the Creative Commons Attribution 4.0 License.
the Creative Commons Attribution 4.0 License.
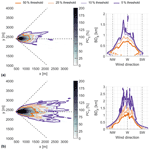
Assessing the representativity of NH3 measurements influenced by boundary-layer dynamics and the turbulent dispersion of a nearby emission source
Margreet C. van Zanten
Bart J. H. van Stratum
Jordi Vilà-Guerau de Arellano
This study presents a fine-scale simulation approach to assess the representativity of ammonia (NH3) measurements in the proximity of an emission source. Close proximity to emission sources (< 5 km) can introduce a bias in regionally representative measurements of the NH3 molar fraction and flux. Measurement sites should, therefore, be located a significant distance away from emission sources, but these requirements are poorly defined and can be difficult to meet in densely agricultural regions. This study presents a consistent criterion to assess the regional representativity of NH3 measurements in proximity to an emission source, calculating variables that quantify the NH3 plume dispersion using a series of numerical experiments at a fine resolution (20 m). Our fine-scale simulation framework with explicitly resolved turbulence enables us to distinguish between the background NH3 and the emission plume, including realistic representations of NH3 deposition and chemical gas–aerosol transformations. We introduce the concept of blending distance based on the calculation of turbulent fluctuations to systematically analyze the impact of the emission plume on simulated measurements, relative to this background NH3. We perform a suite of systematic numerical experiments for flat homogeneous grasslands, centered around the CESAR Observatory at Cabauw, to analyze the sensitivity of the blending distance, varying meteorological factors, emission/deposition and NH3 dependences. Considering these sensitivities, we find that NH3 measurements at this measurement site should be located at a minimum distance of 0.5–3.0 and 0.75–4.5 km from an emission source for NH3 molar fraction and flux measurements, respectively. The simulation framework presented here can easily be adapted to local conditions, and paves the way for future ammonia research to integrate simulations at high spatio-temporal resolutions with observations of NH3 concentrations and fluxes.
- Article
(7241 KB) - Full-text XML
- BibTeX
- EndNote
Excess atmospheric nitrogen leads to an increased public health risk through the formation of particulate matter and causes environmental damage; nitrogen deposition leads to eutrophication, ecosystem acidification and shifts in climate change (Erisman and Schaap, 2004; Sutton et al., 2008; Behera et al., 2013; Erisman et al., 2013; Smit and Heederik, 2017). There can be serious societal consequences when nitrogen deposition critical loads are exceeded, as is the case in the Netherlands where the nitrogen crisis threatens the Dutch environment and economy (Stokstad, 2019). Atmospheric ammonia (NH3) plays a key role in this process, mainly originating from agricultural activities and accounting for two-thirds of all nitrogen deposition in the Netherlands between 2005 and 2016 (Wichink Kruit and van Pul, 2018).
It is therefore important to have a network of NH3 concentration and deposition measurements used for model validation and (trend) monitoring (Wichink Kruit et al., 2021). For these purposes, the measurement sites in such a network must be representative of a larger region. One requirement for these regional measurement sites is that they are located a sufficient distance away from local NH3 sources, as local emissions introduce a bias in observations (EMEP/CCC, 2001; Wichink Kruit et al., 2021). Positioning measurement sites a sufficient distance away from local sources is a challenge in densely agricultural areas like the Netherlands and regions all across the world with intensive livestock farming, e.g., North-West Germany, the province of Lleida in Spain, the state of North Carolina in the USA or the Hai River basin in China.
The emitted NH3 is transported and mixed within the convective boundary layer (CBL) through turbulent dispersion. The field of turbulent plume dispersion is extensively researched using both observations and turbulent-resolved models. However, these studies typically focus on concentration peaks of highly toxic/flammable gases (Mylne and Mason, 1991; Ardeshiri et al., 2021; Cassiani et al., 2020), quantification of the emission strength and position (Shah et al., 2020; Ražnjević et al., 2022), or on statistical descriptions of the emission plume (Barad, 1958; Dosio et al., 2003; Vrieling and Nieuwstadt, 2003; Dosio and Vilà-Guerau de Arellano, 2006), typically used in chemistry transport models, e.g., OPS (Sauter et al., 2018), LOTOS-EUROS (Schaap et al., 2008) or EMEP MSC-W (Simpson et al., 2012). These transport models typically operate with resolutions at a kilometer scale (1–50 km) and parameterized turbulence, making them unsuitable to study the impact of local NH3 sources on nearby measurement sites at the subkilometer scale.
Furthermore, plume dispersion studies generally focus on chemically inert gases, e.g., methane (Shah et al., 2020; Ražnjević et al., 2022). Ammonia is highly reactive; surface–atmosphere exchanges and chemical gas–aerosol transformations play an important role in the NH3 budget (Fowler et al., 1998; Van Oss et al., 1998; Nemitz et al., 2004; aan de Brugh et al., 2013; Behera et al., 2013; Shen et al., 2016; Schulte et al., 2021). Additionally, ammonia emissions in densely agricultural areas are released and mixed into a background concentration, a result of long range transport of NH3 (10–100 km). Yearly averaged background concentrations can vary from 1–2 µg m−3 (e.g., in coastal regions) up to up to tens of µg m−3 in regions with intensive agricultural activity, which is the focus on this study (van Zanten et al., 2017).
In this study, we investigate the impact of a typical ammonia emission source on the regional representativeness of NH3 concentration and flux measurements. The novelty of our approach is twofold:
-
We use a fine-scale large-eddy simulation (LES) model with explicitly resolved turbulence at a very high spatio-temporal resolution (10–100 m and 10 s–1 min).
-
We include realistic representations of surface–atmosphere exchanges, chemical gas–aerosol transformations and a background ammonia concentration.
Following this approach, we combine fine-scale simulations, where turbulence is explicitly resolved, with theoretical concepts on turbulent emission plume dispersion. We then translate this knowledge to practical applications for the measurement community. The aim is to carry out a systematic analysis of how meteorological factors, including boundary-layer dynamics, deposition, chemical transformation and model resolution influence the relationships between emission and receptor. To this end, we introduce and analyze the concept of a blending distance (BD), i.e., the horizontal distance at which the emission plume can be considered well-mixed with respect to the background NH3. With the concept of blending distance, we aim to provide an estimate of the minimum required distance from a typical NH3 emission source for regionally representative measurements.
2.1 NH3 turbulent dispersion in DALES
To understand the variations of the NH3 budget due to turbulence and heterogeneous sources and sinks of ammonia, our approach is twofold: (a) an explicit simulation of processes that govern turbulent dispersion and mixing of NH3 and (b) identifying their individual contributions to the NH3 molar fraction and surface–atmosphere exchange. For the former, we use the large-eddy simulation technique, with explicitly resolved turbulence, at very high resolution. To this end, we conduct our numerical experiments using a modified version of the Dutch Atmospheric Large-Eddy Simulation (DALES) version 4.2 (Heus et al., 2010; Ouwersloot et al., 2017), with the original v4.2 freely available online (https://doi.org/10.5281/zenodo.3759193, Arabas et al., 2020). DALES explicitly resolves processes at scales ranging from 100 meters to kilometers, using filtered Navier–Stokes equations with the Boussinesq approximation. The filter size is generally equal to the grid size of the simulations, with subfilter-scale processes being parameterized using one-and-a-half-order closure. The numerical experiments presented here are performed using a 20 m × 20 m × 5 m grid for a 10 km × 4.8 km × 3 km domain (500 × 240 × 600 grid points). Atmospheric NH3 is added to DALES as a passive scalar in ppb, of which the spatial evolution is solved simultaneously with the thermodynamic variables. The boundary conditions for scalars and meteorological variables are periodic, unless stated otherwise.
The atmospheric ammonia budget is further governed by surface–atmosphere exchanges and chemical gas–particle transformations (Schulte et al., 2021). We use a simplified yet realistic approach in our representation of these processes. The NH3 surface–atmosphere exchange is modeled by a constant homogeneous deposition of 0.045 ppb m s−1 (about 0.032 µg m−2 s−1), representative of the observed yearly average NH3 dry deposition in the Netherlands (https://www.rivm.nl/stikstof/meten/drogedepositieNH3, last access: 23 June 2022; Stolk et al., 2014).
The representation of the chemical gas–aerosol transformations follows the approach of the OPS model, applying a percentage per hour change in the molar fraction of gaseous NH3 to the whole domain (van Jaarsveld, 2004). This simplified yet realistic representation of chemistry as a net removal process will reduce the reach of the emission plume. However, the model is unable to resolve potential nonlinear effects of turbulent mixing on the chemical reaction rate within the plume. Turbulent dispersion of the emission plume is characterized by macromixing (meandering) and micromixing (in-plume mixing) (Vilà-Guerau de Arellano et al., 1990; Galmarini et al., 1995). The former is mainly carried out by large-scale turbulent eddies and is related to the average dispersion of the plume. Micromixing is carried out by turbulent eddies smaller than the plume and is related to the fluctuations of NH3 and its chemical reactants. The reaction rate can slow down close to the emission source, as macromixing is the dominant dispersion process here and little micromixing occurs to supply chemical reactants from outside the plume. The extent to which turbulent mixing can limit the chemical reactions within the plume depends on the ratio of the turbulent timescales and the timescale of chemistry (Damköhler number) (Galmarini et al., 1995; Meeder and Nieuwstadt, 2000). When the timescales of chemistry are similar to the turbulent timescales, as is the case for ammonia (aan de Brugh et al., 2013), the reduction in the chemical reaction rate close to the source can be significant (Vilà-Guerau de Arellano et al., 2004).
Special attention is placed on the representation of one NH3 emission source in our domain, a dairy barn. Agricultural activity accounts for over 90 % of the NH3 emissions in the Netherlands and the European Union (Anys et al., 2020; Vonk et al., 2020; van Bruggen et al., 2021). Dairy farms account for approximately 50 % of these agricultural NH3 emissions, with approximately 15 000 farms with about 100 cows each on average in the Netherlands (van der Peet et al., 2018; WUR, 2021). A typical cubicle stable for 80 cows has a yearly emission of about 800 kg NH3 yr−1 and requires 10 m2 per cow (800 m2 in total) (Remmelink et al., 2020, Table 10.19; RIVM, 2021, type A1). Contrary to the closed-off and air-filtered housing for pigs and chickens, a dairy barn is open and the ammonia-rich air can freely escape. Therefore, we can represent a typical 80-dairy-cow barn as a surface emission source (Theobald et al., 2012), with an emission flux of 45 ppb m s−1 (about 32 µg m−2 s−1) over an area of 800 m2.
