the Creative Commons Attribution 4.0 License.
the Creative Commons Attribution 4.0 License.
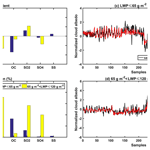
Evaluation of the CMIP6 marine subtropical stratocumulus cloud albedo and its controlling factors
Bida Jian
Jiming Li
Guoyin Wang
Yuxin Zhao
Yarong Li
Jing Wang
Min Zhang
Jianping Huang
The cloud albedo in the marine subtropical stratocumulus regions plays a key role in regulating the regional energy budget. Based on 12 years of monthly data from multiple satellite datasets, the long-term, monthly and seasonal cycle of averaged cloud albedo in five stratocumulus regions were investigated to intercompare the atmosphere-only simulations between phases 5 and 6 of the Coupled Model Intercomparison Project (AMIP5 and AMIP6). Statistical results showed that the long-term regressed cloud albedos were underestimated in most AMIP6 models compared with the satellite-driven cloud albedos, and the AMIP6 models produced a similar spread as AMIP5 over all regions. The monthly averaged values and seasonal cycle of cloud albedo of AMIP6 ensemble mean showed a better correlation with the satellite-driven observations than that of the AMIP5 ensemble mean. However, the AMIP6 model still failed to reproduce the values and amplitude in some regions. By employing the Modern-Era Retrospective Analysis for Research and Applications Version 2 (MERRA-2) data, this study estimated the relative contributions of different aerosols and meteorological factors on the long-term variation of marine stratocumulus cloud albedo under different cloud liquid water path (LWP) conditions. The multiple regression models can explain ∼ 65 % of the changes in the cloud albedo. Under the monthly mean LWP ≤ 65 g m−2, dust and black carbon dominantly contributed to the changes in the cloud albedo, while dust and sulfur dioxide aerosol contributed the most under the condition of 65 g m−2 < LWP ≤ 120 g m−2. These results suggest that the parameterization of cloud–aerosol interactions is crucial for accurately simulating the cloud albedo in climate models.
- Article
(9224 KB) - Full-text XML
-
Supplement
(2642 KB) - BibTeX
- EndNote
One of the critical parameters in regulating the distribution of solar radiation in the atmosphere and surface is cloud albedo, which is the proportion of incoming solar radiation reflected by clouds (Mueller et al., 2011; Wall et al., 2018). A change in the cloud albedo over low-level clouds can cause a significant alteration in the planetary albedo (Engström et al., 2014) and even could offset the warming caused by doubled the amount of carbon dioxide (Latham et al., 2008). Recent studies employing the cloud-system-resolving and plume models have shown that changes in the cloud albedo are largely dependent on aerosol and meteorological conditions (Wang et al., 2011; Stuart et al., 2013; Kravitz et al., 2014). However, there are still non-neglectable uncertainties in simulations (Bender et al., 2016).
This study specifically focused on the cloud albedo in the subtropical marine stratocumulus regions as it is particularly difficult to reproduce the cloud properties by numerical models (Eyring et al., 2016), which results in a larger uncertainty in energy budget simulations and climate predictions (Wood, 2012). The subtropical marine stratocumulus regions are mainly covered by low-level clouds that usually reflect most of the solar radiation and significantly contribute to the planetary albedo (Seethala et al., 2015). In addition, the contribution of the cloud albedo to planetary albedo over these dark oceans could be tremendous compared with those over snow- or ice-covered regions with a high surface albedo (Mueller et al., 2011). However, it is a challenge to accurately estimate the cloud albedo in regions where there are different types of clouds for evaluating the cloud albedo resulting from the relationship between the planetary albedo and cloud fractions at a monthly scale (Bender et al., 2011, 2019).
To date, climate models have continuously advanced in main physical processes, model structures and initial conditions to improve the capability to reproduce numerous observed climate events (Van Weverberg et al., 2018; Huang et al., 2018). Many studies have paid attention to understanding the cloud albedo and its controlling factors over the subtropical marine stratocumulus regions for reducing the uncertainty in models' outputs (Latham et al., 2008; Wood, 2012; Engström et al., 2014; Bender et al., 2019). The cloud albedos obtained from regressing satellite observations in five typical subtropical marine stratocumulus regions exhibited distinct characteristics, ranging from 0.32 to 0.39, and noticeable diurnal variations (Bender et al., 2011; Engström et al., 2014), which may be induced by respective aerosols and meteorological conditions in each region. For example, the southeast Atlantic stratocumulus region (Namibian) is a typical region with massive biomass burning aerosol loading (Wilcox, 2010), while a dominant aerosol loading type in the Canarian region is dust (Waquet et al., 2013). As the value of cloud albedo is usually determined by the cloud optical thickness (COT) and the solar zenith angle (Wood, 2012), the main factors (i.e., the cloud droplet number and sizes) controlling the COT may affect changes in the cloud albedo (George and Wood, 2010; Xie et al., 2013; Bender et al., 2016). Further, the cloud droplet amount and the droplet sizes are affected by cloud condensation nuclei (CCN) and cloud liquid water content (Zhao et al., 2012), and it is crucial to understand the interactions in key dynamical and microphysical processes controlling CCN with regard to improving the model capacity to simulate the cloud albedo (Bender et al., 2016; Rosenfeld et al., 2019).
Regarding the microphysical processes, the aerosol–cloud–radiation interactions over the subtropical marine stratocumulus regions have been actively examined in previous studies (Wang et al., 2011; Bender et al., 2016, 2019; Zhao et al., 2018). Among them, some studies have demonstrated the effect of aerosols on the marine stratocumulus cloud albedo (Twomey effect). In other words, an increase in aerosols can result in smaller droplet sizes and more droplets, leading to a higher cloud albedo (Twomey, 1974, 1977). However, the cloud–aerosol interactions are complex and varying with aerosol types due to their different effects on clouds. Unfortunately, the Intergovernmental Panel on Climate Change currently lacks confidence in estimating the global indirect effects of aerosol (Boucher et al., 2013). Furthermore, the semi-direct effects of absorbing aerosols (e.g., black carbon) are also difficult to be quantified by numerical models (Herbert et al., 2020). Given different model experiments from the Coupled Model Intercomparison Project phase 5 (CMIP5), Frey et al. (2017) estimated the impact of anthropogenic sulfate and non-sulfate aerosol forcing on changing the cloud albedo and concluded that absorbing aerosols play a key role in offsetting the cloud brightening to a certain degree. Regarding the dynamical processes, previous studies found that the dynamical factors (e.g., vertical velocity or instability) can influence not only the vapor supersaturation, leading to the activation of CCN (Twomey, 1959; Lu et al., 2012; Rosenfeld et al., 2019), but also the cloud droplet number and effective radius and cloud optical depth by the entrainment and detrainment of air above the clouds (Fuchs et al., 2018; Yang et al., 2019; Scott et al., 2020). Based on satellite observations, Chen et al. (2014) investigated the effects of aerosols on marine warm clouds, and they found that the response of liquid water path (LWP) to aerosol loading strongly depends on lower-tropospheric stability (LTS) and free-tropospheric moisture. Moreover, the free-troposphere relative humidity is also considered as a critical factor in regulating the cloud albedo because it is closely related to the cloud-top entrainment and drying process that influences the cloud albedo effect (Betts and Ridgway, 1989).
However, most of these studies are based on rapid cloud adjustment to study the effects of specific meteorological factors or aerosol–cloud interactions over the marine subtropical stratocumulus regions. Few systematic studies focus on the effects of meteorological factors and various aerosol types on the cloud albedo and changes at the monthly scale. Furthermore, it is also crucial to evaluate the performance of current climate models to accurately project the cloud albedo responses to climate change. By the intercomparison of outputs between CMIP3 and CMIP5, Engström et al. (2014) found that the regressed regional-averaged cloud albedo and intermodal spread of CMIP5 in the subtropical marine stratocumulus regions are more comparable with the satellite observations compared with those of CMIP3. Given the release of up-to-date CMIP6, as in the previous study, it is necessary to systematically evaluate the performance of CMIP5 and CMIP6 in reproducing the cloud albedo for understanding advances in the skill of climate models to resolve long-standing problems in the marine stratocumulus regions. Based on multiple satellite datasets, this study evaluated the performance of 10 CMIP5/AMIP (Atmospheric Model Intercomparison Project) and 28 CMIP6/AMIP outputs. As an essential part of CMIP experiments, the AMIP outputs forced by observed sea surface temperatures (SSTs) and sea ice concentrations (Eyring et al., 2016) were used in the study. By employing the reanalysis data, this study quantitatively estimated the contributions of each factor to the marine stratocumulus cloud albedo to identify main factors dominating the long-term variations in the marine stratocumulus cloud albedo. This study will provide useful information for comprehensively understanding the impacts of different aerosol types and meteorological factors on cloud albedo changes.
The article is organized as follows. The datasets and methods are given in Sect. 2. The comparison of performances between CMIP5 and CMIP6 is presented in Sect. 3.1. The impacts of different aerosol types and meteorological factors on the cloud albedo are described in Sect. 3.2. Lastly, Sect. 4 addresses the conclusions and discussion.
This study compiled multiple satellite datasets, 10 CMIP5/AMIP outputs, 28 CMIP6/AMIP outputs and reanalysis data not only to evaluate the performance of CMIP5 and CMIP6 outputs but also to investigate the variations in the cloud albedo over the typical subtropical marine stratocumulus regions. Since spatial resolutions vary with climate models, all data were interpolated to a 1.0∘ × 1.0∘ spatial resolution and monthly temporal resolution for fairly evaluating and intercomparing the performance. The following sections provide more details on the satellite datasets, CMIP5, CMIP6 and reanalysis data.
