the Creative Commons Attribution 4.0 License.
the Creative Commons Attribution 4.0 License.
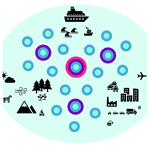
Opinion: Insights into updating Ambient Air Quality Directive 2008/50/EC
Hilkka Timonen
Jarkko V. Niemi
Hanna E. Manninen
Topi Rönkkö
Tareq Hussein
Pak Lun Fung
Sasu Tarkoma
Mikko Laakso
Erkka Saukko
Aino Ovaska
Markku Kulmala
Ari Karppinen
Lasse Johansson
Tuukka Petäjä
As evidence of adverse health effects due to air pollution continues to increase, the World Health Organization (WHO) recently published its latest edition of the global air quality guidelines (World Health Organization, 2021). Although not legally binding, the guidelines aim to provide a framework in which policymakers can combat air pollution by formulating evidence-based air quality management strategies. In the light of this, the European Union has stated its intent to revise the current ambient air quality directive (2008/50/EC) to more closely resemble the newly published WHO guidelines (European Commission, 2020). This article provides an informed opinion on selected features of the air quality directive that we believe would benefit from a reassessment. The selected features include discussion about (1) air quality sensors as a part of a hierarchical observation network, (2) the number of minimum sampling points and their siting criteria, and (3) new target air pollution parameters for future consideration.
- Article
(728 KB) - Full-text XML
- BibTeX
- EndNote
Air pollution continues to be one of the top-ranking mortality risk factors in the global burden on disease analysis, with different estimates linking air pollution to 3.3 million to 9 million premature deaths globally (Burnett et al., 2018; Lelieveld et al., 2015; World Health Organization, 2021). Air pollution consists of both gas- and particle-phase components that originate from a variety of different anthropogenic and natural sources. Typical anthropogenic sources are related to combustion processes, such as vehicular exhaust emissions and residential wood burning, whereas natural sources include, for instance, wildfires and volcano eruptions (e.g., Aguilera et al., 2021; Rönkkö et al., 2017). From a health effects point of view, particulate matter (PM2.5 and PM10) is the most detrimental to human health, although trace gases such as NO2 and O3 also have a contribution (European Environment Agency, 2020). Besides regulated mass-based PM2.5 and PM10 parameters, typically evaluated nonregulated particle metrics also include particle number and surface-area-based concentrations, particle size distributions, and black carbon or elemental carbon (BC or EC, respectively) (e.g., de Jesus et al., 2019; Wu and Boor, 2020). Exposure to air pollution occurs both indoors and outdoors, although people spend most of their time indoors (World Health Organization, 2006).
To combat poor outdoor air quality, the World Health Organization (WHO) has produced a series of guidelines to support policymakers in setting air quality management strategies. Although these guidelines are neither standards nor legally binding criteria, they are based on expert evaluation of scientific evidence and are, thus, a valuable source of information. The earliest guidelines were published in 1987, with subsequent revisions being presented in 2000 and 2006. The newest edition, which was published in 2021, represents the most up-to-date understanding of air pollution and its impacts on human lives (World Health Organization, 2021). In light of this, the European Union has stated its intent to revise the current Ambient Air Quality Directive (AAQD) 2008/50/EC (European Council, 2008) to more closely resemble the new WHO guidelines (European Commission, 2020). The purpose of this article is to provide an expert opinion on how the AAQD should be developed, considering the latest technological and scientific advances in air quality monitoring. Two open consultation rounds have taken place during which experts (open from 1 February to 1 March 2021) and the wider community (open from 23 September to 16 December 2021) were welcome to express their views on the AAQD revision. The results of the expert survey can be found at https://ec.europa.eu/environment/air/quality/documents/20210831_SR9 Phase 1 Report_TechAnnex.pdf (last access: 10 February 2022).
