the Creative Commons Attribution 4.0 License.
the Creative Commons Attribution 4.0 License.
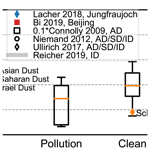
Ice-nucleating particles from multiple aerosol sources in the urban environment of Beijing under mixed-phase cloud conditions
Cuiqi Zhang
Zhijun Wu
Jingchuan Chen
Jie Chen
Lizi Tang
Wenfei Zhu
Xiangyu Pei
Shiyi Chen
Ping Tian
Limin Zeng
Zamin A. Kanji
Ice crystals occurring in mixed-phase clouds play a vital role in global precipitation and energy balance because of the unstable equilibrium between coexistent liquid droplets and ice crystals, which affects cloud lifetime and radiative properties, as well as precipitation formation. Satellite observations proved that immersion freezing, i.e., ice formation on particles immersed within aqueous droplets, is the dominant ice nucleation (IN) pathway in mixed-phase clouds. However, the impact of anthropogenic emissions on atmospheric IN in the urban environment remains ambiguous. In this study, we present in situ observations of ambient ice-nucleating particle number concentration (NINP) measured at mixed-phase cloud conditions (−30 ∘C, relative humidity with respect to liquid water RHw= 104 %) and the physicochemical properties of ambient aerosol, including chemical composition and size distribution, at an urban site in Beijing during the traditional Chinese Spring Festival. The impact of multiple aerosol sources such as firework emissions, local traffic emissions, mineral dust, and urban secondary aerosols on NINP is investigated. The results show that NINP during the dust event reaches up to 160 # L−1 (where “#” represents number of particles), with an activation fraction (AF) of 0.0036 % ± 0.0011 %. During the rest of the observation, NINP is on the order of 10−1 to 10 # L−1, with an average AF between 0.0001 % and 0.0002 %. No obvious dependence of NINP on the number concentration of particles larger than 500 nm (N500) or black carbon (BC) mass concentration (mBC) is found throughout the field observation. The results indicate a substantial NINP increase during the dust event, although the observation took place at an urban site with high background aerosol concentration. Meanwhile, the presence of atmospheric BC from firework and traffic emissions, along with urban aerosols formed via secondary transformation during heavily polluted periods, does not influence the observed INP concentration. Our study corroborates previous laboratory and field findings that anthropogenic BC emission has a negligible effect on NINP and that NINP is unaffected by heavy pollution in the urban environment under mixed-phase cloud conditions.
- Article
(8202 KB) - Full-text XML
- BibTeX
- EndNote
Ice crystals in clouds can form via homogeneous freezing of aqueous droplets below −38 ∘C or via heterogeneous ice nucleation (IN) with the aid of foreign interfaces offered by atmospheric ice-nucleating particles (INPs) through immersion/contact freezing of existing droplets at higher temperature or direct deposition/condensation of water vapor below water saturation (Pruppacher and Klett, 1997; Vali et al., 2015; Kanji et al., 2017). Mixed-phase clouds occur where super-cooled liquid water droplets coexist with ice crystals and are normally sustained between −38 and 0 ∘C in the atmosphere, with ice melting rapidly at warmer temperature and droplets freezing homogeneously at colder temperature (Boucher et al., 2013; Korolev et al., 2017). The Wegener–Bergeron–Findeisen process in mixed-phase clouds favors ice crystal growth at the cost of liquid droplet evaporation (Wegener, 1911; Bergeron, 1935; Findeisen, 1938), leading to ice water content and ice crystal size change, which further result in changes of mixed-phase cloud lifetime and radiative properties, as well as global precipitation patterns (Cantrell and Heymsfield, 2005; Field and Heymsfield, 2015; Mülmenstädt et al., 2015; Korolev et al., 2017; Heymsfield et al., 2020). Satellite observations demonstrate that the predominant ice formation pathway in mixed-phase clouds is immersion freezing (e.g., Ansmann et al., 2008; de Boer et al., 2011; Silber et al., 2021). In this mode, INPs immersed within supercooled aqueous droplets provide an interface that decreases the liquid–solid phase transition energy barrier and aids droplet freezing (Pruppacher and Klett, 1997; Vali et al., 2015; Kanji et al., 2017).
Most of the particles in highly populated urban areas originate from local emissions, including ground transportation, cooking, coal, and biomass burning, leading to significant production of carbonaceous particles, including organic compounds and elemental carbon, as well as inorganic salts (e.g., Sun et al., 2016). Apart from local emissions, regional transportation, such as transportation of mineral dusts and pollutants from adjacent areas, also contributes significantly to urban particle population under appropriate meteorological conditions, during which aging can significantly modify particle physicochemical properties, such as chemical composition, morphology, and mixing state (e.g., Lin et al., 2016; Sun et al., 2016; Hua et al., 2018; X. Zhang et al., 2020; Lei et al., 2021; Li et al., 2021). Previous studies have confirmed that several kinds of atmospheric particles, including mineral dusts, carbonaceous particles, and biological species, can act as immersion INPs (Hoose and Möhler, 2012; Murray et al., 2012; Kanji et al., 2017, and references therein). When present at atmospherically relevant amounts in droplets, mineral dusts mostly catalyze supercooled aqueous droplet freezing below −15 ∘C (Hoose and Möhler, 2012, and references therein; Murray et al., 2012; Kanji et al., 2017, and references therein), while biological species, such as pollen, fungal spores, and viruses, generally exhibit immersion IN activity below −5 ∘C and are fully activated below −10 to −20 ∘C (e.g., Chou, 2011; Conen et al., 2015; Polen et al., 2016; Kanji et al., 2017; Conen et al., 2022; Porter et al., 2022). The reported atmospheric immersion INP number concentration (NINP) values were measured between −5 and −38 ∘C and were normally on the order of 10−2 to 103 # L−1 (e.g., Rogers et al., 1998; DeMott et al., 2010; Chen et al., 2018; Porter et al., 2022).
Among all types of airborne particles, mineral dusts are commonly acknowledged as a major source of effective atmospheric immersion INPs (e.g., DeMott et al., 2003; Archuleta et al., 2005; Kanji and Abbatt, 2006; Welti et al., 2009; DeMott et al., 2010; Atkinson et al., 2013; Cziczo et al., 2013; DeMott et al., 2015; Chen et al., 2021). It was also reported that crystalline ammonium sulfate could nucleate ice heterogeneously below water saturation (Abbatt et al., 2006). But the effectiveness of carbonaceous particles and inorganic salts acting as INPs under mixed-phase cloud conditions remains elusive (Schill et al., 2016; Chen et al., 2018; Kanji et al., 2020; Schill et al., 2020; Wolf et al., 2020). Although certain types of black carbon (BC) and organic particles exhibited INP activity at temperatures below −38 ∘C through deposition IN (Murray et al., 2010; Mahrt et al., 2018; Nichman et al., 2019; C. Zhang et al., 2020), field observations (Chen et al., 2018; Adams et al., 2020) and laboratory experiments (Schill et al., 2016; Kanji et al., 2020; Schill et al., 2020) suggest that carbonaceous particles might not affect ice crystal formation via immersion mode. Besides, organic coatings are likely to impede carbonaceous particles from acting as effective deposition INPs at temperatures below −38 ∘C (Nichman et al., 2019; C. Zhang et al., 2020).
Previous modeling work confirmed that anthropogenic INP emission could alter the size of ice crystals in clouds and change cloud lifetime and global precipitation pattern (Zhao et al., 2019). Yet, there is limited published direct evidence on the contribution of anthropogenic particles to ice crystal formation in highly populated areas (Knopf et al., 2010; Corbin et al., 2012; Chen et al., 2018; Che et al., 2019, 2021). Knopf et al. (2010) used filter samples collected from a highly populated urban area in Mexico City and an optical IN microscopy technique to report that anthropogenic particles dominated by organic components might catalyze ice formation well below water saturation at temperature below −38 ∘C. Such organic-rich anthropogenic particles also demonstrated ice formation potential via immersion pathway above −38 ∘C in their study. Corbin et al. (2012) suggested that coupling atmospheric dust, elemental carbon, and biomass burning particle concentration together could provide the best estimation for atmospheric INP concentration in downtown Toronto at −34 ∘C just below water saturation, but the share of each particle category remained unclear due to limited data. Chen et al. (2018) quantified offline immersion INP concentration between −6 and −25 ∘C using filter samples collected every 12 h during a heavily polluted 2016 wintertime in Beijing. Even though a high level PM2.5 with complex chemical composition was sampled during the pollution period in the urban area, these aerosols did not act as superior INPs, and the highest INP concentration measured at −25 ∘C was below 10 # L−1, similar to what was observed in remote regions such as the Swiss Alps (Boose et al., 2016a; Lacher et al., 2017). The INP concentration reported by Chen et al. (2018) was insensitive to particle number concentration and particle chemistry in an atmosphere that was dominated by anthropogenic emissions. The absence of a correlation between immersion INP concentration and particle number during a pollution period was further supported by Bi et al. (2019) in an online immersion INP concentration field observation at a suburban site in Beijing during May to June 2018, using a continuous-flow diffusion chamber (CFDC) operated above water saturation between −20 to −30 ∘C. The lack of online particle chemistry information impedes aerosol source correlation in these studies (Chen et al., 2018; Bi et al., 2019). Che et al. (2019) reported a positive correlation between the total atmospheric INP concentration and air pollution degree during springtime in Beijing. INP concentration was measured by a Bigg's mixing cloud chamber for 1 month in 2017, and the total atmospheric INP concentration could reach 1500 # L−1 at −30 ∘C (Che et al., 2019, 2021).
Currently, a knowledge gap still exists on the magnitude and dominant source of ambient INPs in highly populated urban areas, as well as the dependence of INP concentration on anthropogenic particle emission, hampering the estimation of global atmospheric INP concentrations (Boucher et al., 2013; Seinfeld and Pandis, 2016). In this paper, we report the in situ INP concentration measured at mixed-phase cloud conditions (−30 ∘C, relative humidity with respect to liquid water of 104 %, i.e., RHw=104 %) during the traditional Chinese Spring Festival at an urban site in Beijing. Urban particle emission sources were distinguished based on the online chemical characterization using an aerosol chemical speciation monitor (ACSM). The correlations between immersion INP concentration, meteorology condition, and aerosol physiochemical properties are also explored.
2.1 Sampling
The sampling site (39∘59′20′′ N, 116∘18′26′′ E) is located on the roof of a six-floor building (∼ 30 m a.g.l.) at Peking University, which is adjacent to the northwestern 4th Ring Road of Beijing. The site lies about 250 m west of a busy street with heavy traffic. At the sampling site, meteorological parameters, including wind speed, wind direction, RHw, and temperature, were measured by a weather station (MetOne Inc.). The mass concentrations of particulate matter (PM) with aerodynamic diameter (da) smaller than 2.5 and 10 µm (PM2.5 and PM10, respectively) were measured by a tapered-element oscillating microbalance (TEOM) monitor. The temporal resolutions of meteorology and PM data were 1 min.
