the Creative Commons Attribution 4.0 License.
the Creative Commons Attribution 4.0 License.
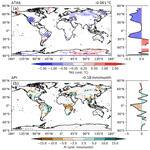
Fire–climate interactions through the aerosol radiative effect in a global chemistry–climate–vegetation model
Chenguang Tian
Jun Zhu
Hong Liao
Yang Yang
Yadong Lei
Xinyi Zhou
Yimian Ma
Yang Cao
Fire emissions influence radiation, climate, and ecosystems through aerosol radiative effects. These can drive rapid atmospheric and land surface adjustments which feed back to affect fire emissions. However, the magnitude of such feedback remains unclear on the global scale. Here, we quantify the impacts of fire aerosols on radiative forcing and the fast atmospheric response through direct, indirect, and albedo effects based on the two-way simulations using a well-established chemistry–climate–vegetation model. Globally, fire emissions cause a reduction of 0.565 ± 0.166 W m−2 in net radiation at the top of the atmosphere with dominant contributions by the aerosol indirect effect (AIE). Consequently, terrestrial surface air temperature decreases by 0.061 ± 0.165 ∘C with coolings of >0.25 ∘C over the eastern Amazon, the western US, and boreal Asia. Both the aerosol direct effect (ADE) and AIE contribute to such cooling, while the aerosol albedo effect (AAE) exerts an offset warming, especially at high latitudes. Land precipitation decreases by 0.180 ± 0.966 mm per month (1.78 % ± 9.56 %) mainly due to the inhibition in central Africa by AIE. Such a rainfall deficit further reduces regional leaf area index (LAI) and lightning ignitions, leading to changes in fire emissions. Globally, fire emissions reduce by 2 %–3 % because of the fire-induced fast responses in humidity, lightning, and LAI. The fire aerosol radiative effects may cause larger perturbations to climate systems with likely more fires under global warming.
- Article
(7066 KB) - Full-text XML
-
Supplement
(2179 KB) - BibTeX
- EndNote
Fire occurs all year round in both hemispheres, burning about 1 % of the earth's surface and emitting roughly 2–3 Pg (1015 g) carbon into the atmosphere every year (van der Werf et al., 2017). Fire activities are strongly influenced by fuel availability, ignition/suppression, and climate conditions (Flannigan et al., 2009). The fuel type, continuity, and amount affect fire occurrence and spread probability (Flannigan et al., 2013). Lightning discharge is the most important natural source of fire ignition (Macias Fauria and Johnson, 2006). Human activities affect fire patterns by adding ignition sources or by suppressing processes (Andela et al., 2017). Compared to the above factors, climate shows a more dominant role in modulating fire activities through the changes in fuel moisture and spread conditions (Flannigan and Harrington, 1988).
Fire exerts prominent impacts on earth systems and human society through various processes. Biomass burning emits a large amount of trace gases and aerosol particles into the troposphere, affecting air quality at the local and downwind regions (Yue and Unger, 2018). In situ observations showed that about one-third of the background particles in the free troposphere of North America originated from biomass burning (Hudson et al., 2004). Extremely intense fires can even inject aerosols into the stratosphere, where the particles were transported globally (Yu et al., 2019). Fire-induced air pollution can reduce global terrestrial productivity of unburned forests (Yue and Unger, 2018), leading to weakened carbon uptake by ecosystems. The global transport of fire air pollution also causes large threats to public health by increasing the risks of diseases and mortality (Liu et al., 2015). It is estimated that fire-induced particulate matter causes more than 33 000 deaths globally each year (Chen et al., 2021).
Aerosols from fires can cause substantial impact on climate via the radiative effect owing to their different optical and chemical properties (Xu et al., 2021). The aerosol radiative effect represents the fast atmospheric adjustment or response before changing global mean surface air temperature (TAS). First, aerosols scatter and/or absorb solar radiation through the aerosol direct effect (ADE), leading to an altered energy budget and climate variables (Carslaw et al., 2010). There is no agreement on the sign of ADE of biomass-burning aerosols at the global scale. Some studies (Heald et al., 2014; Veira et al., 2015; Zou et al., 2020) predicted positive forcing, while others (Ward et al., 2012; Jiang et al., 2016; Grandey et al., 2016) yielded negative forcing (−0.2 to 0.2 W m−2), mainly because of the large uncertainties in the absorption of fire-emitted black carbon (BC) (Carslaw et al., 2010; IPCC, 2014). Second, aerosols can serve as cloud condensation nuclei (CCN) or ice nuclei to affect the microphysical properties of clouds. Such an aerosol indirect effect (AIE) further influences the climate system through the changes in cloud albedo and lifetime (Twomey, 1974; Albrecht, 1989). Globally, fire aerosols account for ∼30 % of the total CCN (Andreae et al., 2004), and the overall negative AIE of fire aerosol is stronger than ADE in magnitude (Liu et al., 2014; Ward et al., 2012; Jiang et al., 2016). Third, deposition of fire-emitted BC aerosols reduces surface albedo and promotes ice/snow melting, which is called the aerosol albedo effect (AAE) (Hansen and Nazarenko, 2004; Warren and Wiscombe, 1980). Compared with other two effects, AAE shows more regional characteristics (Kang et al., 2020). These fire-induced disturbances in radiative fluxes further alter meteorological and hydrologic variables, which in turn affect fire activities through the changes in fuel moisture and weather conditions.
The impacts of fire-induced rapid adjustments on fire activity at the global scale have not been fully assessed. While observations revealed fire-induced perturbations to the regional climate (Bali et al., 2017; Zhuravleva et al., 2017), its feedback to fire activities is difficult to be isolated from the influences of background climate. Models provide unique tools to explore fire–climate interactions resulting from the aerosol radiative effect especially at the regional to global scales. However, they are not routinely included in most earth system models. The IPCC (Intergovernmental Panel on Climate Change) Sixth Assessment Report (AR6) did not provide a quantitative assessment of such feedback as well (IPCC, 2021). In this study, we explore the impacts of the fire aerosol radiative effect on climate and the consequent feedbacks to fire emissions by using a well-established fire parameterization coupled to a chemistry–climate–vegetation model, ModelE2-YIBs (Yue and Unger, 2015). The main objectives are (1) to isolate the radiative effects of fire aerosols through ADE, AIE, and AAE processes and (2) to quantify the feedback of fire-induced rapid adjustments to fire emissions.
2.1 Data
We use the emissions from the Global Fire Emission Database version 4.1s (GFED4.1s) to validate the simulated fire emissions. GFED4.1s provides monthly fire emission fluxes of various air pollutants based on satellite retrieval of area burned from the Moderate Resolution Imaging Spectroradiometer (MODIS) (van der Werf et al., 2017). Area burned in GFED4.1s is mainly derived from the MODIS burned-area product (Giglio et al., 2013), taking into account “small” fires outside the burned-area maps based on active fire detections (Randerson et al., 2012). The gridded fire emission dataset has a spatial resolution of and is available for every month from July 1997. To compute anthropogenic ignition and suppression effects (see Sect. 2.3), we use a downscaled population density dataset from Gao (2017, 2020). Monthly sea surface temperature (SST) and sea ice concentration (SIC) obtained from the Hadley Centre Sea Ice and Sea Surface Temperature (HadISST) dataset (Rayner et al., 2003) are used as the boundary conditions for the climate model.
2.2 ModelE2-YIBs model
The chemistry–climate–vegetation model ModelE2-YIBs is used to simulate the two-way coupling between fire aerosols and climate systems. The ModelE2-YIBs is composed of the NASA Goddard Institute for Space Studies (GISS) ModelE2 (Schmidt et al., 2014) model and the Yale Interactive terrestrial Biosphere Model (YIBs) (Yue and Unger, 2015). GISS ModelE2 is a global climate–chemistry model with a horizontal resolution of latitude by longitude and 40 vertical layers extending to the stratosphere (0.1 hPa). The dynamics and physics codes are executed every 30 min, and the radiation code is calculated every 2.5 h.