In the current AAQD documentation, air quality observations comprise four modes (Table 1): (1) fixed measurements, (2) indicative measurements, (3) modeling, and (4) objective estimation. In the current hierarchy, the uncertainties are shown in descending order from the most stringent and accurate to the least stringent and accurate. The two most accurate modes – fixed and indicative measurements – are based on the actual measurements, whereas the two least accurate modes – modeling and objective estimation – are based on mathematical and expert estimations of the concentrations. The main weakness of the measurement-based observation modes is their cost and laborious nature; establishing and maintaining a measurement station, especially a station classified as a “fixed measurement” facility (see point 1 in Table 1 for more information), requires considerable resources, leading to a scarcity of stations and poor spatial coverage of measurements. The modeling and objective estimation observation modes require far less continuous effort and can essentially cover any spatial domain, but they potentially provide less reliable, mathematically derived data. The hierarchical observation framework, in which different tier-level observation modes can be used in parallel to complement each other's strengths and weaknesses, is well justified; the authority conducting monitoring may use a combination of techniques that best fits its needs and resources.
Table 1Current Ambient Air Quality Directive (AAQD) observation modes and their measurement uncertainties adapted from the AAQD (European Council, 2008).
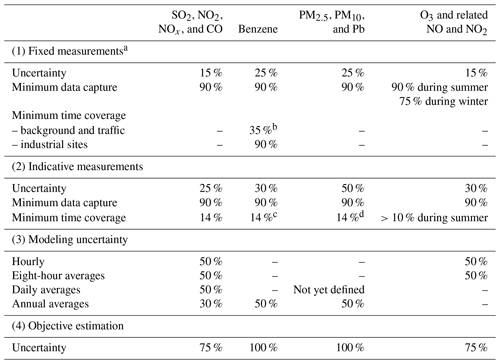
a Member states may apply random measurements instead of continuous measurements for benzene, lead, and particulate matter if they can demonstrate to the commission that the uncertainty, including the uncertainty due to random sampling, meets the quality objective of 25 % and that the time coverage is still larger than the minimum time coverage for indicative measurements. Random sampling must be evenly distributed over the year in order to avoid skewing of results. The uncertainty due to random sampling may be determined by the procedure laid down in ISO 11222:2002 “Air Quality – Determination of the Uncertainty of the Time Average of Air Quality Measurements”. If random measurements are used to assess the requirements of the PM10 limit value, the 90.4 percentile (to be lower than or equal to 50 µg m−3) should be evaluated instead of the number of exceedances, which is highly influenced by data coverage. b Distributed over the year to be representative of various conditions for climate and traffic. c A total of 1 d measurement a week at random, evenly distributed over the year, or 8 weeks evenly distributed over the year. d One measurement a week at random, evenly distributed over the year, or 8 weeks evenly distributed over the year.
Currently, the devices used for measurement-based (fixed or indicative measurements; no modeling or objective estimation) online observations are almost exclusively limited to the most accurate fixed measurement types. This is at least partly due to the long and costly process of acquiring a device “type approval”, which is common practice if regulatory measurements are to be made. Type approval means that the measurement device adheres to the specifications outlined in a standard (e.g., EN 16450 for automated PM measurements) and that the adherence to this standard has been verified by an accredited laboratory (e.g., TÜV Rheinland). Because the type-approval process is the same and the cost is the same for both fixed and indicative measurement devices, there is little incentive for a company to pursue instruments other than those with the more stringent and accurate fixed measurement classification. This is problematic when considering the recent emergence and proven usefulness of air quality sensors (e.g., Petäjä et al., 2021). Although sensors have no formal definition, they are typically perceived as small, standalone devices that are easy to use and easy to deploy within the city infrastructure due to their wireless communication features. Furthermore, their cost is usually a fraction (e.g., < EUR 5000) of a conventional fixed measurement monitor. Air quality sensors and their respective performance have been studied intensively for several years (e.g., Alfano et al., 2020), and they have been used successfully in several voluntary, nonregulatory applications, such as in the wildfire smoke map provided by the US EPA (https://fire.airnow.gov, last access: 10 February 2022). The consensus within the research community is that, while sensors are unlikely to be a direct replacement for the established fixed measurement monitors, their capability to cost-efficiently complement existing monitoring networks as indicative measurement devices is a novel and valuable feature (Peltier, 2020). However, the current formulation and procedure for device type approval does not facilitate extensive integration of sensors into air quality management strategies that aim to fulfill the legal obligations set by the AAQD, and a new testing protocol is needed if this is to be changed. It is likely that the cost of owning and operating sensors would increase as a result of formal performance testing and improved overall quality. Nevertheless, we believe that standardization is the most appropriate way to proceed at this stage, and it remains to be seen how the cost structure of sensors eventually compares to the conventional high-cost instruments.