Ambient air was sampled through a stainless-steel tube with an inner diameter of 12.7 mm. The tube inlet was arched so that it was bent facing downwards (see Fig. 1) to prevent water contamination, with d10 and d50 (the da at which 10 % and 50 % particle could be transported to instruments through the inlet, respectively) being ∼ 20 and 13.4 µm, respectively (Brockmann, 2011, Eqs. 6-23 to 6-29). The sample flow was then split and pumped into different instruments. The relative humidity of the sample stream (RHw, sample) was kept below 2 % by passing samples through two consecutive 47 cm Nafion™ dryers (Perma Pure, LLC.) using 4 L min−1 nitrogen as sheath gas during the experiment. A schematic of the setup is shown in Fig. 1.
2.2 Instrumentation
2.2.1 Particle number size distribution
Submicron particle number size distribution was measured by a scanning mobility particle sizer (SMPS, model 3082, comprising a 3082 classifier, a 3081 long DMA,1 and a 3776 CPC;2 TSI Inc.). The sampling flow rate of the SMPS was set to 0.3 L min−1 with a sheath-to-sample ratio of 10:1, resulting in an electrical mobility size range from 14.6 to 710.5 nm. An impactor was installed at the DMA inlet to remove particles larger than 735 nm. Furthermore, multiple charging correction was applied when processing the submicron particle number size distribution.
An aerodynamic particle sizer (APS, model 3021; TSI Inc.) was used to provide number size distribution for ambient aerosols with da ranging from 0.542 to 19.81 µm every minute. The aerodynamic particle number size distribution obtained from the APS could be converted to particle mobility size (dm) distribution by assuming the effective density of ambient particles to be 1.5 g cm−3, which is commonly used for urban atmosphere (Khlystov et al., 2004; Chen et al., 2018; Qiao et al., 2018; An et al., 2019). The sampling flow rate of the APS was 1 L min−1.
2.2.2 Particle chemical composition
Real-time nonrefractory PM1 (da smaller than 1.0 µm) mass loading and chemical composition were measured by an ACSM (Aerodyne Inc.) equipped with a quadrupole analyzer (Ng et al., 2011). The sampling flow rate of the ACSM was 0.1 L min−1. A PM2.5 cyclone was installed upstream of the ACSM inlet to prevent inlet clogging by particles with da larger than 2.5 µm. The 50 % transmission efficiency range of the ACSM is ∼ 60–660 nm (Liu et al., 2007). The time resolution of an ACSM scan was set to 15 min. Meanwhile, BC mass concentration was monitored by a multiangle absorption photometer (MAAP, model 5012; Thermo, Inc.) with a temporal resolution of 1 min.
2.2.3 Ice-nucleating particle (INP) concentration
In situ immersion INP concentration was measured by a horizontal ice nucleation chamber (HINC) at fixed lamina conditions throughout the observation period, i.e., with a lamina temperature () of −30 ± 0.2 ∘C and RHw=104 ± 2.2 % (equivalent to RHi=140 ± 3.0 %, where the subscript i denotes ice). HINC is a CFDC-type instrument made of two flat parallel copper plates. The temperature of each plate is controlled independently to create supersaturation along the chamber centerline lamina. To minimize the impact of convection, the top plate of the HINC is warmer than the bottom plate. Ice crystal size and number were measured by a six-channel optical particle counter (OPC; MetOne Inc.). The injector position (and thus the flow structure of HINC in this study) is identical to the settings by Lacher et al. (2017). Estimated particle gravitational settling within the HINC is consistent with the OPC measurement by Lacher et al. (2017), i.e., ∼ 23.5 % for 1 µm particle, 46.6 % for 1.5 µm particles, and 100 % for particles larger than 5 µm (Brockmann, 2011, Eqs. 6-51 to 6-53). Therefore, only particles larger than 5 µm that were detected by the HINC OPC are counted as ice crystals. The INP concentration measurements in this study are representative of ambient particles smaller than 1.5 µm. For more detailed HINC design and operating principle information, please refer to Lacher et al. (2017) and Kanji and Abbatt (2009). In addition, the gravitational settling values estimated for 1, 2, and 5 µm particles in the tubing upstream of the HINC inlet are estimated to be ∼ 2.1 %, 7.7 %, and 41.0 %, respectively (Brockmann, 2011, Eqs. 6-51 to 6-53). Such sampling line loss is considered to be negligible, because particles larger than 1 µm were scarce during the observation (Table 1).
In this study, both warm and cold walls of the HINC lined with glass-fiber filter paper were wetted with ∼ 150 mL deionized water each day before the experiment start or after running the experiment for 4 h. After draining for ∼ 15 min, the wall temperatures of warm and cold walls would be set to −21.2 and −38.8 ∘C, respectively, to achieve desired lamina temperature (−30 ∘C) and RHw (104 %). The sampling flow rate of the HINC was 0.26 L min−1, surrounded by a 2.57 L min−1 particle-free nitrogen sheath gas. During the experiment, sampling air would pass through a particle filter for 5 min after every 15 min of measurement to quantify HINC background count detected by the OPC. The HINC background counts follow a Poisson distribution, on which the average background count is determined. Average ice crystal concentration (equivalent to NINP) of the 15 min measurement period is calculated by firstly subtracting the average background particle counts from measurement counts and secondly converting particle counts to number concentration using HINC sampling flow rate. The subtraction might produce negative NINP when the signal of the OPC during the measurement is undistinguishable from background noise. Therefore, this study reports positive NINP as is, and replaces negative NINP with the minimum quantifiable concentration of the OPC (0.26 # L−1), following the method in Lacher et al. (2017). Ambient particle number concentration entering the HINC was monitored by a CPC (model 3775, d50=4 nm; TSI Inc.) connected in parallel with HINC at the aerosol inlet (see Fig. 1).
Activation fraction (AF) and ice-active surface site density (nS) were selected as IN activity parameters in this study (Vali et al., 2015; Kanji et al., 2017). AF is the ratio between ice crystal number concentration at HINC outlet (calculated from OPC counts, as stated above) and total particle number concentration at HINC inlet (measured by CPC). Symbol nS is defined as the number of ice-active surface sites per unit surface area of INPs, and it allows IN activity intercomparison between different aerosol species and different studies as a normalized parameter (Hoose and Möhler, 2012; Vali et al., 2015). In this study, total surface area S for polydisperse ambient particles was firstly derived by assuming particles to be spherical and integrating the particle mobility size distribution (Lacher et al., 2018; Bi et al., 2019; Chen et al., 2021). Dividing NINP by S yields nS (Connolly et al., 2009; Hoose and Möhler, 2012; Niemand et al., 2012; Vali et al., 2015; Lacher et al., 2018; Bi et al., 2019).
3.1 Overview
The observation lasted from 10 to 28 February, overlapping with the traditional Chinese Spring Festival for the year 2021. Figure 2 displays (a) the chemical composition of nonrefractory PM1 mass concentration and (b) particle number size distribution. Figure 3 shows the ambient meteorological conditions, including (a) wind speed and wind direction, (b) temperature and RHw, and (c) PM2.5 and PM10 mass concentrations and NINP. Figure 4 presents the BC mass concentration (mBC) variation during the observation in addition to NINP. The grey shading in Figs. 2–4 indicates the IN experiment time periods.
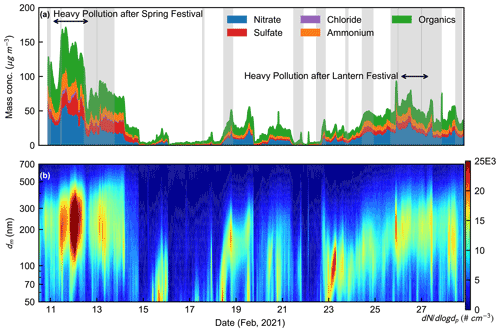
Figure 2Time series of (a) nonrefractory PM1 mass concentration and (b) particle number size distribution. The grey shading and black arrows in (a) indicate IN experiment time periods and heavy pollution after celebrations, respectively.
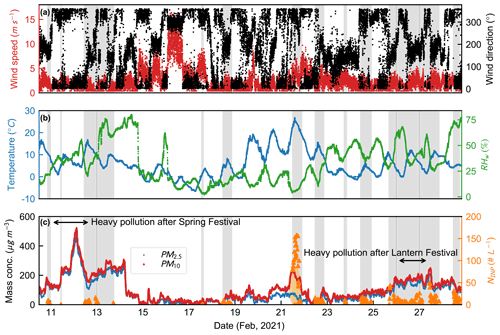
Figure 3Times series of (a) wind speed and direction, (b) ambient temperature and relative humidity with respect to liquid water (RHw), and (c) PM2.5 and PM10 mass concentrations and immersion INP number concentration (NINP). The grey shading in each panel indicates IN experiment time periods; the orange shading and arrows in (b) mark the dust event on 21 February 2021 and the heavy pollution after celebrations, respectively.
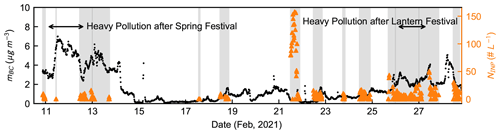
Figure 4Time series of black carbon (BC) particle mass concentration (mBC) and NINP. The grey shading and black arrows indicate IN experiment time periods and heavy pollution after celebrations, respectively.
On the nights of 11 and 26 February, fireworks were lit for celebrations of the Spring Festival and Lantern Festival. Large amounts of particles emerged after the celebrations due to firework emissions, as indicated by the sharp growth of nonrefractory PM1 mass concentration comprising organic components and chloride salts in Fig. 2a. Up to 7 µg m−3 of BC particles mainly originating from firework emissions were also detected during and after the celebrations, as illustrated in Fig. 4. Apart from the firework emissions, the mass concentration of nitrate and sulfate also increased significantly (Fig. 2a) under the relatively stagnant and humid meteorological conditions after the nights of 11 and 26 February (Fig. 3b), indicating secondary pollutant formation (e.g., Wu et al., 2018). Such secondary pollutant transformation processes produce particles in the accumulation mode, as illustrated by the high level of particle concentration peaking between 200–300 nm in Fig. 2b. During heavy pollution after the Spring Festival and Lantern Festival, PM2.5 mass concentrations, respectively, approached ∼ 550 and 200 µg m−3 (Fig. 3c). The synergetic heavy pollution induced by secondary pollutant formation and firework emission is marked with arrows in Figs. 2–4.
On 18 February, the first workday after the Spring Festival holiday (11 to 17 February), people swarmed into downtown Beijing, and the mass concentrations of nonrefractory PM1 and PM2.5 increased during rush hours, as can be seen in Figs. 2a and 3c, respectively. mBC also increased because of increasing use of passenger vehicles during rush hours on workdays after 18 February, as shown in Fig. 4.