2.1 CERES and MODIS
Estimating the cloud albedo requires multiple atmospheric variables such as the top of atmosphere (TOA) downward and upward (all-sky) shortwave fluxes, cloud liquid water path (LWP), and cloud fractions. In this study, the TOA downward and upward shortwave fluxes were obtained from the Clouds and the Earth's Radiant Energy System (CERES; Wielicki et al., 1996) Single Scanner Footprint (SSF) monthly Ed4A datasets. The LWP and cloud fractions were obtained from the Moderate Resolution Imaging Spectroradiometer (MODIS; Platnick et al., 2003) collection 6.1 level 3 monthly products during the period from 2003 to 2014, i.e., MODIS MYD08_M3 (Aqua) and MOD08_M3 (Terra) products, respectively. The spatial resolutions of these products are 1.0∘ × 1.0∘. The CERES TOA shortwave fluxes were converted from broadband (0.2–5.0 µm) radiances by applying empirical angular distribution models to correct the instrument's incomplete spectral response (Loeb et al., 2001). Then, the real-time fluxes were aggregated to produce 24 h mean fluxes from empirical diurnal albedo models that create meteorology conditions at the overflight time (Loeb et al., 2018). It is worth noting that the aforementioned data processing may introduce some potential uncertainties, e.g., diurnal correction error, radiance-to-flux conversion error (one standard deviation, 1σ) and instrument calibration error (1σ). For example, the uncertainty in the monthly combined regional all-sky shortwave flux was 6.2 W m−2 (CERES_SSF1deg-Hour/Day/Month_Ed4A Data Quality Summary, 2021), in which the calculation of the diurnal correction uncertainty was driven by comparisons with Geostationary Earth Radiation Budget data (Doelling et al., 2013). In addition, the cloud fraction, a fraction of MODIS cloudy pixels to the total pixels at each grid box, is determined based on daytime scenes entirely and represents all cloud phases (Platnick et al., 2003). As the CERES and MODIS instruments are both carried on board Aqua (Equator crossing local time: 11:30) and Terra (Equator crossing local time: 10:30) satellites in polar orbits, we averaged the Aqua and Terra products to obtain the observed combined all-sky albedo, cloud fraction, LWP and cloud albedo as in the works of Engström et al. (2015) and Bender et al. (2017). Time representation errors can be caused by the averaged cloud observations at two time points to represent the daily average. However, recent studies found that the time representation error was significant for short-term studies (up to 14 %) while negligible for long-term statistical analysis (Wang and Zhao, 2017; Zhao et al., 2019a).
2.2 CMIP5/AMIP and CMIP6/AMIP
The 10 CMIP5/AMIP and 28 CMIP6/AMIP outputs include all variables necessary to estimate the cloud albedo, i.e., monthly mean TOA downward and upward (all-sky) fluxes and total cloud fractions (Taylor et al., 2012; Eyring et al., 2016). This study used 10 climate models that provide both CMIP5 and CMIP6 outputs and implemented the intercomparison of performance for the regressed cloud albedo during the period from 2003 to 2008. Furthermore, this study evaluated the cloud albedo for 28 CMIP6/AMIP outputs during the period from 2003 to 2014. Tables 1–2 show the characteristics of CMIP5 and CMIP6 models. Note that there is a considerable discrepancy in the total cloud fractions between the CMIP models and MODIS observations which is caused by different definitions, cloud overlap algorithms and different threshold assumptions for cloud formation (Engström et al., 2015). Moreover, the total cloud fractions in the climate models are usually calculated based on daytime and nighttime cloud fractions, while the observed cloud fractions are only for the daytime. As used in Engström et al. (2015), this study also employed the total cloud fractions as there are no available MODIS simulator outputs for CMIP6. Although uncertainty in cloud fraction remains, a previous study also demonstrated that the time representation error was negligible for long-term statistical analysis (Wang and Zhao, 2017).
2.3 MERRA-2
The study employed the Modern-Era Retrospective analysis for Research and Applications Version 2 (MERRA-2) which provides a long-term aerosol and atmospheric reanalysis record (1980–present) at 0.625∘ × 0.5∘ resolution based on the Goddard Earth Observing System Model, version 5.12.4 (Gelaro et al., 2017). The aerosol reanalysis has been produced by a global data assimilation system that combines satellite- and ground-based observed aerosols with meteorological conditions. Here, the mass mixing ratios of different aerosol types and air density at different levels from the 3-hourly aerosol product (inst3_3d_aer_Nv) and meteorological data from the monthly atmosphere product (instM_3d_asm_Np and instM_2d_asm_Nx) were collected to represent the monthly regional aerosol and meteorological conditions. The outputs of MERRA-2 reanalysis were used during the continuous period from 2003 to 2014 with satellite observation records. As selected in McCoy et al. (2017) and Li et al. (2018), the impacts of different aerosol types on marine stratocumulus cloud albedo were evaluated based on the mass concentrations of hydrophilic black carbon (BC), hydrophilic organic carbon (OC), sulfate aerosol (SO4), sulfur dioxide (SO2), the smallest particles dust (DU; i.e., 0.1–1 µm size) and sea salt (SS; 0.03–0.1 µm size) at the 910 hPa level. The meteorological variables include the monthly vertical velocity at 900 and 700 hPa (omega900 and omega700), surface pressure, relative humidity at 700 hPa (RH700), air temperature, the eastward wind, the northward wind, and surface skin temperature data. In addition, the estimated inversion strength (EIS) and horizontal temperature advection at the surface (SSTadv) factors were also calculated. Finally, all of these meteorological parameters were used to investigate the meteorological effects on the cloud albedo (the units for aerosol mass concentrations, relative humidity, vertical velocity, EIS and SSTadv are kg m−3, %, Pa s−1, K and K s−1, respectively).
2.4 Methods
The planetary albedo (α) can be calculated mainly from the cloud fraction f (Bender et al., 2011) as expressed in Eq. (1):
where αcloud and αclear denote the albedo under cloudy-sky and clear-sky conditions, respectively. For a given region where the cloud and surface type are homogeneous (i.e., constant αcloud and αclear), a change in α should be driven by a change in the cloud fraction f. The cloud albedo can be estimated by the derivative of Eq. (1) as in Eq. (2):
The invariable αcloud and αclear should be applied for the same cloud type and ocean region. In this light, as in the works in Klein and Hartmann (1993), this study also analyzed only five marine stratocumulus regions: Peruvian (10–20∘ S, 80–90∘ W; A1), Namibian (10–20∘ S, 0–10∘ E; A2), Californian (20–30∘ N, 120–130∘ W; A3), Australian (25–35∘ S, 95–105∘ E; A4) and Canarian (15–25∘ N, 25–35∘ W; A5). Previous study (Engström et al., 2014) has also demonstrated that there is a near-linear relationship between cloud cover and planetary albedo in these regions. Figure 1 illustrates the locations of the above stratocumulus regions and the near-global distribution of combined planetary albedo averaged from Aqua and Terra during the continuous period from 2003 to 2014. Here, EIS is defined in Wood and Bretherton (2006):
where the lower-tropospheric stability (LTS) is defined as the difference in potential temperature between 700 hPa and the surface, Γ850m is the moist-adiabatic lapse rate at 850 hPa, and Z700 and ZLCL are the height of the 700 hPa level and the lifting condensation level relative to the surface, respectively. As in Wood and Bretherton (2006), we assumed the surface relative humidity of 80 % to simplify the calculation of surface dew point temperature. ZLCL was calculated based on the method of Georgakakos and Bras (1984). In addition, SSTadv was obtained by Eq. (4) as in Qu et al. (2015):
where u and v represent the eastward and northward horizontal wind components at 1000 hPa, respectively, and ϕ and λ are latitude and longitude, respectively. RE is the mean Earth radius, and SST is the surface skin temperature. A positive/negative SSTadv indicates warm/cold advection. The SSTadv can affect the moisture transport within the cloud layer by influencing the surface sensible and latent heat fluxes and, consequently, influence the thickness of marine stratocumulus clouds (George and Wood, 2010).
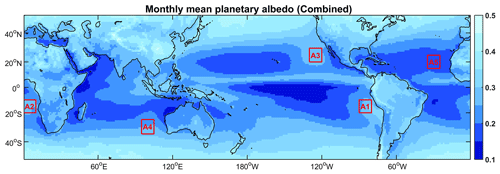
Figure 1Near-global distribution of combined planetary albedo averaged from Aqua and Terra during 2003–2014. Red rectangular boxes indicate the five regions used chosen for the analysis: (A1) Peruvian, (A2) Namibian (A3) Californian, (A4) Australian and (A5) Canarian.
In the study, to avoid influence from a seasonal cycle, the long-term mean analyses are implemented with deseasonalized monthly mean data processed by removing a mean seasonal cycle and then adding the monthly mean value to the interannual anomaly data. The selection of variables is a crucial step to build a multiple linear regression model of the monthly cloud albedo as a function of meteorological factors and aerosol types under two different LWP scenarios (LWP < 65 g m−2 and 65 g m−2 < LWP < 120 g m−2). This study selected suitable variables based on correlation analysis. If the correlation between the cloud albedo and a candidate is significant at a 90 % confidence level, the variable was considered as a predictor factor. Furthermore, the partial least squares were used to reduce the collinearity between the selected variables (McCoy et al., 2017). The regression model of cloud albedo αcloud is as follows:
where a and b are regression coefficients, c is a constant term, Mi represents the ith meteorological predictor, I is the number of meteorological predictor variables, Aj is the jth aerosols predictor, and J is the number of aerosol predictor variables.
The relative contributions of each predictor to the change in the cloud albedo (Huang and Yi, 1991) were evaluated using Eq. (6):
where m is the number of the monthly samples, a is the number of predictors, and Tij is the product of the regression coefficients of each term (bj) and predictor variables (xij).
After removing the effect of meteorological factors, we further investigated the pure relationship between aerosols and the cloud albedo using the partial correlations between αcloud and log 10A, as expressed in Eq. (7):
where rαcloud⋅log10A, rαcloud⋅M and rlog10A⋅M are the total correlation between each variable pair, and rαcloudlog10A⋅M is the correlation between αcloud and log 10A which eliminates the effects of meteorological factors M. More details on the partial correlation are described in Jiang et al. (2018) and Engström and Ekman (2010).
3.1 Satellite observations and CMIP5/6 simulations
The first two columns in Fig. 2 (from panels a to e) show the estimated long-term mean cloud albedo corresponding to the correlation between planetary albedo and cloud fraction over the five regions from the observations and 22 AMIP5 and AMIP6 models, including 10 individual models and an ensemble mean for AMIP5 and AMIP6 (represented by AMIP5-MEM and AMIP6-MEM), during the period from 2003 to 2008. For the combined satellite observations, the correlation coefficient values are above 0.85 in all regions. The correlation over the Peruvian region was the largest (∼ 0.95), while a relatively weak correlation (∼ 0.88) appeared in the Canarian region. Such a high correlation between planetary albedo and cloud fraction further indicates the homogeneity of cloud and surface types over these regions. The regressed cloud albedo from the satellite ranged from 0.30 to 0.42 for the five stratocumulus regions, which is consistent with previous studies (Bender et al., 2011; Engström et al., 2014). As the values averaged over Aqua and Terra albedos and cloud fractions were used as the observations in this study, the regressed cloud albedo values need to be within the range of the Aqua and Terra values (Engström et al., 2014). Regarding the AMIP5 and AMIP6 models, a higher correlation (> 0.8) appeared for most models in the five regions, especially higher in the Australian and Canarian regions. In the Peruvian, Namibian and Californian regions, the correlations of the observations were relatively higher than those of most climate models, while the observed correlation was approximately close to the median value of model simulations in the Australian and Canarian regions.