The gas phase chemistry scheme considers 156 chemical reactions among 51 species, including NOx–HOx–Ox–CO–CH4 chemistry and different species of volatile organic compounds. Aerosol species in ModelE2 include sulfate, nitrate, ammonium, sea salt, dust, BC, and organic carbon (OC), which are interactively calculated and tracked for both mass and number concentrations. Heterogeneous chemistry on dust surfaces and NOx-dependent secondary organic aerosol production from isoprene and terpenes is included in the model (Bauer et al., 2007b; Tsigaridis and Kanakidou, 2007). The thermodynamic gas–aerosol equilibrium module is used to calculate the phase partitioning of the –– ––Na+–Ca2+–Mg2+–K+–H2O system (Metzger et al., 2006; Bauer et al., 2007a). The aerosol microphysical scheme is based on the quadrature method of moments, which incorporates nucleation, gas particle mass transfer, new particle formation, particle emissions, aerosol phase chemistry, condensational growth, and coagulation (Bauer et al., 2008). The residence time of aerosol species varies greatly in space and time due to different removal rates. Turbulent dry deposition is determined by a resistance-in-series scheme, which is closely coupled to the boundary layer scheme and implemented between the surface layer (10 m) and the ground (Koch et al., 2006). The wet deposition consists of several processes including scavenging within and below clouds, evaporation of falling rainout, transportation along convective plumes, and detrainment and evaporation from convective plumes (Koch et al., 2006; Shindell et al., 2006).
In ModelE2, gases can be converted to aerosols through chemical reactions, while aerosols affect photolysis and provide reaction surface for gases. For example, the formation of sulfate aerosols is driven by modeled oxidants (Bell et al., 2005), and the chemical production of nitrate aerosols is dependent on nitric acid and gaseous ammonia (Bauer et al., 2007b). Moreover, the disturbances of aerosols on climate systems via direct, indirect, and albedo effects are considered in ModelE2. Aerosol optical parameters are calculated by the Mie scattering theory using a complex refractive index depending on chemical speciation and particle size. The first AIE is estimated by the prognostic treatment of cloud droplet number concentration, which is a function of species-dependent contact nucleation, auto-conversion, and immersion freezing (Menon et al., 2008, 2010). The AAE of BC is considered by estimating the decline in surface albedo as a function of aerosol concentrations at the top layer of snow or ice (Koch and Hansen, 2005). We note that average BC deposition to snow estimated by measurement-based average scavenging ratios is used as a climatological proxy to the physical process of BC deposition (Hansen and Nazarenko, 2004). The latter involves size-resolved and meteorologically dependent BC deposition fluxes, as would be found in a chemical transport model but is not used here due to computational constraints. More detailed descriptions of ModelE2 can be found in Schmidt et al. (2014). It has been extensively evaluated for meteorological and chemical variables against observations, reanalysis products, and other models and widely used for studies of climate systems, atmospheric components, and their interactions (Schmidt et al., 2014).
YIBs is a process-based vegetation model that dynamically simulates tree growth and terrestrial carbon fluxes with prescribed fractions of nine plant functional types (PFTs), including deciduous broadleaf forest, evergreen needleleaf forest, evergreen broadleaf forest, tundra, shrubland, grassland, and cropland. Essential biological processes such as photosynthesis, phenology, and autotrophic and heterotrophic respiration are considered and parameterized using the state-of-the-art schemes (Yue and Unger, 2015). Dynamic daily leaf area index (LAI) is estimated based on carbon allocation which is updated every 10 d and prognostic phenology which is dependent instantaneously on temperature and drought conditions. Simulated tree height, phenology, gross primary productivity, and LAI agree well with site-level observations and/or satellite retrievals (Yue and Unger, 2015). The YIBs model joined the dynamic global vegetation model inter-comparison project TRENDY and showed reasonable performance of carbon fluxes against available observations (Friedlingstein et al., 2020). In the coupled model, ModelE2 provides meteorological drivers to YIBs, which feeds back to alter land surface water and energy fluxes through changes in stomatal conductance, surface albedo, and LAI. By incorporating YIBs into ModelE2, the new coupled model ModelE2-YIBs can simulate interactions between terrestrial ecosystems and climate systems through the exchange of water and energy fluxes and chemical components (Yue and Unger, 2015; Yue et al., 2017).
2.3 Fire parameterization
We implemented the active global fire parameterization from Pechony and Shindell (2009) into the ModelE2-YIBs model. The parameterization considers key fire-related processes including fuel flammability, lightning and human ignitions, and human suppressions. Flammability is a unitless metric indicating conditions favorable for fire occurrence and is calculated using the vapor pressure deficit (VPD, hPa), precipitation (R, mm d−1), and LAI (m2 m−2) as follows:
where LAI represents vegetation density and is dynamically calculated by YIBs model. CR is a constant set to 2. VPD is a vital indicator of flammability conditions:
where es is the saturation vapor pressure and RH is surface relative humidity. es can be calculated by the Goff–Gratch equation:
where est is 1013.246 hPa and
where a, b, c, d, f, and h are constants set to −7.90298, 5.02808, , 11.344, , and −3.49149, respectively. Ts is the boiling point of water and equal to 373.16 K. VPD and LAI in Eq. (1) are calculated in half-hourly and daily time steps, respectively, while 30 d running average precipitation is employed to avoid unrealistically huge flammability fluctuations. It should be noted that the response of flammability to abovementioned factors may not be instantaneous but may occur over time. For example, a reduction in precipitation in one season at a given location may reduce foliage growth and hence reduce the fuel available for combustion in another season.
The natural and anthropogenic ignition rates determine whether the fire can actually occur. If the ignition rate is zero, the resulting fire emissions will be zero, regardless of flammability. The natural ignition rate IN depends on the cloud-to-ground lightning (CoGL) strike rate, which is simulated by ModelE2 following the parameterization of Price and Rind (1994):
where H is the cloud depth (unit: km).
Humans influence fire activity by adding ignition sources and suppressing fire events, the rates of which increase with population and to some extent counteract each other. The anthropogenic ignition rate IA (number km−2 per month) is calculated as follows (Venevsky et al., 2002):
where PD is population density (number km−2). stands for ignition potentials of human activity, assuming that people in scarcely populated areas interact more with the natural ecosystems and therefore produce more ignition potential. α is the number of potential ignitions per person per month and set to 0.03.
In principle, the successful suppression of fires is dependent on early detection. It is reasonably assumed that fires are detected earlier and suppressed more effectively in highly populated areas. Therefore, the fraction of non-suppressed fires FNS can be expressed as
where c1, c2, and ω are constants and set to 0.05, 0.95, and 0.05, respectively. The selection of constant values in Eq. (7) is done in a heuristic way, due to lack of quantified data globally. It assumes that up to 95 % of fires are suppressed in the densely populated regions but that only 5 % are suppressed in unpopulated areas.
With the calculation of flammability (Flam), ignition (IN and IA), and non-suppression (FNS), the fire count density Nfire (unit: number km−2) at a specific time step can be derived as
Finally, fire emissions of trace gases and particulate matters (FireEmis) are calculated as
where EF is the PFT-specific emission factor of an air pollutant such as BC, OC, NOx, NH3, SO2, CO, alkenes, and paraffin. For each species, simulated gridded emissions are grouped by the dominant PFT and compared to annual total emissions from GFED4.1s over the same grids. The EF is then calibrated to minimize the root-mean-square error between the simulated and GFED data for all land grids. Such calibration adjusts only the global total amount of fire emissions without changing the spatiotemporal pattern predicted by the parameterization. The EF is the intrinsic attribution of wildfire emissions that should not vary greatly with climatic conditions. The fire-emitted minerals or dust-like materials are not implemented in the current model, given that these species are not included in the current version of GFED4.1s.
