the Creative Commons Attribution 4.0 License.
the Creative Commons Attribution 4.0 License.
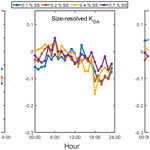
Measurement report: Distinct size dependence and diurnal variation in organic aerosol hygroscopicity, volatility, and cloud condensation nuclei activity at a rural site in the Pearl River Delta (PRD) region, China
Mingfu Cai
Baoling Liang
Qibin Sun
Li Liu
Min Shao
Weiwei Hu
Wei Chen
Qicong Song
Wei Li
Yuwen Peng
Zelong Wang
Duohong Chen
Haobo Tan
Hanbin Xu
Fei Li
Xuejiao Deng
Tao Deng
Jiaren Sun
Organic aerosol (OA) has a significant contribution to cloud formation and hence climate change. However, high uncertainties still exist in its impact on global climate, owing to the varying physical properties affected by the complex formation and aging processes. In this study, the hygroscopicity, volatility, cloud condensation nuclei (CCN) activity, and chemical composition of particles were measured using a series of online instruments at a rural site in the Pearl River Delta (PRD) region of China in fall 2019. During the campaign, the average hygroscopicity of OA (κOA) increased from 0.058 at 30 nm to 0.09 at 200 nm, suggesting a higher oxidation state of OA at larger particle sizes, supported by a higher fraction of extremely low volatility OA (ELVOA) for larger size particles. Significantly different diurnal patterns of κOA were observed between Aitken mode particles and accumulation mode particles. For Aitken mode particles (30–100 nm), the κOA values showed daily minima (0.02–0.07) during daytime, while the accumulation mode exhibited a daytime peak (∼ 0.09). Coincidently, a daytime peak was observed for both aged biomass burning organic aerosol (aBBOA) and less oxygenated organic aerosol (LOOA) based on source apportionment, which was attributed to the aging processes and gas–particle partitioning through photochemical reactions. In addition, the fraction of semi-volatile OA (SVOA) was higher at all measured sizes during daytime than during nighttime. These results indicate that the formation of secondary OA (SOA) through gas–particle partitioning can generally occur at all diameters, while the aging processes of pre-existing particles are more dominated in the accumulation mode. Furthermore, we found that applying a fixed κOA value (0.1) could lead to an overestimation of the CCN number concentration (NCCN) up to 12 %–19 % at 0.1 %–0.7 % supersaturation (SS), which was more obvious at higher SS during daytime. Better prediction of NCCN could be achieved by using size-resolved diurnal κOA, which indicates that the size dependence and diurnal variations in κOA can strongly affect the NCCN at different SS values. Our results highlight the need for accurately evaluating the atmospheric evolution of OA at different size ranges and their impact on the physicochemical properties and hence climate effects.
- Article
(3081 KB) -
Supplement
(1232 KB) - BibTeX
- EndNote
The impact of aerosol particles on global climate is widely known, including absorbing and scattering solar radiation and acting as cloud condensation nuclei (CCN). However, the extent of their contribution to the climate forcing is still unclear. Organic aerosol (OA) as a dominant component of fine particles (Jimenez et al., 2009) may contribute the uncertainties of climate effects of particles, mainly owing to unknown sources, physical properties, formation, and aging mechanisms (Volkamer et al., 2006; Kuang et al., 2020b; Rastak et al., 2017). Numerous studies show that secondary organic aerosol (SOA) accounts for a large OA fraction in most atmospheric environments (e.g., Huang et al., 2014; Shrivastava et al., 2017; Kanakidou et al., 2005; Hallquist et al., 2009). Nevertheless, both primary OA (POA) and SOA in the ambient air remain poorly characterized in terms of the formation mechanism and atmospheric evolution, and their particle diameter can vary on a large scale. Their impact on the global climate and atmospheric chemistry is still highly uncertain.
A combination of Aerodyne aerosol mass spectrometer (AMS) or aerosol chemical species monitor (ACSM) with positive matrix factorization (PMF) is widely used for investigating the OA evolution in the atmosphere (Li et al., 2013; Huang et al., 2018, 2014; Chen et al., 2015; Jimenez et al., 2009). For instance, Qin et al. (2017) found that hydrocarbon-like OA (HOA) from traffic emission contributed up to 40 % of OA during nighttime, owing to daytime traffic restrictions on heavy vehicles in urban Guangzhou. Kuang et al. (2020a) reported a dominant contribution to oxygenated OA (OOA) through aqueous-phase reaction in the North China Plain (NCP). Guo et al. (2020) observed different SOA mechanisms between clean and pollution episodes in the Pearl River Delta (PRD) region. Nevertheless, the investigation of bulk OA is still insufficient in understanding the aerosol climate effects without the size-resolved characterization. The OA size distribution is largely dependent on its composition, sources, and aging level. Li et al. (2012) observed various mass distribution patterns for different species in airborne particulate organics and reported that dehydrated sugars, fossil-fuel-derived n-alkanes, and PAHs showed a unimodal distribution, while non-dehydrated sugars and plant wax were derived as n-alkanes which presented a bimodal pattern. In the urban region, the Aitken mode was mainly dominated by HOA owing to traffic emissions (Zhang et al., 2005b; Cai et al., 2020). In the marine atmosphere, the size distribution of fine-mode POA was found to be independent of sea salt, while coarse-mode particles tended to be internally mixed with sea salt (Gantt and Meskhidze, 2013). Similarly, the OA physical properties were also found to be size-dependent. Deng et al. (2018) reported a higher OA hygroscopicity (κOA≈ 0.22) at about 150 nm than that (κOA≈ 0.19) at sub-100 nm at a forest site. In contrast, Zhao et al. (2015) measured size-dependent hygroscopicity and chemical composition for SOA from various procedures and found that κOA of SOA from α-pinene photooxidation decreased from 0.17 at 50 nm to 0.07 at 200 nm, which was attributed to the higher oxidation degree for smaller particles.
The size dependence of OA properties in the aforementioned studies might exert impact on the CCN prediction, which is mainly determined by their sources and formation processes. Cai et al. (2018) found that NCCN at 0.1 % SS was underestimated by about 10 % if a κOA value of 0.1 was used. A model simulation from Liu and Wang (2010) showed that an increase of about 40 %–80 % for the CCN concentration was obtained by increasing the κ value of POA from 0 to 0.1. Wang et al. (2008) reported that the uncertainties of the first indirect aerosol effect varied from −0.2 to 0.2 W m−2 for a κOA value of 0 to 0.25. Rastak et al. (2017) showed that using a single-parameter framework of κOA in evaluating the climate effects of aerosol could lead to significant errors (about −1.02 W m−2), which is the same order as the climate forcing of anthropogenic aerosol during the industrial period. These results further highlight a need for the understanding of the relationship between the OA evolution processes and its impact on the CCN activity at different particle sizes.
The OA hygroscopicity and volatility can provide information about the evolution of OA, given that they are often related to the chemical composition of the particles. A positive correlation between the hygroscopicity values and the oxidation degree of OA, including the ratio of atomic oxygen to atomic carbon (O:C), the oxidation state (), or the mass fraction of 44 (for ) ion fragments in the organic spectra (f44) from chemical composition, was widely reported in the literature (Wu et al., 2013; Pajunoja et al., 2015; Chang et al., 2010). Kim et al. (2020) found that the κOA was positively and negatively correlated with OOA and HOA at different size ranges, respectively. Deng et al. (2019) reported a decreasing trend of κOA at a size range of 100–360 nm during daytime in a forest environment, suggesting the formation of biogenic SOA (BSOA) through photochemical oxidation of biogenic volatile organic compounds (BVOCs). The OA volatility, specifically saturation vapor concentration (C∗), is linked to the gas–particle partitioning and aging processes. In general, the C∗ value decreases with an increase in the oxidation degree and the number of atomic carbon (Donahue et al., 2011). May et al. (2013) found that most of the biomass-burning POA was semi-volatile. Saha et al. (2017) showed a lower volatility of OA in the afternoon hours using a dual-thermodenuder (TD) system, probably owing to photochemical oxidation of OA. Hong et al. (2017) derived the OA volatility distribution by a combination of the VTMDA measurement and a multi-component evaporation dynamics model and found a moderate (R≈ 0.4) correlation between the OA groups obtained by the VTDMA data and the PMF results, respectively.
In this study, we investigate physical properties of OA at different size ranges and evaluate their influence on the atmospheric CCN concentration. A rural field measurement was conducted at the Heshan site in the Pearl River Delta (PRD) region, China, during fall 2019 (October and November). The hygroscopicity, volatility, size-resolved CCN activity, and chemical composition were measured by a series of online instruments. The size-resolved hygroscopicity and volatility distribution of organics were investigated. PMF was employed to analyze the sources and processes of OA. The impact of diurnal variation and size dependence of κOA on the NCCN prediction at different supersaturation (SS) was assessed.
2.1 Measurement site
The field measurements were conducted at the Heshan supersite in Guangdong Province, China during fall 2019 (27 September to 17 November 2019). This supersite (22∘42′39.1′′ N, 112∘55′35.9′′ E) is located in the southwest of the PRD region and surrounded by farms and villages, with an altitude of about 40 m. All sample particles first passed through a Nafion dryer (model MD-700, Perma Pure Inc., USA) to maintain a relative humidity (RH) lower than 30 %. The schematic diagram of the experimental setup can be found in Fig. S1 in the Supplement. Detailed descriptions of the measurement site and some instruments can be found in Cai et al. (2021a).
2.2 Instrumentation
2.2.1 Aerosol hygroscopicity and volatility measurement
Size-resolved hygroscopicity and volatility of particles were measured by a H/V-TDMA (model M3000, Bmet Inc., China). The instrument consists of two differential mobility analyzers (DMA1 and DMA2, model 3081 L, TSI Inc., USA), a Nafion humidifier (model MD-700, Perma Pure Inc., USA), a heater tube, and a condensation particle counter (CPC, model 3788, TSI Inc., USA). The instrument was operated in H- and V-mode during the measurement with a cycle time of about 3–4 h. The dried sample particles were firstly charged by an X-ray neutralizer and then classified by DMA1 at six diameters (30, 50, 80, 100, 150, and 200 nm). In the H-mode, the chosen particles with a specific dry diameter (D0) were sequentially humidified by the Nafion humidifier to achieve 90 % of RH. A combination of DMA2 and CPC was employed to measure the size distribution of humidified particles (). The hygroscopic growth factor (GF) at a certain dry diameter can be defined as
In the V-mode, the selected particles from DMA1 were heated in the heater tube at 100, 150, 200, and 250 ∘C, respectively. Similar to the H mode, the size distribution of heated particles along with particles at room temperature (25 ∘C) was measured by the DMA2 and CPC. The volatility shrink factor (VSF) at a certain diameter and temperature is then defined as
Before the campaign, standard polystyrene latex spheres (PSLs; with a size of 20, 50, and 200 nm), ammonium sulfate, and sodium chloride were used to calibrate the diameter classification of DMAs, hygroscopicity measurement, and transport efficiency of particles in the heater tube, respectively. For the H/V-TDMA data, the TDMAfit algorithm (Stolzenburg and McMurry, 2008) was applied to fit the growth factor and volatility shrink factor probability density function (GF-PDF and VSF-PDF) with various DMA transfer functions. The detailed data inversion processes can be found in Tan et al. (2013a).
2.2.2 The size-resolved CCN activity and particle number size distribution measurement
A combination of a cloud condensation nuclei counter (CCNc, model 200, DMT Inc., USA) and a scanning mobility particle sizer (SMPS, model 3938L75, TSI Inc., USA) was employed to measure size-resolved CCN activity. The supersaturation (SS) of each column (A and B) of CCNc was set to be 0.1 %, 0.2 %, and 0.4 % (for column A) and 0.7 %, 0.9 %, and 1.0 % (for column B), respectively. During the measurement, the SMPS was operated at a scanning mode. The sample particles were firstly neutralized by an X-ray neutralizer (model 3088, TSI Inc., USA) and were subsequently classified by a DMA. The classified particles were then split into three paths: one to a CPC (model 3756, TSI Inc., USA) for measurement of particle number concentration (with a flow rate of 0.6 L min−1) and two to the CCNc for measurement of the CCN number concentration (NCCN) at a specific SS (with a flow rate of 0.5 L min−1).