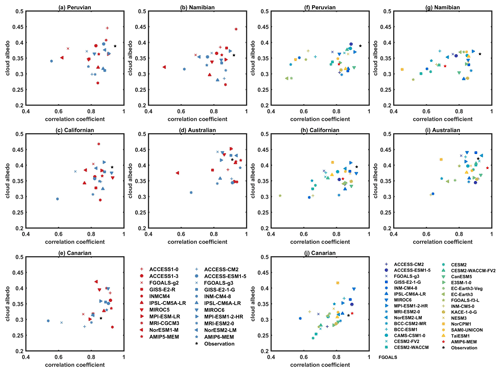
Figure 2The estimated long-term mean cloud albedo and corresponding correlation coefficient from the relation between planetary albedo and cloud fraction (a–e) from satellite observations (black symbol), 11 AMIP5 (red symbols) models and 11 AMIP6 (blue symbols) models during 2003–2008 and (f–j) from satellite observations and 29 AMIP6 models during 2003–2014 over the (a, f) Peruvian, (b, g) Namibian (c, h) Californian, (d, i) Australian and (e, j) Canarian regions.
Although previous studies indicated that some CMIP6 models updated the cloud physical parameterization in the new version (Seland et al., 2020; Kawai et al., 2019), the correlation coefficients of the AMIP6 models between planetary albedo and cloud fraction showed a lower value than those of the AMIP5, indicating that the linear relationship between cloud fraction and planetary albedo in the AMIP6 models' simulations is not superior to that of AMIP5. While the AMIP6 simulations displayed a similar spread in the estimated cloud albedo for all regions, some AMIP6 models produced a lower correlation coefficient than those of the AMIP5 models (e.g., AMIP6/INM-CM4-8). Notably, the AMIP5-MEM and AMIP6-MEM always produced a worse correlation relationship and more irrational cloud albedo values, indicating that the AMIP5 and AMIP6 models have a lack of skill in simulating cloud properties over the marine stratocumulus regions.
The third and fourth columns in Fig. 2 (from panel f to j) also show the estimated long-term mean cloud albedo and the correlation between planetary albedo and cloud fraction over the five regions for the observations and 29 AMIP6 models from 2003 to 2014. The simulated correlation exhibited a larger spread in the Peruvian and Namibian regions than those in other regions, indicating that the AMIP6 models have a lack of capacity to capture the linear relationship between planetary albedo and cloud fraction. The cloud albedos were underestimated in most CMIP6/AMIP models compared with the satellite-based cloud albedos. The Australian (0.30–0.43) and Canarian (0.24–0.42) regions displayed a larger intermodel variability in the cloud albedo than other regions due to a poor skill in simulating the cloud properties (e.g., LWP and COT). Over the Canarian regions, the correlation and cloud albedo of AMIP6-MEM showed good agreement with those of the satellite observations compared with those of the individual AMIP6 models, resulting from the offsetting effect between models. Overall, the AMIP6 models reproduced the cloud albedo and correlation well in the Australian region while having a higher uncertainty in the model's simulations, i.e., a larger intermodal spread, in the Peruvian region (Engström et al., 2014).
Engström et al. (2014) also found that CMIP5 models simulating a higher cloud cover have a tendency to produce a smaller cloud albedo value. Darker clouds can offset the contribution of the higher cloud cover to the planetary albedo, resulting in a relatively consistent model-driven planetary albedo. This is a presentation of the “too few, too bright” problem that persists in general circulation models (GCMs; Nam et al., 2012). To validate whether or not this problem has been improved in the AMIP6 models, we compared the relationship between regressed cloud albedo and cloud fraction (See Fig. S1 in the Supplement). The correlations driven by the 28 AMIP6 models were −0.28, 0.19, −0.11, −0.71 and 0.43 for the Peruvian, Namibian, Californian, Australian and Canarian regions, respectively. Compared with the results from the CMIP5 models (Engström et al., 2014), noticeable progress was found in the Namibian and Californian regions, while a high negative correlation was simulated in the Australian region, indicating that the new generation models need to be further improved to resolve the long-standing problem.
The monthly cloud albedo time series regressed from the satellite, MEM and AMIP6-MEM for the 6-year period from 2003 to 2008 over the five regions are shown in Fig. 3a–e. The temporal correlations (R5/R6) and corresponding confidence value (P5/P6) between simulated (AMIP5-MEM/AMIP6-MEM) and satellite-regressed monthly cloud albedo time series are also given in Fig. 3a–e. Note that the smoothed time series were produced by 12-month smoothing. The statistical results showed that the R5/R6 values were 0.62/0.78, 0.44/0.55, 0.38/0.45, 0.75/0.74 and 0.00/0.05 for the Peruvian, Namibian, Californian, Australian and Canarian regions, respectively. Among them, the correlations only in the Canarian region were insignificant (i.e., P5/P6 = 1.00/0.70). A high positive correlation appeared in the Australian region (> 0.70), indicating that the changes in the cloud albedo are well captured by the models.
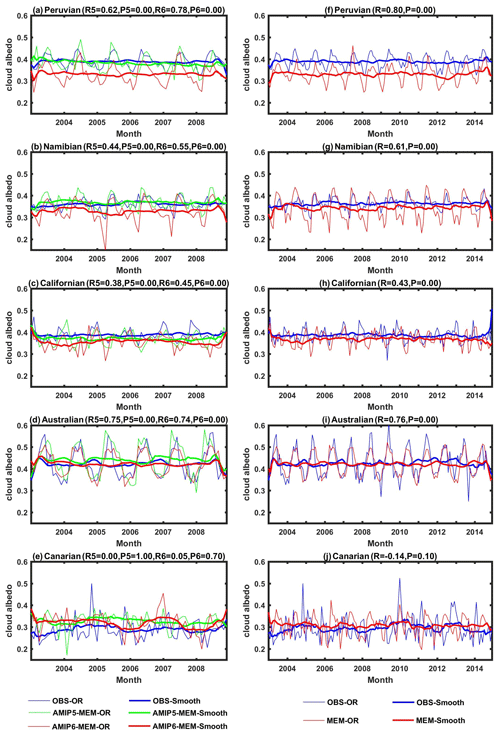
Figure 3Monthly mean time series of estimated cloud albedo (a–e) from AMIP5 and AMIP6 multimodel ensemble mean during 2003–2008 and (f–j) from AMIP6 multimodel ensemble mean during 2003–2014 compared with satellite observations over the (a, f) Peruvian, (b, g) Namibian, (c, h) Californian, (d, i) Australian and (e, j) Canarian regions.
Compared with AMIP5-MEM, the regressed monthly cloud albedo of AMIP6-MEM showed a better correlation with the satellite-regressed values. However, the performance of AMIP5-MEM in reproducing monthly cloud albedo and its amplitude (the difference between the maximum and minimum values of cloud albedo) was better than that of AMIP6-MEM. Furthermore, the monthly cloud albedos obtained from the satellite and models displayed an obvious seasonal cycle in all regions except for the Canarian region. This may be related to the fact that a weaker linear relationship between monthly cloud cover and planetary albedo may exist in the Canarian region, resulting in a significant change in the estimated cloud albedo (see Fig. S2).
In addition, the monthly cloud albedo time series for the satellite and AMIP6-MEM for the period from 2003 to 2014 in the five regions are also shown in Fig. 3f–j, which are consistent with Fig. 3a–e, indicating that the simulation capability of the AMIP6-MEM in different regions does not improve significantly with the expansion of the simulation time and the increase in the model numbers. The amplitudes of the cloud albedo simulated from the model were larger than those of the satellite in the Peruvian, Namibian and Californian regions and smaller in the Australian and Canarian regions. Note that in the Australian region, the monthly cloud albedo exhibited a larger variation than that in other regions based on the satellite-based observations, which means that the cloud optical properties (e.g., COT and cloud effective radius) have been considerably changed within the Australian region.
This study further assessed the performance of the AMIP6 models in reproducing the cloud albedo time series. Figure 4a–e provide the Taylor diagrams (Taylor, 2001) for the five regions, which include the correlation coefficients, the centered root mean square error (RMSE, the green circle), and the standard deviation value between individual AMIP6 models and the satellite-based observations. The centered RMSE and the standard deviation values represent the model's ability to reproduce the phase and amplitude of the variable, respectively. Correlation coefficients greatly varied by region, ranging from negative (Peruvian, Namibian and Canarian) to positive values. Compared with other regions, most of the models showed a high positive correlation (> 0.6) in the Peruvian region. The model-driven cloud albedo was most poorly correlated with the observations in the Canarian region, e.g., < 0.4 or negative values. In contrast, in the Australian region, all models showed a significant positive correlation (> 0.4). The standard deviation values of the models in the Peruvian, Namibian and Californian regions were in the range of 0.02–0.09, 0.02–0.11 and 0.03–0.10, respectively, and 0.03 for the satellite-based observations. This result indicates that most of the models overestimate the amplitude of the cloud albedo time series in the regions. Some models produced the standard deviation values of cloud albedos 3 times larger than the observations. It is evident that the standard deviation values of the simulated cloud albedo in the Australian region were closer to the observed value than that of other regions, indicating that the AMIP6 models also perform well in simulating the amplitude of the monthly cloud albedo time series in this region. Overall, the intermodal variability in the correlation coefficient, RMSE and standard deviation values was the smallest in the Australian region, while the largest was in the Peruvian region.
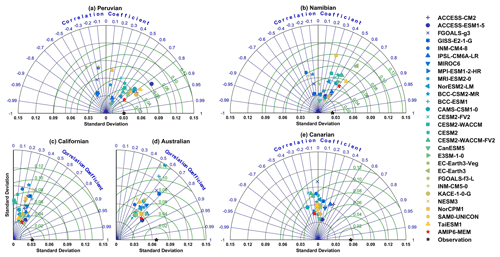
Figure 4Taylor diagrams for monthly estimated cloud albedo between individual AMIP6 models and satellite observations during 2003–2014 over the (a) Peruvian, (b) Namibian, (c) Californian, (d) Australian and (e) Canarian regions. The green circles indicate the centered root mean square error.
Further, Fig. 5 shows the annual cycles of the cloud albedo estimated by the satellite and AMIP5 and AMIP6 models for the five regions. The seasonal variation in the cloud albedo in each region takes a shape of a single peak distribution. In terms of similarity among regions, the cloud albedo in all regions reached the maximum value during the boreal winter season, i.e., December to January in the Northern Hemisphere and June to July in the Southern Hemisphere. Many previous studies have demonstrated that the seasonal variations in marine cloud properties (e.g., cloud fraction, LWP and cloud thickness) are strongly affected by meteorological conditions (Lin et al., 2009; Wood, 2012; Dong et al., 2014). Employing a 19-month record of ground-based lidar and radar observations from the Atmospheric Radiation Measurement Program Azores site, for example, Dong et al. (2014) found that the seasonal variations in cloud thickness and LWP are closely related to the seasonal synoptic patterns (e.g., transport of water vapor, relative humidity, high and low pressure system). Furthermore, the influence of aerosol loading is non-neglectable. While the aerosols act as CCN, the concentration of CCN can significantly influence the cloud albedo of low clouds (Twomey, 1974). On the other hand, absorbing aerosols near stratocumulus may enhance absorbing solar energy, resulting in an influence on the dynamical evolution of stratocumulus causing a change in the cloud albedo (Wilcox, 2010). The seasonal cycle of the cloud albedo in the Australian region showed the largest amplitude among the five regions (ranging from 0.37 to 0.52), while the amplitudes in other regions were less than 0.10. Such a result means that the meteorological conditions and aerosol loadings of the cloud system in the Australian region have a relatively larger seasonal variation compared with those in other regions.