Compared to fire indexes, such as the Canadian Forest Fire Weather Index system (Wagner, 1987), this fire parameterization shows advantages in integrating the effects of meteorology, vegetation, natural ignition, and human activities (both ignition and suppression) on fires. Furthermore, it is physically straightforward and has been validated based on global observations (Pechony and Shindell, 2009; Hantson et al., 2020). In ModelE2-YIBs, fire emissions are affected by environmental factors following above parameterizations. In turn, the radiative effects of fire-emitted aerosols feed back to affect those climatic and ecological factors. Note that the changes in the environmental factors may result in changes to fire emissions later. We consider only the fire emissions at the surface due to the large uncertainties in depicting fire plume height (Sofiev et al., 2012; Ke et al., 2021). The fire emissions include both primary aerosols and trace gases, the latter of which react with other species to form the secondary aerosols. These particles could be transported across the globe by three-dimensional atmospheric circulation and eventually removed through either dry or wet deposition.
2.4 Simulations
We perform four groups of sensitivity experiments (Table 1) with the ModelE2-YIBs model to quantify the fire–climate interactions through different radiative processes. The first group with the suffix “AD” considers only the ADE. The second (third) group with the suffix “AD_AI” (“AD_AA”) considers both ADE and AIE (ADE and AAE). The fourth group with the suffix “AD_AI_AA” includes all three aerosol radiative effects (ADE, AIE, and AAE). Within each group, two runs are performed with (YF) or without (NF) fire emissions. For YF simulations, fire-induced aerosols including primarily emitted and secondarily formed are dynamically calculated based on fire parameterization (see Sect. 2.3) and atmospheric transport. These fire emissions cause radiative perturbations and the consequent fast atmospheric adjustments, which feed back to influence fire emissions. For NF simulations, fire emissions are calculated offline at each step without perturbing the climate system, which can be considered to mean that there is no fire emission. By comparing the climatic variables from the YF and NF runs in the first group, we isolate the impacts of fire aerosols on climate through ADE. By comparing the climatic effects from the first and second (third) groups, we isolate the AIE (AAE) of fire aerosols. By comparing the climatic variables from YF and NF runs in the fourth group, the overall effect (ADE + AIE + AAE) is obtained. Besides, the differences in fire emissions between simulations of “YF_AD_AI_AA” and “NF_AD_AI_AA” represent the feedback of fire-aerosol-induced environmental perturbations. Note that fire-emitted gas phase species also perturb radiation via atmospheric absorption and/or feedback from rapid adjustment; these perturbations are far less than aerosol forcing and could be ignored.
Table 1Summary of simulations using ModelE2-YIBs.
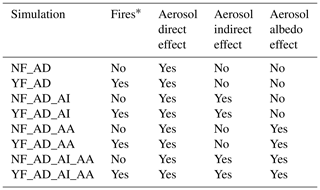
* All simulations predict fire emissions, but the runs with NF do not feed the fire aerosols into the model to perturb radiative fluxes.
For each simulation, climatological mean CO2 concentrations, SST and SIC, and population density during 1995–2005 are used as boundary conditions to drive the model. Such a configuration ignores the year-to-year variability in climate systems, which may cause significant changes in annual fire emissions (Burton et al., 2020). Each simulation is integrated for 25 years with the first 5 years spinning up and the last 20 years averaged. A two-tailed Student's t test is performed to assess 90 % confidence levels of the predicted radiative and climatic responses (p<0.1). The global mean or sum value is depicted in the form of the mean value ± standard deviation. In this study, downward (upward) radiative/heat fluxes are defined as positive (negative). Given that the model is driven by prescribed SST and SIC, only the rapid adjustments of atmospheric variables are taken into account, and we mainly focus on climate changes over the land grid. The radiative effect simulated with such a model configuration is termed the effective radiative forcing (ERF) (IPCC, 2014).
3.1 Model evaluation
Simulated fire emissions of BC and OC show hotspots in the tropics, such as the Amazon, the Sahel, central Africa, and Southeast Asia (Fig. S1 in the Supplement). The large tropical fire emissions are related to abundant vegetation and/or distinct dry seasons. Compared to GFED4.1s data, ModelE2-YIBs slightly underestimates boreal fire emissions especially over northern Asia and North America. On the global scale, fire releases 1.85 ± 0.01 Tg C yr−1 (1 Tg=1012 g) of BC and 16.8 ± 0.92 Tg C yr−1 of OC in ModelE2-YIBs, close to the 1.86 Tg C yr−1 of BC and 16.4 Tg C yr−1 of OC estimated by GFED4.1s. In general, ModelE2-YIBs reasonably captures the spatial distribution of fire emissions, with high spatial correlations of 0.67 (p<0.01) for BC and 0.58 (p<0.01) for OC and low normalized mean biases of 0.6 % for BC and 2.4 % for OC against satellite-based observations.
3.2 Fire-induced radiative perturbations
Figure S2 in the Supplement shows the fire-induced changes in aerosol optical depth (AOD) at 550 nm. Fire emissions largely enhance surface aerosols especially over tropical regions. Hotspots are located in southern Africa and South America with regional enhancement larger than 0.05. In addition, large enhancement is also found at boreal high latitudes (>0.01). At the global scale, fires enhance AOD by 0.006 ± 0.001 with 0.010 ± 0.001 over land.
Fire aerosols cause large perturbations in net radiation at the top of the atmosphere (TOA). Globally, the net radiation at TOA decreases by 0.565 ± 0.166 W m−2 for fire aerosols (Fig. 1a). Regionally, negative changes are predicted over central Africa, western South America, western North America, and the boreal high latitudes. Diagnosis shows that fire-induced AIE dominates the reduction in TOA flux with a global value of −0.440 ± 0.264 W m−2 (Fig. 1c), accounting for 78 % of the total TOA radiative effect by fire aerosols. The spatial correlation coefficient is 0.62 over land grids between the perturbations by all aerosol effects and that by AIE alone. Compared to AIE, the changes in TOA radiative fluxes are much smaller for fire ADE (−0.058 ± 0.213 W m−2, Fig. 1b) and AAE (−0.016 ± 0.283 W m−2, Fig. 1d) with limited perturbations on land.
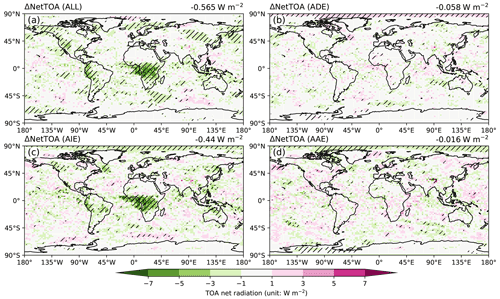
Figure 1Changes in net radiation flux at the top of the atmosphere due to (a) total effects, (b) aerosol direct effect (ADE), (c) aerosol indirect effect (AIE), and (d) aerosol albedo effect (AAE) of fire aerosols. Positive values represent the increase in downward radiation. Global average value is shown at the top of each panel. Slashes denote areas with significant (p<0.1) changes.
Fire aerosols decrease net shortwave radiation reaching the surface up to 9 W m−2 in central Africa and 7 W m−2 in Amazon (Fig. 2a), where biomass-burning emissions are most intense (Fig. S1). Such a pattern is in general consistent with the changes in TOA fluxes (Fig. 1a), leading to an average reduction of −1.227 ± 0.216 W m−2 in the shortwave radiation over global land. The fire-induced ADE alone reduces land surface shortwave radiation by 0.654 ± 0.353 W m−2 with the maximum center in the Amazon (Fig. S3a in the Supplement). As a comparison, the fire-induced AIE causes a smaller reduction of −0.553 ± 0.518 W m−2 with the hotspot in central Africa (Fig. S3c). The net effect of AAE (0.263 ± 0.551 W m−2) by fire aerosols is positive mainly because fire AAE reduces surface albedo and increases shortwave radiation over the Tibetan Plateau and boreal high latitudes (Fig. S3e). However, the magnitude of AAE is much smaller compared to that of ADE and AIE.