We identify the individual contributions of ammonia sources to the NH3 molar fraction and surface–atmosphere exchange, with each source of NH3 represented by a unique scalar. In this study, these sources are identified as a background molar fraction (NH3,bg) and the NH3 emission plume (NH3,plume) from a surface emission source. The sum of these two unique scalars represents the total atmospheric ammonia (NH3,total), as would be observed by in-field observations. Here, we modify DALES v4.2 to force the NH3,plume molar fraction to zero at both x edges of the domain (west and east), preventing circulation of the emission plume in x direction.
Further modifications to DALES v4.2 are made to include the remaining processes governing the variability of the atmospheric ammonia budget. The scalar surface flux (Ftotal), representing surface atmosphere exchange, is divided between a flux acting on the background scalar (Fbg) and another flux acting on the emission plume scalar (Fplume). The magnitude of these two fluxes is weighted by their respective molar fractions (NH3,bg and NH3,plume), relative to the total NH3 molar fraction, e.g., for NH3,bg.
The final modification adds an additional term to the change in the scalar molar fraction . This modified change in the scalar molar fraction reads , with Rchem representing the gain/loss rate in % h−1 and subscript S representing the scalar molar fraction, which can be substituted by either NH3,plume or NH3,bg. The modified DALES v4.2 code used in this study is also freely available online (https://doi.org/10.4121/19869478.v1, Schulte et al., 2022).
2.2 Numerical experiments
We simulate the meteorological conditions observed on 8 May 2008 at the Ruisdael CESAR Observatory (https://ruisdael-observatory.nl/cesar/, last access: 23 June 2022) at Cabauw in the Netherlands (51.971∘ N, 4.927∘ E), as described by aan de Brugh et al. (2013) and Barbaro et al. (2014, 2015). The supersite, with a 213 m high mast, is located on flat (agricultural) grassland with an average height of 0.1 m, and the surface elevation changes are, at most, a few meters over 20 km. 8 May 2008 is selected as it is widely studied and includes measurements of the NH3 molar fraction. In May 2008, the intensive observational campaign IMPACT/EUCAARI was held, which included ammonia concentration measurements by a MARGA system (Aan de Brugh et al., 2012; Mensah et al., 2012) and several additional meteorological variables, including vertical profiles and radiosondes (Kulmala et al., 2011). The meteorology of this day is described in detail by Barbaro et al. (2014), where the experiment is called CESAR2008. Figures 2 and 3 by Barbaro et al. (2014) show vertical profiles and time series of, among other variables, potential temperature, specific humidity, surface fluxes and boundary layer height. The case can be characterized as typical clear-sky, fair-weather conditions with an absence of large-scale heat advection. The model is initialized following the conditions as described by Barbaro et al. (2014), and the initial and prescribed meteorological values of the experiment can be found in Barbaro et al. (2014, their Table 1).
In the morning, a 1500 m residual layer leads to an overshooting of the boundary layer height around 10:30 CEST, up to roughly 1800 m. In the afternoon (12:30–17:00 CEST), CBL growth is weak and the thermodynamic conditions remain relatively constant (Barbaro et al., 2014). Therefore, we only study the turbulent dispersion in the afternoon, when the impact of boundary layer dynamics on the NH3 budget is minimal. The wind speed is moderate at 5.5 to 7 m s−1 in the afternoon, resulting in strong shear production near the surface and a strong momentum entrainment at the CBL top. The convective timescale (τ) in the afternoon is typical for convective fair-weather conditions, increasing from 18 to 27 min between 12:30 and 17:00 CEST. The Monin–Obukhov length fluctuates around approximately −50 m.
The numerical experiments are split into three phases: the meteorological spin-up phase, the buffer phase and the analysis phase. During the meteorological spin-up, 08:00–12:30 CEST, the ammonia surface–atmosphere exchange and chemical transformations are not active. These processes are activated at the start of the buffer phase, from 12:30–14:00 CEST. Entrainment is still an important factor until around 13:00 CEST, causing large fluctuations of the NH3 molar fraction (> 4 ppb), as will be discussed in Sect. 3.1. The CBL is considered well-mixed around 13:00 CEST, but we extend the buffer phase with one more hour. We do so to minimize the impact of earlier entrainment on the one-hour moving average used to calculate statistics during the analysis phase. The analysis phase therefore starts at 14:00 CEST until the collapse of the CBL around 17:00 CEST. The analysis phase is the focus of this study and it is when we analyze the impact of the emission plume on (simulated) point measurements of the NH3 concentration and flux.
2.3 Quantifying the emission plume impact on NH3 measurements
Inspired by the plume observation study by Mylne and Mason (1991), we introduce three variables to assess the presence of the emitted NH3 plume and relevance of the plume fluctuations to nearby observations. These variables – intermittency factor (I), fluctuation intensity (fI) and NH3 flux (F) – are all defined by fluctuations in the NH3 molar fraction. Fluctuations in the NH3 molar fraction result from the turbulent mixing of differences in NH3, caused by local sinks and sources. NH3 fluctuations are therefore found in the background molar fraction as a result of ammonia-poor air near the surface (deposition) and the top of the CBL (entrainment). NH3 fluctuations are further enhanced in proximity to heterogeneous surfaces. A strong local emission source (e.g., a dairy barn), as presented in this study, will cause an emission plume as the enhanced NH3 molar fraction is mixed with the background molar fraction through turbulent mixing. Turbulent models like DALES explicitly resolve this turbulent mixing at a high spatial–temporal resolution and can provide valuable information in the interpretation of in-field observations where surface heterogeneity plays an important role.
We first introduce the intermittency factor (I) to quantify the detectability of the emission plume. Intermittency is defined as the proportion of time during which the plume molar fraction is above the detection limit of instruments typically used to measure atmospheric ammonia, as seen in Fig. 1 and Eq. (1), where N is the number of time steps.
Note that the intermittency is calculated for each individual grid point during the analysis window (14:00–17:00 CEST), at 10 s temporal resolution. We set the NH3 detection limit at 0.25 ppb, similar to the detection limit of the miniDOAS instrument used in the Dutch ammonia monitoring network (Berkhout et al., 2017). The concept of intermittency cannot be applied to NH3,bg or NH3,total, as the background molar fraction always exceeds 0.25 ppb in our numerical experiments, which would result in an intermittency of 1. We therefore only calculate the intermittency for NH3,plume to analyze the detectability of the emission plume.
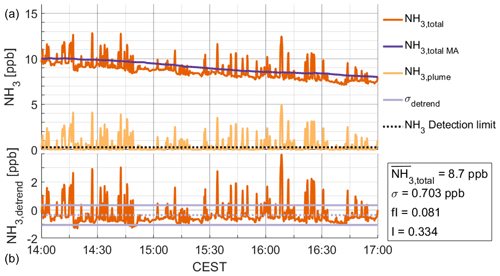
Figure 1Panel (a) shows 10 s time series of NH3,total (orange) and NH3,plume (yellow) during the analysis phase, at 250 m from the emission source. The detrended NH3,total (orange) is shown in panel (b). Fluctuation intensity and intermittency are calculated following Eqs. (4) and (1), respectively, based on the mean NH3,total, standard deviation (light purple) and NH3 detection limit (dotted black).
The second variable, fluctuation intensity (fI), determines the magnitude of the NH3 fluctuations, i.e., NH3 standard deviation (), relative to the mean NH3 molar fraction (). Fluctuation intensity is defined following Eq. (2):
The fluctuation intensity quantifies the level of turbulent mixing. High fI indicates that there are large fluctuations in the measured NH3, which can introduce a positive bias in measurements. In the field of plume dispersion, high fI is found close to the source where plume meandering dominates the mixing process (Dosio and Vilà-Guerau de Arellano, 2006), or at the edge of the emission plume as a result of lateral entrainment of air from outside the plume (Mylne and Mason, 1991; Gailis et al., 2007; Ražnjević et al., 2022). When analyzing the fluctuation intensity of NH3,total, we have a consistent reference for the fluctuation intensity in NH3,bg. Comparing the fI for the total ammonia (fItotal) to the fI for the background ammonia (fIbg) enables us to quantify the relative impact of the emitted NH3 plume to a simulated measurement. When fItotal is of the same order of magnitude as fIbg, we consider the emission plume indistinguishable from the background NH3, i.e., the plume is well mixed. Note that for NH3,plume, the average NH3 concentration is (very close to) zero outside the emission plume, which could lead to an infinitely large fluctuation intensity following Eq. (2). Therefore, fI is only calculated inside the plume using an arbitrary requirement of > 10−5 ppb.
Figure 1 shows a downward trend in NH3,bg and NH3,total, resulting from surface deposition and the loss by chemical gas–aerosol transformations. To minimize the impact of this downward trend on , we detrend the simulated molar fraction by subtracting a 1 h leading moving average (NH3,MA), following Eq. (3) and shown in Fig. 1. The detrended molar fraction (NH3,detrend) is assumed to only represent turbulent fluctuations and is used to calculate the standard deviation to derive fluctuation intensity. By using NH3,detrend to calculate , the fluctuation intensity follows from Eq. (4).
Finally, we introduce the 30 min NH3 flux, studied to mimic the in-field ammonia eddy covariance flux measurements and calculated following Eq. (5). The flux presented in this study is the average 30 min flux for each individual grid point over the analysis phase between 14:00 and 17:00 CEST.
2.4 The concept of blending distance
We use the fluctuation intensity and flux to quantify the impact of the emission plume on the simulated NH3 molar fraction and flux measurements, by introducing the concept of blending distance. The blending distance is based on the percentage change (PCX) in the simulated NH3 measurements resulting from the emission plume, i.e., the percentage change between NH3,total and NH3,bg. PCX is calculated following Eq. (6), where X can be substituted by either fI or F.
Based on this percentage change, we define a threshold for which we assume that the impact of the emission plume is negligible. The blending distance (BDX) is defined as the maximum distance at which PCX drops below the threshold level (e.g., PCX < 25 %), following Eq. (7).
In this study, we present blending distances based on an arbitrary set of threshold levels, ranging from 5 % to 50 %.
The concept of the blending distance is applied to the fluctuation intensity (BDfI) and the NH3 flux (BDF), to quantify the impact on the simulated NH3 measurements of NH3 molar fraction and flux, respectively. For context, we also present the intermittency in Sect. 3.2 to quantify the detectability of the plume.
2.5 Blending distance sensitivity
A key aspect of the study is to determine the sensitivity of the concept of the blending distance to variations in meteorological and NH3 pollution factors. This is in order to study the impact of each process on the blending distance and to identify the driving variables. We study the sensitivity of the blending distance for fluctuation intensity and NH3 flux by varying the geostrophic wind speed (ug), initial background molar fraction (Cbg) at the start of the analysis phase, emission strength (E), deposition strength (D), chemical conversion rate (R), simulation height (H) and model grid resolution (Δ). Table 1 presents the suite of numerical experiments in this study. A single numerical experiment was performed for the sensitivity studies of the NH3 background, emission, deposition and chemistry, each with separate scalars for NH3,bg and NH3,plume. This single experiment, which does not include the variations in the geostrophic wind speed nor the high-resolution experiment, generates just under 1 TB of model output with a computational cost of about 64 000 SBU (System Billing Unit, i.e., the usage of one processor of the Cartesius supercomputer system for 1 h).