The particle number size distribution (PNSD) in a size range of 1 nm–10 µm was measured by a suite of instruments including a diethylene glycol scanning mobility particle sizer (DEG-SMPS, model 3938E77, TSI Inc., USA), a SMPS (model 3938L75, TSI Inc., USA), and an aerodynamic particle sizer (APS, model 3321, TSI Inc., USA). The detailed description of these instruments can be found in Cai et al. (2021a). Before the measurement, the SMPSs were calibrated with PSLs (20, 50, and 200 nm), and the CCNc was calibrated with ammonium sulfate ((NH4)2SO4) particles at selected SSs (0.1 %, 0.2 %, 0.4 %, 0.7 %, 0.9 %, and 1.0 %, Sect. S1 in the Supplement).
2.2.3 Aerosol chemical composition measurement
The size-resolved chemical composition of ambient aerosol particles was measured by a soot particle aerosol mass spectrometer (SP-AMS, Aerodyne Research, Inc., USA). The principle and operation of the instrument are generally the same as a high-resolution time-of-flight aerosol mass spectrometer (HR-ToF-AMS) (Canagaratna et al., 2007). In addition to an original tungsten vaporizer (∼ 600 ∘C), a soot-particle module which mainly contains a Nd:YAG (1064 nm) laser was integrated into the HR-ToF-AMS for vaporizing refractory species (Onasch et al., 2012). As a result, the SP-AMS can provide chemical information for non-refractory species (nitrate, sulfate, ammonium, chloride, and organics) as well as refractory species such as refractory black carbon (rBC) and several metals. During the campaign, the SP-AMS was run between V mode (only tungsten vaporizer) and SP mode (tungsten and laser vaporizers) with a time resolution of 1 min. In order to minimize disturbance caused by mode switch, 15 min averaged data are used in the present study. More details on the quantification using ionization efficiency, composition-dependent collection efficiency, and external instrument as well as software for SP-AMS data analysis could be found in Kuang et al. (2021).
Facilitated by the time-of-flight chamber in the SP-AMS, the particle mass size distribution can be measured in submicrometer size range, specifically 40 to 800 nm in vacuum aerodynamic diameter (Dva). The mass size distribution for relevant AMS species was used in this study for investigating the link between particle chemical composition and volatility/hygroscopicity. Since SP-AMS provided the size distribution versus Dva, the equation below was used to convert Dva into mobility diameter (Dp).
where S is the shape factor, ρp is the particle density, and ρ0 is the density for water (1 kg m−3). In this study, we estimate that the particles were close to sphere due to high RH in the PRD, and thus a shape factor of 0.8 was applied. An overall particle density of 1.6 kg m−3 is used.
Based on high-resolution data from the SP-AMS, source apportionment was performed for organic aerosols (OAs) in the bulk PM1 with positive matrix factorization (PMF, Paatero, 1997; Paatero and Tapper, 1994) following the instruction in Ulbrich et al. (2009). The input data, selection of solutions, mass spectral profile, and time series of each factor can be found in Kuang et al. (2021). In brief, OA measured at the Heshan site could be divided into six components with identified sources and processes, including two from primary sources and four factors corresponding to secondary formation: a hydrocarbon-like OA (HOA) contributed mainly by vehicle exhausts mixed with cooking emissions, a biomass burning OA (BBOA) related to biomass burning combustion from the surrounding villages, an aged BBOA (aBBOA), a more oxygenated OA (MOOA) from regional transport, a less oxygenated OA (LOOA) provided by daytime photochemical formation, and a nighttime-formed OA (Night-OA) related to secondary formation during nighttime.
2.3 Methodology
2.3.1 Estimates of hygroscopicity
The hygroscopicity parameter κ can be obtained under subsaturation conditions by the H/V-TDMA measurement and supersaturation conditions by the CCNc measurement. The κ value (κHTDMA) can be estimated from the growth factor measured by the H/V-TDMA (Petters and Kreidenweis, 2007):
where σs/a is the surface tension of the solution–air interface and the solution is temporarily assumed to be pure water (0.0728 N m−1 at 298.15 K), Mw is the molecular weight of water (0.018 kg mol−1), R is the universal gas constant (8.314 ), T is the thermodynamic temperature in kelvin (298.15 K), ρw is the density of water (about 997.04 kg m−3 at 298.15 K), and Dp is the particle diameter in meters.
For the CCNc measurement, the κ value (κCCN) is calculated from the critical supersaturation (Sc) and the critical diameter (D50) by the following equation (Petters and Kreidenweis, 2007):
The critical diameter, D50, is defined as the diameter at which 50 % of the particles are activated at a specific SS and can be obtained from the NCCN and NCN measured by the CCNc and SMPS system:
where the B and C are fitting coefficients.
2.3.2 Derivation of the size-resolved hygroscopicity of organic matter
The size-resolved chemical composition is adopted to derive the size-dependent hygroscopicity of organic matter (κOA). However, the AMS cannot provide sufficient information of the size-resolved species, especially for small size particles (< 100 nm) owing to the low mass concentration. Thalman et al. (2017) proposed a method to reconstruct the size-resolved chemical composition, which combines a time-resolved bulk mass concentration and an average mass distribution. Nevertheless, the variation in mass distribution was not considered in this method. In this study, a bimodal lognormal distribution function method was adopted, and the 1 h average mass distribution was fitted to obtain the reconstructed size-resolved chemical composition. The average mass distribution with bimodal lognormal fitted modes of each species was shown in Fig. S2 in the Supplement.
According to the ZSR mixing rule (Zdanovskii, 1948; Stokes and Robinson, 1966), the hygroscopicity of particles (κAMS) can be calculated based on the SP-AMS measurement, assuming an internal mixing state for all particles:
where κi is the κ value of each component and εi is the volume fraction of corresponding species in particles. The mole concentrations of the inorganic species are estimated based on the , , and measured by the AMS (Gysel et al., 2007).
Here n denotes the number of moles of each component (, , and ), and εorg and εBC were obtained from mass concentration measured by the SP-AMS. The density and κ value of each component are listed in Table 1.
Table 1The density and the κ value of the related species used in this study.
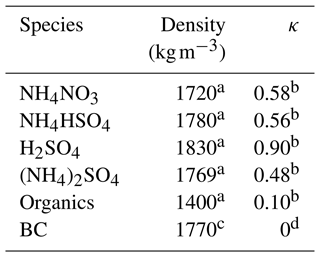
a From Gysel et al. (2007). b From (Cai et al., 2018). c From Deng et al. (2019). d Assumed to be 0.
The κOA can be calculated based on the size-resolved chemical composition and H/V-TMDA measurement using the following equation:
Table 2Thermophysical properties of each component used in the multi-component evaporation dynamics model.
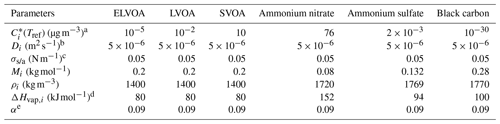
a From Hong et al. (2017). b From Riipinen et al. (2010). c From Riipinen et al. (2010). d The ΔHvap values of organics are obtained from the sensitivity test shown in Fig. S4, and the values of inorganic species are from Hong et al. (2017). e Obtained from the sensitivity test shown in Fig. S4.
2.3.3 Volatility data
During the heating process, some particles could be lost between DMA1 and DMA2 due to complete evaporation (CV), thermophoresis, and Brownian diffusion (Philippin et al., 2004). Owing to these losses, the V-mode measurement does not represent the actual volatility distribution of the monodisperse particles. The sodium chloride (NaCl) particles, which do not evaporate at the set temperature in this measurement, were used to determine the particle losses owing to thermophoretic forces and diffusion. The size- and temperature-dependent transmission efficiency (η(DpT)) of NaCl in the heater was shown in Fig. S3 in the Supplement. Thus, the number fraction of the CV group (NFCV(DpT)) at a certain diameter and temperature can be calculated using the following equation (Cheung et al., 2016):
where N′(DpT) is the number concentration of particles at a specific diameter and temperature after heating, which was measured by the CPC in the H/V-TDMA. The N(Dp) is the number concentration of particles with a diameter Dp before heating, which was provided by the SMPS measurement. The volume fraction remaining (VFR) after heating for the measured particles can be obtained according to the following equation:
where i represents the ith VSF bin, and NFi is the number fraction of particles with VSFi, which is calculated based on the VSF-PDF (c(VSFDpT)):
The mass fraction remaining (MFR) was assumed to be proportional to VFR, assuming that the density of particles was constant before and after heating.
2.3.4 Multi-component evaporation dynamics model
Based on the volatility basis set (VBS) framework (Donahue et al., 2011), the organic matter was classified into three organic groups based on the saturation concentration (C∗(Tref), Tref = 298.15 K): extremely low-volatility organic aerosol (ELVOA, C∗ = 10−5 µg m−3), low-volatility organic aerosol (LVOA, C∗ = 10−2 µg m−3), and semi-volatile organic aerosol (SVOA, C∗ = 10 µg m−3).
A multi-component evaporation dynamics model described by Lee et al. (2011) was used to simulate the evaporation of particles in the heated tube of the H/V-TDMA by solving the mass transfer regime equation, in order to obtain the size-resolved distribution of the aforementioned three OA groups. The MFR, residence time (about 4.11 s) in the heater tube, temperature of the heater tube, particle number concentration, particle sizes, chemical composition, and thermophysical properties of each species (Table 2) were input into the model. The particles were assumed to be internally mixed with organic and inorganic species, including three organic groups, NH4NO3, (NH4)2SO4, and black carbon (BC). The mass transfer of each component i between the aerosol and gas phases in the transition regime was calculated from the following equation:
where mp,i (µg) is the mass of species i in a single particle, Ci,g (µg m−3) is its gas-phase concentration, Di (m2 s−1) is the diffusion coefficient for species i in air, Dp (m) is the particle diameter, f(Kn,α) is a correction term to account for non-continuum mass transfer depending on Knudsen number (Kn) and mass accommodation coefficient (α), fi is the mole fraction of species i, is the saturation concentration at temperature (T) of the heater tube, Mi (kg mol−1) is the molecular weight of species i, ρi (kg m−3) is its density, and Np(Dp) (cm−3) is the number concentration of particles with a diameter Dp.
The correction term f(Kn,α) is determined by the following equation (Seinfeld and Pandis, 2016):
where λi is the mean free path of species i in the air, which is defined as . The ci is the mean speed of species i and .
The temperature-dependent is estimated from the Clausius–Clapeyron equation:
where ΔHvap,i (kJ mol−1) is the enthalpy of vaporization. The known mass fractions of NH4NO3, (NH4)2SO4, and BC were calculated based on the SP-AMS measurement. The time step of the model was set to be 10−3 s. The characteristics of each species were listed in Table 2. The mass fraction of each organic group in different particle sizes was derived by minimizing the squared residual (SSR) values, . The non-linear constrained optimization function “fmincon” in MATLAB (version 2016a, Mathworks Inc.) was used to obtain the optimal fitted result. A constraint of is used.
The modeled MFR is strongly dependent on the values of vaporization enthalpy (ΔHvap) and mass accommodation coefficient (α) (Lee et al., 2010, 2011). Thus, a sensitivity test is performed to determine the ΔHvap of OA and α based on the campaign average data (Fig. S4 in the Supplement). A linear relationship was adopted between ΔHvap and , ΔHvap = , where a and b are fitting parameters (Epstein et al., 2010). The a and b values are set to be [0, 4, 8, 12] and [50, 80, 100, 150, 200] in the sensitivity test, respectively, along with α = [0.01, 0.09, 0.1, 0.5, 0.7, 0.9, 1]. The results show that the measured MFR was reproduced well (with the lowest SSR of 0.0205, Fig. S5 in the Supplement) by using ΔHvap = 80 kJ mol−1 with α of 0.09, 0.1, and 0.7, respectively. For simplicity, ΔHvap = 80 kJ mol−1 and α = 0.09 are considered to be the best estimation and adopted in the simulation of the whole campaign datasets. The extracted α value was consistent with the values (α≤0.1) reported previously (Saha et al., 2015; Park et al., 2013; Saleh et al., 2008; Cappa and Jimenez, 2010), indicating significant resistance to mass transfer during evaporation. In addition, the ΔHvap of OA is of the same magnitude (80–150 kJ mol−1) as reported in the literature (Hong et al., 2017; Saha et al., 2017; Riipinen et al., 2010).
