the Creative Commons Attribution 4.0 License.
the Creative Commons Attribution 4.0 License.
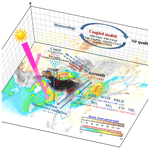
Two-way coupled meteorology and air quality models in Asia: a systematic review and meta-analysis of impacts of aerosol feedbacks on meteorology and air quality
Chao Gao
Aijun Xiu
Xuelei Zhang
Qingqing Tong
Hongmei Zhao
Shichun Zhang
Guangyi Yang
Mengduo Zhang
Atmospheric aerosols can exert an influence on meteorology and air quality through aerosol–radiation interaction (ARI) and aerosol–cloud interaction (ACI), and this two-way feedback has been studied by applying two-way coupled meteorology and air quality models. As one of the regions with the highest aerosol loading in the world, Asia has attracted many researchers to investigate the aerosol effects with several two-way coupled models (WRF-Chem, WRF-CMAQ, GRAPES-CUACE, WRF-NAQPMS, and GATOR-GCMOM) over the last decade. This paper attempts to offer a bibliographic analysis regarding the current status of applications of two-way coupled models in Asia, related research focuses, model performances, and the effects of ARI and/or ACI on meteorology and air quality. There were a total of 160 peer-reviewed articles published between 2010 and 2019 in Asia meeting the inclusion criteria, with more than 79 % of papers involving the WRF-Chem model. The number of relevant publications has an upward trend annually, and East Asia, India, and China, as well as the North China Plain are the most studied areas. The effects of ARI and both ARI and ACI induced by natural aerosols (particularly mineral dust) and anthropogenic aerosols (bulk aerosols, different chemical compositions, and aerosols from different sources) are widely investigated in Asia. Through the meta-analysis of surface meteorological and air quality variables simulated by two-way coupled models, the model performance affected by aerosol feedbacks depends on different variables, simulation time lengths, selection of two-way coupled models, and study areas. Future research perspectives with respect to the development, improvement, application, and evaluation of two-way coupled meteorology and air quality models are proposed.
- Article
(6068 KB) -
Supplement
(630 KB) - BibTeX
- EndNote
Atmospheric pollutants can affect local weather and global climate via many mechanisms, as extensively summarized in the Intergovernmental Panel on Climate Change (IPCC) reports (IPCC, 2007, 2013, 2021), and also exhibit impacts on human health and ecosystems (Lelieveld et al., 2015; Wu and Zhang, 2018). Atmospheric pollutants can modify the radiation energy balance, thus influencing meteorological conditions (Gray et al., 2010; Yiğit et al., 2016). Compared to other climate agents, short-lived and localized aerosols could induce changes in meteorology and climate through aerosol–radiation interaction (ARI; Tremback et al., 1986; Satheesh and Moorthy, 2005) and aerosol–cloud interaction (ACI; Martin and Leight, 1949; Lohmann and Feichter, 2005) or both (Sud and Walker, 1990; Haywood and Boucher, 2000). ARI (previously known as the direct effect and semi-direct effect) is based on scattering and absorbing solar radiation by aerosols as well as cloud dissipation by heating (McCormick and Ludwig, 1967; Ackerman et al., 2000; Koch and Del Genio, 2010; Wilcox, 2012). ACI (known as the indirect effect) is concerned with aerosols altering albedo and lifetime of clouds (Twomey, 1977; Albrecht, 1989; Lohmann and Feichter, 2005). As our knowledge base of aerosol–radiation–cloud interactions that involve extremely complex physical and chemical processes has been expanding, accurately assessing the effects of these interactions still remains a big challenge (Rosenfeld et al., 2008, 2019; Fan et al., 2016; Kuniyal and Guleria, 2019).
The interactions between air pollutants and meteorology can be investigated by observational analyses and/or air quality models. So far, many observational studies using measurement data from a variety of sources have been conducted to analyze these interactions (Wendisch et al., 2002; Bellouin et al., 2008; Groß et al., 2013; Rosenfeld et al., 2019). Yu et al. (2006) reviewed research work that adopted satellite and ground-based measurements to estimate the ARI-induced changes in radiative forcing and the associated uncertainties in the analysis. Yoon et al. (2019) analyzed the effects of aerosols on the radiative forcing based on the Aerosol Robotic Network observations and demonstrated that these effects depend on aerosol types. On the other hand, since the uncertainties in ARI estimations have been associated with ACI (Kuniyal and Guleria, 2019), simultaneous assessments of both ARI and ACI effects are needed and have gradually been conducted via satellite observations (Sekiguchi et al., 2003; Quaas et al., 2008; Illingworth et al., 2015; Kant et al., 2019). In the early stages, observational studies of ACI effects were based on several cloud parameters mainly derived from surface-based microwave radiometer (Kim et al., 2003; Liu et al., 2003) and cloud radar (Feingold et al., 2003; Penner et al., 2004). Later on, with the further development of satellite observation technology and enhanced spatial resolution of satellite measurement compared against traditional ground observations, satellite-retrieved cloud parameters (effective cloud droplet radius, liquid water path – LWP, and cloud cover) were utilized to identify the ACI effects studies on a cloud scale (Goren and Rosenfeld, 2014; Rosenfeld et al., 2014). Moreover, in order to clarify whether aerosols affect precipitation positively or negatively, the effects of ACI on cloud properties and precipitation were widely investigated but with various answers (Andreae and Rosenfeld, 2008; Rosenfeld et al., 2014; Casazza et al., 2018; Fan et al., 2018). Analyses of satellite and/or ground observations revealed that increased aerosols could suppress (enhance) precipitation in drier (wetter) environments (Rosenfeld, 2000; Rosenfeld et al., 2008; Z. Li et al., 2011; Donat et al., 2016). Most recently, Rosenfeld et al. (2019) further used satellite-derived cloud information (droplet concentration and updraft velocity at cloud base, LWP at cloud cores, cloud geometrical thickness, and cloud fraction) to single out ACI under a certain meteorological condition and found that the cloudiness change caused by aerosol in marine low-level clouds was much greater than previous analyses (Sato and Suzuki, 2019). Despite the fact that the aforementioned studies significantly improved our understanding of aerosol effects, many limitations still exist, such as low temporal resolution of satellite data, low spatial resolution of ground monitoring sites, and lack of vertical distribution information on aerosol and cloud (Yu et al., 2006; Rosenfeld et al., 2014; Sato and Suzuki, 2019).
Numerical models can also be used to study the interactions between air pollutants and meteorology. Air quality models simulate physical and chemical processes in the atmosphere (ATM) and are classified as offline and online models (El-Harbawi, 2013). Offline models (also known as traditional air quality models) require outputs from meteorological models to subsequently drive chemical models (Seaman, 2000; Byun and Schere, 2006; Ramboll Environment and Health, 2008). Compared to online models, offline models are usually computationally efficient but incapable of capturing two-way feedbacks between chemistry and meteorology (North et al., 2014). Online models or coupled models are designed and developed to consider the two-way feedbacks and have attempted to accurately simulate both meteorology and air quality (Grell et al., 2005; Wong et al., 2012; Briant et al., 2017). Two-way coupled models can be generally categorized as integrated and access models based on whether they use a coupler to exchange variables between meteorological and chemical modules (Baklanov et al., 2014). As Zhang (2008) pointed out, Jacobson (1994, 1997a) and Jacobson et al. (1996a) pioneered the development of a fully coupled model named the Gas, Aerosol, Transport, Radiation, General Circulation, Mesoscale, and Ocean Model (GATOR-GCMOM) in order to investigate all the processes related to ARI and ACI. Currently, there are three representative two-way coupled meteorology and air quality models, namely the Weather Research and Forecasting-Chemistry (WRF-Chem) (Grell et al., 2005), WRF coupled with Community Multiscale Air Quality (CMAQ) (Wong et al., 2012), and WRF coupled with a multi-scale chemistry-transport model for atmospheric composition analysis and forecast (WRF-CHIMERE) (Briant et al., 2017). WRF-Chem is an integrated model that includes various chemical modules in the meteorological model (i.e., WRF) without using a coupler. For the remaining two models, which are access models, WRF-CMAQ uses a subroutine called aqprep (Wong et al., 2012) as its coupler, while WRF-CHIMERE uses general coupling software named the Ocean Atmosphere Sea Ice Soil–Model Coupling Toolkit (Craig et al., 2017). With more growing interest in coupled models and their developments, applications, and evaluations, two review papers thoroughly summarized the related works published before 2008 (Zhang, 2008) and 2014 (Baklanov et al., 2014). Zhang (2008) provided an overview of the developments and applications of five coupled models in the United States (US) and the treatments of chemical and physical processes in these coupled models with an emphasis on ACI-related processes. Another paper presented a systematic review of the similarities and differences of 18 integrated or access models in Europe and discussed the descriptions of interactions between meteorological and chemical processes in these models as well as the model evaluation methodologies involved (Baklanov et al., 2014). Some of these coupled models can be used to investigate the interactions between air quality and meteorology at regional scales but also at global and hemispheric scales (Jacobson, 2001; Grell et al., 2011; Xing et al., 2015a; Mailler et al., 2017); large-scale studies were not included in the two review papers by Zhang (2008) and Baklanov et al. (2014). These reviews only focused on application and evaluation of coupled models in the US and Europe, but there is still no systematic review targeting two-way coupled model applications in Asia.
Compared to the US and Europe, Asia has been suffering from more severe air pollution in the past 3 decades (Bollasina et al., 2011; Rohde and Muller, 2015; Gurjar et al., 2016) due to the rapid industrialization, urbanization, and population growth together with unfavorable meteorological conditions (Jeong and Park, 2017; Li et al., 2017a; Lelieveld et al., 2018). The interactions between atmospheric pollution and meteorology in Asia, which have received a lot of attention from the scientific community, are investigated using extensive observations and a certain number of numerical simulations (Wang et al., 2010; Li et al., 2016; Nguyen et al., 2019a). Based on airborne, ground-based, and satellite-based observations, multiple important experiments have been carried out to analyze properties of radiation, cloud, and aerosols in Asia, as briefly reviewed by Lin et al. (2014b). Recent observational studies confirmed that increasing aerosol loadings play important roles in the radiation budget (Eck et al., 2018; Benas et al., 2020), cloud properties (Dahutia et al., 2019; Yang et al., 2019), and precipitation intensity along with vertical distributions of precipitation types (Guo et al., 2014, 2018). According to previous observational studies in Southeast Asia (SEA), Tsay et al. (2013) and Lin et al. (2014b) comprehensively summarized the spatiotemporal characteristics of biomass burning (BB) aerosols and clouds as well as their interactions. Li et al. (2016) analyzed how ARI or ACI influenced climate and meteorology in Asia utilizing observations and climate models. With regard to the impacts of aerosols on cloud, precipitation, and climate in East Asia (EA), a detailed review of observations and modeling simulations has also been presented by Z. Li et al. (2019). Since the 2000s, substantial progress has been made in climate–air pollution interactions in Asia based on regional climate model simulations, which have been summarized by Li et al. (2016). Moreover, starting from the year 2010, with the development and availability of two-way coupled meteorology and air quality models, more and more modeling studies have been conducted to explore the ARI and/or ACI effects in Asia (H. Wang et al., 2010; J. Wang et al., 2014; Sekiguchi et al., 2018; Nguyen et al., 2019a). In recent studies, a series of WRF-Chem and WRF-CMAQ simulations were performed to assess the consequences of ARI for radiative forcing, planetary boundary layer height (PBLH), precipitation, and fine particulate matter (PM2.5) and ozone concentrations (J. Wang et al., 2014; Huang et al., 2016; Sekiguchi et al., 2018; Nguyen et al., 2019b). Different from the currently released version of WRF-CMAQ (based on WRF version 4.3 and CMAQ version 5.3.3) that only includes ARI, WRF-Chem with ACI (starting from WRF-Chem version 3.0; Chapman et al., 2009) has been implemented for analyzing the complicated aerosol effects that lead to variations of cloud properties, precipitation, and PM2.5 concentrations (Zhao et al., 2017; Z. Liu et al., 2018; Park et al., 2018; Bai et al., 2020). To quantify the individual or joint effects of ARI and/or ACI on meteorological variables and pollutant concentrations, several modeling studies have been performed in Asia (B. Zhang et al., 2015; X. Zhang et al., 2018; Ma et al., 2016; Chen et al., 2019b). In addition, model comparisons (including offline and online models) targeting EA have been carried out recently under the Model Inter-Comparison Study for Asia (MICS-Asia) Phase III (M. Gao et al., 2018a; Chen et al., 2019a; J. Li et al., 2019). As mentioned above, even though there are already several reviews regarding observational studies of ARI and/or ACI (Tsay et al., 2013; N.-H. Lin et al., 2014; Z. Li et al., 2016, 2019) it is necessary to conduct a systematic review in Asia focusing on applications of two-way coupled meteorology and air quality models as well as simulated variations of meteorology and air quality induced by aerosol effects.
This paper is constructed as follows: Sect. 2 describes the methodology for literature searching, paper inclusion, and analysis; Sect. 3 summarizes the basic information about publications as well as developments and applications of coupled models in Asia, and Sect. 4 provides the recent overviews of their research points. Sections 5 to 6 present a systematic review and meta-analysis of the effects of aerosol feedbacks on model performance, meteorology, and air quality in Asia. The summary and perspective are provided in Sect. 7.
2.1 Criteria and synthesis
Since 2010, in Asia, regional studies of aerosol effects on meteorology and air quality based on coupled models have been increasing gradually; therefore, in this study we performed a systematic search of the literature to identify relevant studies from 1 January 2010 to 31 December 2019. In order to find all the relevant papers in English, Chinese, Japanese, and Korean, we deployed serval science-based search engines, including Google Scholar, the Web of Science, the China National Knowledge Infrastructure, the Japan Information Platform for S&T Innovation, and the Korean Studies Information Service System. The different keywords and their combinations for paper searching are as follows: (1) model-related keywords including “coupled model”, “two-way”, “WRF”, “NU-WRF”, “WRF-Chem”, “CMAQ”, “WRF-CMAQ”, “CAMx”, “CHIMERE”, “WRF-CHIMERE” and “GATOR-GCMOM”; (2) effect-related keywords including “aerosol radiation interaction”, “ARI”, “aerosol cloud interaction”, “ACI”, “aerosol effect”, and “aerosol feedback”; (3) air-pollution-related keywords including “air quality”, “aerosol”, “PM2.5”, “O3”, “CO”, “SO2”, “NO2”, “dust”, “BC”, “black carbon”, “blown carbon”, “carbonaceous”, and “primary pollutants”; (4) meteorology-related keywords including “meteorology”, “radiation”, “wind”, “temperature”, “specific humidity”, “relative humidity”, “planetary boundary layer”, “cloud”, and “precipitation”; (5) region-related keywords including “Asia”, “East Asia”, “Northeast Asia”, “South Asia”, “Southeast Asia”, “Far East”, “China”, “India”, “Japan”, “Korea”, “Singapore”, “Thailand”, “Malaysia”, “Nepal”, “North China Plain”, “Yangtze River Delta”, “Pearl River Delta”, “middle reaches of the Yangtze River”, “Sichuan Basin”, “Guanzhong Plain”, “Northeast China”, “Northwest China” “East China”, “Tibet Plateau”, “Taiwan”, “northern India”, “southern India”, “Gangetic Basin”, and “Kathmandu Valley”.
After applying the search engines and the keyword combinations mentioned above, we found 946 relevant papers. In order to identify which papers should be included or excluded in this paper, the following criteria were applied: (1) duplicate literature was deleted; (2) studies using coupled models in Asia with aerosol feedbacks turned on were included, and observational studies of aerosol effects were excluded; (3) publications involving coupled climate models were excluded. According to these criteria, not only regional studies, but also studies using the coupled models at global or hemispheric scales involving Asia or its subregions were included. Then, we carefully examined all the included papers and further checked the listed references in each paper to make sure that no related paper was neglected. A flowchart that illustrates the detailed procedures applied for article identification is presented in Fig. A1 (note: although the deadline for literature searching is 2019, any literature published in 2020 is also included). There were a total of 160 publications included in our study.
2.2 Analysis method
To summarize the current status of coupled models applied in Asia and quantitatively analyze the effects of aerosol feedbacks on model performance as well as meteorology and air quality, we carried out a series of analyses based on data extracted from the selected papers. We firstly compiled the publication information from the included papers as well as the information regarding model name, simulated time period, study region, simulation design, and aerosol effects. Secondly, we summarized the important findings of two-way coupled model applications in Asia according to different aerosol sources and components to clearly determine the major research focuses in past studies. Finally, we gathered all the simulated results of meteorological and air quality variables with and without aerosol effects and their statistical indices (SIs). For questionable results, quality assurance was conducted after personal communications with the original authors to decide whether they were deleted and/or corrected. All the extracted publication and statistical information was exported into an Excel file, which is provided in Table S1. Moreover, we performed quantitative analyses of the effects of aerosol feedbacks through the following steps. (1) We discussed whether meteorological and air quality variables were overestimated or underestimated based on their SIs. Then, variations of the SIs of these variables were further analyzed in detail with and without turning on ARI and/or ACI in two-way coupled models. (2) We investigated the SIs of simulation results at different simulation time lengths and spatial resolutions in coupled models. (3) More detailed inter-model comparisons of model performance based on the compiled SIs among different coupled models are conducted. (4) Differences in simulation results with and without aerosol feedbacks were grouped by study regions and timescales (yearly, seasonal, monthly, daily, and hourly). Toward a better understanding of the complicated interactions between air quality and meteorology in Asia, the results sections in this paper are organized following the above analysis methods (1)–(3) and presented in Sect. 5, and the results following method (4) are presented in Sect. 6. In addition, Excel and Python were used to conduct data processing and plotting in this study.
3.1 Summary of applications of coupled models in Asia
A total of 160 articles were selected according to the inclusion criteria, and their basic information was compiled in Table 1. In Asia, five two-way coupled models are applied to study the ARI and ACI effects. These include GATOR-GCMOM, two commonly used models, i.e., WRF-Chem and WRF-CMAQ, and two locally developed models, i.e., the global–regional assimilation and prediction system coupled with the Chinese Unified Atmospheric Chemistry Environment forecasting system (GRAPES-CUACE) and WRF coupled with the nested air quality prediction modeling system (WRF-NAQPMS). A total of 127 out of 160 papers involved the applications of WRF-Chem in Asia since its two-way coupled version was publicly available in 2006 (Fast et al., 2006). WRF-CMAQ was applied in only 16 studies due to its later initial release in 2012 (Wong et al., 2012). GRAPES-CUACE was developed by the China Meteorological Administration and introduced in detail in Zhou et al. (2008, 2012, 2016), then firstly utilized in Wang et al. (2010) to estimate impacts of aerosol feedbacks on meteorology and the dust cycle in EA. The coupled version of WRF-NAQPMS was developed by the Institute of Atmospheric Physics, Chinese Academy of Sciences, and improved the prediction accuracy of haze pollution in the North China Plain (NCP) (Z. Wang et al., 2014). Note that GRAPES-CUACE and WRF-NAQPMS were only applied in China. There were only three published papers about the applications of GATOR-GCMOM in northeastern Asia (NEA), NCP, and India. In the included papers, 93, 33, and 31 studies targeted various areas in China, EA, and India, respectively. There were 79 papers regarding effects of ARI (7 health), 63 for both ARI and ACI (1 health), and 18 for ACI. ACI studies were much fewer than ARI-related ones, which indicated that ACI-related studies need to be paid more attention in the future. Considering that the choices of cloud microphysics and radiation schemes can affect coupled models' results (Baró et al., 2015; Jimenez et al., 2016), the schemes used in the selected studies are also summarized in Table 1. This table presents a concise overview of coupled models' applications in Asia with the purpose of providing basic information regarding models, study periods and areas, aerosol effects, scheme selections, and references. More complete information is summarized in Table S1 including model version, horizontal resolution, vertical layer, aerosol- and gas-phase chemical mechanisms, photolysis rate, PBL, land surface, surface layer, cumulus, urban canopy schemes, meteorological initial and boundary conditions (ICs and BCs), chemical ICs and BCs, spin-up time, and anthropogenic and natural emissions.
It should be noted that in Table 1 there are four model intercomparison studies that aimed at evaluating model performance, identifying error sources and uncertainties, and providing optimal model setups. By comparing simulations from two coupled models (WRF-Chem and Spectral Radiation-Transport Model for Aerosol Species) (Takemura et al., 2003) in India (Govardhan et al., 2016), it was found that the spatial distributions of various aerosol species (black carbon –BC, mineral dust, and sea salt) were similar with the two models. Based on the intercomparisons of WRF-Chem simulations in different areas, Yang et al. (2017) revealed that aerosol feedbacks could enhance PM2.5 concentrations in the Indo-Gangetic Plain but suppress the concentrations in the Tibetan Plateau (TP). Targeting China and India, M. Gao et al. (2018b) also applied the WRF-Chem model to quantify the contributions of different emission sectors to aerosol radiative forcings, suggesting that reducing the uncertainties in emission inventories was critical, especially for India. Moreover, for the NCP region, M. Gao et al. (2018a) presented a comparison study with multiple online models under the MICS-Asia Phase III and pointed out noticeable discrepancies in the simulated secondary inorganic aerosols under heavy haze conditions and the importance of accurate predictions of wind speed at 10 m above the surface (WS10) by these models. Comprehensive comparative studies for Asia have been emerging lately but are still limited compared to those for North America and Europe, such as the Air Quality Model Evaluation International Initiative Phase II (Brunner et al., 2015; Campbell et al., 2015; Im et al., 2015a, b; Kong et al., 2015; Makar et al., 2015a, b; K. Wang et al., 2015; Forkel et al., 2016).
Table 1Basic information on coupled model applications in Asia during 2010–2019.

† Unclear; * a preprint version of this study was available online on 31 October 2019 and was formally published on 1 January 2020. (EA: East Asia, NEA: northeastern Asia, SEA: Southeast Asia, EC: eastern China, NCP: North China Plain, YRD: Yangtze River Delta, SEC: southeastern China, NWC: northwestern China, TP: Tibetan Plateau, MRYR: middle reaches of the Yangtze River, SWC: southwestern China; PRD: Pearl River Delta).
3.2 Spatiotemporal distribution of publications
To gain an overall understanding of applications of coupled models in Asia, the spatial distributions of study areas from the selected literature and the temporal variations of the annual publication numbers were extracted from Table 1 and summarized. Figure 1 illustrates the spatial distributions of study regions as well as the number of papers involving coupled models in Asia (Fig. 1a) and China (Fig. 1b). In this figure, the color and number in the pie charts represent individual (WRF-Chem, WRF-CMAQ, GRAPES-CUACE, WRF-NAQPMS, and GATOR-GCMOM) or multiple coupled models and the quantity of corresponding articles, respectively. At subregional scales, most studies targeted EA where high anthropogenic aerosol loading occurred in recent decades, mainly using WRF-Chem and WRF-CMAQ (Fig. 1a). For other subregions, such as NEA, SEA, central Asia (CA), and western Asia (WA), there were rather limited research activities taking into account aerosol feedbacks with two-way coupled models. National-scale applications of two-way coupled models targeted mostly modeling domains covering India and China, but much less work has been carried out in other countries, such as Japan and Korea, where air pollution levels are much lower. With respect to various areas in China (Fig. 1b), the research activities concentrated mostly in NCP and secondly in eastern China (EC), then in the Yangtze River Delta (YRD) and Pearl River Delta (PRD) areas. WRF-Chem was the most popular model applied in all areas, but there were a few applications of GPRAPES-CUACE and WRF-NAQPMS in EC and NCP.
Figure 2 depicts the temporal variations of research activities with two-way coupled models in Asia over the period of 2010 to 2019. The total number of papers related to two-way coupled models had an obvious upward trend in the past decade. Prior to 2014, applications of two-way coupled models in Asia were scarce, with about one to six publications per year. A noticeable increase in research activities emerged starting from 2014, and the growth was rapid from 2014 to 2016 at a rate of seven to nine more papers per year, especially in China. It could be related to the Action Plan on Prevention and Control of Atmospheric Pollution (2013–2017) implemented by the Chinese government. The growth was rather flat during 2016–2018 before reaching a peak of 31 articles in 2019. In addition, the pie charts in Fig. 2 indicate that modeling activities had been picking up with a diversified pattern in the study domain from 2010 to 2019. The modeling domains extended from EA to China and India and then several subregions in Asia and various areas in China. For EA and India, investigations of aerosol feedbacks based on two-way coupled models rose from one to two papers per year during 2010–2013 to four to eight during 2014–2019. Since 2014, most model simulations were carried out with a focus on areas with severe air pollution in China, especially the NCP area with five to seven publications per year.
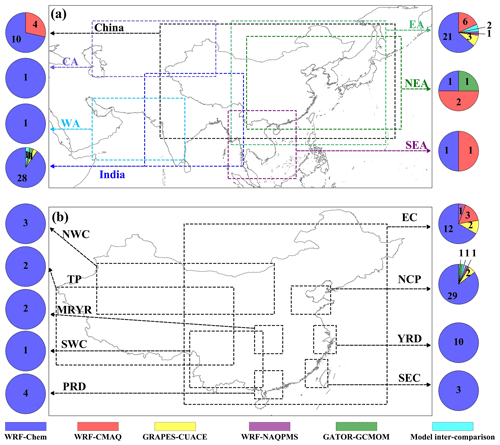
Figure 1The spatial distributions of study domains as well as the two-way coupled modeling publication numbers in different subregions or countries of Asia (a) and areas of China (b). (EA: East Asia, NEA: northeastern Asia, SEA: Southeast Asia, EC: eastern China, NCP: North China Plain, YRD: Yangtze River Delta, SEC: southeastern China, NWC: northwestern China, TP: Tibetan Plateau, MRYR: middle reaches of the Yangtze River, SWC: southwestern China; PRD: Pearl River Delta).
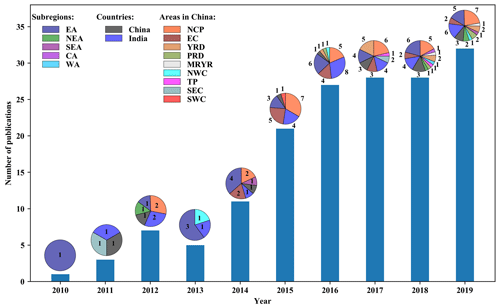
Figure 2The temporal variations of study activities adopting two-way coupled models in Asia during 2010–2019. (EA: East Asia, NEA: northeastern Asia, SEA: Southeast Asia, EC: eastern China, NCP: North China Plain, YRD: Yangtze River Delta, SEC: southeastern China, NWC: northwestern China, TP: Tibetan Plateau, MRYR: middle reaches of the Yangtze River, SWC: southwestern China; PRD: Pearl River Delta).
3.3 Summary of modeling methodologies
The physiochemical processes involved with ARI and ACI are sophisticated in actual conditions of the atmospheric environment, but their representations in two-way coupled models can be rather different. Also, simulation results depend on how these models are configured and set up. Therefore, the treatments of aerosol and cloud microphysics, aerosol–radiation–cloud interactions in WRF-Chem, WRF-CMAQ, GRAPES-CUACE, WRF-NAQPMS, and GATOR-GCMOM applied in Asia, and the various aspects of how the modeling studies are set up in the selected papers are summarized in Tables 2–5, respectively, and outlined in this section.
Aerosol microphysics processes consist of particle nucleation, coagulation, condensation and evaporation, gas–particle mass transfer, inorganic aerosol thermodynamic equilibrium, aqueous chemistry, and formation of secondary organic aerosol (SOA). Their representations in a variety of aerosol mechanisms offered in the five two-way coupled models applied in Asia and relevant references are compiled in Table 2. Note that the GOCART scheme in WRF-Chem is based on a bulk aerosol mechanism that is not able to consider the details of these microphysics processes. The binary homogeneous nucleation schemes with and without hydration developed by different authors are applied in the five coupled models for simulating new particle formation, and GATOR-GCMOM also adopts the ternary nucleation parameterization scheme for H2SO4, NH3, and H2O vapors. All five coupled models calculate the aerosol–aerosol coagulation rate coefficients based on the Brownian coagulation theory, with certain enhancements in GATOR-GCMOM as stated in detail by Jacobson (1999). The dynamic condensation–evaporation approaches of inorganic gases (e.g., H2SO4, NH3, HNO3, and HCl) and organic gases (VOCs) based on the Fuchs–Sutugin expression are implemented in various aerosol mechanisms offered by WRF-Chem, WRF-CMAQ, GRAPES-CUACE, and WRF-NAQPMS, while GATOR-GCMOM deploys the condensation–evaporation approach in which several terms of processes are factored in the 3-D equations of discrete size-resolved aerosol growth (Jacobson, 2012a). The mass transfer between gaseous and aerosol particles is treated via two typical methods (i.e., bulk equilibrium and kinetic) in most coupled models, and the hybrid and Henry's law equilibrium methods are also applied in the MADRID (WRF-Chem) and the sixth- and seventh-generation CMAQ aerosol modules (AERO6/AERO7) (WRF-CMAQ), respectively. Different versions of the ISORROPIA module, the Model for an Aerosol Reacting System-version A (MARS-A), the Multicomponent Equilibrium Solver for Aerosols with the Multicomponent Taylor Expansion Method (MESA-MTEM), and the EQUIlibrium SOLVer version 2 (EQUISOLV II) modules are implemented for computing the inorganic aerosol thermodynamic equilibrium in these two-way coupled models. For aqueous chemistry, the bulk aqueous chemistry scheme and variations of CMAQ's standard aqueous chemistry module (AQCHEM) are the most applied, and the CBM-IV aqueous chemistry scheme, the Regional Acid Deposition Model (RADM) aqueous chemistry module, and the size-resolved aqueous chemistry module are utilized as well. Multiple approaches have been incorporated into the five coupled models for calculating SOA formation and include the volatility basis set (VBS) approach, approaches considering reversible absorption or combined absorption and dissolution, fixed or bulk two-product yield approaches, and the approach of time-dependent organic condensation and evaporation considering vapor pressure.
Table 2Treatments of aerosol microphysics processes in two-way coupled models (WRF-Chem, WRF-CMAQ, GRAPES-CUACE, WRF-NAQPMS, and GATOR-GCMOM) applied in Asia.
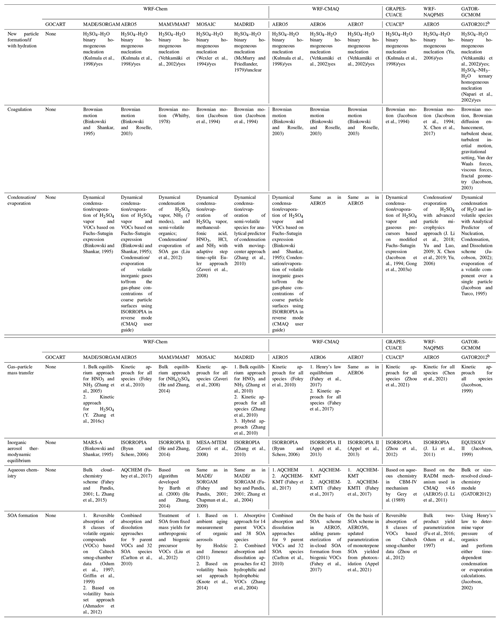
a CUACE is the aerosol mechanism implemented in the GRAPES-CUACE model (Zhou et al., 2012). b GATOR2012 is the aerosol mechanism implemented in the GATOR-GCMOM model (Jacobson, 2012b).