Table 1Parameter names, symbols, reference values and their respective variations for the sensitivity study of the blending distance, with the reference settings highlighted in bold.
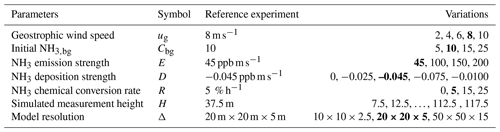
The sensitivity study is structured from large-scale processes to small-scale processes and modeling numerics. Starting with mesoscale processes, we vary the geostrophic wind speed to study the impact of the atmospheric stability on blending distance, i.e., a shear or convection-dominated CBL. Atmospheric stability plays a key role in the turbulent mixing of local sources (emission) and sinks (entrainment and deposition), affecting both the fluctuations in the background molar fraction and the mixing of the emission plume (Dosio et al., 2003). Next, we study the sensitivity of BD to different levels of the background NH3 at the start of the analysis window, representing different levels of regional NH3 pollution. Additionally, varying the background levels of ammonia changes the NH3 inversion at the top of the CBL, affecting the impact of entrainment. Next, the emission strength is varied in order to study the local effect of different emission strengths.
Furthermore, we study the sensitivity of both BDfI and BDF to NH3 deposition and the chemical gas–aerosol transformation. These are dynamic processes, i.e., experiencing clear diurnal and seasonal variability, mainly related to temperature, humidity and pollution levels (Wichink Kruit et al., 2010; van Zanten et al., 2010; aan de Brugh et al., 2013). Our simulation approach, with a simplified representation of deposition and chemistry, allows us to distinctly study the role of these two processes.
Finally, we study the sensitivity of BD to choices made in the numerical setup of the experiments. We vary the height of the simulated measurements. The numerical experiments are generally taken at a simulated height of 37.5 m. This is a trade-off between simulating measurements close to the surface to mimic in-field observations and the resolved turbulent kinetic energy (TKEres) of the model. The TKEres at the lowest level of DALES (at 2.5 m) is zero due to the no-slip boundary at the surface (Heus et al., 2010). When we aim for a TKEres of 75 % at all three (vertical) resolutions, we find TKEres of 76 %, 95 % and 96 % for the low, middle and high resolution at 37.5 m (36.25 m for high resolution). Additionally, it is also expected that varying the measurement height will allow practical insight for in-field observations. Finally, the sensitivity of the blending distance to changes in resolution is studied with two new numerical experiments with higher and lower resolutions of 10 m × 10 m × 2.5 m (1000 × 480 × 1200 grid points) and 50 m × 50 m × 15 m (200 × 96 × 200 grid points), respectively.
3.1 Qualitative analysis of the NH3 emission plume impact
The concept of blending distance is based on fluctuations in the NH3 molar fraction. To better understand the sources of these fluctuations, we first study the time series of a “virtual” point measurement at 250 m horizontal distance from the emission source, shown in Fig. 2a. Our simulation framework allows us to distinguish the individual contributions of NH3,bg (purple) and NH3,plume (light purple) to NH3,total (orange). Here we find that the large NH3,total fluctuations are mainly ascribed to NH3,plume.
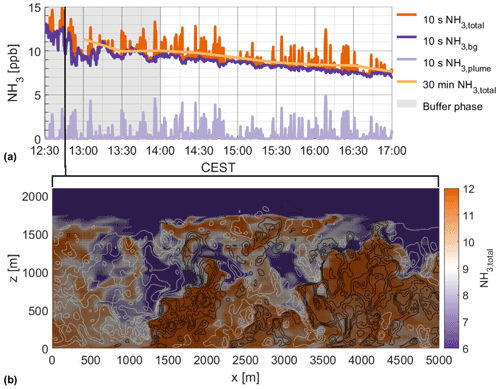
Figure 210 s time series (a) of NH3,total (orange), NH3,bg (purple) and NH3,plume (light purple) during the buffer phase (gray area) and the analysis phase, taken at 250 m distance from the emission source. The large high-frequency fluctuations shown (> 4 ppb) are not captured by the 30 min average of NH3,total (light orange). The vertical xz cross-section at 12:46 CEST (b) displays high spatial variability during the buffer phase in NH3,total (> 4 ppb) over short distances (hundreds of meters). The black/white contour lines represent upward/downward wind speeds in steps of 0.5 m s−1.
As discussed in Sect. 2.3, fluctuations are also found in the background molar fraction (NH3,bg), leading to non-zero fIbg. Fluctuations NH3,bg are a result of heterogeneous turbulent mixing. In this study, the fluctuations are caused by vertical gradients only, as we use a homogeneous surface in the simulation of NH3,bg. These vertical gradients are found near the surface and at the top of the CBL. At the surface, the surface–atmosphere exchange (deposition) decreases the NH3 molar fraction, which results in a vertical gradient in NH3,bg. At the top of the CBL, the vertical gradient is a result of the turbulent exchange with the free troposphere (entrainment). Figure 2b shows that the intrusion of NH3-low air masses from the free troposphere is transported by the downdraft subsidence motions, resulting in large fluctuations in NH3,bg in the boundary layer. As shown in Fig. 2a, the amplitude of these fluctuations can reach 4 ppb and can last for over 5 min. When averaging over 30 min, even the large fluctuations between 12:30 and 13:15 CEST are filtered out, but these high-frequency turbulent fluctuations could still be present in raw measurement data of high-resolution in-field observations.
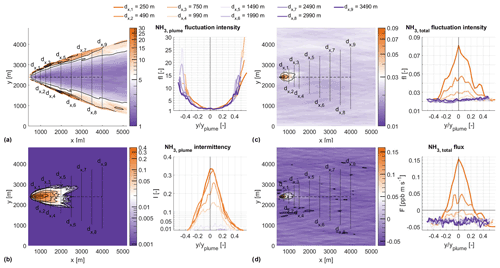
Figure 3The xy cross-sections at 37.5 m with y transects through the NH3 emission plume for the NH3,plume fluctuation intensity (a), intermittency (b), NH3,total fluctuation intensity (c) and NH3,total flux (d). The plume transects are labeled dx,1 to dx,9 for increasing x distance from the NH3 emission source and normalized by plume width for NH3,plume > 10−5 ppb. The data presented are calculated during the analysis phase (14:00 and 17:00 CEST) at 37.5 m.
Now that we understand the source of the NH3 fluctuations, we take a closer look at the emission plume without any background NH3. The xy plot in Fig. 3a shows low fI in the plume center (≈ 2) and a strong increase near the plume edges, up to fI ≈ 30. This is echoed by the plume transects, as they show the typical “U-shape” found for Gaussian plumes (Mylne and Mason, 1991; Gailis et al., 2007; Ražnjević et al., 2022). These high fI values at the edges of the plume are a result of very low average molar fractions combined with low intermittency. This leads to a high standard deviation, relative to the very low averaged molar fraction, at the plume edges. Without background NH3, it is at the edges of the plume that in-plume lateral entrainment of ammonia-free air happens, diluting the emission plume by turbulent mixing.
The intermittency cross-section in Fig. 3b shows that maximum I is only a little over 0.3, resulting from the meandering of the plume. Figure 3b also shows that with an NH3 detection limit of 0.25 ppb, the plume can be detected up to a distance of about 2.0 km from the source.
The cross-section of fI changes dramatically when analyzing NH3,total, the sum of NH3,bg and NH3,plume. With the addition of a non-zero background molar fraction, fI can be calculated over the whole domain, as shown in Fig. 3c. Now, we find a much lower fluctuation intensity, with a maximum of 0.08 for NH3,total compared to 30 for NH3,plume. The U-shape shown in the transect of Fig. 3a is replaced by an approximately Gaussian shape, with the highest fluctuation intensities at the centerline of the plume. This centerline fI decreases with distance from the source and becomes indistinguishable from the out-of-plume fI after approximately 1 km distance, i.e., a rough estimate for BDfI.
Finally, Fig. 3d shows that the emission plume leads to a positive flux (emission) for NH3,total in proximity of the emission source, while the flux is negative (deposition) outside the plume. Note that significant fluctuations are found in the flux over the full domain, with ppb m s−1 (prescribed ppb m s−1) for NH3,bg. Similar to fItotal in Fig. 3c, the transects for the NH3 flux are approximately Gaussian in shape, with peak values close to the plume centerline at = 0. After approximately 1 km at the approximate plume centerline, the in-plume flux becomes visually indistinguishable from the background, i.e., a rough estimate for BDF. This positive anomaly is the result of the emission source being within the footprint of these receptors.
3.2 Quantitative analysis of the NH3 emission plume impact
We apply the concept of blending distance in Fig. 4 to the fluctuation intensity (Fig. 4a), flux (Fig. 4b) and intermittency (Fig. 4c). The markers represent the value at each individual grid point on the 37.5 m horizontal plane, the continuous orange line represents the grid point with the highest value within a 50 m moving window (maximum), the orange dotted line represents the plume centerline and the purple dashed and continuous lines represent the blending distances for their respective threshold.
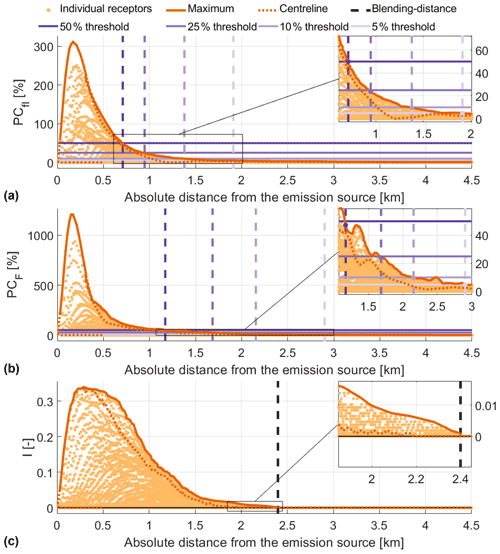
Figure 4The percentage change of NH3,total relative to NH3,bg against absolute distance from the NH3 emission source. The panels show fluctuation intensity (a), NH3 flux (b) and intermittency (c). The maximum value within a 50 m moving window (orange) and the plume centerline (dotted orange) are highlighted. Blending distances (purple dashed) are calculated based on four thresholds at 5 %, 10 %, 25 % and 50 % (purple continuous).
