In the size range of 40–100 nm, the particle number concentration is primarily affected by the condensation growth of particles below 40 nm, which is closely related to chemical components such as SOA and nitrate. Prior to the evaluation of particle number concentration, we first evaluate the compositions of PM2.5 and criteria air pollutants including PM2.5, PM10, O3, SO2, CO, and NO2, showing relatively low biases compared to observations (Sect. S1 and Figs. S1 and S2 in the Supplement).
3.2.1 Bias correction of particle number concentration at 10–40 nm
In this study, as shown in Fig. 2, comparisons of CN10−40 between simulations (red line in Fig. 2a) and observations (black line in Fig. 2a) of the six NPF events mentioned in the previous section in Qingdao in February 2017 indicate that the model overestimates CN10−40 with a mean fractional bias of 48 %. As one of the major processes affecting the particle number concentration of 10–40 nm, nucleation is governed by the particle nucleation rate of 1 nm particles (cm−3 s−1), which is closely associated with the concentration of H2SO4. For instance, in a commonly applied activation mechanism, the nucleation rate calculated is . Note that KACT is the nucleation coefficient considering the physical properties and chemical species of nucleation process under different environments, indicating that a lumped chemical species is included in the scheme reflected primarily in the nucleation coefficient k, set as s−1 based on previous studies (Sihto et al., 2006; Riipinen et al., 2007). Dong et al. (2019) simulated NPF occurring in the summer of 2008 in the United States using the NPF-explicit WRF-Chem based on the activation mechanism, which overestimated, by nearly double, the particle number concentration as 10–63 nm, even when the KACT decreased by 1 order of magnitude (set at a very low value of 10−7 s−1). Therefore, it is likely that the overestimation of particle number concentration in the smaller particle size segment is probably due to the bias of simulated sulfuric acid.
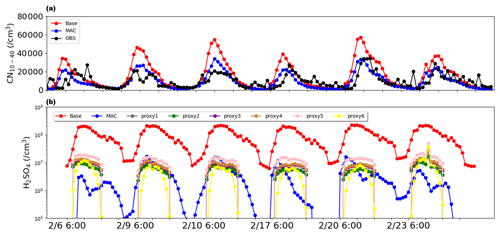
Figure 2Time series of (a) CN10−40 on NPF days, where red and blue represent Base and MAC simulation results, respectively, and black represents observation results; (b) sulfuric acid gas concentration obtained by simulation and by proxies (dark gray: Eq. 5, green: Eq. 6, purple: Eq. 7, brown: Eq. 8, pink: Eq. 9, yellow: Eq. 10). All times are local (LT).
Measurement of sulfuric acid gases in the lower troposphere is challenging due to the generally low ambient concentration of sulfuric acid (106–107 molec cm−3). Different methods have been proposed to estimate ambient sulfuric acid concentrations based on observations such as SO2 (Petäjä et al., 2009; Lu et al., 2019; Mikkonen et al., 2011). For instance, Petäjä et al. (2009) proposed a linear method to approximate observed H2SO4 concentration in Hyytiälä, southern Finland. Moreover, a recent study by Lu et al. (2019) proposed a nonlinear method to construct a number of proxies for gaseous sulfuric acid concentration (Eqs. 5–9), indicating that compared to the linear method in Petäjä et al. (2009), a nonlinear relationship can provide more accurate H2SO4 concentration in Beijing during the February–March 2018 period. In addition, we also used another sulfuric acid nonlinear proxy (Eq. 10) based on long-term observations in Germany, Finland, the United States, etc. (Mikkonen et al., 2011). In this study, we adopt the above six nonlinear proxy methods (referred to as proxy5 to proxy10) to estimate H2SO4 in Qingdao.
where [SO2], [O3], and [NOx] (molecule cm−3) represent the concentrations of observed SO2, O3, and NOx, respectively. “Radiation” (W m−2) is global radiation. RH (%) is the relative humidity, and CS (s−1) is the condensation sink, which is calculated based on observed particle distribution.
The simulated H2SO4 concentration from the Base simulation (dots in Fig. 2b) is compared with observations obtained by proxies (see Fig. 2b), indicating that Base simulations apparently overestimate by 1 order of magnitude compared to the H2SO4 estimated by proxies. The overestimation has been frequently reported previously, i.e., over Beijing (Matsui et al., 2011), which ascribes the bias to the overestimation of the SO2 concentration. In a more recent study, the sensitivity of H2SO4 to SO2 is tested, and the result shows that even when SO2 is reduced to an unrealistically low level, the simulated H2SO4 is still more than 1 order of magnitude higher than the observed value (Lai et al., 2022), suggesting that the SO2 concentration cannot fully explain the overestimation.
In addition to the precursor of H2SO4, the mass accommodation coefficient (α), representing the probability of the impaction of a gaseous molecule on a liquid surface and its entering the bulk liquid phase, is another important factor affecting the concentration of sulfuric acid gas. In the public release of WRF-Chem, the mass accommodation coefficient is typically set to a low value of 0.1 for all gas species under different volatility during the condensation process, including H2SO4 (Davidovits et al., 2004; Zaveri et al., 2008). Recent studies indicate that the low mass accommodation coefficient value may not be applicable to the low-volatility gases, which tend to have a mean mass accommodation coefficient value of 0.7 and be close to unity (Krechmer et al., 2017). In fact, an earlier study has indicated based on experimental determination, where the mass accommodation coefficient of H2SO4 vapor in sulfuric acid aqueous solution was measured, that the best fit value was 0.65. Accordingly, a sensitivity simulation was conducted by adjusting the mass accommodation coefficient of H2SO4 from 0.1 to 0.65, referred to as MAC.
This simulation brought the H2SO4 concentration (see Fig. 2b) much closer to the calculated results from proxies, and the corresponding biases reduced by approximately 1 order of magnitude. Notably, the MAC simulation decreases the overestimation of sulfuric acid gas concentration, resulting in a lower particle formation rate. The MAC simulation also significantly reduces overestimation of CN10−40 (Fig. 2a), and mean fractional bias compared to observations decreases from 48 % to 1 %.
3.2.2 Improvement of particle number concentration simulations at 40–100 nm
The number concentration of particles in the 40–100 nm range is mainly affected by the coagulation and condensation processes. While the coagulation process tends to largely affect ultrafine particles below 10 nm than those with larger sizes (Wu et al., 2011), the condensation growth of particles during gas–particle partitioning at sizes of 10–40 nm, to a large extent, governs the variations in number concentration of 40–100 nm particles. The condensation process is primarily controlled by gas–particle partitioning of chemical species, which may change the chemical composition of particles, such as organic compounds and inorganics including sulfate, nitrate, and ammonium.
Among the species contributing to the condensation growth of particles at 10–40 nm, organic compounds with c* of 10−2 µg m−3 play the dominant role (Pierce et al., 2011). In the current model setting, the low-volatility organic matter of 10−2 µg m−3 comes from two gas-phase sources, including the direct emission of primary organic aerosol (POA) and SOA formed from S/IVOC (SI-SOA), conducive to condensation on particles. While the condensation of gaseous SOA is in general reasonable, the gas-phase emissions of POA may be problematic. For instance, previous studies suggested that POA is in gas phase close to the emissions source. However, with rapid dilution and cooling in the atmosphere away from the source, most POA condenses to the particle phase (Roldin et al., 2011a, b; Shrivastava et al., 2008). Therefore, away from the emissions source, being in the particle phase, POA will not be involved in the growth of newly formed particles. Therefore, POA may not contribute to particle growth away from the emission sources, which caused different size distributions of POA compared to when it was emitted in the gas phase (Fig. S3a vs. Fig. S3b). Emitting low-volatility POA in the particle phase eliminates the unreasonable quasi-banana shape pattern exhibiting concomitant growth of newly formed particles with increasing mass concentration of POA.
The composition analysis (Fig. S3c) of the 10–40 nm particles mass from the model results indicates that the organic compounds mentioned above only account for 21 % of total mass (sulfates, nitrates, ammonium salts, and organics) in this size range. The dominant species is nitrate, which accounts for 51 % of total mass, exhibiting inconsistencies with previous studies, which in general indicate a much smaller contribution of nitrate. For instance, Liu et al. (2014a) suggested that over the North China Plain in summer 2009, organic matter accounted for 77 % of particles around 30 nm, while the sum of SO, NO and NH only accounted for 18 %. Recent observations conducted in Beijing also indicated that particles at 8–40 nm are mainly composed of organic matter (with a mass fraction of ∼ 80 %) and sulfate (with mass fraction of ∼ 13 %), while nitrate content is very low (with mass fraction of ∼ 3 %; Li et al., 2022). Another study showed that nitrate accounted for 7 %–8 % at urban sites and 17 % at rural sites of particle mass in the 7–30 nm range in the United States in 2007 (Bzdek et al., 2012). Therefore, the potentially overestimated nitrate fraction in the 10–40 nm range modeled in this study is tightly associated with the condensation process, with the specific reasons explained below.