Note that the decomposition of particles during the heating process is ignored in the model. Kiyoura and Urano (1970) suggested that ammonium sulfate would decompose to ammonium bisulfate (NH4HSO4) or triammonium hydrogen sulfate (NH4)3H(SO4)2 and ammonia (NH3) when heated to around 160–180 ∘C. Wang and Hildebrandt Ruiz (2018) also observed thermal decomposition of organics and ammonium sulfate during evaporation by using a filter inlet for gases and aerosols chemical-ionization mass spectrometer (FIGAERO-CIMS). It suggests that, besides sublimation, decomposition might occur during evaporation of particles. However, the mechanisms of decomposition are complex and remain unclear, which is difficult to simulate in our model. We roughly estimated uncertainty caused by the decomposition and found that ignoring the decomposition of organics would lead to an underestimation of SVOA, while the decomposition of (NH4)2SO4 played a minor role in the simulation (Sect. S2 in the Supplement). However, the exact effects are still highly uncertain. We hence exclude the decomposition of particles from the model for simplicity.
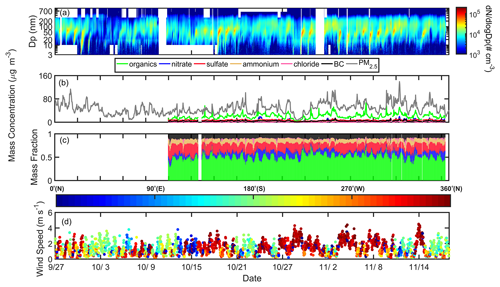
Figure 1The temporal profile of the measured variables during the campaign. (a) Particle number size distribution; (b) PM1 chemical composition measured by the SP-AMS along with mass concentration of PM2.5; (c) mass fraction of each species; (d) wind speed and direction. The color code in (d) represents the wind direction.
3.1 Overview
Figure 1 shows the temporal profile of PNSD (a), aerosol chemical composition and total mass concentration of PM2.5 (b), mass fraction of each component (c), and wind speed and direction (d) during the measurements. Note that the SP-AMS measurement started on 12 October. According to the PNSD data, a total number of 20 new particle formation (NPF) events were observed during the whole campaign. The background particles mainly exhibited unimodal distribution, which peaked at a size range of about 80–150 nm. The average particle number concentration (NCN) in the size range of 3–1000 nm was about 12 700 cm−3, much lower than that from the rural measurement (18 150 cm−3) in 2006 in the PRD region (Rose et al., 2010). A wide accumulation mode was observed during the period with a prevalent north wind direction, implying that the air mass from the north could bring pollutants from the city cluster around Guangzhou to the measurement site.
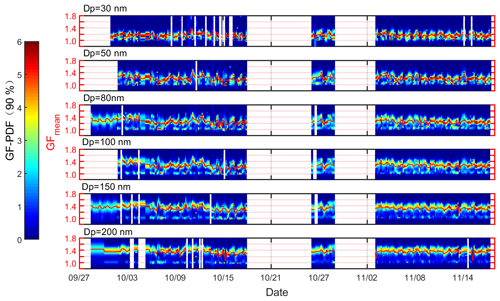
Figure 2The temporal profile of GF-PDF at the measured diameters (30, 50, 80, 100, 150, and 200 nm). The color code denotes the probability density, and the red solid line represents the mean GF (GFmean).
Table 3The average and standard deviation values (mean ± SD) of NCCN, AR, D50, and κCCN at 0.1 %, 0.2 %, 0.4 %, 0.7 %, 0.9 %, and 1.0 % SS during the campaign.

The chemical composition and the corresponding mass fraction measured by the SP-AMS (Fig. 1b and c) were consistent with those of PNSD, which showed a significantly high mass concentration of organics when the wind was from the north. The average mass fraction of PM1 was dominated by organics (51.8 %), followed by sulfate (17.5 %), nitrate (10.2 %), BC (9.9 %), ammonium (8.8 %), and chloride (1.7 %). The mass concentration of organics varied from 3.3 to 123.4 µg m−3, with an average value of 20.3 µg m−3, lower than the value (25.7 µg m−3) reported in Guangzhou City (Qin et al., 2017), but significantly higher than that was observed (4.1 µg m−3) in Hong Kong (Lee et al., 2013). The mass distribution of the chemical species at the Heshan site was similar to that measured in inland China (Chen et al., 2015; Huang et al., 2014), which was dominated by organics from anthropogenic emissions. A distinguished and reproducible diurnal pattern of the mass fraction was observed during the measurement (Fig. 1c), implying that the particle composition was more affected by local emission or photochemical production than other pathways. Organics showed a diurnal pattern with bimodal peaks in the afternoon and evening, which will be discussed later in Sect. 3.3. The temporal profile of GF-PDF (Fig. 2) measured by the H/V-TDMA was consistent with that of chemical composition, which showed a significant diurnal pattern. This suggested that particles at all diameters could be affected by atmospheric chemical processes and local emissions, which will be further discussed in Sect. 3.3. The H/V-TDMA data from 18–26 October and 29 October to 3 November were not available due to instrumental failure. In general, the GF-PDF exhibited a bimodal distribution for particles larger than 30 nm, with a significant more-hygroscopic (MH, GF > 1.33) or less-hygroscopic (LH, 1.11 < GF < 1.33) mode and a less obvious non-hygroscopic mode (NH, GF < 1.11), indicating that these particles were partly externally mixed. The NH mode with primary emissions (e.g., fresh black carbon and some organics) was more obvious in a size range of 50–150 nm than others, suggesting that these particles were more affected by local anthropogenic emissions. The above observation was supported by the size distribution of the BC mass faction (Fig. S7 in the Supplement), which peaked at a size range of about 50–150 nm. Besides, the MH mode shifted to a higher GF value with an increase in particle sizes, implying that larger particles were more aged with a higher fraction of inorganic salt (Fig. S7) and well separated from the freshly emitted counterparts. A similar phenomenal pattern was previously observed in the urban environment, including the PRD region (Hong et al., 2018; Cai et al., 2017; Jiang et al., 2016; Tan et al., 2013b), the North China Plain (Liu et al., 2011; Ma et al., 2016), and other city regions around the world (Yuan et al., 2020; Mochida et al., 2006; Massling et al., 2005).
Table 3 summarizes the NCCN, activation ratio (AR), D50, and κCCN values at 0.1 %, 0.2 %, 0.4 %, 0.7 %, 0.9 %, and 1.0 % SS during the campaign. The activation ratio is defined as the ratio of NCCN to NCN, that is, . The average NCCN at 0.1 %, 0.2 %, 0.4 %, 0.7 %, 0.9 %, and 1.0 % SS was about 2507, 4322, 5854, 6834, 7497, and 7862 cm−3, respectively. The NCCN at 0.7 % SS was lower than that measured (7900 cm−3 at 0.7 % SS) in urban Guangzhou (Cai et al., 2018) and at a suburban site (14 400 cm−3 at 0.864 % SS) in the North China Plain (Zhang et al., 2020) but significantly higher than that measured at an urban site (2776 cm−3 at 0.68 % SS) in São Paulo, Brazil (Almeida et al., 2014). The average AR at the above six SS values was 0.20, 0.34, 0.45, 0.52, 0.57, and 0.60. The AR at 0.7 % SS was lower than the measured value (0.64 at 0.7 % SS) in urban Guangzhou (Cai et al., 2018), while the corresponding D50 (52.56 nm) was lower than that (58.45 nm) in the Guangzhou campaign, implying a higher CCN activity at this site. Thus, the lower AR in this fall campaign suggested that particles were more centered at smaller sizes, which might be attributed to frequently occurring NPF at the Heshan site. The average κ values obtained using the HTDMA fall in a range of 0.1–0.17 at 30–200 nm (Fig. S8a in the Supplement), which were possibly attributed to a high fraction of organic matter (Fig. S7). The κAMS is slightly higher than the κHTDMA, and the differences become larger with decreasing particle sizes. This was probably due to the overestimated κOA at a small size range, which will be discussed in the next section. The hygroscopicity parameter κ values obtained by the CCNc method were 0.48, 0.47, 0.31, 0.22, 0.20, and 0.20 at the above SS values, which were much higher than those measured by the HTDMA in this study. This significant discrepancy between the measured κCCN and κHTDMA values might suggest that the water uptake behavior is different under super- and sub-saturation conditions, which is likely attributed to the surfactant effect. It was reported that organic matter in the particles could serve as a surfactant and lower surface tension by about 0.01–0.032 N m−1, leading to a higher CCN activity and thus a higher κCCN (Petters and Kreidenweis, 2013; Ovadnevaite et al., 2017; Liu et al., 2018). According to Eqs. (4) and (5), the κCCN was more susceptibly affected by the value of surface tension than that of κHTDMA, which would lead to the discrepancy between κCCN and κHTDMA values. The surfactant effect is closely related to the presence of liquid–liquid phase separation (LLPS) for organic-containing particles at high RH (Renbaum-Wolff et al., 2016; Ruehl and Wilson, 2014). Once LLPS occurred, the organic film on the droplet surface would decrease surface tension and enhance water uptake. For particles of organic–inorganic mixture, the LLPS can occur when the O:C is lower than 0.8 (Bertram et al., 2011; Song et al., 2012a, b; Schill and Tolbert, 2013). The average O:C obtained using AMS is about 0.53 in this campaign, suggesting that the LLPS likely occurred at supersaturation conditions. Meanwhile, the variation in the discrepancy between κCCN and κHTDMA is statistically insignificant during clean and polluted periods (Fig. S8b and c), implying that the surfactant effect was hardly affected by pollution conditions. Note that surface tension effect is not the only factor which leads to a higher κCCN. It was found that κCCN could be higher than κHTDMA, since the existence of the slightly soluble compounds inhibits water uptake under subsaturation conditions (Zhao et al., 2016; Pajunoja et al., 2015; Dusek et al., 2011; Petters et al., 2009; Hong et al., 2014; Hansen et al., 2015). Other factors, such as different parameters used in the CCNc and HTMDA calibration and function groups associated with the carbon chain, can lead to a gap between κHTDMA and κCCN (Rose et al., 2008; Wex et al., 2009). More future work is needed to better understand this water uptake mechanism and to improve the prediction of aerosol–cloud–climate interactions.
3.2 The average size-resolved hygroscopicity and volatility of OA
The composition of organics could vary on a large scale with diameters due to different sources and aging processes, which would further affect their properties. Figure 3 presents the average size-resolved hygroscopicity and volatility of OA. The κOA values (vertical red lines in Fig. 3) ranged from 0.058 to 0.09, within the range (0.05–0.15 at 100 nm) previously reported in the PRD region (Hong et al., 2018) and slightly higher than that (0.03–0.06 at 250 nm) at a mountain site in Germany (Wu et al., 2013). In general, the κOA values increased with particle sizes from 0.058 at 30 nm to 0.09 at 150 and 200 nm, similar to the feature observed in urban and forest environments (Kim et al., 2020; Deng et al., 2019). The increases in the κOA values with particle sizes could be explained by the oxidation level of organic aerosols (Massoli et al., 2010; Lambe et al., 2011; Xu et al., 2021). Specifically, the hygroscopicity of OA was often found to be positively correlated to its oxidation level (Mei et al., 2013; Lambe et al., 2011), which was usually represented by f44, ratio, or . Thus, the higher κOA values at larger particle diameters in this study might correspond to a higher aging degree of these particles, and this was confirmed by the increasing trend of f44 with particle diameters, i.e., the increasing fraction of in OA in large particles (Fig. S9 in the Supplement). Previous field studies also indicated that f44 increased with particle diameters (Kim et al., 2020; Cai et al., 2018), leading to a higher κOA value.
Besides the hygroscopicity of OA, we observed the size dependence of volatility. As shown in Fig. 3, the mass fraction of ELVOA increases from 0.16 to 0.30 with the particle diameter, indicating that the particles could be more aged at larger diameters, consistent with the higher κOA values as discussed above. The ELVOA fraction in this campaign was higher than that in Beijing in summer (0.13) measured by a thermodenuder (TD) coupled to an AMS (Xu et al., 2019), but similar to that in Athens (0.3) using a similar TD system (Louvaris et al., 2017). The SVOA generally contributed 42 %–57 % to the OA at all measured sizes, comparable to the values reported in Centreville and Raleigh (66 %–75 %, Saha et al., 2017), Beijing (64 %, Xu et al., 2019), and Mexico City (39 %–73 %, Cappa and Jimenez, 2010). Note that the relationship between volatility and oxidation state of OA is not usually strong. Saha et al. (2017) reported weak correlations (R < 0.3) between the mean volatility () and the mean oxidation state (). Hong et al. (2017) also found that the volatility distribution of OA derived from the combined V-TDMA and evaporation dynamic model could not be fully explained by the organic factions determined by the PMF analysis based on the AMS data. This is probably because the volatility was dependent on not only the but also the number of atomic carbon (Donahue et al., 2011). In spite of this, the size-resolved volatility distribution can provide a rough estimate of the aging degree of OA.