We interpret the calculation of the blending distance based on four arbitrary threshold levels (5 %, 10 %, 25 % and 50 %) for fI and F, shown in Fig. 4a and b. The distance at which the maximum value of PCX drops below the threshold level is the blending distance. The sensitivity of BD to these thresholds will be discussed in detail in Sect. 4.1, using Figs. 6 and 7. Additionally, the intermittency in Fig. 4c shows that the emission plume is quantifiable up to over 2.4 km distance.
Starting with the fluctuation intensity (Fig. 4a), PCfI peaks at a relative change of about 300 %, caused by the NH3 emission plume. BDfI decreases nonlinearly from 0.7 to 1.9 km with the thresholds decreasing from 50 % to 5 %. Figure 4b shows that the emission plume has a larger impact on NH3 flux measurements than on the fluctuation intensity of the NH3 molar fraction. The large difference between the emission strength (45 ppb m s−1) and the deposition (−0.045 ppb m s−1) results in a maximum PCF of about 1200 % in close proximity to the emission source. The long tail of PCF indicates that the turbulent fluctuations in the emission plume affect flux measurements over several kilometers. As a result, BDF increases from 1.2 to 2.9 km for decreasing thresholds, significantly longer distances than our 1 km qualitative estimate based on Fig. 3d. Note that Fig. 3d shows that the flux change signs in proximity to the emission source and that this sign change is not reflected in Fig. 4b.
Figure 4 shows that the centerline and the maximum statistics give similar results, indicating that the highest values of PC are found at the plume centerline, however, with some variability. These variabilities are visualized in Fig. 5, which shows the spatial structure of the percentage change in grayscale for the fluctuation intensity (PCfI) in Fig. 5a and the ammonia flux (PCF) in Fig. 5b. The colored lines in these panels represent the blending distances for different thresholds. The right panels show these same blending distances for different angles from the plume centerline (W), representing different wind directions. Figure 5 shows large variability in the blending distance, especially for the 5 % and 10 % threshold levels, as a result of the chaotic nature of turbulence.
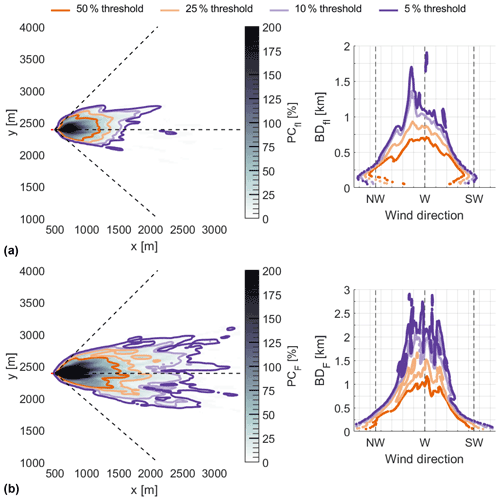
Figure 5The left panels show the spatial structure of the percentage change in grayscale for the fluctuation intensity (PCfI) in (a) and the ammonia flux (PCF) in (b). The colored contour lines show the locations where the 50 % (orange), 25 % (light orange), 10 % (light purple) and 5 % (purple) thresholds are met, representing the blending distance (BD). The right panels show these blending distances as a function of different angles from the plume centerline (W), with these angles representing the wind direction.
4.1 The sensitivity of blending distance to meteorological and NH3 pollution variables
We study the sensitivities of BDfI and BDF to a range of meteorological, NH3 pollution parameters, and model resolution and simulated measurement height (Table 1). The results of the sensitivity study are shown in Figs. 6 and 7, for an arbitrary set of thresholds ranging from 5 % (orange dashed) to 50 % (orange dotted), representing the maximum acceptable difference in fI and F caused by the emission plume in %.
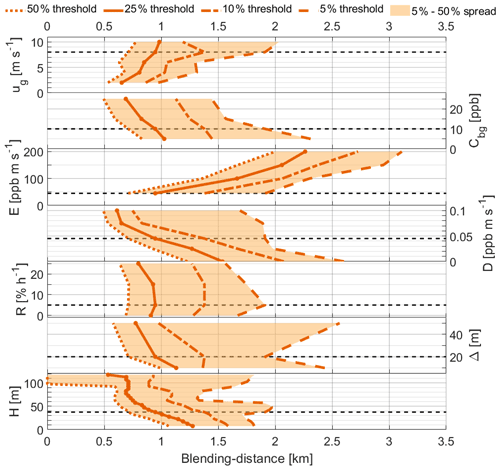
Figure 6The sensitivity of BDfI to the geostrophic wind speed (ug), initial background molar fraction (Cbg), emission strength (E), deposition strength (D), chemical reaction rate (R), model resolution (Δ) and simulated measurement height (H). BDfI is determined for threshold levels ranging from 5 % (orange dashed) to 50 % (orange dotted).
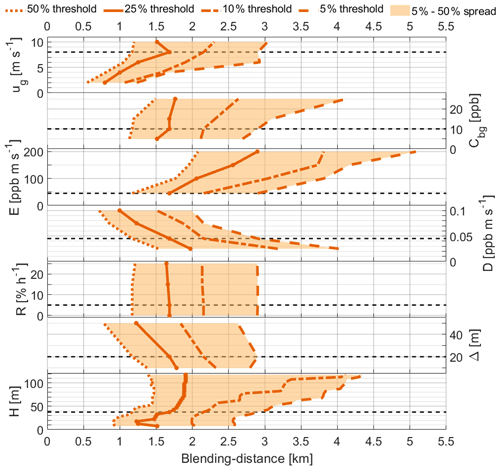
Figure 7The sensitivity of BDF to the geostrophic wind speed (ug), initial background molar fraction (Cbg), emission strength (E), deposition strength (D), chemical reaction rate (R), model resolution (Δ) and simulated measurement height (H). BDF is determined for threshold levels ranging from 5 % (orange dashed) to 50 % (orange dotted).
Starting with BDfI, Fig. 6 shows that BDfI ranges roughly between 0.5 and 3.0 km, a first-order estimate of the minimum distance for NH3 molar fraction measurements. There is a negative correlation between BDfI and the choice in threshold, i.e., increasing the threshold level decreases BDfI. We generally find that BDfI decreases nonlinearly by approximately 1.0 km when increasing the threshold level from 10 % to 50 %, halving BDfI, highlighted by the large difference between the 10 % (dashed-dotted) and 5 % (dashed) threshold levels for both BDfI and BDF. We discuss the individual variables of Fig. 6 from top to bottom, starting at the mesoscale (ug), down to the micrometer scale (R), and finishing with the model resolution and simulated measurement height.
The geostrophic wind speed (ug) is one of the main drivers of turbulent mixing and transport of the plume (Dosio et al., 2003; Vrieling and Nieuwstadt, 2003; Dosio and Vilà-Guerau de Arellano, 2006). Figure 6 shows a positive correlation between BDfI and ug. By varying ug, we move from a convection-driven boundary layer (ug = 2 m s−1) to more shear-driven meteorological conditions (ug = 10 m s−1). In a convection-driven boundary layer, turbulent mixing is rather weak and the NH3 emission plume rises from the surface as convection plumes are the main drivers of turbulent mixing. Under these conditions, in-plume molar fractions are very high, but horizontal transport of the emission plume is weak, resulting in a low BDfI. For shear-driven conditions, the NH3 emission plume tends to stick to the surface as the increased horizontal wind speed enhances horizontal transport and turbulent mixing. The enhanced horizontal transport and emission plume sticking to the surface should significantly increase BDfI, but the enhanced turbulent mixing counteracts these processes by reducing the NH3,plume molar fraction and fluctuations. This is shown in Fig. 6 and explains why the sensitivity of BDfI increases for lower threshold levels (5 %), as smaller plume fluctuations will reach long distances in shear-driven conditions.
One panel below, Fig. 6 shows a negative correlation between BDfI and the initial background molar fraction (Cbg), i.e., the regional level of NH3 pollution. The first cause of the negative correlation is the higher average molar fraction, which lowers the relative weight of the NH3,plume fluctuations (σplume) when fItotal is calculated following Eq. (2). Additionally, increasing NH3,bg leads to a large difference in the NH3 air mass characteristics at the top of the boundary layer. The exchange between the boundary layer and free tropospheric air masses through entrainment increases σbg at 37.5 m from 0.13 ppb (Cbg = 5 ppb) to 0.38 ppb (Cbg = 25 ppb), resulting in an increased fIbg. Both processes reduce the magnitude of PCfI for increasing Cbg, resulting in a decrease of BDfI.
At the local scale, Fig. 6 shows a clear positive and negative correlation when varying emission strength (E) and deposition strength (D), respectively. Both variables directly affect one of the main drivers of turbulent mixing – heterogeneity. Increasing the NH3 emission strength of the local (heterogeneous) source directly increases fIplume, increasing BDfI. Varying the deposition, on the other hand, directly affects the vertical gradient of the NH3 molar fraction near the surface, increasing fIbg for increasing D and therefore reducing BDfI.
We only briefly touch upon the chemical conversion rate (R), as Fig. 6 shows that varying R does not significantly affect BDfI. R is applied uniformly to the 3D domain and has little effect on turbulent mixing. Note that our simplified representation of chemistry could lead to a potential underestimation of the impact of chemistry on BDfI, as our approach is unable to resolve potential nonlinear effects of turbulent mixing on the in-plume chemical reaction rate near the emission source (see discussion in Sect. 4.2).
Next, we vary the model resolution (Δ) in Fig. 6 and find that BDfI is weakly sensitive to the model resolution. The results indicate that the calculation of the blending distance does benefit by increasing the simulation resolution. However, there is a trade-off between the computational costs of the simulation and the resolution.
Finally, Fig. 6 shows two regimes in the sensitivity of BDfI to the simulated measurement height (H). For the 50 % threshold, BD decreases by about 500 m with height up to 90 m. Above 90 m, there is a transition where BDfI rapidly goes to zero. In this second regime, the simulated measurements are located above the plume centerline. Thereon, fIplume rapidly decreases in height until PCfI does not reach the 50 % threshold and BDfI becomes zero. This rapid decrease is a result of the simulated measurements being located above the emission plume, as the height of the plume does not reach above 150 m for the first 1.5 km horizontal distance. The height of this transition increases with decreasing threshold levels as the thresholds become more sensitive to smaller NH3,plume fluctuations.
Figure 7 shows the results of the sensitivity study for BDF (Table 1). Both the blending distance for molar fraction measurements (BDfI) and for flux measurements (BDF) can be interpreted as an inverse footprint analysis, as we estimate the area affected by the emission source. The results of the sensitivity study of BDF, however, are different from the BDfI results, as the footprints for flux and molar fraction measurements are not the same. The footprint for flux measurements is smaller than those of molar fraction measurements (Rannik et al., 2000; Kljun et al., 2003; Vesala et al., 2008). However, comparing BDfI to the footprint of NH3 molar fraction measurements is not straightforward, as BDfI is based on the NH3 fluctuation intensity, not the molar fraction. It is therefore interesting to determine whether the results of the sensitivity study of BDF will differ compared to the results of BDfI.