The condensation of nitric acid on particles is highly constrained by the particle acidity. The acidity in smaller particles (i.e., 10–40 nm) tends to be higher than that in large particles, primarily due to the larger condensation of H2SO4 (Lu et al., 2022), and particles of sizes greater than 40 nm have much weaker acidity or are nearly neutral. For example, observed evidence has shown that acidic ultrafine particles accounted for a large proportion of ultrafine particles from 22 December 2010 to 15 January 2011 in Hong Kong, e.g., 65 % for particles within 5.5–30 nm (Wang et al., 2014).
In the model, a particle is determined to be in solid phase when the ambient relative humidity is lower than the mutual deliquescence relative humidity of the particles (Zaveri et al., 2005, 2008), which is in general suitable for particles dominated by inorganics. In the study area, the results indicate that in most conditions, relative humidity is relatively low and the particles are in solid phase, in which the condensation process is not affected by particle acidity and the condensation of nitric acid on particles is directly calculated based on the gas–particle equilibrium concentration (Zaveri et al., 2008). However, for particles below 40 nm, the main compositions are likely to be organic matter (Zhu et al., 2014; Ehn et al., 2014), which tends to be in liquid phase (Virtanen et al., 2011; Cheng et al., 2015), under which the condensation of nitric acid is strongly constrained by acidity. Therefore, the phase misrepresentation ignores the weakening effect of acidity on nitric acid condensation, resulting in too-high nitrate therein.
To overcome this issue, we propose a ratio method for condensation (RACD) to partition the condensation of nitric acid on particles under 40 nm, by applying a ratio of the number concentration of non-acidic particles to ultrafine particles. The method is based on two assumptions: (1) little condensation of nitric acid on particles with strong acidity (Lu et al., 2022) and (2) the condensation of nitric acid on particles is proportional to the ratio of the number concentration of non-acidic ultrafine particles to the total particles, despite the existence of uncertainties. Figure S4 depicts the average particle number concentration and acid particle in the 1 to 40 nm range, calculated based on Wang et al. (2014). The ratio of non-acidic particles is 8 % for particles below 10 nm, 18 % for particles at 10–15.8 nm, 30 % for particles at 15.8–25.1 nm, and 55 % for particles at 25.1–39.8 nm (Fig. S4). Note that the ratio is based on measurements acquired at a single site in Hong Kong; therefore, more observational studies are needed to warrant the robustness of the method. Alternatively, the condensation of nitric acid on particles in bins from 1 to 40 nm is completely suppressed, referred to as NOCD.
The simulation results based on the two methods (RACD and NOCD) are shown in Fig. 3. Compared to MAC, RACD simulations reduce previously noted overestimation of particle number concentration in the 40–100 nm size range (Fig. 3b), with the mean fractional bias decreasing from 83 % to 63 %. In addition to the amount of nitrate condensation during the particle growth mentioned above, the overestimation of particle number concentrations in the 40–100 nm range may be attributed to the nucleation process. More specifically, in the H2SO4–H2O binary nucleation mechanism used in this study, when the concentration of sulfuric acid gas is reduced (Sect. 3.2.1), the resulting decrease in nucleation rate leads to a slight decrease in particle number concentration at 40–100 nm relative to Base (mean fractional bias from 98 % to 83 %). Apart from that, it may also be related to the choice of nucleation parameterization scheme. For example, using the global chemical transport model GEOS-Chem with a nucleation mechanism in which formation rate is a function of the concentrations of sulfuric acid and low-volatility organics, Yu et al. (2015) overestimated the concentration of particles in the 10–100 nm range by 161 % at nine sites in the summer in North America. A possible explanation for this overestimation was given by the uncertainty in the predicted concentration of organic compounds involved in organics-mediated nucleation parameterization. After they switched to another scheme of the ion-mediated nucleation mechanism, without organic matter, the number becomes 27 % lower than the observations (Yu et al., 2015). The test based on different schemes is beyond the scope of the study and is therefore not investigated.
Moreover, the overestimation of particles over 100 nm (CN100−1000; Fig. 3c), which have a strong influence on CCN, also decreases in the RACD simulation. Thus, the mean fractional bias decreases from 25 % (MAC) to 1 %. Note that the slight increase in CN10−40 through the application of RACD can be linked to the decrease in nitrate condensation, and it leads to weakened particle growth and enhanced particle number concentration at 10–40 nm (Fig. 3a). The alternative method, by completely removing the nitrate condensation (NOCD), yields even better performance in particle number concentration of 40–100 nm (mean fractional bias of 34 %), indicating the feasibility of reducing the nitrate condensation. The proportion of nitrate simulated by RACD is 23 %, closer to values reported in past observations (Bzdek et al., 2011, 2012), while the nitrate (1 %) in the scenario of NOCD seems to be too low. Considering the limited observational information obtained based on previous studies, RACD is applied in this study.
In addition to Qingdao, we evaluate the model performance over a few other sites, including one site over urban Beijing and another over the rural area of Gucheng, yielding consistent improvements in model simulations (Sect. S2; Figs. S5–S7). Moreover, we select another empirical scheme, e.g., kinetics, and one classical nucleation scheme, indicating that the empirical scheme is in general a good option in this study. (Sect. S2; Figs. S8–S10; Tables S1–S3).
3.3 Substantial contributions of SI-SOA to CCN
Compared with the original model setting, after adjusting the growth process of ultrafine particles (RACD), the number concentration of particles tends to decrease, especially for particles above 40 nm. Ultrafine particles above 40 nm are important sources of CCN (Dusek et al., 2006), in this way, the number concentration of CCN also tends to decline. In addition, in the Base case, we found that the model overestimated CCN0.4 % and CCN0.6 %, with mean fractional bias being 64 % and 87 %, respectively. After adjusting the condensation growth process of ultrafine particles, under high supersaturation (i.e., CCN0.4 % and CCN0.6 %), the capability of the model in reproducing the CCN is improved. RACD reduces the overestimation of CCN0.4 % and CCN0.6 %, with mean fractional bias reduced to 30 % and 56 %, respectively, although the overestimation still exists (Fig. S11b, c). However, for low supersaturation (i.e., CCN0.2 %), the decrease in number concentration of CCN is too large and the mean fractional bias decreases from 7 % to −45 % (Fig. S11a); therefore, the bias will be further adjusted later.
In addition to the growth process, the remaining overestimation of CCN under high SS and underestimation of CCN over low SS is likely to be influenced by the chemical compositions involved in the activation of ultrafine particles into CCN. Specifically, ultrafine particles can grow up to CCN size under certain SS (Pierce and Adams, 2007). This process is influenced by both particle size and hygroscopicity, and hygroscopicity is closely related to the chemical composition of particles (Petters and Kreidenweis, 2007). In particular, inorganic compounds generally increase particle hygroscopicity, increasing CCN. SOA has dual effects on CCN since it decreases particle hygroscopicity and also promotes the growth of particles, and these two effects are competitive with each other (Wu et al., 2015; Zaveri et al., 2021). Ultrafine particles must grow to a critical size to be activated into CCN (Dusek et al., 2006). SOA act as a major contributor in promoting the condensational growth of ultrafine particles to the critical size, facilitating particle activation into CCN. In contrast, SOA tends to reduce the hygroscopicity of particles, leading to a diminished ability of activation into CCN (Wu et al., 2015). These two competing effects work together and modulate the number of CCN. Moreover, considering that SI-SOA is the main SOA component of ultrafine particles (Fig. S11d), the effect of SI-SOA on CCN is explored in this study.
Considering SI-SOA is a product of S/IVOC oxidation, the oxidation rate of S/IVOC is tightly associated with CCN, which likely affects the bias of CCN. In the original model setup, the oxidation rate is set to be a constant of cm3 molec−1 s−1 for all S/IVOC. However, a recent study (Wu et al., 2021b) proposed that the oxidation rate can be as high as cm3 molec−1 s−1 such as for polycyclic aromatic hydrocarbons (PAHs). This is close to the original model value but it can be as low as half (i.e., cm3 molec−1 s−1) of the original modeling setting for S/IVOC species except PAHs (O-S/IVOCs). It is noteworthy that the oxidation rates of and in general represent the upper and lower bounds (Zhao et al., 2016; Wu et al., 2021b).