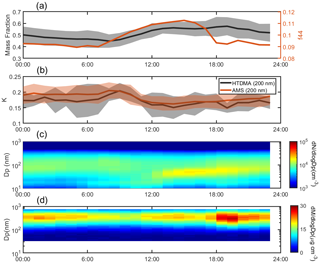
Figure 4The campaign average diurnal variation in mass fraction of organics and f44 in bulk PM1 (a), the κ values at 200 nm obtained by HTDMA (κHTDMA) and AMS (κAMS) measurements (b), and the PNSD (c) and mass distribution of organics (d). The shaded area represents standard deviation.
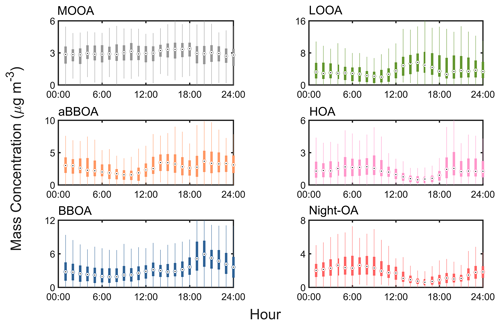
Figure 5The diurnal variation (displayed in boxplot) mass concentration of the deconvolved OA factors from PMF analysis of AMS data, including more oxygenated OA (MOOA), less oxygenated OA (LOOA), aged biomass burning OA (aBBOA), hydrocarbon-like OA (HOA), biomass burning OA (BBOA), and nighttime OA (night-OA).
3.3 The diurnal variation in OA hygroscopicity and volatility
As discussed in Sect. 3.2, the hygroscopicity and volatility of OA could vary on a large range with particle diameters, which might be attributed to photochemical reactions and the OA sources. In this section, the diurnal variation in hygroscopicity and volatility of OA at different particle sizes was investigated, in combination with the PMF results. In general, the mass fraction of organics showed an obvious diurnal pattern during the whole campaign, with two peaks at about 14:00 and 19:00 LT (Fig. 4a), implying significant impacts of photochemical reactions and local emissions. Based on the PMF results (Fig. 5), the afternoon peak was attributed to secondary organic aerosol (SOA) formation (aBBOA and LOOA) during daytime, while the evening peak was explained by local residential activity (e.g., biomass burning and cooking, HOA and BBOA), as will be discussed later. A similar late-afternoon peak was observed in Hong Kong (Lee et al., 2013), where the OA enhancement was mainly contributed by traffic emissions. The f44 remained at a high level during daytime, consistent with strong photochemical reactions. A similar diurnal pattern was observed in the urban and suburban regions (Hong et al., 2018; Hu et al., 2016; Thalman et al., 2017), suggesting the consistent aging processes of pre-existing OA. In contrast, Deng et al. (2019) reported a relatively low OA oxidation state during daytime in a forest environment, which could be explained by the SOA formation through photochemical oxidation of BVOCs.
The calculated κHTDMA and κAMS (the blue and red lines in Fig. 4b, respectively) values at 200 nm based on Eqs. (4) and (7) both reached minimum during daytime, which was consistent with high OA fractions. This may be explained by lower hygroscopicity of OA than inorganics as found in previous studies (Pajunoja et al., 2015; Zhao et al., 2015; Kuang et al., 2020b) as well as the low κi values shown in Table 1. Although OA in a higher oxidation state could be hydrophilic (Massoli et al., 2010), the primary OA is usually considered to be hydrophobic substances, and their mixture would be less hygroscopic (usually with average κ = 0.1). The κAMS values were generally consistent with those of the κHTDMA during daytime while the overestimated κOA was observed during nighttime. This implies a lower κOA value than 0.1 at 200 nm during the nighttime, probably due to less oxidation processes at night than those under the sunlight.
The average diurnal profile of PNSD is shown in Fig. 4c. Besides a stable accumulation mode peaking at around 100 nm, a significantly growing mode of particle number from 20 to 80 nm was observed from 12:00 to 20:00 LT, which could be attributed to the frequently occurring NPF during the campaign (Fig. 1a).
The size-resolved diurnal variations in κOA were explored in Fig. 6. Note that the κOA values are presented in 2 h resolution due to the low data coverage (Figs. 1 and 2). In general, a significantly different pattern was observed between the Aitken mode and the accumulation mode. For Aitken mode particles (30–100 nm), the κOA values were higher (0.05–0.1) before dawn than those (0.02–0.07) during daytime, while this trend began to overturn at 150 and 200 nm, where the κOA values peaked at noon (∼ 0.09, Fig. 6). As reported in literature, the hygroscopicity of organics was partly dependent on the aging degree (Liu et al., 2021; Zhao et al., 2016; Kim et al., 2020). The diurnal characteristics of the size-resolved κOA indicate that the OA in small particles (30–100 nm) was fresh and became aged in large particles. For the same campaign, Kuang et al. (2021) reported the bulk κOA of PM1 based on aerosol optical hygroscopicity measurements, which could provide high-time-resolution data of κOA. The relationship between κOA and different OA factors was investigated, which showed a negative correlation (R = −0.25) between LOOA and κOA and a positive correlation (R = 0.35) between aBBOA and κOA. Thus, the decrease in κOA for Aitken mode particles during daytime might be attributed to the daytime formation of LOOA through gas–particle partitioning (Fig. 5). A similar phenomenon was reported by Deng et al. (2019) in a forest environment, which might be attributed to the photochemical reactions of BVOCs. Therefore, OA in small particles might be less aged and was primarily contributed by photochemical oxidation of VOCs. In contrast, it is likely that the accumulation mode particles became aged through photochemical oxidation during daylight, as evidenced by higher fractions of ELVOA at 200 nm and higher κOA (Figs. 6 and 7) during daytime. According to the PMF analysis, the daytime formation of aBBOA likely resulted from the aging processes of primary OA or biomass-burning-related precursors (Fig. 5). As suggested by Kuang et al. (2021), the daytime formation of aBBOA (Fig. 5) would lead to an increase in κOA, which likely explained the noontime κOA peak at 150 and 200 nm. It suggested that the OA in the accumulation mode was more influenced by the aging processes through photochemical reactions (leading to aBBOA formation).
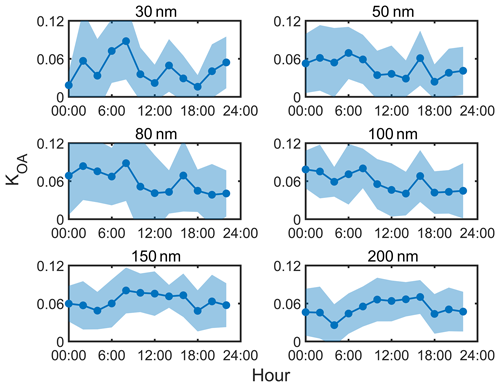
Figure 6The average (solid line) and standard deviation (shaded area) diurnal variation in κOA at different particle diameters.
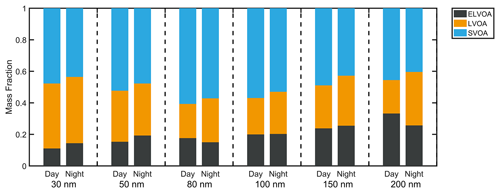
Figure 7The size-resolved volatility distribution during daytime (08:00–16:00 LT) and nighttime (20:00 to 04:00 LT) based on the median time of each cycle owing to the limited time resolution.
The average size-resolved volatility distribution of OA during daytime (08:00 to 16:00 LT) and nighttime (20:00 to 04:00 LT) was demonstrated in Fig. 7. A higher fraction of semi-volatile organic aerosol (SVOA) was observed at six measured sizes (30, 50, 80, 100, 150, and 200 nm) during daytime. SVOA was usually related to primary emission (e.g., traffic, biomass burning) and gas–particle partitioning (Donahue et al., 2012; Jathar et al., 2020; Hong et al., 2017; Saha et al., 2017). Two primary emission factors, BBOA and HOA, remained at a relative low level during daytime, suggesting that the higher fraction of SVOA during daylight might originate more from gas–particle partitioning. Note that gas–particle partitioning (leading to LOOA formation) could occur at all measured diameters, as shown by the higher daytime fractions of SVOA (Fig. 7). In summary, the above results indicate that the negative effect of LOOA on κOA might exist at all diameters, while the positive effect of aBBOA was more dominant at larger particle sizes.
Meanwhile, the decreasing trend of κOA was observed from 18:00 to 24:00 at 80 and 100 nm, which might be related to the high mass fraction of OA from primary emissions (HOA and BBOA, Fig. 5), owing to their hydrophobic nature. These two primary factors were associated with traffic emissions, cooking, and biomass burning. Zhang et al. (2005b) constructed the size distribution of HOA based on the size-resolved 44 and 57 from the AMS measurement and showed that HOA was dominant (∼ 75 %) in ultrafine particles (Dva < 100 nm). The size-resolved PMF results from Sun et al. (2012) also indicated a high mass fraction of HOA (0.3–0.4) in Aitken mode particles. The mass distribution of BC could be used to represent the distribution of primary OA (Cubison et al., 2008; Wang et al., 2010; Zhang et al., 2005a) due to similar source origins for BC and HOA/BBOA. The average mass fraction of BC peaked at about 80–100 nm (Fig. S7a), suggesting that HOA and BBOA might be dominant at this size range. The BC peaks at 80 and 100 nm were consistent with those of the SVOA mass fraction (Fig. 3), which was attributed to biomass burning as similar characteristics for the BC peak were shown in other studies (May et al., 2013; Huffman et al., 2009; Donahue et al., 2011). Furthermore, this conclusion was supported by the hygroscopicity measurements as a significant NH mode for 80–100 nm particles was found (Fig. 2). Overall, these results highlight that the diurnal variation in physicochemical properties of OA could vary in a large range with particle diameters, and further investigation is needed.
3.4 Implication for CCN activity
The CCN activity and its prediction are essential in global climate modeling and evaluation. A κOA value of 0.1–0.15 was widely adopted in the prediction of NCCN based on aerosol chemical composition (Meng et al., 2014; Wang et al., 2010; Almeida et al., 2014). As discussed in Sect. 3.3, the κOA values might be dependent on particle sizes and vary diurnally, which in turn affect NCCN. Here, different κOA values were adopted to predict NCCN, and the impact of κOA on NCCN was investigated through comparison between the predicted and measured NCCN. Note that we only discussed the NCCN at 0.1 %, 0.2 %, 0.4 %, and 0.7 % SS, since the D50 at higher SS (0.9 % and 1.0 %) was within a narrow range (35–60 nm).
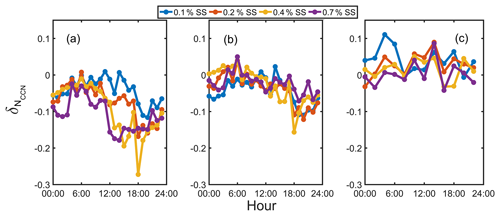
Figure 8The average diurnal variation in at 0.1 %, 0.2 %, 0.4 %, and 0.7 % SS based on fixed κOA (a), SR κOA (b), and SR diurnal κOA (c).
The NCCN at a certain SS can be calculated using PNSD and D50:
where ni is the particle distribution function at , and D50 is determined from the κAMS using Eqs. (5) and (7). The D50 at 0.1 %, 0.2 %, 0.4 %, and 0.7 % SS ranged from about 130–160, 90–110, 60–80, and 45–60 nm, respectively. Three κOA schemes were proposed to predict NCCN: (1) fixed κOA, where κOA was assumed to be 0.1 for all size particles; (2) size-resolved κOA (SR κOA), where κOA was taken from average size-resolved κOA (κOA at 50, 80, 100, and 150 nm for 0.7 %, 0.4 %, 0.2 %, and 0.1 % SS, respectively) in Sect. 3.2; and (3) size-resolved diurnal κOA (SR diurnal κOA), where κOA was the average diurnal value of κOA at each diameter (κOA at 50, 80, 100, and 150 nm for 0.7 %, 0.4 %, 0.2 %, and 0.1 % SS, respectively) as shown in Sect. 3.3. The κAMS was calculated based on the chemical composition at the corresponding D50 range. Note that the NCCN prediction based on the SR diurnal κOA scheme was presented in 2 h time resolution, and the particles were assumed to be internally mixed in Eq. (16). The internal mixing assumption could slightly increase the predicted NCCN by about 6 %–10 % (Sect. S3 in the Supplement). As aforementioned, organics can increase the CCN activity by decreasing surface tension, which might lead to significant discrepancy between κHTDMA and κCCN in this campaign (Fig. S8). In addition, this effect could result in a significant underestimation of NCCN (Ovadnevaite et al., 2017; Liu et al., 2018; Good et al., 2010; Noziere, 2016).