When analyzing Fig. 7, we find that there are indeed differences between BDF and BDfI. BDF is significantly longer, ranging from 0.75 to roughly 5 km, indicating that NH3 flux measurements are more sensitive to the emission plume. Note that we removed the results for D = 0 ppb m s−1. Here, Fbg approaches zero, resulting in infinitely large PCF and unrealistic BDF values, following Eq. (6).
One of main differences between BDF and BDfI is found in the sensitivity to the threshold levels (5 % to 50 %). BDF is more sensitive to the different threshold levels compared to BDfI. This is in agreement with the results shown in Fig. 4b, where we discussed that PCF is significantly larger than PCfI, with a longer tail. As a result, the nonlinear effect of the aforementioned long tail in PCF (Fig. 4b) increases BDF for low threshold levels. Despite these differences, the same arbitrary set of thresholds is used for both BDfI and BDF.
Significant differences between BDF and BDfI are also found in the sensitivity to the geostrophic wind speed (ug) and the simulated measurement height (H). Both variables directly affect the footprint of the simulated flux measurements. In shear-driven turbulent conditions (high ug), the footprint of the measurement is elongated compared to convective conditions. This reduces the width of the footprint and lengthens the up-wind distance at which the emission source can be measured, thus, increasing BDF. Increasing H also increases the footprint of the measurements, but there is no elongation of the footprint. As a result, BDF has a strong positive correlation to ug, but is only weakly correlated to H, except for the lower threshold levels.
Figure 7 appears to show that BDF has a weak positive correlation with increasing Cbg. This is mainly attributed to an increase in the spatial variations of the background NH3 flux, which increases from ppb m s−1 for Cbg = 10 ppb (Fig. 3d) to for Cbg = 25 ppb. As a result, the fluctuations in PCF shown in Fig. 4b, increase in amplitude and frequency, which particularly affects the low threshold levels of 5 % and 10 %.
There are also strong similarities between the sensitivity of BDF and BDfI. Both Figs. 6 and 7 show that the blending distance is only weakly sensitive to the chemical reaction rate (R) and the model resolution (Δ). For both molar fraction and flux measurements, the emission strength (E), deposition (D) and, to a lesser extent, the geostrophic wind speed (u3) are the driving variables of the blending distance.
4.2 Uncertainty of the blending distance estimation
The turbulent dispersion of the emission plume is chaotic by nature and driven by a wide range of factors. We therefore carry out a systematic analysis on how these factors and the model resolution influence the relationships between emissions and simulated in-field measurements. The chaotic nature of turbulence results in random variations in both the emitted NH3 (Fig. 3a and b) and the background NH3 (Fig. 3c and d). These random fluctuations lead to variability in the calculation of the blending distances, leading to uncertainty in the blending distances presented in this study. The variability increases when using the lower threshold levels (e.g., 5 % and 10 %), as is visualized and discussed in Sect. 3.1 and 3.2. The variability could be reduced by increasing the length of the analysis window, i.e., increasing the averaging time to filter out the small and short spatio-temporal turbulence variability.
Increasing the length analysis window, however, means that the blending distance is calculated using a wider range of boundary layer dynamics and variations in the thermodynamic variables. Boundary layer dynamics are especially relevant in the morning and early afternoon when the boundary layer grows and air from the residual layer and free troposphere is entrained, or in the afternoon when turbulence decays (Pino et al., 2006). It leads to entrainment being one of the dominant processes driving the NH3 diurnal variability (Wichink Kruit et al., 2007; Schulte et al., 2021). In Fig. 2, we show that it leads to large fluctuations in NH3,bg, significantly increasing fIbg. We therefore filter out the impact of boundary layer dynamics and variations in the thermodynamic variables with our choice of analysis window from 14:00 and 17:00 CEST, in order to find a first-order estimate of the blending distance. We do recommend a follow-up study on the role of boundary dynamics.
Finally, there is a downside to our simplified representation of chemical transformations in that it is applied uniformly to the 3D domain. In reality, the equilibrium molar fractions for these chemical transformations are related to temperature and humidity, and results in a near-surface NH3 gradient of the NH3 molar fraction (aan de Brugh et al., 2013). Therefore, we are likely to underestimate the role of chemical transformations and overestimate BDfI, as turbulent mixing of this near-surface gradient increases fIbg.
The blending distance cannot be captured by a single number. This is partly due to the uncertainty involved in calculating the blending distance, but the blending distances is, most of all, an integrated variable. Several processes are captured by the blending distance in one single variable, including the chaotic nature of turbulent plume dispersion, convective and shear-induced turbulence, atmospheric pollution levels and surface heterogeneity. As shown in Sect. 4.1, each of these processes impacts the blending distance differently. Despite its complexity, the blending distance is a useful variable since it is an integrated variable; all the aforementioned processes are represented in this distance at which the impact of an emission plume is negligible with respect to the background.
The applicability of the results presented here depends not only on the meteorological and NH3 pollution factors, but also on the physical context of the measurement site. This study is based on the Ruisdael CESAR Observatory at Cabauw, which is located on flat agricultural grassland with surface elevation changing only by a few meters over 20 km. A different physical context, like a heterogeneous surface which changes turbulent properties (Ouwersloot et al., 2011), is likely to significantly affect the resulting blending distances. With the simulation framework presented here, the blending distance can be calculated for specific weather conditions and for the physical context of the measurement site, providing a more accurate assessment of the impact of nearby emissions on NH3 observations at a specific measurement site. The results presented in this study provide a valuable first estimate of and discussion on a typical blending distance and its driving variables.
4.3 Blending distance literature for passive tracers
Evaluating the blending distance results against typical literature on plume dispersion is a difficult exercise. The topic is generally not mentioned as these studies focus on the release of passive scalars in an unpolluted environment, and only few studies even research (near) surface releases (Cassiani et al., 2020). Normalization of both distance from the source and the in-plume molar fraction further complicates the interpretation of literature results.
We therefore try to estimate the order of magnitude of the blending distance based on the in-plume molar fraction and fluctuation intensity of plume dispersion modeling studies. Following figures by Dosio et al. (2003) and Dosio and Vilà-Guerau de Arellano (2006), we find that the in-plume molar fraction rapidly decreases for a convection-driven boundary layer ( ≥ 40 and ≤ 0.2) at the surface up to roughly 6 km distance, after which, it starts to level off. Similar results are found for the fluctuation intensity; however, the results are less pronounced for the near-surface release experiments. The 6 km distance approximately doubles for shear-driven boundary layers ( ∼ 40 and ∼ 0.46). The observations shown in Fig. 10 by Mylne and Mason (1991) show that the observed fluctuation intensity also decreases with distance, but levels after roughly 15 km distance from the emission source. We use these distances at which the plume statistics start to level off as an estimate of the order of magnitude of the blending distance, indicating that the blending distance could be in the order of several kilometers (6 to 15 km), based on plume dispersion literature.
These rough estimates of the 6 to 15 km distance are significantly larger than the blending distances presented in this study. Such long distances between source and measurement site would not make feasible requirements in densely agricultural regions, but are likely an overestimation of the blending distance. These estimates are based on the molar fraction and fI of the emission plume, with no representation of background ammonia levels. The latter is especially important, as we show in Sect. 3.1 and 3.2 that the impact of the emission plume rapidly decreases relative to the turbulent background ammonia, while the emission plume itself can be detected for several kilometers as indicated by the intermittency.
4.4 Blending distance literature for ammonia measurements
Articles on ammonia measurements in close proximity to an emission source implicitly include all relevant processes. These studies could also provide a qualitative, perhaps more realistic, evaluation of the NH3 blending distance results presented here. In-field measurements show that the NH3 molar fraction exponentially decreases with distance from the source, with measurements close to the background molar fraction after 300 to 500 m (Fowler et al., 1998; Sommer et al., 2009; Shen et al., 2016). Similar results were obtained in an intercomparison study of short-range atmospheric dispersion models by Theobald et al. (2012), at horizontal resolutions of 25–50 m and receptors at 100 m intervals along four radial directions (N, E, S and W). However, these measurements are typically arranged a few lines downwind of the source, with only a handful of measurements over a distance of 300 to 1000 m. At these short distances, plume dispersion is dominated by meandering of the plume (Nieuwstadt, 1992), and the in-plume molar fraction measurements are underestimated as a result, especially given the averaging times of these measurements ranging from several hours up to multiple weeks.
Finally, we can evaluate our findings against measurement site requirements of air quality networks. The Dutch air quality network and the EMEP (European Monitoring and Evaluation Programme) network do set requirements for the minimum distance from emission sources and no references to scientific studies are provided. Back in 1990, the Dutch network required a minimum distance for NH3 sites of 300–500 m from NH3 point or area sources, depending on source strength (Boermans and Erisman, 1990). This is in line with the literature on measurements in proximity to emission sources discussed earlier, but closer than the blending distances presented here. Currently, no hard requirements are in place in the Netherlands, although the potential impact of NH3 sources is still recognized (Wichink Kruit et al., 2021). At a European level, EMEP measurement sites require a 2 km minimum distance for measurements nearby the stabling of animals and manure applications, depending on the number of animals and field size (Schaug, 1988; EMEP/CCC, 2001). This 2 km distance is in line with our recommendations, although the results in this study indicate that distances below 2 km could also be sufficient.
4.5 Towards an NH3 virtual test bed: integrating fine-scale simulations with advanced observations
This study is the first that specifically addresses the regional representativity of ammonia measurements in proximity to an emission source. The systematic analysis presented in Figs. 6 and 7 can be used as a reference when interpreting in-field NH3 measurements. Additionally, the simulation framework can be applied to individual locations to study the representativity of (potential new) measurement sites under local conditions by using the concept of blending distance. One can expand the simulation framework to include multiple sources, area sources – each with a unique passive scalar – and heterogeneous surface conditions (Ouwersloot et al., 2011) to simulate local NH3 conditions.
The DALES model proved to be flexible, allowing for simulations of a convective, sheared convective, stable and cloud-topped boundary layer (Verzijlbergh et al., 2009; Heus et al., 2010). The fine-scale simulation framework will be included in the Ruisdael CESAR Observatory at Cabauw, a nationwide observatory for measurements and modeling of the atmosphere and air quality. It can be a powerful tool in future ammonia research, e.g., in preparation of (emission) measurement campaigns or to improve interpretation of NH3 (flux) measurements. Furthermore, we want to stress that the methods presented here are not limited to ammonia, but can be used for any gas for which relevant processes occur at high spatio-temporal resolutions.