To delve into how oxidation rate affects CCN, we set up a few numerical experiments (Table 3) to investigate the response of CCN to the oxidation rate of S/IVOC at three supersaturations (0.6 %, 0.4 %, 0.2 %), including cases of High_Yield and Low_Yield. As it is shown in Fig. 4, decreasing the oxidation rate (Low_Yield) leads to a reduction in ∼ 10 % of CCN at high supersaturation (i.e., CCN0.6 %) as compared to the High_Yield simulation. This behavior is a consequence of the decrease in particle number concentrations associated with Low_Yield, particularly of the particles close to the critical diameter (40–100 nm). In this case, the effect of particle size dominates the hygroscopicity. In contrast, at a lower supersaturation (CCN0.2 %), CCN increases by 42 % when the oxidation rate is switched from a high to a low value, which is due to the smaller fraction of SI-SOA contributing to particulate mass when the oxidation rate is low. In this case, relative to SOA, a larger fraction of other particle constituents such as inorganics increases the volume-weighted particle hygroscopicity (Dusek et al., 2006), which causes the increase in CCN number. This means that the effect of hygroscopicity on CCN surpasses the influence on particle size at low supersaturations. This conclusion is consistent with the observation conducted by Ma et al. (2016) in the North China Plain in 2013, which suggested that along with the decrease in SS, the particles that can be activated into CCN are more sensitive to changes in particle hygroscopicity. Similarly, based on observational data in northern China in summer, Wang et al. (2023) found that CN in 2020 is lower than that in 2014 due to particulate pollution control; however, the particles become more easily activated, attributable to the larger extent of the decrease in organic matter compared to inorganics, leading to enhanced particle hygroscopicity and more conduciveness to activation.
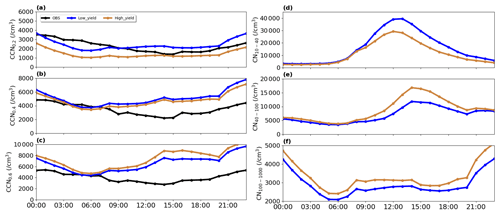
Figure 4Average diurnal variation in (a) CCN0.2 %, (b), CCN0.4 % and (c) CCN0.6 % and (d) CN10−40, (e) CN40−100, and (f) CN100−1000 on NPF days in Qingdao on 5–24 February 2017, in Low_yield and High_yield simulations, shown as blue and brown lines; black lines represent observation results.
Furthermore, compared to the high yield of SI-SOA, the low SI-SOA yield results in a high CCN concentration under low SS and low CCN concentration under high SS. Therefore, both the underestimation of CCN0.2 % (mean fractional bias of −45 %) and overestimation of CCN0.6 % (mean fractional bias of 56 %) mentioned above are improved, with the mean fractional biases of CCN0.2 % and CCN0.6 % reaching 7 % and 43 %, respectively (Fig. 4a, c). This result suggests that the oxidation rate of S/IVOC is possibly closer to a low value. This is understandable based on Wu et al. (2021b), who found that the amount of O-S/IVOCs, which corresponds to a low oxidation rate, is in general much larger (i.e., 20 times) than that of PAHs with a high oxidation rate.
In addition to the single site of Qingdao, we further explore the impact of SI-SOA yield on CCN with larger spatial coverage (Fig. 5). Consistent with the mechanism revealed over Qingdao, even from a larger spatial perspective, a lower oxidation rate of S/IVOC essentially enhances CCN at a lower SS (e.g., CCN0.2 %; Fig. 5a), with the greatest increase over the North China Plain area (Fig. 5a), and this weakens CCN (i.e., by 10 %–20 % over Beijing–Tianjin–Hebei) at a higher SS (Fig. 5c), particularly over the dense emission area (Fig. S12). It is worth noting that in the two-species VBS mechanism used in our study, all S/IVOC in the inventory is calculated based on a constant emission ratio of S/IVOC to POA from all source categories (Shrivastava et al., 2011), which may miss part of S/IVOC due to different emission ratios of POA from different sources (Chang et al., 2022). In addition, the simplified VBS mechanism used in our study does not take into account the multi-step oxidation of organic species, which may introduce some uncertainties. To be more specific, in the two-species VBS mechanism, SI-SOA with effective saturation concentrations (c*) of 10−2 µg m−3 is formed by the vapor-phase oxidation of S/IVOC vapors with c* of 105 µg m−3, reducing volatility by 7 orders of magnitude. The process of one-step oxidation does not mean representing a physical process but parameterizing the mean effect of a complex process of SOA formation (Shrivastava et al., 2011). However, in the real atmosphere, gaseous VOCs often undergo multi-generational oxidation to form SOA (Garmash et al., 2020), during which the properties and composition of SOA change substantially. For instance, by adding the formation chemistry associated with multi-generational oxidation, Zhao et al. (2020) found improved simulations of a vertical aerosol profile in the Amazon free troposphere compared to the simplified VBS mechanism.
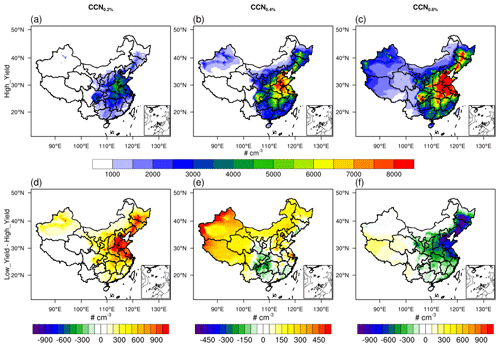
Figure 5Spatial distributions of CCN concentrations at different supersaturations (SS): (a) and (d) are CCN0.2 %, (b) and (e) are CCN0.4 %, and (c) and (f) are CCN0.6 %. The top panels exhibit the results from the High_Yield simulation, and the bottom panels show the difference between the Low_Yield and High_Yield simulations.
3.4 Contribution of nucleation to CCN under different SI-SOA yields
Considering the importance of nucleated particles on CCN (Yu et al., 2020; Westervelt et al., 2013), we further investigate the influence of nucleation on CCN under different SI-SOA yield conditions discussed above.
As shown in Fig. 6, in simulations close to the original model setting (High_Yield), when SS is low (i.e., SS = 0.2 %), the nucleation process tends to reduce the CCN by ∼ 10 %–50 %. In contrast, when the SS is high (0.6 %), the nucleation results in a significant increase in CCN in most regions of China. When the yield of SI-SOA is adjusted to a lower level, the nucleation process has a positive contribution to CCN under both low and high SS, in particular, when SS is low (0.2 %) and there is a sign reversal (i.e., from negative, Fig. 6a, to positive, Fig. 6d). With the decrease in SI-SOA yield, the contribution of NPF to CCN is approximately 10 %–20 %, mainly concentrated in eastern China. The primary mechanism lies in that, along with the decrease in SI-SOA yield, the smaller fraction of SI-SOA yields an increase in hygroscopicity, which surpasses the suppression effect on particle growth due to reduced SI-SOA formation. In the real atmosphere, when supersaturation is usually low, e.g., about ∼ 0.1 % in polluted areas (Kalkavouras et al., 2019; Hudson and Noble, 2014), CCN will likely reduce with increasing oxidation rate of S/IVOC and corresponding SI-SOA formation.
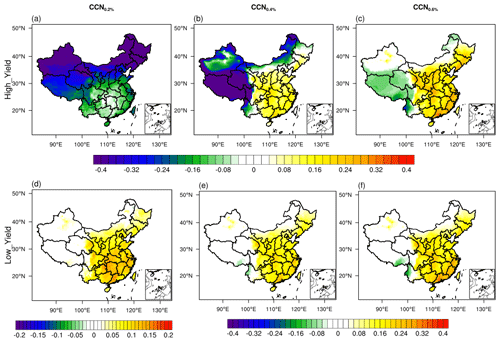
Figure 6Spatial distribution of the contribution of nucleation to CCN calculated by the ratio of the difference between the parameterization with and without nucleation to the parameterization with nucleation under different SI-SOA yields in China in February 2017. (a), (d) CCN0.2 %, (b), (e) CCN0.4 %, and (c), (f) CCN0.6 %. The upper panels and lower panels represent High_Yield and Low_Yield simulations, respectively.
In addition to the linear H2SO4 nucleation mechanism, an additional empirical scheme of kinetics nucleation is selected, which assumes that the nucleation rate is proportional to the square of the concentration of sulfuric acid (J= K[H2SO4]2), in order to investigate the effect of nucleation on CCN. Substantially positive contributions of nucleation to CCN is found when the low SI-SOA yield is applied, consistent with that shown based on the linear H2SO4 nucleation scheme (Fig. S13). However, nucleation contributes positively to CCN even when the SI-SOA yield is high in the quadratic H2SO4 nucleation scheme (e.g., kinetics nucleation scheme). When more sulfuric acid molecules participate in nucleation under this scheme than the linear H2SO4 nucleation scheme, the particles are more easily hygroscopically activated into CCN, which is equivalent to the effect of a reduction in organic components in the linear H2SO4 nucleation scheme (e.g., activation-type nucleation scheme). The results from this study show the importance of assessing the simulated effects of the nucleation scheme on not only the formation and growth process of particles but also climate factors such as CCN using observations.