In addition to aerosol microphysics processes, the cloud properties included in cloud microphysics schemes and the treatment of aerosol–cloud processes in the five two-way coupled models are different in terms of hydrometeor classes, cloud droplet size distribution, aerosol water uptake, in- and below-cloud scavenging, hydrometeor–aerosol coagulations, and sedimentation of aerosols and cloud droplets (Table 3). Among the microphysics schemes implemented in the five coupled models, mass concentrations of different hydrometeors (including cloud water, rain, ice, snow, or graupel) are included, but their number concentrations are only considered if the cloud microphysics schemes are two-moment or three-moment. The single modal approach with either lognormal or gamma distribution and the sectional approach with discrete size distributions for cloud droplets are applied in different microphysics schemes. Based on the Mie theory, WRF-Chem, WRF-CMAQ, GRAPES-CUACE, WRF-NAQPMS, and GATOR-GCMOM calculate cloud radiative properties (including the extinction, scattering, and absorption coefficients, single-scattering albedo, and asymmetry factor of liquid and ice clouds) in their radiation schemes (e.g., RRTMG, GODDARD, GATOR2012). In the atmosphere, the hygroscopic growth of aerosols due to water uptake is parameterized based on the Köhler or Zdanovskii–Stokes–Robinson theory, and the hysteresis effects depending on the deliquescence and crystallization RH are taken into account in the five coupled models. The removal processes of aerosol particles include wet removal and sedimentation. Aerosol particles in accumulation and coarse modes can act as cloud condensation nuclei (CCN) or ice nuclei (IN) via activations in cloud, which can further develop to different types of hydrometeors (cloud water, rain, ice, snow, and graupel) and then gradually form precipitation. These processes are called in-cloud scavenging or rainout. The aerosol particles below cloud base also can be coagulated with the falling hydrometeors, which is known as below-cloud scavenging or washout. Representations of both in- and below-cloud scavenging processes are based on the scavenging rate approach in aerosol mechanisms of WRF-Chem, WRF-CMAQ, GRAPES-CUACE, and WRF-NAQPMS but not GATOR-GCMOM. Size-resolved sedimentation of aerosols is computed from one model layer to layers below down to the surface layer using setting velocity in most coupled models, and the MOSAIC aerosol mechanism in WRF-Chem only considers the sedimentation in the lowest model level (Marelle et al., 2017).
Table 3Compilation of cloud properties and aerosol–cloud processes in two-way coupled models (WRF-Chem, WRF-CMAQ, GRAPES-CUACE, WRF-NAQPMS, and GATOR-GCMOM) applied in Asia.
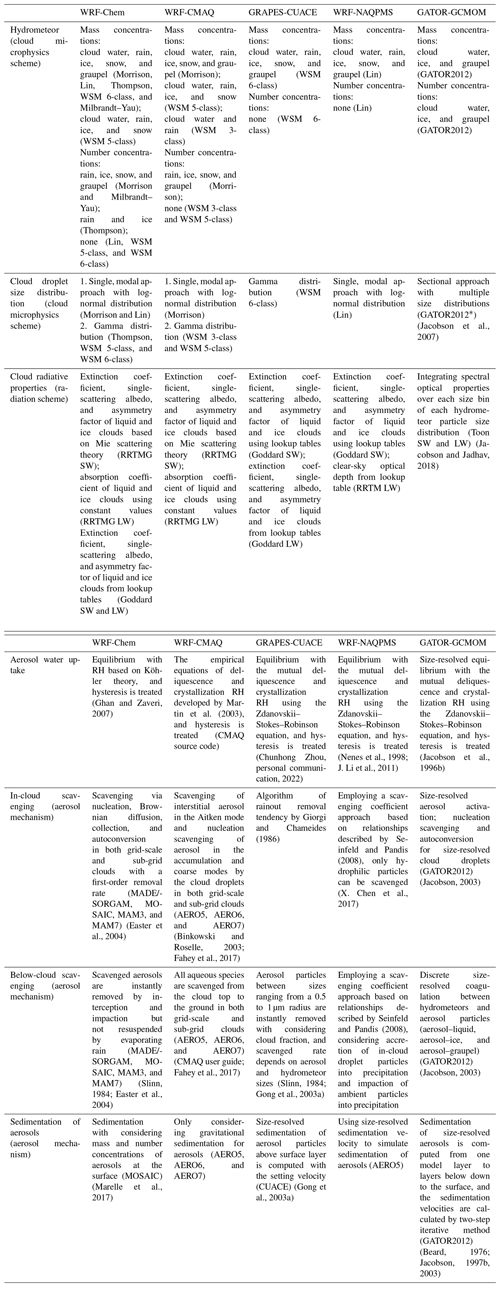
∗ GATOR2012 refers to either the aerosol or cloud microphysics scheme used in Jacobson (2012b).
Table 4 further lists various aspects with regards to how ARI and ACI are calculated in the five two-way coupled models (WRF-Chem, WRF-CMAQ, GRAPES-CUACE, WRF-NAQPMS, and GATOR-GCMOM) applied in Asia. Note that the information in this table was extracted from the latest released version of WRF-Chem (version 4.3.3) and WRF-CMAQ (based on WRF v4.3 and CMAQ v5.3.3) as well as relevant references for GRAPES-CUACE (H. Wang et al., 2015), WRF-NAQPMS (Z. Wang et al., 2014), and GATOR-GCMOM (Jacobson, 2012a). These models all use the Mie theory to compute ARI effects but differ in representations of aerosol optical properties and radiation schemes. To simplify the calculation, aerosol species simulated by the chemistry module and/or model are put into different groups (Table 4), and the refractive indices of these groups are directly from the Optical Properties of Aerosols and Clouds (OPAC) database (Hess et al., 1998) in WRF-Chem and WRF-CMAQ (Table B6 in Appendix B). In WRF-Chem, the aerosol optical properties (AOD, extinction, scattering, and absorption coefficients, single-scattering albedo, and asymmetry factor) are calculated in terms of four spectral intervals (listed in Table B6 in Appendix B) and then interpolated and/or extrapolated to 11 (14) SW intervals defined in the GODDARD (RRTMG) scheme. For SW and LW radiation in both WRF-CMAQ and WRF-Chem, these optical parameters are computed at each of the corresponding spectral intervals in the RRTMG scheme. The aerosol optical property for LW radiation is considered only at five thermal windows (listed in Table B6) in WRF-CMAQ. No detailed information regarding how aerosol optical properties and relevant parameters are calculated in GRAPES-CUACE and WRF-NAQPMS can be found from the relevant references.
With respect to ACI effects, the simulated aerosol characteristics (such as mass, size distribution and species) are utilized for the calculation of cloud droplet activation and aerosol resuspension based on the Köhler theory (Abdul-Razzak and Ghan, 2002) in several (one) microphysics schemes (scheme) in WRF-Chem (GRAPES-CUACE). GATOR-GCMOM is the first two-way coupled model adding IN activation processes including heterogeneous and homogeneous freezing (Jacobson, 2003). None of the other four two-way coupled models consider the IN formation processes (including immersion freezing, deposition freezing, contact freezing, and condensation freezing), but they have been included in some specific versions of WRF-Chem (Keita et al., 2020; Lee et al., 2020), which are not yet in the latest release version 4.3.3 of WRF-Chem.
Table 4Summary of relevant information regarding calculations of aerosol–radiation interaction (ARI) and aerosol–cloud interaction (ACI) in two-way coupled models (WRF-Chem, WRF-CMAQ, GRAPES-CUACE, WRF-NAQPMS, and GATOR-GCMOM) applied in Asia.
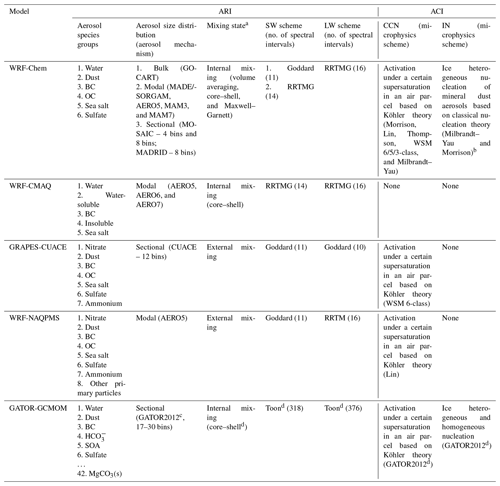
a Specific versions of WRF-Chem, WRF-NAQPMS and GATOR-GCMOM have the ability to simulate aerosol aging (H. Zhang et al., 2014; X. Chen et al., 2017; J. Li et al., 2018; Jacobson, 2012b). b Some specific versions of WRF-Chem consider IN (Keita et al., 2020; Lee et al., 2020). c The shortwave and longwave radiation calculations in GATOR-GCMOM are based on the algorithm of Toon et al. (1989). d GATOR2012 refers to either the aerosol or cloud microphysics scheme used in Jacobson (2012b).
How accurately ARI and ACI are simulated also relies on the representation of aerosol composition and size distribution in two-way coupled models. Table 5 presents the treatments of aerosol compositions and size distributions in the five two-way coupled models applied in Asia. As shown in Tables 4 and 5, GATOR-GCMOM considered more detailed aerosol species groups with as many as 42 kinds, and other coupled models considered different numbers of species groups (such as six, five, seven, and eight aerosol species groups in WRF-Chem, CMAQ, NAQPMS, and CUACE, respectively). Three typical representation approaches of size distribution (bulk, modal, and sectional methods) are adopted by the five two-way coupled models, and WRF-Chem offers all three approaches, but other models only support one specific option. The Global Ozone Chemistry Aerosol Radiation and Transport (GOCART) model (Ginoux et al., 2001) in WRF-Chem is the only one that is based on a combination of bulk (for water, BC, OC, and sulfate aerosols) and sectional (for dust and sea salt aerosols) approaches. The widely used modal and sectional approaches in five coupled models and their detailed numerical settings of aerosol size distribution (namely, geometric diameter and standard deviation for the modal approach and bin ranges for the sectional method) are listed in Table 5. Regarding the modal method, same parameter values for Aitken and accumulation modes as well as geometric diameters for the coarse mode in the latest version of WRF-Chem (v4.3.3) and the older version of WRF-CMAQ (before v5.2) are set as default, except the standard deviations for the coarse mode, which are slightly different. In the official version of WRF-CMAQ released after v5.2, there are some modifications to the default setting of geometric diameters in Aitken, accumulation, and coarse modes from 0.01 to 0.015, 0.07 to 0.08, and 1.0 to 0.6 µm, respectively. For the GRAPES-CUACE model, the parameters of size distribution for certain aerosol species in the accumulation mode were updated from its older version (Zhou et al., 2012) to the newer one (Zhang et al., 2021). With respect to the sectional approach, 4 or 8 (from 0.039 to 10 µm), 12 (from 0.005 to 20.48 µm), and 14 (from 0.002 to 50 µm) particle size bins are defined in WRF-Chem, CUACE, and GATOR-GCMOM, respectively.
Table 5Summary of numerical representations of aerosol size distribution and composition in two-way coupled models (WRF-Chem, WRF-CMAQ, GRAPES-CUACE, WRF-NAQPMS, and GATOR-GCMOM) applied in Asia.
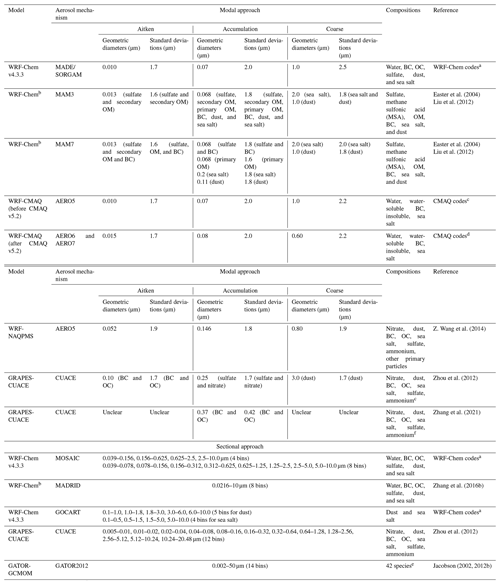
a Official released version of WRF-Chem. b Specific version of WRF-Chem. c https://github.com/USEPA/CMAQ/blob/5.1/models/CCTM/aero/aero6/AERO_DATA.F (last access: 20 March 2022). d https://github.com/USEPA/CMAQ/blob/5.2/CCTM/src/aero/aero6/AERO_DATA.F (last access: 20 March 2022). e More detailed components are presented in the first column of Table 2. f Initial size distribution is a trimodal lognormal distribution.
Not only the choice of methodologies for ARI and ACI calculations can impact simulation results, but also the various aspects regarding the setup of modeling studies by applying two-way coupled models. The extra and/or auxiliary information about model configuration, including horizontal and vertical resolutions, aerosol- and gas-phase chemical mechanisms, PBL schemes, meteorological and chemical ICs and BCs, and anthropogenic and natural emissions, were extracted from the 160 papers and are presented in Table S4 of the Supplement, which is organized in the same order as Table 1.
For two-way coupled model applications in Asia, horizontal resolutions were set from a few to several hundred kilometers, sometimes with nests, and vertical resolutions were from 15 to about 50–70 levels, with only one study performed at 100 levels for studying a fog case (Z. Wang et al., 2019). K. Wang et al. (2018) evaluated the impacts of horizontal resolutions on simulation results and found that surface meteorological variables were better modeled at finer resolution, but there were no significant improvements of ACI-related meteorological variables and certain chemical species between different grid resolutions. By applying a single column model and then WRF-Chem with ARI, Z. Wang et al. (2019) revealed that better representation of PBL structure and relevant variables with finer vertical resolution from the surface to the PBL top could reduce model biases noticeably, but balancing between vertical resolution and computational resources was important as well. Among the 160 applications of two-way coupled models in Asia, the frequently used aerosol module and gas-phase chemistry mechanism in WRF-CMAQ (WRF-Chem) were AERO6 (MOSAIC and MADE/SORGAM) and CB05 (CBMZ and RADM2), respectively. For PBL schemes, most studies selected YSU in WRF-Chem and ACM2 in WRF-CMAQ. Regarding meteorological ICs and BCs, the FNL data were the first choice, and outputs from the Model for Ozone and Related Chemical Tracer (MOZART) were used to generate chemical ICs and BCs by most researchers. Georgiou et al. (2018) also revealed that boundary conditions of dust and O3 played an important role in WRF-Chem simulations. The modeling applications in Asia utilized global (EDGAR), regional (e.g., MIX, INTEX-B, and REAS), and national (e.g., MEIC and JEI-DB) anthropogenic emission inventories. Natural emission sources, such as mineral dust (Shao, 2004), biomass burning (FINN, Wiedinmyer et al., 2011, and GFED, Giglio et al., 2010), biogenic VOCs (MEGAN; Guenther et al., 2006), and sea salt (Gong et al., 1997), were also considered. It should be noted that only one paper by Gao et al. (2017c) reported that the WRF-Chem model with the Gridpoint Statistical Interpolation (GSI) data assimilation could improve the simulation accuracy during a wintertime pollution period.
4.1 Feedbacks of natural aerosols
4.1.1 Mineral dust aerosols
Due to the fact that dust storm events frequently occurred over Asia during 2000–2010, the research community has focused on dust transportation and associated climatic effects (Gong et al., 2003b; Zhang et al., 2003a, b; Yasunari and Yamazaki, 2009; Lee et al., 2010; Choobari et al., 2014). Also, the detailed processes and physiochemical mechanisms of dust storms have been well understood and reviewed in detail (Shao and Dong, 2006; Uno et al., 2006; Huang et al., 2014; S. Chen et al., 2017b). To probe the radiative feedbacks of dust aerosols in Asia, Wang et al. (2010, 2013) initiated modeling studies by a two-way coupled model, i.e., the GRAPES-CUACE model, to simulate direct radiative forcing (DRF) of dust and revealed that the feedback effects of dust aerosols could lead to decreasing surface wind speeds and then suppress dust emissions. Further modeling simulations by the same model (Wang and Niu, 2013) indicated that considering dust radiative effects did not substantially improve the model performance of the air temperature at 2 m above the surface (T2), even when assimilating data from in situ and satellite observations into the model. Subsequently, several similar studies based on another two-way coupled model (WRF-Chem with the GOCART scheme) were conducted to investigate dust radiative forcing (including shortwave radiative forcing – SWRF – and longwave radiative forcing – LWRF) and ARI effects of dust on meteorological variables (PBLH, T2 and WS10) in different regions of Asia (Kumar et al., 2014; Chen et al., 2014; Jin et al., 2015, 2016b; L. Liu et al., 2016; Bran et al., 2018; Su and Fung, 2018a, b; Zhou et al., 2018). These studies demonstrated that dust aerosols could induce negative radiative forcing (cooling effect) at the top of the atmosphere (TOA) as well as the surface (including both Earth's and sea surfaces) and positive radiative forcing (warming effect) in the ATM (Wang et al., 2013; Chen et al., 2014; Kumar et al., 2014; M. M. Li et al., 2017a; Bran et al., 2018; Li and Sokolik, 2018; Su and Fung, 2018b). More thorough analyses of the radiative effects of dust in Asia (Wang et al., 2013; Li and Sokolik, 2018) pointed out that dust aerosols played opposite roles in the shortwave and longwave bands so that the dust SWRF at TOA and the surface (cooling effects) as well as in the ATM (warming effects) was offset partially by the dust LWRF (warming effects at TOA and the surface but cooling effects in the ATM). It was noteworthy that adding a more detailed mineralogical composition to the dust emissions for WRF-Chem could alter the dust SWRF at TOA from cooling to warming and then lead to a positive net radiative forcing at TOA (Li and Sokolik, 2018). These different conclusions showed some degree of uncertainty in the coupled model simulations of dust aerosols' radiative forcing that needs to be further investigated in the future.
Dust aerosols can act not only as water-insoluble cloud condensation nuclei (CCN) (Kumar et al., 2009) but also as ice nuclei (IN) (Lohmann and Diehl, 2006) since they are referred to as ice-friendly (Thompson and Eidhammer, 2014). Therefore, activation and heterogeneous ice nucleation parameterizations (INPTs) with respect to dust aerosols were developed and incorporated into WRF-Chem to explore ACI effects as well as both ARI and ACI effects of dust aerosols in Asia (Jin et al., 2015, 2016b; Y. Zhang et al., 2015a; Su and Fung, 2018a, b; K. Wang et al., 2018). During dust storms, including the adsorption activation of dust particles played vital roles in the simulations of ACI-related cloud properties, with a 45 % increase in cloud droplet number concentration (CDNC) compared to a simpler aerosol activation scheme in WRF-Chem (K. Wang et al., 2018). More sophisticated INPTs implemented in WRF-Chem that take dust particles into account as IN resulted in substantial modifications of cloud and ice properties as well as surface meteorological variables and air pollutant concentrations in model simulations (Y. Zhang et al., 2015a; Su and Fung, 2018b). Y. Zhang et al. (2015a) determined that dust aerosols acting either as CCN or IN made model results rather different regarding radiation, T2, precipitation, and number concentrations of cloud water and ice. Su and Fu (2018b) described the ACI effects of dust as having fewer impacts on the radiative forcing than its ARI effects, and dust particles could promote (demote) ice (liquid) clouds in the middle to upper (lower to middle) troposphere over EA. With turning on both ARI and ACI effects of dust, fewer low-level clouds and more mid- and high-level clouds were detected that contributed to cooling at the Earth's surface and in the lower atmosphere as well as warming in the middle to upper troposphere (Su and Fung, 2018b). Mineral dust particles transported by the westerly and southwesterly winds from the Middle East (ME) affected the radiative forcing at TOA and the Earth's surface as well as in the ATM by the dust-induced ARI and ACI in the Arabian Sea and the Indian subcontinent, subsequently changing the circulation patterns, cloud properties, and characteristics related to the India summer monsoon (ISM; Jin et al., 2015, 2016a). Moreover, the effects of dust on precipitation are not only complex but also highly uncertain, as evidenced by several modeling investigations targeting a variety of areas in Asia (Jin et al., 2015, 2016a, b; Y. Zhang et al., 2015a; Su and Fung, 2018b). Less precipitation from model simulations including dust effects was found at EA, and dust particles acting mainly as CCN or IN influenced precipitation in a rather different way (Y. Zhang et al., 2015a). A positive response of ISM rainfall to dust particles from the ME was reported by Jin et al. (2015) and was less affected by dust storms from local sources and NWC (Jin et al., 2016b). Jin et al. (2016a) further elucidated the fact that the impacts of ME dust on ISM rainfall were highly sensitive to the imaginary refractive index of the dust setting in the model, so accurate simulations of the dust–rainfall interaction depended on more precise representation of radiative absorptions of dust in two-way coupled models. About a 20 % increase or decrease in rainfall due to the dust effects was detected in different areas over EA from the WRF-Chem simulations (Su and Fung, 2018b). However, it should be mentioned that a few studies targeting DRF of dust in Asia based on WRF-Chem simulations but without enabling aerosol–radiation feedbacks (Ashrafi et al., 2017; S. Chen et al., 2017b; Tang et al., 2018) were not included in this paper.
Along with the modeling research on the effects of dust aerosols on meteorology, their impacts on air quality in Asia were explored using two-way coupled models (Wang et al., 2013; Chen et al., 2014; Kumar et al., 2014; M. M. Li et al., 2017a; Li and Sokolik, 2018). Many early modeling research work involving two-way coupled models with dust only looked into the ARI or direct radiative effects of dust particles, which are described as follows. Taking a springtime dust storm from the Thar Desert into consideration in WRF-Chem, the modeled aerosol optical depth (AOD) and Ångström exponent (as indicators of aerosol optical properties and unique proxies for the surface particulate matter pollution) demonstrated that turning on the ARI effects of dust could reduce biases in their simulations, but were underestimated in northern India (Kumar et al., 2014). Wang et al. (2013) pointed out that in EA, including the longwave radiative effects of dust in the GRAPES-CUACE dust model lowered relative errors of the modeled AOD by 15 % compared to simulations only considering shortwave effects of dust. Comparisons against both satellite and in situ observations showed that the WRF-Chem model was able to capture the general spatiotemporal variations of the optical properties and size distribution of dust particles over the main dust sources in EA, such as the Taklimakan and Gobi deserts, but overestimated AOD during summer and fall and also exhibited positive (negative) biases in the fine (coarse) mode of dust particles (Chen et al., 2014). Besides the ARI effects of dust, the heterogeneous chemistry on dust particles' surface added in WRF-Chem accounted for 80 % of the net reductions of O3, NO2, NO3, N2O5, HNO3, •OH, HO2•, and H2O2 when a springtime dust storm struck the Nanjing megacity of EC (M. M. Li et al., 2017a). In CA, AOD was overestimated by the WRF-Chem model, but its simulation improved when more detailed mineral components of dust particles were incorporated in the model (Li and Sokolik, 2018). Later on, more investigations started to focus on both ARI and ACI effects of dust aerosols. With consideration of ARI as well as both ARI and ACI of dust particles from the ME, during the ISM period, the WRF-Chem model reproduced AOD spatial distributions but underpredicted (overpredicted) AOD over the Arabian Sea (the Arabian Peninsula) compared with satellite observations and AOD reanalysis data (Jin et al., 2015, 2016a, b). In EA, K. Wang et al. (2018) demonstrated that including both ARI and ACI effects of dust in WRF-Chem caused lower O3 concentrations, and by incorporating INPTs, the WRF-Chem model simulated the surface PM10 concentrations (Su and Fung, 2018a) well with reduced (elevated) surface concentrations of OH, O3, SO, and PM2.5 (CO, NO2, and SO2) (Y. Zhang et al., 2015a). It is worth noting that how to partition dust particles into the fine mode and coarse mode or initialize their size distribution in coupled models can affect simulations in many ways and requires more detailed measurements at the source areas and further modeling studies.
4.1.2 Wildfire, sea salt, and volcanic ash
In the maritime SEA region, peat and forest fires triggered by El Niño induced drought conditions and released a huge amount of smoke particles, which promoted dire air pollution problems in the downstream areas, and their ARI effects simulated by WRF-Chem enhanced radiative forcing at the TOA and the atmospheric stability (Ge et al., 2014). Ge et al. (2014) also pointed out that the ARI effects of these fires impaired (intensified) sea breeze during daytime (land breeze at nighttime) over this region so that their impacts on cloud cover could be positive or negative in different areas and time periods (day or night). Sea salt and volcanic ash are also important natural aerosols for regions near seashores as are active volcanoes and surrounding areas, but modeling studies of their ARI and ACI effects are relatively scarce in Asia. Based on WRF-Chem simulations, Kedia et al. (2019a) demonstrated that the feedbacks of sea salt aerosols impacted convective and non-convective precipitation rather variously in different areas of the Indian subcontinent. Jiang et al. (2019a, b) also used WRF-Chem with and without sea salt emissions to evaluate the effects of sea salt on rainfall in Guangdong Province of China, but unfortunately, no feedbacks were considered in the simulations. So far there has been no investigation targeting aerosol effects of volcanic ash from eruptions in Asia using coupled models.
4.2 Feedbacks of anthropogenic aerosols
Atmospheric pollutants from anthropogenic sources are the leading causes of heavy pollution events occurring in Asia due to the acceleration of urbanization, industrialization, and population growth in recent decades, particularly in China and India, and their ARI and/or ACI effects on meteorology and air quality have been quantitatively examined using two-way coupled models (Kumar et al., 2012a, b; Li and Liao, 2014; J. Wang et al., 2014; B. Zhang et al., 2015; M. Gao et al., 2016a; Yao et al., 2017; Z. Wang et al., 2018; Archer-Nicholls et al., 2019; Bharali et al., 2019). This modeling research work has been primarily focused on the ARI and/or ACI effects of anthropogenic aerosols, their specific chemical components (especially the light-absorbing aerosols, i.e., BC and brown carbon – BrC), and aerosols originating from different sources. The major findings are outlined as follows with respect to the effects of anthropogenic aerosol feedbacks on meteorology and air quality.
Concerning the meteorological responses, most papers treated anthropogenic aerosols as a whole to explore their effects on meteorological variables based on coupled model simulations by enabling ARI and/or ACI in WRF-Chem, WRF-CMAQ, WRF-CMAQ, GRAPES-CUACE, and WRF-NAQPMS (Kumar et al., 2012b; J. Wang et al., 2014; Z. Wang et al., 2014; H. Wang et al., 2015; B. Zhang et al., 2015; X. Zhang et al., 2018; Zhao et al., 2017; Nguyen et al., 2019a, b; Bai et al., 2020). Generally, the main ARI effects of anthropogenic aerosols resulted in decreases in SWRF, T2, WS10, and PBLH, as well as increases in surface relative humidity (RH2) and temperature in the ATM, which further suppressed PBL development (Y. Gao et al., 2015; Xing et al., 2015c; M. M. Li et al., 2017b; Zhang et al., 2018; Nguyen et al., 2019a, b). H. Wang et al. (2015) utilized GRAPES-CUACE with ARI to study a summer haze case in the NCP area and discovered that the ARI effects made the subtropical high less intense (−14 hPa) to help pollutants in the area dissipate. In Asia, ACI effects of anthropogenic aerosols on cloud properties and precipitation are relatively complex. On the one hand, anthropogenic aerosols activated as CCN enhanced CDNC and LWP and then slowed down the precipitation onset, but their impacts on precipitation amounts varied in different seasons and areas in China (Zhao et al., 2017). Targeting a summertime rainstorm in the middle reaches of the Yangtze River (MRYR) in China, sensitivity studies using WRF-Chem unveiled that CDNC, cloud water contents, and precipitation decreased (increased) with low (high) anthropogenic emission scenarios due to the ACI effects, and these variations tended to depend on atmospheric humidity (Bai et al., 2020). The modeling investigations with WRF-Chem aiming at the ISM (Kedia et al., 2019a) and a disastrous flood event in southwestern China (SWC) (Fan et al., 2015) pointed out that the simulated convective process was suppressed and convective (non-convective) precipitation was inhibited (enhanced) by the ARI and ACI effects of accumulated anthropogenic aerosols, but these effects could invigorate convection and rainfall in the downwind mountainous area at nighttime (Fan et al., 2015). On the other hand, how anthropogenic aerosols act in the ice nucleation processes is still open to debate (Zhao et al., 2019), and these processes need to be represented accurately in two-way coupled models. However, until now no study has been performed to simulate the ACI effects of anthropogenic aerosol serving as IN in Asia using two-way coupled models. Therefore, in Asia, further investigations are needed targeting cloud and/or ice processes involving anthropogenic aerosols (including their size, composition, and mixing state) in two-way coupled models. Meanwhile, several studies not only discussed aerosol feedbacks but also focused on the additional effects of topography or the urban heat island on meteorology (Zhong et al., 2015, 2017; D. Wang et al., 2019). Utilizing the GATOR-GCMOM model at global and local scales, Jacobson et al. (2015, 2019) explored the impacts of land use changes due to the unprecedented expansions of megacities, such as Beijing and New Delhi in Asia, from 2000 to 2009 on meteorology and air quality.
Hitherto there were several attempts to ascertain the effects of different chemical components of anthropogenic aerosols on meteorology in Asia (Huang et al., 2015; Ding et al., 2016, 2019; J. Gao et al., 2018; Z. Wang et al., 2018; Archer-Nicholls et al., 2019). First of all, Asia is the region in the world with the highest BC emissions due to burning of a large amount of fossil fuels and biomass, and this has increasingly attracted many researchers to probe into the ARI and/or ACI effects of BC (IPCC, 2013). As the most important absorbing aerosol, BC induced the largest mean DRF at the TOA (positive), in the ATM (positive), and at the surface (negative) over China during 2006 (Huang et al., 2015). Ding et al. (2016) and Z. Wang et al. (2018) further applied WRF-Chem with feedbacks to investigate how aerosol–PBL interactions involving BC suppressed the PBL development, which deteriorated air quality in Chinese cities and was described as the “dome effect” (namely, BC warms the atmosphere and cools the surface, suppresses the PBL development, and eventually results in more accumulation of pollutants). This dome effect of BC promoted advection–radiation fog and fog–haze formation in the YRD area through altering the land–sea circulation pattern and increasing the moisture level (Ding et al., 2019). J. Gao et al. (2018) also pointed out that BC in the ATM modified the vertical profiles of heating rate and equivalent potential temperature in Nanjing, China. In India, the ARI effects of BC enhanced convective activities, meridional flows, and rainfall in northeastern India during the pre-monsoon season but could either enhance or suppress precipitation during the monsoon season in different parts of the Indian subcontinent (Soni et al., 2018). Moreover, the ARI effects of BC on surface meteorological variables were larger than its ACI effects in EC (Archer-Nicholls et al., 2019; Ding et al., 2019). Besides BC, the BrC portion of organic aerosol (OA) emitted from agriculture residue burning (ARB) was included in WRF-Chem with the parameterization scheme suggested by Saleh et al. (2014), and the model simulations in EC revealed that at the TOA, the net DRF of OA was −0.22 W m−2 (absorption and scattering DRF were +0.21 and −0.43 W m−2, respectively), but the BC's DRF was still the highest (+0.79 W m−2) (Yao et al., 2017). As mentioned above, it is obvious that ARI and ACI effects of different aerosol components are substantially distinctive, and many other aerosol compositions (e.g., sulfate, nitrate, and ammonium) besides BC and BrC should be taken into considerations in future modeling studies in Asia.