We recommend expanding the simulation framework to create a testbed to study NH3 at high spatio-temporal resolutions, including all processes relevant to the NH3 diurnal variability. The main additions should be a dynamic parameterization of the surface–atmosphere exchange, e.g., DEPAC (van Zanten et al., 2010) and a thermodynamic chemistry module, e.g., ISORROPIA version 2 (Fountoukis and Nenes, 2007). With these additions, on top of the existing possibility to distinguish between background and emitted NH3, the fine-scale simulation framework with explicitly resolved turbulence will be well suited to study short-range dispersion of ammonia, e.g., deposition in close proximity to emission sources and the impact of turbulent micromixing on the chemical reaction rate. These studies are typically performed using models where turbulence is parameterized or Gaussian plume models are used (Loubet et al., 2006; Sommer et al., 2009; van der Swaluw et al., 2017). Furthermore, the addition of a thermodynamic chemistry module can lead to new insights on NH3 flux measurements. The equilibrium molar fractions of the NH3 gas–aerosol transformations depend on atmospheric temperature and humidity, resulting in a near-surface molar fraction gradient. This gradient leads to an underestimation of the NH3 deposition flux of about 0.02 µg m−2 s−1, when using the flux-gradient method (Nemitz et al., 2004). With these additions to the simulation framework, the virtual NH3 testbed can be used improve the interpretation of NH3 flux measurements.
This paper presents a fine-scale simulation framework with which we assess the regional representativity of NH3 molar fractions and flux measurements in proximity to a typical NH3 emission source. We aim to translate concepts from the fields of plume dispersion and fine-scale simulations to support the analysis of NH3 observations in areas characterized by NH3 (point) source emissions. This includes realistic representations of NH3 surface–atmosphere exchange and chemical gas–aerosol transformations. The concept of a blending distance is introduced to systematically analyze the impact of the emitted NH3 on simulated measurements, relative to a background concentration. Following this approach, we define a first-order estimate of a minimum distance requirement between regional representative measurements and a typical NH3 emission source.
By means of fine-scale simulation of atmospheric NH3, we investigate the representativity of NH3 measurements from kilometer to meter scales in proximity to a typical emission source. The fine-scale simulation framework presented has proven to be a powerful and flexible tool for future research on ammonia, or any gas for which the relevant processes occur at high spatio-temporal resolutions. The simulation framework, with explicitly resolved turbulence, not only enables us to quantify the variability in NH3 measurements, but also to analyze and quantify the individual contribution of the NH3 emission plume. The concept of blending distance presents a consistent criterion, based on second-order statistics, for the minimum distance at which the impact of the emitted NH3 is estimated to be indistinguishable from the variability of the background NH3. Following this approach, we perform several numerical experiments to analyze the sensitivity of the blending distance to a variety of meteorological and NH3 pollution variables, centered around the flat grassland at Ruisdael CESAR Observatory at Cabauw. This systematic analysis shows a strong sensitivity to the emission strength, deposition and threshold level used in the calculation, and to the stability of the (convective or shear dominated) boundary layer. Furthermore, we find that the blending distances differ for NH3 molar fraction and flux measurements, with flux measurements being more sensitive to the NH3 emission plume. Following this sensitivity analysis, we conclude that NH3 measurements at the CESAR Observatory should be taken at a minimum distance of 0.5–3.0 km or 0.75–4.5 km from an emission source for measurements of the NH3 molar fraction or flux, respectively.
The modified version of the Dutch Atmospheric Large-Eddy Simulation (DALES) version 4.2, including data processing scripts and documentation, is available at https://doi.org/10.4121/19869478.v1 (Schulte et al., 2022). The original DALES version 4.2 code is available at https://doi.org/10.5281/zenodo.3759193 (Arabas et al., 2020). All data presented in this study can reproduced using the code and input files provided at https://doi.org/10.4121/19869478.v1 (Schulte et al., 2022).
RBS and JVGdA worked on the conceptualization and developed the methodology of the numerical experiments. RBS and BJHvS made modifications to the software, i.e., the DALES model. The numerical experiments were performed by RBS, who also analyzed and visualized resulting data. The manuscript draft was written by RBS and reviewed by both JVGdA and MCvZ. Finally, the project was supervised by JVGdA and MCvZ.
The contact author has declared that neither they nor their co-authors have any competing interests.
Publisher’s note: Copernicus Publications remains neutral with regard to jurisdictional claims in published maps and institutional affiliations.
This project is part of the Monitoring of dry ammonia deposition (project no. 36.7) project of the Dutch National Institute of Public Health and the Environment (RIVM). The numerical simulations were performed with the supercomputer facilities at SURFsara and financially sponsored by the Netherlands Organization for Scientific Research (NWO) Physical Science Division (project no. 2021/ENW/01081379).
This research has been supported by the Rijksinstituut voor Volksgezondheid en Milieu (project no. 36.7: Monitoring of dry ammonia deposition) and the Netherlands Organization for Scientific Research (NWO) Physical Science Division (project no. 2021/ENW/01081379).
This paper was edited by Stefano Galmarini and reviewed by two anonymous referees.
Aan de Brugh, J. M. J., Henzing, J. S., Schaap, M., Morgan, W. T., van Heerwaarden, C. C., Weijers, E. P., Coe, H., and Krol, M. C.: Modelling the partitioning of ammonium nitrate in the convective boundary layer, Atmos. Chem. Phys., 12, 3005–3023, https://doi.org/10.5194/acp-12-3005-2012, 2012. a
aan de Brugh, J. M. J., Ouwersloot, H. G., Vilà-Guerau de Arellano, J., and Krol, M. C.: A large-eddy simulation of the phase transition of ammonium nitrate in a convective boundary layer, J. Geophys. Res.-Atmos., 118, 826–836, https://doi.org/10.1002/jgrd.50161, 2013. a, b, c, d, e
Anys, M., Ullrich, B., Gager, M., and Pinterits, M.: European Union emission inventory report 1990–2018: under the UNECE Convention on Long-range Transboundary Air Pollution, Tech. Rep. TH-AL-20-013-EN-N, European Environment Agency, Kongens Nytorv 6, 1050 Copenhagen K, Denmark, https://www.eea.europa.eu/ds_resolveuid/c48fe5a189e5484095dcb509da927a36 (last access: 23 June 2022), 2020. a
Arabas, S., Axelsen, S., Attema, J., Beets, C., Boeing, S. J., Cuijpers, H., de Bruine, M., Chylik, J., van der Dussen, J., van Heerwaarden, C., Heus, T., Jansson, F., Jonker, H., Moene, A., Ouwersloot, H., van den Oord, G., de Roode, S., Neggers, R., Pedruzo, X., Siebesma, P., Sikma, M., van Stratum, B. J. H., Vilà-Guerau de Arellano, J., and van Zanten, M. C.: dalesteam/dales: DALES 4.2.1, Zenodo [code], https://doi.org/10.5281/zenodo.3759193, 2020. a, b
Ardeshiri, H., Cassiani, M., Park, S. Y., Stohl, A., Pisso, I., and Dinger, A. S.: On the Convergence and Capability of the Large-Eddy Simulation of Concentration Fluctuations in Passive Plumes for a Neutral Boundary Layer at Infinite Reynolds Number, Bound.-Lay. Meteorol., 176, 291–327, https://doi.org/10.1007/s10546-020-00537-6, 2021. a
Barad, M. L.: Project Prairie Grass, a field program in diffusion, vol. 1, Tech. Rep. No. 59, vol. I, Report AFCRC-TR-58-235(I), Air Force Cambridge Research Labs, https://www.harmo.org/jsirwin/PGrassVolumeI.pdf (last access: 23 June 2022), 1958. a
Barbaro, E., Vilà-Guerau de Arellano, J., Ouwersloot, H. G., Schröter, J. S., Donovan, D. P., and Krol, M. C.: Aerosols in the convective boundary layer: Shortwave radiation effects on the coupled land-atmosphere system, J. Geophys. Res.-Atmos., 119, 5845–5863, https://doi.org/10.1002/2013JD021237, 2014. a, b, c, d, e, f
Barbaro, E., Krol, M., and Vilà-Guerau de Arellano, J.: Numerical simulation of the interaction between ammonium nitrate aerosol and convective boundary-layer dynamics, Atmos. Environ., 105, 202–211, https://doi.org/10.1016/j.atmosenv.2015.01.048, 2015. a
Behera, S. N., Sharma, M., Aneja, V. P., and Balasubramanian, R.: Ammonia in the atmosphere: a review on emission sources, atmospheric chemistry and deposition on terrestrial bodies, Environ. Sci. Pollut. R., 20, 8092–8131, https://doi.org/10.1007/s11356-013-2051-9, 2013. a, b
Berkhout, A. J. C., Swart, D. P. J., Volten, H., Gast, L. F. L., Haaima, M., Verboom, H., Stefess, G., Hafkenscheid, T., and Hoogerbrugge, R.: Replacing the AMOR with the miniDOAS in the ammonia monitoring network in the Netherlands, Atmos. Meas. Tech., 10, 4099–4120, https://doi.org/10.5194/amt-10-4099-2017, 2017. a