In this study, WRF-Chem NPF-explicit simulations, with a linear H2SO4 nucleation scheme (e.g., activation-type nucleation scheme), are used to investigate observed wintertime NPF events and their contribution to CCN in China. Based on observations in a typical coastal city of Qingdao, as well as in the cities of Beijing and Gucheng over the North China Plain, we identify high biases of the model-simulated CN and CCN concentrations. Therefore, we updated and improved the parameterization setting of particle growth in the model, mainly by (1) adjusting the mass accommodation coefficient (α) from the default value of 0.1 to 0.65, an important parameter for sulfuric acid condensation; (2) proportionally reducing the condensation amount of nitric acid on particles below 40 nm; and (3) changing the emitted low-volatility POA from the gas to the particle phase. Through these adjustments, the capability of the model in reproducing CN and CCN is substantially improved, leading to better agreement with the observed results, which significantly reduces the overestimation of CN10−40 (mean fractional bias decreases from 48 % to 1 %) and CN40−100 (mean fractional bias decreases from 98 % to 63 %).
For CCN, due to the crucial role of SI-SOA in promoting the growth of ultrafine particles, on the basis of previous studies, we lower the oxidation rate of S/IVOC and hence the production rate of SI-SOA, which weakens the growth of particles to reach the critical size of CCN activation but enhances particulate hygroscopicity favoring the activation into CCN. When the yield of SI-SOA is adjusted to the lower bound of literature values, CCN0.6 % is reduced by ∼ 10 % and is closer to observations. At low SS (CCN0.2 %), the decrease in SI-SOA yield has greater effects on the increase in particle hygroscopicity compared to the effect of the reduction in particle size due to the decrease in condensation growth. This results in an increase in CCN (as large as ∼ 42 %), in better agreement with observations. Under low SS conditions, common in the atmosphere, a 2.5-fold reduction in SI-SOA yield results in a substantial increase in CCN that switches from a negative contribution of new particle formation to CCN from approx. −50 % to −10 % to a positive contribution of approx. 10 % to 20 %.
In addition to activation nucleation scheme, we have also tested a few other schemes such as the quadratic H2SO4 nucleation scheme (e.g., kinetics nucleation scheme). Under this scheme, the abovementioned bias-corrected method is applicable to improving the simulations of concentrations of CN and CCN. It is noteworthy that the dependence of CCN on the SI-SOA yield has diminished, showing that under both high and low yields of SI-SOA, there are positive contributions of NPF to CCN. This is likely due to the increase in the amount of sulfuric acid involved in nucleation, making it more hygroscopic and easier to activate into CCN, and the high content of inorganic species makes them less sensitive to changes in SI-SOA yield, which deserves further investigation.
Code is available upon reasonable request to the corresponding author (yanggao@ouc.edu.cn).
Data is available upon request to the corresponding author (yanggao@ouc.edu.cn).
The supplement related to this article is available online at: https://doi.org/10.5194/acp-23-10713-2023-supplement.
CPZ and SFH designed the experiments, conducted the numerical experiment, and wrote the article. YG and WYH conceived the idea and the experiment design. ZB, MS, and LA discussed the experimental design and analysis. All co-authors contributed to the discussion and writing of the article.
At least one of the (co-)authors is a member of the editorial board of Atmospheric Chemistry and Physics. The peer-review process was guided by an independent editor, and the authors also have no other competing interests to declare.
Publisher's note: Copernicus Publications remains neutral with regard to jurisdictional claims in published maps and institutional affiliations.
This research was supported by grants from the National Natural Science Foundation of China (grant no. 42122039) and Fundamental Research Funds for the Central Universities (grant nos. 202341001 and 202072001). Yuhang Wang was supported by the National Science Foundation Atmospheric Chemistry Program. Manish Shrivastava was supported by the US Department of Energy (DOE) Office of Science, Office of Biological and Environmental Research (BER), through the Early Career Research Program and the Atmospheric System Research (ASR) program.
This paper was edited by Zhibin Wang and reviewed by three anonymous referees.
Arghavani, S., Rose, C., Banson, S., Lupascu, A., Gouhier, M., Sellegri, K., and Planche, C.: The Effect of Using a New Parameterization of Nucleation in the WRF-Chem Model on New Particle Formation in a Passive Volcanic Plume, Atmosphere, 13, 15, https://doi.org/10.3390/atmos13010015, 2022.
Buchholz, R. R., Emmons, L. K., and Tilmes, S.: The CESM2 Development Team, CESM2.1/CAM-Chem Instantaneous Output for Boundary Conditions, UCAR/NCAR – Atmospheric Chemistry Observations and Modeling Laboratory, Lat: 10 to 70, Lon: 80 to 150, February 2017–March 2017, https://doi.org/10.5065/NMP7-EP60, 2019.
Bzdek, B., Zordan, C., Luther, G., and Johnston, M.: Nanoparticle Chemical Composition During New Particle Formation, Aerosol Sci. Technol., 45, 1041–1048, https://doi.org/10.1080/02786826.2011.580392, 2011.
Bzdek, B. R., Zordan, C. A., Pennington, M. R., Luther III, G. W., and Johnston, M. V.: Quantitative Assessment of the Sulfuric Acid Contribution to New Particle Growth, Environ. Sci. Technol., 46, 4365–4373, https://doi.org/10.1021/es204556c, 2012.
Cai, C., Zhang, X., Wang, K., Zhang, Y., Wang, L., Zhang, Q., Duan, F., He, K., and Yu, S.-C.: Incorporation of new particle formation and early growth treatments into WRF/Chem: Model improvement, evaluation, and impacts of anthropogenic aerosols over East Asia, Atmos. Environ., 124, 262–284, https://doi.org/10.1016/j.atmosenv.2015.05.046, 2016.
Cai, J., Chu, B., Yao, L., Yan, C., Heikkinen, L. M., Zheng, F., Li, C., Fan, X., Zhang, S., Yang, D., Wang, Y., Kokkonen, T. V., Chan, T., Zhou, Y., Dada, L., Liu, Y., He, H., Paasonen, P., Kujansuu, J. T., Petäjä, T., Mohr, C., Kangasluoma, J., Bianchi, F., Sun, Y., Croteau, P. L., Worsnop, D. R., Kerminen, V. M., Du, W., Kulmala, M., and Daellenbach, K. R.: Size-segregated particle number and mass concentrations from different emission sources in urban Beijing, Atmos. Chem. Phys., 20, 12721–12740, https://doi.org/10.5194/acp-20-12721-2020, 2020.
Carter, W.: Documentation of the SAPRC-99 Chemical Mechanism for VOC Reactivity Assessment, Final Report to California Air Resources Board, http://www.engr.ucr.edu/~carter/reactdat.htm (last access: 4 June 2022), 2000.
Chang, X., Zhao, B., Zheng, H., Wang, S., Cai, S., Guo, F., Gui, P., Huang, G., Wu, D., Han, L., Xing, J., Hanyang, M., Hu, R., Liang, C., xu, Q., Xionghui, Q., Ding, D., Liu, K., Han, R., and Donahue, N.: Full-volatility emission framework corrects missing and underestimated secondary organic aerosol sources, One Earth, 5, 403–412, https://doi.org/10.1016/j.oneear.2022.03.015, 2022.
Chen, F. and Dudhia, J.: Coupling and advanced land surface/hydrology model with the Penn State-NCAR MM5 modeling system. Part I: Model implementation and sensitivity, Mon. Weather Rev., 129, 569–585, https://doi.org/10.1175/1520-0493(2001)129<0569:CAALSH>2.0.CO;2, 2000.
Cheng, Y., Su, H., Koop, T., Mikhailov, E., and Pöschl, U.: Size dependence of phase transitions in aerosol nanoparticles, Nat. Commun., 6, 5923, https://doi.org/10.1038/ncomms6923, 2015.
Chrit, M., Sartelet, K., Sciare, J., Majdi, M., Nicolas, J., Petit, J. E., and Dulac, F.: Modeling organic aerosol concentrations and properties during winter 2014 in the northwestern Mediterranean region, Atmos. Chem. Phys., 18, 18079–18100, https://doi.org/10.5194/acp-18-18079-2018, 2018.