ARB is a common practice in many Asian countries after harvesting and before planting, and it can deteriorate air quality quickly as one of the most important sources of anthropogenic aerosols, so it has been attracting much attention among the public and scientists worldwide (Reid et al., 2005; Koch and Del Genio, 2010; J. Chen et al., 2017; Yan et al., 2018; Hodshire et al., 2019). Recently, the effects of ARB aerosols on meteorology has been widely explored using the two-way coupled model (WRF-Chem) in many Asian countries and regions, such as EC (Huang et al., 2016; Wu et al., 2017; Yao et al., 2017; M. Li et al., 2018), southern China (SC) (Huang et al., 2019), and South Asia (SA) (Singh et al., 2020). In general, when ARB occurred, the WRF-Chem simulations from all the studies showed that the changes in radiative forcing induced by ARB aerosols were greater than by those from other anthropogenic sources, especially in the ATM. Also, all the modeling studies indicated that ARB aerosols reduced (increased) radiative forcing at the surface (in the ATM), cooled (warmed) the surface (the atmosphere), and increased (decreased) atmospheric stability (PBLH). Furthermore, the WRF-Chem simulations with ARI demonstrated that light-absorbing carbonaceous aerosols (CAs) from ARB caused daytime (nighttime) precipitation to decrease (increase) over Nanjing in EC during a post-harvest ARB event (Huang et al., 2016). Yao et al. (2017) pointed out that their WRF-Chem simulations in EC exhibited a larger direct radiative effect (DRE) induced by BC from ARB at the TOA than previous studies. Lately, several modeling studies using WRF-Chem targeted the effects of ARI and both ARI and ACI due to ARB aerosols from countries in the Indochina, SEA, and SA regions during the planting and harvesting time (Zhou et al., 2018; Dong et al., 2019; Huang et al., 2019; Singh et al., 2020). Zhou et al. (2018) investigated how ARB aerosols from SEA mixed with mineral dust and other anthropogenic aerosols while being lifted to the middle to lower troposphere over the source region and transported to the YRD area and then affected meteorology and air quality there. The influences of ARI and ACI caused by ARB aerosols from Indochina were contrary: namely, the ARI (ACI) effects made the atmosphere over SC warmer (cooler) and drier (wetter), and the ARI effects hindered cloud formation and suppressed precipitation there (Huang et al., 2019). Dong et al. (2019) found that the warming ARI effects of ARB aerosols were smaller over the source region (i.e., SEA) than the downwind region (i.e., SC) with cloudier conditions. Annual simulations regarding the ARI effects of ARB aerosols from SA (especially Myanmar and Punjab) indicated that CAs released by ARB reduced the radiative forcing at the TOA but did not change the precipitation processes much when only the ARI effects were considered in WRF-Chem (Singh et al., 2020).
Besides ARB, to our best knowledge, there were only a few research works quantitatively assessing the effects of anthropogenic aerosols from different emission sources on meteorology using WRF-Chem. M. Gao et al. (2018b) evaluated the responses of radiative forcing in China and India to aerosols from five emission sectors (power, industry, residential, BB, and transportation) and found that the power (residential) sector was the dominant contributor to the negative (positive) DRF at the TOA over both countries due to high emissions of sulfate and nitrate precursors (BC), and the total sectoral contributions were in the order of power > residential > industry > BB > transportation (power > residential > transportation > industry > BB) for China (India) during 2013. To pinpoint the ARI and ACI effects, Archer-Nicholls et al. (2019) reported that during January 2014, the aerosols from the residential emission sector induced larger SWRF (+1.04 W m−2) than LWRF (+0.18 W m−2) at the TOA and their DRF (+0.79 W m−2) was the largest, followed by their semi-direct effects (+0.54 W m−2) and indirect effects (−0.29 W m−2) over EC. This study further emphasized that a realistic ratio of BC to total carbon from residential emissions was critical for accurate simulations of the ARI and ACI effects with two-way coupled models.
In terms of anthropogenic aerosol effects on air quality, the responses of PM2.5 have been widely investigated (J. Wang et al., 2014; Z. Wang et al., 2014; H. Wang et al., 2015; Y. Gao et al., 2015; M. Gao et al., 2016b; J. Gao et al., 2018; B. Zhang et al., 2015; X. Zhang et al., 2018; Zhao et al., 2017; Chen et al., 2019b; Nguyen et al., 2019a, b; Wu et al., 2019a), but fewer studies explored the responses of O3 and other species (Kumar et al., 2012b; B. Zhang et al., 2015; Xing et al., 2017; J. Li et al., 2018; Nguyen et al., 2019a, b). As summarized by Wu et al. (2019a) in their Table 1, observations and model simulations with WRF-Chem, WRF-CMAQ, WRF-CMAQ, GRAPES-CUACE, and WRF-NAQPMS all pointed out that the ARI effects promoted higher PM2.5 concentrations in China (J. Wang et al., 2014; Z. Wang et al., 2014; H. Wang et al., 2015; Y. Gao et al., 2015; M. Gao et al., 2016b; B. Zhang et al., 2015; X. Zhang et al., 2018; Chen et al., 2019b), and this was also true in other areas of Asia (e.g., India, EA, continental SEA) (M. Gao et al., 2018b; Nguyen et al., 2019a, b) during different seasons. At the same time, all the modeling investigations revealed that the positive aerosol–meteorology feedbacks could further exacerbate pollution problems during heavy haze episodes. Based on WRF-Chem simulations, the ACI effects on PM2.5 were negligible compared to the ARI effects over EC (B. Zhang et al., 2015) but subject to a certain degree of uncertainty with no consideration of the ACI effects induced by cumulus clouds in the model (Y. Gao et al., 2015). Annual WRF-Chem simulations for 2014 by Zhang et al. (2018) indicated that even though the ARI effects had bigger impacts on PM2.5 during wintertime than the ACI effects, the ARI and ACI impacts on PM2.5 were similar during other seasons, and the increase in PM2.5 due to the ACI effects was more noticeable in the wet season than the dry season. Using the process analysis method to distinguish the contributions of different physical and chemical processes to PM2.5 over the NCP area, Chen et al. (2019b) applied WRF-Chem with ARI and ACI and found that besides local emissions and regional transport processes, vertical mixing contributed the most to the accumulation and dispersion of PM2.5 compared to chemistry and advection, and the ARI effects changed the vertical mixing contribution to daily PM2.5 variation from negative to positive. Regarding surface O3 concentrations, all the two-way coupled models with ARI, ACI, and both ARI and ACI predicted a reduced photolysis rate and O3 concentrations under heavy pollution conditions through the radiation attenuation induced by aerosols and clouds. Further analyses indicated that the ARI effects impacted O3 positively through reducing vertical dispersions (WRF-CMAQ, Xing et al., 2017), reducing O3 more during wintertime than summertime in EC (WRF-NAQPMS, J. Li et al., 2018), and suppressing (enhanced) O3 in the dry (wet) season in continental SEA (WRF-CMAQ, Nguyen et al., 2019b). Xing et al. (2017) applied the process analysis method in WRF-CMAQ with ARI and revealed that the impacts of ARI on the contributions of atmospheric dynamics and photochemistry processes to O3 over China varied in winter and summer months, and ARI induced the largest changes in photochemistry (dry deposition) of surface O3 at noontime in January (July). The process analysis in WRF-Chem with ARI and ACI indicated that the vertical mixing process played the most important role among the other physical and chemical processes (advection and photochemistry) in surface O3 growth during 10:00–14:00 local time in Nanjing, China (Gao et al., 2018a). ARI and ACI not only affected PM2.5 and O3, but also other chemical species. For instance, CO and SO2 increased due to ARI and ACI over EC (B. Zhang et al., 2015), ARI caused midday (daily average) OH increase (decrease) in July (January) over China (Xing et al., 2017), SO2, NO2, BC, SO, and NO were enhanced but OH was reduced over China by ACI (Zhao et al., 2017), and ARI impacted SO2 and NO2 positively over EA (Nguyen et al., 2019a). Wu et al. (2019b) further analyzed how the aerosol liquid water involved in ARI and chemical processes (i.e., photochemistry and heterogeneous reactions) influenced radiation and PM2.5 (especially secondary aerosols) over NCP during an intense haze event. Moreover, evaluations and sensitivity studies indicated that turning on aerosol feedbacks could improve the model performance for surface PM2.5, particularly during severe haze episodes (B. Zhang et al., 2015; X. Zhang et al., 2018; J. Li et al., 2018; H. Wang et al., 2018).
With reference to the feedback effects of anthropogenic aerosol compositions on air quality, most modeling research work with WRF-Chem has focused on the ARI and ACI effects of BC and BrC, especially the dome effect that prompted the accumulation of pollutants (aerosols and O3) near the surface and in the PBL (Li and Liao, 2014; Ding et al., 2016, 2019; J. Gao et al., 2018; Z. Wang et al., 2018). At the same time, the ARI effects of BC undermined the low-level wind convergence and then led to a decrease in aerosols (sulfate and nitrate) and O3 (Li and Liao, 2014). With the process analysis methodology in WRF-Chem, J. Gao et al. (2018) indicated that compared to simulations without BC, the BC and PBL interaction slowed the O3 growth from late morning to early afternoon somewhat before O3 reached its maximum value at noon due to less vertical mixing in the PBL.
Studies on the feedback effects of aerosols from different emission sectors on air quality were relatively limited and mainly involved with ARB emissions and assessments of emission controls during certain major air pollution events. Jena et al. (2015) applied WRF-Chem with aerosol feedbacks and investigated O3 and its precursors in SA due to regional ARB. Based on WRF-Chem simulations with enabling ARI and ACI, Wu et al. (2017) determined that aerosols emitted from ARB could be mixed and/or coated with urban aerosols while being transported to cities and contributed to heavy air pollution events there, such as in Nanjing, China. The ARI effects induced by ARB aerosols on O3 and NO2 concentrations (−1 % and 2 %, respectively) were small compared to the contribution of precursors emitted from ARB to O3 chemistry (40 %) in the ARB zone (M. Li et al., 2018). Pollutants emitted from natural and anthropogenic BB over Indochina affected pollution levels over SC, and their ACI effects removed aerosols more efficiently than the ARI effects that could make BB aerosols last longer in the ATM (Huang et al., 2019). Gao et al. (2017b) and Zhou et al. (2019) both utilized WRF-Chem to evaluate what role the ARI effects played when dramatic emission reductions were implemented during the week of the Asia Pacific Economic Cooperation Summit and concluded that the ARI reduction induced by decreased emissions led to a 6.7 %–10.9 % decline in PM2.5 concentrations in Beijing.
4.3 Human health effects
Poor air quality poses risks to human health (Brunekreef and Holgate, 2002; Manisalidis et al., 2020); therefore, in the past several decades, air quality models have been used in epidemiology-related research to establish quantitative relationships between concentrations of various pollutants and the burden of disease (including mortality and/or morbidity) as well as associated economic loss (Conti et al., 2017). In Asia, there were several studies that applied coupled air quality models with feedbacks to assess human health effects of air pollutants under historical and future scenarios (M. Gao et al., 2015, 2017c; Ghude et al., 2016; Xing et al., 2016; Wang et al., 2017; Conibear et al., 2018a, b; Hong et al., 2019; Zhong et al., 2019). By applying WRF-Chem with ARI and ACI, M. Gao et al. (2015) estimated the health and financial impacts induced by an intense air pollution event that happened in the NCP area during January 2013 and concluded that the mortality, morbidity, and financial losses over the Beijing area were USD 690, 69 070, and 253.8 million, respectively. Targeting the same case, Gao et al. (2017c) pointed out that turning on the data assimilation of surface PM2.5 observations in WRF-Chem not only improved model simulations but also made the premature death numbers increase by 2 % in the NCP area compared to simulations without the PM2.5 data assimilation. In India, WRF-Chem simulations with aerosol feedbacks and updated population data revealed that the number of premature deaths related to chronic obstructive pulmonary disease (COPD) caused by PM2.5 (O3) was 570 000 (12 000), resulting in shortened life expectancy (3.4±1.1 years) and financial expenses (USD 640 million) during 2011 (Ghude et al., 2016). Based on WRF-CMAQ simulations with ARI for 21 years (1990–2010), Xing et al. (2016) pointed out that in EA the population-weighted PM2.5-induced mortality had an upward trend from 1990 (+3187) to 2010 (+3548), and the mean mortality caused by ARI-enhanced PM2.5 was 3.68 times more than the decrease by ARI-reduced temperature. The same 21-year simulations also showed that from 1990 to 2010, the PM2.5-related mortalities in EA and SA rose by 21 % and 85 %, respectively, while they declined in Europe and high-income North America by 67 % and 58 %, respectively (Wang et al., 2017). Conibear et al. (2018a) applied WRF-Chem with ARI to study how different emission sectors affected human health in India and demonstrated that the residential energy use sector played the most critical role among other sectors and caused 511 000 premature deaths in 2014. Furthermore, Conibear et al. (2018b) investigated future PM2.5 pollution levels as well as health impacts in India under different emission scenarios (business as usual and two emission control pathways) and deduced that the burden of disease driven by PM2.5 and population factors (growth and aging) in 2050 increased by 75 % under the business-as-usual scenario but decreased by 9 % and 91 % under the International Energy Agencies New Policy Scenario and Clean Air Scenario, respectively, compared with that in 2015. The sensitivity study using WRF-Chem with ARI under a variety of emission scenarios, population projections, and concentration response functions (CRFs) for the years of 2008 and 2050 demonstrated that CRFs (future emission projections) were the main sources of uncertainty in the total mortality estimations related to PM2.5 (O3) in China (Zhong et al., 2019). Applying a suite of models, including WRF-CMAQ with ARI, climate, and epidemiology, Hong et al. (2019) inferred that under Representative Concentration Pathway 4.5, future mortalities could be 12 100 and 8900 per year in China led by PM2.5 and O3, respectively, and the climate-driven weather extremes could add 39 % and 6 % to future mortalities due to a stable atmosphere and heat waves, respectively. Ten Hoeve and Jacobson (2012) applied GATOR-GCMOM and a human exposure model to estimate the local and worldwide health effects induced by the 2011 Fukushima nuclear accident and a hypothetical one in California, US.
Even though there are a certain number of research papers using two-way coupled models to quantify the effects of aerosol feedbacks on regional meteorology and air quality in Asia, model performances impacted by considering aerosol effects varied to some extent. This section provides a summary of model performance by presenting the SIs of meteorology and air quality variables as shown in Table S2. These SIs were collected from the selected papers supplying these indices and defined as papers with SIs (PSIs) (listed in Tables B2–B3 of Appendix B). As mentioned in Sect. 3, investigations of ACI effects were very limited, and there were no former studies simultaneously exploring aerosol feedbacks with and without both ARI and ACI turned on. Here, we only compared the SIs for simulations with and without ARI in the same study, as summarized in Appendix Tables B4–B5. It should be pointed out that all the reported evaluation results either from individual models or inter-model comparison studies were extracted and put into Table S2.
5.1 Model performance for meteorology variables
With certain emissions, accurate simulations of meteorological elements are critical to air quality modeling and prediction (Seaman, 2000; Bauer et al., 2015; Appel et al., 2017; Saylor et al., 2019). Targeting meteorological variables, we summarized their SIs and further analyzed the variations of SIs on different simulated timescales and among multiple models.
5.1.1 Overall performance
Figure 3 shows the compiled statistical indicators (correlation coefficient – R – in black; mean bias – MB – and root mean square error – RMSE – in blue) of T2 (∘C), RH2 (%), and specific humidity (SH2, g kg−1) at 2 m, as well as WS10 (m s−1) from PSIs (a–d) and simulations with and without ARI (marked as ARI and NO-ARI in e–h). In this figure and the following figures, NP and NS are the number of publications and samples with SIs, respectively, and are summed up in Appendix Table B2. In these two tables, we also listed the NS of positive (red upward arrow) and negative (blue downward arrow) biases for the meteorological and air quality variables in parentheses in the MB column. Note that NS in Fig. 3e–h and Appendix Table B4 counted the samples of SIs provided by the simulations simultaneously with and without ARI. Also, the 5th, 25th, 75th, and 95th percentiles of SIs are illustrated in box-and-whisker plots; the dashed line in the box is the mean value (not median), and the circles are outliers.
The evaluations for T2 (Fig. 3a) from PSIs revealed that in Asia coupled models performed rather well for temperature (mean R=0.90) with RMSE ranging from 0.64 to 5.90 ∘C, but 60 % of samples showed the tendency towards temperature underestimations (mean value of MB = −0.20 ∘C) with the largest average MB (−0.31 ∘) occurring during winter months (70 samples). Underestimations of temperature have been reported not only from modeling studies by using WRF or coupled models, but also in Asia, Europe, and North America (García-Díez et al., 2013; Brunner et al., 2015; Makar et al., 2015b; Yahya et al., 2015; Gao et al., 2019). The WRF simulations in China (Gao et al., 2019) and the US (US Environmental Protection Agency, 2019) also showed wintertime cold biases of T2, but in Europe warm biases were reported (García-Díez et al., 2013). This temperature bias was probably related to the impacts of model resolutions (Kuik et al., 2016), urban canopies (Liao et al., 2014), and PBL schemes (Hu et al., 2013). With the ARI turned on in the coupled models, modeled temperatures (limited papers with 12 samples) were improved somewhat. The mean correlation coefficient increased from 0.93 to 0.95, and RMSE decreased slightly (Fig. 3e), but average MB of temperature decreased from −0.98 to −1.24 ∘C. In short, temperatures from PSIs or simulations with and without ARI turned on agreed well with observations but were mostly underestimated, and the negative bias of T2 simulated by models with ARI turned on got worse; the reasons behind it will be explained in Sect. 6.
Figure 3b and c illustrate that RH2 was simulated reasonably well (mean R=0.73) and the modeled SH2 was also well correlated with observations (R varied between 0.85 and 1.00). RH2 and SH2 from more than half of the samples had slightly positive and negative mean biases with average MB values of 0.4 % and −0.01 g kg−1, respectively. The overestimations of RH2 could be caused by the negative bias of T2 (Cuchiara et al., 2014). Compared with results without ARI effects, statistics of RH2 and SH2 from simulations with ARI showed better R and RMSE. However, the increased positive mean biases (average MBs of RH2 and SH2 were from 6.4 % to 7.6 % and from 0.07 to 0.11 g kg−1, respectively) indicated that turning on ARI could cause further overprediction of humidity variables. Overall, the modeled RH2 and SH2 were in good agreement with observations with slight overestimations and underestimations, respectively, and the limited studies showed that RH2 and SH2 simulated by models with ARI turned on had marginally larger positive biases relative to the results without ARI.
Compared with the correlation coefficients of T2, RH2, and SH2, mean R (0.59) of WS10 was the smallest with a large fluctuation ranging from 0.14 to 0.98 (Fig. 3d). The meta-analysis also indicated that most modeled WS10 tended to be overestimated (81 % of the samples) with the average MB value of 0.79 m s−1, and the mean RMSE value was 2.76 m s−1. The general overpredictions of WS10 by WRF (Mass and Ovens, 2011) and coupled models (Y. Gao et al., 2015; M. Gao et al., 2018a) have been explained by possible out-of-date geographical data, coarse model resolutions, and lack of better representations of urban canopy physics. The PSIs with ARI effects suggested that the correlation of wind speed was slightly improved (mean R from 0.56 to 0.57), and the average RMSE and positive MB decreased by 0.003 and 0.051 m s−1, respectively (Fig. 3h). The collected SIs indicated relatively poor performance of modeled WS10 (most wind speeds were overestimated) compared to T2 and humidity, but turning on ARI in coupled models could improve WS10 simulations somewhat.
Besides the SIs discussed above, very limited papers reported the normalized mean error (NME) (%) of surface meteorological variables (T2, SH2, RH2, and WS10) simulated by two-way coupled models (WRF-Chem and WRF-CMAQ) in Asia, which is summarized in Table B7. The evaluations with two-way coupled models in Asia showed that the overall mean percent errors of T2, SH2, RH2, and WS10 were 22.71 %, 10.32 %, 13.94 %, and 51.28 %, respectively. The ranges of NME (%) values were quite wide for T2 (from −0.48 % to 270.20 %) and WS10 (from 0.33 % to 112.28 %) reported by the limited studies. Note that no NME of surface meteorological variables simulated by two-way coupled models simultaneously with and without enabling the ARI effects was mentioned in these studies.
5.1.2 Comparisons of SIs for meteorology using different coupled models
Also, to examine how different coupled models (i.e., WRF-Chem, WRF-CMAQ, WRF-NAQPMS, GRAPES-CUACE, and GATOR-GCMOM) performed in Asia with respect to meteorological variables, the SIs were extracted from PSIs in terms of these five coupled models and displayed in Fig. 4. The SIs for T2, RH2, SH2, and WS10 from WRF-NAQPMS, GRAPES-CUACE, and GATOR-GCMOM simulations were missing or had rather limited samples so that the discussion here only focuses on the WRF-Chem and WRF-CMAQ simulations. Moreover, the SIs sample size from studies involving WRF-Chem was generally larger than that involving WRF-CMAQ, except for SH2.
As seen in Fig. 4a, the modeled T2 by both WRF-CMAQ and WRF-Chem was well correlated with observations, but WRF-CMAQ (mean R=0.95) outperformed WRF-Chem (mean R=0.90) to some extent. On the other hand, WRF-CMAQ underestimated T2 (mean MB ∘C), but WRF-Chem slightly overestimated it (mean MB =0.09 C) (Fig. 4e). The RMSE of modeled T2 by both models was at a similar level with mean RMSE values of 2.51 and 2.31 ∘C by WRF-CMAQ and WRF-Chem simulations, respectively (Fig. 4i).
Both WRF-Chem and WRF-CMAQ performed better for SH2 (mean R=0.96 and 0.97, respectively) than RH2 (mean R=0.75 and 0.73, respectively) (Fig. 4b and c), which might be due to the influence of temperature on RH2 (Bei et al., 2017). Also, the modeled RH2 (SH2) by WRF-Chem correlated better (worse) with observations than those by WRF-CMAQ. The mean RMSE of modeled RH2 (Fig. 4j) by WRF-Chem (11.1 %) was lower than that by WRF-CMAQ (14.3 %), but the mean RMSE of modeled SH2 (Fig. 4k) by WRF-Chem (2.25 g kg−1) was higher than that by WRF-CMAQ (0.71 g kg−1). It is seen in Fig. 4f and d that WRF-CMAQ overestimated RH2 and SH2 (average MB were 5.30 % and 0.07 g kg−1, respectively), and WRF-Chem underpredicted RH2 (average MB %) and SH2 (average MB, g kg−1). Generally, the modeled RH2 and SH2 were reproduced more reasonably by WRF-Chem than by WRF-CMAQ.
The modeled WS10 by both WRF-Chem and WRF-CMAQ (Fig. 4d) correlated with observations on the same level with the mean R of 0.56. The mean RMSEs of modeled WS10 by WRF-Chem and WRF-CMAQ were 1.54 and 2.28 m s−1, respectively, as depicted in Fig. 4l. Both models overpredicted WS10 to some extent with average MBs of 0.55 m s−1 (WRF-CAMQ) and 0.84 m s−1 (WRF-Chem), respectively. These results demonstrated that overall WRF-CMAQ and WRF-Chem had similar model performance for WS10.
In general, WRF-CMAQ performed better than WRF-Chem for T2 but worse for humidity (RH2 and SH2), and both models' performance for WS10 was very similar. WRF-Chem overestimated T2, RH2, and WS10 and underestimated SH2 slightly, while WRF-CMAQ overpredicted humidity and WS10 but underpredicted T2. Compared to WRF-Chem and WRF-CMAQ, the very few SIs samples indicated that for the meteorological variables excluding SH2, WRF-NAQPMS simulations matched observations better than GRAPES-CUACE simulations, but more applications and statistical analyses of these two models are needed to make this kind of comparison conclusive.
5.2 Model performance for air quality variables
5.2.1 Overall performance
The results of the overall statistical evaluation for the online air quality simulations are presented in Fig. 5, and all labels and colors indicate that the SIs are the same as those for meteorological variables. In this figure and following figures, NP and NS are the number of publications and samples with SIs, respectively, and are summed up in Table B3. In Fig. 5a, the correlation between the simulated and observed PM2.5 concentrations from PSIs shows that in Asia coupled models performed relatively well for PM2.5 (mean R=0.63), but RMSE was between −87.60 and 80.90, and more than half of the samples of simulated PM2.5 were underestimated (mean MB µg m−3). Note that NS in Fig. 5c and d and Table B5 counted the samples of SIs provided by the simulations simultaneously with and without ARI. With the ARI turned on in the coupled models, modeled PM2.5 concentrations (limited papers with 15 samples) were improved somewhat. The mean R slightly increased from 0.71 to 0.72, and mean absolute MB decreased from 4.10 to 1.33 µg m−3 (Fig. 5c), but RMSE of PM2.5 concentrations slightly increased from 35.40 to 36.20 µg m−3. In short, PM2.5 with and without ARI agreed well with observations but was mostly underestimated, and PM2.5 bias simulated by models became overpredicted.
Compared with PM2.5, mean R (0.59) of O3 was relatively smaller (Fig. 5b). The statistical analysis also showed the most modeled O3 concentrations tended to be overestimated (76 % of the samples) with the average MB value of 8.05 µg m−3, and the mean RMSE value was 32.65 µg m−3. The 14 PSIs with ARI effects suggested that the correlation of O3 was slightly improved (mean R from 0.58 to 0.64), and the average RMSE and MB were decreased by 15.93 and 1.55 µg m−3, respectively (Fig. 5d). The collected studies indicated relatively poor performance of modeled O3 compared to PM2.5, but turning on ARI in coupled models improved O3 simulations somewhat.
In addition to the SIs analyzed above and similar to the surface meteorological variables, the NME (%) of PM2.5 and O3 is listed in Table B7. The limited studies with WRF-Chem and WRF-CMAQ indicated that the overall mean percent errors of PM2.5 and O3 were 47.63 % (from 29.55 % to 104.70 %) and 43.03 % (from 21.10 % to 127.00 %), respectively. With the ARI effects enabled in WRF-Chem in different seasons over the China domain, the NME (%) of PM2.5 increased slightly during most seasons, except during a spring month with little change (Zhang et al., 2018). Another study by Nguyen et al. (2019b) revealed that the NME (%) of PM2.5 and O3 simulated by WRF-CMAQ became a little worse in SEA compared to the simulations without ARI.
5.2.2 Comparisons of SIs for air quality using different coupled models
Figure 6 shows the SIs for PM2.5 and O3 from different coupled models, and only WRF-Chem and WRF-CMAQ simulations are discussed for the same reason as in Sect. 5.1.2. The modeled PM2.5 by WRF-CMAQ (mean R=0.69) outperformed WRF-Chem (mean R=0.62) to some extent (Fig. 6a), and the RMSE of modeled PM2.5 by WRF-CMAQ (33.24 µg m−3) was smaller than that by WRF-Chem (56.16 µg m−3). With respect to MB, WRF-CMAQ overestimated PM2.5 (mean MB µg m−3), but WRF-Chem slightly underestimated it (mean µg m−3) (Fig. 6c). Figure 6b shows that the modeled O3 by WRF-CMAQ (0.60) correlated better with observations than those by WRF-Chem (0.47), but the mean RMSE of modeled O3 (Fig. 6f) by WRF-Chem (27.13 µg m−3) was lower than that by WRF-CMAQ (35.19 µg m−3). It is seen in Fig. 6d that both WRF-CMAQ and WRF-Chem overestimated O3, with mean MBs of 11.98 and 7.21 µg m−3, respectively. Generally, the modeled PM2.5 and O3 were reproduced more reasonably by WRF-CMAQ than by WRF-Chem, even though there were many more samples available from WRF-Chem simulations than WRF-CMAQ simulations.
Aerosol feedbacks not only impact the performances of two-way coupled models but also the simulated meteorological and air quality variables to a certain extent. In this section, we collected and quantified the variations (Table S3) of these variables induced by ARI and/or ACI from the modeling studies in Asia. Due to limited sample sizes in the collected papers, the target variables only include radiative forcing, surface meteorological parameters (T2, RH2, SH2, and WS10), PBLH, cloud, precipitation, and PM2.5 and gaseous pollutants.
6.1 Impacts of aerosol feedbacks on meteorology
6.1.1 Radiative forcing
With regard to radiative forcing, most studies with two-way coupled models in Asia focused on the effects of dust aerosols (Dust), BC emitted from ARB (ARB_BC) and anthropogenic sources (Anthro_BC), and total anthropogenic aerosols (Anthro). Figure 7 presents the variations of simulated SWRF and LWRF at the bottom of the atmosphere (BOT), TOA, and in the ATM due to aerosol feedbacks, and detailed information on these variations is compiled in Table S5. In this figure, the color bars show the range of radiative forcing variations, and the black tick marks inside the color bars represent these variations extracted from all the collected papers. It should be noted that in this figure all the radiative forcing variations were plotted regardless of temporal resolutions of data reporting and simulation durations. Apparently in Asia, most studies targeted the SWRF variations induced by anthropogenic aerosols at the BOT that exhibited the largest differences ranging from −140.00 to −0.45 W m−2, with the most variations (88 % of samples) concentrated in the range of −50.00 to −0.45 W m−2. The SWRF variations due to anthropogenic aerosols in the ATM and at the TOA were −2.00 to +120.00 and −6.50 to 20.00 W m−2, respectively. There were many fewer studies reporting LWRF variations caused by anthropogenic aerosols, which ranged from −10.00 to +5.78, −1.91 to +3.94, and −4.26 to +1.21 W m−2 at the BOT, TOA, and in the ATM, respectively.
Considering BC from anthropogenic sources and ARB, they both led to positive SWRF at the TOA (with mean values of 2.69 and 7.55 W m−2, respectively) and in the ATM (with mean values of 11.70 and 25.45 W m−2, respectively) but negative SWRF at the BOT (with mean values of −18.43 and −14.39 W m−2, respectively). The responses of LWRF to Anthro_BC and ARB_BC at the BOT (in the ATM) on average were 4.01 and 0.72 W m−2 (−1.89 and −3.24 W m−2), respectively, and weak at the TOA (+0.92 and −0.53 W m−2, respectively). The SWRF variations induced by dust were in the range of −233.00 to −1.94, −140.00 to +25.70 W m−2, and +1.44 to +164.80 W m−2 at the BOT, TOA, and in the ATM, respectively. The LWRF variations caused by dust were the largest (with mean values of 22.83, +5.20, and −22.12 W m−2 at the BOT, TOA, and in the ATM, respectively) compared to the ones caused by anthropogenic aerosols and BC aerosols from anthropogenic sources and ARB.