Boermans, G. M. F. and Erisman, J. W.: Meetstrategieontwikkeling voor het representativiteitsonderzoek als onderdeel van het additioneel meetprogramma ammoniak: fenomenologie van NH3 en meetritsimulaties, Tech. Rep. 222105001, National Institute for Public Health and the Environment (RIVM), Antonie van Leeuwenhoeklaan 9, 3721, MA Bilthoven, the Netherlands, http://hdl.handle.net/10029/255566 (last access: 23 June 2022), 1990. a
Cassiani, M., Bertagni, M., Marro, M., and Salizzoni, P.: Concentration Fluctuations from Localized Atmospheric Releases, Bound.-Lay. Meteorol., 177, 461–510, https://doi.org/10.1007/s10546-020-00547-4, 2020. a, b
Dosio, A. and Vilà-Guerau de Arellano, J.: Statistics of Absolute and Relative Dispersion in the Atmospheric Convective Boundary Layer: A Large-Eddy Simulation Study, J. Atmos. Sci., 63, 1253–1272, https://doi.org/10.1175/JAS3689.1, 2006. a, b, c, d
Dosio, A., Vilà-Guerau de Arellano, J., Holtslag, A. A. M., and Builtjes, P. J. H.: Dispersion of a Passive Tracer in Buoyancy- and Shear-Driven Boundary Layers, J. Appl. Meteorol., 42, 1116–1130, https://doi.org/10.1175/1520-0450(2003)042<1116:DOAPTI>2.0.CO;2, 2003. a, b, c, d
EMEP/CCC: EMEP manual for sampling and chemical analysis, Tech. Rep. EMEP/CCC-Report 1/95, O-7726, Chemical Co-ordination Centre of EMEP (EMEP/CCC), https://projects.nilu.no/ccc/manual/download/cccr1-95rev.pdf (last access: 23 June 2022), 2001. a, b
Erisman, J. and Schaap, M.: The need for ammonia abatement with respect to secondary PM reductions in Europe, Environ. Pollut., 129, 159–163, https://doi.org/10.1016/j.envpol.2003.08.042, 2004. a
Erisman, J. W., Galloway, J. N., Seitzinger, S., Bleeker, A., Dise, N. B., Petrescu, A. M. R., Leach, A. M., and de Vries, W.: Consequences of human modification of the global nitrogen cycle, Philos. T. Roy. Soc. B, 368, 20130116, https://doi.org/10.1098/rstb.2013.0116, 2013. a
Fountoukis, C. and Nenes, A.: ISORROPIA II: a computationally efficient thermodynamic equilibrium model for K+–Ca2+–Mg2+––Na+–––Cl−–H2O aerosols, Atmos. Chem. Phys., 7, 4639–4659, https://doi.org/10.5194/acp-7-4639-2007, 2007. a
Fowler, D., Pitcairn, C. E. R., Sutton, M. A., Flechard, C., Loubet, B., Coyle, M., and Munro, R. C.: The mass budget of atmospheric ammonia in woodland within 1 km of livestock buildings, Environ. Pollut., 102, 343–348, https://doi.org/10.1016/S0269-7491(98)80053-5, 1998. a, b
Gailis, R. M., Hill, A., Yee, E., and Hilderman, T.: Extension of a fluctuating plume model of tracer dispersion to a sheared boundary layer and to a large array of obstacles, Bound.-Lay. Meteorol., 122, 577–607, https://doi.org/10.1007/s10546-006-9118-9, 2007. a, b
Galmarini, S., Vilà-Guerau de Arellano, J., and Duynkerke, P.: The effect of micro-scale turbulence on the reaction rate in a chemically reactive plume, Atmos. Environ., 29, 87–95, https://doi.org/10.1016/1352-2310(94)00224-9, 1995. a, b
Heus, T., van Heerwaarden, C. C., Jonker, H. J. J., Pier Siebesma, A., Axelsen, S., van den Dries, K., Geoffroy, O., Moene, A. F., Pino, D., de Roode, S. R., and Vilà-Guerau de Arellano, J.: Formulation of the Dutch Atmospheric Large-Eddy Simulation (DALES) and overview of its applications, Geosci. Model Dev., 3, 415–444, https://doi.org/10.5194/gmd-3-415-2010, 2010. a, b, c
Kljun, N., Kormann, R., Rotach, M. W., and Meixer, F. X.: Comparison of the lagrangian footprint model LPDM-B with an analytical footprint model, Bound.-Lay. Meteorol., 106, 349–355, https://doi.org/10.1023/A:1021141223386, 2003. a
Kulmala, M., Asmi, A., Lappalainen, H. K., Baltensperger, U., Brenguier, J.-L., Facchini, M. C., Hansson, H.-C., Hov, Ø., O'Dowd, C. D., Pöschl, U., Wiedensohler, A., Boers, R., Boucher, O., de Leeuw, G., Denier van der Gon, H. A. C., Feichter, J., Krejci, R., Laj, P., Lihavainen, H., Lohmann, U., McFiggans, G., Mentel, T., Pilinis, C., Riipinen, I., Schulz, M., Stohl, A., Swietlicki, E., Vignati, E., Alves, C., Amann, M., Ammann, M., Arabas, S., Artaxo, P., Baars, H., Beddows, D. C. S., Bergström, R., Beukes, J. P., Bilde, M., Burkhart, J. F., Canonaco, F., Clegg, S. L., Coe, H., Crumeyrolle, S., D'Anna, B., Decesari, S., Gilardoni, S., Fischer, M., Fjaeraa, A. M., Fountoukis, C., George, C., Gomes, L., Halloran, P., Hamburger, T., Harrison, R. M., Herrmann, H., Hoffmann, T., Hoose, C., Hu, M., Hyvärinen, A., Hõrrak, U., Iinuma, Y., Iversen, T., Josipovic, M., Kanakidou, M., Kiendler-Scharr, A., Kirkevåg, A., Kiss, G., Klimont, Z., Kolmonen, P., Komppula, M., Kristjánsson, J.-E., Laakso, L., Laaksonen, A., Labonnote, L., Lanz, V. A., Lehtinen, K. E. J., Rizzo, L. V., Makkonen, R., Manninen, H. E., McMeeking, G., Merikanto, J., Minikin, A., Mirme, S., Morgan, W. T., Nemitz, E., O'Donnell, D., Panwar, T. S., Pawlowska, H., Petzold, A., Pienaar, J. J., Pio, C., Plass-Duelmer, C., Prévôt, A. S. H., Pryor, S., Reddington, C. L., Roberts, G., Rosenfeld, D., Schwarz, J., Seland, Ø., Sellegri, K., Shen, X. J., Shiraiwa, M., Siebert, H., Sierau, B., Simpson, D., Sun, J. Y., Topping, D., Tunved, P., Vaattovaara, P., Vakkari, V., Veefkind, J. P., Visschedijk, A., Vuollekoski, H., Vuolo, R., Wehner, B., Wildt, J., Woodward, S., Worsnop, D. R., van Zadelhoff, G.-J., Zardini, A. A., Zhang, K., van Zyl, P. G., Kerminen, V.-M., S Carslaw, K., and Pandis, S. N.: General overview: European Integrated project on Aerosol Cloud Climate and Air Quality interactions (EUCAARI) – integrating aerosol research from nano to global scales, Atmos. Chem. Phys., 11, 13061–13143, https://doi.org/10.5194/acp-11-13061-2011, 2011. a
Loubet, B., Cellier, P., Milford, C., and Sutton, M. A.: A coupled dispersion and exchange model for short-range dry deposition of atmospheric ammonia, Q. J. Roy. Meteor. Soc., 132, 1733–1763, https://doi.org/10.1256/qj.05.73, 2006. a
Meeder, J. and Nieuwstadt, F.: Large-eddy simulation of the turbulent dispersion of a reactive plume from a point source into a neutral atmospheric boundary layer, Atmos. Environ., 34, 3563–3573, https://doi.org/10.1016/S1352-2310(00)00124-2, 2000. a
Mensah, A. A., Holzinger, R., Otjes, R., Trimborn, A., Mentel, Th. F., ten Brink, H., Henzing, B., and Kiendler-Scharr, A.: Aerosol chemical composition at Cabauw, The Netherlands as observed in two intensive periods in May 2008 and March 2009, Atmos. Chem. Phys., 12, 4723–4742, https://doi.org/10.5194/acp-12-4723-2012, 2012. a
Mylne, K. R. and Mason, P. J.: Concentration fluctuation measurements in a dispersing plume at a range of up to 1000 m, Q. J. Roy. Meteor. Soc., 117, 177–206, https://doi.org/10.1002/qj.49711749709, 1991. a, b, c, d, e
Nemitz, E., Sutton, M. A., Wyers, G. P., Otjes, R. P., Mennen, M. G., van Putten, E. M., and Gallagher, M. W.: Gas-particle interactions above a Dutch heathland: II. Concentrations and surface exchange fluxes of atmospheric particles, Atmos. Chem. Phys., 4, 1007–1024, https://doi.org/10.5194/acp-4-1007-2004, 2004. a, b
Nieuwstadt, F.: A large-eddy simulation of a line source in a convective atmospheric boundary layer – I. Dispersion characteristics, Atmos. Environ. A-Gen., 26, 485–495, https://doi.org/10.1016/0960-1686(92)90331-E, 1992. a
Ouwersloot, H. G., Vilà-Guerau de Arellano, J., van Heerwaarden, C. C., Ganzeveld, L. N., Krol, M. C., and Lelieveld, J.: On the segregation of chemical species in a clear boundary layer over heterogeneous land surfaces, Atmos. Chem. Phys., 11, 10681–10704, https://doi.org/10.5194/acp-11-10681-2011, 2011. a, b
Ouwersloot, H. G., Moene, A. F., Attema, J. J., and Vilà-Guerau de Arellano, J.: Large-Eddy Simulation Comparison of Neutral Flow Over a Canopy: Sensitivities to Physical and Numerical Conditions, and Similarity to Other Representations, Bound.-Lay. Meteorol., 162, 71–89, https://doi.org/10.1007/s10546-016-0182-5, 2017. a
Pino, D., Jonker, H., Vilà-Guerau de Arellano, J., and Dosio, A.: Role of Shear and the Inversion Strength During Sunset Turbulence Over Land: Characteristic Length Scales, Bound.-Lay. Meteorol., 121, 537–556, https://doi.org/10.1007/s10546-006-9080-6, 2006. a
Rannik, Ü., Aubinet, M., Kurbanmuradov, O., Sabelfeld, K. K., Markkanen, T., and Vesala, T.: Footprint Analysis For Measurements Over A Heterogeneous Forest, Bound.-Lay. Meteorol., 97, 137–166, https://doi.org/10.1023/A:1002702810929, 2000. a
Ražnjević, A., van Heerwaarden, C., van Stratum, B., Hensen, A., Velzeboer, I., van den Bulk, P., and Krol, M.: Technical note: Interpretation of field observations of point-source methane plume using observation-driven large-eddy simulations, Atmos. Chem. Phys., 22, 6489–6505, https://doi.org/10.5194/acp-22-6489-2022, 2022. a, b, c, d
Remmelink, G., van Middelkoop, J., Ouweltjes, W., and Wemmenhove, H.: Handboek melkveehouderij 2020/21, over the year 2019/20, no. 44 in Handboek/Wageningen Livestock Research, Wageningen Livestock Research, https://doi.org/10.18174/529557, 2020. a
RIVM: Landbouw, Emissiefactoren diercategorieën, Hoofdcategorie A: Rundvee, RIVM, https://www.infomil.nl/onderwerpen/landbouw/emissiearme-stalsystemen/emissiefactoren-per/map-staltypen/hoofdcategorie/, last access: 28 January 2021. a