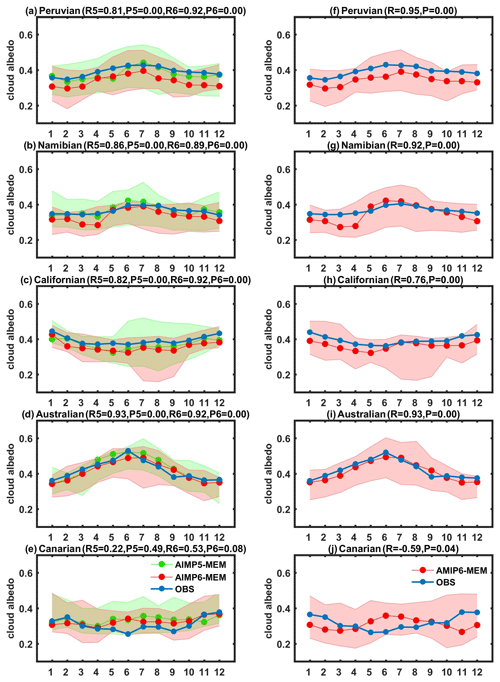
Figure 5Annual cycles of the cloud albedo estimated by (a–e) AMIP5 and AMIP6 multimodel ensemble mean during 2003–2008 and (f–j) AMIP6 multimodel ensemble mean during 2003–2014 compared with satellite observations over the (a, f) Peruvian, (b, g) Namibian, (c, h) Californian, (d, i) Australian and (e, j) Canarian regions. The green and red shaded areas indicate the range of the cloud albedo simulated by AMIP5 and AMIP6 models, respectively. The temporal correlations (R5/R6/R value) and P5/P6/P value (if P5/P6/P < 0.10, indicating the correlation R5/R6/R is significant) for the seasonal cycles of the cloud albedo obtained from satellite-based observations and models are given in parentheses.
The COT usually increases with an increase in cloud LWP, resulting in an increase in the cloud albedo (Wood, 2012). Gryspeerdt et al. (2019) also concluded that LWP is the main factor controlling liquid cloud albedo. Thus, this study investigated the seasonal variation of LWP and found that the change in LWP is strongly correlated with the change in cloud albedo in the Peruvian, Australian and Canarian regions (see Fig. S3). For the Namibian region, however, many studies have shown that the continuous transportation of absorbing biomass burning aerosols from Africa to the region during the African biomass burning season from August to October (Das et al., 2017) can reside above the clouds, resulting in an increase in the cloud albedo by thickening the stratocumulus (Wilcox, 2010, 2012). Zuidema et al. (2018) also found that the biomass burning aerosols generally exist in the boundary layer at the earlier time of the biomass burning seasons and are mainly located above the clouds in September to October, which is caused by the northwestward transportation of the biomass burning aerosols from the African continent. However, Fig. 5b shows that the peak of the cloud albedo occurred in July and then continuously decreased from August to October in the Namibian region, indicating that it is difficult to explain the changes in the cloud albedo by the negative semi-direct effect of the biomass burning aerosols. This result is consistent with the work of Bender et al. (2016) which concluded that the direct effect and positive semi-direct effect are the main aerosol effects (Wilcox, 2012). That is, clouds become darker under a polluted environment. Regarding the seasonal cycles of cloud droplet number concentration, Nd, we found that the seasonal cycles of the cloud albedo in the Namibian region were highly correlated with those of Nd obtained from the Cloud-Aerosol Lidar and Infrared Pathfinder Satellite Observations (CALIPSO) (Li et al., 2018), whereas the seasonal cycles of Nd and the cloud albedo showed opposite seasonal changes to each other in the California region. The relationship between the Nd and the cloud albedo varies with different regions, which may be caused by the effect of meteorological conditions. These results indicate that it is a challenge to study the variability in the cloud albedo over the marine stratocumulus regions under various meteorological and aerosol conditions.
Figure 5a–e show the seasonal cycles of cloud albedo in the five regions during a period from 2003 to 2008 for the AMIP5 and AMIP6 and the satellite-based observations. Shaded areas in Fig. 5 represent the range of the cloud albedo simulated by the 22 models. The R5/R6 and P5/P6 values for the seasonal cycles of the cloud albedo obtained from the models and the satellite-based observations are also given in Fig. 5. For the AMIP5-MEM and AMIP6-MEM, the correlations of the cloud albedo seasonal cycles between the models and the observations are highly positive in all regions (R5/R6 > 0.6), except for the Canarian region (R5/R6 = 0.22/0.53). The R values were the largest in the Namibian (R5/R6 = 0.82/0.92) and Australian regions (R5/R6 = 0.93/0.92). Overall, the results of AMIP6 were slightly superior to those of AMIP5, especially in the Canarian region. However, the seasonal cycles of cloud albedo estimated from the AMIP6-MEM in the Canarian region for 12 years from 2003 to 2014 (Fig. 5j) exhibited a significant negative correlation with that of the satellite-based observations, indicating AMIP6-MEM still has a lack of skill in capturing the seasonal cycle of the cloud albedo in this region even if the numbers of AMIP6 models increases.
3.2 The impacts of different aerosol types and meteorological factors on cloud albedo changes
Cloud liquid water may affect the COT, which is subsequently influencing the cloud albedo (Wood, 2012). Furthermore, the change in LWP also may influence the relationship between aerosols and cloud properties (Roberts et al., 2008; Gryspeerdt et al., 2019; Douglas and L'Ecuyer, 2019). For example, the effect of aerosols on the cloud albedo may be weakened by a change in the LWP (Han et al., 2002; Twohy, 2005). Based on in situ observations, recent studies found that the relationship between aerosol concentration and cloud droplet effective radius changes from negative to positive when liquid water content increases (Qiu et al., 2017; Zhao et al., 2019b). Considering the effect of LWP, this study evaluated the impact of meteorological parameters and aerosol types on the cloud albedo at different LWP ranges in order to evaluate the influence of LWP on cloud albedo. Firstly, the 720 monthly sample data points obtained from the five regions were divided into two groups based on the range of monthly mean LWP values: LWP ≤ 65 g m−2 and 65 g m−2 < LWP ≤ 120 g m−2. Here, the threshold of 65 g m−2 for LWP was chosen to evenly split the samples.
Figure 6a–b show the regression coefficients in the partial correlation calculation and the relative contributions for individual variables related to cloud albedo changes under different LWP conditions. Normalized variables were incorporated into the regression models. There is a considerable discrepancy in the results between the two groups. For the lower LWP bin (i.e., LWP ≤ 65 g m−2), the results showed that the regression coefficient of BC/SO2/SS to the cloud albedo was positive, while DU- and OC-related coefficients were negative, which indicates that the cloud albedo increases with increasing BC/SO2/SS and decreases with increasing DU/OC. Figure 6b also clearly shows that DU, BC and OC make a larger contribution to the change in the cloud albedo compared with other predictors, e.g., omega900, EIS and RH700. Under LWP > 65 g m−2, the contribution of DU to the cloud albedo was the largest. In addition, SO2 and SO4 also considerably contributed to the cloud albedo.
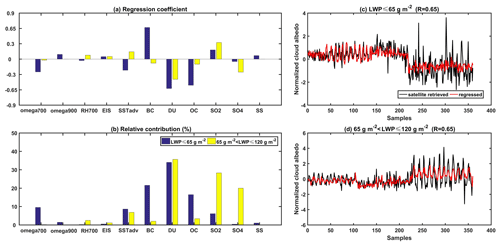
Figure 6The (a) regression coefficients and corresponding (b) relative contribution of each predictor variable related to cloud albedo from the multilinear regression models under two LWP conditions: LWP ≤ 65 g m−2 (blue) and 65 g m−2 < LWP ≤ 120 g m−2 (yellow). Note that for ease of comparison, 11 variables are given in the figure, and variables without values are not predictive variables of the sample group. The satellite- and model-driven normalized cloud albedo trained in two sample groups: (c) LWP ≤ 65 g m−2 and (d) 65 g m−2 < LWP ≤ 120 g m−2. The correlations (R value) between satellite- and model-driven normalized cloud albedo are given in parentheses.
In addition to the effects of LWP, the difference in the relative contribution may be induced by the regional variability in aerosol types. A smaller LWP mainly appeared in the Namibian and Canarian regions where the main aerosol types are DU and BC, while lower BC loadings were found in the regions with a larger LWP (Fig. S4). While the positive coefficient for BC reflects the indirect effect of aerosols on the cloud albedo, the negative dependency of BC may represent the direct and semi-direct effects of absorbing aerosols (Johnson et al., 2004; Bender et al., 2016). For example, Johnson et al. (2004) found that absorbing aerosols in clouds can make the clouds warmer and thinner, resulting in a decrease in cloud albedo. Moreover, McCoy et al. (2018) found a negative dependence of Nd on BC in regions with low BC loadings. This means that a decrease in the cloud albedo may be associated with a decrease in Nd. The dependence of Nd on OC has also been investigated in previous studies (McCoy et al., 2018; Li et al., 2018), and a negative dependence of Nd on OC has been found in some marine regions. The negative sensitivities of OC to the cloud albedo may be attributed to a decrease in Nd with an increase in OC.
Dust is a crucial predictor of the cloud albedo, and the coefficient of DU was negative for the two datasets divided in this study, which may be induced by the semi-direct effects of absorbing aerosols. In the literature, many studies have examined the impacts of dust aerosols on stratocumulus (Doherty and Evan, 2014; Amiri-Farahani et al., 2017). For example, Karydis et al. (2011) showed that aged dust reduces Nd by consuming the supersaturation of clouds. However, McCoy et al. (2017) estimated the indirect effect of aerosol from satellite observations and reanalysis data and found that the dust has a limited impact on Nd in different stratocumulus regions. Pradelle et al. (2002) employing satellite observations also investigated the effect of Saharan dust on marine stratocumulus clouds and found that minimum cloud albedo values appeared in regions with the most dust particles. They also found that the dust in a stratiform cloud may decrease the initial CCN and increase the effective droplet radius, which causes a reduction in the cloud albedo (Pradelle and Cautenet, 2002). In addition, a recent study also showed that the dust aerosol can even further influence the meteorological environment that the clouds form by both suppressing the SST and affecting the temperature and humidity profile (Sun and Zhao, 2020). A significant influence of dust on the cloud albedo in this study may be driven by the collected samples in the five regions where the cloud albedo and dust highly vary with the regions.