During the whole observation, there was a minor difference between ambient PM2.5 and PM10 mass concentrations (PM10–2.5) except for 21 February afternoon (Table 1), when there was a significant increase of PM10 mass concentration as highlighted by the orange shading in Fig. 3c, implying that large particles with da ranging between 2.5 and 10 µm were present, which were most likely to be mineral dust particles (e.g., Park et al., 2004; Hoffmann et al., 2008; Rai et al., 2021). Besides, aerodynamic particle number size distribution exhibited a clear shift towards the larger end during the dust event (Fig. A1) with a mode size around 1 µm, which further confirmed the presence of large particles during the dust event. Aerosol optical depth (AOD) derived from MODIS Aqua Deep Blue Collection 6 dataset at 550 nm (Acker and Leptoukh, 2007) also shows elevated aerosol loading on 21 February afternoon at the sampling site compared to 20 February (Fig. A2). Based on measured particle mass concentration level and meteorological conditions, the observation days are categorized into different scenarios, i.e., dust event, clean, and heavy pollution days, as summarized in Table 1.
Table 1The date, number concentrations of immersion INPs (NINP) and ambient particles (NCPC), activation fraction (AF), ice-active surface site density (nS), number concentrations of particles ranging from 500 nm to 1.5 µm (N500) and 1000 nm to 1.5 µm (N1000), mass concentrations of BC particles (mBC) and ammonium salt (mammo.), PM2.5, PM10, and PM10–2.5 for each scenario. The numbers are average values, and numbers in parentheses denote 1 standard deviation (σ) from the average.
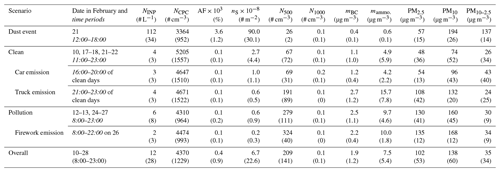
In the following sections, the data collected in this study are categorized into aforementioned scenarios. The correlation between NINP and the physiochemical properties of ambient particles, including particle number concentration and chemical composition, in each scenario and the potential source of immersion INPs are discussed. We also compare data to NINP measured under similar conditions reported in the literature, with particular attention to the IN activity and INP source attribution.
3.2 Contribution of mineral dust to NINP during the dust event
In the afternoon of 21 February 2021, a dust event occurred at the sampling site, as indicated clearly by the significant difference between PM10 and PM2.5 mass concentrations in Fig. 3c. PM10 mass concentration reached 250 µg m−3 and was 3 to 5 times as much as the PM2.5 mass concentration during the dust event.
The dust event is characterized with substantially higher AF (0.0036 % ± 0.0011 %) and nS (9.0 × 109 ± 3.0 × 109 # m−2) compared to other days, as listed in Table 1. During the dust event, NINP was 1 to 2 orders of magnitude higher than clean days, ranging from 40 to 160 # L−1. Meanwhile, the ambient particle number concentration entering HINC (NCPC) during the dust event is only half to two-thirds that of the clean-day concentration level (Table 1), leading to the distinguishably higher AF and nS. The significant increase of NINP in the dust event complies with the results by Bi et al. (2019), who reported NINP as high as 2800 # L−1 measured at −30 ∘C and RHw=106.5 % during a desert dust event at a rural sampling site in suburban Beijing. nS during the dust event is also on the same order of magnitude with Bi et al. (2019) and Lacher et al. (2018), as shown in Fig. 5. However, most of the parameterizations obtained from laboratory experiments using Asian dust (AD) samples tend to overestimate nS by 1–2 orders of magnitude (Connolly et al., 2009; Niemand et al., 2012; Ullrich et al., 2017), except for the size-segregated parameterization proposed by Reicher et al. (2019) that spans from 1.9 × 109 # m−2 for submicron particles to 4.2 × 1010 # m−2 for supermicron particles. The 24 h back trajectory analysis at three heights (20, 500, and 1000 m, Fig. A3) using the National Oceanic and Atmospheric Administration (NOAA) HYSPLIT (HYbrid Single-Particle Lagrangian Integrated Trajectory) model suggests that the air parcel during the dust event is from the northwestern direction of Beijing, originating from the Mongolia Gobi Desert. The back trajectory analysis by Bi et al. (2019) also suggested that air parcels from Mongolia Gobi Desert tended to carry loads of desert dust, leading to higher NINP.
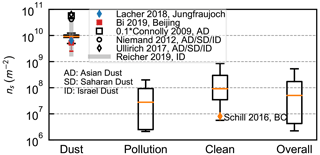
Figure 5Ice-active surface site density (nS) for different scenarios. The median (horizontal orange lines), 25th and 75th percentiles (lower and upper boxes), and 10th and 90th percentiles (lower and upper whiskers) are shown.
Previous laboratory studies have shown that larger particles, especially those larger than 500 or 1000 nm, exhibit superior INP activity based on surface active site density theory (e.g., Connolly et al., 2009; Welti et al., 2009; Lüönd et al., 2010; Hoose and Möhler, 2012; Ardon-Dryer and Levin, 2014; Chen et al., 2021). Bi et al. (2019) observed a significant increase of NINP when ambient particle peak size shifted towards the larger end of size spectra (exceeding 1000 nm) during springtime dust events in rural Beijing. N500 is generally below 50 # cm−3 during springtime dust events in Beijing (Bi et al., 2019) and is comparable to the results presented in Fig. 6a. To quantify the impact of N500 on NINP, linear regression analysis between logarithms of NINP and N500 was performed for the dust event using the ordinary least square (OLS) method, as shown in Fig. 6a. The triangles denote measured data, and the blue markers are the predicted data using the fitted linear regression parameters. The Pearson's correlation coefficient (r) between log 10(NINP) and log 10(N500) is −0.3 (Fig. 6a), reflecting that N500 is slightly correlated with NINP and might have limited impact on NINP during the dust event. Besides, the significant difference between PM10 and PM2.5 mass concentrations (Fig. 3c and Table 1) implies that large (dust) particles (occupies high mass concentration but low number concentration) with high IN activity appeared during the dust event. An earlier study in an eastern Mediterranean urban region claimed that immersion IN activity of particles collected during dust storms correlated well (R2=0.47) with PM10–2.5 between −10 and −30 ∘C (Ardon-Dryer and Levin, 2014). To explore the connection between PM10–2.5 and NINP in the urban environment, correlation analysis between NINP and PM10–2.5 data collected during the dust event was also conducted using OLS linear regression. The correlation between NINP and PM10–2.5 at −30 ∘C (, Fig. C1) during the dust event in this study suggests that PM10–2.5 had a moderate negative correlation with NINP in the urban environment, which is stronger yet not statistically significant. As suggested by previous studies (e.g., Atkinson et al., 2013; Kaufmann et al., 2016; Iwata and Matsuki, 2018), dust mineralogy might be a superior immersion IN driving factor instead of N500 and PM10–2.5 in the urban environment and worth further exploration.
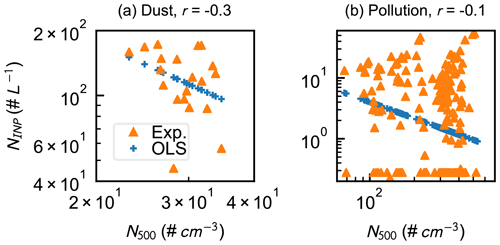
Figure 6Correlations between N500 and NINP for (a) dust event and (b) heavy pollution. The r above each panel is the Pearson's correlation coefficient for the linear regression fitting of the experiment data using ordinary least square (OLS) method. Blue markers indicate predicted NINP using fitted linear regression parameters.
The upper panel of Fig. 7 displays the diurnal profile of measured NINP and mass concentration of atmospheric ammonium (mammo.) during the dust event. It can be seen that, except for 13:00 (UTC+8), the NINP profile seems to follow the mammo. profile. Wu et al. (2020) reported that ammonium ions could form and accumulate on mineral dust surface in the form of ammonium nitrate in the highly populated urban environment. Previous laboratory studies have shown that the ammonium content on mineral dust surface might promote their IN activity due to strengthened ammonium ion surface adsorption followed by the formation of an ice-favorable structure on dust particle surfaces (Boose et al., 2016b; Kumar et al., 2018; Whale et al., 2018; Kumar et al., 2019). To quantify the correlation between atmospheric ammonium content and NINP during the dust event and to investigate whether the observed enhancement of mineral dust IN activity by ammonium salts in previous studies (Boose et al., 2016b; Kumar et al., 2018; Whale et al., 2018; Kumar et al., 2019) still holds for the urban environment, linear regression analysis between NINP and mammo. was performed, as shown in the lower panel of Fig. 7. The blue markers are fitted NINP based on the OLS regression parameters, and the blue shading refers to the ±1σ range (calculated from measured NINP) from fitted NINP. The NINP exhibits a moderate positive correlation with mammo. (r=0.5), with more than 60 % of measured NINP falling into the shaded area, suggesting that NINP might be associated with mammo. during dust events in the urban environment. It should be noted that NINP also has a weak positive correlation with (r=0.4, Table C1) during the dust event, but previous studies have confirmed that ammonium content, instead of anion species, is more likely to be the driving force in altering the immersion IN activity of mineral dusts (e.g., Kumar et al., 2018; Whale et al., 2018; Kumar et al., 2019). More field observations in urban areas, as well as systematic laboratory studies using natural mineral dust samples (e.g., Saharan dust and Asian dust, etc.), are required to further investigate the connection between mineral dust surface characteristics and IN activity, as well as the underlying mechanism.
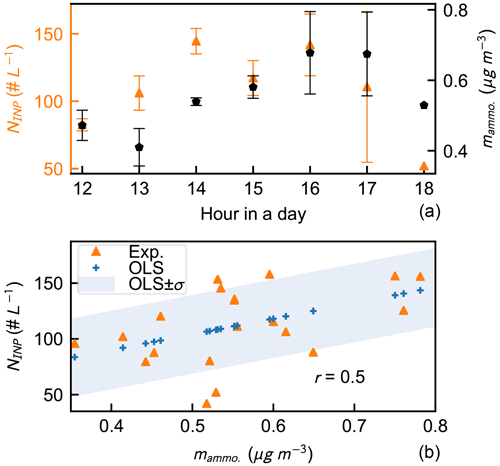
Figure 7Diurnal profile of (a) and correlation between (b) NINP and mammo. during the dust event. The blue markers in panel (b) indicate the predicted NINP using fitted linear regression parameters. The blue shading indicates ±1σ of measured NINP from predicted NINP.