Sauter, F., van Zanten, M., van der Swaluw, E., Aben, J., de Leeuw, F., and van Jaarsveld, H.: The OPS-model: Description of OPS 4.5.2, Tech. rep., National Institute for Public Health and the Environment (RIVM), https://www.rivm.nl/media/ops/OPS-model.pdf (last access: 23 June 2022), 2018. a
Schaap, M., Timmermans, R. M. A., Roemer, M., Boersen, G. A. C., Builtjes, P. J. H., Sauter, F. J., Velders, G. J. M., and Beck, J. P.: The LOTOS–EUROS model: description, validation and latest developments, Int. J. Environ. Poll., 32, 270–290, https://doi.org/10.1504/IJEP.2008.017106, 2008. a
Schaug, J.: Quality assurance plane for EMEP, Tech. Rep. EMEP/CCC-Report 1/88, Norwegian Institute for Air Research, https://projects.nilu.no/ccc/reports/cccr1-88.pdf (last access: 23 June 2022), 1988. a
Schulte, R., van Zanten, M., Rutledge-Jonker, S., Swart, D., Wichink Kruit, R., Krol, M., van Pul, W., and Vilà-Guerau de Arellano, J.: Unraveling the diurnal atmospheric ammonia budget of a prototypical convective boundary layer, Atmos. Environ., 249, 118153, https://doi.org/10.1016/j.atmosenv.2020.118153, 2021. a, b, c
Schulte, R., van Zanten, M., van Stratum, B., and Vilà-Guerau de Arellano, J.: DALES v4.2 modified for ammonia plume dispersion and blending-distance estimations, 4TU.ResearchData [code], https://doi.org/10.4121/19869478.v1, 2022. a, b, c
Shah, A., Pitt, J. R., Ricketts, H., Leen, J. B., Williams, P. I., Kabbabe, K., Gallagher, M. W., and Allen, G.: Testing the near-field Gaussian plume inversion flux quantification technique using unmanned aerial vehicle sampling, Atmos. Meas. Tech., 13, 1467–1484, https://doi.org/10.5194/amt-13-1467-2020, 2020. a, b
Shen, J., Chen, D., Bai, M., Sun, J., Coates, T., Lam, S. K., and Li, Y.: Ammonia deposition in the neighbourhood of an intensive cattle feedlot in Victoria, Australia, Sci. Rep., 6, 2045–2322, https://doi.org/10.1038/srep32793, 2016. a, b
Simpson, D., Benedictow, A., Berge, H., Bergström, R., Emberson, L. D., Fagerli, H., Flechard, C. R., Hayman, G. D., Gauss, M., Jonson, J. E., Jenkin, M. E., Nyíri, A., Richter, C., Semeena, V. S., Tsyro, S., Tuovinen, J.-P., Valdebenito, Á., and Wind, P.: The EMEP MSC-W chemical transport model – technical description, Atmos. Chem. Phys., 12, 7825–7865, https://doi.org/10.5194/acp-12-7825-2012, 2012. a
Smit, L. A. M. and Heederik, D.: Impacts of Intensive Livestock Production on Human Health in Densely Populated Regions, GeoHealth, 1, 272–277, https://doi.org/10.1002/2017GH000103, 2017. a
Sommer, S., Øtergård, H., Løfstrøm, P., Andersen, H., and Jensen, L.: Validation of model calculation of ammonia deposition in the neighbourhood of a poultry farm using measured NH3 concentrations and N deposition, Atmos. Environ., 43, 915–920, https://doi.org/10.1016/j.atmosenv.2008.10.045, 2009. a, b
Stokstad, E.: Nitrogen crisis threatens Dutch environment- and economy, Science, 366, 1180–1181, https://doi.org/10.1126/science.366.6470.1180, 2019. a
Stolk, A., Noordijk, H., and van Zanten, M.: Drogedepositiemetingen van ammoniak in Natura 2000-gebied Bargerveen, Tech. Rep. 680029001, National Institute for Public Health and the Environment (RIVM), Antonie van Leeuwenhoeklaan 9, 3721, MA Bilthoven, the Netherlands, https://rivm.openrepository.com/handle/10029/320523 (last access: 23 June 2022), 2014. a
Sutton, M. A., Erisman, J. W., Dentener, F., and Möller, D.: Ammonia in the environment: From ancient times to the present, Environ. Pollut., 156, 583–604, https://doi.org/10.1016/j.envpol.2008.03.013, 2008. a
Theobald, M. R., Løfstrøm, P., Walker, J., Andersen, H. V., Pedersen, P., Vallejo, A., and Sutton, M. A.: An intercomparison of models used to simulate the short-range atmospheric dispersion of agricultural ammonia emissions, Environ. Model. Softw., 37, 90–102, https://doi.org/10.1016/j.envsoft.2012.03.005, 2012. a, b
van Bruggen, C., Bannink, A., Groenestein, C., Huijsmans, J., Lagerwerf, L., Luesink, H., Ros, M., Velthof, G., Vonk, J., and van der Zee, T.: Emissies naar lucht uit de landbouw berekend met NEMA voor 1990–2019, no. 203 in WOt-technical report, Wettelijke Onderzoekstaken Natuur & Milieu, https://doi.org/10.18174/544296, 2021. a
van der Peet, G., Leenstra, F., Vermeij, I., Bondt, N., Puister, L., and van Os, J.: Feiten en cijfers over de Nederlandse veehouderijsectoren 2018, no. 1134 in Wageningen Livestock Research rapport, Wageningen Livestock Research, https://doi.org/10.18174/464128, 2018. a
van der Swaluw, E., de Vries, W., Sauter, F., Aben, J., Velders, G., and van Pul, A.: High-resolution modelling of air pollution and deposition over the Netherlands with plume, grid and hybrid modelling, Atmos. Environ., 155, 140–153, https://doi.org/10.1016/j.atmosenv.2017.02.009, 2017. a
van Jaarsveld, J.: The Operational Priority Substances model: Description and validation of OPS-Pro 4.1, Tech. Rep. 500045001, National Institute for Public Health and the Environment (RIVM), Antonie van Leeuwenhoeklaan 9, 3721, MA Bilthoven, the Netherlands, https://www.rivm.nl/publicaties/operational-priority-substances-model (last access: 23 June 2022), 2004. a
Van Oss, R., Duyzer, J., and Wyers, P.: The influence of gas-to-particle conversion on measurements of ammonia exchange over forest, Atmos. Environ., 32, 465–471, https://doi.org/10.1016/S1352-2310(97)00280-X, 1998. a
van Zanten, M., Wichink Kruit, R., Hoogerbrugge, R., Van der Swaluw, E., and van Pul, W.: Trends in ammonia measurements in the Netherlands over the period 1993–2014, Atmos. Environ., 148, 352–360, https://doi.org/10.1016/j.atmosenv.2016.11.007, 2017. a
van Zanten, M. C., Sauter, F. J., Wichink Kruit, R. J., van Jaarsveld, J. A., and van Pul, W. A. J.: Description of the DEPAC module: Dry deposition modelling with DEPAC-GCN2010, Tech. Rep. 680180001, National Institute for Public Health and the Environment (RIVM), https://www.rivm.nl/bibliotheek/rapporten/680180001.pdf (last access: 23 June 2022), 2010. a, b
Verzijlbergh, R. A., Jonker, H. J. J., Heus, T., and Vilà-Guerau de Arellano, J.: Turbulent dispersion in cloud-topped boundary layers, Atmos. Chem. Phys., 9, 1289–1302, https://doi.org/10.5194/acp-9-1289-2009, 2009. a
Vesala, T., Kljun, N., Rannik, Ü., Rinne, J., Sogachev, A., Markkanen, T., Sabelfeld, K., Foken, T., and Leclerc, M.: Flux and concentration footprint modelling: State of the art, Environ. Pollut., 152, 653–666, https://doi.org/10.1016/j.envpol.2007.06.070, 2008. a
Vilà-Guerau de Arellano, J., Talmon, A. M., and Builtjes, P.: A chemically reactive plume model for the NO-NO2-O3 system, Atmos. Environ. A-Gen., 24, 2237–2246, https://doi.org/10.1016/0960-1686(90)90255-L, 1990. a
Vilà-Guerau de Arellano, J., Dosio, A., Vinuesa, J.-F., Holtslag, A. A. M., and Galmarini, S.: The dispersion of chemically reactive species in the atmospheric boundary layer, Meteorol. Atmos. Phys., 87, 23–38, https://doi.org/10.1007/s00703-003-0059-2, 2004. a
Vonk, J., Arets, E., Bannink, A., van Bruggen, C., Groenestein, C., Huijsmans, J., Lagerwerf, L., Luesink, H., Ros, M., Schelhaas, M., van der Zee, T., and Velthof, G.: Referentieraming van emissies naar de lucht uit landbouw en landgebruik tot 2030, met doorkijk naar 2035: Achtergronddocument bij de Klimaat- en Energieverkenning 2020, no. 1278 in Rapport/Wageningen Livestock Research, Wageningen Livestock Research, https://doi.org/10.18174/533503, 2020. a
Vrieling, A. and Nieuwstadt, F.: Turbulent dispersion from nearby point sources – interference of the concentration statistics, Atmos. Environ., 37, 4493–4506, https://doi.org/10.1016/S1352-2310(03)00576-4, 2003. a, b
Wichink Kruit, R. and van Pul, W. A. J.: Ontwikkelingen in de stikstofdepositie, Rijksinstituut voor Volksgezondheid en Milieu (RIVM), RIVM briefrapport 2018-0117, https://doi.org/10.21945/RIVM-2018-0117, 2018. a
Wichink Kruit, R., Bleeker, A., Braam, M., van Goethem, T., Hoogerbrugge, R., Rutledge-Jonker, S., Stefess, G., Stolk, A., van der Swaluw, E., Voogt, M., and van Pul, A.: Op weg naar een optimale meetstrategie voor stikstof, Rijksinstituut voor Volksgezondheid en Milieu (RIVM), techreport, https://doi.org/10.21945/RIVM-2021-0118, 2021. a, b, c
Wichink Kruit, R. J., van Pul, W. A. J., Otjes, R. P., Hofschreuder, P., Jacobs, A. F. G., and Holtslag, A. A. M.: Ammonia fluxes and derived canopy compensation points over non-fertilized agricultural grassland in The Netherlands using the new GRadient Ammonia – High Accuracy – Monitoring (GRAHAM), Atmos. Environ., 41, 1275–1287, https://doi.org/10.1016/j.atmosenv.2006.09.039, 2007. a
Wichink Kruit, R. J., van Pul, W. A. J., Sauter, F. J., van den Broek, M., Nemitz, E., Sutton, M. A., Krol, M., and Holtslag, A. A. M.: Modeling the surface–atmosphere exchange of ammonia, Atmos. Environ., 44, 945–957, https://doi.org/10.1016/j.atmosenv.2009.11.049, 2010. a
WUR: Dutch Farm Accountancy Data Network, agriculture, WUR, https://www.agrimatie.nl/Binternet.aspx?ID=2&Bedrijfstype=2@3&SelectedJaren=2020&GroteKlassen=Alle+bedrijven, last access: 21 June 2021. a