Under LWP ≤ 65 g m−2, the coefficient of SS was a small positive value, while the correlation coefficient of sea salt was insignificant under LWP > 65 g m−2, which means that these variables are not suitable as a predictor for estimating the cloud albedo. This is consistent with the results of McCoy et al. (2017, 2018) which indicate that the Nd is weakly dependent on the SS, although sea salt is an effective CCN. McCoy et al. (2018) have also validated the influence of SS on Nd with up-to-date observations. As submicron SS in the MERRA-2 reanalysis data can be simply predicted from wind speed and SST by a parameterization (Jaeglé et al., 2011; McCoy et al., 2018; Li et al., 2018), the effect of SS on the cloud albedo may be dependent on the relationship between the cloud albedo and near-surface wind speed, which may explain the limited effect of SS on the cloud albedo.
The coefficients of SO2 were positive for both datasets. In addition, the Twomey effect for SO2 was further pronounced under the condition with higher LWP. The previous studies (e.g., McCoy et al., 2017; Li et al., 2018) showed that SO4 plays a key role in modulating Nd. Although their results showed significant positive coefficients of SO4 with Nd, this study found an unexpected negative correlation of SO4 with the cloud albedo. Such a result may be driven by the fact that the sulfate aerosol particles and dust are externally mixed. The previous studies showed that sulfate-covered dust can act as CCN, which may induce a decrease in the cloud albedo by enhancing the collision–coalescence progress of droplets (Levin et al., 1996; Rosenfeld et al., 2001).
The results of this study showed a weak dependency of the cloud albedo with omega900, RH700 and EIS. Under LWP ≤ 65 g m−2, the upward vertical velocity and RH700 have an unexpected negative but weak effect on the cloud albedo, and the relative contributions of omega900 and RH700 are negligible. Under LWP > 65 g m−2, no significant correlation between the cloud albedo and omega900 was found. Note that the analysis of this study employed the average data at the monthly scale rather than raw satellite measurements at an instantaneous scale, which may make the cloud albedo less sensitive to omega900. The coefficient of RH700 was positive, and the relative contribution was about 4 %. Generally, drier free-troposphere humidity usually drives stronger entrainment of dry air, which induces evaporating and raising lifted condensation level, resulting in a reduced cloud thickness (Wood, 2012; Eastman and Wood, 2018). The positive dependency of the cloud albedo on EIS was identified for the two datasets divided in this study, which may be caused by stronger inversions linked to increased stability and reduced vertical exchange at cloud top, resulting in thicker low clouds by keeping moisture trapped in the marine boundary layer (MBL) (Scott et al., 2020). Compared to other meteorological factors, the contribution of SSTadv to cloud albedo was larger and non-negligible in both datasets (7 %–9 %). Under LWP ≤ 65 g m−2, the SSTadv showed a negative coefficient. The cold advection usually thickens clouds by reducing low-level stability and transporting more moisture into the MBL (George and Wood, 2010; Scott et al., 2020). Under LWP > 65 g m−2, the coefficient of SSTadv was positive, which is hard to be explained by the aforementioned mechanism. By analyzing the correlation between LWP and SSTadv, we found that there was no significant correlation between them. This indicates that the surface temperature advection may affect cloud albedo in other ways than by affecting the moisture in cloud layers. Furthermore, dust can affect the meteorological environment through radiative effects; consequently, the positive coefficient found in this study may be a reflection of their effects (Sun and Zhao, 2020; Huang et al., 2021). The coefficients of the omega700 were negative for both datasets. The downdraft allowed dry air above the cloud to enter the clouds, causing evaporation and making cloud droplets smaller and fewer, resulting in reducing the cloud albedo (Yang et al., 2019). Note that the role of omega700 was very weak under the condition with higher LWP, and its contribution was negligible.
The analysis of the relative contribution of each predictor variable was similar to the results of the coefficients. Under LWP ≤ 65 g m−2, DU and BC contributed approximately 63 % of the variations of the cloud albedo in the regression model. Note that the contribution of omega700 and SSTadv was non-negligible, accounting for 18 %. Under LWP > 65 g m−2, the contribution of DU and SO2 to the change in cloud albedo was about 61 %. DU has the largest relative contribution to the cloud albedo changes (∼ 35 %) in both datasets.
The normalized satellite-based and model-driven cloud albedos under different cloud water conditions are shown in Fig. 6c–d, in which the correlation (R) between the two cloud albedos is given in parentheses. A larger R value indicates a better model. Both of the correlation coefficients are greater than 0.65, indicating the regression model properly captures the changes in the cloud albedo for the two datasets. A considerable part of the variation in cloud albedo can be explained by the change in meteorological parameters and mass concentrations of different aerosol types.
In addition, to verify the sensitivity of the results to input data, we employ different datasets to perform the multilinear regression. The monthly Multisensor Advanced Climatology of Liquid Water Path (MAC-LWP) is used to test the sensitivity of the results to the input LWP data (Elsaesser et al., 2017). Considering the differences in retrieval methods and values of the MODIS LWP and MAC-LWP datasets (Greenwald, 2009), we used the threshold of 55 g m−2 for MAC-LWP to better split the samples evenly. The regressed results are given in Fig. S5. We can see that the results did not change significantly, indicating that the regressed results are relatively robust. For the reanalyzed dataset, ERA-5 reanalysis is considered to be the most state-of-the-art reanalysis with higher temporal and spatial resolutions (Hersbach et al., 2019). We also used the ERA-5 data to perform the multiple regression model (see Fig. S6). Although the results change slightly, the changed results do not affect the main conclusions.
Table 3Total correlations between the cloud albedo and different aerosol types, as well as the partial correlations to eliminate the influence of three meteorological parameters individually or simultaneously under different LWP conditions. The value above is under the condition of LWP ≤ 65 g m−2. The value in parentheses is under the condition of 65 g m−2 < LWP ≤ 120 g m−2.
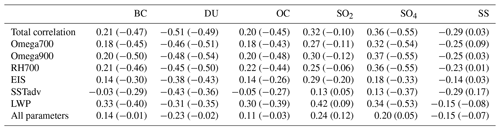
It is also found from Fig. 6 that changes in LWP can also cause an alteration of the relationship between aerosol and the cloud albedo. To further investigate the influence of meteorological factors on the relationship, the partial correlations were calculated to eliminate the influence of meteorological parameters individually or simultaneously. If the partial correlation is similar to the total correlation, it means that the influence of meteorological factors on the relationship is limited. In contrast, the influence of meteorological factors on the relationship may be significant if the partial correlation and the total correlation are the opposite sign. Given six meteorological parameters (omega700, omega900, RH700, EIS, SSTadv and LWP) considered in this study, the total correlation and partial correlation between the cloud albedo and different aerosols for two sample groups are given in Table 3. Under LWP ≤ 65 g m−2, the correlations of all aerosol types were weakened when eliminating the effects of meteorological factors. When the influence of EIS and LWP were eliminated, the correlation of DU becomes much weaker, indicating that the correlation of DU is sensitive to EIS and LWP. On the contrary, the correlations of BC, OC and SO4 were stronger when the influence of LWP was eliminated. In addition, most aerosol types were sensitive to SSTadv except for the SS. The correlation of BC/OC ranged from 0.21/0.20 to -0.03/-0.05 by eliminating the influence of SSTadv, indicating that the relationship between BC/OC and the cloud albedo is extremely sensitive to the influences of SSTadv. Under LWP > 65 g m−2, the correlations of all aerosol types varied significantly by eliminating the influence of all meteorological parameters. For example, the correlation of BC/DU/OC ranged from -0.47/-0.49/-0.45 to -0.01/-0.02/-0.03. This indicates that the cloud–aerosol interaction is more sensitive to the response of meteorological conditions at higher LWP conditions. Although the contribution of meteorological parameters to the change in the cloud albedo is only a small part based on the relative contribution calculation, its influence on cloud–aerosol interactions is not negligible.
The cloud albedo in the marine subtropical stratocumulus regions has a key role in regulating the regional energy budget. However, climate models have a lack of skill to properly capture the cloud properties over the regions. Therefore, the CMIP6 has more of a possibility to improve some long-standing model biases, e.g., the low cloud simulation over tropical oceans and surface processes (Stouffer et al., 2017). Accordingly, considerable improvements in reproducing the observed seasonal planetary albedo over the subtropical stratocumulus have been found in CMIP6 (Jian et al., 2020). To enhance the confidence in climate predictions, it is necessary to systematically evaluate and compare the performance of CMIP5 and CMIP6 models and to further study the processes that contribute to the cloud albedo using the satellite-driven and reanalysis data. This study investigated the performances of CMIP6 models in reproducing the cloud albedo in the five marine subtropical stratocumulus regions from 2003 to 2014.
For the long-term regressed values, the cloud albedos were underestimated in most AMIP6 models compared with the satellite-driven cloud albedos. The AMIP6 models produced a similar spread of AMIP5 in all regions, and even some AMIP6 models performed worse than AMIP5. The monthly cloud albedo of AMIP6-MEM showed better correlation with the satellite-driven observations than that of AMIP5-MEM. However, this study found a lack of skill in reproducing the values and amplitude in some regions (e.g., Peruvian and Namibian), indicating that the cloud parameterization between two generations of AMIP models needs to be further improved to produce more accurate predictions. This study also found that most AMIP6 models overestimated the amplitude of the cloud albedo in all regions except for the Australian region, i.e., simulating higher seasonal variations. Overall, the AMIP6 models performed the best in the Australian region and the worst in the Canarian region. The seasonal cycle of cloud albedo of AMIP6-MEM was correlated better with satellite-driven observations than that of AMIP5-MEM. For the Australian region, the model-driven seasonal cycle of the cloud albedo was almost consistent with that of the satellite-driven observations, which indicates the superiority of model performance in this region.
Employing the satellite and reanalysis data, we further evaluated the impacts of different aerosol types and meteorological factors on the cloud albedo. Changes in aerosol types and meteorological factors explained ∼ 65 % of the changes in the cloud albedo. However, the controlling factors and their contribution rates varied with LWP conditions. Under the monthly mean LWP ≤ 65 g m−2, DU and BC dominantly contributed to the changes in the cloud albedo, while DU and SO2 contributed the most under the condition of 65 g m−2 < LWP ≤ 120 g m−2. Although the contributions of aerosols were significant, the influence of meteorological factors on the cloud–aerosol interactions cannot be ignored.