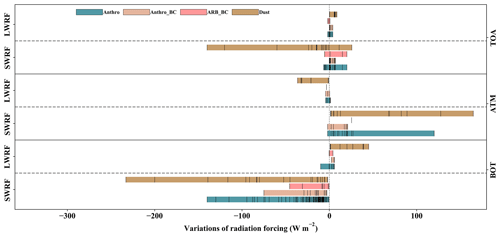
Figure 7Variations of shortwave and longwave radiative forcing (SWRF and LWRF) simulated by two-way coupled models (WRF-Chem, WRF-CMAQ, GRAPES-CUACE, WRF-NAQPMS, and GATOR-GCMOM) with aerosol feedbacks at the bottom and top of the atmosphere (BOT and TOA) as well as in the atmosphere (ATM) in Asia.
As shown in Fig. 7, SWRF variations at the BOT caused by total aerosols (sum of Anthro, Anthro_BC, ARB_BC, and dust) have been widely assessed in Asia. Therefore, we further analyzed their spatiotemporal distributions and inter-regional differences, which are displayed in Fig. 8. Figure 8a presents the SWRF variations over different areas of Asia (the acronyms used in Fig. 8 are listed in Table B1) at different timescales. In Asia, almost 41 % of the selected papers investigated SWRF in terms of its monthly variations, 36 % its hourly and daily variations, and 23 % its seasonal and yearly variations. Most studies reported that aerosol-induced SWRF variations primarily occurred in NCP, EA, China, and India. At the hourly scale, the range of SWRF decrease was from −350.00 to −5.90 W m−2 (mean value of −106.92 W m−2) during typical pollution episodes, and significant variations occurred in EA. The daily and monthly mean SWRF reductions varied from −73.71 to −5.58 W m−2 and −82.20 to −0.45 W m−2, respectively, with relatively large perturbations in NCP. At the seasonal and yearly scales, the SWRF changes ranged from −22.54 to −3.30 and −30.00 to −2.90 W m−2 with mean values of −11.28 and −11.82 W m−2, respectively, with EA as the most researched area.
To identify the differences of aerosol-induced SWRF variations between high- (Asia) and low-polluted regions (Europe and North America), their inter-regional comparisons are depicted in Fig. 8b. This figure does not include information about temporal resolutions of data reporting and durations of model simulations with ARI and/or ACI, but it intends to delineate the range of SWRF changes due to aerosol feedbacks. The SWRF variations fluctuated from −233.00 to −0.45, −100.00 to −1.00, and −600.00 to −1.00 W m−2 in Asia, Europe, and North America, respectively. It should be pointed out that the two extreme values were caused by dust (−233.00 W m−2) in Asia and wildfires (−600.00 W m−2) in North America. Overall, the median value of SWRF reductions due to ARI and/or ACI in Asia (−15.92 W m−2) was larger than those in North America (−10.50 W m−2) and Europe (−7.00 W m−2).
6.1.2 Temperature, wind speed, humidity, and PBLH
The impact of aerosols on radiation can influence the energy balance, which eventually alters other meteorological variables. The summary of aerosol-induced variations of T2, WS10, RH2, SH2, and PBLH in different regions of Asia as well as at different temporal scales is provided in Table 6. In this table, the minimum and maximum values were collected from the corresponding papers, and the mean values were calculated by adding all the variations from these papers and then dividing by the number of samples.
Overall, aerosol effects led to decreases in T2, WS10, and PBLH with average changes of −0.65 ∘C, −0.13 m s−1, and −60.70 m, respectively, as well as increases in humidity (mean ΔRH2 = 2.56 %) in most regions of Asia. On average, the hourly aerosol-induced changes in surface meteorological variables (T2, WS10, and RH2) and PBLH were the largest among the different timescales. At the hourly timescale, the mean variations of T2, WS10, RH2, and PBLH due to ARI and/or ACI were −1.85 ∘C, −0.32 m s−1, 4.60 %, and −165.84 m, respectively, with their absolute maximum values in EC, YRD, NCP, and NCP, respectively. Compared to variations at the hourly timescale, smaller daily variations of T2, WS10, RH2, and PBLH were caused by aerosol effects, and their mean values were −0.63 ∘C, −0.15 m s−1, +2.89 %, and −34.61 m, respectively. The largest daily variations of T2, WS10, RH2, and PBLH occurred in NCP, EC, EC, and SEC, respectively. For other timescales (monthly, seasonal, and yearly), the respective mean variations of T2, RH2, and PBLH induced by aerosol effects were comparable. However, the WS10 perturbations at the monthly timescale were about 2 to 3 times higher than those at the seasonal and yearly timescales. High variations at the monthly, seasonal, and yearly timescales were reported in NCP (T2, RH2, and PBLH), EA (T2, WS10, and PBLH), and PRD (T2 and PBLH). In addition, compared to T2 and PBLH, the aerosol-induced variations of WS10 and humidity were less revealed.
6.1.3 Cloud and precipitation
In the included publications, only a few papers focused on the effects of aerosol feedbacks on cloud properties (cloud fraction, LWP, ice water path - IWP, CDNC and cloud effective radius) and precipitation characteristics (amount, spatial distribution, peak occurrence, and onset time) using two-way coupled models in Asia, as shown in Table 7. In this table, the abbreviations representing aerosol emission sources (dust, ARB_BC, Anthro_BC, and Anthro) and regions in Asia are defined in Table B1. The plus and minus signs indicate increase and decrease, respectively.
The variations of cloud properties and precipitation characteristics induced by ARI and/or ACI are rather complex and not uniform in different parts of Asia and time periods. BC from both ARB and anthropogenic sources reduced cloud fraction through ARI and both ARI and ACI in several areas in China. ARI and/or ACI induced by anthropogenic aerosols could increase or decrease cloud fraction and affect cloud fraction differently in various atmospheric layers and time periods. Considering EA and subareas in China, anthropogenic aerosols tended to increase LWP through ARI and ACI as well as ACI alone but decrease LWP in some areas of SC (ARI and ACI) at noon and in the afternoon during summertime and NC (ACI) in winter. ARI and ACI induced by anthropogenic BC aerosols had negative effects on LWP except during daytime in CC. Dust aerosols increased both LWP and IWP through ACI in EA, which was reported only by one study. The increase (decrease) in CDNC caused by the ARI and ACI effects of anthropogenic (anthropogenic BC) aerosols in EC during summertime was reported. Through ACI, anthropogenic aerosols affected CDNC positively in EA and China. Compared to anthropogenic aerosols, dust aerosols could have much larger positive impacts on CDNC via ACI in springtime over EA. The ACI effects of anthropogenic aerosols reduced the cloud effective radius over China (January) and EA (July).
Among all the variables describing cloud properties and precipitation characteristics, the variations of precipitation amount were studied the most using two-way coupled models in Asia. How turning on ARI and/or ACI in coupled models can change precipitation amount is not unidirectional and depends on many factors, including different aerosol sources, areas, emission levels, atmospheric humidity, precipitation types, seasons, and time of a day. Under high emission levels as well as at slightly different humidity levels of RH >85 % with increasing emissions, the ACI effects of anthropogenic aerosols induced precipitation increase in the MRYR area of China. Over the same area, precipitation decreased due to the ACI effects of anthropogenic aerosols with low emission levels and RH <80 %. In PRD, wintertime precipitation was enhanced by the ACI effects of anthropogenic aerosols but inhibited by ARI. In South Korea (SK), summertime precipitation was both enhanced and inhibited by the ACI and ARI effects of anthropogenic aerosols. In locations upwind (downwind) of Beijing, rainfall amount was raised (lowered) by the ARI effects of anthropogenic aerosols but lowered (raised) by ACI. Both ARI and ACI induced by anthropogenic aerosols had positive impacts on total, convective, and stratiform rain in India during the summer season, and the increase in convective rain was larger than those of stratiform. Summertime precipitation amounts could be enhanced or inhibited at various subareas inside simulation domains over India, China, and Korea and during daytime or nighttime due to ARI and ACI of anthropogenic aerosols. Over China, dust-induced ACI decreased (increased) springtime precipitation in CC (western part of NC), and over India, dust aerosols from local sources and ME had positive impacts on total, convective, and stratiform rain through ARI and ACI. Simulations in India also revealed that precipitation could be increased in some subareas but decreased in another, and absorptive (non-absorptive) dust enhanced (inhibited) summertime precipitation via ARI and ACI. The ARI (ACI) effects of BC from ARB caused precipitation reduction (increase) in SEC, but CAs emitted from ARB (ARB_CAs) caused rainfall enhancement in Myanmar. During the pre-monsoon season, ARI induced by anthropogenic BC could lead to +42 % variations of precipitation in northeastern India (NEI) and −5 % to −8 % in southern India (SI) during the monsoon season. Considering both ARI and ACI effects, BC from ARB and sea salt aerosols enhanced or inhibited precipitation in different parts of India, and BC from anthropogenic sources enhanced (inhibited) nighttime (daytime) rainfall in CC (NC and SC) at the rate of +1 to +4 mm d−1 (−2 to −6 mm d−1) during the summer season. With respect to spatial variations, 6.5 % larger rainfall area in PRD was caused by ARI and ACI effects under 50 % reduced anthropogenic emissions. ACI induced by anthropogenic aerosols tended to delay the peak occurrence time and onset time of precipitation by 1 to 9 h in China and South Korea.
Table 7Summary of changes in cloud properties and precipitation characteristics due to aerosol feedbacks simulated by two-way coupled models (WRF-Chem, WRF-CMAQ, GRAPES-CUACE, WRF-NAQPMS, and GATOR-GCMOM) in Asia.
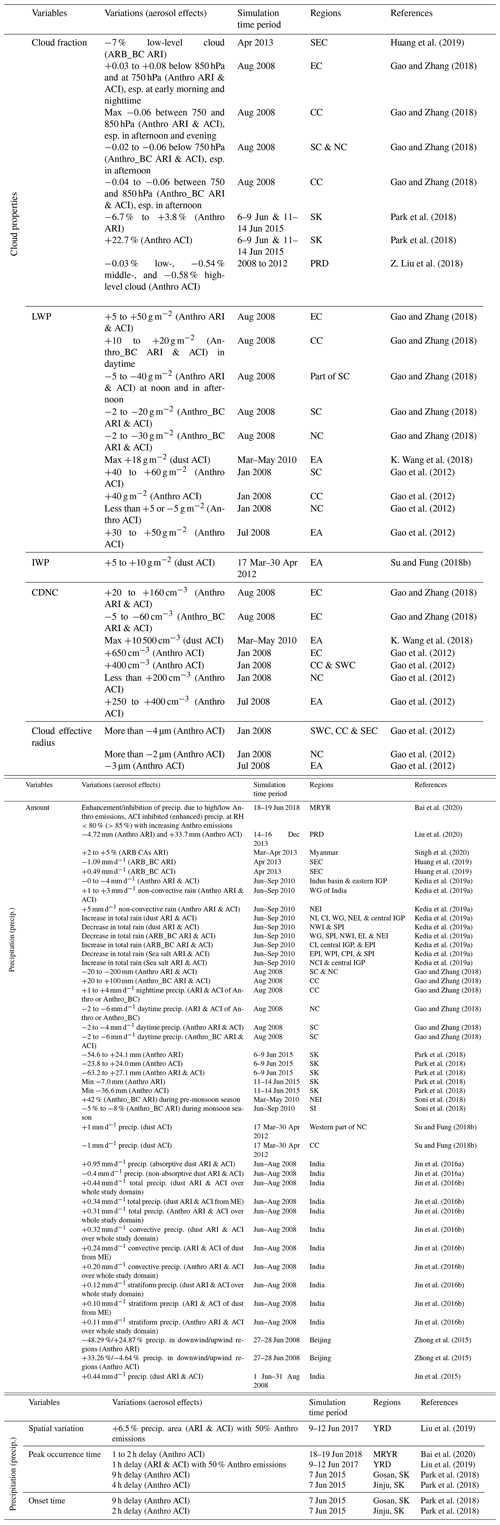
Note – SEC: southeastern China, EC: eastern China, CC: central China, SC: southern China, NC: northern China, SK: South Korea, PRD: Pearl River Delta, EA: East Asia, SWC: southwestern China, MRYR: Middle reaches of the Yangtze River, IGP: Indo-Gangetic Plain, WG: western Ghats, NEI: northeastern India, NI: northern India, CI: central India, NWI: northwestern India, SPI: southern peninsula of India, EI: eastern India, EPI: eastern peninsula of India, WPI: western peninsula of India, CPI: central peninsula of India, NCI: northern central India, SI: southern India.
6.2 Impacts of aerosol feedbacks on air quality
Aerosol effects not only gave rise to changes in meteorological variables but also air quality. Table 8 (the minimum, maximum, and mean values were defined in the same way as in Table 6) summarizes the variations of atmospheric pollutant concentrations induced by aerosol effects in different regions of Asia and at different timescales. In Asia, most modeling studies with coupled models targeted the impacts of aerosol feedbacks on surface PM2.5 and O3 concentrations, with only a few focusing on other gaseous pollutants.
Simulation results showed that turning on aerosol feedbacks in coupled models generally made PM2.5 concentrations increase in different regions of Asia at various timescales, which stemmed from a decrease in shortwave radiation, T2, WS10, and PBLH and an increase in RH2. Some studies did show negative impacts of aerosol effects on hourly, daily, and seasonal PM2.5 at some areas that could be attributed to ACI effects, changes in transport and dispersion patterns, reductions in humidity levels, and secondary aerosol formation (B. Zhang et al., 2015; Yang et al., 2017; Zhan et al., 2017; K. Wang et al., 2018). Similar to the perturbations of surface meteorological variables due to aerosol effects, the hourly PM2.5 variations and the range were the largest compared to those at other timescales. The largest PM2.5 increases were reported in NCP, SEC, EA, SEA, and PRD at the hourly, daily, monthly, seasonal, and yearly timescales with average values of 23.48, 14.73, 16.50, 1.12, and 2.90 µg m−3, respectively.
In addition to PM2.5, gaseous pollutants (O3, NO2, SO2, CO, and NH3) are impacted by ARI and/or ACI effects as well. As shown in Table 8, general reductions of ozone concentrations were reported in Asia across all the modeling domains and timescales based on coupled models' simulations. However, the influences of aerosol feedbacks on atmospheric dynamics and stability, as well as photochemistry (photolysis rate and ozone formation regimes) could make ozone concentrations increase somewhat in summer months or during the wet season (Xing et al., 2017; Jung et al., 2019; Nguyen et al., 2019b). The largest hourly, daily, monthly, seasonal, and annual variations of O3 occurred in YRD (−32.80 µg m−3), EC (−5.97 µg m−3), China (−23.90 µg m−3), EA (−4.48 µg m−3), and EA (−2.76 µg m−3). Along with reduced O3 due to ARI and/or ACI, NO2 concentrations were enhanced with average changes of +12.30 µg m−3 (YRD) at the hourly scale and +0.66 µg m−3 (EA) at both the seasonal and yearly scales, which could be attributed to slower photochemical reactions, strengthened atmospheric stability, and O3 titration (Nguyen et al., 2019b). Regarding other gaseous pollutants, limited studies pointed out that daily and annual SO2 concentrations increased in NEA and EA due to lower PBLH induced by the ARI effects of anthropogenic aerosols (Jung et al., 2019; Nguyen et al., 2019b). The seasonal SO2 reduction was rather large, which is related to higher PBLH induced by the ACI effects of dust aerosols in the NCP area of EA (K. Wang et al., 2018). The slight increase in seasonal SO2 was reported in the whole domain of EA due to lower PBLH caused by ARI effects of anthropogenic aerosols (Nguyen et al., 2019b). There was only one study that depicted an increased CO (NH3) concentration in EC (NEA) due to both the ARI and ACI (ARI) effects of anthropogenic aerosols, but these results may not be conclusive.
Two-way coupled models have been applied in the US and Europe extensively and then in Asia due to frequent occurrences of severe air pollution events accompanied by rapid economic growth in the region. Until now, no comprehensive study has been conducted to elucidate the recent advances in two-way coupled models' applications in Asia. This paper provides a critical overview of the current status and research focuses of related modeling studies using two-way coupled models in Asia between 2010 and 2019, and it summarizes the effects of aerosol feedbacks on meteorological and air quality variables from these studies.
By systematically searching peer-reviewed publications with several scientifically based search engines along with a variety of keyword combinations and applying certain selection criteria, 160 relevant papers were identified. Our bibliometric analysis results (as schematically illustrated in Fig. 9) showed that in Asia, research activities with two-way coupled models have increased gradually in the past decade, and five two-way coupled models (WRF-Chem, WRF-CMAQ, WRF-NAQPMS, GRAPES-CUACE, and GATOR-GCMOM) were extensively utilized to explore the ARI and/or ACI effects in Asia focusing on several high aerosol loading areas (e.g., EA, India, China, and NCP) during wintertime and/or severe pollution events, with fewer investigations looking into other areas and seasons with low pollution levels. Among the 160 papers, nearly 82 % of them focused on ARI (72 papers) and both ARI and ACI effects (60 papers), but papers only considering ACI effects were relatively limited. The ARI and/or ACI effects of natural mineral dust, BC and BrC from anthropogenic sources, and BC from ARB were mostly investigated, while a few studies quantitatively assessed the health impacts induced by aerosol effects.
Meta-analysis results revealed that enabling aerosol effects in two-way coupled models could improve their simulation and/or forecast capabilities of meteorology and air quality in Asia, but a wide range of differences occurred among the previous studies, perhaps due to various model configurations (selections of model versions and parameterization schemes) and large uncertainties related to ACI processes and their treatments in models. Compared to the US and Europe, the aerosol-induced decrease in the shortwave radiative forcing was larger because of higher air pollution levels in Asia. The overall decrease (increase) in T2, WS10, PBLH, and O3 (RH2, PM2.5, and other gaseous pollutant concentrations) caused by ARI and/or ACI effects was reported from the modeling studies using two-way coupled models in Asia. The ranges of aerosol-induced variations of T2, PBLH, PM2.5, and O3 concentrations were larger than other meteorological and air quality variables. For variables of CO, SO2, NO2, and NH3, reliable estimates could not be obtained due to insufficient numbers of samples in past studies.
Even though noticeable progress toward the application of two-way coupled meteorology and air quality models has been made in Asia and the world during the last decade, several limitations are still presented. Enabling aerosol feedbacks leads to higher computational cost compared to offline models, but this shortcoming can be overcome with the new developments of cluster computing technology (i.e., GPU-accelerated computing and cloud computing; GPU – Graphics Processing Unit). The latest advances in the measurements and research of cloud properties, precipitation characteristics, and physiochemical characteristics of aerosols that play pivotal roles in CCN or IN activation mechanisms can guide the improvements and enhancements in two-way coupled models, especially to abate the uncertainties in simulating ACI effects. Special attention needs to be paid to assessing the accuracies of different methodologies in terms of ARI and ACI calculations in two-way coupled models in Asia and other regions. Besides the five two-way coupled models mentioned in this paper, more models capable of simulating aerosol feedbacks (such as WRF-CHIMERE and WRF-GEOS-Chem) have become available, and projects covering more comprehensive intercomparisons of these coupled models should be conducted in Asia. Future assessments of the ARI and/or ACI effects should pay extra attention to their impacts on dry and wet deposition simulated by two-way coupled models. So far, the majority of two-way coupled model simulations and evaluations have focused on episodic air pollution events occurring in certain areas, and therefore their long-term applications and evaluations are necessary; their real-time forecasting capabilities should be explored as well.
Table B2The compiled number of publications (NP) and number of samples (NS) for papers providing statistical indices (SIs) of meteorological variables.
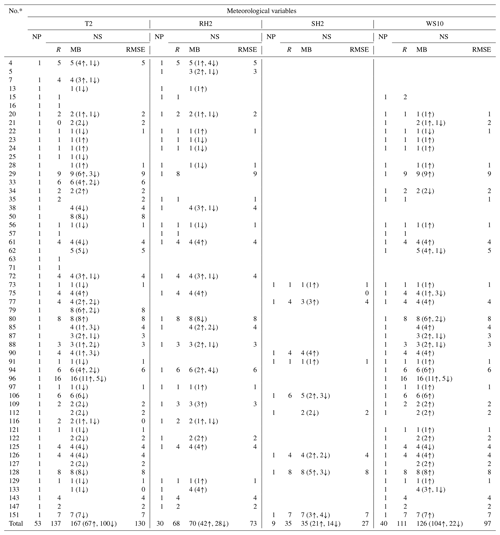
Note that “No.*” is consistent with “No.” in Table 1, and ↑ and ↓ mark overestimations and underestimations of variables, respectively, along with their number of samples.
Table B3The compiled number of publications (NP) and number of samples (NS) for papers providing statistical indices (SIs) of air quality variables.
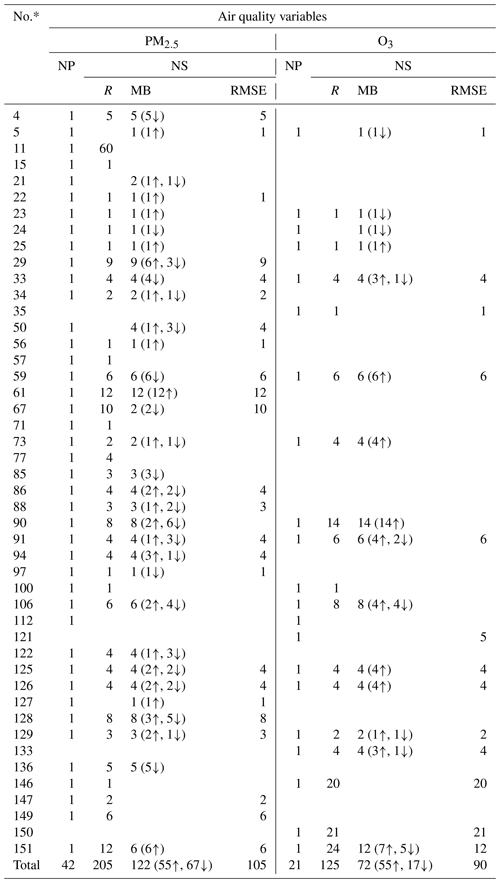
Note that “No.*” is consistent with “No.” in Table 1, and ↑ and ↓ mark overestimations and underestimations of variables, respectively, along with their number of samples.
Table B4The compiled number of publications (NP) and number of samples (NS) for papers simultaneously providing the statistical indices (SIs) of meteorological variables simulated by coupled models (WRF-Chem, WRF-CMAQ, GRAPES-CUACE, WRF-NAQPMS, and GATOR-GCMOM) with and without ARI.
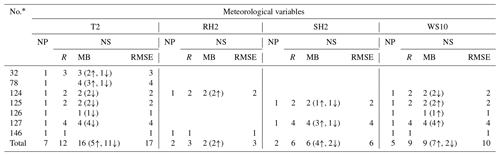
Note that “No.*” is consistent with “No.” in Table 1, and ↑ and ↓ mark overestimations and underestimations of variables, respectively, along with their number of samples.
Table B5The compiled number of publications (NP) and number of samples (NS) for papers simultaneously providing the statistical indices (SIs) of air quality variables simulated by coupled models (WRF-Chem, WRF-CMAQ, GRAPES-CUACE, WRF-NAQPMS, and GATOR-GCMOM) with and without ARI.
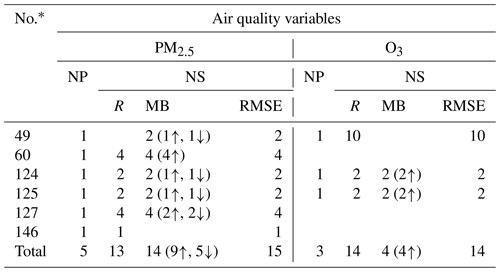
Note that “No.*” is consistent with “No.” in Table 1, and ↑ and ↓ mark overestimations and underestimations of variables, respectively, along with their number of samples.
Table B6Description of refractive indices and radiation schemes used in the WRF-Chem and WRF-CMAQ in Asia.
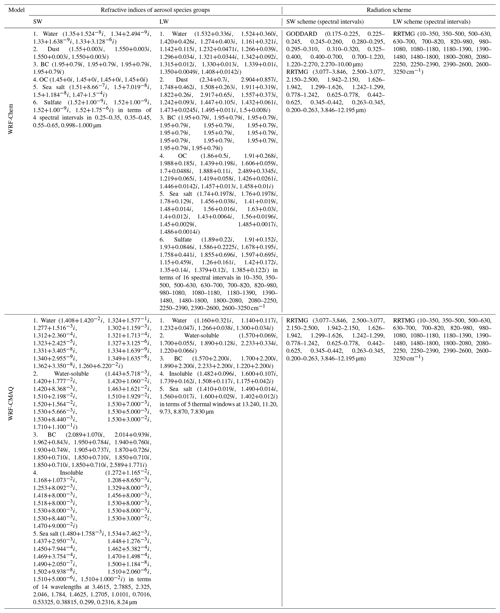
C1 Comparisons of SIs at different temporal scales for meteorology
To probe the model performance of simulated T2, RH2, SH2, and WS2 at different temporal scales, the SIs of these meteorological variables from PSIs were grouped according to the simulation time (yearly, seasonal, monthly, and daily) and plotted in Fig. C1. Note that the seasonal results contained SIs values from simulations lasting more than 1 month and less than or equal to 3 months. Here in Fig. C1, NP and NS were the number of PSIs and samples with SIs at different timescales, respectively, and also their total values were the same as the ones listed in Table S2. The correlations between simulated and observed T2 (Fig. C1a) at the seasonal (mean R=0.97 with the smallest sample size), yearly (0.91), and monthly (0.90) scales were stronger than that at the daily scale (0.87), indicating that long-term simulations of T2 were well reproduced by coupled models. As shown in Fig. C1e, the T2 underestimation mentioned above (Fig. 3a) also appeared in the seasonal, monthly, and yearly simulations (average MB , −0.15, and −0.34 ∘C, respectively), but the daily T2 values were overestimated (average MB =0.07 ∘C). It should be noted that T2 at the monthly scale was underpredicted mainly during winter months (16 samples). Regarding the mean RMSE, its value (Fig. C1i) at the daily scale was the largest (0.97 ∘C) in comparison with that at the other temporal scales.
Given that no SIs were available for RH2 at the seasonal scale, results at other timescales were discussed here. Figure C1b presents simulated RH2 at the daily scale with the best correlation coefficient (mean R=0.74), followed by those at the monthly (0.73) and yearly (0.71) scales. Except overestimation (average MB =3.6 %) at the yearly scale (Fig. C1f), modeled RH2 values were underestimated at the monthly (average MB %) and daily (average MB %) scales. Therefore, coupled models calculated RH2 reasonably well in short-term simulations. However, at the daily scale, RMSE of modeled RH2 (Fig. C1j) showed a relatively large fluctuation ranging from 6.2 % to 21.3 %.
Lacking SIs for SH2 at the daily scale, only those at other timescales were compared. Even though NP and NS were very limited, the modeled SH2 (Fig. C1c) exhibited especially good correlation with observations with the mean R values exceeding 0.95 at the yearly, seasonal, and monthly scales (0.99, 0.97, and 0.96, respectively) but had the largest mean RMSE (2.09 g kg−1) at the yearly scale (Fig. C1k). Also, both overestimations and underestimations of modeled SH2 (Fig. C1g) were reported at different timescales with average MB values as 0.15, −0.02, and −0.14 g kg−1 for yearly, seasonal, and monthly simulations, respectively. Generally, the long-term simulations of SH2 agreed better with observations than the short-term ones.
As seen in Fig. C1d, the modeled WS10 at the monthly scale (mean R=0.68) correlated with observations better than that at the daily, yearly, and seasonal scales (mean R=0.62, 0.48, and 0.46, respectively). The simulations at all temporal scales tended to overestimate WS10 compared against observations (Fig. C1h), and their average MB values were 0.80 m s−1 (seasonal), 0.86 m s−1 (monthly), 0.64 m s−1 (yearly), and 0.62 m s−1 (daily). The short-term simulations of WS10 better matched observations compared to the long-term ones. At the same time, the largest mean RMSE (1.79 m s−1) of simulated WS10 (Fig. C1l) appeared at the seasonal scale.
C2 Comparisons of SIs at different temporal scales for air quality
Figure C2 depicts the SIs of simulated PM2.5 and O3 at yearly, seasonal, monthly, and daily scales. The correlation between simulated and observed PM2.5 (Fig. C2a) at the monthly scale (mean R=0.68) was largest compared to those at the yearly (0.64), seasonal (0.59), and daily (0.57) scales. All the simulated PM2.5 values were underestimated, with the average daily, monthly, seasonal, and yearly MB as −4.13, −1.46, −0.28, and −1.89 µg m−3, respectively (Fig. C2c). As displayed in Fig. C2e, the mean RMSE at the monthly scale was the largest (61.57 µg m−3).
Regarding correlation between simulated and observed O3 (Fig. C2b), it was the best at the daily scale (mean R=0.77). Modeled O3 values were overestimated at the seasonal (average MB µg m−3), monthly (average MB µg m−3), and yearly (average MB µg m−3) scales but underestimated at the daily scale (average MB µg m−3) (Fig. C2d). Note that no RMSE for O3 simulation was available at the daily scale, and the RMSE at the yearly scale (Fig. C2f) had a relatively large fluctuation ranging from 0.21 to 71 µg m−3. Therefore, coupled models' calculated O3 matched observations in short-term simulations well.
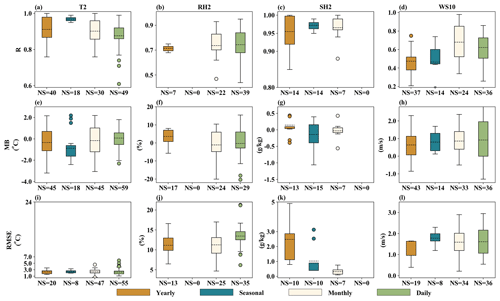
Figure C1The statistical indices of modeled meteorological variables at different temporal scales (yearly, seasonal, monthly, and daily) from past studies in Asia.
The related dataset can be downloaded from https://doi.org/10.5281/zenodo.6141615 (Gao et al., 2022), and this dataset includes basic information (Table S1), performance metrics (Table S2), quantitative effects of aerosol feedbacks on meteorological and air quality variables (Table S3), model configuration and setup (Table S4), and aerosol-induced variations of simulated shortwave and longwave radiative forcing (Table S5) extracted from collected studies of applications of two-way coupled meteorology and air quality models in Asia.
The supplement related to this article is available online at: https://doi.org/10.5194/acp-22-5265-2022-supplement.