NINP values measured during the dust event are compared with previous empirical parameterizations with a particular focus on mineral dusts to link parameterizations with the observation and gain better insight into the deviation between measured and predicted NINP. Almost all measured NINP data lie within a factor of 2.5 of the predicted NINP using the widely used parameterization proposed by DeMott et al. (2010) based on 14-year field observation data, as shown by the brown squares and shading in Fig. 8. This parameterization links NINP at a specific temperature with the exponential form of N500 and takes not just mineral dusts but all ambient IN-active aerosol types into consideration. It should be noted that most measured NINP values lie above the DeMott et al. (2010) parameterization predicted NINP. Such underestimation by the DeMott et al. (2010) parameterization for ammonium-rich mineral dusts was also reported earlier in a Saharan dust plume observation, and it was attributed to not taking the IN activity enhancement of mineral dusts by trace amount atmospheric ammonium into consideration (Boose et al., 2016b). Another empirical NINP parameterization proposed by DeMott et al. (2015) is specifically for mineral dusts based on laboratory measurement. However, it tends to systematically overestimate NINP during the dust event by up to an order of magnitude (3 to 13 times higher than the measured values) as shown by the blue squares in Fig. 8. Such overestimation on NINP during the dust event by previous parameterizations might be caused by omission of mineral dust chemical composition and mixing state change during transportation (e.g., Tobo et al., 2010; Wang et al., 2014; Li et al., 2016; Tang et al., 2016; Wu et al., 2020). Previous laboratory experiments suggest that even though mixing with organics might not affect the immersion IN activity of mineral dusts (Tobo et al., 2012; Wex et al., 2014; Kanji et al., 2019), mixing with sulfuric acids (Cziczo et al., 2009; Eastwood et al., 2009; Chernoff and Bertram, 2010; Niedermeier et al., 2011; Tobo et al., 2012; Augustin-Bauditz et al., 2014) and ammonium and sulfate salts (Cziczo et al., 2009; Iwata and Matsuki, 2018; Kumar et al., 2018; Whale et al., 2018; Kumar et al., 2019) could suppress the immersion IN activity of mineral dusts to different degrees. However, current parameterizations could represent the upper limit of atmospheric INP number concentration in global models, and we suggest that future parameterizations should include the influence of atmospheric processes (such as photo-oxidation and gaseous species condensation) on mineral dust IN activity to achieve more realistic prediction.
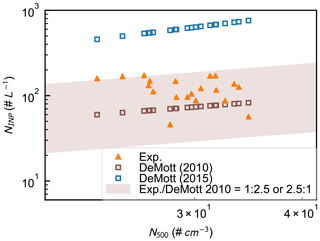
Figure 8Intercomparison of measured (solid orange triangles) and predicted (hollow blue and brown squares) NINP during the dust event. The prediction is based on the parameterizations that link NINP with the number concentration of particles larger than 500 nm (N500) at a specific temperature (DeMott et al., 2010, 2015).
3.3 Contribution of black carbon (BC) to NINP
3.3.1 Traffic emission
Clean days, when compared with heavy pollution or dusty days, provide an ideal background to investigate the impact of primary particle emission sources, especially local traffic emission (from clean days), on NINP in urban regions. The major particle formation pathway in gasoline passenger vehicle exhausts is volatile organic compound (VOC) nucleation, producing large numbers of nanoparticles (diameter smaller than 50 nm) with low mass concentration (Raza et al., 2018 and references therein). On the other hand, diesel engine particle emission is dominated by BC particles ranging between 80–200 nm (Kittelson, 1998). The increase of mass concentrations of organics (morg.) and BC between 16:00 to 20:00 (UTC+8) in Fig. 9 corresponds to the evening rush hours, during which the emission of gasoline passenger vehicles dominates ambient particle population in the urban region. There is a further increase of morg. and mBC after 20:00 (UTC+8) followed by a plateau in Fig. 9. According to Beijing municipal administrative regulation, heavy-duty diesel trucks for goods transportation, as well as gasoline passenger vehicles with foreign plates (issued by cities other than Beijing), are only permitted to enter urban Beijing after 20:00 (UTC+8). Increasing emission from on-road heavy-duty diesel trucks and gasoline passenger vehicles with foreign plates are highly likely to be responsible for the increasing morg. and mBC after 20:00 (UTC+8; e.g., Hua et al., 2018; Zhang et al., 2019).
NINP of clean days ranges between 0.3 to 16 # L−1, which is the same order of magnitude as the immersion NINP results by Schill et al. (2016), who reported NINP for both freshly emitted and aged BC on the order of 10−1 to 101 # L−1 measured at similar experiment condition (−30 ∘C and RHw=105 %) to this study, using BC generated from an off-road diesel engine. AF and nS values reported by Schill et al. (2016) lie in the lower range of measured AF and nS associated with vehicle emission periods (Table 1 and Fig. 5). To investigate the impact of traffic emission on NINP, linear regression analysis is performed between NINP and mBC, a widely used cursor of traffic emission. As shown in Fig. 10a, the correlation between NINP and mBC is poor (r= 0), implying that NINP is independent of mBC on clean days. Recently, Kanji et al. (2020) also reported that BC might not act as effective immersion INPs based on laboratory experiments. The absence of a relationship between NINP and mBC on clean days is consistent with previous findings (Schill et al., 2016; Kanji et al., 2020; Schill et al., 2020), which suggests that BC associated with vehicle emission might not act as active immersion INPs in the urban atmosphere.
3.3.2 Firework emission
Heavy pollution accompanied by the presence of substantially higher mass concentrations of nonrefractory PM1, PM2.5 (128 ± 44 µg m−3), and BC (up to 6 µg m−3) occurred during 12–13 and 24–27 February, as shown in Figs. 2–4. The N500 was also substantially higher during heavy pollution than other days (Table 1). As stated above, large amounts of particles comprising carbonaceous material and chloride emerged after Spring Festival and Lantern Festival celebrations due to firework emissions which also contain trace amounts of potassium and other metal elements (Jiang et al., 2015; Kong et al., 2015; Cao et al., 2017).
With almost 10 times as much N500 as that of the dust event during heavy pollution, it was expected that a substantially higher INP concentration would be observed if these large particles are effective INPs. However, the particle population during heavy pollution did not exhibit superior IN activity, with the majority of NINP falling into the range of 0 to 25 # L−1. The log 10(NINP) exhibits a weak negative correlation with log 10(N500) in Fig. 6b (). As shown in Fig. 10b, the OLS linear regression results further suggest that NINP is likely to be independent of mBC during heavily polluted days (r=0). The independence of NINP on mBC is compliant with the results by Adams et al. (2020), in which there was a substantial growth (by more than an order of magnitude) of ambient particle number concentration and mBC from combustion and firework emissions, but no significant NINP change was observed. Chen et al. (2018) conducted offline NINP measurements using filtered samples collected at the same sampling site as this study during heavy pollution, and they found no dependence of NINP on the mass concentrations of PM2.5 or BC nor on N500. As mentioned before, the synergetic heavy pollution after festival celebrations was induced by secondary pollutant formation via liquid-phase reaction (e.g., Wang et al., 2018; Wu et al., 2018) and firework emission. Under the mixed-phase cloud conditions (−30 ∘C, RHw=104 %) in this study, such particles are very likely to become aqueous droplets or contain liquid films on solid particles, which might require conditions for homogeneous freezing to nucleate ice.
In situ observation of NINP and physiochemical properties, including chemical composition and size distribution, of ambient particles at an urban site in Beijing during the traditional Chinese Spring Festival has been performed at mixed-phase cloud conditions (−30 ∘C, RHw=104 %) for 18 d. The impact of different scenarios, such as the synergetic heavy pollution induced by secondary aerosol formation and firework emissions, a dust event, and local traffic emissions on NINP, has been explored. NINP was investigated in relation to N500 and mBC. The relationships between mammo. and NINP, as well as between PM10–2.5 and NINP, during the dust event are also presented. The results show that NINP values , as well as AF, of ambient particles during the dust event are substantially higher than all other scenarios. NINP could reach 160 # L−1 during the dust event, while it ranges from 10−1 to 101 # L−1 on other days. AF and nS during the dust event (0.0036 % ± 0.0011 % and 9.0 × 109 ± 3.0 × 109 # m−2) are 20 to 30 times higher than clean (0.0001 % ± 0.0001 % and 2.7 × 108 ± 4.4 × 108 # m−2) and heavily polluted days (0.0002 % ± 0.0002 % and 6.5 × 107 ± 9.3 × 107 # m−2). During the dust event, NINP values exhibit a moderate positive correlation with mammo. (r=0.5) and a moderate negative correlation with PM10–2.5 (). The parameterization proposed by DeMott et al. (2010) predicts more than 60 % of measured NINP within a factor of 2.5 during the dust event. Mass concentration measurements suggest that large amounts of aerosols containing chloride and BC appeared after the celebrations on the nights of 11 and 26 February due to firework emission. Meanwhile, the stagnant and humid meteorology conditions provide ideal conditions for secondary aerosol formation. But there is no significant difference between NINP on heavily polluted and clean days, implying that the urban aerosols from multiple sources with complex chemistry might not be effective INPs. Besides, the diurnal increase of mBC from petrol passenger vehicle emissions during rush hours and from diesel truck emissions after 20:00 (UTC+8) on clean days does not lead to distinguishable higher NINP, implying that local traffic emission also has a negligible impact on NINP. Our study reveals that immersion INP population in the urban environment has increased substantially during the East Asian dust event. Furthermore, our results agree with previous literature from laboratory and field studies showing that atmospheric BC from both local traffic and firework emissions has negligible effects on mixed-phase cloud formation and that NINP is unaffected by heavy pollution.
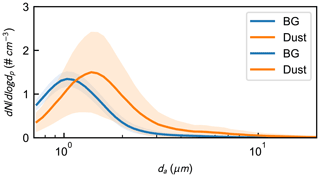
Figure A1Aerodynamic diameter (da) size distribution of particles before (00:00–06:00, 21 February; “BG”) and during the dust event (11:00–18:00, 21 February; “Dust”).
The HINC lamina condition calibration was performed by injection of 200 nm ammonium nitrate aqueous droplets into HINC at four lamina temperatures, i.e., −45, −40, −35, and −30 ∘C. HINC was operated in RH scan mode during the calibration, in which HINC lamina temperature was maintained constant by varying the wall temperatures simultaneously, resulting in a temperature gradient and supersaturation in the chamber (Lacher et al., 2017). The RHi within the HINC lamina changed continuously from 100 % to 160 % at each lamina temperature. The concentration of the ammonium nitrate solution was 0.0025 mol L−1. The solution was atomized by a nebulizer (TSI Inc.) using 1.5 L min−1 nitrogen gas. The flow stream was dried to RHw<2 % by passing it through a 47 cm Nafion™ dryer, and it was then size selected by a DMA (model 3081 long; TSI Inc.). Particle number concentration entering the HINC was measured online by a CPC (model 3775; TSI Inc.).
Figure B1 shows the AF as a function of RHw at −30 ∘C for 200 nm ammonium nitrate aqueous droplets. Different OPC channels (>1, >2, >3, >5 µm) are marked with different colors. It is worth noting that given the flow structure of HINC in this study (Sect. 2.2.3), only particles larger than 5 µm detected by the HINC OPC would be recognized as detectable ice crystals (below −38 ∘C) or water droplets (above −38 ∘C). As shown in Fig. B1, the 200 nm ammonium nitrate aqueous droplets start to grow upon water saturation (black dots), followed by more rapid growth with increasing RHw (grey dots). However, there is no growth in the >5 µm channel until RHw exceeds 106 %, corresponding to the presence of detectable water droplets larger than 5 µm. Therefore, the HINC should be operated below 106 % at −30 ∘C to avoid erroneous counts of large (>5 µm) water droplets rather than ice crystals.