Due to the limitations of polar-orbiting satellite observations, this study did not obtain a complete diurnal cycle of cloud properties and radiation flux, which may induce a bias in the results of this study. The diurnal cycle of marine subtropical stratocumulus cloud albedo is usually significant due to the diurnal cycle of solar energy (Wood, 2012). The maximum cloud thickness usually occurs in the morning and gradually decreases over the afternoon due to absorbing solar radiation in the cloud layer (Wood et al., 2002; Christensen et al., 2013). It is a challenge to evaluate how much of the cloud albedo bias contributes to the diurnal cycle of cloud albedo. Therefore, it is necessary to evaluate the diurnal cycle of cloud albedo in the marine subtropical stratocumulus regions for reducing the uncertainties in cloud radiation interactions in GCMs. Note that the “too bright, too few” problem was improved in the Namibian and Californian regions in AMIP6. However, even if some models can simulate the cloud albedo more reasonably, it is questionable if other cloud properties can be captured (e.g., total cloud fraction), consequently resulting in significant biases in radiation (see Fig. S7). Therefore, we need to pay more attention to improve the calculation of total cloud fraction in the GCMs. Recently, some studies are devoted to improving cloud overlap parameterization for accurately simulating the cloud fractions in GCMs (Li et al., 2018, 2019). Accordingly, it is also necessary to evaluate the improvement of the cloud overlap scheme on cloud radiation interaction using long-term satellite-driven observations and reanalysis data.
The CERES datasets are available from the CERES website: https://ceres.larc.nasa.gov/data/#single-scanner-footprint-ssf (CERES Science Team, 2019). The MODIS datasets are available from the Level-1 and Atmosphere Archive & Distribution System (LAADS) Distributed Active Archive Center (DAAC) website: https://ladsweb.modaps.eosdis.nasa.gov/archive/allData/61 (MODIS Science Team, 2019). The MERRA-2 reanalysis products are downloaded from the MERRA-2 website: https://disc.gsfc.nasa.gov/datasets?keywords=MERRA-2 (Global Modeling and Assimilation Office, 2019). The CMIP5 and CMIP6 products are downloaded from the Earth System Grid Federation (ESGF) website: https://esgf-node.llnl.gov/projects/esgf-llnl/ (ESGF, 2019).
The supplement related to this article is available online at: https://doi.org/10.5194/acp-21-9809-2021-supplement.
BJ and JL organized the paper and carried out its execution. BJ prepared the manuscript with contributions from all co-authors. JL conceptualized the paper and revised the whole manuscript. GW provided the computing resources. YL downloaded the data and maintained the research data. YZ, JW and MZ processed the raw model output data into consistent gridded format for comparison with the satellite dataset. JH provided consultations and acquired the financial support for this study. All authors contributed to the discussion of the results and reviewed the manuscript.
The authors declare that they have no conflict of interest.
Publisher’s note: Copernicus Publications remains neutral with regard to jurisdictional claims in published maps and institutional affiliations.
This research was jointly supported by the Strategic Priority Research Program of the Chinese Academy of Sciences (XDA2006010301) and the National Science Fund for Excellent Young Scholars (42022037). We would like to thank the CERES, MODIS, CMIP5, CMIP6 and MERRA-2 science teams for providing excellent and accessible data products that made this study possible.
This research has been supported by the Strategic Priority Research Program of the Chinese Academy of Sciences (grant no. XDA2006010301) and the National Science Fund for Excellent Young Scholars (grant no. 42022037).
This paper was edited by Zhanqing Li and reviewed by two anonymous referees.
Amiri-Farahani, A., Allen, R. J., Neubauer, D., and Lohmann, U.: Impact of Saharan dust on North Atlantic marine stratocumulus clouds: importance of the semidirect effect, Atmos. Chem. Phys., 17, 6305–6322, https://doi.org/10.5194/acp-17-6305-2017, 2017.
Betts, A. K. and Ridgway, W.: Climatic equilibrium of the atmospheric convective boundary layer over a tropical ocean, J. Atmos. Sci., 46, 2621–2641, https://doi.org/10.1175/1520-0469(1989)046<2621:CEOTAC>2.0.CO;2, 1989.
Bender, F. A. M., Charlson, R. J., Ekman, A. M. L., and Leahy, L. V.: Quantification of Monthly Mean Regional-Scale Albedo of Marine Stratiform Clouds in Satellite Observations and GCMs, J. Appl. Meteorol. Clim., 50, 2139–2148, https://doi.org/10.1175/jamc-d-11-049.1, 2011.
Bender, F. A. M., Engström, A., and Karlsson, J.: Factors Controlling Cloud Albedo in Marine Subtropical Stratocumulus Regions in Climate Models and Satellite Observations, J. Climate, 29, 3559–3587, https://doi.org/10.1175/Jcli-D-15-0095.1, 2016.
Bender, F. A. M., Engström, A., Wood, R., and Charlson, R. J.: Evaluation of Hemispheric Asymmetries in Marine Cloud Radiative Properties, J. Climate, 30, 4131–4147, https://doi.org/10.1175/Jcli-D-16-0263.1, 2017.
Bender, F. A. M., Frey, L., McCoy, D. T., Grosvenor, D. P., and Mohrmann, J. K.: Assessment of aerosol–cloud–radiation correlations in satellite observations, climate models and reanalysis, Clim. Dynam., 52, 4371–4392, https://doi.org/10.1007/s00382-018-4384-z, 2019.
Boucher, O., Randall, D., Artaxo, P., Bretherton, C., Feingold, G., Forster, P., Kerminen, V.-M., Kondo, Y., Liao, H., Lohmann, U., Rasch, P., Satheesh, S. K., Sherwood, S., Stevens, B., and Zhang, X. Y.: Clouds and aerosols, in: Climate change 2013: the physical science basis. Contribution of Working Group I to the Fifth Assessment Report of the Intergovernmental Panel on Climate Change, Cambridge University Press, Cambridge, United Kingdom and New York, NY, USA, 2013.
CERES Science Team: Single Scanner Footprint (SSF), available at: https://ceres.larc.nasa.gov/data/#single-scanner-footprint-ssf (last access: 25 June 2021), 2019.
Chen, Y. C., Christensen, M. W., Stephens, G. L., and Seinfeld, J. H.: Satellite-based estimate of global aerosol-cloud radiative forcing by marine warm clouds, Nat. Geosci., 7, 643–646, https://doi.org/10.1038/NGEO2214, 2014.
Christensen, M. W., Carrio, G. G., Stephens, G. L., and Cotton, W. R.: Radiative Impacts of Free-Tropospheric Clouds on the Properties of Marine Stratocumulus, J. Atmos. Sci., 70, 3102–3118, https://doi.org/10.1175/jas-d-12-0287.1, 2013.
Das, S., Harshvardhan, H., Bian, H., Chin, M., Curci, G., Protonotariou, A. P., Mielonen, T., Zhang, K., Wang, H., and Liu, X.: Biomass burning aerosol transport and vertical distribution over the South African-Atlantic region, J. Geophys. Res., 122, 6391–6415, https://doi.org/10.1002/2016jd026421, 2017.
Doelling, D. R., Loeb, N. G., Keyes, D. F., Nordeen, M. L., Morstad, D., Nguyen, C., Wielicki, B. A., Young, D. F., and Sun, M.: Geostationary Enhanced Temporal Interpolation for CERES Flux Products, J. Atmos. Ocean Tech., 30, 1072–1090, https://doi.org/10.1175/jtech-d-12-00136.1, 2013.
Doherty, O. M. and Evan, A. T.: Identification of a new dust-stratocumulus indirect effect over the tropical North Atlantic, Geophys. Res. Lett., 41, 6935–6942, https://doi.org/10.1002/2014gl060897, 2014.
Elsaesser, G. S., O'Dell, C. W., Lebsock, M. D., Bennartz, R., Greenwald, T. J., and Wentz, F. J.: The Multisensor Advanced Climatology of Liquid Water Path (MAC-LWP), J. Climate, 30, 10193–10210, https://doi.org/10.1175/jcli-d-16-0902.1, 2017.
Dong, X., Xi, B., Kennedy, A., Minnis, P., and Wood, R.: A 19-Month Record of Marine Aerosol- Cloud-Radiation Properties Derived from DOE ARM Mobile Facility Deployment at the Azores. Part I: Cloud Fraction and Single-Layered MBL Cloud Properties, J. Climate, 27, 3665–3682, https://doi.org/10.1175/jcli-d-13-00553.1, 2014.
Douglas, A. and L'Ecuyer, T.: Quantifying variations in shortwave aerosol–cloud–radiation interactions using local meteorology and cloud state constraints, Atmos. Chem. Phys., 19, 6251–6268, https://doi.org/10.5194/acp-19-6251-2019, 2019.
Eastman, R. and Wood, R.: The Competing Effects of Stability and Humidity on Subtropical Stratocumulus Entrainment and Cloud Evolution from a Lagrangian Perspective, J. Atmos. Sci., 75, 2563–2578, https://doi.org/10.1175/jas-d-18-0030.1, 2018.
Engström, A. and Ekman, A. M. L.: Impact of meteorological factors on the correlation between aerosol optical depth and cloud fraction, Geophys. Res. Lett., 37, L18814, https://doi.org/10.1029/2010gl044361, 2010.
Engström, A., Bender, F. A. M., and Karlsson, J.: Improved Representation of Marine Stratocumulus Cloud Shortwave Radiative Properties in the CMIP5 Climate Models, J. Climate, 27, 6175–6188, https://doi.org/10.1175/jcli-d-13-00755.1, 2014.
Engström, A., Bender, F. A. M., Charlson, R. J., and Wood, R.: The nonlinear relationship between albedo and cloud fraction on near-global, monthly mean scale in observations and in the CMIP5 model ensemble, Geophys. Res. Lett., 42, 9571–9578, https://doi.org/10.1002/2015GL066275, 2015.
ESGF: ESGF@DOE/LLNL, available at: https://esgf-node.llnl.gov/projects/esgf-llnl/ (last access: 25 June 2021), 2019.
Eyring, V., Bony, S., Meehl, G. A., Senior, C. A., Stevens, B., Stouffer, R. J., and Taylor, K. E.: Overview of the Coupled Model Intercomparison Project Phase 6 (CMIP6) experimental design and organization, Geosci. Model Dev., 9, 1937–1958, https://doi.org/10.5194/gmd-9-1937-2016, 2016.
Frey, L., Bender, F. A.-M., and Svensson, G.: Cloud albedo changes in response to anthropogenic sulfate and non-sulfate aerosol forcings in CMIP5 models, Atmos. Chem. Phys., 17, 9145–9162, https://doi.org/10.5194/acp-17-9145-2017, 2017.
Fuchs, J., Cermak, J., and Andersen, H.: Building a cloud in the southeast Atlantic: understanding low-cloud controls based on satellite observations with machine learning, Atmos. Chem. Phys., 18, 16537–16552, https://doi.org/10.5194/acp-18-16537-2018, 2018.
Greenwald, T. J.: A 2 year comparison of AMSR-E and MODIS cloud liquid water path observations, Geophys. Res. Lett., 36, L20805, https://doi.org/10.1029/2009GL040394, 2009.