Chu, B., Kerminen, V. M., Bianchi, F., Yan, C., Petäjä, T., and Kulmala, M.: Atmospheric new particle formation in China, Atmos. Chem. Phys., 19, 115–138, https://doi.org/10.5194/acp-19-115-2019, 2019.
Dal Maso, M., Kulmala, M., Riipinen, I., and Wagner, R.: Formation and growth of fresh atmospheric aerosols: Eight years of aerosol size distribution data from SMEAR II, Hyytiälä, Finland, Boreal Environ. Res., 10, 323–336, 2005.
Davidovits, P., Worsnop, D. R., Jayne, J. T., Kolb, C. E., Winkler, P., Vrtala, A., Wagner, P. E., Kulmala, M., Lehtinen, K. E. J., Vesala, T., and Mozurkewich, M.: Mass accommodation coefficient of water vapor on liquid water, Geophys. Res. Lett., 31, L22111, https://doi.org/10.1029/2004GL020835, 2004.
Donahue, N. M., Robinson, A. L., Stanier, C. O., and Pandis, S. N.: Coupled Partitioning, Dilution, and Chemical Aging of Semivolatile Organics, Environ. Sci. Technol., 40, 2635–2643, https://doi.org/10.1021/es052297c, 2006.
Dong, C., Matsui, H., Spak, S., Kalafut-Pettibone, A., and Stanier, C.: Impacts of New Particle Formation on Short-term Meteorology and Air Quality as Determined by the NPF-explicit WRF-Chem in the Midwestern United States, Aerosol Air Qual. Res., 19, 204–220, https://doi.org/10.4209/aaqr.2018.05.0163, 2019.
Dusek, U., Frank, G. P., Hildebrandt, L., Curtius, J., Schneider, J., Walter, S., Chand, D., Drewnick, F., Hings, S., Jung, D., Borrmann, S., and Andreae, M. O.: Size Matters More Than Chemistry for Cloud-Nucleating Ability of Aerosol Particles, Science, 312, 1375–1378, https://doi.org/10.1126/science.1125261, 2006.
Ehn, M., Thornton, J., Kleist, E., Sipilä, M., Junninen, H., Pullinen, I., Springer, M., Rubach, F., Tillmann, R., Lee, B., Lopez-Hilfiker, F., Andrés, S., Acir, I. H., Rissanen, M., Jokinen, T., Schobesberger, S., Kangasluoma, J., Kontkanen, J., Nieminen, T., and Mentel, T.: A large source of low-volatility secondary organic aerosol, Nature, 506, 476–479, https://doi.org/10.1038/nature13032, 2014.
Fanourgakis, G. S., Kanakidou, M., Nenes, A., Bauer, S. E., Bergman, T., Carslaw, K. S., Grini, A., Hamilton, D. S., Johnson, J. S., Karydis, V. A., Kirkevåg, A., Kodros, J. K., Lohmann, U., Luo, G., Makkonen, R., Matsui, H., Neubauer, D., Pierce, J. R., Schmale, J., Stier, P., Tsigaridis, K., van Noije, T., Wang, H., Watson-Parris, D., Westervelt, D. M., Yang, Y., Yoshioka, M., Daskalakis, N., Decesari, S., Gysel-Beer, M., Kalivitis, N., Liu, X., Mahowald, N. M., Myriokefalitakis, S., Schrödner, R., Sfakianaki, M., Tsimpidi, A. P., Wu, M., and Yu, F.: Evaluation of global simulations of aerosol particle and cloud condensation nuclei number, with implications for cloud droplet formation, Atmos. Chem. Phys., 19, 8591–8617, https://doi.org/10.5194/acp-19-8591-2019, 2019.
Garmash, O., Rissanen, M. P., Pullinen, I., Schmitt, S., Kausiala, O., Tillmann, R., Zhao, D., Percival, C., Bannan, T. J., Priestley, M., Hallquist, Å. M., Kleist, E., Kiendler-Scharr, A., Hallquist, M., Berndt, T., McFiggans, G., Wildt, J., Mentel, T. F., and Ehn, M.: Multi-generation OH oxidation as a source for highly oxygenated organic molecules from aromatics, Atmos. Chem. Phys., 20, 515–537, https://doi.org/10.5194/acp-20-515-2020, 2020.
Grell, G. A.: Prognostic Evaluation of Assumptions Used by Cumulus Parameterizations, Mon. Weather Rev., 121, 764–787, https://doi.org/10.1175/1520-0493(1993)121<0764:PEOAUB>2.0.CO;2, 1993.
Guo, S., Hu, M., Zamora, M. L., Peng, J., Shang, D., Zheng, J., Du, Z., Wu, Z., Shao, M., Zeng, L., Molina, M. J., and Zhang, R.: Elucidating severe urban haze formation in China, P. Natl. Acad. Sci. USA, 111, 17373–17378, https://doi.org/10.1073/pnas.1419604111, 2014.
Hong, S.-Y., Noh, Y., and Dudhia, J.: A New Vertical Diffusion Package with an Explicit Treatment of Entrainment Processes, Mon. Weather Rev., 134, 2318–2341, https://doi.org/10.1175/MWR3199.1, 2006.
Hudson, J. and Noble, S.: CCN and Vertical Velocity Influences on Droplet Concentrations and Supersaturations in Clean and Polluted Stratus Clouds, J. Atmos. Sci., 71, 312–331, https://doi.org/10.1175/JAS-D-13-086.1, 2014.
Jimenez, J. L., Canagaratna, M. R., Donahue, N. M., Prevot, A. S. H., Zhang, Q., Kroll, J. H., DeCarlo, P. F., Allan, J. D., Coe, H., Ng, N. L., Aiken, A. C., Docherty, K. S., Ulbrich, I. M., Grieshop, A. P., Robinson, A. L., Duplissy, J., Smith, J. D., Wilson, K. R., Lanz, V. A., Hueglin, C., Sun, Y. L., Tian, J., Laaksonen, A., Raatikainen, T., Rautiainen, J., Vaattovaara, P., Ehn, M., Kulmala, M., Tomlinson, J. M., Collins, D. R., Cubison, M. J., E, Dunlea, J., Huffman, J. A., Onasch, T. B., Alfarra, M. R., Williams, P. I., Bower, K., Kondo, Y., Schneider, J., Drewnick, F., Borrmann, S., Weimer, S., Demerjian, K., Salcedo, D., Cottrell, L., Griffin, R., Takami, A., Miyoshi, T., Hatakeyama, S., Shimono, A., Sun, J. Y., Zhang, Y. M., Dzepina, K., Kimmel, J. R., Sueper, D., Jayne, J. T., Herndon, S. C., Trimborn, A. M., Williams, L. R., Wood, E. C., Middlebrook, A. M., Kolb, C. E., Baltensperger, U., and Worsnop, D. R.: Evolution of Organic Aerosols in the Atmosphere, Science, 326, 1525–1529, https://doi.org/10.1126/science.1180353, 2009.
Kalkavouras, P., Bougiatioti, A., Kalivitis, N., Stavroulas, I., Tombrou, M., Nenes, A., and Mihalopoulos, N.: Regional new particle formation as modulators of cloud condensation nuclei and cloud droplet number in the eastern Mediterranean, Atmos. Chem. Phys., 19, 6185–6203, https://doi.org/10.5194/acp-19-6185-2019, 2019.
Kerminen, V.-M., Chen, X., Vakkari, V., Petäjä, T., Kulmala, M., and Bianchi, F.: Atmospheric new particle formation and growth: Review of field observations, Environ. Res. Lett., 13, 03003, https://doi.org/10.1088/1748-9326/aadf3c, 2018.
Krechmer, J. E., Day, D. A., Ziemann, P. J., and Jimenez, J. L.: Direct Measurements of Gas/Particle Partitioning and Mass Accommodation Coefficients in Environmental Chambers, Environ. Sci. Technol., 51, 11867–11875, https://doi.org/10.1021/acs.est.7b02144, 2017.
Kulmala, M., Laakso, L., Lehtinen, K. E. J., Riipinen, I., Dal Maso, M., Anttila, T., Kerminen, V. M., Hõrrak, U., Vana, M., and Tammet, H.: Initial steps of aerosol growth, Atmos. Chem. Phys., 4, 2553–2560, https://doi.org/10.5194/acp-4-2553-2004, 2004.
Kulmala, M., Petäjä, T., Nieminen, T., Sipilä, M., Manninen, H. E., Lehtipalo, K., Dal Maso, M., Aalto, P. P., Junninen, H., Paasonen, P., Riipinen, I., Lehtinen, K. E. J., Laaksonen, A., and Kerminen, V.-M.: Measurement of the nucleation of atmospheric aerosol particles, Nat. Protoc., 7, 1651–1667, https://doi.org/10.1038/nprot.2012.091, 2012.