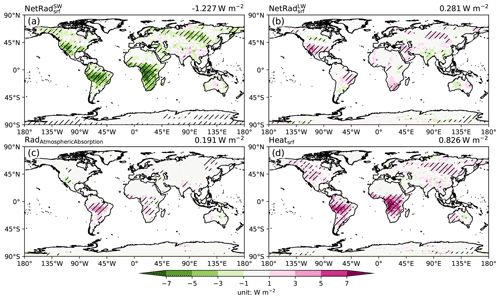
Figure 2Changes in (a) surface (srf) net shortwave (SW) radiation, (b) surface net longwave (LW) radiation, (c) atmospheric absorbed radiation, and (d) surface heat flux (sensible + latent) over land grids caused by fire aerosols. Positive values represent the increase in downward radiation/heat for (a), (b), and (d) and absorption for (c). Global land average value is shown at the top of each panel. Slashes denote areas with significant (p<0.1) changes.
Changes in surface longwave radiation (Fig. 2b) are much smaller than those in shortwave radiation (Fig. 2a). Regionally, positive changes are predicted in the western US, eastern Amazon, and South Africa, where fire-induced surface cooling (Fig. 3a) decreases the upward longwave radiation. On the global scale, fire aerosols cause a decrease of 0.281 ± 0.371 W m−2 in surface upward longwave radiation. As a result, fire aerosols induce a net atmospheric absorption of 0.191 ± 0.227 W m−2 over land grids (Fig. 2c). The reductions in surface shortwave radiation are largely balanced by changes in heat fluxes at the surface, which shows an average decrease of 0.826 ± 0.311 W m−2 in the upward fluxes over land grids (Fig. 2d). Fire ADE and AIE lead to reductions of 0.503 ± 0.289 and 0.432 ± 0.411 W m−2 in surface upward heat fluxes, respectively (Fig. S3b and d). Changes in sensible heat account for 82.2 % of the changes in total heat reduction, much higher than the contributions of 17.8 % by latent heat fluxes (Fig. S4 in the Supplement). Regionally, the upward sensible heat decreases in the western US and Amazon mainly due to fire ADE, while the upward latent heat decreases in central Africa mainly by fire AIE (Fig. S5 in the Supplement).
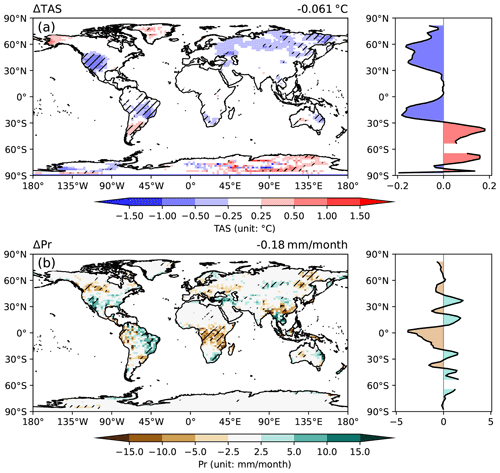
Figure 3Changes in (a) surface air temperature (TAS) and (b) precipitation (Pr) over land grids caused by fire aerosols. The zonal averages of these changes are shown by the side of each panel. Global land average value is shown at the top of each panel. Slashes denote areas with significant (p<0.1) changes.
3.3 Fire-induced fast climatic responses
In response to the perturbations in radiative fluxes, land TAS decreases by 0.061 ± 0.165 ∘C globally for fire aerosols (Fig. 3a). Such cooling is mainly located in the western US, the Amazon, and boreal Asia, following the large reductions in shortwave radiation (Fig. 2a). Meanwhile, moderate warming is predicted at the high latitudes of both hemispheres especially over the areas covered with land ice such as Greenland and Antarctica. Sensitivity experiments show that both ADE (Fig. 4a) and AIE (Fig. 4c) of fire aerosols result in net cooling globally, with regional reductions in TAS over boreal Asia and North America. In contrast, the fire AAE causes increases in TAS over boreal Asia and North America (Fig. 4e), where the deposition of BC aerosols reduces surface albedo. Consequently, the fire AAE results in a global warming of 0.054 ± 0.163 ∘C, which in part offsets the cooling effects by the ADE and AIE of fire aerosols.
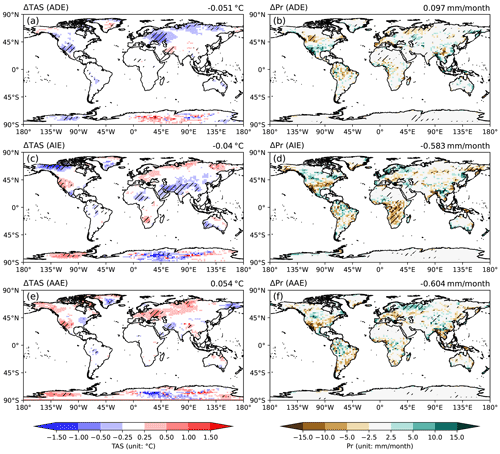
Figure 4Changes in (a, c, e) surface air temperature and (b, d, f) precipitation over land grids due to (a, b) ADE, (c, d) AIE, and (e, f) AAE of fire aerosols. Global land average value is shown at the top of each panel. Slashes denote areas with significant (p<0.1) changes.
Meanwhile, global land precipitation decreases by 0.180 ± 0.966 mm per month (1.78 % ± 9.56 %) with great spatial heterogeneity (Fig. 3b). Decreased precipitation is predicted over central Africa, boreal North America, and eastern Siberia. In contrast, increased rainfall is predicted in the western US, the eastern Amazon, and northern Asia. The reduction in precipitation is mainly contributed by fire AIE, which reduces cloud droplet size and inhibits local rainfall in central Africa (Fig. 4d). Consequently, latent heat fluxes are reduced to compensate the rainfall deficit in central Africa (Fig. S4b).
3.4 Fast response feedback on fire emissions
The fire-aerosol-induced fast response in precipitation, VPD, lightning, and LAI can feed back to affect fire emissions. However, these changes may have contrasting impacts on fire activities. For example, the aerosol-induced reduction in precipitation in central Africa (Fig. 3b) increases local VPD (Fig. 5a) and consequently causes more fire emissions. Meanwhile, such an enhanced drought condition inhibits plant growth and decreases local LAI (Fig. 5c), which has negative impacts on fire emissions by reducing fuel density. Furthermore, the fire AIE inhibits the development of convective clouds, which limits cloud height and the number of cloud-to-ground lightning strikes in central Africa (Fig. 5b), leading to reduced ignition sources and fire emissions.
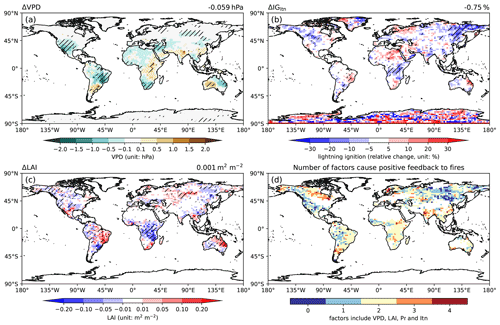
Figure 5Changes in (a) vapor pressure deficit (VPD), (b) lightning (ltn) ignition (IG), and (c) leaf area index (LAI) over land grids induced by fire aerosols. Global land average value is shown at the top of each panel. Slashes denote areas with significant (p<0.1) changes. The number of factors whose changes induced by fire aerosols cause positive feedback to fire emissions is shown in (d). Only grids with fire-emitted OC larger than (colored domain in Fig. S1b) are shown in (d).