Although the testing protocol should be less exhaustive in order for companies to apply for device type approval at a lower threshold, it is equally important that no major compromises are being made with respect to the quality criteria of the testing protocol; the sensors should still be able to measure target pollutants with an adequate certainty over a certain (e.g., 1-year) time span (Kaduwela and Wexler, 2021). When considering the type-approval process of PM measurement systems specifically (EN 16450), which is perhaps the most laborious of the classical target pollutants (PM, O3, NO2, SO2, and CO), a straightforward way to ease the burden of testing would be to replace the use of gravimetric reference measurement with a type-approved automated reference monitor. This would eliminate much of the manual work in field tests (e.g., filter weighing) and, thus, reduce cost. Another simple way to ease the burden of testing could be to reduce the minimum amount of 24 h averaged measurement samples (currently 160) required for the equivalency comparison. In the testing protocol, an important point of focus should be the sensor intra-unit precision. Distinguishing sensor imprecision from the true spatial variability of pollutant concentrations is paramount, as one of the most useful applications for sensors is ultradense monitoring networks (Popoola et al., 2018). In practice, if the uncertainty of a measurement from one node to another is too large, identifying and assessing the local concentration hot spots and emission sources will not be possible. When considering dense monitoring networks alone, it could be argued that the absolute sensor accuracy is secondary to its intra-unit precision.
Table 2Minimum number of sampling points per zone or agglomerate as a function of population (European Council, 2008).
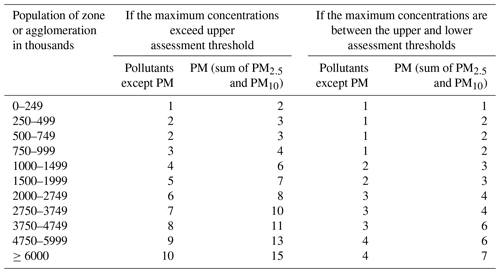
When testing and evaluating sensors, the manufacturer should make it clear if the data generated by a sensor are based on actual measurements of the pollutant itself or if they are based on the combination of, for example, machine learning and secondary data (Li et al., 2020). This is important to ensure transparency. If the sensor relies heavily on data post-processing and advanced conversion methods, the data should be treated as modeling data. In our view, this is because the data then more closely resemble a mathematical prediction rather than an independent measurement. The exact boundary between a measurement and a prediction can be debated, and some empirical corrections may be justifiable and reasonable (Schneider et al., 2019). However, as multiple studies have shown that sensors may entail detrimental flaws (Castell et al., 2017; Giordano et al., 2021), it is very important to distinguish between a real and valid observation and, for example, an AI-based extrapolation of the originally insufficient and inaccurate data.
The authors are aware of the technical specification “CEN/TC 264/WG 42 – Air quality sensors” being under development, and publication of this specification is awaited with interest.
In the European Union, the member states define their zones and agglomerates in which air quality monitoring takes place. Typically, the division between areas follows the administrative unit boarders, although joint efforts, where neighboring units conduct air quality monitoring together, are also possible. In general, the minimum number of fixed sampling points for a specific area is then determined according to the population of that area, as shown in Table 2. As population is the sole factor determining the minimum number of sampling points, this may lead to cases where the number of minimum sampling points between two vastly different zones or agglomerates is strikingly similar. For example, Finnish Lapland – one of the monitoring zones in Finland – covers an area of approximately 100 000 km2 and has 175 000 inhabitants, whereas the Helsinki metropolitan area – also a monitoring zone in Finland – covers an area of 1500 km2 and has 1.2 million inhabitants. According to Table 2, if the maximum concentrations are between the upper and lower assessment thresholds, the Finnish Lapland monitoring zone requires a total of two sampling points (one per 87 500 inhabitants) whereas the Helsinki metropolitan area requires five sampling points (one per 240 000 inhabitants). In our view, the proportions of the required sampling points are imbalanced, and the difference in required sampling points should be greater between the two areas. A fairly similar example to that of Finland can be found in Norway between the Oslo metropolitan agglomerate (five sampling points; one per 206 000 inhabitants) and the Troms and Finnmark zone (two sampling points; one per 117 000 inhabitants). A more sophisticated approach would be to connect the minimum number of sampling points to the population density rather than to just population alone. Population density data are readily available online (https://ghsl.jrc.ec.europa.eu/ghs_pop2019.php, last access: 10 February 2022). Implementation of this connection could be done in a multitude of ways, and it should be ensured that the scaling is appropriate; on average, the number of sampling points should increase rather than decrease. Taking population density into account, the determination of the amount of observation points would more closely track the real exposure that citizens are subjected to; thus, the directive would better fulfill its main purpose of protecting human health.