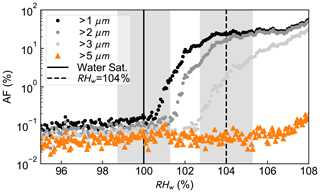
Figure B1AF as a function of RHw at −30 ∘C for 200 nm ammonium nitrate aqueous droplets detected in different HINC OPC channels. Vertical solid and dash black lines represent water saturation (RHw=100 %) and RHw=104 %, respectively. Grey shading indicates the average variation of RHw along HINC lamina centerline.
Figure B2 shows the IN onset or water droplet survival points for 200 nm ammonium nitrate during the calibration. The IN onset or water droplet survival points are defined as the temperature and RH when 0.1 % of aerosols entering HINC are activated as detectable ice crystals or water droplets by the HINC OPC in the >5 µm channel. The blue and black solid lines represent water saturation (RHw=100 %) and homogeneous freezing threshold (Koop et al., 2000), respectively. The error bars in Fig. B2 represent 1 standard deviation of temperature and RHi along HINC lamina centerline for each individual RHi scan. During the calibration, the average variation of lamina RHw was less than 1.2 % (corresponding to a 1.8 % variance of lamina RHi). Lamina RHw suffers larger variation as RH increases, resulting in RHw=108 % ± 2.1 % at −30 ∘C. The variance of lamina temperature was below 0.2 K throughout the calibration process. As shown in Fig. B2, the IN onset point of 200 nm ammonium nitrate at −40 ∘C lies on the calculated homogeneous freezing threshold. The IN onset at −45 ∘C exceeds the homogeneous freezing threshold by 3.5 %, yet it is still below the water saturation line. When the lamina temperature is above −38 ∘C, water drops require RHw substantially higher than 104 % (dashed line) to be detected in the >5 µm OPC channel; as such, we are confident that signals arising in the >5 µm OPC channel at RHw=104 % are due to ice crystal formation.
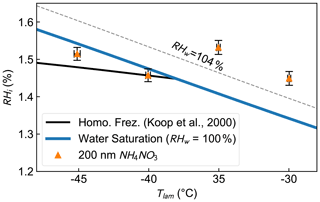
Figure B2Phase diagram of the IN onset (below −38 ∘C) or water droplet detection in the >5 µm OPC channel (above −38 ∘C) for freezing or water droplet formation onto 200 nm dry diameter ammonium nitrate particles. The solid blue and black lines represent the water saturation line and the homogeneous freezing threshold of 200 nm aqueous droplets (Koop et al., 2000), respectively. The horizontal and vertical error bars represent the variation of temperature and RHi along HINC lamina centerline.
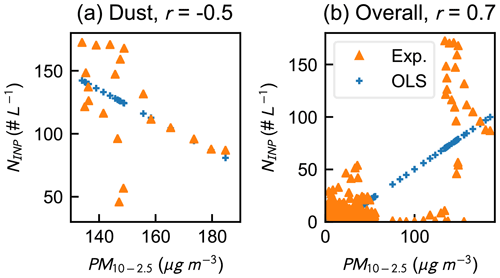
Figure C1Correlations between PM10–2.5 and NINP in the (a) dust event and (b) observation. The r above each panel is Pearson's correlation coefficient. Blue markers indicate the predicted NINP using fitted linear regression parameters.
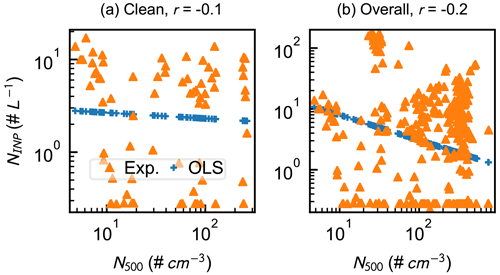
Figure C2Correlations between N500 and NINP for (a) clean days and (b) the observation. The r above each panel is Pearson's correlation coefficient. Blue markers indicate the predicted NINP using fitted linear regression parameters.
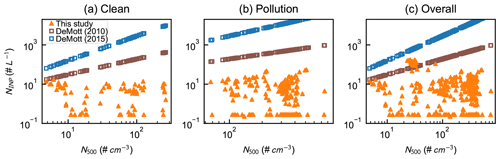
Figure C3Intercomparison of measured (solid orange triangles) and predicted (hollow blue and brown squares) NINP on clean (a) and heavy pollution (b) days, as well as during the observation (c). The prediction is based on the parameterizations that link NINP with the number concentration of particles larger than 500 nm (N500) at a specific temperature (DeMott et al., 2010, 2015).
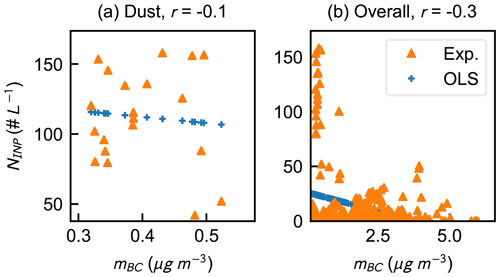
Figure C4Correlations between mBC and NINP during the (a) dust event and (b) observation. The r above each panel is Pearson's correlation coefficient. Blue markers indicate the predicted NINP using fitted linear regression parameters.
Data inquires can be directed to the corresponding author (Zhijun Wu, zhijunwu@pku.edu.cn).
CZ and ZW designed the experiments and methodology. CZ and JingC conducted the field observation. CZ, WZ, and LT performed aerosol chemical and size distribution analyses. CZ, JieC, XP, and JingC calibrated the HINC. SC and LZ provided the meteorology data. MH, ZW, ZAK, PT, and SG supervised the observation. CZ, ZW, and ZAK prepared the article with input from all co-authors.
The contact author has declared that neither they nor their co-authors have any competing interests.
Publisher’s note: Copernicus Publications remains neutral with regard to jurisdictional claims in published maps and institutional affiliations.
Aerosol optical depth data used in this paper were produced with the Giovanni online data system, developed and maintained by the NASA GES DISC.
This work was supported by National Natural Science Foundation of China (NSFC, grant nos. 41875149, 42011530121). Zamin A. Kanji received funding from the Atmospheric Physics chair, ETH Zürich.
This paper was edited by Anne Perring and reviewed by two anonymous referees.
Abbatt, J. P. D., Benz, S., Cziczo, D. J., Kanji, Z., Lohmann, U., and Möhler, O.: Solid Ammonium Sulfate Aerosols as Ice Nuclei: A Pathway for Cirrus Cloud Formation, Science, 313, 1770–1773, https://doi.org/10.1126/science.1129726, 2006.
Acker, J. G. and Leptoukh, G.: Online analysis enhances use of NASA Earth science data, Eos, Transactions American Geophysical Union, 88, 14–17, https://doi.org/10.1029/2007EO020003, 2007.
Adams, M. P., Tarn, M. D., Sanchez-Marroquin, A., Porter, G. C. E., O'Sullivan, D., Harrison, A. D., Cui, Z., Vergara-Temprado, J., Carotenuto, F., Holden, M. A., Daily, M. I., Whale, T. F., Sikora, S. N. F., Burke, I. T., Shim, J. U., McQuaid, J. B., and Murray, B. J.: A Major Combustion Aerosol Event Had a Negligible Impact on the Atmospheric Ice-Nucleating Particle Population, J. Geophys. Res.-Atmos., 125, e2020JD032938, https://doi.org/10.1029/2020JD032938, 2020.
An, Z., Huang, R.-J., Zhang, R., Tie, X., Li, G., Cao, J., Zhou, W., Shi, Z., Han, Y., Gu, Z., and Ji, Y.: Severe haze in northern China: A synergy of anthropogenic emissions and atmospheric processes, P. Natl. Acad. Sci. USA, 116, 8657–8666, https://doi.org/10.1073/pnas.1900125116, 2019.
Ansmann, A., Tesche, M., Althausen, D., Müller, D., Seifert, P., Freudenthaler, V., Heese, B., Wiegner, M., Pisani, G., Knippertz, P., and Dubovik, O.: Influence of Saharan dust on cloud glaciation in southern Morocco during the Saharan Mineral Dust Experiment, J. Geophys. Res.-Atmos., 113, D04210, https://doi.org/10.1029/2007JD008785, 2008.
Archuleta, C. M., DeMott, P. J., and Kreidenweis, S. M.: Ice nucleation by surrogates for atmospheric mineral dust and mineral dust/sulfate particles at cirrus temperatures, Atmos. Chem. Phys., 5, 2617–2634, https://doi.org/10.5194/acp-5-2617-2005, 2005.
Ardon-Dryer, K. and Levin, Z.: Ground-based measurements of immersion freezing in the eastern Mediterranean, Atmos. Chem. Phys., 14, 5217–5231, https://doi.org/10.5194/acp-14-5217-2014, 2014.
Atkinson, J. D., Murray, B. J., Woodhouse, M. T., Whale, T. F., Baustian, K. J., Carslaw, K. S., Dobbie, S., O'Sullivan, D., and Malkin, T. L.: The importance of feldspar for ice nucleation by mineral dust in mixed-phase clouds, Nature, 498, 355–358, https://doi.org/10.1038/nature12278, 2013.
Augustin-Bauditz, S., Wex, H., Kanter, S., Ebert, M., Niedermeier, D., Stolz, F., Prager, A., and Stratmann, F.: The immersion mode ice nucleation behavior of mineral dusts: A comparison of different pure and surface modified dusts, Geophys. Res. Lett., 41, 7375–7382, https://doi.org/10.1002/2014GL061317, 2014.
Bergeron, T.: On the physics of cloud and precipitation, in: Proceedings of the Fifth Assembly of International Union of Geodesy and Geophysics, Lisbon, Portugal, 14 to 23 September 1933, 156–178, 1935.
Bi, K., McMeeking, G. R., Ding, D. P., Levin, E. J. T., DeMott, P. J., Zhao, D. L., Wang, F., Liu, Q., Tian, P., Ma, X. C., Chen, Y. B., Huang, M. Y., Zhang, H. L., Gordon, T. D., and Chen, P.: Measurements of Ice Nucleating Particles in Beijing, China, J. Geophys. Res.-Atmos., 124, 8065–8075, https://doi.org/10.1029/2019JD030609, 2019.
Boose, Y., Kanji, Z. A., Kohn, M., Sierau, B., Zipori, A., Crawford, I., Lloyd, G., Bukowiecki, N., Herrmann, E., Kupiszewski, P., Steinbacher, M., and Lohmann, U.: Ice Nucleating Particle Measurements at 241 K during Winter Months at 3580 m MSL in the Swiss Alps, J. Atmos. Sci., 73, 2203–2228, https://doi.org/10.1175/jas-d-15-0236.1, 2016a.
Boose, Y., Sierau, B., García, M. I., Rodríguez, S., Alastuey, A., Linke, C., Schnaiter, M., Kupiszewski, P., Kanji, Z. A., and Lohmann, U.: Ice nucleating particles in the Saharan Air Layer, Atmos. Chem. Phys., 16, 9067–9087, https://doi.org/10.5194/acp-16-9067-2016, 2016b.