To illustrate the joint impacts of fire-aerosol-induced fast climate responses, we count the number out of the four factors contributing positive effects to fire emissions over land grids (Fig. 5d). The larger (smaller) number indicates a higher possibility of increasing (decreasing) fire emissions. Most areas show a neutral number of 2, indicating offsetting effects of the changes in fire-prone factors. Only 13.5 % of land grids show numbers higher than 2 with a sparse distribution. In contrast, 32.1 % of land grids show numbers smaller than 2, especially for the grids over Siberia and the western US where the increased rainfall (Fig. 3b) and decreased VPD (Fig. 5a) inhibit fire emissions. Furthermore, the regional reductions in lightning ignition or LAI promote the inhibition effects. As a result, fire emissions in YF_AD_AI_AA slightly decrease by 31.0 ± 35.9 Gg yr−1 (1.7 %) for BC and 493.6 ± 566.8 Gg yr−1 (2.9 %) for OC compared to NF_AD_AI_AA in which fire emissions do not perturb climate (Fig. 6).
We used the chemistry–climate–vegetation coupled model ModelE2-YIBs to quantify fire–climate interactions through ADE, AIE, and AAE. Globally, fire aerosols decrease TOA net radiation by 0.565 ± 0.166 W m−2, dominated by the AIE over central Africa. Surface net solar radiation also exhibits widespread reductions especially over fire-prone areas with compensations from the decreased sensible and latent heat fluxes. Following the changes in radiation, land TAS decreases by 0.061 ± 0.165 ∘C, and precipitation decreases by 0.180 ± 0.966 mm per month, albeit with regional inconsistencies. The surface cooling is dominated by fire ADE and AIE, while the drought tendency is mainly contributed by fire AIE with hotspots in central Africa. AAE also plays an important role by introducing a warming tendency at the mid-to-high latitudes. These fire-induced fast climatic responses further affect VPD, LAI, and lightning ignitions, leading to reductions in global fire emissions of BC by 2 % and OC by 3 %. It may seem counter-intuitive that reduced precipitation would decrease wildfire emissions, while the observation-based data show that the fire-precipitation correlations are not negative in all regions (Fig. S6 in the Supplement). In this study, the inhibition of precipitation in central Africa (Fig. 3b) reduces regional LAI (Fig. 5c) and decreases fuel availability for fire occurrence, resulting in a positive correlation between fire and precipitation that matches the observed relationship in Africa (Fig. S6). However, in North America, Eurasia, and the Amazon basin, precipitation is anti-correlated with fire emissions. These differences may reflect the seasonal variation in rainfall in the different regions.
Our predicted reduction of 0.565 ± 0.166 W m−2 in TOA radiation by fire aerosols is close to the estimate of −0.51 W m−2 reported by Jiang et al. (2016) and −0.59 W m−2 by Zou et al. (2020) using different models with prescribed SST/SIC and fire-induced ADE, AIE, and AAE (Table 2). Within such change, fire ADE alone makes a moderate contribution of −0.016 ± 0.283 W m−2, falling within the range of −0.2 to 0.2 W m−2 from other studies. The large uncertainty in fire ADE is likely related to the discrepancies in the BC absorption among climate models, which cause varied net effects when offsetting the radiative perturbations of scattering aerosols. As a comparison, fire AIE in our model induces a significant radiative effect of −0.440 ± 0.264 W m−2. However, such a magnitude is much smaller than previous estimates of −0.7 to −1.1 W m−2 using different models (Table 2). We further estimated a limited fire AAE of −0.016 ± 0.283 W m−2, consistent with previous findings showing an insignificant role of AAE by fire aerosols (Ward et al., 2012; Jiang et al., 2016). Our estimates of reductions in TAS and precipitation also fall within the range of previous studies (Table 2).
Table 2Comparison of the simulated fire-induced change in radiative forcing (RF) at TOA and surface climate with previous studies.
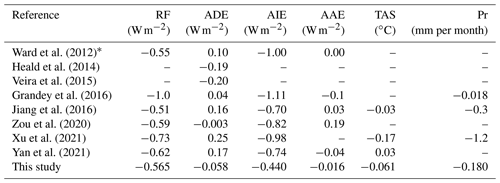
* Other effects of fire-induced change on radiative turbulence are considered in this paper.
Our estimates are subject to some limitations and uncertainties. First, we considered only the fast climatic responses of land surface with prescribed SST and SIC in the simulations. Although most fire-induced AOD changes are located on land (Fig. S2), the air–sea interaction may cause complex climatic responses to aerosol radiative effects. In a recent study, Jiang et al. (2020) emphasized the role of slow feedback contributed by fire aerosols on global precipitation reduction by using a coupled model. Such an air–sea interaction will modify the magnitude and/or spatial pattern of fast climatic responses revealed in this study and should be explored in future studies with coupled ocean models. Second, the nonlinear effects of different radiative processes may influence the attribution results. In this study, we isolate the effects of AIE and AAE by subtracting variables between different groups following the approaches by Bauer and Menon (2012). However, the additive perturbations from individual processes are not equal to the total perturbations with all processes in one simulation. For example, the sum of three processes causes changes in TOA radiation by −0.513 ± 0.324 W m−2 (Fig. 1b–d), surface temperature by −0.037 ± 0.160 ∘C (Fig. 4a, c, and e), and precipitation by −1.090 ± 1.122 mm per month (Fig. 4b, d, and f). These perturbations are weaker than the net effects of 0.565 ± 0.166 W m−2 (Fig. 1a) in radiation and −0.061 ± 0.165 ∘C in temperature (Fig. 3a) but much stronger than that of −0.18 ± 0.96 mm per month in precipitation (Fig. 3b) predicted by the simulation with all three processes. As a result, the nonlinear feedbacks among different radiative processes may magnify or offset the final climatic responses to fire aerosols. Third, considering the complex nature of fire activities, the fire parameterization in this study does not incorporate all fire-related processes (e.g., the influence of wind). In addition, the simulations omit several factors influencing fire emissions (e.g., moist content of fuels) and aerosol radiative effects (e.g., fire plume height). For example, studies show significant impacts of plume rise on the vertical distribution of fire aerosols and the consequent radiative effects (Walter et al., 2016). The impacts of human activity on fire emissions are calculated as a function of population density without considerations of differences in economy, education, and policies. These auxiliary factors may increase the spatial heterogeneity of fire aerosol radiative effects and deserve further explorations in the future studies.
Despite these limitations, we made the first attempt to assess the two-way interaction between fire emissions and climate via aerosol radiative effects. Our results show that fire-emitted aerosols cause negative ERF of 0.565 ± 0.166 W m−2, which is about 20 % of the anthropogenic ERF due to the increased greenhouse gases and aerosols from 1950 to 2019 (IPCC, 2021). Such a fire ERF largely reduces regional TAS and precipitation, leading to further changes in fire emissions. Although the reduction of 2 % to 3 % in fire emissions by the fire–climate interaction through the aerosol radiative effect seems limited, such a change is a result of several complex feedbacks that may exert offsetting effects, and the relative magnitude of individual factors may vary spatially. Both the number of factors and the magnitude of their effects will determine the overall response. Furthermore, our simulations reveal a strong inhibition effect of fire aerosols on LAI in central Africa due to the aerosol-induced drought intensification. Such negative effects on ecosystems are inconsistent with previous estimates that showed certain fertilization effects by fire aerosols (Yue and Unger, 2018), mainly because the rainfall deficit overweighs the diffuse fertilization effects of aerosols. With likely more fires under global warming (Abatzoglou et al., 2019), our results suggested complex and uncertain perturbations by fire emissions to the climate and ecosystem through fire–climate interactions.
The Hadley Centre Sea Ice and Sea Surface Temperature dataset was obtained from https://www.metoffice.gov.uk/hadobs/hadisst/ (Rayner et al., 2003). Population data can be downloaded from https://doi.org/10.7927/q7z9-9r69 (Gao, 2020). GFED data were obtained from https://daac.ornl.gov/VEGETATION/guides/fire_emissions_v4_R1.html (van der Werf et al., 2017). Model data from this study are available from the corresponding author upon request.
The supplement related to this article is available online at: https://doi.org/10.5194/acp-22-12353-2022-supplement.