Kulmala, M., Petäjä, T., Ehn, M., Thornton, J., Sipilä, M., Worsnop, D. R., and Kerminen, V.-M.: Chemistry of Atmospheric Nucleation: On the Recent Advances on Precursor Characterization and Atmospheric Cluster Composition in Connection with Atmospheric New Particle Formation, Annu. Rev. Phys. Chem., 65, 21–37, https://doi.org/10.1146/annurev-physchem-040412-110014, 2013.
Kulmala, M., Dada, L., Daellenbach, K. R., Yan, C., Stolzenburg, D., Kontkanen, J., Ezhova, E., Hakala, S., Tuovinen, S., Kokkonen, T. V., Kurppa, M., Cai, R., Zhou, Y., Yin, R., Baalbaki, R., Chan, T., Chu, B., Deng, C., Fu, Y., Ge, M., He, H., Heikkinen, L., Junninen, H., Liu, Y., Lu, Y., Nie, W., Rusanen, A., Vakkari, V., Wang, Y., Yang, G., Yao, L., Zheng, J., Kujansuu, J., Kangasluoma, J., Petäjä, T., Paasonen, P., Järvi, L., Worsnop, D., Ding, A., Liu, Y., Wang, L., Jiang, J., Bianchi, F., and Kerminen, V.-M.: Is reducing new particle formation a plausible solution to mitigate particulate air pollution in Beijing and other Chinese megacities?, Faraday Discuss., 226, 334–347, https://doi.org/10.1039/D0FD00078G, 2021.
Lai, S., Hai, S., Gao, Y., Wang, Y., Sheng, L., Lupascu, A., Ding, A., Nie, W., Qi, X., Huang, X., Chi, X., Zhao, C., Zhao, B., Shrivastava, M., Fast, J. D., Yao, X., and Gao, H.: The striking effect of vertical mixing in the planetary boundary layer on new particle formation in the Yangtze River Delta, Sci. Total Environ., 829, 154607, https://doi.org/10.1016/j.scitotenv.2022.154607, 2022.
Lee, S.-H., Gordon, H., Yu, H., Lehtipalo, K., Haley, R., Li, Y., and Zhang, R.: New Particle Formation in the Atmosphere: From Molecular Clusters to Global Climate, J. Geophys. Res, 124, 7098–7146, https://doi.org/10.1029/2018JD029356, 2019.
Li, K., Zhu, Y., Gao, H., and Yao, X.: A comparative study of cloud condensation nuclei measured between non-heating and heating periods at a suburb site of Qingdao in the North China, Atmos. Environ., 112, 40–53, https://doi.org/10.1016/j.atmosenv.2015.04.024, 2015.
Li, M., Liu, H., Geng, G., Hong, C., Liu, F., Song, Y., Tong, D., Zheng, B., Cui, H., Man, H., Zhang, Q., and He, K.: Anthropogenic emission inventories in China: a review, Natl. Sci. Rev., 4, 834–866, https://doi.org/10.1093/nsr/nwx150, 2017.
Li, X., Li, Y., Cai, R., Yan, C., Qiao, X., Guo, Y., Deng, C., Yin, R., Chen, Y., Li, Y., Yao, L., Sarnela, N., Zhang, Y., Petäjä, T., Bianchi, F., Liu, Y., Kulmala, M., Hao, J., Smith, J. N., and Jiang, J.: Insufficient Condensable Organic Vapors Lead to Slow Growth of New Particles in an Urban Environment, Environ. Sci. Technol., 56, 9936–9946, https://doi.org/10.1021/acs.est.2c01566, 2022.
Liu, H. J., Zhao, C. S., Nekat, B., Ma, N., Wiedensohler, A., van Pinxteren, D., Spindler, G., Müller, K., and Herrmann, H.: Aerosol hygroscopicity derived from size-segregated chemical composition and its parameterization in the North China Plain, Atmos. Chem. Phys., 14, 2525–2539, https://doi.org/10.5194/acp-14-2525-2014, 2014a.
Liu, M. and Matsui, H.: Secondary Organic Aerosol Formation Regulates Cloud Condensation Nuclei in the Global Remote Troposphere, Geophys. Res. Lett., 49, e2022GL100543, https://doi.org/10.1029/2022GL100543, 2022.
Liu, X. H., Zhu, Y. J., Zheng, M., Gao, H. W., and Yao, X. H.: Production and growth of new particles during two cruise campaigns in the marginal seas of China, Atmos. Chem. Phys., 14, 7941–7951, https://doi.org/10.5194/acp-14-7941-2014, 2014b.
Lovejoy, E. R., Curtius, J., and Froyd, K. D.: Atmospheric ion-induced nucleation of sulfuric acid and water, J. Geophys. Res.-Atmos., 109, D08204, https://doi.org/10.1029/2003JD004460, 2004.
Lu, H., Wang, G., and Guo, H.: Ambient acidic ultrafine particles in different land-use areas in two representative Chinese cities, Sci. Total Environ., 830, 154774, https://doi.org/10.1016/j.scitotenv.2022.154774, 2022.
Lu, Y., Yan, C., Fu, Y., Chen, Y., Liu, Y., Yang, G., Wang, Y., Bianchi, F., Chu, B., Zhou, Y., Yin, R., Baalbaki, R., Garmash, O., Deng, C., Wang, W., Liu, Y., Petäjä, T., Kerminen, V. M., Jiang, J., Kulmala, M., and Wang, L.: A proxy for atmospheric daytime gaseous sulfuric acid concentration in urban Beijing, Atmos. Chem. Phys., 19, 1971–1983, https://doi.org/10.5194/acp-19-1971-2019, 2019.
Lupascu, A., Easter, R., Zaveri, R., Shrivastava, M., Pekour, M., Tomlinson, J., Yang, Q., Matsui, H., Hodzic, A., Zhang, Q., and Fast, J. D.: Modeling particle nucleation and growth over northern California during the 2010 CARES campaign, Atmos. Chem. Phys., 15, 12283–12313, https://doi.org/10.5194/acp-15-12283-2015, 2015.
Ma, N., Zhao, C., Tao, J., Wu, Z., Kecorius, S., Wang, Z., Größ, J., Liu, H., Bian, Y., Kuang, Y., Teich, M., Spindler, G., Müller, K., van Pinxteren, D., Herrmann, H., Hu, M., and Wiedensohler, A.: Variation of CCN activity during new particle formation events in the North China Plain, Atmos. Chem. Phys., 16, 8593–8607, https://doi.org/10.5194/acp-16-8593-2016, 2016.
Matsui, H., Koike, M., Kondo, Y., Takegawa, N., Wiedensohler, A., Fast, J. D., and Zaveri, R. A.: Impact of new particle formation on the concentrations of aerosols and cloud condensation nuclei around Beijing, J. Geophys. Res.-Atmos., 116, D19208, https://doi.org/10.1029/2011JD016025, 2011.
Matsui, H., Koike, M., Takegawa, N., Kondo, Y., Takami, A., Takamura, T., Yoon, S., Kim, S. W., Lim, H. C., and Fast, J. D.: Spatial and temporal variations of new particle formation in East Asia using an NPF-explicit WRF-chem model: North-south contrast in new particle formation frequency, J. Geophys. Res.-Atmos., 118, 11647–611663, https://doi.org/10.1002/jgrd.50821, 2013.
Merikanto, J., Napari, I., Vehkamäki, H., Anttila, T., and Kulmala, M.: New parameterization of sulfuric acid-ammonia-water ternary nucleation rates at tropospheric conditions, J. Geophys. Res.-Atmos., 112, D15207, https://doi.org/10.1029/2006JD007977, 2007.
Merikanto, J., Spracklen, D. V., Mann, G. W., Pickering, S. J., and Carslaw, K. S.: Impact of nucleation on global CCN, Atmos. Chem. Phys., 9, 8601–8616, https://doi.org/10.5194/acp-9-8601-2009, 2009.
Mikkonen, S., Romakkaniemi, S., Smith, J. N., Korhonen, H., Petäjä, T., Plass-Duelmer, C., Boy, M., McMurry, P. H., Lehtinen, K. E. J., Joutsensaari, J., Hamed, A., Mauldin Iii, R. L., Birmili, W., Spindler, G., Arnold, F., Kulmala, M., and Laaksonen, A.: A statistical proxy for sulphuric acid concentration, Atmos. Chem. Phys., 11, 11319–11334, https://doi.org/10.5194/acp-11-11319-2011, 2011.