In the current AAQD, the definitions and instructions regarding the siting of sampling points are sometimes poorly formulated. This has been outlined exceptionally well by Maiheu and Janssen (2019) in their report Assessing the spatial representativeness of AQ sampling points. Some examples of poor formulation include the use of vague terms such as “some meters away” and “edge of major junction”, which can both be interpreted in a multitude of ways. Here, we propose that, rather than trying to define an ultimate set of all-encompassing siting guidelines, it is worth considering the extent to which it would be appropriate to rely on the expertise and judgment of the local air quality authorities. The potential abuse of liberty with respect to artificially improving air quality by situating stations solely in low-concentration areas is a real problem, and some boundary conditions are necessary. Nevertheless, in reality, practicalities such as the availability of power and local regulations have a significant impact on the final site location. As technology evolves, sensors may eventually play a key role in solving this dilemma; however, to date, the clear and well-defined siting criteria and practical challenges related to the deployment of monitors are at odds with one another. With respect to solving the problem of “spatial representativeness” and prioritizing siting criteria, measurement at two different sites instead of only one solves these problems in many cases: there is no need to decide between two equally worthy measurement locations if the amount of sampling points allows both locations to be covered. Fundamentally, the necessity for siting criteria arises from the scarcity of measurements, and the hierarchical monitoring approach is the right tool to address it. This further underlines the need for and utility of dense sensor networks.
The primary motivation of ambient air quality monitoring is the protection of human health. Epidemiological studies have shown that particulate matter is the dominant pollutant causing morbidity and premature deaths (European Environment Agency, 2020); however, to some degree, the explicit mechanisms driving the adverse health effects are still unclear (Fiordelisi et al., 2017). Mass-based parameters, PM2.5 and PM10, have been used as target parameters due to the clear evidence of their association with adverse health effects (Burnett et al., 2018). Ultrafine particles have also been considered a factor, but the current literature suggests that, besides their short-term association with inflammatory and cardiovascular changes, there is insufficient evidence showing that they drive negative health effects independently of other particulate matter constituents (Ohlwein et al., 2019). Exposure to BC also entails negative health effects, and it has been shown to be an important detrimental component of PM2.5 (Yang et al., 2019). Additionally, the climatic effects of BC further underline its importance with respect to air quality monitoring (Bond et al., 2013). Perhaps an often overlooked parameter is pollen, which causes symptoms of irritation in as many as one in four people in Europe each year (Bauchau and Durham, 2004). Pollen originates from plants and, besides reducing exposure to it by personal behavioral changes, it is questionable if mitigation measures could be taken to reduce its concentrations; thus, it is not reasonable to propose limit values for pollen. Nevertheless, out of all of the particulate matter parameters and constituents, the adverse health effects of pollen are the most visible and evident in people's daily lives. Similarly to sensor technology, measurement techniques for pollen have advanced, and monitors capable of online concentration measurement and species identification are currently available. Lastly, the monitoring of ambient aerosol parameters that are included in European emission regulations could significantly improve both the understanding of emission sources and the emission mitigation actions; for instance, regulatory emission measurements made for vehicles in road traffic include the measurement of exhaust solid particle number, which is currently not monitored in traffic-influenced urban air.