Boucher, O., Randall, D., Artaxo, P., Bretherton, C., Feingold, G., Forster, P., Kerminen, V.-M., Kondo, Y., Liao, H., Lohmann, U., Rasch, P., Satheesh, S. K., Sherwood, S., Stevens, B., and Zhang, X. Y.: Clouds and Aerosols, in: Climate Change 2013: The Physical Science Basis. Contribution of Working Group I to the Fifth Assessment Report of the Intergovernmental Panel on Climate Change, edited by: Stocker, T. F., Qin, D., Plattner, G.-K., Tignor, M., Allen, S. K., Boschung, J., Nauels, A., Xia, Y., Bex, V., and Midgley, P. M., Cambridge University Press, Cambridge, United Kingdom and New York, NY, USA, 571–658, https://doi.org/10.1017/CBO9781107415324.016, 2013.
Brockmann, J. E.: Aerosol Transport in Sampling Lines and Inlets, in: Aerosol Measurement: Principles, Techniques, and Applications, 3rd edn., edited by: Baron, P. A., Kulkarni, P., and Willeke, K., Wiley Online Books, John Wiley & Sons, Inc., Hoboken, New Jersey, 68–105, ISBN 9781118001684, https://doi.org/10.1002/9781118001684.ch6, 2011.
Cantrell, W. and Heymsfield, A.: Production of Ice in Tropospheric Clouds: A Review, B. Am. Meteorol. Soc., 86, 795–808, https://doi.org/10.1175/bams-86-6-795, 2005.
Cao, X., Zhang, X., Tong, D. Q., Chen, W., Zhang, S., Zhao, H., and Xiu, A.: Review on physicochemical properties of pollutants released from fireworks: environmental and health effects and prevention, Environ. Rev., 26, 133–155, https://doi.org/10.1139/er-2017-0063, 2017.
Che, Y., Dang, J., Fang, W., Shen, X., Sun, J., Chen, Y., and Qian, Y.: Measurements of natural ice nucleating particles in Beijing in the spring of 2017, Atmos. Environ., 200, 170–177, https://doi.org/10.1016/j.atmosenv.2018.12.020, 2019.
Che, Y., Zhang, J., Zhao, C., Fang, W., Xue, W., Yang, W., Ji, D., Dang, J., Duan, J., Sun, J., Shen, X., and Zhou, X.: A study on the characteristics of ice nucleating particles concentration and aerosols and their relationship in spring in Beijing, Atmos. Res., 247, 105196, https://doi.org/10.1016/j.atmosres.2020.105196, 2021.
Chen, J., Wu, Z., Augustin-Bauditz, S., Grawe, S., Hartmann, M., Pei, X., Liu, Z., Ji, D., and Wex, H.: Ice-nucleating particle concentrations unaffected by urban air pollution in Beijing, China, Atmos. Chem. Phys., 18, 3523–3539, https://doi.org/10.5194/acp-18-3523-2018, 2018.
Chen, J., Wu, Z., Chen, J., Reicher, N., Fang, X., Rudich, Y., and Hu, M.: Size-resolved atmospheric ice-nucleating particles during East Asian dust events, Atmos. Chem. Phys., 21, 3491–3506, https://doi.org/10.5194/acp-21-3491-2021, 2021.
Chernoff, D. I., and Bertram, A. K.: Effects of sulfate coatings on the ice nucleation properties of a biological ice nucleus and several types of minerals, J. Geophys. Res.-Atmos., 115, D20205, https://doi.org/10.1029/2010JD014254, 2010.
Chou, C.: Investigation of ice nucleation properties onto soot, bioaerosol and mineral dust during different measurement campaigns, PhD thesis, ETH Zürich, https://doi.org/10.3929/ethz-a-006541204, 2011.
Conen, F., Rodríguez, S., Hülin, C., Henne, S., Herrmann, E., Bukowiecki, N., and Alewell, C.: Atmospheric ice nuclei at the high-altitude observatory Jungfraujoch, Switzerland, Tellus B, 67, 25014, https://doi.org/10.3402/tellusb.v67.25014, 2015.
Conen, F., Einbock, A., Mignani, C., and Hüglin, C.: Measurement report: Ice-nucleating particles active ≥ −15 ∘C in free tropospheric air over western Europe, Atmos. Chem. Phys., 22, 3433–3444, https://doi.org/10.5194/acp-22-3433-2022, 2022.
Connolly, P. J., Möhler, O., Field, P. R., Saathoff, H., Burgess, R., Choularton, T., and Gallagher, M.: Studies of heterogeneous freezing by three different desert dust samples, Atmos. Chem. Phys., 9, 2805–2824, https://doi.org/10.5194/acp-9-2805-2009, 2009.
Corbin, J. C., Rehbein, P. J. G., Evans, G. J., and Abbatt, J. P. D.: Combustion particles as ice nuclei in an urban environment: Evidence from single-particle mass spectrometry, Atmos. Environ., 51, 286–292, https://doi.org/10.1016/j.atmosenv.2012.01.007, 2012.
Cziczo, D. J., Froyd, K. D., Gallavardin, S. J., Moehler, O., Benz, S., Saathoff, H., and Murphy, D. M.: Deactivation of ice nuclei due to atmospherically relevant surface coatings, Environ. Res. Lett., 4, 044013, https://doi.org/10.1088/1748-9326/4/4/044013, 2009.
Cziczo, D. J., Froyd, K. D., Hoose, C., Jensen, E. J., Diao, M., Zondlo, M. A., Smith, J. B., Twohy, C. H., and Murphy, D. M.: Clarifying the dominant sources and mechanisms of cirrus cloud formation, Science, 340, 1320–1324, 2013.
de Boer, G., Morrison, H., Shupe, M. D., and Hildner, R.: Evidence of liquid dependent ice nucleation in high-latitude stratiform clouds from surface remote sensors, Geophys. Res. Lett., 38, L01803, https://doi.org/10.1029/2010GL046016, 2011.
DeMott, P. J., Cziczo, D. J., Prenni, A. J., Murphy, D. M., Kreidenweis, S. M., Thomson, D. S., Borys, R., and Rogers, D. C.: Measurements of the concentration and composition of nuclei for cirrus formation, P. Natl. Acad. Sci. USA, 100, 14655–14660, https://doi.org/10.1073/pnas.2532677100, 2003.
DeMott, P. J., Prenni, A. J., Liu, X., Kreidenweis, S. M., Petters, M. D., Twohy, C. H., Richardson, M. S., Eidhammer, T., and Rogers, D. C.: Predicting global atmospheric ice nuclei distributions and their impacts on climate, P. Natl. Acad. Sci. USA, 107, 11217–11222, https://doi.org/10.1073/pnas.0910818107, 2010.
DeMott, P. J., Prenni, A. J., McMeeking, G. R., Sullivan, R. C., Petters, M. D., Tobo, Y., Niemand, M., Möhler, O., Snider, J. R., Wang, Z., and Kreidenweis, S. M.: Integrating laboratory and field data to quantify the immersion freezing ice nucleation activity of mineral dust particles, Atmos. Chem. Phys., 15, 393–409, https://doi.org/10.5194/acp-15-393-2015, 2015.
Eastwood, M. L., Cremel, S., Wheeler, M., Murray, B. J., Girard, E., and Bertram, A. K.: Effects of sulfuric acid and ammonium sulfate coatings on the ice nucleation properties of kaolinite particles, Geophys. Res. Lett., 36, L02811, https://doi.org/10.1029/2008GL035997, 2009.
Field, P. R. and Heymsfield, A. J.: Importance of snow to global precipitation, Geophys. Res. Lett., 42, 9512–9520, https://doi.org/10.1002/2015GL065497, 2015.
Findeisen, W.: Kolloid-meteorologische Vorgänge bei Neiderschlags-bildung, Meteorol. Z, 55, 121–133, 1938.
Heymsfield, A. J., Schmitt, C., Chen, C.-C.-J., Bansemer, A., Gettelman, A., Field, P. R., and Liu, C.: Contributions of the Liquid and Ice Phases to Global Surface Precipitation: Observations and Global Climate Modeling, J. Atmos. Sci., 77, 2629–2648, https://doi.org/10.1175/JAS-D-19-0352.1, 2020.
Hoffmann, C., Funk, R., Sommer, M., and Li, Y.: Temporal variations in PM10 and particle size distribution during Asian dust storms in Inner Mongolia, Atmos. Environ., 42, 8422–8431, https://doi.org/10.1016/j.atmosenv.2008.08.014, 2008.
Hoose, C. and Möhler, O.: Heterogeneous ice nucleation on atmospheric aerosols: a review of results from laboratory experiments, Atmos. Chem. Phys., 12, 9817–9854, https://doi.org/10.5194/acp-12-9817-2012, 2012.
Hua, Y., Wang, S., Jiang, J., Zhou, W., Xu, Q., Li, X., Liu, B., Zhang, D., and Zheng, M.: Characteristics and sources of aerosol pollution at a polluted rural site southwest in Beijing, China, Sci. Total Environ., 626, 519–527, https://doi.org/10.1016/j.scitotenv.2018.01.047, 2018.
Iwata, A. and Matsuki, A.: Characterization of individual ice residual particles by the single droplet freezing method: a case study in the Asian dust outflow region, Atmos. Chem. Phys., 18, 1785–1804, https://doi.org/10.5194/acp-18-1785-2018, 2018.
Jiang, Q., Sun, Y. L., Wang, Z., and Yin, Y.: Aerosol composition and sources during the Chinese Spring Festival: fireworks, secondary aerosol, and holiday effects, Atmos. Chem. Phys., 15, 6023–6034, https://doi.org/10.5194/acp-15-6023-2015, 2015.
Kanji, Z. A. and Abbatt, J. P. D.: Laboratory studies of ice formation via deposition mode nucleation onto mineral dust and n-hexane soot samples, J. Geophys. Res., 111, D16204, https://doi.org/10.1029/2005jd006766, 2006.
Kanji, Z. A. and Abbatt, J. P. D.: The University of Toronto Continuous Flow Diffusion Chamber (UT-CFDC): A Simple Design for Ice Nucleation Studies, Aerosol Sci. Technol., 43, 730–738, https://doi.org/10.1080/02786820902889861, 2009.
Kanji, Z. A., Ladino, L. A., Wex, H., Boose, Y., Burkert-Kohn, M., Cziczo, D. J., and Krämer, M.: Overview of Ice Nucleating Particles, Meteor. Mon., 58, 1.1–1.33, https://doi.org/10.1175/amsmonographs-d-16-0006.1, 2017.
Kanji, Z. A., Sullivan, R. C., Niemand, M., DeMott, P. J., Prenni, A. J., Chou, C., Saathoff, H., and Möhler, O.: Heterogeneous ice nucleation properties of natural desert dust particles coated with a surrogate of secondary organic aerosol, Atmos. Chem. Phys., 19, 5091–5110, https://doi.org/10.5194/acp-19-5091-2019, 2019.
Kanji, Z. A., Welti, A., Corbin, J. C., and Mensah, A. A.: Black Carbon Particles Do Not Matter for Immersion Mode Ice Nucleation, Geophys. Res. Lett., 47, e2019GL086764, https://doi.org/10.1029/2019gl086764, 2020.