Morrison, H., Thompson, G., and Tatarskii, V.: Impact of Cloud Microphysics on the Development of Trailing Stratiform Precipitation in a Simulated Squall Line: Comparison of One and Two-Moment Schemes, Mon. Weather Rev., 137, 991–1007, https://doi.org/10.1175/2008MWR2556.1, 2009.
Nieminen, T., Kerminen, V. M., Petäjä, T., Aalto, P. P., Arshinov, M., Asmi, E., Baltensperger, U., Beddows, D. C. S., Beukes, J. P., Collins, D., Ding, A., Harrison, R. M., Henzing, B., Hooda, R., Hu, M., Hõrrak, U., Kivekäs, N., Komsaare, K., Krejci, R., Kristensson, A., Laakso, L., Laaksonen, A., Leaitch, W. R., Lihavainen, H., Mihalopoulos, N., Németh, Z., Nie, W., O'Dowd, C., Salma, I., Sellegri, K., Svenningsson, B., Swietlicki, E., Tunved, P., Ulevicius, V., Vakkari, V., Vana, M., Wiedensohler, A., Wu, Z., Virtanen, A., and Kulmala, M.: Global analysis of continental boundary layer new particle formation based on long-term measurements, Atmos. Chem. Phys., 18, 14737–14756, https://doi.org/10.5194/acp-18-14737-2018, 2018.
Petäjä, T., Mauldin, I. R. L., Kosciuch, E., McGrath, J., Nieminen, T., Paasonen, P., Boy, M., Adamov, A., Kotiaho, T., and Kulmala, M.: Sulfuric acid and OH concentrations in a boreal forest site, Atmos. Chem. Phys., 9, 7435–7448, https://doi.org/10.5194/acp-9-7435-2009, 2009.
Petters, M. D. and Kreidenweis, S. M.: A single parameter representation of hygroscopic growth andhttps://doi.org/10.5194/acp-7-1961-2007, 2007.
Pierce, J. R., Riipinen, I., Kulmala, M., Ehn, M., Petäjä, T., Junninen, H., Worsnop, D. R., and Donahue, N. M.: Quantification of the volatility of secondary organic compounds in ultrafine particles during nucleation events, Atmos. Chem. Phys., 11, 9019–9036, https://doi.org/10.5194/acp-11-9019-2011, 2011.
Pöschl, U., Canagaratna, M., Jayne, J. T., Molina, L. T., Worsnop, D. R., Kolb, C. E., and Molina, M. J.: Mass Accommodation Coefficient of H2SO4 Vapor on Aqueous Sulfuric Acid Surfaces and Gaseous Diffusion Coefficient of H2SO4 in N2 H2O, J. Phys. Chem. A, 102, 10082–10089, https://doi.org/10.1021/jp982809s, 1998.
Qiao, X., Yan, C., Li, X., Guo, Y., Yin, R., Deng, C., Li, C., Nie, W., Wang, M., Cai, R., Huang, D., Wang, Z., Yao, L., Worsnop, D. R., Bianchi, F., Liu, Y., Donahue, N. M., Kulmala, M., and Jiang, J.: Contribution of Atmospheric Oxygenated Organic Compounds to Particle Growth in an Urban Environment, Environ. Sci. Technol., 55, 13646–13656, https://doi.org/10.1021/acs.est.1c02095, 2021.
Ren, J., Chen, L., Fan, T., Liu, J., Jiang, S., and Zhang, F.: The NPF Effect on CCN Number Concentrations: A Review and Re-Evaluation of Observations From 35 Sites Worldwide, Geophys. Res. Lett., 48, e2021GL095190, https://doi.org/10.1029/2021GL095190, 2021.
Riipinen, I., Sihto, S.-L., Kulmala, M., Arnold, F., Dal Maso, M., Birmili, W., Saarnio, K., Teinilä, K., Kerminen, V.-M., Laaksonen, A., and Lehtinen, K. E. J.: Connections between atmospheric sulphuric acid and new particle formation during QUEST III–IV campaigns in Heidelberg and Hyytiälä, Atmos. Chem. Phys., 7, 1899–1914, https://doi.org/10.5194/acp-7-1899-2007, 2007.
Roldin, P., Swietlicki, E., Massling, A., Kristensson, A., Löndahl, J., Eriksson, A., Pagels, J., and Gustafsson, S.: Aerosol ageing in an urban plume – implication for climate, Atmos. Chem. Phys., 11, 5897–5915, https://doi.org/10.5194/acp-11-5897-2011, 2011a.
Roldin, P., Swietlicki, E., Schurgers, G., Arneth, A., Lehtinen, K. E. J., Boy, M., and Kulmala, M.: Development and evaluation of the aerosol dynamics and gas phase chemistry model ADCHEM, Atmos. Chem. Phys., 11, 5867–5896, https://doi.org/10.5194/acp-11-5867-2011, 2011b.
Saha, S., Moorthi, S., Wu, X., Wang, J., Nadiga, S., Tripp, P., Behringer, D., Hou, Y.-T., Chuang, H.-y., Iredell, M., Ek, M., Meng, J., Yang, R., Mendez, M. P., van den Dool, H., Zhang, Q., Wang, W., Chen, M., and Becker, E.: The NCEP Climate Forecast System Version 2, J. Clim., 27, 2185–2208, https://doi.org/10.1175/JCLI-D-12-00823.1, 2014.
Shen, X., Sun, J., Zhang, X., Zhang, Y., Wang, Y., Tan, K., Wang, P., Zhang, L., Qi, X., Che, H., Zhang, Z., Zhong, J., Zhao, H., and Ren, S.: Comparison of Submicron Particles at a Rural and an Urban Site in the North China Plain during the December 2016 Heavy Pollution Episodes, J. Meteorol. Res., 32, 26–37, https://doi.org/10.1007/s13351-018-7060-7, 2018.
Shrivastava, M., Fast, J., Easter, R., Gustafson Jr., W. I., Zaveri, R. A., Jimenez, J. L., Saide, P., and Hodzic, A.: Modeling organic aerosols in a megacity: comparison of simple and complex representations of the volatility basis set approach, Atmos. Chem. Phys., 11, 6639–6662, https://doi.org/10.5194/acp-11-6639-2011, 2011.
Shrivastava, M. K., Lane, T. E., Donahue, N. M., Pandis, S. N., and Robinson, A. L.: Effects of gas particle partitioning and aging of primary emissions on urban and regional organic aerosol concentrations, J. Geophys. Res.-Atmos., 113, D18301, https://doi.org/10.1029/2007JD009735, 2008.
Sihto, S. L., Kulmala, M., Kerminen, V. M., Dal Maso, M., Petäjä, T., Riipinen, I., Korhonen, H., Arnold, F., Janson, R., Boy, M., Laaksonen, A., and Lehtinen, K. E. J.: Atmospheric sulphuric acid and aerosol formation: implications from atmospheric measurements for nucleation and early growth mechanisms, Atmos. Chem. Phys., 6, 4079–4091, https://doi.org/10.5194/acp-6-4079-2006, 2006.
Sihto, S. L., Mikkilä, J., Vanhanen, J., Ehn, M., Liao, L., Lehtipalo, K., Aalto, P. P., Duplissy, J., Petäjä, T., Kerminen, V. M., Boy, M., and Kulmala, M.: Seasonal variation of CCN concentrations and aerosol activation properties in boreal forest, Atmos. Chem. Phys., 11, 13269–13285, https://doi.org/10.5194/acp-11-13269-2011, 2011.
Tewari, M., Chen, F., Wang, W., Dudhia, J., LeMone, M. A., Mitchell, K., Ek, M., Gayno, G., Wegiel, J., and Cuenca, R. H.: Implementation and verification of the unified noah land surface model in the WRF model [presentation], in: 20th Conference on Weather Analysis and Forecasting/16th Conference on Numerical Weather Prediction, 11–15, http://n2t.net/ark:/85065/d7fb523p (last access: 20 June 2022), 2004.
Virtanen, A., Kannosto, J., Kuuluvainen, H., Arffman, A., Joutsensaari, J., Saukko, E., Hao, L., Yli-Pirilä, P., Tiitta, P., Holopainen, J. K., Keskinen, J., Worsnop, D. R., Smith, J. N., and Laaksonen, A.: Bounce behavior of freshly nucleated biogenic secondary organic aerosol particles, Atmos. Chem. Phys., 11, 8759–8766, https://doi.org/10.5194/acp-11-8759-2011, 2011.
Wang, D.-W., Guo, H., and Chan, C.: Diffusion Sampler for Measurement of Acidic Ultrafine Particles in the Atmosphere, Aerosol Sci. Technol., 48, 1236–1246, https://doi.org/10.1080/02786826.2014.978937, 2014.