XY conceived the study. XY and CT designed the research and performed the model runs. CT completed data analysis and the first draft. XY reviewed and edited the manuscript. JZ, HL, YY, and YL advised on concepts and methods. XZ, HZ, YM, and YC helped with data collection. All authors contributed to the discussion of the results and to the finalization of the paper.
The contact author has declared that none of the authors has any competing interests.
Publisher's note: Copernicus Publications remains neutral with regard to jurisdictional claims in published maps and institutional affiliations.
The authors are grateful to Paul A. Makar and another anonymous reviewer for their constructive comments that have improved this study.
This research has been supported by the National Key Research and Development Program of China (grant no. 2019YFA0606802).
This paper was edited by Johannes Quaas and reviewed by Paul A. Makar and one anonymous referee.
Abatzoglou, J. T., Williams, A. P., and Barbero, R.: Global Emergence of Anthropogenic Climate Change in Fire Weather Indices, Geophys. Res. Lett., 46, 326–336, https://doi.org/10.1029/2018gl080959, 2019.
Albrecht, B. A.: Aerosols, Cloud Microphysics, and Fractional Cloudiness, Science, 245, 1227–1230, https://doi.org/10.1126/science.245.4923.1227, 1989.
Andela, N., Morton, D. C., Giglio, L., Chen, Y., van der Werf, G. R., Kasibhatla, P. S., DeFries, R. S., Collatz, G. J., Hantson, S., Kloster, S., Bachelet, D., Forrest, M., Lasslop, G., Li, F., Mangeon, S., Melton, J. R., Yue, C., and Randerson, J. T.: A human-driven decline in global burned area, Science, 356, 1356, https://doi.org/10.1126/science.aal4108, 2017.
Andreae, M. O., Rosenfeld, D., Artaxo, P., Costa, A. A., Frank, G. P., Longo, K. M., and Silva-Dias, M. A. F.: Smoking Rain Clouds over the Amazon, Science, 303, 1337–1342, https://doi.org/10.1126/science.1092779, 2004.
Bali, K., Mishra, A. K., and Singh, S.: Impact of anomalous forest fire on aerosol radiative forcing and snow cover over Himalayan region, Atmos. Environ., 150, 264–275, https://doi.org/10.1016/j.atmosenv.2016.11.061, 2017.
Bauer, S. E. and Menon, S.: Aerosol direct, indirect, semidirect, and surface albedo effects from sector contributions based on the IPCC AR5 emissions for preindustrial and present-day conditions, J. Geophys. Res., 117, D01206, https://doi.org/10.1029/2011JD016816, 2012.
Bauer, S. E., Koch, D., Unger, N., Metzger, S. M., Shindell, D. T., and Streets, D. G.: Nitrate aerosols today and in 2030: a global simulation including aerosols and tropospheric ozone, Atmos. Chem. Phys., 7, 5043–5059, https://doi.org/10.5194/acp-7-5043-2007, 2007a.
Bauer, S. E., Mishchenko, M. I., Lacis, A. A., Zhang, S., Perlwitz, J., and Metzger, S. M.: Do sulfate and nitrate coatings on mineral dust have important effects on radiative properties and climate modeling?, J. Geophys. Res., 112, D06307, https://doi.org/10.1029/2005JD006977, 2007b.
Bauer, S. E., Wright, D. L., Koch, D., Lewis, E. R., McGraw, R., Chang, L.-S., Schwartz, S. E., and Ruedy, R.: MATRIX (Multiconfiguration Aerosol TRacker of mIXing state): an aerosol microphysical module for global atmospheric models, Atmos. Chem. Phys., 8, 6003–6035, https://doi.org/10.5194/acp-8-6003-2008, 2008.
Bell, N., Koch, D., and Shindell, D. T.: Impacts of chemistry-aerosol coupling on tropospheric ozone and sulfate simulations in a general circulation model, J. Geophys. Res., 110, D14305, https://doi.org/10.1029/2004JD005538, 2005.
Burton, C., Betts, R. A., Jones, C. D., Feldpausch, T. R., Cardoso, M., and Anderson, L. O.: El Niño Driven Changes in Global Fire 2015/16, Front. Earth Sci., 8, 199, https://doi.org/10.3389/feart.2020.00199, 2020.
Carslaw, K. S., Boucher, O., Spracklen, D. V., Mann, G. W., Rae, J. G. L., Woodward, S., and Kulmala, M.: A review of natural aerosol interactions and feedbacks within the Earth system, Atmos. Chem. Phys., 10, 1701–1737, https://doi.org/10.5194/acp-10-1701-2010, 2010.
Chen, G., Guo, Y., Yue, X., Tong, S., Gasparrini, A., Bell, M. L., Armstrong, B., Schwartz, J., Jaakkola, J. J. K., Zanobetti, A., Lavigne, E., Nascimento Saldiva, P. H., Kan, H., Royé, D., Milojevic, A., Overcenco, A., Urban, A., Schneider, A., Entezari, A., Vicedo-Cabrera, A. M., Zeka, A., Tobias, A., Nunes, B., Alahmad, B., Forsberg, B., Pan, S.-C., Íñiguez, C., Ameling, C., De la Cruz Valencia, C., Åström, C., Houthuijs, D., Van Dung, D., Samoli, E., Mayvaneh, F., Sera, F., Carrasco-Escobar, G., Lei, Y., Orru, H., Kim, H., Holobaca, I.-H., Kyselý, J., Teixeira, J. P., Madureira, J., Katsouyanni, K., Hurtado-Díaz, M., Maasikmets, M., Ragettli, M. S., Hashizume, M., Stafoggia, M., Pascal, M., Scortichini, M., de Sousa Zanotti Stagliorio Coêlho, M., Valdés Ortega, N., Ryti, N. R. I., Scovronick, N., Matus, P., Goodman, P., Garland, R. M., Abrutzky, R., Garcia, S. O., Rao, S., Fratianni, S., Dang, T. N., Colistro, V., Huber, V., Lee, W., Seposo, X., Honda, Y., Guo, Y. L., Ye, T., Yu, W., Abramson, M. J., Samet, J. M., and Li, S.: Mortality risk attributable to wildfire-related PM2.5 pollution: a global time series study in 749 locations, The Lancet Planetary Health, 5, e579–e587, https://doi.org/10.1016/S2542-5196(21)00200-X, 2021.
Flannigan, M. and Harrington, J. B.: A Study of the Relation of Meteorological Variables to Monthly Provincial Area Burned by Wildfire in Canada (1953–80), J. Appl. Meteorol. Clim., 27, 441–452, https://doi.org/10.1175/1520-0450(1988)027<0441:ASOTRO>2.0.CO;2, 1988.
Flannigan, M., Krawchuk, M. A., de Groot, W. J., Wotton, B. M., and Gowman, L. M.: Implications of changing climate for global wildland fire, Int. J. Wildland Fire, 18, 483–507, https://doi.org/10.1071/WF08187, 2009.
Flannigan, M., Cantin, A. S., de Groot, W. J., Wotton, M., Newbery, A., and Gowman, L. M.: Global wildland fire season severity in the 21st century, Forest Ecol. Manag., 294, 54–61, https://doi.org/10.1016/j.foreco.2012.10.022, 2013.