Kaufmann, L., Marcolli, C., Hofer, J., Pinti, V., Hoyle, C. R., and Peter, T.: Ice nucleation efficiency of natural dust samples in the immersion mode, Atmos. Chem. Phys., 16, 11177–11206, https://doi.org/10.5194/acp-16-11177-2016, 2016.
Khlystov, A., Stanier, C., and Pandis, S. N.: An Algorithm for Combining Electrical Mobility and Aerodynamic Size Distributions Data when Measuring Ambient Aerosol Special Issue of Aerosol Science and Technology on Findings from the Fine Particulate Matter Supersites Program, Aerosol Sci. Technol., 38, 229–238, https://doi.org/10.1080/02786820390229543, 2004.
Kittelson, D. B.: Engines and nanoparticles: a review, J. Aerosol Sci., 29, 575–588, https://doi.org/10.1016/S0021-8502(97)10037-4, 1998.
Knopf, D. A., Wang, B., Laskin, A., Moffet, R. C., and Gilles, M. K.: Heterogeneous nucleation of ice on anthropogenic organic particles collected in Mexico City, Geophys. Res. Lett., 37, L11803, https://doi.org/10.1029/2010gl043362, 2010.
Kong, S. F., Li, L., Li, X. X., Yin, Y., Chen, K., Liu, D. T., Yuan, L., Zhang, Y. J., Shan, Y. P., and Ji, Y. Q.: The impacts of firework burning at the Chinese Spring Festival on air quality: insights of tracers, source evolution and aging processes, Atmos. Chem. Phys., 15, 2167–2184, https://doi.org/10.5194/acp-15-2167-2015, 2015.
Koop, T., Luo, B., Tsias, A., and Peter, T.: Water activity as the determinant for homogeneous ice nucleation in aqueous solutions, Nature, 406, 611–614, https://doi.org/10.1038/35020537, 2000.
Korolev, A., McFarquhar, G., Field, P. R., Franklin, C., Lawson, P., Wang, Z., Williams, E., Abel, S. J., Axisa, D., Borrmann, S., Crosier, J., Fugal, J., Krämer, M., Lohmann, U., Schlenczek, O., Schnaiter, M., and Wendisch, M.: Mixed-Phase Clouds: Progress and Challenges, Meteor. Mon., 58, 5.1–5.50, https://doi.org/10.1175/AMSMONOGRAPHS-D-17-0001.1, 2017.
Kumar, A., Marcolli, C., Luo, B., and Peter, T.: Ice nucleation activity of silicates and aluminosilicates in pure water and aqueous solutions – Part 1: The K-feldspar microcline, Atmos. Chem. Phys., 18, 7057–7079, https://doi.org/10.5194/acp-18-7057-2018, 2018.
Kumar, A., Marcolli, C., and Peter, T.: Ice nucleation activity of silicates and aluminosilicates in pure water and aqueous solutions – Part 3: Aluminosilicates, Atmos. Chem. Phys., 19, 6059–6084, https://doi.org/10.5194/acp-19-6059-2019, 2019.
Lacher, L., Lohmann, U., Boose, Y., Zipori, A., Herrmann, E., Bukowiecki, N., Steinbacher, M., and Kanji, Z. A.: The Horizontal Ice Nucleation Chamber (HINC): INP measurements at conditions relevant for mixed-phase clouds at the High Altitude Research Station Jungfraujoch, Atmos. Chem. Phys., 17, 15199–15224, https://doi.org/10.5194/acp-17-15199-2017, 2017.
Lacher, L., DeMott, P. J., Levin, E. J. T., Suski, K. J., Boose, Y., Zipori, A., Herrmann, E., Bukowiecki, N., Steinbacher, M., Gute, E., Abbatt, J. P. D., Lohmann, U., and Kanji, Z. A.: Background Free-Tropospheric Ice Nucleating Particle Concentrations at Mixed-Phase Cloud Conditions, J. Geophys. Res.-Atmos., 123, 10506–10525, https://doi.org/10.1029/2018JD028338, 2018.
Lei, L., Zhou, W., Chen, C., He, Y., Li, Z., Sun, J., Tang, X., Fu, P., Wang, Z., and Sun, Y.: Long-term characterization of aerosol chemistry in cold season from 2013 to 2020 in Beijing, China, Environ. Pollut., 268, 115952, https://doi.org/10.1016/j.envpol.2020.115952, 2021.
Li, J., Gao, W., Cao, L., Xiao, Y., Zhang, Y., Zhao, S., Liu, Z., Liu, Z., Tang, G., Ji, D., Hu, B., Song, T., He, L., Hu, M., and Wang, Y.: Significant changes in autumn and winter aerosol composition and sources in Beijing from 2012 to 2018: Effects of clean air actions, Environ. Pollut., 268, 115855, https://doi.org/10.1016/j.envpol.2020.115855, 2021.
Li, Z., Lau, W. K. M., Ramanathan, V., Wu, G., Ding, Y., Manoj, M. G., Liu, J., Qian, Y., Li, J., Zhou, T., Fan, J., Rosenfeld, D., Ming, Y., Wang, Y., Huang, J., Wang, B., Xu, X., Lee, S. S., Cribb, M., Zhang, F., Yang, X., Zhao, C., Takemura, T., Wang, K., Xia, X., Yin, Y., Zhang, H., Guo, J., Zhai, P. M., Sugimoto, N., Babu, S. S., and Brasseur, G. P.: Aerosol and monsoon climate interactions over Asia, Rev. Geophys., 54, 866–929, https://doi.org/10.1002/2015RG000500, 2016.
Lin, J., An, J., Qu, Y., Chen, Y., Li, Y., Tang, Y., Wang, F., and Xiang, W.: Local and distant source contributions to secondary organic aerosol in the Beijing urban area in summer, Atmos. Environ., 124, 176–185, https://doi.org/10.1016/j.atmosenv.2015.08.098, 2016.
Liu, P. S. K., Deng, R., Smith, K. A., Williams, L. R., Jayne, J. T., Canagaratna, M. R., Moore, K., Onasch, T. B., Worsnop, D. R., and Deshler, T.: Transmission Efficiency of an Aerodynamic Focusing Lens System: Comparison of Model Calculations and Laboratory Measurements for the Aerodyne Aerosol Mass Spectrometer, Aerosol Sci. Technol., 41, 721–733, https://doi.org/10.1080/02786820701422278, 2007.
Lüönd, F., Stetzer, O., Welti, A., and Lohmann, U.: Experimental study on the ice nucleation ability of size-selected kaolinite particles in the immersion mode, J. Geophys. Res.-Atmos., 115, D14201, https://doi.org/10.1029/2009jd012959, 2010.
Mahrt, F., Marcolli, C., David, R. O., Grönquist, P., Barthazy Meier, E. J., Lohmann, U., and Kanji, Z. A.: Ice nucleation abilities of soot particles determined with the Horizontal Ice Nucleation Chamber, Atmos. Chem. Phys., 18, 13363–13392, https://doi.org/10.5194/acp-18-13363-2018, 2018.
Mülmenstädt, J., Sourdeval, O., Delanoë, J., and Quaas, J.: Frequency of occurrence of rain from liquid-, mixed-, and ice-phase clouds derived from A-Train satellite retrievals, Geophys. Res. Lett., 42, 6502–6509, https://doi.org/10.1002/2015GL064604, 2015.
Murray, B. J., Wilson, T. W., Dobbie, S., Cui, Z., Al-Jumur, S. M. R. K., Möhler, O., Schnaiter, M., Wagner, R., Benz, S., Niemand, M., Saathoff, H., Ebert, V., Wagner, S., and Kärcher, B.: Heterogeneous nucleation of ice particles on glassy aerosols under cirrus conditions, Nat. Geosci., 3, 233–237, https://doi.org/10.1038/ngeo817, 2010.
Murray, B. J., O'Sullivan, D., Atkinson, J. D., and Webb, M. E.: Ice nucleation by particles immersed in supercooled cloud droplets, Chem. Soc. Rev., 41, 6519–6554, https://doi.org/10.1039/c2cs35200a, 2012.
Ng, N. L., Herndon, S. C., Trimborn, A., Canagaratna, M. R., Croteau, P. L., Onasch, T. B., Sueper, D., Worsnop, D. R., Zhang, Q., Sun, Y. L., and Jayne, J. T.: An Aerosol Chemical Speciation Monitor (ACSM) for Routine Monitoring of the Composition and Mass Concentrations of Ambient Aerosol, Aerosol Sci. Technol., 45, 780–794, https://doi.org/10.1080/02786826.2011.560211, 2011.
Nichman, L., Wolf, M., Davidovits, P., Onasch, T. B., Zhang, Y., Worsnop, D. R., Bhandari, J., Mazzoleni, C., and Cziczo, D. J.: Laboratory study of the heterogeneous ice nucleation on black-carbon-containing aerosol, Atmos. Chem. Phys., 19, 12175–12194, https://doi.org/10.5194/acp-19-12175-2019, 2019.
Niedermeier, D., Hartmann, S., Clauss, T., Wex, H., Kiselev, A., Sullivan, R. C., DeMott, P. J., Petters, M. D., Reitz, P., Schneider, J., Mikhailov, E., Sierau, B., Stetzer, O., Reimann, B., Bundke, U., Shaw, R. A., Buchholz, A., Mentel, T. F., and Stratmann, F.: Experimental study of the role of physicochemical surface processing on the IN ability of mineral dust particles, Atmos. Chem. Phys., 11, 11131–11144, https://doi.org/10.5194/acp-11-11131-2011, 2011.
Niemand, M., Möhler, O., Vogel, B., Vogel, H., Hoose, C., Connolly, P., Klein, H., Bingemer, H., DeMott, P., Skrotzki, J., and Leisner, T.: A Particle-Surface-Area-Based Parameterization of Immersion Freezing on Desert Dust Particles, J. Atmos. Sci., 69, 3077–3092, https://doi.org/10.1175/JAS-D-11-0249.1, 2012.
Park, S. H., Song, C. B., Kim, M. C., Kwon, S. B., and Lee, K. W.: Study on Size Distribution of Total Aerosol and Water-Soluble Ions During an Asian Dust Storm Event at Jeju Island, Korea, Environ. Monit. Assess., 93, 157–183, https://doi.org/10.1023/B:EMAS.0000016805.04194.56, 2004.
Polen, M., Lawlis, E. and Sullivan, R. C.: The unstable ice nucleation properties of Snomax® bacterial particles, J. Geophys. Res.-Atmos., 121, 11666–11678, https://doi.org/10.1002/2016jd025251, 2016.
Porter, G. C. E., Adams, M. P., Brooks, I. M., Ickes, L., Karlsson, L., Leck, C., Salter, M. E., Schmale, J., Siegel, K., Sikora, S. N. F., Tarn, M. D., Vüllers, J., Wernli, H., Zieger, P., Zinke, J., and Murray, B. J.: Highly active ice-nucleating particles at the summer North Pole, J. Geophys. Res.-Atmos., 127, e2021JD036059, https://doi.org/10.1029/2021JD036059, 2022.