Here, we evaluate the surface tension effect by comparing κHTDMA and κCCN as a function of σs/a (Fig. S10 in the Supplement). The κCCN reached κHTDMA when the σs/a values were set to be about 0.059 N m−1 at 0.7 %, 0.9 %, and 1.0 % SS; 0.053 N m−1 at 0.4 % SS; 0.047 N m−1 at 0.2 % SS; and 0.049 N m−1 at 0.1 % SS. Thus, we adopted σs/a values of 0.049, 0.047, 0.053, and 0.059 N m−1 to predict NCCN at 0.1 %, 0.2 %, 0.4 %, and 0.7 % SS, respectively. In general, the NCCN prediction could be significantly improved by considering the surfactant effect (Fig. S12 in the Supplement). The NCCN was slightly overestimated by using reduced σs/a values, which was probably due to using a fixed κOA value. This bias could be corrected by adopting the SR κOA scheme (Fig. S13 in the Supplement).
The deviation of the NCCN prediction () at a certain SS is defined as (Cai et al., 2021b)
where NCCN,m(SS) is the measured NCCN at a specific SS. A negative indicates an overestimate of NCCN, and vice versa.
Figure 8 shows the at different SS values for the three κOA schemes. The fixed κOA scheme generally gave a negative value of (−0.18 to −0.02) at 0.7 % SS, indicating an NCCN overestimation, due to lower κOA values for smaller particles. A significant diurnal pattern of was observed at all SS values. The was relatively higher during daytime at 0.1 % SS, while an opposite pattern was shown at high SS, consistent with the size-dependent variation in κOA (Fig. 6). Hence, the fixed κOA scheme could lead to an obvious discrepancy in the NCCN prediction as SS increased. The results based on the SR κOA scheme showed that the minimum value at 0.7 % SS increased from −0.18 in the fixed κOA scheme to −0.08, indicating the improvement for the NCCN prediction at high SS (Fig. 8b). However, only minor improvement was observed at SS lower than 0.4 % because of the low employed κOA (about 0.08), which was close to the κOA value (0.1) adopted in the fixed κOA scheme. A significant difference of was still observed in the diurnal pattern at high and low SS, implying the impact of the diurnal variation in κOA on the NCCN prediction. To further investigate this impact, the SR diurnal κOA scheme was employed to calculate , and the results were shown in Fig. 8c. The value at 0.7 % SS varied from −0.04 to 0.09 with an average value of 0, whereas it ranged from 0 to 0.11 at 0.1 % SS. Hence, the discrepancies of among different SS values became minor compared to the other two schemes as a relatively flat diurnal pattern of was observed at all SS values. It implies that better prediction of NCCN could be achieved by considering the diurnal variation and the size dependence of κOA.
A rural field measurement was conducted at the Heshan supersite in the PRD region of China during October and November 2019. We investigated the diurnal variation and size dependence in the hygroscopicity and volatility of OA in combination with the PMF analysis of the AMS data. The impacts of OA on the CCN number concentration at different SS values were discussed for various given size-dependent κOA values.
In general, the average κOA values varied from 0.058 at 30 nm to 0.09 at 200 nm, indicating a higher oxidation degree of OA at larger sizes than at smaller sizes. This is consistent with particle volatility: the mass fraction of ELVOA increased (0.16–0.30) with increasing particle diameters.
Our results suggest that the formation and aging processes of OA might vary with particle sizes. An oppositely diurnal pattern of κOA was observed between Aitken mode (30–100 nm) and accumulation mode (150 and 200 nm) particles, suggesting different atmospheric evolution processes of OA at different diameters. The gas–particle partitioning could decrease the κOA, while the aging processes of preexisting particles could enhance the hygroscopicity of OA. For Aitken mode particles (30–100 nm), the κOA values reached a minimum (0.02–0.07) during daytime. Meanwhile, a daytime peak was observed for the κOA value (∼ 0.09) in the accumulation mode (150 and 200 nm), suggesting that the aging processes of preexisting particles were more dominant at accumulation mode particles. In addition, the mass faction of SVOA was higher during daytime at all measured diameters, implying that the formation of LOOA through gas–particle partitioning was independent of particle diameters.
The impact of the size-resolved diurnal variation in κOA on the NCCN was investigated. The use of fixed κOA (κOA = 0.1) overestimated the NCCN up to 18 % at 0.7 % SS. The diurnal deviation became obvious at 0.7 % SS and minor at 0.1 % SS during daytime, owing to the size-dependent variation in κOA. The NCCN prediction at 0.7 % SS was improved if the SR κOA scheme was used, while the diurnal variation in still existed. Better predictions can be obtained by using SR diurnal κOA. Our results highlight that the physical properties of OA can vary in a large range at different size ranges due to the formation and aging processes, and the size-resolved diurnal variation in κOA plays an important role in the NCCN prediction at different SS values. Further studies on the size-resolved physicochemical properties of OA should be performed in different environments to better understand their impact on cloud formation and hence climate.
Data from the measurements are available at https://doi.org/10.6084/m9.figshare.18094277.v1 (Cai et al., 2022).
The supplement related to this article is available online at: https://doi.org/10.5194/acp-22-8117-2022-supplement.
MC, SH, BY, and LL designed the research. MC, SH, MS, BY, YP, ZW, DC, WC, QSo, WL, BL, and QSu performed the measurements. MC, SH, BL, QSu, LL, BY, WH, WC, QSo, WL, YP, ZW, HT, HX, FL, XD, TD, JS, and JZ analyzed the data. MC, SH, and LL wrote the paper with contributions from all co-authors.
The contact author has declared that neither they nor their co-authors have any competing interests.
Publisher's note: Copernicus Publications remains neutral with regard to jurisdictional claims in published maps and institutional affiliations.
Additional support from the crew of the Heshan supersite and Guangdong Environmental Monitoring Center is greatly acknowledged.
This work was supported by the National Key R&D Plan of China (grant nos. 2019YFC0214605, 2019YFE0106300, and 2018YFC0213904), Key-Area Research and Development Program of Guangdong Province (grant no. 2019B110206001), National Natural Science Foundation of China (grant nos. 41877302, 91644225, 41775117, and 41807302), Guangdong Natural Science Funds for Distinguished Young Scholar (grant no. 2018B030306037), Guangdong Innovative and Entrepreneurial Research Team Program (grant no. 2016ZT06N263), Guangdong Province Key Laboratory for Climate Change and Natural Disaster Studies (grant no. 2020B1212060025), Guangdong Basic and Applied Basic Research Foundation (grant nos. 2019A1515110790 and 2019A1515110791), Science and Technology Research project of Guangdong Meteorological Bureau (grant no. GRMC2018M07), the Natural Science Foundation of Guangdong Province, China (grant no. 2016A030311007), Science and Technology Innovation Team Plan of Guangdong Meteorological Bureau (grant no. GRMCTD202003), and Science and Technology Program of Guangdong Province (Science and Technology Innovation Platform Category, grant no. 2019B121201002).
This paper was edited by Anne Perring and reviewed by two anonymous referees.
Almeida, G. P., Brito, J., Morales, C. A., Andrade, M. F., and Artaxo, P.: Measured and modelled cloud condensation nuclei (CCN) concentration in São Paulo, Brazil: the importance of aerosol size-resolved chemical composition on CCN concentration prediction, Atmos. Chem. Phys., 14, 7559–7572, https://doi.org/10.5194/acp-14-7559-2014, 2014.
Bertram, A. K., Martin, S. T., Hanna, S. J., Smith, M. L., Bodsworth, A., Chen, Q., Kuwata, M., Liu, A., You, Y., and Zorn, S. R.: Predicting the relative humidities of liquid-liquid phase separation, efflorescence, and deliquescence of mixed particles of ammonium sulfate, organic material, and water using the organic-to-sulfate mass ratio of the particle and the oxygen-to-carbon elemental ratio of the organic component, Atmos. Chem. Phys., 11, 10995–11006, https://doi.org/10.5194/acp-11-10995-2011, 2011.
Cai, J., Chu, B., Yao, L., Yan, C., Heikkinen, L. M., Zheng, F., Li, C., Fan, X., Zhang, S., Yang, D., Wang, Y., Kokkonen, T. V., Chan, T., Zhou, Y., Dada, L., Liu, Y., He, H., Paasonen, P., Kujansuu, J. T., Petäjä, T., Mohr, C., Kangasluoma, J., Bianchi, F., Sun, Y., Croteau, P. L., Worsnop, D. R., Kerminen, V.-M., Du, W., Kulmala, M., and Daellenbach, K. R.: Size-segregated particle number and mass concentrations from different emission sources in urban Beijing, Atmos. Chem. Phys., 20, 12721–12740, https://doi.org/10.5194/acp-20-12721-2020, 2020.
Cai, M., Tan, H., Chan, C. K., Mochida, M., Hatakeyama, S., Kondo, Y., Schurman, M. I., Xu, H., Li, F., and Shimada, K.: Comparison of Aerosol Hygroscopcity, Volatility, and Chemical Composition between a Suburban Site in the Pearl River Delta Region and a Marine Site in Okinawa, Aerosol Air Qual. Res., 17, 3194–3208, https://doi.org/10.4209/aaqr.2017.01.0020, 2017.
Cai, M., Tan, H., Chan, C. K., Qin, Y., Xu, H., Li, F., Schurman, M. I., Liu, L., and Zhao, J.: The size-resolved cloud condensation nuclei (CCN) activity and its prediction based on aerosol hygroscopicity and composition in the Pearl Delta River (PRD) region during wintertime 2014, Atmos. Chem. Phys., 18, 16419–16437, https://doi.org/10.5194/acp-18-16419-2018, 2018.
Cai, M., Liang, B., Sun, Q., Liu, L., Yuan, B., Shao, M., Huang, S., Peng, Y., Wang, Z., Tan, H., Li, F., Xu, H., Chen, D., and Zhao, J.: The important roles of surface tension and growth rate in the contribution of new particle formation (NPF) to cloud condensation nuclei (CCN) number concentration: evidence from field measurements in southern China, Atmos. Chem. Phys., 21, 8575–8592, https://doi.org/10.5194/acp-21-8575-2021, 2021a.
Cai, M. F., Liang, B. L., Sun, Q. B., Zhou, S. Z., Yuan, B., Shao, M., Tan, H. B., Xu, Y. S., Ren, L. H., and Zhao, J.: Contribution of New Particle Formation to Cloud Condensation Nuclei Activity and its Controlling Factors in a Mountain Region of Inland China, J. Geophys. Res. Atmos., 126, e2020JD034302, https://doi.org/10.1029/2020JD034302, 2021b.
Cai, M., Huang, S., Li, L., Yuan, B., Shao, M., and Zhao, J.: Distinct size dependence and diurnal variation of OA hygroscopicity, volatility, and CCN activity at a rural site in the Pearl River Delta (PRD) region, China, figshare [data set], https://doi.org/10.6084/m9.figshare.18094277.v1, 2022.
Canagaratna, M. R., Jayne, J. T., Jimenez, J. L., Allan, J. D., Alfarra, M. R., Zhang, Q., Onasch, T. B., Drewnick, F., Coe, H., Middlebrook, A., Delia, A., Williams, L. R., Trimborn, A. M., Northway, M. J., DeCarlo, P. F., Kolb, C. E., Davidovits, P., and Worsnop, D. R.: Chemical and microphysical characterization of ambient aerosols with the aerodyne aerosol mass spectrometer, Mass Spectrom. Rev., 26, 185–222, https://doi.org/10.1002/mas.20115, 2007.
Cappa, C. D. and Jimenez, J. L.: Quantitative estimates of the volatility of ambient organic aerosol, Atmos. Chem. Phys., 10, 5409–5424, https://doi.org/10.5194/acp-10-5409-2010, 2010.
Chang, R. Y.-W., Slowik, J. G., Shantz, N. C., Vlasenko, A., Liggio, J., Sjostedt, S. J., Leaitch, W. R., and Abbatt, J. P. D.: The hygroscopicity parameter (κ) of ambient organic aerosol at a field site subject to biogenic and anthropogenic influences: relationship to degree of aerosol oxidation, Atmos. Chem. Phys., 10, 5047–5064, https://doi.org/10.5194/acp-10-5047-2010, 2010.