CG, AX, XZ, and QT carried out the data collection, related analysis, figure plotting, and paper writing. HZ, SZ, GY, and MZ were involved with the original research plan and made suggestions for the paper writing.
The contact author has declared that neither they nor their co-authors have any competing interests.
Publisher's note: Copernicus Publications remains neutral with regard to jurisdictional claims in published maps and institutional affiliations.
The authors are very grateful to many researchers who provided detailed information on the two-way coupled models and related research work. The list includes but is not limited to Xueshun Chen, Zifa Wang, Yi Gao, Meigen Zhang, and Baozhu Ge (Institute of Atmospheric Physics, Chinese Academy of Sciences); Chunhong Zhou (Chinese Academy of Meteorological Sciences); Yang Zhang (Northeastern University); Mark Zachary Jacobson (Stanford University); Tianliang Zhao (Nanjing University of Information Science & Technology); Xin Huang (Nanjing University); Chun Zhao (University of Science and Technology of China); Junhua Yang and Shichang Kang (Northwest Institute of Eco-Environment and Resources, Chinese Academy of Sciences); Sachin Ghude (Ministry of Earth Sciences Government of India); and Luke Conibear (University of Leeds). We would also like to express our deepest appreciation to the editor, James Allan, and two anonymous reviewers for their constructive comments and suggestions, which helped to improve the quality and readability of this article.
This study was financially sponsored by the National Key Research and Development Program of China (grant nos. 2017YFC0212304 and 2019YFE0194500), the Talent Program of Chinese Academy of Sciences, and the National Natural Science Foundation of China (grant nos. 42171142, 41771071, and 41571063).
This paper was edited by James Allan and reviewed by two anonymous referees.
Abdul-Razzak, H. and Ghan, S. J.: A parameterization of aerosol activation 3. Sectional representation, J. Geophys. Res.-Atmos., 107, AAC 1-1–AAC 1-6, https://doi.org/10.1029/2001JD000483, 2002.
Ackerman, A. S., Toon, O. B., Stevens, D. E., Heymsfield, A. J., Ramanathan, V., and Welton, E. J.: Reduction of tropical cloudiness by soot, Science, 288, 1042–1047, https://doi.org/10.1126/science.288.5468.1042, 2000.
Ahmadov, R., McKeen, S. A., Robinson, A. L., Bahreini, R., Middlebrook, A. M., De Gouw, J. A., Meagher, J., Hsie, E., Edgerton, E., and Shaw, S.: A volatility basis set model for summertime secondary organic aerosols over the eastern United States in 2006, J. Geophys. Res.-Atmos., 117, D06301, https://doi.org/10.1029/2011JD016831, 2012.
Albrecht, B. A.: Aerosols, cloud microphysics, and fractional cloudiness, Science, 245, 1227–1230, https://doi.org/10.1126/science.245.4923.1227, 1989.
An, Z., Huang, R.-J., Zhang, R., Tie, X., Li, G., Cao, J., Zhou, W., Shi, Z., Han, Y., and Gu, Z.: Severe haze in northern China: A synergy of anthropogenic emissions and atmospheric processes, P. Natl. Acad. Sci. USA, 116, 8657–8666, https://doi.org/10.1073/pnas.1900125116, 2019.
Andreae, M. O. and Rosenfeld, D.: Aerosol-cloud-precipitation interactions. Part 1. The nature and sources of cloud-active aerosols, Earth-Science Rev., 89, 13–41, https://doi.org/10.1016/j.earscirev.2008.03.001, 2008.
Appel, K. W., Pouliot, G. A., Simon, H., Sarwar, G., Pye, H. O. T., Napelenok, S. L., Akhtar, F., and Roselle, S. J.: Evaluation of dust and trace metal estimates from the Community Multiscale Air Quality (CMAQ) model version 5.0, Geosci. Model Dev., 6, 883–899, https://doi.org/10.5194/gmd-6-883-2013, 2013.
Appel, K. W., Napelenok, S. L., Foley, K. M., Pye, H. O. T., Hogrefe, C., Luecken, D. J., Bash, J. O., Roselle, S. J., Pleim, J. E., Foroutan, H., Hutzell, W. T., Pouliot, G. A., Sarwar, G., Fahey, K. M., Gantt, B., Gilliam, R. C., Heath, N. K., Kang, D., Mathur, R., Schwede, D. B., Spero, T. L., Wong, D. C., and Young, J. O.: Description and evaluation of the Community Multiscale Air Quality (CMAQ) modeling system version 5.1, Geosci. Model Dev., 10, 1703–1732, https://doi.org/10.5194/gmd-10-1703-2017, 2017.
Appel, K. W., Bash, J. O., Fahey, K. M., Foley, K. M., Gilliam, R. C., Hogrefe, C., Hutzell, W. T., Kang, D., Mathur, R., Murphy, B. N., Napelenok, S. L., Nolte, C. G., Pleim, J. E., Pouliot, G. A., Pye, H. O. T., Ran, L., Roselle, S. J., Sarwar, G., Schwede, D. B., Sidi, F. I., Spero, T. L., and Wong, D. C.: The Community Multiscale Air Quality (CMAQ) model versions 5.3 and 5.3.1: system updates and evaluation, Geosci. Model Dev., 14, 2867–2897, https://doi.org/10.5194/gmd-14-2867-2021, 2021.
Archer-Nicholls, S., Lowe, D., Lacey, F., Kumar, R., Xiao, Q., Liu, Y., Carter, E., Baumgartner, J., and Wiedinmyer, C.: Radiative effects of residential sector emissions in China: sensitivity to uncertainty in black carbon emissions, J. Geophys. Res.-Atmos., 124, 5029–5044, https://doi.org/10.1029/2018JD030120, 2019.
Ashrafi, K., Motlagh, M. S., and Neyestani, S. E.: Dust storms modeling and their impacts on air quality and radiation budget over Iran using WRF-Chem, Air Qual. Atmos. Heal., 10, 1059–1076, https://doi.org/10.1007/s11869-017-0494-8, 2017.
Bai, Y., Qi, H., Zhao, T., Zhou, Y., Liu, L., Xiong, J., Zhou, Z., and Cui, C.: Simulation of the responses of rainstorm in the Yangtze River Middle Reaches to changes in anthropogenic aerosol emissions, Atmos. Environ., 220, 117081, https://doi.org/10.1016/j.atmosenv.2019.117081, 2020.
Baklanov, A., Schlünzen, K., Suppan, P., Baldasano, J., Brunner, D., Aksoyoglu, S., Carmichael, G., Douros, J., Flemming, J., Forkel, R., Galmarini, S., Gauss, M., Grell, G., Hirtl, M., Joffre, S., Jorba, O., Kaas, E., Kaasik, M., Kallos, G., Kong, X., Korsholm, U., Kurganskiy, A., Kushta, J., Lohmann, U., Mahura, A., Manders-Groot, A., Maurizi, A., Moussiopoulos, N., Rao, S. T., Savage, N., Seigneur, C., Sokhi, R. S., Solazzo, E., Solomos, S., Sørensen, B., Tsegas, G., Vignati, E., Vogel, B., and Zhang, Y.: Online coupled regional meteorology chemistry models in Europe: current status and prospects, Atmos. Chem. Phys., 14, 317–398, https://doi.org/10.5194/acp-14-317-2014, 2014.
Baró, R., Jiménez-Guerrero, P., Balzarini, A., Curci, G., Forkel, R., Grell, G., Hirtl, M., Honzak, L., Langer, M., and Pérez, J. L.: Sensitivity analysis of the microphysics scheme in WRF-Chem contributions to AQMEII phase 2, Atmos. Environ., 115, 620–629, https://doi.org/10.1016/j.atmosenv.2015.01.047, 2015.
Barth, M. C., Rasch, P. J., Kiehl, J. T., Benkovitz, C. M., and Schwartz, S. E.: Sulfur chemistry in the NCAR CCM: Description, evaluation, features and sensitivity to aqueous chemistry, J. Geophys. Res., 105, 1387–1415, https://doi.org/10.1029/1999JD900773, 2000.
Bauer, P., Thorpe, A., and Brunet, G.: The quiet revolution of numerical weather prediction, Nature, 525, 47–55, https://doi.org/10.1038/nature14956, 2015.
Beard, K. V.: Terminal velocity and shape of cloud and precipitation drops aloft, J. Atmos. Sci., 33, 851–864, https://doi.org/10.1175/1520-0469(1976)033<0851:TVASOC>2.0.CO;2, 1976.
Bei, N., Wu, J., Elser, M., Feng, T., Cao, J., El-Haddad, I., Li, X., Huang, R., Li, Z., Long, X., Xing, L., Zhao, S., Tie, X., Prévôt, A. S. H., and Li, G.: Impacts of meteorological uncertainties on the haze formation in Beijing–Tianjin–Hebei (BTH) during wintertime: a case study, Atmos. Chem. Phys., 17, 14579–14591, https://doi.org/10.5194/acp-17-14579-2017, 2017.
Beig, G., Chate, D. M., Ghude, S. D., Mahajan, A. S., Srinivas, R., Ali, K., Sahu, S. K., Parkhi, N., Surendran, D., and Trimbake, H. R.: Quantifying the effect of air quality control measures during the 2010 Commonwealth Games at Delhi, India, Atmos. Environ., 80, 455–463, https://doi.org/10.1016/j.atmosenv.2013.08.012, 2013.
Bellouin, N., Jones, A., Haywood, J., and Christopher, S. A.: Updated estimate of aerosol direct radiative forcing from satellite observations and comparison against the Hadley Centre climate model, J. Geophys. Res.-Atmos., 113, D10205, https://doi.org/10.1029/2007JD009385, 2008.
Benas, N., Meirink, J. F., Karlsson, K.-G., Stengel, M., and Stammes, P.: Satellite observations of aerosols and clouds over southern China from 2006 to 2015: analysis of changes and possible interaction mechanisms, Atmos. Chem. Phys., 20, 457–474, https://doi.org/10.5194/acp-20-457-2020, 2020.
Bennartz, R., Fan, J., Rausch, J., Leung, L. R., and Heidinger, A. K.: Pollution from China increases cloud droplet number, suppresses rain over the East China Sea, Geophys. Res. Lett., 38, L09704, https://doi.org/10.1029/2011GL047235, 2011.
Bharali, C., Nair, V. S., Chutia, L., and Babu, S. S.: Modeling of the effects of wintertime aerosols on boundary layer properties over the Indo Gangetic Plain, J. Geophys. Res.-Atmos., 124, 4141–4157, https://doi.org/10.1029/2018JD029758, 2019.
Bhattacharya, A., Chakraborty, A., and Venugopal, V.: Role of aerosols in modulating cloud properties during active–break cycle of Indian summer monsoon, Clim. Dynam., 49, 2131–2145, https://doi.org/10.1007/s00382-016-3437-4, 2017.
Binkowski, F. S. and Roselle, S. J.: Models-3 Community Multiscale Air Quality (CMAQ) model aerosol component 1. Model description, J. Geophys. Res.-Atmos., 108, 4183, https://doi.org/10.1029/2001JD001409, 2003.
Binkowski, F. S. and Shankar, U.: The regional particulate matter model: 1. Model description and preliminary results, J. Geophys. Res.-Atmos., 100, 26191–26209, https://doi.org/10.1029/95JD02093, 1995.
Bollasina, M. A., Ming, Y., and Ramaswamy, V.: Anthropogenic aerosols and the weakening of the South Asian summer monsoon, Science, 334, 502–505, https://doi.org/10.1126/science.1204994, 2011.
Bran, S. H., Jose, S., and Srivastava, R.: Investigation of optical and radiative properties of aerosols during an intense dust storm: A regional climate modeling approach, J. Atmos. Solar-Terr. Phy., 168, 21–31, https://doi.org/10.1016/j.jastp.2018.01.003, 2018.
Briant, R., Tuccella, P., Deroubaix, A., Khvorostyanov, D., Menut, L., Mailler, S., and Turquety, S.: Aerosol–radiation interaction modelling using online coupling between the WRF 3.7.1 meteorological model and the CHIMERE 2016 chemistry-transport model, through the OASIS3-MCT coupler, Geosci. Model Dev., 10, 927–944, https://doi.org/10.5194/gmd-10-927-2017, 2017.
Brunekreef, B. and Holgate, S. T.: Air pollution and health, Lancet, 360, 1233–1242, https://doi.org/10.1016/S0140-6736(02)11274-8, 2002.
Brunner, D., Savage, N., Jorba, O., Eder, B., Giordano, L., Badia, A., Balzarini, A., Baro, R., Bianconi, R., and Chemel, C.: Comparative analysis of meteorological performance of coupled chemistry-meteorology models in the context of AQMEII phase 2, Atmos. Environ., 115, 470–498, https://doi.org/10.1016/j.atmosenv.2014.12.032, 2015.
Byun, D. and Schere, K. L.: Review of the governing equations, computational algorithms, and other components of the Models-3 Community Multiscale Air Quality (CMAQ) modeling system, Appl. Mech. Rev., 59, 51–77, https://doi.org/10.1115/1.2128636, 2006.
Campbell, P., Zhang, Y., Yahya, K., Wang, K., Hogrefe, C., Pouliot, G., Knote, C., Hodzic, A., San Jose, R., and Perez, J. L.: A multi-model assessment for the 2006 and 2010 simulations under the Air Quality Model Evaluation International Initiative (AQMEII) phase 2 over North America: Part I. Indicators of the sensitivity of O3 and PM2.5 formation regimes, Atmos. Environ., 115, 569–586, https://doi.org/10.1016/j.atmosenv.2014.12.026, 2015.
Campbell, P., Zhang, Y., Wang, K., Leung, R., Fan, J., Zheng, B., Zhang, Q., and He, K.: Evaluation of a multi-scale WRF-CAM5 simulation during the 2010 East Asian Summer Monsoon, Atmos. Environ., 169, 204–217, https://doi.org/10.1016/j.atmosenv.2017.09.008, 2017.
Carlton, A. G., Bhave, P. V, Napelenok, S. L., Edney, E. O., Sarwar, G., Pinder, R. W., Pouliot, G. A., and Houyoux, M.: Model representation of secondary organic aerosol in CMAQv4.7, Environ. Sci. Technol., 44, 8553–8560, https://doi.org/10.1021/es100636q, 2010.
Casazza, M., Lega, M., Liu, G., Ulgiati, S., and Endreny, T. A.: Aerosol pollution, including eroded soils, intensifies cloud growth, precipitation, and soil erosion: A review, J. Clean. Prod., 189, 135–144, https://doi.org/10.1016/j.jclepro.2018.04.004, 2018.
Chang, S.: Characteristics of aerosols and cloud condensation nuclei (CCN) over China investigated by the two-way coupled WRFCMAQ air quality model, MS thesis, College of Environmental and Resource Sciences, Zhejiang University, China, 79 pp., 2018.
Chapman, E. G., Gustafson Jr., W. I., Easter, R. C., Barnard, J. C., Ghan, S. J., Pekour, M. S., and Fast, J. D.: Coupling aerosol-cloud-radiative processes in the WRF-Chem model: Investigating the radiative impact of elevated point sources, Atmos. Chem. Phys., 9, 945–964, https://doi.org/10.5194/acp-9-945-2009, 2009.
Chen, D.-S., Ma, X., Xie, X., Wei, P., Wen, W., Xu, T., Yang, N., Gao, Q., Shi, H., and Guo, X.: Modelling the effect of aerosol feedbacks on the regional meteorology factors over China, Aerosol. Air. Qual. Res., 15, 1559–1579, https://doi.org/10.4209/aaqr.2014.11.0272, 2015.
Chen, J., Li, C., Ristovski, Z., Milic, A., Gu, Y., Islam, M. S., Wang, S., Hao, J., Zhang, H., and He, C.: A review of biomass burning: Emissions and impacts on air quality, health and climate in China, Sci. Total Environ., 579, 1000–1034, https://doi.org/10.1016/j.scitotenv.2016.11.025, 2017.
Chen, L., Gao, Y., Zhang, M., Fu, J. S., Zhu, J., Liao, H., Li, J., Huang, K., Ge, B., Wang, X., Lam, Y. F., Lin, C.-Y., Itahashi, S., Nagashima, T., Kajino, M., Yamaji, K., Wang, Z., and Kurokawa, J.: MICS-Asia III: multi-model comparison and evaluation of aerosol over East Asia, Atmos. Chem. Phys., 19, 11911–11937, https://doi.org/10.5194/acp-19-11911-2019, 2019a.
Chen, L., Zhu, J., Liao, H., Gao, Y., Qiu, Y., Zhang, M., Liu, Z., Li, N., and Wang, Y.: Assessing the formation and evolution mechanisms of severe haze pollution in the Beijing–Tianjin–Hebei region using process analysis, Atmos. Chem. Phys., 19, 10845–10864, https://doi.org/10.5194/acp-19-10845-2019, 2019b.
Chen, S., Huang, J., Zhao, C., Qian, Y., Leung, L. R., and Yang, B.: Modeling the transport and radiative forcing of Taklimakan dust over the Tibetan Plateau: A case study in the summer of 2006, J. Geophys. Res.-Atmos., 118, 797–812, https://doi.org/10.1002/jgrd.50122, 2013.
Chen, S., Zhao, C., Qian, Y., Leung, L. R., Huang, J., Huang, Z., Bi, J., Zhang, W., Shi, J., and Yang, L.: Regional modeling of dust mass balance and radiative forcing over East Asia using WRF-Chem, Aeolian Res., 15, 15–30, https://doi.org/10.1016/j.aeolia.2014.02.001, 2014.
Chen, S., Huang, J., Kang, L., Wang, H., Ma, X., He, Y., Yuan, T., Yang, B., Huang, Z., and Zhang, G.: Emission, transport, and radiative effects of mineral dust from the Taklimakan and Gobi deserts: comparison of measurements and model results, Atmos. Chem. Phys., 17, 2401–2421, https://doi.org/10.5194/acp-17-2401-2017, 2017a.
Chen, S., Huang, J., Qian, Y., Zhao, C., Kang, L., Yang, B., Wang, Y., Liu, Y., Yuan, T., and Wang, T.: An overview of mineral dust modeling over East Asia, J. Meteorol. Res., 31, 633–653, https://doi.org/10.1007/s13351-017-6142-2, 2017b.
Chen, X., Wang, Z., Yu, F., Pan, X., Li, J., Ge, B., Wang, Z., Hu, M., Yang, W., and Chen, H.: Estimation of atmospheric aging time of black carbon particles in the polluted atmosphere over central-eastern China using microphysical process analysis in regional chemical transport model, Atmos. Environ., 163, 44–56, https://doi.org/10.1016/j.atmosenv.2017.05.016, 2017.
Chen, X., Yang, W., Wang, Z., Li, J., Hu, M., An, J., Wu, Q., Wang, Z., Chen, H., and Wei, Y.: Improving new particle formation simulation by coupling a volatility-basis set (VBS) organic aerosol module in NAQPMS+APM, Atmos. Environ., 204, 1–11, https://doi.org/10.1016/j.atmosenv.2019.01.053, 2019.
Chen, X., Yu, F., Yang, W., Sun, Y., Chen, H., Du, W., Zhao, J., Wei, Y., Wei, L., Du, H., Wang, Z., Wu, Q., Li, J., An, J., and Wang, Z.: Global–regional nested simulation of particle number concentration by combing microphysical processes with an evolving organic aerosol module, Atmos. Chem. Phys., 21, 9343–9366, https://doi.org/10.5194/acp-21-9343-2021, 2021.
Chen, Y., Zhang, Y., Fan, J., Leung, L.-Y. R., Zhang, Q., and He, K.: Application of an online-coupled regional climate model, WRF-CAM5, over East Asia for examination of ice nucleation schemes: Part I. Comprehensive model evaluation and trend analysis for 2006 and 2011, 3, 627–667, https://doi.org/10.3390/cli3030627, 2015.
Choobari, O. A., Zawar-Reza, P., and Sturman, A.: The global distribution of mineral dust and its impacts on the climate system: A review, Atmos. Res., 138, 152–165, https://doi.org/10.1016/j.atmosres.2013.11.007, 2014.
Conibear, L., Butt, E. W., Knote, C., Arnold, S. R., and Spracklen, D. V.: Residential energy use emissions dominate health impacts from exposure to ambient particulate matter in India, Nat. Commun., 9, 1–9, https://doi.org/10.1038/s41467-018-02986-7, 2018a.
Conibear, L., Butt, E. W., Knote, C., Arnold, S. R., and Spracklen, D. V.: Stringent Emission Control Policies Can Provide Large Improvements in Air Quality and Public Health in India, GeoHealth, 2, 196–211, https://doi.org/10.1029/2018gh000139, 2018b.
Conti, G. O., Heibati, B., Kloog, I., Fiore, M., and Ferrante, M.: A review of AirQ Models and their applications for forecasting the air pollution health outcomes, Environ. Sci. Pollut. Res., 24, 6426–6445, https://doi.org/10.1007/s11356-016-8180-1, 2017.
Craig, A., Valcke, S., and Coquart, L.: Development and performance of a new version of the OASIS coupler, OASIS3-MCT_3.0, Geosci. Model Dev., 10, 3297–3308, https://doi.org/10.5194/gmd-10-3297-2017, 2017.
Cuchiara, G. C., Li, X., Carvalho, J., and Rappenglück, B.: Intercomparison of planetary boundary layer parameterization and its impacts on surface ozone concentration in the WRF/Chem model for a case study in Houston/Texas, Atmos. Environ., 96, 175–185, https://doi.org/10.1016/j.atmosenv.2014.07.013, 2014.
Dahutia, P., Pathak, B., and Bhuyan, P. K.: Vertical distribution of aerosols and clouds over north-eastern South Asia: Aerosol-cloud interactions, Atmos. Environ., 215, 116882, https://doi.org/10.1016/j.atmosenv.2019.116882, 2019.
Ding, A. J., Huang, X., Nie, W., Sun, J. N., Kerminen, V., Petäjä, T., Su, H., Cheng, Y. F., Yang, X., and Wang, M. H.: Enhanced haze pollution by black carbon in megacities in China, Geophys. Res. Lett., 43, 2873–2879, https://doi.org/10.1002/2016GL067745, 2016.
Ding, Q., Sun, J., Huang, X., Ding, A., Zou, J., Yang, X., and Fu, C.: Impacts of black carbon on the formation of advection–radiation fog during a haze pollution episode in eastern China, Atmos. Chem. Phys., 19, 7759–7774, https://doi.org/10.5194/acp-19-7759-2019, 2019.
Dipu, S., Prabha, T. V, Pandithurai, G., Dudhia, J., Pfister, G., Rajesh, K., and Goswami, B. N.: Impact of elevated aerosol layer on the cloud macrophysical properties prior to monsoon onset, Atmos. Environ., 70, 454–467, https://doi.org/10.1016/j.atmosenv.2012.12.036, 2013.
Donat, M. G., Lowry, A. L., Alexander, L. V, O'Gorman, P. A., and Maher, N.: More extreme precipitation in the world's dry and wet regions, Nat. Clim. Chang., 6, 508–513, https://doi.org/10.1038/nclimate2941, 2016.
Dong, X., Fu, J. S., Huang, K., Zhu, Q., and Tipton, M.: Regional Climate Effects of Biomass Burning and Dust in East Asia: Evidence From Modeling and Observation, Geophys. Res. Lett., 46, 11490–11499, https://doi.org/10.1029/2019GL083894, 2019.
Easter, R. C., Ghan, S. J., Zhang, Y., Saylor, R. D., Chapman, E. G., Laulainen, N. S., Abdul-Razzak, H., Leung, L. R., Bian, X., and Zaveri, R. A.: MIRAGE: Model description and evaluation of aerosols and trace gases, J. Geophys. Res.-Atmos., 109, D20210, https://doi.org/10.1029/2004JD004571, 2004.
Eck, T. F., Holben, B. N., Reid, J. S., Xian, P., Giles, D. M., Sinyuk, A., Smirnov, A., Schafer, J. S., Slutsker, I., and Kim, J.: Observations of the interaction and transport of fine mode aerosols with cloud and/or fog in Northeast Asia from Aerosol Robotic Network and satellite remote sensing, J. Geophys. Res.-Atmos., 123, 5560–5587, https://doi.org/10.1029/2018JD028313, 2018.
El-Harbawi, M.: Air quality modelling, simulation, and computational methods: a review, Environ. Rev., 21, 149–179, https://doi.org/10.1139/er-2012-0056, 2013.
Fahey, K. M. and Pandis, S. N.: Optimizing model performance: variable size resolution in cloud chemistry modeling, Atmos. Environ., 35, 4471–4478, https://doi.org/10.1016/S1352-2310(01)00224-2, 2001.
Fahey, K. M., Carlton, A. G., Pye, H. O. T., Baek, J., Hutzell, W. T., Stanier, C. O., Baker, K. R., Appel, K. W., Jaoui, M., and Offenberg, J. H.: A framework for expanding aqueous chemistry in the Community Multiscale Air Quality (CMAQ) model version 5.1, Geosci. Model Dev., 10, 1587–1605, https://doi.org/10.5194/gmd-10-1587-2017, 2017.
Fan, J., Rosenfeld, D., Yang, Y., Zhao, C., Leung, L. R., and Li, Z.: Substantial contribution of anthropogenic air pollution to catastrophic floods in Southwest China, Geophys. Res. Lett., 42, 6066–6075, https://doi.org/10.1002/2015GL064479, 2015.
Fan, J., Wang, Y., Rosenfeld, D., and Liu, X.: Review of aerosol-cloud interactions: Mechanisms, significance, and challenges, J. Atmos. Sci., 73, 4221–4252, https://doi.org/10.1175/JAS-D-16-0037.1, 2016.
Fan, J., Rosenfeld, D., Zhang, Y., Giangrande, S. E., Li, Z., Machado, L. A. T., Martin, S. T., Yang, Y., Wang, J., and Artaxo, P.: Substantial convection and precipitation enhancements by ultrafine aerosol particles, Science, 359, 411–418, https://doi.org/10.1126/science.aan8461, 2018.
Fast, J. D., Gustafson Jr, W. I., Easter, R. C., Zaveri, R. A., Barnard, J. C., Chapman, E. G., Grell, G. A., and Peckham, S. E.: Evolution of ozone, particulates, and aerosol direct radiative forcing in the vicinity of Houston using a fully coupled meteorology-chemistry-aerosol model, J. Geophys. Res.-Atmos., 111, D21305, https://doi.org/10.1029/2005JD006721, 2006.
Feingold, G., Eberhard, W. L., Veron, D. E., and Previdi, M.: First measurements of the Twomey indirect effect using ground-based remote sensors, Geophys. Res. Lett., 30, 1287, https://doi.org/10.1029/2002GL016633, 2003.
Feng, Y., Kotamarthi, V. R., Coulter, R., Zhao, C., and Cadeddu, M.: Radiative and thermodynamic responses to aerosol extinction profiles during the pre-monsoon month over South Asia, Atmos. Chem. Phys., 16, 247–264, https://doi.org/10.5194/acp-16-247-2016, 2016.
Foley, K. M., Roselle, S. J., Appel, K. W., Bhave, P. V., Pleim, J. E., Otte, T. L., Mathur, R., Sarwar, G., Young, J. O., Gilliam, R. C., Nolte, C. G., Kelly, J. T., Gilliland, A. B., and Bash, J. O.: Incremental testing of the Community Multiscale Air Quality (CMAQ) modeling system version 4.7, Geosci. Model Dev., 3, 205–226, https://doi.org/10.5194/gmd-3-205-2010, 2010.
Forkel, R., Brunner, D., Baklanov, A., Balzarini, A., Hirtl, M., Honzak, L., Jiménez-Guerrero, P., Jorba, O., Pérez, J. L., and San José, R.: A multi-model case study on aerosol feedbacks in online coupled chemistry-meteorology models within the cost action ES1004 EuMetChem, in: Air pollution modeling and its application XXIV, Springer, 23–28, https://doi.org/10.1007/978-3-319-24478-5_4, 2016.
Fu, P., Aggarwal, S. G., Chen, J., Li, J., Sun, Y., Wang, Z., Chen, H., Liao, H., Ding, A., and Umarji, G. S.: Molecular markers of secondary organic aerosol in Mumbai, India, Environ. Sci. Technol., 50, 4659–4667, https://doi.org/10.1021/acs.est.6b00372, 2016.
Gao, C., Zhang, X., Xiu, A., Huang, L., Zhao, H., Wang, K., and Tong, Q.: Spatiotemporal distribution of biogenic volatile organic compounds emissions in China, Acta Sci. Circumstantiae, 39, 4140–4151, https://doi.org/10.13671/j.hjkxxb.2019.0243, 2019.
Gao, C., Xiu, A., Zhang, X., Tong, Q., Zhao, H., Zhang, S., Yang, G., and Zhang, M.: Data used to create figures and tables in the ACP manuscript “Two-way coupled meteorology and air quality models in Asia: a systematic review and meta-analysis of impacts of aerosol feedbacks on meteorology and air quality” by Gao et al. (2022), Zenodo [data set], https://doi.org/10.5281/zenodo.6141615, 2022.
Gao, J., Zhu, B., Xiao, H., Kang, H., Pan, C., Wang, D., and Wang, H.: Effects of black carbon and boundary layer interaction on surface ozone in Nanjing, China, Atmos. Chem. Phys., 18, 7081–7094, https://doi.org/10.5194/acp-18-7081-2018, 2018.
Gao, M., Guttikunda, S. K., Carmichael, G. R., Wang, Y., Liu, Z., Stanier, C. O., Saide, P. E., and Yu, M.: Health impacts and economic losses assessment of the 2013 severe haze event in Beijing area, Sci. Total Environ., 511, 553–561, https://doi.org/10.1016/j.scitotenv.2015.01.005, 2015.
Gao, M., Carmichael, G. R., Saide, P. E., Lu, Z., Yu, M., Streets, D. G., and Wang, Z.: Response of winter fine particulate matter concentrations to emission and meteorology changes in North China, Atmos. Chem. Phys., 16, 11837–11851, https://doi.org/10.5194/acp-16-11837-2016, 2016a.
Gao, M., Carmichael, G. R., Wang, Y., Saide, P. E., Yu, M., Xin, J., Liu, Z., and Wang, Z.: Modeling study of the 2010 regional haze event in the North China Plain, Atmos. Chem. Phys., 16, 1673–1691, https://doi.org/10.5194/acp-16-1673-2016, 2016b.
Gao, M., Carmichael, G. R., Wang, Y., Saide, P. E., Liu, Z., Xin, J., Shan, Y., and Wang, Z.: Chemical and Meteorological Feedbacks in the Formation of Intense Haze Events, in: Air Pollution in Eastern Asia: An Integrated Perspective, Springer, 437–452, https://doi.org/10.1007/978-3-319-59489-7_21, 2017a.
Gao, M., Liu, Z., Wang, Y., Lu, X., Ji, D., Wang, L., Li, M., Wang, Z., Zhang, Q., and Carmichael, G. R.: Distinguishing the roles of meteorology, emission control measures, regional transport, and co-benefits of reduced aerosol feedbacks in “APEC Blue”, Atmos. Environ., 167, 476–486, https://doi.org/10.1016/j.atmosenv.2017.08.054, 2017b.