Monitoring additional particulate parameters would undoubtedly be useful from a scientific point of view, and the newly published WHO guidelines support this, as the publication recommends the monitoring of ultrafine particles as well as BC and EC (World Health Organization, 2021). However, the monitoring of the additional parameters would require new and extensive standardization with respect to the new parameter-specific instrumentation and expensive investments by monitoring bodies in these new instruments. Therefore, it may be more appropriate to suggest that the scientific community monitors these additional parameters with supporting, research-type “supersite” stations, which remain outside of the regulatory monitoring framework. Naturally, air quality authorities capable of conducting more extensive, voluntary-based monitoring are encouraged to do so, and initiating the preparation for instrument standardization would be useful regardless of the direction in which the AAQD is headed in the future. As illustrated in Fig. 1, the idea of a supersite station is to support and complement existing regulatory monitoring and to provide novel insights into air quality (e.g., Kulmala et al., 2021). The instrumentation could cover measurements for the additional parameters described previously as well as for the more advanced parameters such as the chemical composition of particles. Moreover, due to the extensive measurement arsenal, new observation technologies could be benchmarked and calibrated rapidly. The more in-depth data generated by the supersites would enable scientists and policymakers to assess the needs and directions of future air quality regulation.
Accurate and reliable fixed measurement monitors are, and should remain, the backbone of regulatory air quality monitoring. The technological development of air quality sensors is advancing rapidly, but further development is needed for the sensors to have a major role in regulatory air quality monitoring. The full benefit of the new observational capacities of small sensors can be obtained when a hierarchical observation system is considered (e.g., Hari et al., 2016). This would allow for the verification of the sensor network data quality against the higher-quality observation sites in the vicinity. The hierarchical network would also allow for science-based development and the rapid deployment of novel air quality devices as soon as they become available.
Instead of determining the siting criteria to match all of the possible urban environments, more trust should be placed in local air quality expert analysis; in some cases, applying multiple sensors could be the way forward. Furthermore, the population density should be considered as the basis for determining the required amount of air quality observations. This would lead to an optimized air quality monitoring network providing more representative data in terms of population exposure. At the same time, specific focus should be placed upon constraining the intra-sensor variability to ensure that the true spatial variability of concentrations and sensor imprecision are not mixed with each other.
The monitoring of additional air quality parameters, such as ultrafine particles and BC, would be beneficial in terms of gaining a better understanding of air pollution, and research-type supersite stations operated by the scientific community or, where possible, local air quality authorities could provide the platform for such monitoring. While supporting and complementing a regulatory observation network, the more in-depth data generated by the supersites could help with navigating the future air quality directive requirements.
No data sets were used in this article.
JK, HT, and TP drafted the preliminary version of the article. All authors contributed to the ideation, commenting, writing, and editing of the text. JK finalized the paper.
At least one of the (co-)authors is a member of the editorial board of Atmospheric Chemistry and Physics. The peer-review process was guided by an independent editor, and the authors also have no other competing interests to declare.
Publisher’s note: Copernicus Publications remains neutral with regard to jurisdictional claims in published maps and institutional affiliations.
This research has been supported by the European Union's Horizon 2020 Research and Innovation program (grant agreement no. 101036245) within the framework of the RI-URBANS (Research Infrastructures Services Reinforcing Air Quality Monitoring Capacities in European Urban & Industrial AreaS) project; Business Finland and participating companies via the CITYZER (project nos. 3021/31/2015 and 2883/31/2015) and BC Footprint (project nos. 528/31/2019 and 530/31/2019) projects; the European Regional Development Fund Urban Innovative Actions initiative (HOPE – Healthy Outdoor Premises for Everyone; project no. UIA03-240); the Regional Innovations and Experimentations (AIKO) funds, governed by the Helsinki-Uusimaa Regional Council (project no. HAQT, AIKO014); Academy of Finland Flagship funding (grant nos. 337549, 337551, and 337552); and the Technology Industries of Finland Centennial Foundation Urban Air Quality 2.0 project.
This paper was edited by James Allan and Barbara Ervens and reviewed by two anonymous referees.