Chen, C., Sun, Y. L., Xu, W. Q., Du, W., Zhou, L. B., Han, T. T., Wang, Q. Q., Fu, P. Q., Wang, Z. F., Gao, Z. Q., Zhang, Q., and Worsnop, D. R.: Characteristics and sources of submicron aerosols above the urban canopy (260 m) in Beijing, China, during the 2014 APEC summit, Atmos. Chem. Phys., 15, 12879–12895, https://doi.org/10.5194/acp-15-12879-2015, 2015.
Cheung, H. H. Y., Tan, H., Xu, H., Li, F., Wu, C., Yu, J. Z., and Chan, C. K.: Measurements of non-volatile aerosols with a VTDMA and their correlations with carbonaceous aerosols in Guangzhou, China, Atmos. Chem. Phys., 16, 8431–8446, https://doi.org/10.5194/acp-16-8431-2016, 2016.
Cubison, M. J., Ervens, B., Feingold, G., Docherty, K. S., Ulbrich, I. M., Shields, L., Prather, K., Hering, S., and Jimenez, J. L.: The influence of chemical composition and mixing state of Los Angeles urban aerosol on CCN number and cloud properties, Atmos. Chem. Phys., 8, 5649–5667, https://doi.org/10.5194/acp-8-5649-2008, 2008.
Deng, Y., Kagami, S., Ogawa, S., Kawana, K., Nakayama, T., Kubodera, R., Adachi, K., Hussein, T., Miyazaki, Y., and Mochida, M.: Hygroscopicity of Organic Aerosols and Their Contributions to CCN Concentrations Over a Midlatitude Forest in Japan, J. Geophys. Res.-Atmos., 123, 9703–9723, https://doi.org/10.1029/2017JD027292, 2018.
Deng, Y., Yai, H., Fujinari, H., Kawana, K., Nakayama, T., and Mochida, M.: Diurnal variation and size dependence of the hygroscopicity of organic aerosol at a forest site in Wakayama, Japan: their relationship to CCN concentrations, Atmos. Chem. Phys., 19, 5889–5903, https://doi.org/10.5194/acp-19-5889-2019, 2019.
Donahue, N. M., Epstein, S. A., Pandis, S. N., and Robinson, A. L.: A two-dimensional volatility basis set: 1. organic-aerosol mixing thermodynamics, Atmos. Chem. Phys., 11, 3303–3318, https://doi.org/10.5194/acp-11-3303-2011, 2011.
Donahue, N. M., Kroll, J. H., Pandis, S. N., and Robinson, A. L.: A two-dimensional volatility basis set – Part 2: Diagnostics of organic-aerosol evolution, Atmos. Chem. Phys., 12, 615–634, https://doi.org/10.5194/acp-12-615-2012, 2012.
Dusek, U., Frank, G. P., Massling, A., Zeromskiene, K., Iinuma, Y., Schmid, O., Helas, G., Hennig, T., Wiedensohler, A., and Andreae, M. O.: Water uptake by biomass burning aerosol at sub- and supersaturated conditions: closure studies and implications for the role of organics, Atmos. Chem. Phys., 11, 9519–9532, https://doi.org/10.5194/acp-11-9519-2011, 2011.
Epstein, S. A., Riipinen, I., and Donahue, N. M.: A Semiempirical Correlation between Enthalpy of Vaporization and Saturation Concentration for Organic Aerosol, Environ. Sci. Technol., 44, 743–748, https://doi.org/10.1021/es902497z, 2010.
Gantt, B. and Meskhidze, N.: The physical and chemical characteristics of marine primary organic aerosol: a review, Atmos. Chem. Phys., 13, 3979–3996, https://doi.org/10.5194/acp-13-3979-2013, 2013.
Good, N., Topping, D. O., Allan, J. D., Flynn, M., Fuentes, E., Irwin, M., Williams, P. I., Coe, H., and McFiggans, G.: Consistency between parameterisations of aerosol hygroscopicity and CCN activity during the RHaMBLe discovery cruise, Atmos. Chem. Phys., 10, 3189–3203, https://doi.org/10.5194/acp-10-3189-2010, 2010.
Guo, J., Zhou, S., Cai, M., Zhao, J., Song, W., Zhao, W., Hu, W., Sun, Y., He, Y., Yang, C., Xu, X., Zhang, Z., Cheng, P., Fan, Q., Hang, J., Fan, S., Wang, X., and Wang, X.: Characterization of submicron particles by time-of-flight aerosol chemical speciation monitor (ToF-ACSM) during wintertime: aerosol composition, sources, and chemical processes in Guangzhou, China, Atmos. Chem. Phys., 20, 7595–7615, https://doi.org/10.5194/acp-20-7595-2020, 2020.
Gysel, M., Crosier, J., Topping, D. O., Whitehead, J. D., Bower, K. N., Cubison, M. J., Williams, P. I., Flynn, M. J., McFiggans, G. B., and Coe, H.: Closure study between chemical composition and hygroscopic growth of aerosol particles during TORCH2, Atmos. Chem. Phys., 7, 6131–6144, https://doi.org/10.5194/acp-7-6131-2007, 2007.
Hallquist, M., Wenger, J. C., Baltensperger, U., Rudich, Y., Simpson, D., Claeys, M., Dommen, J., Donahue, N. M., George, C., Goldstein, A. H., Hamilton, J. F., Herrmann, H., Hoffmann, T., Iinuma, Y., Jang, M., Jenkin, M. E., Jimenez, J. L., Kiendler-Scharr, A., Maenhaut, W., McFiggans, G., Mentel, Th. F., Monod, A., Prévôt, A. S. H., Seinfeld, J. H., Surratt, J. D., Szmigielski, R., and Wildt, J.: The formation, properties and impact of secondary organic aerosol: current and emerging issues, Atmos. Chem. Phys., 9, 5155–5236, https://doi.org/10.5194/acp-9-5155-2009, 2009.
Hansen, A. M. K., Hong, J., Raatikainen, T., Kristensen, K., Ylisirniö, A., Virtanen, A., Petäjä, T., Glasius, M., and Prisle, N. L.: Hygroscopic properties and cloud condensation nuclei activation of limonene-derived organosulfates and their mixtures with ammonium sulfate, Atmos. Chem. Phys., 15, 14071–14089, https://doi.org/10.5194/acp-15-14071-2015, 2015.
Hong, J., Häkkinen, S. A. K., Paramonov, M., Äijälä, M., Hakala, J., Nieminen, T., Mikkilä, J., Prisle, N. L., Kulmala, M., Riipinen, I., Bilde, M., Kerminen, V.-M., and Petäjä, T.: Hygroscopicity, CCN and volatility properties of submicron atmospheric aerosol in a boreal forest environment during the summer of 2010, Atmos. Chem. Phys., 14, 4733–4748, https://doi.org/10.5194/acp-14-4733-2014, 2014.
Hong, J., Äijälä, M., Häme, S. A. K., Hao, L., Duplissy, J., Heikkinen, L. M., Nie, W., Mikkilä, J., Kulmala, M., Prisle, N. L., Virtanen, A., Ehn, M., Paasonen, P., Worsnop, D. R., Riipinen, I., Petäjä, T., and Kerminen, V.-M.: Estimates of the organic aerosol volatility in a boreal forest using two independent methods, Atmos. Chem. Phys., 17, 4387–4399, https://doi.org/10.5194/acp-17-4387-2017, 2017.
Hong, J., Xu, H., Tan, H., Yin, C., Hao, L., Li, F., Cai, M., Deng, X., Wang, N., Su, H., Cheng, Y., Wang, L., Petäjä, T., and Kerminen, V.-M.: Mixing state and particle hygroscopicity of organic-dominated aerosols over the Pearl River Delta region in China, Atmos. Chem. Phys., 18, 14079–14094, https://doi.org/10.5194/acp-18-14079-2018, 2018.
Hu, W., Hu, M., Hu, W., Jimenez, J. L., Yuan, B., Chen, W., Wang, M., Wu, Y., Chen, C., Wang, Z., Peng, J., Zeng, L., and Shao, M.: Chemical composition, sources, and aging process of submicron aerosols in Beijing: Contrast between summer and winter, J. Geophys. Res.-Atmos., 121, 1955–1977, https://doi.org/10.1002/2015JD024020, 2016.
Huang, R.-J., Zhang, Y., Bozzetti, C., Ho, K.-F., Cao, J.-J., Han, Y., Daellenbach, K. R., Slowik, J. G., Platt, S. M., and Canonaco, F.: High secondary aerosol contribution to particulate pollution during haze events in China, Nature, 514, 218–222, https://doi.org/10.1038/nature13774, 2014.
Huang, S., Wu, Z., Poulain, L., van Pinxteren, M., Merkel, M., Assmann, D., Herrmann, H., and Wiedensohler, A.: Source apportionment of the organic aerosol over the Atlantic Ocean from 53∘ N to 53∘ S: significant contributions from marine emissions and long-range transport, Atmos. Chem. Phys., 18, 18043–18062, https://doi.org/10.5194/acp-18-18043-2018, 2018.
Huffman, J. A., Docherty, K. S., Aiken, A. C., Cubison, M. J., Ulbrich, I. M., DeCarlo, P. F., Sueper, D., Jayne, J. T., Worsnop, D. R., Ziemann, P. J., and Jimenez, J. L.: Chemically-resolved aerosol volatility measurements from two megacity field studies, Atmos. Chem. Phys., 9, 7161–7182, https://doi.org/10.5194/acp-9-7161-2009, 2009.
Jathar, S. H., Sharma, N., Galang, A., Vanderheyden, C., Takhar, M., Chan, A. W. H., Pierce, J. R., and Volckens, J.: Measuring and modeling the primary organic aerosol volatility from a modern non-road diesel engine, Atmos. Environ., 223, 117221, https://doi.org/10.1016/j.atmosenv.2019.117221, 2020.
Jiang, R., Tan, H., Tang, L., Cai, M., Yin, Y., Li, F., Liu, L., Xu, H., Chan, P. W., and Deng, X.: Comparison of aerosol hygroscopicity and mixing state between winter and summer seasons in Pearl River Delta region, China, Atmos. Res., 169, 160–170, 2016.
Jimenez, J. L., Canagaratna, M., Donahue, N., Prevot, A., Zhang, Q., Kroll, J. H., DeCarlo, P. F., Allan, J. D., Coe, H., and Ng, N.: Evolution of organic aerosols in the atmosphere, Science, 326, 1525–1529, 2009.
Kanakidou, M., Seinfeld, J. H., Pandis, S. N., Barnes, I., Dentener, F. J., Facchini, M. C., Van Dingenen, R., Ervens, B., Nenes, A., Nielsen, C. J., Swietlicki, E., Putaud, J. P., Balkanski, Y., Fuzzi, S., Horth, J., Moortgat, G. K., Winterhalter, R., Myhre, C. E. L., Tsigaridis, K., Vignati, E., Stephanou, E. G., and Wilson, J.: Organic aerosol and global climate modelling: a review, Atmos. Chem. Phys., 5, 1053–1123, https://doi.org/10.5194/acp-5-1053-2005, 2005.
Kim, N., Yum, S. S., Park, M., Park, J. S., Shin, H. J., and Ahn, J. Y.: Hygroscopicity of urban aerosols and its link to size-resolved chemical composition during spring and summer in Seoul, Korea, Atmos. Chem. Phys., 20, 11245–11262, https://doi.org/10.5194/acp-20-11245-2020, 2020.
Kiyoura, R. and Urano, K.: Mechanism, Kinetics, and Equilibrium of Thermal Decomposition of Ammonium Sulfate, Ind. Eng. Chem. Proc. D. D., 9, 489–494, https://doi.org/10.1021/i260036a001, 1970.
Kuang, Y., He, Y., Xu, W., Yuan, B., Zhang, G., Ma, Z., Wu, C., Wang, C., Wang, S., Zhang, S., Tao, J., Ma, N., Su, H., Cheng, Y., Shao, M., and Sun, Y.: Photochemical Aqueous-Phase Reactions Induce Rapid Daytime Formation of Oxygenated Organic Aerosol on the North China Plain, Environ. Sci. Technol., 54, 3849–3860, https://doi.org/10.1021/acs.est.9b06836, 2020a.
Kuang, Y., Xu, W., Tao, J., Ma, N., Zhao, C., and Shao, M.: A Review on Laboratory Studies and Field Measurements of Atmospheric Organic Aerosol Hygroscopicity and Its Parameterization Based on Oxidation Levels, Curr. Pollut. Rep., 6, 410–424, https://doi.org/10.1007/s40726-020-00164-2, 2020b.