Friedlingstein, P., O'Sullivan, M., Jones, M. W., Andrew, R. M., Hauck, J., Olsen, A., Peters, G. P., Peters, W., Pongratz, J., Sitch, S., Le Quéré, C., Canadell, J. G., Ciais, P., Jackson, R. B., Alin, S., Aragão, L. E. O. C., Arneth, A., Arora, V., Bates, N. R., Becker, M., Benoit-Cattin, A., Bittig, H. C., Bopp, L., Bultan, S., Chandra, N., Chevallier, F., Chini, L. P., Evans, W., Florentie, L., Forster, P. M., Gasser, T., Gehlen, M., Gilfillan, D., Gkritzalis, T., Gregor, L., Gruber, N., Harris, I., Hartung, K., Haverd, V., Houghton, R. A., Ilyina, T., Jain, A. K., Joetzjer, E., Kadono, K., Kato, E., Kitidis, V., Korsbakken, J. I., Landschützer, P., Lefèvre, N., Lenton, A., Lienert, S., Liu, Z., Lombardozzi, D., Marland, G., Metzl, N., Munro, D. R., Nabel, J. E. M. S., Nakaoka, S.-I., Niwa, Y., O'Brien, K., Ono, T., Palmer, P. I., Pierrot, D., Poulter, B., Resplandy, L., Robertson, E., Rödenbeck, C., Schwinger, J., Séférian, R., Skjelvan, I., Smith, A. J. P., Sutton, A. J., Tanhua, T., Tans, P. P., Tian, H., Tilbrook, B., van der Werf, G., Vuichard, N., Walker, A. P., Wanninkhof, R., Watson, A. J., Willis, D., Wiltshire, A. J., Yuan, W., Yue, X., and Zaehle, S.: Global Carbon Budget 2020, Earth Syst. Sci. Data, 12, 3269–3340, https://doi.org/10.5194/essd-12-3269-2020, 2020.
Gao, J.: Global 1 km Downscaled Population Base Year and Projection Grids Based on the Shared Socioeconomic Pathways, Revision 01, NASA Socioeconomic Data and Applications Center (SEDAC) [data set], https://doi.org/10.7927/q7z9-9r69, 2020.
Giglio, L., Randerson, J. T., and van der Werf, G. R.: Analysis of daily, monthly, and annual burned area using the fourth-generation global fire emissions database (GFED4), J. Geophys. Res.-Biogeo., 118, 317–328, https://doi.org/10.1002/jgrg.20042, 2013.
Grandey, B. S., Lee, H.-H., and Wang, C.: Radiative effects of interannually varying vs. interannually invariant aerosol emissions from fires, Atmos. Chem. Phys., 16, 14495–14513, https://doi.org/10.5194/acp-16-14495-2016, 2016.
Hansen, J. and Nazarenko, L.: Soot climate forcing via snow and ice albedos, P. Natl. Acad. Sci. USA, 101, 423–428, https://doi.org/10.1073/pnas.2237157100, 2004.
Hantson, S., Kelley, D. I., Arneth, A., Harrison, S. P., Archibald, S., Bachelet, D., Forrest, M., Hickler, T., Lasslop, G., Li, F., Mangeon, S., Melton, J. R., Nieradzik, L., Rabin, S. S., Prentice, I. C., Sheehan, T., Sitch, S., Teckentrup, L., Voulgarakis, A., and Yue, C.: Quantitative assessment of fire and vegetation properties in simulations with fire-enabled vegetation models from the Fire Model Intercomparison Project, Geosci. Model Dev., 13, 3299–3318, https://doi.org/10.5194/gmd-13-3299-2020, 2020.
Heald, C. L., Ridley, D. A., Kroll, J. H., Barrett, S. R. H., Cady-Pereira, K. E., Alvarado, M. J., and Holmes, C. D.: Contrasting the direct radiative effect and direct radiative forcing of aerosols, Atmos. Chem. Phys., 14, 5513–5527, https://doi.org/10.5194/acp-14-5513-2014, 2014.
Hudson, P. K., Murphy, D. M., Cziczo, D. J., Thomson, D. S., de Gouw, J. A., Warneke, C., Holloway, J., Jost, H.-J., and Hübler, G.: Biomass-burning particle measurements: Characteristic composition and chemical processing, J. Geophys. Res., 109, D23S27, https://doi.org/10.1029/2003JD004398, 2004.
IPCC: Contribution of Working Groups I, II and III to the Fifth Assessment Report of the Intergovernmental Panel on Climate Change, Geneva, Switzerland, 151 pp., ISBN 978-92-9169-143-2, 2014.
IPCC: Climate Change 2021: The Physical Science Basis. Contribution of Working Group I to the Sixth Assessment Report of the Intergovernmental Panel on Climate Change, Cambridge University Press, 2021.
Jiang, Y., Lu, Z., Liu, X., Qian, Y., Zhang, K., Wang, Y., and Yang, X.-Q.: Impacts of global open-fire aerosols on direct radiative, cloud and surface-albedo effects simulated with CAM5, Atmos. Chem. Phys., 16, 14805–14824, https://doi.org/10.5194/acp-16-14805-2016, 2016.
Jiang, Y., Yang, X.-Q., Liu, X., Qian, Y., Zhang, K., Wang, M., Li, F., Wang, Y., and Lu, Z.: Impacts of Wildfire Aerosols on Global Energy Budget and Climate: The Role of Climate Feedbacks, J. Climate, 33, 3351–3366, https://doi.org/10.1175/JCLI-D-19-0572.1, 2020.
Kang, S., Zhang, Y., Qian, Y., and Wang, H.: A review of black carbon in snow and ice and its impact on the cryosphere, Earth-Sci. Rev., 210, 103346, https://doi.org/10.1016/j.earscirev.2020.103346, 2020.
Ke, Z., Wang, Y., Zou, Y., Song, Y., and Liu, Y.: Global Wildfire Plume-Rise Data Set and Parameterizations for Climate Model Applications, J. Geophys. Res.-Atmos., 126, e2020JD033085, https://doi.org/10.1029/2020JD033085, 2021.
Koch, D. and Hansen, J.: Distant origins of Arctic black carbon: A Goddard Institute for Space Studies ModelE experiment, J. Geophys. Res., 110, D23S27, https://doi.org/10.1029/2004JD005296, 2005.
Koch, D., Schmidt, G. A., and Field, C. V.: Sulfur, sea salt, and radionuclide aerosols in GISS ModelE, J. Geophys. Res., 111, D06206, https://doi.org/10.1029/2004JD005550, 2006.
Liu, J. C., Pereira, G., Uhl, S. A., Bravo, M. A., and Bell, M. L.: A systematic review of the physical health impacts from non-occupational exposure to wildfire smoke, Environ. Res., 136, 120–132, https://doi.org/10.1016/j.envres.2014.10.015, 2015.
Liu, Y., Goodrick, S., and Heilman, W.: Wildland fire emissions, carbon, and climate: Wildfire–climate interactions, Forest Ecol. Manag., 317, 80–96, https://doi.org/10.1016/j.foreco.2013.02.020, 2014.
Macias Fauria, M. and Johnson, E. A.: Large-scale climatic patterns control large lightning fire occurrence in Canada and Alaska forest regions, J. Geophys. Res., 111, G04008, https://doi.org/10.1029/2006JG000181, 2006.
Menon, S., Del Genio, A. D., Kaufman, Y., Bennartz, R., Koch, D., Loeb, N., and Orlikowski, D.: Analyzing signatures of aerosol-cloud interactions from satellite retrievals and the GISS GCM to constrain the aerosol indirect effect, J. Geophys. Res., 113, D14S22, https://doi.org/10.1029/2007JD009442, 2008.
Menon, S., Koch, D., Beig, G., Sahu, S., Fasullo, J., and Orlikowski, D.: Black carbon aerosols and the third polar ice cap, Atmos. Chem. Phys., 10, 4559–4571, https://doi.org/10.5194/acp-10-4559-2010, 2010.
Metzger, S., Mihalopoulos, N., and Lelieveld, J.: Importance of mineral cations and organics in gas-aerosol partitioning of reactive nitrogen compounds: case study based on MINOS results, Atmos. Chem. Phys., 6, 2549–2567, https://doi.org/10.5194/acp-6-2549-2006, 2006.
Pechony, O. and Shindell, D. T.: Fire parameterization on a global scale, J. Geophys. Res., 114, D16115, https://doi.org/10.1029/2009JD011927, 2009.
Price, C. and Rind, D.: Modeling Global Lightning Distributions in a General Circulation Model, Mon. Weather Rev., 122, 1930–1939, https://doi.org/10.1175/1520-0493(1994)122<1930:MGLDIA>2.0.CO;2, 1994.