Aguilera, R., Corringham, T., Gershunov, A., and Benmarhnia, T.: Wildfire smoke impacts respiratory health more than fine particles from other sources: observational evidence from Southern California, Nat. Commun., 12, 1493, https://doi.org/10.1038/s41467-021-21708-0, 2021.
Alfano, B., Barretta, L., Giudice, A. Del, De Vito, S., Francia, G., Di Esposito, E., Formisano, F., Massera, E., Miglietta, M. L., and Polichetti, T.: A review of low-cost particulate matter sensors from the developers' perspectives, Sensors (Switzerland), 20, 1–56, https://doi.org/10.3390/s20236819, 2020.
Bauchau, V. and Durham, S. R.: Prevalence and rate of diagnosis of allergic rhinitis in Europe, Eur. Respir. J., 24, 758–764, https://doi.org/10.1183/09031936.04.00013904, 2004.
Bond, T. C., Doherty, S. J., Fahey, D. W., Forster, P. M., Berntsen, T., Deangelo, B. J., Flanner, M. G., Ghan, S., Kärcher, B., Koch, D., Kinne, S., Kondo, Y., Quinn, P. K., Sarofim, M. C., Schultz, M. G., Schulz, M., Venkataraman, C., Zhang, H., Zhang, S., Bellouin, N., Guttikunda, S. K., Hopke, P. K., Jacobson, M. Z., Kaiser, J. W., Klimont, Z., Lohmann, U., Schwarz, J. P., Shindell, D., Storelvmo, T., Warren, S. G., and Zender, C. S.: Bounding the role of black carbon in the climate system: A scientific assessment, J. Geophys. Res.-Atmos., 118, 5380–5552, https://doi.org/10.1002/jgrd.50171, 2013.
Burnett, R. T., Chen, H., Szyszkowicz, M., Fann, N., Hubbell, B., Pope, C. A., Apte, J. S., Brauer, M., Cohen, A., Weichenthal, S., Coggins, J., Di, Q., Brunekreef, B., Frostad, J., Lim, S. S., Kan, H., Walker, K. D., Thurston, G. D., Hayes, R. B., Lim, C. C., Turner, M. C., Jerrett, M., Krewski, D., Gapstur, S. M., Diver, W. R., Ostro, B., Goldberg, D., Crouse, D. L., Martin, R. V., Peters, P., Pinault, L., Tjepkema, M., Van Donkelaar, A., Villeneuve, P. J., Miller, A. B., Yin, P., Zhou, M., Wang, L., Janssen, N. A. H., Marra, M., Atkinson, R. W., Tsang, H., Thach, T. Q., Cannon, J. B., Allen, R. T., Hart, J. E., Laden, F., Cesaroni, G., Forastiere, F., Weinmayr, G., Jaensch, A., Nagel, G., Concin, H., and Spadaro, J. V.: Global estimates of mortality associated with longterm exposure to outdoor fine particulate matter, P. Natl. Acad. Sci. USA, 115, 9592–9597, https://doi.org/10.1073/pnas.1803222115, 2018.
Castell, N., Dauge, F. R., Schneider, P., Vogt, M., Lerner, U., Fishbain, B., Broday, D. M., and Bartonova, A.: Can commercial low-cost sensor platforms contribute to air quality monitoring and exposure estimates?, Environ. Int., 99, 293–302, https://doi.org/10.1016/j.envint.2016.12.007, 2017.
de Jesus, A. L., Rahman, M. M., Mazaheri, M., Thompson, H., Knibbs, L. D., Jeong, C., Evans, G., Nei, W., Ding, A., Qiao, L., Li, L., Portin, H., Niemi, J. V., Timonen, H., Luoma, K., Petäjä, T., Kulmala, M., Kowalski, M., Peters, A., Cyrys, J., Ferrero, L., Manigrasso, M., Avino, P., Buonanno, G., Reche, C., Querol, X., Beddows, D., Harrison, R. M., Sowlat, M. H., Sioutas, C., and Morawska, L.: Ultrafine particles and PM2.5 in the air of cities around the world: Are they representative of each other?, Environ. Int., 129, 118–135, https://doi.org/10.1016/j.envint.2019.05.021, 2019.