Gao, M., Saide, P. E., Xin, J., Wang, Y., Liu, Z., Wang, Y., Wang, Z., Pagowski, M., Guttikunda, S. K., and Carmichael, G. R.: Estimates of health impacts and radiative forcing in winter haze in eastern China through constraints of surface PM2.5 predictions, Environ. Sci. Technol., 51, 2178–2185, https://doi.org/10.1021/acs.est.6b03745, 2017c.
Gao, M., Han, Z., Liu, Z., Li, M., Xin, J., Tao, Z., Li, J., Kang, J.-E., Huang, K., Dong, X., Zhuang, B., Li, S., Ge, B., Wu, Q., Cheng, Y., Wang, Y., Lee, H.-J., Kim, C.-H., Fu, J. S., Wang, T., Chin, M., Woo, J.-H., Zhang, Q., Wang, Z., and Carmichael, G. R.: Air quality and climate change, Topic 3 of the Model Inter-Comparison Study for Asia Phase III (MICS-Asia III) – Part 1: Overview and model evaluation, Atmos. Chem. Phys., 18, 4859–4884, https://doi.org/10.5194/acp-18-4859-2018, 2018a.
Gao, M., Ji, D., Liang, F., and Liu, Y.: Attribution of aerosol direct radiative forcing in China and India to emitting sectors, Atmos. Environ., 190, 35–42, https://doi.org/10.1016/j.atmosenv.2018.07.011, 2018b.
Gao, Y. and Zhang, M.: Changes in the diurnal variations of clouds and precipitation induced by anthropogenic aerosols over East China in August 2008, Atmos. Pollut. Res., 9, 513–525, https://doi.org/10.1016/j.apr.2017.11.013, 2018.
Gao, Y., Zhang, M., Liu, X., and Zhao, C.: Model Analysis of the Anthropogenic Aerosol Effect on Clouds over East Asia, Atmos. Ocean. Sci. Lett., 5, 1–7, https://doi.org/10.1080/16742834.2012.11446968, 2012.
Gao, Y., Zhao, C., Liu, X., Zhang, M., and Leung, L. R.: WRF-Chem simulations of aerosols and anthropogenic aerosol radiative forcing in East Asia, Atmos. Environ., 92, 250–266, https://doi.org/10.1016/j.atmosenv.2014.04.038, 2014.
Gao, Y., Zhang, M., Liu, Z., Wang, L., Wang, P., Xia, X., Tao, M., and Zhu, L.: Modeling the feedback between aerosol and meteorological variables in the atmospheric boundary layer during a severe fog–haze event over the North China Plain, Atmos. Chem. Phys., 15, 4279–4295, https://doi.org/10.5194/acp-15-4279-2015, 2015.
Gao, Y., Zhang, M., Liu, X., and Wang, L.: Change in diurnal variations of meteorological variables induced by anthropogenic aerosols over the North China Plain in summer 2008, Theor. Appl. Climatol., 124, 103–118, https://doi.org/10.1007/s00704-015-1403-4, 2016.
García-Díez, M., Fernández, J., Fita, L., and Yagüe, C.: Seasonal dependence of WRF model biases and sensitivity to PBL schemes over Europe, Q. J. Roy. Meteor. Soc., 139, 501–514, https://doi.org/10.1002/qj.1976, 2013.
Ge, C., Wang, J., and Reid, J. S.: Mesoscale modeling of smoke transport over the Southeast Asian Maritime Continent: coupling of smoke direct radiative effect below and above the low-level clouds, Atmos. Chem. Phys., 14, 159–174, https://doi.org/10.5194/acp-14-159-2014, 2014.
Georgiou, G. K., Christoudias, T., Proestos, Y., Kushta, J., Hadjinicolaou, P., and Lelieveld, J.: Air quality modelling in the summer over the eastern Mediterranean using WRF-Chem: chemistry and aerosol mechanism intercomparison, Atmos. Chem. Phys., 18, 1555–1571, https://doi.org/10.5194/acp-18-1555-2018, 2018.
Gery, M. W., Whitten, G. Z., Killus, J. P., and Dodge, M. C.: A photochemical kinetics mechanism for urban and regional scale computer modeling, J. Geophys. Res.-Atmos., 94, 12925–12956, https://doi.org/10.1029/JD094iD10p12925, 1989.
Ghan, S. J. and Zaveri, R. A.: Parameterization of optical properties for hydrated internally mixed aerosol, J. Geophys. Res.-Atmos., 112, D10201, https://doi.org/10.1029/2006JD007927, 2007.
Ghude, S. D., Chate, D. M., Jena, C., Beig, G., Kumar, R., Barth, M. C., Pfister, G. G., Fadnavis, S., and Pithani, P.: Premature mortality in India due to PM2.5 and ozone exposure, Geophys. Res. Lett., 43, 4650–4658, https://doi.org/10.1002/2016GL068949, 2016.
Giglio, L., Randerson, J. T., van der Werf, G. R., Kasibhatla, P. S., Collatz, G. J., Morton, D. C., and DeFries, R. S.: Assessing variability and long-term trends in burned area by merging multiple satellite fire products, Biogeosciences, 7, 1171–1186, https://doi.org/10.5194/bg-7-1171-2010, 2010.
Ginoux, P., Chin, M., Tegen, I., Prospero, J. M., Holben, B., Dubovik, O., and Lin, S.: Sources and distributions of dust aerosols simulated with the GOCART model, J. Geophys. Res.-Atmos., 106, 20255–20273, https://doi.org/10.1029/2000JD000053, 2001.
Giorgi, F. and Chameides, W. L.: Rainout lifetimes of highly soluble aerosols and gases as inferred from simulations with a general circulation model, J. Geophys. Res.-Atmos., 91, 14367–14376, https://doi.org/10.1029/JD091iD13p14367, 1986.
Gong, S. L., Barrie, L. A., and Blanchet, J.: Modeling sea-salt aerosols in the atmosphere: 1. Model development, J. Geophys. Res.-Atmos., 102, 3805–3818, https://doi.org/10.1029/96JD02953, 1997.
Gong, S. L., Barrie, L. A., Blanchet, J., Von Salzen, K., Lohmann, U., Lesins, G., Spacek, L., Zhang, L. M., Girard, E., and Lin, H.: Canadian Aerosol Module: A size-segregated simulation of atmospheric aerosol processes for climate and air quality models 1. Module development, J. Geophys. Res.-Atmos., 108, AAC-3, https://doi.org/10.1029/2001JD002002, 2003a.
Gong, S. L., Zhang, X. Y., Zhao, T. L., McKendry, I. G., Jaffe, D. A., and Lu, N. M.: Characterization of soil dust aerosol in China and its transport and distribution during 2001 ACE-Asia: 2. Model simulation and validation, J. Geophys. Res.-Atmos., 108, 4262, https://doi.org/10.1029/2002JD002633, 2003b.
Goren, T. and Rosenfeld, D.: Decomposing aerosol cloud radiative effects into cloud cover, liquid water path and Twomey components in marine stratocumulus, Atmos. Res., 138, 378–393, https://doi.org/10.1016/j.atmosres.2013.12.008, 2014.
Govardhan, G., Nanjundiah, R. S., Satheesh, S. K., Krishnamoorthy, K., and Kotamarthi, V. R.: Performance of WRF-Chem over Indian region: Comparison with measurements, J. Earth Syst. Sci., 124, 875–896, https://doi.org/10.1007/s12040-015-0576-7, 2015.
Govardhan, G. R., Nanjundiah, R. S., Satheesh, S. K., Moorthy, K. K., and Takemura, T.: Inter-comparison and performance evaluation of chemistry transport models over Indian region, Atmos. Environ., 125, 486–504, https://doi.org/10.1016/j.atmosenv.2015.10.065, 2016.
Gray, L. J., Beer, J., Geller, M., Haigh, J. D., Lockwood, M., Matthes, K., Cubasch, U., Fleitmann, D., Harrison, G., and Hood, L.: Solar influences on climate, Rev. Geophys., 48, RG4001, https://doi.org/10.1029/2009RG000282, 2010.
Grell, G., Freitas, S. R., Stuefer, M., and Fast, J.: Inclusion of biomass burning in WRF-Chem: impact of wildfires on weather forecasts, Atmos. Chem. Phys., 11, 5289–5303, https://doi.org/10.5194/acp-11-5289-2011, 2011.
Grell, G. A., Peckham, S. E., Schmitz, R., McKeen, S. A., Frost, G., Skamarock, W. C., and Eder, B.: Fully coupled “online” chemistry within the WRF model, Atmos. Environ., 39, 6957–6975, https://doi.org/10.1016/j.atmosenv.2005.04.027, 2005.
Griffin, R. J., Cocker III, D. R., Seinfeld, J. H., and Dabdub, D.: Estimate of global atmospheric organic aerosol from oxidation of biogenic hydrocarbons, Geophys. Res. Lett., 26, 2721–2724, https://doi.org/10.1029/1999GL900476, 1999.
Groß, S., Esselborn, M., Weinzierl, B., Wirth, M., Fix, A., and Petzold, A.: Aerosol classification by airborne high spectral resolution lidar observations, Atmos. Chem. Phys., 13, 2487–2505, https://doi.org/10.5194/acp-13-2487-2013, 2013.
Guenther, A., Karl, T., Harley, P., Wiedinmyer, C., Palmer, P. I., and Geron, C.: Estimates of global terrestrial isoprene emissions using MEGAN (Model of Emissions of Gases and Aerosols from Nature), Atmos. Chem. Phys., 6, 3181–3210, https://doi.org/10.5194/acp-6-3181-2006, 2006.
Guo, J., Deng, M., Fan, J., Li, Z., Chen, Q., Zhai, P., Dai, Z., and Li, X.: Precipitation and air pollution at mountain and plain stations in northern China: Insights gained from observations and modeling, J. Geophys. Res.-Atmos., 119, 4793–4807, https://doi.org/10.1002/2013JD021161, 2014.
Guo, J., Liu, H., Li, Z., Rosenfeld, D., Jiang, M., Xu, W., Jiang, J. H., He, J., Chen, D., Min, M., and Zhai, P.: Aerosol-induced changes in the vertical structure of precipitation: a perspective of TRMM precipitation radar, Atmos. Chem. Phys., 18, 13329–13343, https://doi.org/10.5194/acp-18-13329-2018, 2018.
Gurjar, B. R., Ravindra, K., and Nagpure, A. S.: Air pollution trends over Indian megacities and their local-to-global implications, Atmos. Environ., 142, 475–495, https://doi.org/10.1016/j.atmosenv.2016.06.030, 2016.
Haywood, J. and Boucher, O.: Estimates of the direct and indirect radiative forcing due to tropospheric aerosols: A review, Rev. Geophys., 38, 513–543, https://doi.org/10.1029/1999RG000078, 2000.
He, J. and Zhang, Y.: Improvement and further development in CESM/CAM5: gas-phase chemistry and inorganic aerosol treatments, Atmos. Chem. Phys., 14, 9171–9200, https://doi.org/10.5194/acp-14-9171-2014, 2014.
He, J., Zhang, Y., Wang, K., Chen, Y., Leung, L. R., Fan, J., Li, M., Zheng, B., Zhang, Q., and Duan, F.: Multi-year application of WRF-CAM5 over East Asia-Part I: Comprehensive evaluation and formation regimes of O3 and PM2.5, Atmos. Environ., 165, 122–142, https://doi.org/10.1016/j.atmosenv.2017.06.015, 2017.
Hess, M., Koepke, P., and Schult, I.: Optical properties of aerosols and clouds: The software package OPAC, B. Am. Meteorol. Soc., 79, 831–844, https://doi.org/10.1175/1520-0477(1998)079<0831:OPOAAC>2.0.CO;2, 1998.
Hodshire, A. L., Akherati, A., Alvarado, M. J., Brown-Steiner, B., Jathar, S. H., Jimenez, J. L., Kreidenweis, S. M., Lonsdale, C. R., Onasch, T. B., and Ortega, A. M.: Aging effects on biomass burning aerosol mass and composition: A critical review of field and laboratory studies, Environ. Sci. Technol., 53, 10007–10022, https://doi.org/10.1021/acs.est.9b02588, 2019.
Hodzic, A. and Jimenez, J. L.: Modeling anthropogenically controlled secondary organic aerosols in a megacity: a simplified framework for global and climate models, Geosci. Model Dev., 4, 901–917, https://doi.org/10.5194/gmd-4-901-2011, 2011.
Hong, C., Zhang, Q., Zhang, Y., Tang, Y., Tong, D., and He, K.: Multi-year downscaling application of two-way coupled WRF v3.4 and CMAQ v5.0.2 over east Asia for regional climate and air quality modeling: model evaluation and aerosol direct effects, Geosci. Model Dev., 10, 2447–2470, https://doi.org/10.5194/gmd-10-2447-2017, 2017.
Hong, C., Zhang, Q., Zhang, Y., Davis, S. J., Tong, D., Zheng, Y., Liu, Z., Guan, D., He, K., and Schellnhuber, H. J.: Impacts of climate change on future air quality and human health in China, P. Natl. Acad. Sci. USA, 116, 17193–17200, https://doi.org/10.1073/pnas.1812881116, 2019.
Hu, X., Klein, P. M., and Xue, M.: Evaluation of the updated YSU planetary boundary layer scheme within WRF for wind resource and air quality assessments, J. Geophys. Res.-Atmos., 118, 10–490, https://doi.org/10.1002/jgrd.50823, 2013.
Huang, J., Wang, T., Wang, W., Li, Z., and Yan, H.: Climate effects of dust aerosols over East Asian arid and semiarid regions, J. Geophys. Res.-Atmos., 119, 11–398, https://doi.org/10.1002/2014JD021796, 2014.
Huang, L., Lin, W., Li, F., Wang, Y., and Jiang, B.: Climate impacts of the biomass burning in Indochina on atmospheric conditions over southern China, Aerosol Air Qual. Res., 19, 2707–2720, https://doi.org/10.4209/aaqr.2019.01.0028, 2019.
Huang, X., Song, Y., Zhao, C., Cai, X., Zhang, H., and Zhu, T.: Direct radiative effect by multicomponent aerosol over China, J. Climate, 28, 3472–3495, https://doi.org/10.1175/JCLI-D-14-00365.1, 2015.
Huang, X., Ding, A., Liu, L., Liu, Q., Ding, K., Niu, X., Nie, W., Xu, Z., Chi, X., Wang, M., Sun, J., Guo, W., and Fu, C.: Effects of aerosol–radiation interaction on precipitation during biomass-burning season in East China, Atmos. Chem. Phys., 16, 10063–10082, https://doi.org/10.5194/acp-16-10063-2016, 2016.
Illingworth, A. J., Barker, H. W., Beljaars, A., Ceccaldi, M., Chepfer, H., Clerbaux, N., Cole, J., Delanoë, J., Domenech, C., and Donovan, D. P.: The EarthCARE satellite: The next step forward in global measurements of clouds, aerosols, precipitation, and radiation, B. Am. Meteorol. Soc., 96, 1311–1332, https://doi.org/10.1175/BAMS-D-12-00227.1, 2015.
Im, U., Bianconi, R., Solazzo, E., Kioutsioukis, I., Badia, A., Balzarini, A., Baró, R., Bellasio, R., Brunner, D., and Chemel, C.: Evaluation of operational on-line-coupled regional air quality models over Europe and North America in the context of AQMEII phase 2. Part I: Ozone, Atmos. Environ., 115, 404–420, https://doi.org/10.1016/j.atmosenv.2014.09.042, 2015a.
Im, U., Bianconi, R., Solazzo, E., Kioutsioukis, I., Badia, A., Balzarini, A., Baró, R., Bellasio, R., Brunner, D., and Chemel, C.: Evaluation of operational online-coupled regional air quality models over Europe and North America in the context of AQMEII phase 2. Part II: Particulate matter, Atmos. Environ., 115, 421–441, https://doi.org/10.1016/j.atmosenv.2014.08.072, 2015b.
IPCC: Climate change 2007: Synthesis Report. Contribution of Working Groups I, II and III to the Fourth Assessment Report of the Intergovernmental Panel on Climate Change, edited by: Solomon, S. D., Qin, D., Manning, M., Chen, Z., Marquis, M., Averyt, K. B., Tignor, M., and Miller, H. L., Cambridge University Press, Cambridge, United Kingdom and New York, NY, USA, 2007.
IPCC: Climate change 2014: Synthesis Report. Contribution of Working Groups I, II and III to the Fifth Assessment Report of the Intergovernmental Panel on Climate Change, edited by: Stocker, T. F., Qin, D., Plattner, G.-K., Tignor, M., Allen, S. K., Boschung, J., Nauels, A., Xia, Y., Bex, V., and Midgley, P. M., Cambridge University Press, Cambridge, United Kingdom and New York, NY, USA, 1535 pp., 2013.
IPCC: Climate Change 2021: The Physical Science Basis. Contribution of Working Group I to the Sixth Assessment Report of the Intergovernmental Panel on Climate Change, edited by: Masson-Delmotte, V., Zha, P., Pirani, A., Connors, S. L., Péan, C., Berger, S., Caud, N., Chen, Y., Goldfarb, L., Gomis, M. I., Huang, M., Leitzell, K., Lonnoy, E. Matthews, J. B. R., Maycock, T. K., Waterfield, T., Yelekçi, O., Yu, R., and Zhou, B., Cambridge University Press, https://www.ipcc.ch/report/ar6/wg1/#FullReport (last access: 20 March 2022), 2021.
Jacobson, M. Z.: Developing, coupling, and applying a gas, aerosol, transport, and radiation model to study urban and regional air pollution, Ph.D. thesis, Department of Atmospheric Sciences, University of California, United States, 436 pp., 1994.
Jacobson, M. Z.: Development and application of a new air pollution modeling system – Part III. Aerosol-phase simulations, Atmos. Environ., 31, 587–608, https://doi.org/10.1016/S1352-2310(96)00201-4, 1997a.
Jacobson, M. Z.: Numerical techniques to solve condensational and dissolutional growth equations when growth is coupled to reversible reactions, Aerosol Sci. Technol., 27, 491–498, https://doi.org/10.1080/02786829708965489, 1997b.
Jacobson, M. Z.: Fundamentals of atmospheric modeling, Cambridge University Press, https://web.stanford.edu/group/efmh/jacobson/FAMbook/FAMbook.html (last access: 20 March 2022), 1999.
Jacobson, M. Z.: Strong radiative heating due to the mixing state of black carbon in atmospheric aerosols, Nature, 409, 695–697, https://doi.org/10.1038/35055518, 2001.
Jacobson, M. Z.: Analysis of aerosol interactions with numerical techniques for solving coagulation, nucleation, condensation, dissolution, and reversible chemistry among multiple size distributions, J. Geophys. Res.-Atmos., 107, AAC-2, https://doi.org/10.1029/2001JD002044, 2002.
Jacobson, M. Z.: Development of mixed-phase clouds from multiple aerosol size distributions and the effect of the clouds on aerosol removal, J. Geophys. Res.-Atmos., 108, 4366, https://doi.org/10.1029/2002JD002691, 2003.
Jacobson, M. Z.: History of, processes in, and numerical techniques in GATOR-GCMOM, https://web.stanford.edu/group/efmh/jacobson/GATOR/GATOR-GCMOMHist.pdf (last access: 20 March 2022), 2012a.
Jacobson, M. Z.: Investigating cloud absorption effects: Global absorption properties of black carbon, tar balls, and soil dust in clouds and aerosols, J. Geophys. Res.-Atmos., 117, D06205, https://doi.org/10.1029/2011JD017218, 2012b.
Jacobson, M. Z. and Jadhav, V.: World estimates of PV optimal tilt angles and ratios of sunlight incident upon tilted and tracked PV panels relative to horizontal panels, Sol. Energy, 169, 55–66, https://doi.org/10.1016/j.solener.2018.04.030, 2018.
Jacobson, M. Z. and Turco, R. P.: Simulating condensational growth, evaporation, and coagulation of aerosols using a combined moving and stationary size grid, Aerosol Sci. Technol., 22, 73–92, https://doi.org/10.1080/02786829408959729, 1995.
Jacobson, M. Z., Turco, R. P., Jensen, E. J., and Toon, O. B.: Modeling coagulation among particles of different composition and size, Atmos. Environ., 28, 1327–1338, https://doi.org/10.1016/1352-2310(94)90280-1, 1994.
Jacobson, M. Z., Lu, R., Turco, R. P., and Toon, O. B.: Development and application of a new air pollution modeling system-part I: Gas-phase simulations, Atmos. Environ., 30, 1939–1963, https://doi.org/10.1016/1352-2310(95)00139-5, 1996a.
Jacobson, M. Z., Tabazadeh, A., and Turco, R. P.: Simulating equilibrium within aerosols and nonequilibrium between gases and aerosols, J. Geophys. Res.-Atmos., 101, 9079–9091, https://doi.org/10.1029/96JD00348, 1996b.
Jacobson, M. Z., Kaufman, Y. J., and Rudich, Y.: Examining feedbacks of aerosols to urban climate with a model that treats 3-D clouds with aerosol inclusions, J. Geophys. Res.-Atmos., 112, D24205, https://doi.org/10.1029/2007JD008922, 2007.
Jacobson, M. Z., Nghiem, S. V, Sorichetta, A., and Whitney, N.: Ring of impact from the mega-urbanization of Beijing between 2000 and 2009, J. Geophys. Res.-Atmos., 120, 5740–5756, https://doi.org/10.1002/2014JD023008, 2015.
Jacobson, M. Z., Nghiem, S. V, and Sorichetta, A.: Short-term impacts of the megaurbanizations of New Delhi and Los Angeles between 2000 and 2009, J. Geophys. Res.-Atmos., 124, 35–56, https://doi.org/10.1029/2018JD029310, 2019.
Jena, C., Ghude, S. D., Pfister, G. G., Chate, D. M., Kumar, R., Beig, G., Surendran, D. E., Fadnavis, S., and Lal, D. M.: Influence of springtime biomass burning in South Asia on regional ozone (O3): A model based case study, Atmos. Environ., 100, 37–47, https://doi.org/10.1016/j.atmosenv.2014.10.027, 2015.
Jeong, J. I. and Park, R. J.: Winter monsoon variability and its impact on aerosol concentrations in East Asia, Environ. Pollut., 221, 285–292, https://doi.org/10.1016/j.envpol.2016.11.075, 2017.
Jia, X. and Guo, X.: Impacts of Anthropogenic Atmospheric Pollutant on Formation and Development of a Winter Heavy Fog Event, Chinese J. Atmos. Sci., 36, 995–1008, https://doi.org/10.1007/s11783-011-0280-z, 2012.
Jia, X., Quan, J., Zheng, Z., Liu, X., Liu, Q., He, H., and Liu, Y.: Impacts of Anthropogenic Aerosols on Fog in North China Plain, J. Geophys. Res.-Atmos., 124, 252–265, https://doi.org/10.1029/2018JD029437, 2019.
Jiang, B., Huang, B., Lin, W., and Xu, S.: Investigation of the effects of anthropogenic pollution on typhoon precipitation and microphysical processes using WRF-Chem, J. Atmos. Sci., 73, 1593–1610, https://doi.org/10.1175/JAS-D-15-0202.1, 2016.
Jiang, B., Lin, W., Li, F., and Chen, B.: Simulation of the effects of sea-salt aerosols on cloud ice and precipitation of a tropical cyclone, Atmos. Sci. Lett., 20, e936, https://doi.org/10.1002/asl.936, 2019a.
Jiang, B., Lin, W., Li, F., and Chen, J.: Sea-salt aerosol effects on the simulated microphysics and precipitation in a tropical cyclone, J. Meteorol. Res., 33, 115–125, https://doi.org/10.1007/s13351-019-8108-z, 2019b.
Jimenez, P. A., Hacker, J. P., Dudhia, J., Haupt, S. E., Ruiz-Arias, J. A., Gueymard, C. A., Thompson, G., Eidhammer, T., and Deng, A.: WRF-Solar: Description and clear-sky assessment of an augmented NWP model for solar power prediction, B. Am. Meteorol. Soc., 97, 1249–1264, https://doi.org/10.1175/BAMS-D-14-00279.1, 2016.
Jin, Q., Wei, J., Yang, Z.-L., Pu, B., and Huang, J.: Consistent response of Indian summer monsoon to Middle East dust in observations and simulations, Atmos. Chem. Phys., 15, 9897–9915, https://doi.org/10.5194/acp-15-9897-2015, 2015.
Jin, Q., Yang, Z.-L., and Wei, J.: High sensitivity of Indian summer monsoon to Middle East dust absorptive properties, Sci. Rep.-UK, 6, 1–8, https://doi.org/10.1038/srep30690, 2016a.
Jin, Q., Yang, Z.-L., and Wei, J.: Seasonal responses of Indian summer monsoon to dust aerosols in the Middle East, India, and China, J. Climate, 29, 6329–6349, https://doi.org/10.1175/JCLI-D-15-0622.1, 2016b.
Jung, J., Souri, A. H., Wong, D. C., Lee, S., Jeon, W., Kim, J., and Choi, Y.: The Impact of the Direct Effect of Aerosols on Meteorology and Air Quality Using Aerosol Optical Depth Assimilation During the KORUS-AQ Campaign, J. Geophys. Res.-Atmos., 124, 8303–8319, https://doi.org/10.1029/2019JD030641, 2019.
Kajino, M., Ueda, H., Han, Z., Kudo, R., Inomata, Y., and Kaku, H.: Synergy between air pollution and urban meteorological changes through aerosol-radiation-diffusion feedback – A case study of Beijing in January 2013, Atmos. Environ., 171, 98–110, https://doi.org/10.1016/j.atmosenv.2017.10.018., 2017.
Kant, S., Panda, J., and Gautam, R.: A seasonal analysis of aerosol-cloud-radiation interaction over Indian region during 2000–2017, Atmos. Environ., 201, 212–222, https://doi.org/10.1016/j.atmosenv.2018.12.044, 2019.
Kedia, S., Cherian, R., Islam, S., Das, S. K., and Kaginalkar, A.: Regional simulation of aerosol radiative effects and their influence on rainfall over India using WRFChem model, Atmos. Res., 182, 232–242, https://doi.org/10.1016/j.atmosres.2016.07.008, 2016.
Kedia, S., Kumar, S., Islam, S., Hazra, A., and Kumar, N.: Aerosols impact on the convective and non-convective rain distribution over the Indian region: Results from WRF-Chem simulation, Atmos. Environ., 202, 64–74, https://doi.org/10.1016/j.atmosenv.2019.01.020, 2019a.
Kedia, S., Vellore, R. K., Islam, S., and Kaginalkar, A.: A study of Himalayan extreme rainfall events using WRF-Chem, Meteorol. Atmos. Phys., 131, 1133–1143, https://doi.org/10.1007/s00703-018-0626-1, 2019b.
Keita, S. A., Girard, E., Raut, J.-C., Leriche, M., Blanchet, J.-P., Pelon, J., Onishi, T., and Cirisan, A.: A new parameterization of ice heterogeneous nucleation coupled to aerosol chemistry in WRF-Chem model version 3.5.1: evaluation through ISDAC measurements, Geosci. Model Dev., 13, 5737–5755, https://doi.org/10.5194/gmd-13-5737-2020, 2020.
Kim, B., Schwartz, S. E., Miller, M. A., and Min, Q.: Effective radius of cloud droplets by ground-based remote sensing: Relationship to aerosol, J. Geophys. Res.-Atmos., 108, 4740, https://doi.org/10.1029/2003JD003721, 2003.
Knote, C., Hodzic, A., Jimenez, J. L., Volkamer, R., Orlando, J. J., Baidar, S., Brioude, J., Fast, J., Gentner, D. R., Goldstein, A. H., Hayes, P. L., Knighton, W. B., Oetjen, H., Setyan, A., Stark, H., Thalman, R., Tyndall, G., Washenfelder, R., Waxman, E., and Zhang, Q.: Simulation of semi-explicit mechanisms of SOA formation from glyoxal in aerosol in a 3-D model, Atmos. Chem. Phys., 14, 6213–6239, https://doi.org/10.5194/acp-14-6213-2014, 2014.
Koch, D. and Del Genio, A. D.: Black carbon semi-direct effects on cloud cover: review and synthesis, Atmos. Chem. Phys., 10, 7685–7696, https://doi.org/10.5194/acp-10-7685-2010, 2010.
Kong, X., Forkel, R., Sokhi, R. S., Suppan, P., Baklanov, A., Gauss, M., Brunner, D., Barò, R., Balzarini, A., Chemel, C., Curci, G., Jiménez-Guerrero, P., Hirtl, M., Honzak, L., Im, U., Pérez, J. L., Pirovano, G., San Jose, R., Schlünzen, K. H., Tsegas, G., Tuccella, P., Werhahn, J., Žabkar, R., and Galmarini, S.: Analysis of meteorology-chemistry interactions during air pollution episodes using online coupled models within AQMEII phase-2, Atmos. Environ., 115, 527–540, https://doi.org/10.1016/j.atmosenv.2014.09.020, 2015.
Kuik, F., Lauer, A., Churkina, G., Denier van der Gon, H. A. C., Fenner, D., Mar, K. A., and Butler, T. M.: Air quality modelling in the Berlin–Brandenburg region using WRF-Chem v3.7.1: sensitivity to resolution of model grid and input data, Geosci. Model Dev., 9, 4339–4363, https://doi.org/10.5194/gmd-9-4339-2016, 2016.
Kulmala, M., Laaksonen, A., and Pirjola, L.: Parameterizations for sulfuric acid/water nucleation rates, J. Geophys. Res.-Atmos., 103, 8301–8307, https://doi.org/10.1029/97JD03718, 1998.
Kumar, P., Sokolik, I. N., and Nenes, A.: Parameterization of cloud droplet formation for global and regional models: including adsorption activation from insoluble CCN, Atmos. Chem. Phys., 9, 2517–2532, https://doi.org/10.5194/acp-9-2517-2009, 2009.
Kumar, R., Naja, M., Pfister, G. G., Barth, M. C., and Brasseur, G. P.: Simulations over South Asia using the Weather Research and Forecasting model with Chemistry (WRF-Chem): set-up and meteorological evaluation, Geosci. Model Dev., 5, 321–343, https://doi.org/10.5194/gmd-5-321-2012, 2012a.
Kumar, R., Naja, M., Pfister, G. G., Barth, M. C., Wiedinmyer, C., and Brasseur, G. P.: Simulations over South Asia using the Weather Research and Forecasting model with Chemistry (WRF-Chem): chemistry evaluation and initial results, Geosci. Model Dev., 5, 619–648, https://doi.org/10.5194/gmd-5-619-2012, 2012b.
Kumar, R., Barth, M. C., Pfister, G. G., Naja, M., and Brasseur, G. P.: WRF-Chem simulations of a typical pre-monsoon dust storm in northern India: influences on aerosol optical properties and radiation budget, Atmos. Chem. Phys., 14, 2431–2446, https://doi.org/10.5194/acp-14-2431-2014, 2014.