Kuang, Y., Huang, S., Xue, B., Luo, B., Song, Q., Chen, W., Hu, W., Li, W., Zhao, P., Cai, M., Peng, Y., Qi, J., Li, T., Wang, S., Chen, D., Yue, D., Yuan, B., and Shao, M.: Contrasting effects of secondary organic aerosol formations on organic aerosol hygroscopicity, Atmos. Chem. Phys., 21, 10375–10391, https://doi.org/10.5194/acp-21-10375-2021, 2021.
Lambe, A. T., Onasch, T. B., Massoli, P., Croasdale, D. R., Wright, J. P., Ahern, A. T., Williams, L. R., Worsnop, D. R., Brune, W. H., and Davidovits, P.: Laboratory studies of the chemical composition and cloud condensation nuclei (CCN) activity of secondary organic aerosol (SOA) and oxidized primary organic aerosol (OPOA), Atmos. Chem. Phys., 11, 8913–8928, https://doi.org/10.5194/acp-11-8913-2011, 2011.
Lee, B. H., Kostenidou, E., Hildebrandt, L., Riipinen, I., Engelhart, G. J., Mohr, C., DeCarlo, P. F., Mihalopoulos, N., Prevot, A. S. H., Baltensperger, U., and Pandis, S. N.: Measurement of the ambient organic aerosol volatility distribution: application during the Finokalia Aerosol Measurement Experiment (FAME-2008), Atmos. Chem. Phys., 10, 12149–12160, https://doi.org/10.5194/acp-10-12149-2010, 2010.
Lee, B.-H., Pierce, J. R., Engelhart, G. J., and Pandis, S. N.: Volatility of secondary organic aerosol from the ozonolysis of monoterpenes, Atmos. Environ., 45, 2443–2452, 2011.
Lee, B. P., Li, Y. J., Yu, J. Z., Louie, P. K., and Chan, C. K.: Physical and chemical characterization of ambient aerosol by HR-ToF-AMS at a suburban site in Hong Kong during springtime 2011, J. Geophys. Res.-Atmos., 118, 8625–8639, 2013.
Li, J., Wang, G., Zhou, B., Cheng, C., Cao, J., Shen, Z., and An, Z.: Airborne particulate organics at the summit (2060 m, a.s.l.) of Mt. Hua in central China during winter: Implications for biofuel and coal combustion, Atmos. Res., 106, 108–119, https://doi.org/10.1016/j.atmosres.2011.11.012, 2012.
Li, Y. J., Lee, B. Y. L., Yu, J. Z., Ng, N. L., and Chan, C. K.: Evaluating the degree of oxygenation of organic aerosol during foggy and hazy days in Hong Kong using high-resolution time-of-flight aerosol mass spectrometry (HR-ToF-AMS), Atmos. Chem. Phys., 13, 8739–8753, https://doi.org/10.5194/acp-13-8739-2013, 2013.
Liu, J., Zhang, F., Xu, W., Sun, Y., Chen, L., Li, S., Ren, J., Hu, B., Wu, H., and Zhang, R.: Hygroscopicity of Organic Aerosols Linked to Formation Mechanisms, Geophys. Res. Lett., 48, e2020GL091683, https://doi.org/10.1029/2020GL091683, 2021.
Liu, P., Song, M., Zhao, T., Gunthe, S. S., Ham, S., He, Y., Qin, Y. M., Gong, Z., Amorim, J. C., Bertram, A. K., and Martin, S. T.: Resolving the mechanisms of hygroscopic growth and cloud condensation nuclei activity for organic particulate matter, Nat. Commun., 9, 4076, https://doi.org/10.1038/s41467-018-06622-2, 2018.
Liu, P. F., Zhao, C. S., Göbel, T., Hallbauer, E., Nowak, A., Ran, L., Xu, W. Y., Deng, Z. Z., Ma, N., Mildenberger, K., Henning, S., Stratmann, F., and Wiedensohler, A.: Hygroscopic properties of aerosol particles at high relative humidity and their diurnal variations in the North China Plain, Atmos. Chem. Phys., 11, 3479–3494, https://doi.org/10.5194/acp-11-3479-2011, 2011.
Liu, X. and Wang, J.: How important is organic aerosol hygroscopicity to aerosol indirect forcing?, Environ. Res. Lett., 5, 044010, https://doi.org/10.1088/1748-9326/5/4/044010, 2010.
Louvaris, E. E., Florou, K., Karnezi, E., Papanastasiou, D. K., Gkatzelis, G. I., and Pandis, S. N.: Volatility of source apportioned wintertime organic aerosol in the city of Athens, Atmos. Environ., 158, 138–147, https://doi.org/10.1016/j.atmosenv.2017.03.042, 2017.
Noziere, B.: Don't forget the surface, Science, 351, 1396–1397, https://doi.org/10.1126/science.aaf3253, 2016.
Ma, N., Zhao, C., Tao, J., Wu, Z., Kecorius, S., Wang, Z., Größ, J., Liu, H., Bian, Y., Kuang, Y., Teich, M., Spindler, G., Müller, K., van Pinxteren, D., Herrmann, H., Hu, M., and Wiedensohler, A.: Variation of CCN activity during new particle formation events in the North China Plain, Atmos. Chem. Phys., 16, 8593–8607, https://doi.org/10.5194/acp-16-8593-2016, 2016.
Massling, A., Stock, M., and Wiedensohler, A.: Diurnal, weekly, and seasonal variation of hygroscopic properties of submicrometer urban aerosol particles, Atmos. Environ., 39, 3911–3922, https://doi.org/10.1016/j.atmosenv.2005.03.020, 2005.
Massoli, P., Lambe, A., Ahern, A., Williams, L., Ehn, M., Mikkilä, J., Canagaratna, M., Brune, W., Onasch, T., and Jayne, J.: Relationship between aerosol oxidation level and hygroscopic properties of laboratory generated secondary organic aerosol (SOA) particles, Geophys. Res. Lett., 37, L24801, https://doi.org/10.1029/2010GL045258, 2010.
May, A. A., Levin, E. J. T., Hennigan, C. J., Riipinen, I., Lee, T., Collett Jr., J. L., Jimenez, J. L., Kreidenweis, S. M., and Robinson, A. L.: Gas-particle partitioning of primary organic aerosol emissions: 3. Biomass burning, J. Geophys. Res.-Atmos., 118, 11327–11338, https://doi.org/10.1002/jgrd.50828, 2013.
Mei, F., Setyan, A., Zhang, Q., and Wang, J.: CCN activity of organic aerosols observed downwind of urban emissions during CARES, Atmos. Chem. Phys., 13, 12155–12169, https://doi.org/10.5194/acp-13-12155-2013, 2013.
Meng, J. W., Yeung, M. C., Li, Y. J., Lee, B. Y. L., and Chan, C. K.: Size-resolved cloud condensation nuclei (CCN) activity and closure analysis at the HKUST Supersite in Hong Kong, Atmos. Chem. Phys., 14, 10267–10282, https://doi.org/10.5194/acp-14-10267-2014, 2014.
Mochida, M., Kuwata, M., Miyakawa, T., Takegawa, N., Kawamura, K., and Kondo, Y.: Relationship between hygroscopicity and cloud condensation nuclei activity for urban aerosols in Tokyo, J. Geophys. Res., 111, D23204, https://doi.org/10.1029/2005jd006980, 2006.
Onasch, T. B., Trimborn, A., Fortner, E. C., Jayne, J. T., Kok, G. L., Williams, L. R., Davidovits, P., and Worsnop, D. R.: Soot Particle Aerosol Mass Spectrometer: Development, Validation, and Initial Application, Aerosol Sci. Tech., 46, 804–817, https://doi.org/10.1080/02786826.2012.663948, 2012.
Ovadnevaite, J., Zuend, A., Laaksonen, A., Sanchez, K. J., Roberts, G., Ceburnis, D., Decesari, S., Rinaldi, M., Hodas, N., Facchini, M. C., Seinfeld, J. H., and O' Dowd, C.: Surface tension prevails over solute effect in organic-influenced cloud droplet activation, Nature, 546, 637–641, https://doi.org/10.1038/nature22806, 2017.
Paatero, P.: Least squares formulation of robust non-negative factor analysis, Chemometr. Intell. Lab., 37, 23–35, https://doi.org/10.1016/S0169-7439(96)00044-5, 1997.
Paatero, P. and Tapper, U.: Positive matrix factorization: A non-negative factor model with optimal utilization of error estimates of data values, Environmetrics, 5, 111–126, https://doi.org/10.1002/env.3170050203, 1994.
Pajunoja, A., Lambe, A. T., Hakala, J., Rastak, N., Cummings, M. J., Brogan, J. F., Hao, L., Paramonov, M., Hong, J., and Prisle, N. L.: Adsorptive uptake of water by semisolid secondary organic aerosols, Geophys. Res. Lett., 42, 3063–3068, 2015.
Park, S. H., Rogak, S. N., and Grieshop, A. P.: A Two-Dimensional Laminar Flow Model for Thermodenuders Applied to Vapor Pressure Measurements, Aerosol Sci. Technol., 47, 283–293, https://doi.org/10.1080/02786826.2012.750711, 2013.
Petters, M. D. and Kreidenweis, S. M.: A single parameter representation of hygroscopic growth and cloud condensation nucleus activity, Atmos. Chem. Phys., 7, 1961–1971, https://doi.org/10.5194/acp-7-1961-2007, 2007.
Petters, M. D. and Kreidenweis, S. M.: A single parameter representation of hygroscopic growth and cloud condensation nucleus activity – Part 3: Including surfactant partitioning, Atmos. Chem. Phys., 13, 1081–1091, https://doi.org/10.5194/acp-13-1081-2013, 2013.
Petters, M. D., Wex, H., Carrico, C. M., Hallbauer, E., Massling, A., McMeeking, G. R., Poulain, L., Wu, Z., Kreidenweis, S. M., and Stratmann, F.: Towards closing the gap between hygroscopic growth and activation for secondary organic aerosol – Part 2: Theoretical approaches, Atmos. Chem. Phys., 9, 3999–4009, https://doi.org/10.5194/acp-9-3999-2009, 2009.
Philippin, S., Wiedensohler, A., and Stratmann, F.: Measurements of non-volatile fractions of pollution aerosols with an eight-tube volatility tandem differential mobility analyzer (VTDMA-8), J. Aerosol Sci., 35, 185–203, https://doi.org/10.1016/j.jaerosci.2003.07.004, 2004.
Qin, Y. M., Tan, H. B., Li, Y. J., Schurman, M. I., Li, F., Canonaco, F., Prévôt, A. S. H., and Chan, C. K.: Impacts of traffic emissions on atmospheric particulate nitrate and organics at a downwind site on the periphery of Guangzhou, China, Atmos. Chem. Phys., 17, 10245–10258, https://doi.org/10.5194/acp-17-10245-2017, 2017.
Rastak, N., Pajunoja, A., Acosta Navarro, J. C., Ma, J., Song, M., Partridge, D. G., Kirkevåg, A., Leong, Y., Hu, W. W., Taylor, N. F., Lambe, A., Cerully, K., Bougiatioti, A., Liu, P., Krejci, R., Petäjä, T., Percival, C., Davidovits, P., Worsnop, D. R., Ekman, A. M. L., Nenes, A., Martin, S., Jimenez, J. L., Collins, D. R., Topping, D. O., Bertram, A. K., Zuend, A., Virtanen, A., and Riipinen, I.: Microphysical explanation of the RH-dependent water affinity of biogenic organic aerosol and its importance for climate, Geophys. Res. Lett., 44, 5167–5177, https://doi.org/10.1002/2017GL073056, 2017.
Renbaum-Wolff, L., Song, M., Marcolli, C., Zhang, Y., Liu, P. F., Grayson, J. W., Geiger, F. M., Martin, S. T., and Bertram, A. K.: Observations and implications of liquid–liquid phase separation at high relative humidities in secondary organic material produced by α-pinene ozonolysis without inorganic salts, Atmos. Chem. Phys., 16, 7969–7979, https://doi.org/10.5194/acp-16-7969-2016, 2016.
Riipinen, I., Pierce, J. R., Donahue, N. M., and Pandis, S. N.: Equilibration time scales of organic aerosol inside thermodenuders: Evaporation kinetics versus thermodynamics, Atmos. Environ., 44, 597–607, https://doi.org/10.1016/j.atmosenv.2009.11.022, 2010.