Wang, J., Li, M., Li, L., Zheng, R., Fan, X., Hong, Y., Xu, L., Chen, J., and Hu, B.: Particle number size distribution and new particle formation in Xiamen, the coastal city of Southeast China in wintertime, Sci. Total Environ., 826, 154208, https://doi.org/10.1016/j.scitotenv.2022.154208, 2022.
Wang, Y., Wang, Y., Song, X., Shang, Y., Zhou, Y., Huang, X., and Li, Z.: The impact of particulate pollution control on aerosol hygroscopicity and CCN activity in North China, Environ. Res. Lett., 18, 074028, https://doi.org/10.1088/1748-9326/acde91, 2023.
Westervelt, D. M., Pierce, J. R., Riipinen, I., Trivitayanurak, W., Hamed, A., Kulmala, M., Laaksonen, A., Decesari, S., and Adams, P. J.: Formation and growth of nucleated particles into cloud condensation nuclei: model–measurement comparison, Atmos. Chem. Phys., 13, 7645–7663, https://doi.org/10.5194/acp-13-7645-2013, 2013.
Wu, H., Li, Z., Jiang, M., Liang, C., Zhang, D., Wu, T., Wang, Y., and Cribb, M.: Contributions of traffic emissions and new particle formation to the ultrafine particle size distribution in the megacity of Beijing, Atmos. Environ., 262, 118652, https://doi.org/10.1016/j.atmosenv.2021.118652, 2021a.
Wu, L., Ling, Z., Shao, M., Liu, H., Lu, S., Zhou, S., Guo, J., Mao, J., Hang, J., and Wang, X.: Roles of Semivolatile/Intermediate-Volatility Organic Compounds on SOA Formation Over China During a Pollution Episode: Sensitivity Analysis and Implications for Future Studies, J. Geophys. Res.-Atmos., 126, e2020JD033999, https://doi.org/10.1029/2020JD033999, 2021b.
Wu, Z., Hu, M., Yue, D., Wehner, B., and Wiedensohler, A.: Evolution of particle number size distribution in an urban atmosphere during episodes of heavy pollution and new particle formation, Sci. China Earth Sci., 54, 1772, https://doi.org/10.1007/s11430-011-4227-9, 2011.
Wu, Z. J., Poulain, L., Birmili, W., Größ, J., Niedermeier, N., Wang, Z. B., Herrmann, H., and Wiedensohler, A.: Some insights into the condensing vapors driving new particle growth to CCN sizes on the basis of hygroscopicity measurements, Atmos. Chem. Phys., 15, 13071–13083, https://doi.org/10.5194/acp-15-13071-2015, 2015.
Yao, L., Garmash, O., Bianchi, F., Zheng, J., Yan, C., Kontkanen, J., Junninen, H., Mazon, S., Ehn, M., Paasonen, P., Sipilä, M., Wang, M., Wang, X., Xiao, S., Chen, H., Lu, Y., Zhang, B., Wang, D., Fu, Q., and Wang, L.: Atmospheric new particle formation from sulfuric acid and amines in a Chinese megacity, Science, 361, 278–281, https://doi.org/10.1126/science.aao4839, 2018.
Yu, F.: Quasi-unary homogeneous nucleation of H2SO4-H2O, J. Chem. Phys., 122, 074501, https://doi.org/10.1063/1.1850472, 2005.
Yu, F., Luo, G., Pryor, S. C., Pillai, P. R., Lee, S. H., Ortega, J., Schwab, J. J., Hallar, A. G., Leaitch, W. R., Aneja, V. P., Smith, J. N., Walker, J. T., Hogrefe, O., and Demerjian, K. L.: Spring and summer contrast in new particle formation over nine forest areas in North America, Atmos. Chem. Phys., 15, 13993–14003, https://doi.org/10.5194/acp-15-13993-2015, 2015.
Yu, F., Luo, G., Nair, A. A., Schwab, J. J., Sherman, J. P., and Zhang, Y.: Wintertime new particle formation and its contribution to cloud condensation nuclei in the Northeastern United States, Atmos. Chem. Phys., 20, 2591–2601, https://doi.org/10.5194/acp-20-2591-2020, 2020.
Yuan, Q., Li, W., Zhou, S., Yang, L., Chi, J., Sui, X., and Wang, W.: Integrated evaluation of aerosols during haze-fog episodes at one regional background site in North China Plain, Atmos. Res., 156, 102–110, https://doi.org/10.1016/j.atmosres.2015.01.002, 2015.
Yue, D. L., Hu, M., Zhang, R. Y., Wu, Z. J., Su, H., Wang, Z. B., Peng, J. F., He, L. Y., Huang, X. F., Gong, Y. G., and Wiedensohler, A.: Potential contribution of new particle formation to cloud condensation nuclei in Beijing, Atmos. Environ., 45, 6070–6077, https://doi.org/10.1016/j.atmosenv.2011.07.037, 2011.
Zaveri, R. A., Easter, R. C., and Peters, L. K.: A computationally efficient Multicomponent Equilibrium Solver for Aerosols (MESA), J. Geophys. Res.-Atmos., 110, D24203, https://doi.org/10.1029/2004JD005618, 2005.
Zaveri, R. A., Easter, R. C., Fast, J. D., and Peters, L. K.: Model for Simulating Aerosol Interactions and Chemistry (MOSAIC), J. Geophys. Res.-Atmos., 113, 1–29, https://doi.org/10.1029/2007JD008782, 2008.
Zhang, Q., Jimenez, J. L., Canagaratna, M. R., Allan, J. D., Coe, H., Ulbrich, I., Alfarra, M. R., Takami, A., Middlebrook, A. M., Sun, Y. L., Dzepina, K., Dunlea, E., Docherty, K., DeCarlo, P. F., Salcedo, D., Onasch, T., Jayne, J. T., Miyoshi, T., Shimono, A., Hatakeyama, S., Takegawa, N., Kondo, Y., Schneider, J., Drewnick, F., Borrmann, S., Weimer, S., Demerjian, K., Williams, P., Bower, K., Bahreini, R., Cottrell, L., Griffin, R. J., Rautiainen, J., Sun, J. Y., Zhang, Y. M., and Worsnop, D. R.: Ubiquity and dominance of oxygenated species in organic aerosols in anthropogenically-influenced Northern Hemisphere midlatitudes, Geophys. Res. Lett., 34, L13801, https://doi.org/10.1029/2007GL029979, 2007.
Zhao, B., Wang, S., Donahue, N. M., Jathar, S. H., Huang, X., Wu, W., Hao, J., and Robinson, A. L.: Quantifying the effect of organic aerosol aging and intermediate-volatility emissions on regional-scale aerosol pollution in China, Sci. Rep., 6, 28815, https://doi.org/10.1038/srep28815, 2016.
Zhao, B., Shrivastava, M., Donahue, N. M., Gordon, H., Schervish, M., Shilling, J. E., Zaveri, R. A., Wang, J., Andreae, M. O., Zhao, C., Gaudet, B., Liu, Y., Fan, J., and Fast, J. D.: High concentration of ultrafine particles in the Amazon free troposphere produced by organic new particle formation, P. Natl. Acad. Sci. USA, 117, 25344–25351, https://doi.org/10.1073/pnas.2006716117, 2020.
Zheng, B., Tong, D., Li, M., Liu, F., Hong, C., Geng, G., Li, H., Li, X., Peng, L., Qi, J., Yan, L., Zhang, Y., Zhao, H., Zheng, Y., He, K., and Zhang, Q.: Trends in China's anthropogenic emissions since 2010 as the consequence of clean air actions, Atmos. Chem. Phys., 18, 14095–14111, https://doi.org/10.5194/acp-18-14095-2018, 2018.
Zhu, Y., Sabaliauskas, K., Liu, X., Meng, H., Gao, H., Jeong, C.-H., Evans, G. J., and Yao, X.: Comparative analysis of new particle formation events in less and severely polluted urban atmosphere, Atmospheric Environ., 98, 655–664, https://doi.org/10.1016/j.atmosenv.2014.09.043, 2014.
Zhu, Y., Li, K., Shen, Y., Gao, Y., Liu, X., Yu, Y., Gao, H., and Yao, X.: New particle formation in the marine atmosphere during seven cruise campaigns, Atmos. Chem. Phys., 19, 89–113, https://doi.org/10.5194/acp-19-89-2019, 2019.
Zhu, Y., Shen, Y., Li, K., Meng, H., Sun, Y., Yao, X., Gao, H., Xue, L., and Wang, W.: Investigation of Particle Number Concentrations and New Particle Formation With Largely Reduced Air Pollutant Emissions at a Coastal Semi-Urban Site in Northern China, J. Geophys. Res.-Atmos., 126, e2021JD035419, https://doi.org/10.1029/2021JD035419, 2021.