Kuniyal, J. C. and Guleria, R. P.: The current state of aerosol-radiation interactions: a mini review, J. Aerosol Sci., 130, 45–54, https://doi.org/10.1016/j.jaerosci.2018.12.010, 2019.
Lau, W. K. M., Kim, K.-M., Shi, J.-J., Matsui, T., Chin, M., Tan, Q., Peters-Lidard, C., and Tao, W.-K.: Impacts of aerosol–monsoon interaction on rainfall and circulation over Northern India and the Himalaya Foothills, Clim. Dynam., 49, 1945–1960, https://doi.org/10.1007/s00382-016-3430-y, 2017.
Lee, H.-H., Chen, S.-H., Kumar, A., Zhang, H., and Kleeman, M. J.: Improvement of aerosol activation/ice nucleation in a source-oriented WRF-Chem model to study a winter Storm in California, Atmos. Res., 235, 104790, https://doi.org/10.1016/j.atmosres.2019.104790, 2020.
Lee, Y. C., Yang, X., and Wenig, M.: Transport of dusts from East Asian and non-East Asian sources to Hong Kong during dust storm related events 1996–2007, Atmos. Environ., 44, 3728–3738, https://doi.org/10.1016/j.atmosenv.2010.03.034, 2010.
Lelieveld, J., Evans, J. S., Fnais, M., Giannadaki, D., and Pozzer, A.: The contribution of outdoor air pollution sources to premature mortality on a global scale, Nature, 525, 367, https://doi.org/10.1038/nature15371, 2015.
Lelieveld, J., Bourtsoukidis, E., Brühl, C., Fischer, H., Fuchs, H., Harder, H., Hofzumahaus, A., Holland, F., Marno, D., and Neumaier, M.: The South Asian monsoon-pollution pump and purifier, Science, 361, 270–273, https://doi.org/10.1126/science.aar2501, 2018.
Li, J., Wang, Z., Wang, X., Yamaji, K., Takigawa, M., Kanaya, Y., Pochanart, P., Liu, Y., Irie, H., and Hu, B.: Impacts of aerosols on summertime tropospheric photolysis frequencies and photochemistry over Central Eastern China, Atmos. Environ., 45, 1817–1829, https://doi.org/10.1016/j.atmosenv.2011.01.016, 2011.
Li, J., Chen, X., Wang, Z., Du, H., Yang, W., Sun, Y., Hu, B., Li, J., Wang, W., and Wang, T.: Radiative and heterogeneous chemical effects of aerosols on ozone and inorganic aerosols over East Asia, Sci. Total Environ., 622, 1327–1342, https://doi.org/10.1016/j.scitotenv.2017.12.041, 2018.
Li, J., Nagashima, T., Kong, L., Ge, B., Yamaji, K., Fu, J. S., Wang, X., Fan, Q., Itahashi, S., Lee, H.-J., Kim, C.-H., Lin, C.-Y., Zhang, M., Tao, Z., Kajino, M., Liao, H., Li, M., Woo, J.-H., Kurokawa, J., Wang, Z., Wu, Q., Akimoto, H., Carmichael, G. R., and Wang, Z.: Model evaluation and intercomparison of surface-level ozone and relevant species in East Asia in the context of MICS-Asia Phase III – Part 1: Overview, Atmos. Chem. Phys., 19, 12993–13015, https://doi.org/10.5194/acp-19-12993-2019, 2019.
Li, L. and Liao, H.: Role of the Radiative Effect of Black Carbon in Simulated PM2.5 Concentrations during a Haze Event in China, Atmos. Ocean. Sci. Lett., 7, 434–440, https://doi.org/10.3878/j.issn.1674-2834.14.0023, 2014.
Li, L. and Sokolik, I. N.: The Dust Direct Radiative Impact and Its Sensitivity to the Land Surface State and Key Minerals in the WRF-Chem-DuMo Model: A Case Study of Dust Storms in Central Asia, J. Geophys. Res.-Atmos., 123, 4564–4582, https://doi.org/10.1029/2017JD027667, 2018.
Li, M., Zhang, Q., Kurokawa, J.-I., Woo, J.-H., He, K., Lu, Z., Ohara, T., Song, Y., Streets, D. G., Carmichael, G. R., Cheng, Y., Hong, C., Huo, H., Jiang, X., Kang, S., Liu, F., Su, H., and Zheng, B.: MIX: a mosaic Asian anthropogenic emission inventory under the international collaboration framework of the MICS-Asia and HTAP, Atmos. Chem. Phys., 17, 935–963, https://doi.org/10.5194/acp-17-935-2017, 2017.
Li, M., Wang, T., Xie, M., Li, S., Zhuang, B., Chen, P., Huang, X., and Han, Y.: Agricultural fire impacts on ozone photochemistry over the Yangtze River Delta region, East China, J. Geophys. Res.-Atmos., 123, 6605–6623, https://doi.org/10.1029/2018JD028582, 2018.
Li, M., Wang, T., Xie, M., Li, S., Zhuang, B., Huang, X., Chen, P., Zhao, M., and Liu, J.: Formation and evolution mechanisms for two extreme haze episodes in the Yangtze River Delta region of China during winter 2016, J. Geophys. Res.-Atmos., 124, 3607–3623, https://doi.org/10.1029/2019JD030535, 2019.
Li, M. M., Wang, T., Han, Y., Xie, M., Li, S., Zhuang, B., and Chen, P.: Modeling of a severe dust event and its impacts on ozone photochemistry over the downstream Nanjing megacity of eastern China, Atmos. Environ., 160, 107–123, https://doi.org/10.1016/j.atmosenv.2017.04.010, 2017a.
Li, M. M., Wang, T., Xie, M., Zhuang, B., Li, S., Han, Y., and Chen, P.: Impacts of aerosol-radiation feedback on local air quality during a severe haze episode in Nanjing megacity, eastern China, Tellus B, 69, 1–16, https://doi.org/10.1080/16000889.2017.1339548, 2017b.
Li, Z., Niu, F., Fan, J., Liu, Y., Rosenfeld, D., and Ding, Y.: Long-term impacts of aerosols on the vertical development of clouds and precipitation, Nat. Geosci., 4, 888–894, https://doi.org/10.1038/ngeo1313, 2011.
Li, Z., Lau, W. K. M., Ramanathan, V., Wu, G., Ding, Y., Manoj, M. G., Liu, J., Qian, Y., Li, J., Zhou, T., Fan, J., Rosenfeld, D., Ming, Y., Wang, Y., Huang, J., Wang, B., Xu, X., Lee, S. S., Cribb, M., Zhang, F., Yang, X., Zhao, C., Takemura, T., Wang, K., Xia, X., Yin, Y., Zhang, H., Guo, J., Zhai, P. M., Sugimoto, N., Babu, S. S., and Brasseur, G. P.: Aerosol and monsoon climate interactions over Asia, Rev. Geophys., 54, 866–929, https://doi.org/10.1002/2015RG000500, 2016.
Li, Z., Wang, Y., Guo, J., Zhao, C., Cribb, M. C., Dong, X., Fan, J., Gong, D., Huang, J., and Jiang, M.: East Asian study of tropospheric aerosols and their impact on regional clouds, precipitation, and climate (EAST-AIRCPC), J. Geophys. Res.-Atmos., 124, 13026–13054, https://doi.org/10.1029/2019JD030758, 2019.
Liao, J., Wang, T., Wang, X., Xie, M., Jiang, Z., Huang, X., and Zhu, J.: Impacts of different urban canopy schemes in WRF/Chem on regional climate and air quality in Yangtze River Delta, China, Atmos. Res., 145, 226–243, https://doi.org/10.1016/j.atmosres.2014.04.005, 2014.
Lin, C.-Y., Zhao, C., Liu, X., Lin, N.-H., and Chen, W.-N.: Modelling of long-range transport of Southeast Asia biomass-burning aerosols to Taiwan and their radiative forcings over East Asia, Tellus B, 66, 23733, https://doi.org/10.3402/tellusb.v66.23733, 2014.
Lin, N.-H., Sayer, A. M., Wang, S.-H., Loftus, A. M., Hsiao, T.-C., Sheu, G.-R., Hsu, N. C., Tsay, S.-C., and Chantara, S.: Interactions between biomass-burning aerosols and clouds over Southeast Asia: Current status, challenges, and perspectives, Environ. Pollut., 195, 292–307, https://doi.org/10.1016/j.envpol.2014.06.036, 2014.
Liu, C., Wang, T., Chen, P., Li, M., Zhao, M., Zhao, K., Wang, M., and Yang, X.: Effects of Aerosols on the Precipitation of Convective Clouds: A Case Study in the Yangtze River Delta of China, J. Geophys. Res.-Atmos., 124, 7868–7885, https://doi.org/10.1029/2018JD029924, 2019.
Liu, G., Shao, H., Coakley Jr, J. A., Curry, J. A., Haggerty, J. A., and Tschudi, M. A.: Retrieval of cloud droplet size from visible and microwave radiometric measurements during INDOEX: Implication to aerosols' indirect radiative effect, J. Geophys. Res.-Atmos., 108, AAC 2-1–AAC 2-10, https://doi.org/10.1029/2001JD001395, 2003.
Liu, L., Huang, X., Ding, A., and Fu, C.: Dust-induced radiative feedbacks in north China: A dust storm episode modeling study using WRF-Chem, Atmos. Environ., 129, 43–54, https://doi.org/10.1016/j.atmosenv.2016.01.019, 2016.
Liu, L., Bai, Y., Lin, C., and Yang, H.: Evaluation of Regional Air Quality Numerical Forecasting System in Central China and Its Application for Aerosol Radiative Effect, Meteorol. Mon., 44, 1179–1190, https://doi.org/10.7519/j.issn.1000-0526.2018.09.006, 2018.
Liu, Q., Jia, X., Quan, J., Li, J., Li, X., Wu, Y., Chen, D., Wang, Z., and Liu, Y.: New positive feedback mechanism between boundary layer meteorology and secondary aerosol formation during severe haze events, Sci. Rep.-UK, 8, 1–8, https://doi.org/10.1038/s41598-018-24366-3, 2018.
Liu, X., Easter, R. C., Ghan, S. J., Zaveri, R., Rasch, P., Shi, X., Lamarque, J.-F., Gettelman, A., Morrison, H., Vitt, F., Conley, A., Park, S., Neale, R., Hannay, C., Ekman, A. M. L., Hess, P., Mahowald, N., Collins, W., Iacono, M. J., Bretherton, C. S., Flanner, M. G., and Mitchell, D.: Toward a minimal representation of aerosols in climate models: description and evaluation in the Community Atmosphere Model CAM5, Geosci. Model Dev., 5, 709–739, https://doi.org/10.5194/gmd-5-709-2012, 2012.
Liu, X., Zhang, Y., Zhang, Q., and He, K.: Application of online-coupled WRF/Chem-MADRID in East Asia: Model evaluation and climatic effects of anthropogenic aerosols, Atmos. Environ., 124, 321–336, https://doi.org/10.1016/j.atmosenv.2015.03.052, 2016.
Liu, Z., Yim, S. H. L., Wang, C., and Lau, N. C.: The Impact of the Aerosol Direct Radiative Forcing on Deep Convection and Air Quality in the Pearl River Delta Region, Geophys. Res. Lett., 45, 4410–4418, https://doi.org/10.1029/2018GL077517, 2018.
Liu, Z., Ming, Y., Zhao, C., Lau, N. C., Guo, J., Bollasina, M., and Yim, S. H. L.: Contribution of local and remote anthropogenic aerosols to a record-breaking torrential rainfall event in Guangdong Province, China, Atmos. Chem. Phys., 20, 223–241, https://doi.org/10.5194/acp-20-223-2020, 2020.
Lohmann, U. and Diehl, K.: Sensitivity studies of the importance of dust ice nuclei for the indirect aerosol effect on stratiform mixed-phase clouds, J. Atmos. Sci., 63, 968–982, https://doi.org/10.1175/JAS3662.1, 2006.
Lohmann, U. and Feichter, J.: Global indirect aerosol effects: a review, Atmos. Chem. Phys., 5, 715–737, https://doi.org/10.5194/acp-5-715-2005, 2005.
Ma, X. and Wen, W.: Modelling the Effect of Black Carbon and Sulfate Aerosol on the Regional Meteorology Factors, in: IOP Conf. Ser. Earth Environ. Sci., 12002, https://doi.org/10.1088/1755-1315/78/1/012002, 2017.
Ma, X., Chen, D., Wen, W., Sheng, L., Hu, J., Tong, H., and Wei, P.: Effect of Particle Pollution on Regional Meteorological Factors in China, J. Beijing Univ. Technol., 42, 285–295, https://doi.org/10.11936/bjutxb2015040075, 2016.
Mailler, S., Menut, L., Khvorostyanov, D., Valari, M., Couvidat, F., Siour, G., Turquety, S., Briant, R., Tuccella, P., Bessagnet, B., Colette, A., Létinois, L., Markakis, K., and Meleux, F.: CHIMERE-2017: from urban to hemispheric chemistry-transport modeling, Geosci. Model Dev., 10, 2397–2423, https://doi.org/10.5194/gmd-10-2397-2017, 2017.
Makar, P. A., Gong, W., Hogrefe, C., Zhang, Y., Curci, G., Žabkar, R., Milbrandt, J., Im, U., Balzarini, A., Baró, R., Bianconi, R., Cheung, P., Forkel, R., Gravel, S., Hirtl, M., Honzak, L., Hou, A., Jiménez-Guerrero, P., Langer, M., Moran, M. D., Pabla, B., Pérez, J. L., Pirovano, G., San José, R., Tuccella, P., Werhahn, J., Zhang, J., and Galmarini, S.: Feedbacks between air pollution and weather, part 2: Effects on chemistry, Atmos. Environ., 115, 499–526, https://doi.org/10.1016/j.atmosenv.2014.10.021, 2015a.
Makar, P. A., Gong, W., Milbrandt, J., Hogrefe, C., Zhang, Y., Curci, G., Žabkar, R., Im, U., Balzarini, A., Baró, R., Bianconi, R., Cheung, P., Forkel, R., Gravel, S., Hirtl, M., Honzak, L., Hou, A., Jiménez-Guerrero, P., Langer, M., Moran, M. D., Pabla, B., Pérez, J. L., Pirovano, G., San José, R., Tuccella, P., Werhahn, J., Zhang, J., and Galmarini, S.: Feedbacks between air pollution and weather, Part 1: Effects on weather, Atmos. Environ., 115, 442–469, https://doi.org/10.1016/j.atmosenv.2014.12.003, 2015b.
Manisalidis, I., Stavropoulou, E., Stavropoulos, A., and Bezirtzoglou, E.: Environmental and health impacts of air pollution: A review, Front. Public He., 8, 14, https://doi.org/10.3389/fpubh.2020.00014, 2020.
Marelle, L., Raut, J.-C., Law, K. S., Berg, L. K., Fast, J. D., Easter, R. C., Shrivastava, M., and Thomas, J. L.: Improvements to the WRF-Chem 3.5.1 model for quasi-hemispheric simulations of aerosols and ozone in the Arctic, Geosci. Model Dev., 10, 3661–3677, https://doi.org/10.5194/gmd-10-3661-2017, 2017.
Martin, D. E. and Leight, W. G.: Objective temperature estimates from mean circulation patterns, Mon. Weather Rev., 77, 275–283, https://doi.org/10.1175/1520-0493(1949)077<0275:OTEFMC>2.0.CO;2, 1949.
Martin, S. T., Schlenker, J. C., Malinowski, A., Hung, H., and Rudich, Y.: Crystallization of atmospheric sulfate-nitrate-ammonium particles, Geophys. Res. Lett., 30, 2102, https://doi.org/10.1029/2003GL017930, 2003.
Mass, C. and Ovens, D.: Fixing WRF's high speed wind bias: A new subgrid scale drag parameterization and the role of detailed verification, in: 24th conference on weather and forecasting and 20th conference on numerical weather prediction, preprints, 91st American meteorological society annual meeting, University of Washington, United States, 26 January 2011, 615–617, 2011.
McCormick, R. A. and Ludwig, J. H.: Climate modification by atmospheric aerosols, Science, 156, 1358–1359, https://doi.org/10.1126/science.156.3780.1358, 1967.
McMurry, P. H. and Friedlander, S. K.: New particle formation in the presence of an aerosol, Atmos. Environ., 13, 1635–1651, https://doi.org/10.1016/0004-6981(79)90322-6, 1979.
Miao, Y., Liu, S., Zheng, Y., and Wang, S.: Modeling the feedback between aerosol and boundary layer processes: a case study in Beijing, China, Environ. Sci. Pollut. Res., 23, 3342–3357, https://doi.org/10.1007/s11356-015-5562-8, 2016.
Miao, Y., Guo, J., Liu, S., Zhao, C., Li, X., Zhang, G., Wei, W., and Ma, Y.: Impacts of synoptic condition and planetary boundary layer structure on the trans-boundary aerosol transport from Beijing-Tianjin-Hebei region to northeast China, Atmos. Environ., 181, 1–11, https://doi.org/10.1016/j.atmosenv.2018.03.005, 2018.
Napari, I., Noppel, M., Vehkamäki, H., and Kulmala, M.: Parametrization of ternary nucleation rates for H2SO4-NH3-H2O vapors, J. Geophys. Res.-Atmos., 107, AAC 6-1–AAC 6-6, https://doi.org/10.1029/2002JD002132, 2002.
Nenes, A., Pandis, S. N., and Pilinis, C.: ISORROPIA: A new thermodynamic equilibrium model for multiphase multicomponent inorganic aerosols, Aquat. Geochem., 4, 123–152, https://doi.org/10.1023/A:1009604003981, 1998.
Nguyen, G. T. H., Shimadera, H., Sekiguchi, A., Matsuo, T., and Kondo, A.: Investigation of aerosol direct effects on meteorology and air quality in East Asia by using an online coupled modeling system, Atmos. Environ., 207, 182–196, https://doi.org/10.1016/j.atmosenv.2019.03.017., 2019a.
Nguyen, G. T. H., Shimadera, H., Uranishi, K., Matsuo, T., Kondo, A., and Thepanondh, S.: Numerical assessment of PM2.5 and O3 air quality in continental Southeast Asia: Baseline simulation and aerosol direct effects investigation, Atmos. Environ., 219, 117054, https://doi.org/10.1016/j.atmosenv.2019.117054, 2019b.
North, G. R., Pyle, J. A., and Zhang, F.: Encyclopedia of atmospheric sciences, Academic Press, Cambridge, Massachusetts, United States, 2874 pp., 2014.
Odum, J. R., Jungkamp, T. P. W., Griffin, R. J., Flagan, R. C., and Seinfeld, J. H.: The atmospheric aerosol-forming potential of whole gasoline vapor, Science, 276, 96–99, https://doi.org/10.1126/science.276.5309.96, 1997.
Park, S.-Y., Lee, H.-J., Kang, J.-E., Lee, T., and Kim, C.-H.: Aerosol radiative effects on mesoscale cloud-precipitation variables over Northeast Asia during the MAPS-Seoul 2015 campaign, Atmos. Environ., 172, 109–123, https://doi.org/10.1016/j.atmosenv.2017.10.044, 2018.
Penner, J. E., Dong, X., and Chen, Y.: Observational evidence of a change in radiative forcing due to the indirect aerosol effect, Nature, 427, 231–234, https://doi.org/10.1038/nature02234, 2004.
Quaas, J., Boucher, O., Bellouin, N., and Kinne, S.: Satellite-based estimate of the direct and indirect aerosol climate forcing, J. Geophys. Res.-Atmos., 113, D05204, https://doi.org/10.1029/2007JD008962, 2008.
Qiu, Y., Liao, H., Zhang, R., and Hu, J.: Simulated impacts of direct radiative effects of scattering and absorbing aerosols on surface layer aerosol concentrations in China during a heavily polluted event in february 2014, J. Geophys. Res., 122, 5955–5975, https://doi.org/10.1002/2016JD026309, 2017.
Ramboll Environment and Health: User's Guide: Comprehensive Air quality Model with extensions, Version 7.10, Ramboll, Novato, CA, https://camxwp.azurewebsites.net/Files/CAMxUsersGuide_v7.10.pdf (last access: 20 March 2022), 2020.
Reid, J. S., Koppmann, R., Eck, T. F., and Eleuterio, D. P.: A review of biomass burning emissions part II: intensive physical properties of biomass burning particles, Atmos. Chem. Phys., 5, 799–825, https://doi.org/10.5194/acp-5-799-2005, 2005.
Rohde, R. A. and Muller, R. A.: Air pollution in China: mapping of concentrations and sources, PLoS One, 10, e0135749, https://doi.org/10.1371/journal.pone.0135749, 2015.
Rosenfeld, D.: Suppression of rain and snow by urban and industrial air pollution, Science, 287, 1793–1796, https://doi.org/10.1126/science.287.5459.1793, 2000.
Rosenfeld, D., Lohmann, U., Raga, G. B., O'Dowd, C. D., Kulmala, M., Fuzzi, S., Reissell, A., and Andreae, M. O.: Flood or drought: How do aerosols affect precipitation?, Science, 321, 1309–1313, https://doi.org/10.1126/science.1160606, 2008.
Rosenfeld, D., Andreae, M. O., Asmi, A., Chin, M., de Leeuw, G., Donovan, D. P., Kahn, R., Kinne, S., Kivekäs, N., and Kulmala, M.: Global observations of aerosol-cloud-precipitation-climate interactions, Rev. Geophys., 52, 750–808, https://doi.org/10.1002/2013RG000441, 2014.
Rosenfeld, D., Zhu, Y., Wang, M., Zheng, Y., Goren, T., and Yu, S.: Aerosol-driven droplet concentrations dominate coverage and water of oceanic low-level clouds, Science, 363, 1–9, https://doi.org/10.1126/science.aav0566, 2019.
Saleh, R., Robinson, E. S., Tkacik, D. S., Ahern, A. T., Liu, S., Aiken, A. C., Sullivan, R. C., Presto, A. A., Dubey, M. K., and Yokelson, R. J.: Brownness of organics in aerosols from biomass burning linked to their black carbon content, Nat. Geosci., 7, 647–650, https://doi.org/10.1038/ngeo2220, 2014.
Sarangi, C., Tripathi, S. N., Tripathi, S., and Barth, M. C.: Aerosol-cloud associations over Gangetic Basin during a typical monsoon depression event using WRF-Chem simulation, J. Geophys. Res.-Atmos., 120, 10–974, https://doi.org/10.1002/2015JD023634, 2015.
Satheesh, S. K. and Moorthy, K. K.: Radiative effects of natural aerosols: A review, Atmos. Environ., 39, 2089–2110, https://doi.org/10.1016/j.atmosenv.2004.12.029, 2005.
Sato, Y. and Suzuki, K.: How do aerosols affect cloudiness?, Science, 363, 580–581, https://doi.org/10.1126/science.aaw3720, 2019.
Saylor, R. D., Baker, B. D., Lee, P., Tong, D., Pan, L., and Hicks, B. B.: The particle dry deposition component of total deposition from air quality models: right, wrong or uncertain?, Tellus B, 71, 1550324, https://doi.org/10.1080/16000889.2018.1550324, 2019.
Seaman, N. L.: Meteorological modeling for air-quality assessments, Atmos. Environ., 34, 2231–2259, https://doi.org/10.1016/S1352-2310(99)00466-5, 2000.
Seethala, C., Pandithurai, G., Fast, J. D., Polade, S. D., Reddy, M. S., and Peckham, S. E.: Evaluating WRF-Chem multi-scale model in simulating aerosol radiative properties over the tropics-a case study over India, MAPAN-Journal of Metrology Society of India, 26, 269–284, https://doi.org/10.1007/s12647-011-0025-2, 2011.
Seinfeld, J. and Pandis, S.: Atmospheric chemistry and physics, in: Environment: an interdisciplinary anthology, edited by: Kramer, A. and Rumold, R., Yale University Press, New Haven, United States, 454–468, https://doi.org/10.12987/9780300150315, 2008.
Sekiguchi, A., Shimadera, H., and Kondo, A.: Impact of aerosol direct effect on wintertime PM2.5 simulated by an online coupled meteorology-air quality model over east asia, Aerosol Air Qual. Res., 18, 1068–1079, https://doi.org/10.4209/aaqr.2016.06.0282, 2018.
Sekiguchi, M., Nakajima, T., Suzuki, K., Kawamoto, K., Higurashi, A., Rosenfeld, D., Sano, I., and Mukai, S.: A study of the direct and indirect effects of aerosols using global satellite data sets of aerosol and cloud parameters, J. Geophys. Res.-Atmos., 108, 4699, https://doi.org/10.1029/2002JD003359, 2003.
Shahid, M. Z., Shahid, I., Chishtie, F., Shahzad, M. I., and Bulbul, G.: Analysis of a dense haze event over North-eastern Pakistan using WRF-Chem model and remote sensing, J. Atmos. Solar-Terr. Phy., 182, 229–241, https://doi.org/10.1016/j.jastp.2018.12.007, 2019.
Shao, Y.: Simplification of a dust emission scheme and comparison with data, J. Geophys. Res.-Atmos., 109, D10202, https://doi.org/10.1029/2003JD004372, 2004.
Shao, Y. and Dong, C. H.: A review on East Asian dust storm climate, modelling and monitoring, Glob. Planet. Change, 52, 1–22, https://doi.org/10.1016/j.gloplacha.2006.02.011, 2006.
Shen, H., Shi, H., Shi, H., and Ma, X.: Simulation Study of Influence of Aerosol Pollution on Regional Meteorological Factors in Beijing-Tianjin-Hebei Region, J. Anhui Agric. Sci., 43, 207–210, https://doi.org/10.13989/j.cnki.0517-6611.2015.25.217, 2015.
Shen, X., Jiang, X., Liu, D., Zu, F., and Fan, S.: Simulations of Anthropogenic Aerosols Effects on the Intensity and Precipitation of Typhoon Fitow (1323) Using WRF-Chem Model, Chinese J. Atmos. Sci., 41, 960–974, https://doi.org/10.3878/j.issn.1006-9895.1703.16216, 2017.
Singh, P., Sarawade, P., and Adhikary, B.: Carbonaceous Aerosol from Open Burning and its Impact on Regional Weather in South Asia, Aerosol Air Qual. Res., 20, 419–431, https://doi.org/10.4209/aaqr.2019.03.0146, 2020.
Slinn, W. G. N.: Precipitation scavenging, in: Atmospheric science and power production, edited by: Randerson, D., U.S. Department of Energy, Washington, D.C., United States, 466–532, 1984, 1984.
Soni, P., Tripathi, S. N., and Srivastava, R.: Radiative effects of black carbon aerosols on Indian monsoon: a study using WRF-Chem model, Theor. Appl. Climatol., 132, 115–134, https://doi.org/10.1007/s00704-017-2057-1, 2018.
Srinivas, R., Panicker, A. S., Parkhi, N. S., Peshin, S. K., and Beig, G.: Sensitivity of online coupled model to extreme pollution event over a mega city Delhi, Atmos. Pollut. Res., 7, 25–30, https://doi.org/10.1016/j.apr.2015.07.001, 2016.
Su, L. and Fung, J. C. H.: Investigating the role of dust in ice nucleation within clouds and further effects on the regional weather system over East Asia – Part 1: model development and validation, Atmos. Chem. Phys., 18, 8707–8725, https://doi.org/10.5194/acp-18-8707-2018, 2018a.
Su, L. and Fung, J. C. H.: Investigating the role of dust in ice nucleation within clouds and further effects on the regional weather system over East Asia – Part 2: modification of the weather system, Atmos. Chem. Phys., 18, 11529–11545, https://doi.org/10.5194/acp-18-11529-2018, 2018b.
Sud, Y. C. and Walker, G. K.: A review of recent research on improvement of physical parameterizations in the GLA GCM, National Aeronautics and Space Administration, United States, Open File Rep. 100771, 70 pp., 1990.
Sun, K., Liu, H., Wang, X., Peng, Z., and Xiong, Z.: The aerosol radiative effect on a severe haze episode in the Yangtze River Delta, J. Meteorol. Res., 31, 865–873, https://doi.org/10.1007/s13351-017-7007-4, 2017.
Takemura, T., Nakajima, T., Higurashi, A., Ohta, S., and Sugimoto, N.: Aerosol distributions and radiative forcing over the Asian Pacific region simulated by Spectral Radiation-Transport Model for Aerosol Species (SPRINTARS), J. Geophys. Res.-Atmos., 108, 8659, https://doi.org/10.1029/2002JD003210, 2003.
Tang, Y., Han, Y., Ma, X., and Liu, Z.: Elevated heat pump effects of dust aerosol over Northwestern China during summer, Atmos. Res., 203, 95–104, https://doi.org/10.1016/j.atmosres.2017.12.004, 2018.
Ten Hoeve, J. E. and Jacobson, M. Z.: Worldwide health effects of the Fukushima Daiichi nuclear accident, Energy Environ. Sci., 5, 8743–8757, https://doi.org/10.1039/c2ee22019a, 2012.
Thompson, G. and Eidhammer, T.: A study of aerosol impacts on clouds and precipitation development in a large winter cyclone, J. Atmos. Sci., 71, 3636–3658, https://doi.org/10.1175/JAS-D-13-0305.1, 2014.
Toon, O. B., McKay, C. P., Ackerman, T. P., and Santhanam, K.: Rapid calculation of radiative heating rates and photodissociation rates in inhomogeneous multiple scattering atmospheres, J. Geophys. Res.-Atmos., 94, 16287–16301, https://doi.org/10.1029/JD094iD13p16287, 1989.
Tremback, C., Tripoli, G., Arritt, R., Cotton, W. R., and Pielke, R. A.: The regional atmospheric modeling system, in: Proceedings of an International Conference on Development Applications of Computer Techniques Environmental Studies, Los Angeles, United States, November 1986, 601–607, 1986.
Tsay, S.-C., Hsu, N. C., Lau, W. K.-M., Li, C., Gabriel, P. M., Ji, Q., Holben, B. N., Welton, E. J., Nguyen, A. X., and Janjai, S.: From BASE-ASIA toward 7-SEAS: A satellite-surface perspective of boreal spring biomass-burning aerosols and clouds in Southeast Asia, Atmos. Environ., 78, 20–34, https://doi.org/10.1016/j.atmosenv.2012.12.013, 2013.
Twomey, S.: The influence of pollution on the shortwave albedo of clouds, J. Atmos. Sci., 34, 1149–1152, https://doi.org/10.1175/1520-0469(1977)034<1149:TIOPOT>2.0.CO;2, 1977.
US Environmental Protection Agency: Meteorological Model Performance for Annual 2016 Simulation WRF v3.8, Techn. Support Documentation, https://www.epa.gov/sites/default/files/2020-10/documents/met_model_performance-2016_wrf.pdf (last access: 20 March 2022), 2019.