European Commission: Inception impact assessment, Ref. Ares(2020)7689281, 2020, https://ec.europa.eu/environment/air/documents/Inception impact assessment - Ares(2020)7689281.pdf (last access: 10 February 2022), 2020.
European Council: On Ambient Air Quality and Cleaner Air for Europe 2008/50/EC, Off. J. Eur. Union, 1, 1–44, https://eur-lex.europa.eu/legal-content/EN/TXT/PDF/?uri=CELEX:32008L0050&from=en (last access: 10 February 2022), 2008.
European Environment Agency: Air quality in Europe – 2020 report, https://www.eea.europa.eu//publications/air-quality-in-europe-2020-report (last access: 8 April 2022), 2020.
Fiordelisi, A., Piscitelli, P., Trimarco, B., Coscioni, E., Iaccarino, G., and Sorriento, D.: The mechanisms of air pollution and particulate matter in cardiovascular diseases, Heart Fail. Rev., 22, 337–347, https://doi.org/10.1007/s10741-017-9606-7, 2017.
Giordano, M. R., Malings, C., Pandis, S. N., Presto, A. A., McNeill, V. F., Westervelt, D. M., Beekmann, M., and Subramanian, R.: From low-cost sensors to high-quality data: A summary of challenges and best practices for effectively calibrating low-cost particulate matter mass sensors, J. Aerosol Sci., 158, 105833, https://doi.org/10.1016/j.jaerosci.2021.105833, 2021.
Hari, P., Petäjä, T., Bäck, J., Kerminen, V.-M., Lappalainen, H. K., Vihma, T., Laurila, T., Viisanen, Y., Vesala, T., and Kulmala, M.: Conceptual design of a measurement network of the global change, Atmos. Chem. Phys., 16, 1017–1028, https://doi.org/10.5194/acp-16-1017-2016, 2016.
Kaduwela, A. and Wexler, A.: Use of low-cost air sensors to augment regulatory networks, J. Air Waste Manage. Assoc., 0, 1–2, https://doi.org/10.1080/10962247.2021.1895577, 2021.
Kulmala, M., Dada, L., Daellenbach, K. R., Yan, C., Stolzenburg, D., Kontkanen, J., Ezhova, E., Hakala, S., Tuovinen, S., Kokkonen, T. V., Kurppa, M., Cai, R., Zhou, Y., Yin, R., Baalbaki, R., Chan, T., Chu, B., Deng, C., Fu, Y., Ge, M., He, H., Heikkinen, L., Junninen, H., Liu, Y., Lu, Y., Nie, W., Rusanen, A., Vakkari, V., Wang, Y., Yang, G., Yao, L., Zheng, J., Kujansuu, J., Kangasluoma, J., Petaja, T., Paasonen, P., Jarvi, L., Worsnop, D., Ding, A., Liu, Y., Wang, L., Jiang, J., Bianchi, F., and Kerminen, V. M.: Is reducing new particle formation a plausible solution to mitigate particulate air pollution in Beijing and other Chinese megacities?, Faraday Discuss., 226, 334–347, https://doi.org/10.1039/d0fd00078g, 2021.
Lelieveld, J., Evans, J. S., Fnais, M., Giannadaki, D., and Pozzer, A.: The contribution of outdoor air pollution sources to premature mortality on a global scale, Nature, 525, 367–371, https://doi.org/10.1038/nature15371, 2015.
Li, J., Mattewal, S. K., Patel, S., and Biswas, P.: Evaluation of Nine Low-cost-sensor-based Particulate Matter Monitors, Aerosol Air Qual. Res., 20, 254–270, https://doi.org/10.4209/aaqr.2018.12.0485, 2020.
Maiheu, B. and Janssen, S.: Assessing the spatial representativeness of air quality sampling points – Literature Review, https://ec.europa.eu/environment/air/quality/documents/Report onLiterature Review.PDF (last access: 1 February 2022), 2019.
Ohlwein, S., Kappeler, R., Kutlar Joss, M., Künzli, N., and Hoffmann, B.: Health effects of ultrafine particles: a systematic literature review update of epidemiological evidence, Int. J. Public Health, 64, 547–559, https://doi.org/10.1007/s00038-019-01202-7, 2019.