Pruppacher, H. R. and Klett, J. D.: Microphysics of Clouds and Precipitation, 2 edn., Atmospheric and Oceanographic Sciences Library, volume 18, Springer Netherlands, XXII, 954 pp., eBook ISBN 978-0-306-48100-0, https://doi.org/10.1007/978-0-306-48100-0, 1997.
Qiao, K., Wu, Z., Pei, X., Liu, Q., Shang, D., Zheng, J., Du, Z., Zhu, W., Wu, Y., Lou, S., Guo, S., Chan, C. K., Pathak, R. K., Hallquist, M., and Hu, M.: Size-resolved effective density of submicron particles during summertime in the rural atmosphere of Beijing, China, J. Environ. Sci., 73, 69–77, https://doi.org/10.1016/j.jes.2018.01.012, 2018.
Rai, P., Slowik, J. G., Furger, M., El Haddad, I., Visser, S., Tong, Y., Singh, A., Wehrle, G., Kumar, V., Tobler, A. K., Bhattu, D., Wang, L., Ganguly, D., Rastogi, N., Huang, R.-J., Necki, J., Cao, J., Tripathi, S. N., Baltensperger, U., and Prévôt, A. S. H.: Highly time-resolved measurements of element concentrations in PM10 and PM2.5: comparison of Delhi, Beijing, London, and Krakow, Atmos. Chem. Phys., 21, 717–730, https://doi.org/10.5194/acp-21-717-2021, 2021.
Raza, M., Chen, L., Leach, F., and Ding, S.: A Review of Particulate Number (PN) Emissions from Gasoline Direct Injection (GDI) Engines and Their Control Techniques, Energies, 11, 1417, https://doi.org/10.3390/en11061417, 2018.
Reicher, N., Budke, C., Eickhoff, L., Raveh-Rubin, S., Kaplan-Ashiri, I., Koop, T., and Rudich, Y.: Size-dependent ice nucleation by airborne particles during dust events in the eastern Mediterranean, Atmos. Chem. Phys., 19, 11143–11158, https://doi.org/10.5194/acp-19-11143-2019, 2019.
Rogers, D. C., DeMott, P. J., Kreidenweis, S. M., and Chen, Y.: Measurements of ice nucleating aerosols during SUCCESS, Geophys. Res. Lett., 25, 1383–1386, https://doi.org/10.1029/97GL03478, 1998.
Schill, G. P., Jathar, S. H., Kodros, J. K., Levin, E. J. T., Galang, A. M., Friedman, B., Link, M. F., Farmer, D. K., Pierce, J. R., Kreidenweis, S. M., and DeMott, P. J.: Ice-nucleating particle emissions from photochemically aged diesel and biodiesel exhaust, Geophys. Res. Lett., 43, 5524–5531, https://doi.org/10.1002/2016GL069529, 2016.
Schill, G. P., DeMott, P. J., Emerson, E. W., Rauker, A. M. C., Kodros, J. K., Suski, K. J., Hill, T. C. J., Levin, E. J. T., Pierce, J. R., Farmer, D. K., and Kreidenweis, S. M.: The contribution of black carbon to global ice nucleating particle concentrations relevant to mixed-phase clouds, P. Natl. Acad. Sci. USA, 117, 22705–22711, https://doi.org/10.1073/pnas.2001674117, 2020.
Seinfeld, J. H. and Pandis, S. N.: Atmospheric chemistry and physics: from air pollution to climate change, 3rd edn., John Wiley & Sons, ISBN 1118947401, 2016.
Silber, I., Fridlind, A. M., Verlinde, J., Ackerman, A. S., Cesana, G. V., and Knopf, D. A.: The prevalence of precipitation from polar supercooled clouds, Atmos. Chem. Phys., 21, 3949–3971, https://doi.org/10.5194/acp-21-3949-2021, 2021.
Sun, Y., Du, W., Fu, P., Wang, Q., Li, J., Ge, X., Zhang, Q., Zhu, C., Ren, L., Xu, W., Zhao, J., Han, T., Worsnop, D. R., and Wang, Z.: Primary and secondary aerosols in Beijing in winter: sources, variations and processes, Atmos. Chem. Phys., 16, 8309–8329, https://doi.org/10.5194/acp-16-8309-2016, 2016.
Tang, M., Cziczo, D. J., and Grassian, V. H.: Interactions of Water with Mineral Dust Aerosol: Water Adsorption, Hygroscopicity, Cloud Condensation, and Ice Nucleation, Chem. Rev., 116, 4205–4259, https://doi.org/10.1021/acs.chemrev.5b00529, 2016.
Tobo, Y., Zhang, D., Matsuki, A., and Iwasaka, Y.: Asian dust particles converted into aqueous droplets under remote marine atmospheric conditions, P. Natl. Acad. Sci. USA, 107, 17905–17910, https://doi.org/10.1073/pnas.1008235107, 2010.
Tobo, Y., DeMott, P. J., Raddatz, M., Niedermeier, D., Hartmann, S., Kreidenweis, S. M., Stratmann, F., and Wex, H.: Impacts of chemical reactivity on ice nucleation of kaolinite particles: A case study of levoglucosan and sulfuric acid, Geophys. Res. Lett., 39, L19803, https://doi.org/10.1029/2012GL053007, 2012.
Ullrich, R., Hoose, C., Möhler, O., Niemand, M., Wagner, R., Höhler, K., Hiranuma, N., Saathoff, H., and Leisner, T.: A New Ice Nucleation Active Site Parameterization for Desert Dust and Soot, J. Atmos. Sci., 74, 699–717, https://doi.org/10.1175/jas-d-16-0074.1, 2017.
Vali, G., DeMott, P. J., Möhler, O., and Whale, T. F.: Technical Note: A proposal for ice nucleation terminology, Atmos. Chem. Phys., 15, 10263–10270, https://doi.org/10.5194/acp-15-10263-2015, 2015.
Wang, G. H., Cheng, C. L., Huang, Y., Tao, J., Ren, Y. Q., Wu, F., Meng, J. J., Li, J. J., Cheng, Y. T., Cao, J. J., Liu, S. X., Zhang, T., Zhang, R., and Chen, Y. B.: Evolution of aerosol chemistry in Xi'an, inland China, during the dust storm period of 2013 – Part 1: Sources, chemical forms and formation mechanisms of nitrate and sulfate, Atmos. Chem. Phys., 14, 11571–11585, https://doi.org/10.5194/acp-14-11571-2014, 2014.
Wang, Y., Wu, Z., Ma, N., Wu, Y., Zeng, L., Zhao, C., and Wiedensohler, A.: Statistical analysis and parameterization of the hygroscopic growth of the sub-micrometer urban background aerosol in Beijing, Atmos. Environ., 175, 184–191, https://doi.org/10.1016/j.atmosenv.2017.12.003, 2018.
Wegener, A.: Thermodynamik der Atnwsphäre, J. A. Barth, Leipzig, 331 pp., 1911.
Welti, A., Lüönd, F., Stetzer, O., and Lohmann, U.: Influence of particle size on the ice nucleating ability of mineral dusts, Atmos. Chem. Phys., 9, 6705–6715, https://doi.org/10.5194/acp-9-6705-2009, 2009.
Wex, H., DeMott, P. J., Tobo, Y., Hartmann, S., Rösch, M., Clauss, T., Tomsche, L., Niedermeier, D., and Stratmann, F.: Kaolinite particles as ice nuclei: learning from the use of different kaolinite samples and different coatings, Atmos. Chem. Phys., 14, 5529–5546, https://doi.org/10.5194/acp-14-5529-2014, 2014.
Whale, T. F., Holden, M. A., Wilson, Theodore W., O'Sullivan, D., and Murray, B. J.: The enhancement and suppression of immersion mode heterogeneous ice-nucleation by solutes, Chem. Sci., 9, 4142–4151, https://doi.org/10.1039/C7SC05421A, 2018.
Wolf, M. J., Goodell, M., Dong, E., Dove, L. A., Zhang, C., Franco, L. J., Shen, C., Rutkowski, E. G., Narducci, D. N., Mullen, S., Babbin, A. R., and Cziczo, D. J.: A link between the ice nucleation activity and the biogeochemistry of seawater, Atmos. Chem. Phys., 20, 15341–15356, https://doi.org/10.5194/acp-20-15341-2020, 2020.
Wu, C., Zhang, S., Wang, G., Lv, S., Li, D., Liu, L., Li, J., Liu, S., Du, W., Meng, J., Qiao, L., Zhou, M., Huang, C., and Wang, H.: Efficient Heterogeneous Formation of Ammonium Nitrate on the Saline Mineral Particle Surface in the Atmosphere of East Asia during Dust Storm Periods, Environ. Sci. Technol., 54, 15622–15630, https://doi.org/10.1021/acs.est.0c04544, 2020.
Wu, Z., Chen, J., Wang, Y., Zhu, Y., Liu, Y., Yao, B., Zhang, Y., and Hu, M.: Interactions between water vapor and atmospheric aerosols have key roles in air quality and climate change, Natl. Sci. Rev., 5, 452–454, https://doi.org/10.1093/nsr/nwy063, 2018.
Zhang, C., Zhang, Y., Wolf, M. J., Nichman, L., Shen, C., Onasch, T. B., Chen, L., and Cziczo, D. J.: The effects of morphology, mobility size, and secondary organic aerosol (SOA) material coating on the ice nucleation activity of black carbon in the cirrus regime, Atmos. Chem. Phys., 20, 13957–13984, https://doi.org/10.5194/acp-20-13957-2020, 2020.
Zhang, S., Wu, Y., Yan, H., Du, X., Max Zhang, K., Zheng, X., Fu, L., and Hao, J.: Black carbon pollution for a major road in Beijing: Implications for policy interventions of the heavy-duty truck fleet, Transport. Res. D-Tr. E., 68, 110–121, https://doi.org/10.1016/j.trd.2017.07.013, 2019.
Zhang, X., Zhang, K., Liu, H., Lv, W., Aikawa, M., Liu, B., and Wang, J.: Pollution sources of atmospheric fine particles and secondary aerosol characteristics in Beijing, J. Environ. Sci., 95, 91–98, https://doi.org/10.1016/j.jes.2020.04.002, 2020.
Zhao, B., Wang, Y., Gu, Y., Liou, K. N., Jiang, J. H., Fan, J., Liu, X., Huang, L., and Yung, Y. L.: Ice nucleation by aerosols from anthropogenic pollution, Nat. Geosci., 12, 602–607, https://doi.org/10.1038/s41561-019-0389-4, 2019.
- Abstract
- Introduction
- Methods
- Results and discussion
- Conclusion
- Appendix A: Ambient aerosol characterization and back trajectory analysis of the dust event
- Appendix B: HINC lamina condition calibration
- Appendix C: Additional information
- Data availability
- Author contributions
- Competing interests
- Disclaimer
- Acknowledgements
- Financial support
- Review statement
- References
- Abstract
- Introduction
- Methods
- Results and discussion
- Conclusion
- Appendix A: Ambient aerosol characterization and back trajectory analysis of the dust event
- Appendix B: HINC lamina condition calibration
- Appendix C: Additional information
- Data availability
- Author contributions
- Competing interests
- Disclaimer
- Acknowledgements
- Financial support
- Review statement
- References