Randerson, J. T., Chen, Y., van der Werf, G. R., Rogers, B. M., and Morton, D. C.: Global burned area and biomass burning emissions from small fires, J. Geophys. Res.-Biogeo., 117, G04012, https://doi.org/10.1029/2012JG002128, 2012.
Rayner, N. A., Parker, D. E., Horton, E. B., Folland, C. K., Alexander, L. V., Rowell, D. P., Kent, E. C., and Kaplan, A.: Global analyses of sea surface temperature, sea ice, and night marine air temperature since the late nineteenth century, J. Geophys. Res., 108, , 4407, https://doi.org/10.1029/2002JD002670, 2003 (data available at: https://www.metoffice.gov.uk/hadobs/hadisst/, last access: 15 September 2022).
Schmidt, G. A., Kelley, M., Nazarenko, L., Ruedy, R., Russell, G. L., Aleinov, I., Bauer, M., Bauer, S. E., Bhat, M. K., Bleck, R., Canuto, V., Chen, Y.-H., Cheng, Y., Clune, T. L., Del Genio, A., de Fainchtein, R., Faluvegi, G., Hansen, J. E., Healy, R. J., Kiang, N. Y., Koch, D., Lacis, A. A., LeGrande, A. N., Lerner, J., Lo, K. K., Matthews, E. E., Menon, S., Miller, R. L., Oinas, V., Oloso, A. O., Perlwitz, J. P., Puma, M. J., Putman, W. M., Rind, D., Romanou, A., Sato, M., Shindell, D. T., Sun, S., Syed, R. A., Tausnev, N., Tsigaridis, K., Unger, N., Voulgarakis, A., Yao, M.-S., and Zhang, J.: Configuration and assessment of the GISS ModelE2 contributions to the CMIP5 archive, J. Adv. Model. Earth Sy., 6, 141–184, https://doi.org/10.1002/2013MS000265, 2014.
Shindell, D. T., Faluvegi, G., Unger, N., Aguilar, E., Schmidt, G. A., Koch, D. M., Bauer, S. E., and Miller, R. L.: Simulations of preindustrial, present-day, and 2100 conditions in the NASA GISS composition and climate model G-PUCCINI, Atmos. Chem. Phys., 6, 4427–4459, https://doi.org/10.5194/acp-6-4427-2006, 2006.
Sofiev, M., Ermakova, T., and Vankevich, R.: Evaluation of the smoke-injection height from wild-land fires using remote-sensing data, Atmos. Chem. Phys., 12, 1995–2006, https://doi.org/10.5194/acp-12-1995-2012, 2012.
Tsigaridis, K. and Kanakidou, M.: Secondary organic aerosol importance in the future atmosphere, Atmos. Environ., 41, 4682–4692, https://doi.org/10.1016/j.atmosenv.2007.03.045, 2007.
Twomey, S.: Pollution and the planetary albedo, Atmos. Environ. (1967), 8, 1251–1256, https://doi.org/10.1016/0004-6981(74)90004-3, 1974.
van der Werf, G. R., Randerson, J. T., Giglio, L., van Leeuwen, T. T., Chen, Y., Rogers, B. M., Mu, M., van Marle, M. J. E., Morton, D. C., Collatz, G. J., Yokelson, R. J., and Kasibhatla, P. S.: Global fire emissions estimates during 1997–2016, Earth Syst. Sci. Data, 9, 697–720, https://doi.org/10.5194/essd-9-697-2017, 2017 (data available at: https://daac.ornl.gov/VEGETATION/guides/fire_emissions_v4_R1.html, last access: 15 September 2022).
Veira, A., Kloster, S., Schutgens, N. A. J., and Kaiser, J. W.: Fire emission heights in the climate system – Part 2: Impact on transport, black carbon concentrations and radiation, Atmos. Chem. Phys., 15, 7173–7193, https://doi.org/10.5194/acp-15-7173-2015, 2015.
Venevsky, S., Thonicke, K., Sitch, S., and Cramer, W.: Simulating fire regimes in human-dominated ecosystems: Iberian Peninsula case study, Glob. Change Biol., 8, 984–998, https://doi.org/10.1046/j.1365-2486.2002.00528.x, 2002.
Wagner, V.: Development and structure of the Canadian Forest Fire Weather Index System, Forestry Technical Report, Canadian Forestry Service, ISBN 0-662-15198-4, 1987.
Walter, C., Freitas, S. R., Kottmeier, C., Kraut, I., Rieger, D., Vogel, H., and Vogel, B.: The importance of plume rise on the concentrations and atmospheric impacts of biomass burning aerosol, Atmos. Chem. Phys., 16, 9201–9219, https://doi.org/10.5194/acp-16-9201-2016, 2016.
Ward, D. S., Kloster, S., Mahowald, N. M., Rogers, B. M., Randerson, J. T., and Hess, P. G.: The changing radiative forcing of fires: global model estimates for past, present and future, Atmos. Chem. Phys., 12, 10857–10886, https://doi.org/10.5194/acp-12-10857-2012, 2012.
Warren, S. G. and Wiscombe, W. J.: A Model for the Spectral Albedo of Snow. II: Snow Containing Atmospheric Aerosols, J. Atmos. Sci., 37, 2734–2745, 1980.
Xu, L., Zhu, Q., Riley, W. J., Chen, Y., Wang, H., Ma, P.-L., and Randerson, J. T.: The Influence of Fire Aerosols on Surface Climate and Gross Primary Production in the Energy Exascale Earth System Model (E3SM), J. Climate, 34, 7219–7238, https://doi.org/10.1175/JCLI-D-21-0193.1, 2021.
Yan, H., Zhu, Z., Wang, B., Zhang, K., Luo, J., Qian, Y., and Jiang, Y.: Tropical African wildfire aerosols trigger teleconnections over mid-to-high latitudes of Northern Hemisphere in January, Environ. Res. Lett., 16, 034025, https://doi.org/10.1088/1748-9326/abe433, 2021.
Yu, P., Toon, O. B., Bardeen, C. G., Zhu, Y., Rosenlof, K. H., Portmann, R. W., Thornberry, T. D., Gao, R.-S., Davis, S. M., Wolf, E. T., de Gouw, J., Peterson, D. A., Fromm, M. D., and Robock, A.: Black carbon lofts wildfire smoke high into the stratosphere to form a persistent plume, Science, 365, 587–590, https://doi.org/10.1126/science.aax1748, 2019.
Yue, X. and Unger, N.: The Yale Interactive terrestrial Biosphere model version 1.0: description, evaluation and implementation into NASA GISS ModelE2, Geosci. Model Dev., 8, 2399–2417, https://doi.org/10.5194/gmd-8-2399-2015, 2015.
Yue, X. and Unger, N.: Fire air pollution reduces global terrestrial productivity, Nat. Commun., 9, 5413, https://doi.org/10.1038/s41467-018-07921-4, 2018.
Yue, X., Strada, S., Unger, N., and Wang, A.: Future inhibition of ecosystem productivity by increasing wildfire pollution over boreal North America, Atmos. Chem. Phys., 17, 13699–13719, https://doi.org/10.5194/acp-17-13699-2017, 2017.
Zhuravleva, T. B., Kabanov, D. M., Nasrtdinov, I. M., Russkova, T. V., Sakerin, S. M., Smirnov, A., and Holben, B. N.: Radiative characteristics of aerosol during extreme fire event over Siberia in summer 2012, Atmos. Meas. Tech., 10, 179–198, https://doi.org/10.5194/amt-10-179-2017, 2017.
Zou, Y., Wang, Y., Qian, Y., Tian, H., Yang, J., and Alvarado, E.: Using CESM-RESFire to understand climate–fire–ecosystem interactions and the implications for decadal climate variability, Atmos. Chem. Phys., 20, 995–1020, https://doi.org/10.5194/acp-20-995-2020, 2020.