Peltier, R. E.: An Update on Low-Cost Sensors for the Measurement of Atmospheric Composition, World Meteorological Organization, Geneva, https://library.wmo.int/doc_num.php?explnum_id=10620 (last access: 1 February 2022), 2020.
Petäjä, T., Ovaska, A., Fung, P. L., Poutanen, P., Yli-Ojanperä, J., Suikkola, J., Laakso, M., Mäkelä, T., Niemi, J. V., Keskinen, J., Järvinen, A., Kuula, J., Kurppa, M., Hussein, T., Tarkoma, S., Kulmala, M., Karppinen, A., Manninen, H. E., and Timonen, H.: Added Value of Vaisala AQT530 Sensors as a Part of a Sensor Network for Comprehensive Air Quality Monitoring, Front. Environ. Sci., 9, 1–18, https://doi.org/10.3389/fenvs.2021.719567, 2021.
Popoola, O. A. M., Carruthers, D., Lad, C., Bright, V. B., Mead, M. I., Stettler, M. E. J., Saffell, J. R., and Jones, R. L.: Use of networks of low cost air quality sensors to quantify air quality in urban settings, Atmos. Environ., 194, 58–70, https://doi.org/10.1016/j.atmosenv.2018.09.030, 2018.
Rönkkö, T., Kuuluvainen, H., Karjalainen, P., Keskinen, J., Hillamo, R., Niemi, J. V., Pirjola, L., Timonen, H. J., Saarikoski, S., Saukko, E., Järvinen, A., Silvennoinen, H., Rostedt, A., Olin, M., Yli-Ojanperä, J., Nousiainen, P., Kousa, A., and Dal Maso, M.: Traffic is a major source of atmospheric nanocluster aerosol, P. Natl. Acad. Sci. USA, 114, 7549–7554, https://doi.org/10.1073/pnas.1700830114, 2017.
Schneider, P., Bartonova, A., Castell, N., Dauge, F. R., Gerboles, M., Hagler, G. S. W., Hüglin, C., Jones, R. L., Khan, S., Lewis, A. C., Mijling, B., Müller, M., Penza, M., Spinelle, L., Stacey, B., Vogt, M., Wesseling, J., and Williams, R. W.: Toward a Unified Terminology of Processing Levels for Low-Cost Air-Quality Sensors, Environ. Sci. Technol., 53, 8485–8487, https://doi.org/10.1021/acs.est.9b03950, 2019.
World Health Organization: Air quality guidelines: global update 2005: particulate matter, ozone, nitrogen dioxide, and sulfur dioxide, 484, https://apps.who.int/iris/rest/bitstreams/1344509/retrieve (last access: 8 April 2022), 2006.
World Health Organization: WHO global air quality guidelines. Particulate matter (PM2.5 and PM10), ozone, nitrogen dioxide, sulfur dioxide and carbon monoxide, World Health Organization, Geneva, https://apps.who.int/iris/handle/10665/345329 (last access: 10 February 2022), 2021.
Wu, T. and Boor, B. E.: Urban aerosol size distributions: a global perspective, Atmos. Chem. Phys., 21, 8883–8914, https://doi.org/10.5194/acp-21-8883-2021, 2021.
Yang, Y., Ruan, Z., Wang, X., Yang, Y., Mason, T. G., Lin, H., and Tian, L.: Short-term and long-term exposures to fine particulate matter constituents and health: A systematic review and meta-analysis, Environ. Pollut., 247, 874–882, https://doi.org/10.1016/j.envpol.2018.12.060, 2019.
- Abstract
- Background
- Air quality sensors as a part of a hierarchical observation network
- Determining the minimum amount of sampling points
- Siting criteria of sampling points
- New target parameters for future consideration
- Summary and outlook
- Data availability
- Author contributions
- Competing interests
- Disclaimer
- Financial support
- Review statement
- References
- Abstract
- Background
- Air quality sensors as a part of a hierarchical observation network
- Determining the minimum amount of sampling points
- Siting criteria of sampling points
- New target parameters for future consideration
- Summary and outlook
- Data availability
- Author contributions
- Competing interests
- Disclaimer
- Financial support
- Review statement
- References