Gelaro, R., McCarty, W., Suarez, M. J., Todling, R., Molod, A., Takacs, L., Randles, C., Darmenov, A., Bosilovich, M. G., Reichle, R., Wargan, K., Coy, L., Cullather, R., Draper, C., Akella, S., Buchard, V., Conaty, A., da Silva, A., Gu, W., Kim, G. K., Koster, R., Lucchesi, R., Merkova, D., Nielsen, J. E., Partyka, G., Pawson, S., Putman, W., Rienecker, M., Schubert, S. D., Sienkiewicz, M., and Zhao, B.: The Modern-Era Retrospective Analysis for Research and Applications, Version 2 (MERRA-2), J. Climate, 30, 5419–5454, https://doi.org/10.1175/JCLI-D-16-0758.1, 2017.
Georgakakos, K. P. and Bras, R. L.: A hydrologically useful station precipitation model: 1. Formulation, Water Resour. Res., 20, 1585–1596, https://doi.org/10.1029/WR020i011p01585, 1984.
George, R. C. and Wood, R.: Subseasonal variability of low cloud radiative properties over the southeast Pacific Ocean, Atmos. Chem. Phys., 10, 4047–4063, https://doi.org/10.5194/acp-10-4047-2010, 2010.
Global Modeling and Assimilation Office: MERRA-2, available at: https://disc.gsfc.nasa.gov/datasets?keywords=MERRA-2 (last access: 25 June 2021), 2019.
Gryspeerdt, E., Goren, T., Sourdeval, O., Quaas, J., Mülmenstädt, J., Dipu, S., Unglaub, C., Gettelman, A., and Christensen, M.: Constraining the aerosol influence on cloud liquid water path, Atmos. Chem. Phys., 19, 5331–5347, https://doi.org/10.5194/acp-19-5331-2019, 2019.
Han, Q. Y., Rossow, W. B., Zeng, J., and Welch, R.: Three different behaviors of liquid water path of water clouds in aerosol-cloud interactions, J. Atmos. Sci., 59, 726–735, https://doi.org/10.1175/1520-0469(2002)059<0726:tdbolw>2.0.co;2, 2002.
Herbert, R. J., Bellouin, N., Highwood, E. J., and Hill, A. A.: Diurnal cycle of the semi-direct effect from a persistent absorbing aerosol layer over marine stratocumulus in large-eddy simulations, Atmos. Chem. Phys., 20, 1317–1340, https://doi.org/10.5194/acp-20-1317-2020, 2020.
Hersbach, H., Bell, W., Berrisford, P., Horányi, A., Joaquí, M.-S., Nicolas, J., Radu, R., Schepers, D., Simmons, A., Soci, C., and Dee, D.: Global Reanalysis: Goodbye ERA-Interim, Hello ERA5, ECMWF Newslett., 159, 17–24, 2019.
Huang, J. and Yi, Y.: Inversion of a nonlinear dynamical model from the observation, Science in China, 34B, 1246–1251, 1991.
Huang, J. P., Huang, J. P., Liu, X. Y., Li, C. Y., Ding, L., and Yu, H. P.: The global oxygen budget and its future projection, Sci. Bull., 63, 1180–1186, https://doi.org/10.1016/j.scib.2018.07.023, 2018.
Huang, J. P., Liu, X. Y., He, Y. S., Shen, S. Z., Hou, Z. Q., Li, S. G., Li, C. Y., Yao, L. J., and Huang, J. P.: The oxygen cycle and a habitable Earth, Sci. China Earth Sci., 64, 511–528, https://doi.org/10.1007/s11430-020-9747-1, 2021.
Jaeglé, L., Quinn, P. K., Bates, T. S., Alexander, B., and Lin, J.-T.: Global distribution of sea salt aerosols: new constraints from in situ and remote sensing observations, Atmos. Chem. Phys., 11, 3137–3157, https://doi.org/10.5194/acp-11-3137-2011, 2011.
Jian, B., Li, J., Zhao, Y., He, Y., Wang, J., and Huang, J.: Evaluation of the CMIP6 planetary albedo climatology using satellite observations, Clim. Dynam., 54, 5145–5161, https://doi.org/10.1007/s00382-020-05277-4, 2020.
Jiang, J. H., Su, H., Huang, L., Wang, Y., Massie, S., Zhao, B., Omar, A., and Wang, Z.: Contrasting effects on deep convective clouds by different types of aerosols, Nat. Commun., 9, 3874, https://doi.org/10.1038/s41467-018-06280-4, 2018.
Johnson, B. T., Shine, K. P., and Forster, P. M.: The semi-direct aerosol effect: Impact of absorbing aerosols on marine stratocumulus, Q. J. Roy. Meteor. Soc., 130, 1407–1422, https://doi.org/10.1256/qj.03.61, 2004.
Karydis, V. A., Kumar, P., Barahona, D., Sokolik, I. N., and Nenes, A.: On the effect of dust particles on global cloud condensation nuclei and cloud droplet number, J. Geophys. Res., 116, D23204, https://doi.org/10.1029/2011jd016283, 2011.
Kawai, H., Yukimoto, S., Koshiro, T., Oshima, N., Tanaka, T., Yoshimura, H., and Nagasawa, R.: Significant improvement of cloud representation in the global climate model MRI-ESM2, Geosci. Model Dev., 12, 2875–2897, https://doi.org/10.5194/gmd-12-2875-2019, 2019.
Klein, S. A. and Hartmann, D. L.: THE SEASONAL CYCLE OF LOW STRATIFORM CLOUDS, J. Climate, 6, 1587–1606, https://doi.org/10.1175/1520-0442(1993)006<1587:tscols>2.0.co;2, 1993.
Kravitz, B., Wang, H., Rasch, P. J., Morrison, H., and Solomon, A. B.: Process-model simulations of cloud albedo enhancement by aerosols in the Arctic, Philos. T. R. Soc. A., 372, 20140052, https://doi.org/10.1098/rsta.2014.0052, 2014.
Latham, J., Rasch, P., Chen, C.-C., Kettles, L., Gadian, A., Gettelman, A., Morrison, H., Bower, K., and Choularton, T.: Global temperature stabilization via controlled albedo enhancement of low-level maritime clouds, Philos. T. R. Soc. A., 366, 3969–3987, https://doi.org/10.1098/rsta.2008.0137, 2008.
Levin, Z., Ganor, E., and Gladstein, V.: The effects of desert particles coated with sulfate on rain formation in the eastern Mediterranean, J. Appl. Meteorol., 35, 1511–1523, https://doi.org/10.1175/1520-0450(1996)035<1511:teodpc>2.0.co;2, 1996.
Li, J., Jian, B., Huang, J., Hu, Y., Zhao, C., Kawamoto, K., Liao, S., and Wu, M.: Long-term variation of cloud droplet number concentrations from space-based Lidar, Remote Sens. Environ., 213, 144–161, https://doi.org/10.1016/j.rse.2018.05.011, 2018.
Li, J., Jian, B., Zhao, C., Zhao, Y., Wang, J., and Huang, J.: Atmospheric Instability Dominates the Long-Term Variation of Cloud Vertical Overlap Over the Southern Great Plains Site, J. Geophys. Res., 124, 9691–9701, https://doi.org/10.1029/2019jd030954, 2019.
Lin, W. Y., Zhang, M. H., and Loeb, N. G.: Seasonal Variation of the Physical Properties of Marine Boundary Layer Clouds off the California Coast, J. Climate, 22, 2624–2638, https://doi.org/10.1175/2008jcli2478.1, 2009.
Loeb, N. G., Priestley, K. J., Kratz, D. P., Geier, E. B., Green, R. N., Wielicki, V. A., Hinton, R. O., and Nolan, S. K.: Determination of unfiltered radiances from the clouds and the Earth's Radiant Energy System instrument, J. Appl. Meteorol., 40, 822–835, https://doi.org/10.1175/1520-0450(2001)040<0822:dourft>2.0.co;2, 2001.
Loeb, N. G., Doelling, D. R., Wang, H. L., Su, W. Y., Nguyen, C., Corbett, J. G., Liang, L. S., Mitrescu, C., Rose, F. G., and Kato, S.: Clouds and the Earth's Radiant Energy System (CERES) Energy Balanced and Filled (EBAF) Top-of-Atmosphere (TOA) Edition-4.0 Data Product, J. Climate, 31, 895–918, https://doi.org/10.1175/Jcli-D-17-0208.1, 2018.
Lu, C., Liu, Y., Niu, S., and Vogelmann, A. M.: Observed impacts of vertical velocity on cloud microphysics and implications for aerosol indirect effects, Geophys. Res. Lett., 39, L21808, https://doi.org/10.1029/2012gl053599, 2012.
McCoy, D. T., Bender, F. A. M., Mohrmann, J. K. C., Hartmann, D. L., Wood, R., and Grosvenor, D. P.: The global aerosol-cloud first indirect effect estimated using MODIS, MERRA, and AeroCom, J. Geophys. Res., 122, 1779–1796, https://doi.org/10.1002/2016jd026141, 2017.
McCoy, D. T., Bender, F. A.-M., Grosvenor, D. P., Mohrmann, J. K., Hartmann, D. L., Wood, R., and Field, P. R.: Predicting decadal trends in cloud droplet number concentration using reanalysis and satellite data, Atmos. Chem. Phys., 18, 2035–2047, https://doi.org/10.5194/acp-18-2035-2018, 2018.
MODIS Science Team: Index of /archive/allData/61/, available at: https://ladsweb.modaps.eosdis.nasa.gov/archive/allData/61 (last access: 25 June 2021), 2019.
Mueller, R., Trentmann, J., Traeger-Chatterjee, C., Posselt, R., and Stoeckli, R.: The Role of the Effective Cloud Albedo for Climate Monitoring and Analysis, Remote Sens., 3, 2305–2320, https://doi.org/10.3390/rs3112305, 2011.
Nam, C., Bony, S., Dufresne, J. L., and Chepfer, H.: The “too few, too bright” tropical low-cloud problem in CMIP5 models, Geophys. Res. Lett., 39, L21801, https://doi.org/10.1029/2012gl053421, 2012.
Platnick, S., King, M. D., Ackerman, S. A., Menzel, W. P., Baum, B. A., Riedi, J. C., and Frey, R. A.: The MODIS cloud products: Algorithms and examples from Terra, IEEE T. Geosci. Remote, 41, 459-473, https://doi.org/10.1109/tgrs.2002.808301, 2003.
Pradelle, F. and Cautenet, G.: Radiative and microphysical interactions between marine stratocumulus clouds and Saharan dust – 2. Modeling, J. Geophys. Res., 107, 4413, https://doi.org/10.1029/2000jd000156, 2002.
Pradelle, F., Cautenet, G., and Jankowiak, I.: Radiative and microphysical interactions between marine stratocumulus clouds and Saharan dust – 1. Remote sensing observations, J. Geophys. Res., 107, 4412, https://doi.org/10.1029/2000jd000155, 2002.