Rose, D., Gunthe, S. S., Mikhailov, E., Frank, G. P., Dusek, U., Andreae, M. O., and Pöschl, U.: Calibration and measurement uncertainties of a continuous-flow cloud condensation nuclei counter (DMT-CCNC): CCN activation of ammonium sulfate and sodium chloride aerosol particles in theory and experiment, Atmos. Chem. Phys., 8, 1153–1179, https://doi.org/10.5194/acp-8-1153-2008, 2008.
Rose, D., Nowak, A., Achtert, P., Wiedensohler, A., Hu, M., Shao, M., Zhang, Y., Andreae, M. O., and Pöschl, U.: Cloud condensation nuclei in polluted air and biomass burning smoke near the mega-city Guangzhou, China – Part 1: Size-resolved measurements and implications for the modeling of aerosol particle hygroscopicity and CCN activity, Atmos. Chem. Phys., 10, 3365–3383, https://doi.org/10.5194/acp-10-3365-2010, 2010.
Ruehl, C. R. and Wilson, K. R.: Surface organic monolayers control the hygroscopic growth of submicrometer particles at high relative humidity, J. Phys. Chem. A, 118, 3952–3966, 2014.
Saha, P. K., Khlystov, A., and Grieshop, A. P.: Determining aerosol volatility parameters using a “Dual Thermodenuder” system: application to laboratory-generated organic aerosols, Aerosol Sci. Tech., 49, 620–632, 2015.
Saha, P. K., Khlystov, A., Yahya, K., Zhang, Y., Xu, L., Ng, N. L., and Grieshop, A. P.: Quantifying the volatility of organic aerosol in the southeastern US, Atmos. Chem. Phys., 17, 501–520, https://doi.org/10.5194/acp-17-501-2017, 2017.
Saleh, R., Walker, J., and Khlystov, A.: Determination of saturation pressure and enthalpy of vaporization of semi-volatile aerosols: The integrated volume method, J. Aerosol Sci., 39, 876–887, https://doi.org/10.1016/j.jaerosci.2008.06.004, 2008.
Schill, G. P. and Tolbert, M. A.: Heterogeneous ice nucleation on phase-separated organic-sulfate particles: effect of liquid vs. glassy coatings, Atmos. Chem. Phys., 13, 4681–4695, https://doi.org/10.5194/acp-13-4681-2013, 2013.
Seinfeld, J. H. and Pandis, S. N.: Atmospheric chemistry and physics: from air pollution to climate change, John Wiley & Sons, ISBN 978-1-118-94740-1, 2016.
Shrivastava, M., Cappa, C. D., Fan, J., Goldstein, A. H., Guenther, A. B., Jimenez, J. L., Kuang, C., Laskin, A., Martin, S. T., Ng, N. L., Petaja, T., Pierce, J. R., Rasch, P. J., Roldin, P., Seinfeld, J. H., Shilling, J., Smith, J. N., Thornton, J. A., Volkamer, R., Wang, J., Worsnop, D. R., Zaveri, R. A., Zelenyuk, A., and Zhang, Q.: Recent advances in understanding secondary organic aerosol: Implications for global climate forcing, Rev. Geophys., 55, 509–559, https://doi.org/10.1002/2016RG000540, 2017.
Song, M., Marcolli, C., Krieger, U. K., Zuend, A., and Peter, T.: Liquid-liquid phase separation and morphology of internally mixed dicarboxylic acids/ammonium sulfate/water particles, Atmos. Chem. Phys., 12, 2691–2712, https://doi.org/10.5194/acp-12-2691-2012, 2012a.
Song, M., Marcolli, C., Krieger, U. K., Zuend, A., and Peter, T.: Liquid-liquid phase separation in aerosol particles: Dependence on O:C, organic functionalities, and compositional complexity, Geophys. Res. Lett., 39, L19801, https://doi.org/10.1029/2012GL052807, 2012b.
Stokes, R. and Robinson, R.: Interactions in aqueous nonelectrolyte solutions. I. Solute-solvent equilibria, J. Phys. Chem., 70, 2126–2131, 1966.
Stolzenburg, M. R. and McMurry, P. H.: Equations Governing Single and Tandem DMA Configurations and a New Lognormal Approximation to the Transfer Function, Aerosol Sci. Tech., 42, 421–432, https://doi.org/10.1080/02786820802157823, 2008.
Sun, Y. L., Zhang, Q., Schwab, J. J., Yang, T., Ng, N. L., and Demerjian, K. L.: Factor analysis of combined organic and inorganic aerosol mass spectra from high resolution aerosol mass spectrometer measurements, Atmos. Chem. Phys., 12, 8537–8551, https://doi.org/10.5194/acp-12-8537-2012, 2012.
Tan, H., Xu, H., Wan, Q., Li, F., Deng, X., Chan, P. W., Xia, D., and Yin, Y.: Design and Application of an Unattended Multifunctional H-TDMA System, J. Atmos. Oceanic Tech., 30, 1136–1148, https://doi.org/10.1175/JTECH-D-12-00129.1, 2013a.
Tan, H., Yin, Y., Gu, X., Li, F., Chan, P. W., Xu, H., Deng, X., and Wan, Q.: An observational study of the hygroscopic properties of aerosols over the Pearl River Delta region, Atmos. Environ., 77, 817–826, https://doi.org/10.1016/j.atmosenv.2013.05.049, 2013b.
Thalman, R., de Sá, S. S., Palm, B. B., Barbosa, H. M. J., Pöhlker, M. L., Alexander, M. L., Brito, J., Carbone, S., Castillo, P., Day, D. A., Kuang, C., Manzi, A., Ng, N. L., Sedlacek III, A. J., Souza, R., Springston, S., Watson, T., Pöhlker, C., Pöschl, U., Andreae, M. O., Artaxo, P., Jimenez, J. L., Martin, S. T., and Wang, J.: CCN activity and organic hygroscopicity of aerosols downwind of an urban region in central Amazonia: seasonal and diel variations and impact of anthropogenic emissions, Atmos. Chem. Phys., 17, 11779–11801, https://doi.org/10.5194/acp-17-11779-2017, 2017.
Ulbrich, I. M., Canagaratna, M. R., Zhang, Q., Worsnop, D. R., and Jimenez, J. L.: Interpretation of organic components from Positive Matrix Factorization of aerosol mass spectrometric data, Atmos. Chem. Phys., 9, 2891–2918, https://doi.org/10.5194/acp-9-2891-2009, 2009.
Volkamer, R., Jimenez, J. L., Martini, F. S., Dzepina, K., Qi, Z., Salcedo, D., Molina, L. T., Worsnop, D. R., and Molina, M. J.: Secondary organic aerosol formation from anthropogenic air pollution: Rapid and higher than expected, Geophys. Res. Lett., 33, 254–269, 2006.
Wang, D. S. and Hildebrandt Ruiz, L.: Chlorine-initiated oxidation of n-alkanes under high-NOx conditions: insights into secondary organic aerosol composition and volatility using a FIGAERO–CIMS, Atmos. Chem. Phys., 18, 15535–15553, https://doi.org/10.5194/acp-18-15535-2018, 2018.
Wang, J., Lee, Y.-N., Daum, P. H., Jayne, J., and Alexander, M. L.: Effects of aerosol organics on cloud condensation nucleus (CCN) concentration and first indirect aerosol effect, Atmos. Chem. Phys., 8, 6325–6339, https://doi.org/10.5194/acp-8-6325-2008, 2008.
Wang, J., Cubison, M. J., Aiken, A. C., Jimenez, J. L., and Collins, D. R.: The importance of aerosol mixing state and size-resolved composition on CCN concentration and the variation of the importance with atmospheric aging of aerosols, Atmos. Chem. Phys., 10, 7267–7283, https://doi.org/10.5194/acp-10-7267-2010, 2010.
Wex, H., Petters, M. D., Carrico, C. M., Hallbauer, E., Massling, A., McMeeking, G. R., Poulain, L., Wu, Z., Kreidenweis, S. M., and Stratmann, F.: Towards closing the gap between hygroscopic growth and activation for secondary organic aerosol: Part 1 – Evidence from measurements, Atmos. Chem. Phys., 9, 3987–3997, https://doi.org/10.5194/acp-9-3987-2009, 2009.
Wu, Z. J., Poulain, L., Henning, S., Dieckmann, K., Birmili, W., Merkel, M., van Pinxteren, D., Spindler, G., Müller, K., Stratmann, F., Herrmann, H., and Wiedensohler, A.: Relating particle hygroscopicity and CCN activity to chemical composition during the HCCT-2010 field campaign, Atmos. Chem. Phys., 13, 7983–7996, https://doi.org/10.5194/acp-13-7983-2013, 2013.
Xu, W., Xie, C., Karnezi, E., Zhang, Q., Wang, J., Pandis, S. N., Ge, X., Zhang, J., An, J., Wang, Q., Zhao, J., Du, W., Qiu, Y., Zhou, W., He, Y., Li, Y., Li, J., Fu, P., Wang, Z., Worsnop, D. R., and Sun, Y.: Summertime aerosol volatility measurements in Beijing, China, Atmos. Chem. Phys., 19, 10205–10216, https://doi.org/10.5194/acp-19-10205-2019, 2019.
Xu, W., Chen, C., Qiu, Y., Xie, C., Chen, Y., Ma, N., Xu, W., Fu, P., Wang, Z., Pan, X., Zhu, J., Ng, N. L., and Sun, Y.: Size-resolved characterization of organic aerosol in the North China Plain: new insights from high resolution spectral analysis, Environ. Sci. Atmos., 1, 346–358, https://doi.org/10.1039/D1EA00025J, 2021.
Yuan, L., Zhang, X., Feng, M., Liu, X., Che, Y., Xu, H., Schaefer, K., Wang, S., and Zhou, Y.: Size-resolved hygroscopic behaviour and mixing state of submicron aerosols in a megacity of the Sichuan Basin during pollution and fireworks episodes, Atmos. Environ., 226, 117393, https://doi.org/10.1016/j.atmosenv.2020.117393, 2020.
Zdanovskii, A.: Novyi Metod Rascheta Rastvorimostei Elektrolitov V Mnogokomponentnykh Sistemakh. 1, Zhurnal Fizicheskoi Khimii, 22, 1478–1485, 1948.
Zhang, Q., Canagaratna, M. R., Jayne, J. T., Worsnop, D. R., and Jimenez, J. L.: Time-and size-resolved chemical composition of submicron particles in Pittsburgh: Implications for aerosol sources and processes, J. Geophys. Res.-Atmos., 110, 1984–2012, 2005a.
Zhang, Q., Worsnop, D. R., Canagaratna, M. R., and Jimenez, J. L.: Hydrocarbon-like and oxygenated organic aerosols in Pittsburgh: insights into sources and processes of organic aerosols, Atmos. Chem. Phys., 5, 3289–3311, https://doi.org/10.5194/acp-5-3289-2005, 2005b.
Zhang, Y., Tao, J., Ma, N., Kuang, Y., Wang, Z., Cheng, P., Xu, W., Yang, W., Zhang, S., Xiong, C., Dong, W., Xie, L., Sun, Y., Fu, P., Zhou, G., Cheng, Y., and Su, H.: Predicting cloud condensation nuclei number concentration based on conventional measurements of aerosol properties in the North China Plain, Sci. Tot. Environ., 719, 137473, https://doi.org/10.1016/j.scitotenv.2020.137473, 2020.
Zhao, D. F., Buchholz, A., Kortner, B., Schlag, P., Rubach, F., Kiendler-Scharr, A., Tillmann, R., Wahner, A., Flores, J. M., Rudich, Y., Watne, Å. K., Hallquist, M., Wildt, J., and Mentel, T. F.: Size-dependent hygroscopicity parameter (κ) and chemical composition of secondary organic cloud condensation nuclei, Geophys. Res. Lett., 42, 10920–10928, https://doi.org/10.1002/2015GL066497, 2015.
Zhao, D. F., Buchholz, A., Kortner, B., Schlag, P., Rubach, F., Fuchs, H., Kiendler-Scharr, A., Tillmann, R., Wahner, A., Watne, Å. K., Hallquist, M., Flores, J. M., Rudich, Y., Kristensen, K., Hansen, A. M. K., Glasius, M., Kourtchev, I., Kalberer, M., and Mentel, Th. F.: Cloud condensation nuclei activity, droplet growth kinetics, and hygroscopicity of biogenic and anthropogenic secondary organic aerosol (SOA), Atmos. Chem. Phys., 16, 1105–1121, https://doi.org/10.5194/acp-16-1105-2016, 2016.