Uno, I., Wang, Z., Chiba, M., Chun, Y. S., Gong, S. L., Hara, Y., Jung, E., Lee, S., Liu, M., and Mikami, M.: Dust model intercomparison (DMIP) study over Asia: Overview, J. Geophys. Res.-Atmos., 111, D12213, https://doi.org/10.1029/2005JD006575, 2006.
Vehkamäki, H., Kulmala, M., Napari, I., Lehtinen, K. E. J., Timmreck, C., Noppel, M., and Laaksonen, A.: An improved parameterization for sulfuric acid-water nucleation rates for tropospheric and stratospheric conditions, J. Geophys. Res.-Atmos., 107, AAC 3-1–AAC 3-10, https://doi.org/10.1029/2002JD002184, 2002.
Wang, D., Jiang, B., Lin, W., and Gu, F.: Effects of aerosol-radiation feedback and topography during an air pollution event over the North China Plain during December 2017, Atmos. Pollut. Res., 10, 587–596, https://doi.org/10.1016/j.apr.2018.10.006, 2019a.
Wang, H. and Niu, T.: Sensitivity studies of aerosol data assimilation and direct radiative feedbacks in modeling dust aerosols, Atmos. Environ., 64, 208–218, https://doi.org/10.1016/j.atmosenv.2012.09.066, 2013.
Wang, H., Zhang, X., Gong, S., Chen, Y., Shi, G., and Li, W.: Radiative feedback of dust aerosols on the East Asian dust storms, J. Geophys. Res.-Atmos., 115, D23214, https://doi.org/10.1029/2009JD013430, 2010.
Wang, H., Shi, G., Zhu, J., Chen, B., Che, H., and Zhao, T.: Case study of longwave contribution to dust radiative effects over East Asia, Chinese Sci. Bull., 58, 3673–3681, https://doi.org/10.1007/s11434-013-5752-z, 2013.
Wang, H., Shi, G. Y., Zhang, X. Y., Gong, S. L., Tan, S. C., Chen, B., Che, H. Z., and Li, T.: Mesoscale modelling study of the interactions between aerosols and PBL meteorology during a haze episode in China Jing–Jin–Ji and its near surrounding region – Part 2: Aerosols' radiative feedback effects, Atmos. Chem. Phys., 15, 3277–3287, https://doi.org/10.5194/acp-15-3277-2015, 2015.
Wang, H., Peng, Y., Zhang, X., Liu, H., Zhang, M., Che, H., Cheng, Y., and Zheng, Y.: Contributions to the explosive growth of PM2.5 mass due to aerosol–radiation feedback and decrease in turbulent diffusion during a red alert heavy haze in Beijing–Tianjin–Hebei, China, Atmos. Chem. Phys., 18, 17717–17733, https://doi.org/10.5194/acp-18-17717-2018, 2018.
Wang, J., Wang, S., Jiang, J., Ding, A., Zheng, M., Zhao, B., Wong, D. C., Zhou, W., Zheng, G., Wang, L., Pleim, J. E., and Hao, J.: Impact of aerosol-meteorology interactions on fine particle pollution during China's severe haze episode in January 2013, Environ. Res. Lett., 9, 1–7, https://doi.org/10.1088/1748-9326/9/9/094002, 2014.
Wang, J., Allen, D. J., Pickering, K. E., Li, Z., and He, H.: Impact of aerosol direct effect on East Asian air quality during the EAST-AIRE campaign, J. Geophys. Res.-Atmos., 121, 6534–6554, https://doi.org/10.1002/2016JD025108, 2016.
Wang, J., Xing, J., Mathur, R., Pleim, J. E., Wang, S., Hogrefe, C., Gan, C.-M., Wong, D. C., and Hao, J.: Historical trends in PM2.5-related premature mortality during 1990–2010 across the northern hemisphere, Environ. Health Perspect., 125, 400–408, https://doi.org/10.1289/EHP298, 2017.
Wang, K., Yahya, K., Zhang, Y., Hogrefe, C., Pouliot, G., Knote, C., Hodzic, A., San Jose, R., Perez, J. L., and Jiménez-Guerrero, P.: A multi-model assessment for the 2006 and 2010 simulations under the Air Quality Model Evaluation International Initiative (AQMEII) Phase 2 over North America: Part II. Evaluation of column variable predictions using satellite data, Atmos. Environ., 115, 587–603, https://doi.org/10.1016/j.atmosenv.2014.07.044, 2015.
Wang, K., Zhang, Y., Zhang, X., Fan, J., Leung, L. R., Zheng, B., Zhang, Q., and He, K.: Fine-scale application of WRF-CAM5 during a dust storm episode over East Asia: Sensitivity to grid resolutions and aerosol activation parameterizations, Atmos. Environ., 176, 1–20, https://doi.org/10.1016/j.atmosenv.2017.12.014, 2018.
Wang, L., Fu, J. S., Wei, W., Wei, Z., Meng, C., Ma, S., and Wang, J.: How aerosol direct effects influence the source contributions to PM2.5 concentrations over Southern Hebei, China in severe winter haze episodes, Front. Environ. Sci. Eng., 12, 13, https://doi.org/10.1007/s11783-018-1014-2, 2018.
Wang, Z., Wang, Z., Li, J., Zheng, H., Yan, P., and Li, J.: Development of a meteorology-chemistry two-way coupled numerical model (WRF-NAQPMS) and its application in a severe autumn haze simulation over the Beijing-Tianjin-Hebei area, China. Clim, Environ. Res., 19, 153–163, https://doi.org/10.3878/j.issn.1006-9585.2014.13231, 2014.
Wang, Z., Huang, X., and Ding, A.: Dome effect of black carbon and its key influencing factors: a one-dimensional modelling study, Atmos. Chem. Phys., 18, 2821–2834, https://doi.org/10.5194/acp-18-2821-2018, 2018.
Wang, Z., Huang, X., and Ding, A.: Optimization of vertical grid setting for air quality modelling in China considering the effect of aerosol-boundary layer interaction, Atmos. Environ., 210, 1–13, https://doi.org/10.1016/j.atmosenv.2019.04.042, 2019.
Wang, Z. F., Li, J., Wang, Z., Yang, W., Tang, X., Ge, B., Yan, P., Zhu, L., Chen, X., and Chen, H.: Modeling study of regional severe hazes over mid-eastern China in January 2013 and its implications on pollution prevention and control, Sci. China Earth Sci., 57, 3–13, https://doi.org/10.1007/s11430-013-4793-0, 2014.
Wendisch, M., Keil, A., Müller, D., Wandinger, U., Wendling, P., Stifter, A., Petzold, A., Fiebig, M., Wiegner, M., and Freudenthaler, V.: Aerosol-radiation interaction in the cloudless atmosphere during LACE 98 1. Measured and calculated broadband solar and spectral surface insolations, J. Geophys. Res.-Atmos., 107, LAC 6-1–LAC 6-20, https://doi.org/10.1029/2000JD000226, 2002.
Wexler, A. S., Lurmann, F. W., and Seinfeld, J. H.: Modelling urban and regional aerosols-I. Model development, Atmos. Environ., 28, 531–546, https://doi.org/10.1016/1352-2310(94)90129-5, 1994.
Whitby, K. T.: The physical characteristics of sulfur aerosols, in: Sulfur in the Atmosphere, Elsevier, 135–159, https://doi.org/10.1016/B978-0-08-022932-4.50018-5, 1978.
Wiedinmyer, C., Akagi, S. K., Yokelson, R. J., Emmons, L. K., Al-Saadi, J. A., Orlando, J. J., and Soja, A. J.: The Fire INventory from NCAR (FINN): a high resolution global model to estimate the emissions from open burning, Geosci. Model Dev., 4, 625–641, https://doi.org/10.5194/gmd-4-625-2011, 2011.
Wilcox, E. M.: Direct and semi-direct radiative forcing of smoke aerosols over clouds, Atmos. Chem. Phys., 12, 139–149, https://doi.org/10.5194/acp-12-139-2012, 2012.
Wong, D. C., Pleim, J., Mathur, R., Binkowski, F., Otte, T., Gilliam, R., Pouliot, G., Xiu, A., Young, J. O., and Kang, D.: WRF-CMAQ two-way coupled system with aerosol feedback: software development and preliminary results, Geosci. Model Dev., 5, 299–312, https://doi.org/10.5194/gmd-5-299-2012, 2012.
Wu, J., Bei, N., Hu, B., Liu, S., Zhou, M., Wang, Q., Li, X., Liu, L., Feng, T., Liu, Z., Wang, Y., Cao, J., Tie, X., Wang, J., Molina, L. T., and Li, G.: Aerosol–radiation feedback deteriorates the wintertime haze in the North China Plain, Atmos. Chem. Phys., 19, 8703–8719, https://doi.org/10.5194/acp-19-8703-2019, 2019a.
Wu, J., Bei, N., Hu, B., Liu, S., Zhou, M., Wang, Q., Li, X., Liu, L., Feng, T., Liu, Z., Wang, Y., Cao, J., Tie, X., Wang, J., Molina, L. T., and Li, G.: Is water vapor a key player of the wintertime haze in North China Plain?, Atmos. Chem. Phys., 19, 8721–8739, https://doi.org/10.5194/acp-19-8721-2019, 2019b.
Wu, L., Su, H., and Jiang, J. H.: Regional simulation of aerosol impacts on precipitation during the East Asian summer monsoon, J. Geophys. Res.-Atmos., 118, 6454–6467, https://doi.org/10.1002/jgrd.50527, 2013.
Wu, W. and Zhang, Y.: Effects of particulate matter (PM2.5) and associated acidity on ecosystem functioning: response of leaf litter breakdown, Environ. Sci. Pollut. Res., 25, 30720–30727, https://doi.org/10.1007/s11356-018-2922-1, 2018.
Wu, Y., Han, Y., Voulgarakis, A., Wang, T., Li, M., Wang, Y., Xie, M., Zhuang, B., and Li, S.: An agricultural biomass burning episode in eastern China: Transport, optical properties, and impacts on regional air quality, J. Geophys. Res.-Atmos., 122, 2304–2324, https://doi.org/10.1002/2016JD025319, 2017.
Xie, M., Liao, J., Wang, T., Zhu, K., Zhuang, B., Han, Y., Li, M., and Li, S.: Modeling of the anthropogenic heat flux and its effect on regional meteorology and air quality over the Yangtze River Delta region, China, Atmos. Chem. Phys., 16, 6071–6089, https://doi.org/10.5194/acp-16-6071-2016, 2016.
Xing, J., Mathur, R., Pleim, J., Hogrefe, C., Gan, C.-M., Wong, D. C., and Wei, C.: Can a coupled meteorology–chemistry model reproduce the historical trend in aerosol direct radiative effects over the Northern Hemisphere?, Atmos. Chem. Phys., 15, 9997–10018, https://doi.org/10.5194/acp-15-9997-2015, 2015a.
Xing, J., Mathur, R., Pleim, J., Hogrefe, C., Gan, C.-M., Wong, D. C., Wei, C., Gilliam, R., and Pouliot, G.: Observations and modeling of air quality trends over 1990–2010 across the Northern Hemisphere: China, the United States and Europe, Atmos. Chem. Phys., 15, 2723–2747, https://doi.org/10.5194/acp-15-2723-2015, 2015b.
Xing, J., Mathur, R., Pleim, J., Hogrefe, C., Gan, C., Wong, D. C., Wei, C., and Wang, J.: Air pollution and climate response to aerosol direct radiative effects: A modeling study of decadal trends across the northern hemisphere, J. Geophys. Res.-Atmos., 120, 12–221, https://doi.org/10.1002/2015JD023933, 2015c.
Xing, J., Wang, J., Mathur, R., Pleim, J., Wang, S., Hogrefe, C., Gan, C.-M., Wong, D. C., and Hao, J.: Unexpected benefits of reducing aerosol cooling effects, Environ. Sci. Technol., 50, 7527–7534, https://doi.org/10.1021/acs.est.6b00767, 2016.
Xing, J., Wang, J., Mathur, R., Wang, S., Sarwar, G., Pleim, J., Hogrefe, C., Zhang, Y., Jiang, J., Wong, D. C., and Hao, J.: Impacts of aerosol direct effects on tropospheric ozone through changes in atmospheric dynamics and photolysis rates, Atmos. Chem. Phys., 17, 9869–9883, https://doi.org/10.5194/acp-17-9869-2017, 2017.
Yahya, K., Wang, K., Gudoshava, M., Glotfelty, T., and Zhang, Y.: Application of WRF/Chem over North America under the AQMEII Phase 2: Part I. Comprehensive evaluation of 2006 simulation, Atmos. Environ., 115, 733–755, https://doi.org/10.1016/j.atmosenv.2014.08.063, 2015.
Yan, J., Wang, X., Gong, P., Wang, C., and Cong, Z.: Review of brown carbon aerosols: Recent progress and perspectives, Sci. Total Environ., 634, 1475–1485, https://doi.org/10.1016/j.scitotenv.2018.04.083, 2018.
Yang, J., Duan, K., Kang, S., Shi, P., and Ji, Z.: Potential feedback between aerosols and meteorological conditions in a heavy pollution event over the Tibetan Plateau and Indo-Gangetic Plain, Clim. Dynam., 48, 2901–2917, https://doi.org/10.1007/s00382-016-3240-2, 2017.
Yang, J., Kang, S., Ji, Z., and Chen, D.: Modeling the origin of anthropogenic black carbon and its climatic effect over the Tibetan Plateau and surrounding regions, J. Geophys. Res.-Atmos., 123, 671–692, https://doi.org/10.1002/2017JD027282, 2018.
Yang, T. and Liu, Y.: Impact of anthropogenic pollution on “7.21” extreme heavy rainstorm, J. Meteorol. Sci., 37, 742–752, https://doi.org/10.3969/2016jms.0074, 2017a.
Yang, T. and Liu, Y.: Mechanism analysis of the impacts of aerosol direct effects on a rainstorm, J. Trop. Meteorol., 33, 762–773, https://doi.org/10.16032/j.issn.1004-4965.2017.05.019, 2017b.
Yang, Y., Tang, J., Sun, J., Wang, L., Wang, X., Zhang, Y., Qu, Q., and Zhao, W.: Synoptic Effect of a Heavy Haze Episode over North China, Clim. Environ. Res., 20, 555–570, https://doi.org/10.3878/j.issn.1006-9585.2015.15018, 2015.
Yang, Y., Fan, J., Leung, L. R., Zhao, C., Li, Z., and Rosenfeld, D.: Mechanisms contributing to suppressed precipitation in Mt. Hua of central China. Part I: Mountain valley circulation, J. Atmos. Sci., 73, 1351–1366, https://doi.org/10.1175/JAS-D-15-0233.1, 2016.
Yang, Y., Zhao, C., Dong, X., Fan, G., Zhou, Y., Wang, Y., Zhao, L., Lv, F., and Yan, F.: Toward understanding the process-level impacts of aerosols on microphysical properties of shallow cumulus cloud using aircraft observations, Atmos. Res., 221, 27–33, https://doi.org/10.1016/j.atmosres.2019.01.027, 2019.
Yao, H., Song, Y., Liu, M., Archer-Nicholls, S., Lowe, D., McFiggans, G., Xu, T., Du, P., Li, J., Wu, Y., Hu, M., Zhao, C., and Zhu, T.: Direct radiative effect of carbonaceous aerosols from crop residue burning during the summer harvest season in East China, Atmos. Chem. Phys., 17, 5205–5219, https://doi.org/10.5194/acp-17-5205-2017, 2017.
Yasunari, T. J. and Yamazaki, K.: Impacts of Asian dust storm associated with the stratosphere-to-troposphere transport in the spring of 2001 and 2002 on dust and tritium variations in Mount Wrangell ice core, Alaska, Atmos. Environ., 43, 2582–2590, https://doi.org/10.1016/j.atmosenv.2009.02.025, 2009.
Yiğit, E., Knížová, P. K., Georgieva, K., and Ward, W.: A review of vertical coupling in the Atmosphere–Ionosphere system: Effects of waves, sudden stratospheric warmings, space weather, and of solar activity, J. Atmos. Solar-Terr. Phy., 141, 1–12, https://doi.org/10.1016/j.jastp.2016.02.011, 2016.
Yoo, J.-W., Jeon, W., Park, S.-Y., Park, C., Jung, J., Lee, S.-H., and Lee, H. W.: Investigating the regional difference of aerosol feedback effects over South Korea using the WRF-CMAQ two-way coupled modeling system, Atmos. Environ., 218, 116968, https://doi.org/10.1016/j.atmosenv.2019.116968, 2019.
Yoon, J., Chang, D. Y., Lelieveld, J., Pozzer, A., Kim, J., and Yum, S. S.: Empirical evidence of a positive climate forcing of aerosols at elevated albedo, Atmos. Res., 229, 269–279, https://doi.org/10.1016/j.atmosres.2019.07.001, 2019.
Yu, F.: Binary H2SO4-H2O homogeneous nucleation based on kinetic quasi-unary nucleation model: Look-up tables, J. Geophys. Res.-Atmos., 111, D04201, https://doi.org/10.1029/2005JD006358, 2006.
Yu, F. and Luo, G.: Simulation of particle size distribution with a global aerosol model: contribution of nucleation to aerosol and CCN number concentrations, Atmos. Chem. Phys., 9, 7691–7710, https://doi.org/10.5194/acp-9-7691-2009, 2009.
Yu, H., Kaufman, Y. J., Chin, M., Feingold, G., Remer, L. A., Anderson, T. L., Balkanski, Y., Bellouin, N., Boucher, O., Christopher, S., DeCola, P., Kahn, R., Koch, D., Loeb, N., Reddy, M. S., Schulz, M., Takemura, T., and Zhou, M.: A review of measurement-based assessments of the aerosol direct radiative effect and forcing, Atmos. Chem. Phys., 6, 613–666, https://doi.org/10.5194/acp-6-613-2006, 2006.
Yuan, T., Chen, S., Huang, J., Wu, D., Lu, H., Zhang, G., Ma, X., Chen, Z., Luo, Y., and Ma, X.: Influence of dynamic and thermal forcing on the meridional transport of Taklimakan Desert dust in spring and summer, J. Clim., 32, 749–767, https://doi.org/10.1175/JCLI-D-18-0361.1, 2019.
Zaveri, R. A., Easter, R. C., Fast, J. D., and Peters, L. K.: Model for simulating aerosol interactions and chemistry (MOSAIC), J. Geophys. Res.-Atmos., 113, D13204, https://doi.org/10.1029/2007JD008782, 2008.
Zhan, J., Chang, W., Li, W., Wang, Y., Chen, L., and Yan, J.: Impacts of meteorological conditions, aerosol radiative feedbacks, and emission reduction scenarios on the coastal haze episodes in southeastern China in December 2013, J. Appl. Meteorol. Climatol., 56, 1209–1229, https://doi.org/10.1175/JAMC-D-16-0229.1, 2017.
Zhang, B., Wang, Y., and Hao, J.: Simulating aerosol–radiation–cloud feedbacks on meteorology and air quality over eastern China under severe haze conditionsin winter, Atmos. Chem. Phys., 15, 2387–2404, https://doi.org/10.5194/acp-15-2387-2015, 2015.
Zhang, H., DeNero, S. P., Joe, D. K., Lee, H.-H., Chen, S.-H., Michalakes, J., and Kleeman, M. J.: Development of a source oriented version of the WRF/Chem model and its application to the California regional PM10 PM2.5 air quality study, Atmos. Chem. Phys., 14, 485–503, https://doi.org/10.5194/acp-14-485-2014, 2014.
Zhang, H., Cheng, S., Li, J., Yao, S., and Wang, X.: Investigating the aerosol mass and chemical components characteristics and feedback effects on the meteorological factors in the Beijing-Tianjin-Hebei region, China, Environ. Pollut., 244, 495–502, https://doi.org/10.1016/j.envpol.2018.10.087, 2019.
Zhang, L., Wang, T., Lv, M., and Zhang, Q.: On the severe haze in Beijing during January 2013: Unraveling the effects of meteorological anomalies with WRF-Chem, Atmos. Environ., 104, 11–21, https://doi.org/10.1016/j.atmosenv.2015.01.001, 2015.
Zhang, L., Gong, S., Zhao, T., Zhou, C., Wang, Y., Li, J., Ji, D., He, J., Liu, H., Gui, K., Guo, X., Gao, J., Shan, Y., Wang, H., Wang, Y., Che, H., and Zhang, X.: Development of WRF/CUACE v1.0 model and its preliminary application in simulating air quality in China, Geosci. Model Dev., 14, 703–718, https://doi.org/10.5194/gmd-14-703-2021, 2021.
Zhang, X., Zhang, Q., Hong, C., Zheng, Y., Geng, G., Tong, D., Zhang, Y., and Zhang, X.: Enhancement of PM2.5 Concentrations by Aerosol-Meteorology Interactions Over China, J. Geophys. Res.-Atmos., 123, 1179–1194, https://doi.org/10.1002/2017JD027524, 2018.
Zhang, X. Y., Gong, S. L., Shen, Z. X., Mei, F. M., Xi, X. X., Liu, L. C., Zhou, Z. J., Wang, D., Wang, Y. Q., and Cheng, Y.: Characterization of soil dust aerosol in China and its transport and distribution during 2001 ACE-Asia: 1. Network observations, J. Geophys. Res.-Atmos., 108, 4261, https://doi.org/10.1029/2002JD002632, 2003a.
Zhang, X. Y., Gong, S. L., Zhao, T. L., Arimoto, R., Wang, Y. Q., and Zhou, Z. J.: Sources of Asian dust and role of climate change versus desertification in Asian dust emission, Geophys. Res. Lett., 30, 2272, https://doi.org/10.1029/2003GL018206, 2003b.
Zhang, Y.: Online-coupled meteorology and chemistry models: history, current status, and outlook, Atmos. Chem. Phys., 8, 2895–2932, https://doi.org/10.5194/acp-8-2895-2008, 2008.
Zhang, Y., Pun, B., Vijayaraghavan, K., Wu, S., Seigneur, C., Pandis, S. N., Jacobson, M. Z., Nenes, A., and Seinfeld, J. H.: Development and application of the model of aerosol dynamics, reaction, ionization, and dissolution (MADRID), J. Geophys. Res.-Atmos., 109, D01202, https://doi.org/10.1029/2003JD003501, 2004.
Zhang, Y., Hu, X. M., Howell, G. W., Sills, E., Fast, J. D., Gustafson Jr, W. I., Zaveri, R. A., Grell, G. A., Peckham, S. E., and McKeen, S. A.: Modeling atmospheric aerosols in WRF/CHEM, in: WRF/MM5 Users's Workshop, 27–30 June 2005, Boulder, Colorado, United States, 1–4, 2005.
Zhang, Y., Pan, Y., Wang, K., Fast, J. D., and Grell, G. A.: WRF/Chem-MADRID: Incorporation of an aerosol module into WRF/Chem and its initial application to the TexAQS2000 episode, J. Geophys. Res.-Atmos., 115, D18202, https://doi.org/10.1029/2009JD013443, 2010.
Zhang, Y., Karamchandani, P., Glotfelty, T., Streets, D. G., Grell, G., Nenes, A., Yu, F., and Bennartz, R.: Development and initial application of the global-through-urban weather research and forecasting model with chemistry (GU-WRF/Chem), J. Geophys. Res.-Atmos., 117, D20206, https://doi.org/10.1029/2012JD017966, 2012.
Zhang, Y., Zhang, X., Cai, C., Wang, K., and Wang, L.: Studying Aerosol-Cloud-Climate Interactions over East Asia Using WRF/Chem, in: Air Pollution Modeling and its Application XXIII, Springer, 61–66, https://doi.org/10.1007/978-3-319-04379-1_10, 2014.
Zhang, Y., Chen, Y., Fan, J., and Leung, L.-Y. R.: Application of an online-coupled regional climate model, WRF-CAM5, over East Asia for examination of ice nucleation schemes: part II. Sensitivity to heterogeneous ice nucleation parameterizations and dust emissions, Climate, 3, 753–774, https://doi.org/10.3390/cli3030753, 2015a.
Zhang, Y., Zhang, X., Wang, K., He, J., Leung, L. R., Fan, J., and Nenes, A.: Incorporating an advanced aerosol activation parameterization into WRF-CAM5: Model evaluation and parameterization intercomparison, J. Geophys. Res.-Atmos., 120, 6952–6979, https://doi.org/10.1002/2014JD023051, 2015b.
Zhang, Y., Fan, S., Li, H., and Kang, B.: Effects of aerosol radiative feedback during a severe smog process over eastern China, Acta Meteorol., 74, 465–478, https://doi.org/10.11676/qxxb2016.028, 2016a.
Zhang, Y., He, J., Zhu, S., and Gantt, B.: Sensitivity of simulated chemical concentrations and aerosol-meteorology interactions to aerosol treatments and biogenic organic emissions in WRF/Chem, J. Geophys. Res.-Atmos., 121, 6014–6048, https://doi.org/10.1002/2016JD024882, 2016b.
Zhang, Y., Zhang, X., Wang, K., Zhang, Q., Duan, F., and He, K.: Application of WRF/Chem over East Asia: Part II. Model improvement and sensitivity simulations, Atmos. Environ., 124, 301–320, https://doi.org/10.1016/j.atmosenv.2015.07.023, 2016c.
Zhang, Y., Zhang, X., Wang, L., Zhang, Q., Duan, F., and He, K.: Application of WRF/Chem over East Asia: Part I. Model evaluation and intercomparison with MM5/CMAQ, Atmos. Environ., 124, 285–300, https://doi.org/10.1016/j.atmosenv.2015.07.022., 2016d.
Zhang, Y., Wang, K., and He, J.: Multi-year application of WRF-CAM5 over East Asia-Part II: Interannual variability, trend analysis, and aerosol indirect effects, Atmos. Environ., 165, 222–239, https://doi.org/10.1016/j.atmosenv.2017.06.029, 2017.
Zhao, B., Liou, K., Gu, Y., Li, Q., Jiang, J. H., Su, H., He, C., Tseng, H.-L. R., Wang, S., and Liu, R.: Enhanced PM2.5 pollution in China due to aerosol-cloud interactions, Sci. Rep.-UK, 7, 1–11, https://doi.org/10.1038/s41598-017-04096-8, 2017.
Zhao, B., Wang, Y., Gu, Y., Liou, K.-N., Jiang, J. H., Fan, J., Liu, X., Huang, L., and Yung, Y. L.: Ice nucleation by aerosols from anthropogenic pollution, Nat. Geosci., 12, 602–607, https://doi.org/10.1038/s41561-019-0389-4, 2019.
Zhong, M., Saikawa, E., Liu, Y., Naik, V., Horowitz, L. W., Takigawa, M., Zhao, Y., Lin, N.-H., and Stone, E. A.: Air quality modeling with WRF-Chem v3.5 in East Asia: sensitivity to emissions and evaluation of simulated air quality, Geosci. Model Dev., 9, 1201–1218, https://doi.org/10.5194/gmd-9-1201-2016, 2016.
Zhong, M., Chen, F., and Saikawa, E.: Sensitivity of projected PM2.5- and O3-related health impacts to model inputs: A case study in mainland China, Environ. Int., 123, 256–264, https://doi.org/10.1016/j.envint.2018.12.002, 2019.
Zhong, S., Qian, Y., Zhao, C., Leung, R., and Yang, X.: A case study of urbanization impact on summer precipitation in the Greater Beijing Metropolitan Area: Urban heat island versus aerosol effects, J. Geophys. Res.-Atmos., 120, 10–903, https://doi.org/10.1002/2015JD023753, 2015.
Zhong, S., Qian, Y., Zhao, C., Leung, R., Wang, H., Yang, B., Fan, J., Yan, H., Yang, X.-Q., and Liu, D.: Urbanization-induced urban heat island and aerosol effects on climate extremes in the Yangtze River Delta region of China, Atmos. Chem. Phys., 17, 5439–5457, https://doi.org/10.5194/acp-17-5439-2017, 2017.
Zhou, C., Gong, S., Zhang, X., Liu, H., Xue, M., Cao, G., An, X., Che, H., Zhang, Y., and Niu, T.: Towards the improvements of simulating the chemical and optical properties of Chinese aerosols using an online coupled model-CUACE/Aero, Tellus B, 64, 18965, https://doi.org/10.3402/tellusb.v64i0.18965, 2012.
Zhou, C., Zhang, X., Gong, S., Wang, Y., and Xue, M.: Improving aerosol interaction with clouds and precipitation in a regional chemical weather modeling system, Atmos. Chem. Phys., 16, 145–160, https://doi.org/10.5194/acp-16-145-2016, 2016.
Zhou, C. H., Gong, S. L., Zhang, X. Y., Wang, Y. Q., Niu, T., Liu, H. L., Zhao, T. L., Yang, Y. Q., and Hou, Q.: Development and evaluation of an operational SDS forecasting system for East Asia: CUACE/Dust, Atmos. Chem. Phys., 8, 787–798, https://doi.org/10.5194/acp-8-787-2008, 2008.
Zhou, D., Ding, K., Huang, X., Liu, L., Liu, Q., Xu, Z., Jiang, F., Fu, C., and Ding, A.: Transport, mixing and feedback of dust, biomass burning and anthropogenic pollutants in eastern Asia: a case study, Atmos. Chem. Phys., 18, 16345–16361, https://doi.org/10.5194/acp-18-16345-2018, 2018.
Zhou, M., Zhang, L., Chen, D., Gu, Y., Fu, T.-M., Gao, M., Zhao, Y., Lu, X., and Zhao, B.: The impact of aerosol-radiation interactions on the effectiveness of emission control measures, Environ. Res. Lett., 14, 24002, https://doi.org/10.1088/1748-9326/aaf27d, 2019.
Zhou, Y., Gong, S., Zhou, C., Zhang, L., He, J., Wang, Y., Ji, D., Feng, J., Mo, J., and Ke, H.: A new parameterization of uptake coefficients for heterogeneous reactions on multi-component atmospheric aerosols, Sci. Total Environ., 781, 146372, https://doi.org/10.1016/j.scitotenv.2021.146372, 2021.
Zhuang, B., Jiang, F., Wang, T., Li, S., and Zhu, B.: Investigation on the direct radiative effect of fossil fuel black-carbon aerosol over China, Theor. Appl. Climatol., 104, 301–312, https://doi.org/10.1007/s00704-010-0341-4, 2011.
- Abstract
- Introduction
- Methodology
- Basic overview
- Overview of research focuses in Asia
- Effects of aerosol feedbacks on model performance
- Impacts of aerosol feedbacks in Asia
- Conclusions
- Appendix A
- Appendix B
- Appendix C
- Data availability
- Author contributions
- Competing interests
- Disclaimer
- Acknowledgements
- Financial support
- Review statement
- References
- Supplement
- Abstract
- Introduction
- Methodology
- Basic overview
- Overview of research focuses in Asia
- Effects of aerosol feedbacks on model performance
- Impacts of aerosol feedbacks in Asia
- Conclusions
- Appendix A
- Appendix B
- Appendix C
- Data availability
- Author contributions
- Competing interests
- Disclaimer
- Acknowledgements
- Financial support
- Review statement
- References
- Supplement