Roberts, G. C., Ramana, M. V., Corrigan, C., Kim, D., and Ramanathan, V.: Simultaneous observations of aerosol-cloud-albedo interactions with three stacked unmanned aerial vehicles, P. Natl. Acad. Sci. USA, 105, 7370–7375, https://doi.org/10.1073/pnas.0710308105, 2008.
Rosenfeld, D., Rudich, Y., and Lahav, R.: Desert dust suppressing precipitation: A possible desertification feedback loop, P. Natl. Acad. Sci. USA, 98, 5975–5980, https://doi.org/10.1073/pnas.101122798, 2001.
Rosenfeld, D., Zhu, Y., Wang, M., Zheng, Y., Goren, T., and Yu, S.: Aerosol-driven droplet concentrations dominate coverage and water of oceanic low-level clouds, Science, 363, eaav0566, https://doi.org/10.1126/science.aav0566, 2019.
Seethala, C., Norris, J. R., and Myers, T. A.: How Has Subtropical Stratocumulus and Associated Meteorology Changed since the 1980s?*, J. Climate, 28, 8396–8410, https://doi.org/10.1175/jcli-d-15-0120.1, 2015.
Qiu, Y. M., Zhao, C. F., Guo, J. P., and Li, J. M.: 8-Year ground-based observational analysis about the seasonal variation of the aerosol-cloud droplet effective radius relationship at SGP site, Atmos. Environ., 164, 139–146, https://doi.org/10.1016/j.atmosenv.2017.06.002, 2017.
Qu, X., Hall, A., Klein, S. A., and DeAngelis, A. M.: Positive tropical marine low-cloud cover feedback inferred from cloud-controlling factors, Geophys. Res. Lett., 42, 7767–7775, https://doi.org/10.1002/2015GL065627, 2015.
Scott, R. C., Myers, T. A., Norris, J. R., Zelinka, M. D., Klein, S. A., Sun, M. G., and Doelling, D. R.: Observed Sensitivity of Low-Cloud Radiative Effects to Meteorological Perturbations over the Global Oceans, J. Climate, 33, 7717–7734, https://doi.org/10.1175/Jcli-D-19-1028.1, 2020.
Seland, Ø., Bentsen, M., Olivié, D., Toniazzo, T., Gjermundsen, A., Graff, L. S., Debernard, J. B., Gupta, A. K., He, Y.-C., Kirkevåg, A., Schwinger, J., Tjiputra, J., Aas, K. S., Bethke, I., Fan, Y., Griesfeller, J., Grini, A., Guo, C., Ilicak, M., Karset, I. H. H., Landgren, O., Liakka, J., Moseid, K. O., Nummelin, A., Spensberger, C., Tang, H., Zhang, Z., Heinze, C., Iversen, T., and Schulz, M.: Overview of the Norwegian Earth System Model (NorESM2) and key climate response of CMIP6 DECK, historical, and scenario simulations, Geosci. Model Dev., 13, 6165–6200, https://doi.org/10.5194/gmd-13-6165-2020, 2020.
Stouffer, R. J., Eyring, V., Meehl, G. A., Bony, S., Senior, C., Stevens, B., and Taylor, K. E.: CMIP5 Scientific Gaps and Recommendations for CMIP6, B. Am. Meteorol. Soc., 98, 95–105, https://doi.org/10.1175/bams-d-15-00013.1, 2017.
Stuart, G. S., Stevens, R. G., Partanen, A.-I., Jenkins, A. K. L., Korhonen, H., Forster, P. M., Spracklen, D. V., and Pierce, J. R.: Reduced efficacy of marine cloud brightening geoengineering due to in-plume aerosol coagulation: parameterization and global implications, Atmos. Chem. Phys., 13, 10385–10396, https://doi.org/10.5194/acp-13-10385-2013, 2013.
Sun, Y. and Zhao, C. F.: Influence of Saharan Dust on the Large-Scale Meteorological Environment for Development of Tropical Cyclone Over North Atlantic Ocean Basin, J. Geophys. Res., 125, e2020JD033454, https://doi.org/10.1029/2020JD033454, 2020.
Taylor, K. E.: Summarizing multiple aspects of model performance in a single diagram, J. Geophys. Res., 106, 7183–7192, https://doi.org/10.1029/2000jd900719, 2001.
Taylor, K. E., Stouffer, R. J., and Meehl, G. A.: An overview of CMIP5 and the experiment design, B. Am. Meteorol. Soc., 93, 485–498, 2012.
Twohy, C. H.: Evaluation of the aerosol indirect effect in marine stratocumulus clouds: Droplet number, size, liquid water path, and radiative impact, J. Geophys. Res., 110, D08203, https://doi.org/10.1029/2004jd005116, 2005.
Twomey, S.: The nuclei of natural cloud formation. Part II: The supersaturation in natural clouds and the variation of cloud droplet concentration, Geofis. Pura Appl., 243–249, https://doi.org/10.1007/BF01993560, 1959.
Twomey, S.: Pollution and the planetary albedo, Atmos. Environ., 8, 1251–1256, https://doi.org/10.1016/0004-6981(74)90004-3, 1974.
Twomey, S.: Influence of pollution on shortwave albedo of clouds, J. Atmos. Sci., 34, 1149–1152, https://doi.org/10.1175/1520-0469(1977)034<1149:TIOPOT>2.0.CO;2, 1977.
Van Weverberg, K., Morcrette, C. J., Petch, J., Klein, S. A., Ma, H. Y., Zhang, C., Xie, S., Tang, Q., Gustafson, W. I., Qian, Y., Berg, L. K., Liu, Y., Huang, M., Ahlgrimm, M., Forbes, R., Bazile, E., Roehrig, R., Cole, J., Merryfield, W., Lee, W. S., Cheruy, F., Mellul, L., Wang, Y. C., Johnson, K., and Thieman, M. M.: CAUSES: Attribution of Surface Radiation Biases in NWP and Climate Models near the U.S. Southern Great Plains, J. Geophys. Res., 123, 3612–3644, https://doi.org/10.1002/2017jd027188, 2018.
Wall, C. J., Hartmann, D. L., Thieman, M. M., Smith, W. L., and Minnis, P.: The Life Cycle of Anvil Clouds and the Top-of-Atmosphere Radiation Balance over the Tropical West Pacific, J. Climate, 31, 10059–10080, https://doi.org/10.1175/jcli-d-18-0154.1, 2018.
Wang, H., Rasch, P. J., and Feingold, G.: Manipulating marine stratocumulus cloud amount and albedo: a process-modelling study of aerosol-cloud-precipitation interactions in response to injection of cloud condensation nuclei, Atmos. Chem. Phys., 11, 4237–4249, https://doi.org/10.5194/acp-11-4237-2011, 2011.
Wang, Y. and Zhao, C.: Can MODIS cloud fraction fully represent the diurnal and seasonal variations at DOE ARM SGP and Manus sites?, J. Geophys. Res., 122, 329–343, https://doi.org/10.1002/2016jd025954, 2017.
Waquet, F., Peers, F., Ducos, F., Goloub, P., Platnick, S., Riedi, J., Tanré, D., and Thieuleux, F.: Global analysis of aerosol properties above clouds, Geophys. Res. Lett., 40, 5809–5814, https://doi.org/10.1002/2013gl057482, 2013.
Wielicki, B. A., Barkstrom, B. R., Harrison, E. F., Lee III, R. B., Louis Smith, G., and Cooper, J. E.: Clouds and the Earth's Radiant Energy System (CERES): An earth observing system experiment, B. Am. Meteorol. Soc., 77, 853–868, 1996.
Wilcox, E. M.: Stratocumulus cloud thickening beneath layers of absorbing smoke aerosol, Atmos. Chem. Phys., 10, 11769–11777, https://doi.org/10.5194/acp-10-11769-2010, 2010.
Wilcox, E. M.: Direct and semi-direct radiative forcing of smoke aerosols over clouds, Atmos. Chem. Phys., 12, 139–149, https://doi.org/10.5194/acp-12-139-2012, 2012.
Wood, R.: Stratocumulus Clouds, Mon. Weather Rev., 140, 2373–2423, https://doi.org/10.1175/mwr-d-11-00121.1, 2012.
Wood, R. and Bretherton, C. S.: On the relationship between stratiform low cloud cover and lower-tropospheric stability, J. Climate, 19, 6425–6432, https://doi.org/10.1175/Jcli3988.1, 2006.
Wood, R., Bretherton, C. S., and Hartmann, D. L.: Diurnal cycle of liquid water path over the subtropical and tropical oceans, Geophys. Res. Lett., 29, 2092, https://doi.org/10.1029/2002GL015371, 2002.
Xie, S. C., Liu, X. H., Zhao, C. F., and Zhang, Y. Y.: Sensitivity of CAM5-Simulated Arctic Clouds and Radiation to Ice Nucleation Parameterization, J. Climate, 26, 5981–5999, https://doi.org/10.1175/Jcli-D-12-00517.1, 2013.
Yang, Y., Zhao, C. F., Dong, X. B., Fan, G. C., Zhou, Y. Q., Wang, Y., Zhao, L. J., Lv, F., and Yan, F.: Toward understanding the process-level impacts of aerosols on microphysical properties of shallow cumulus cloud using aircraft observations, Atmos. Res., 221, 27–33, https://doi.org/10.1016/j.atmosres.2019.01.027, 2019.
Zhao, C. F., Klein, S. A., Xie, S. C., Liu, X. H., Boyle, J. S., and Zhang, Y. Y.: Aerosol first indirect effects on non-precipitating low-level liquid cloud properties as simulated by CAM5 at ARM sites, Geophys. Res. Lett., 39, L08806, https://doi.org/10.1029/2012gl051213, 2012.
Zhao, C. F., Lin, Y. L., Wu, F., Wang, Y., Li, Z. Q., Rosenfeld, D., and Wang, Y.: Enlarging Rainfall Area of Tropical Cyclones by Atmospheric Aerosols, Geophys. Res. Lett., 45, 8604–8611, https://doi.org/10.1029/2018GL079427, 2018.
Zhao, C. F., Chen, Y. Y., Li, J. M., Letu, H. S., Su, Y. F., Chen, T. M., and Wu, X. L.: Fifteen-year statistical analysis of cloud characteristics over China using Terra and Aqua Moderate Resolution Imaging Spectroradiometer observations, Int. J. Climatol., 39, 2612–2629, https://doi.org/10.1002/joc.5975, 2019a.
Zhao, C. F., Zhao, L. J., and Dong, X. B.: A Case Study of Stratus Cloud Properties Using In Situ Aircraft Observations over Huanghua, China, Atmos., 10, 19, https://doi.org/10.3390/atmos10010019, 2019b.
Zuidema, P., Sedlacek, A. J., III, Flynn, C., Springston, S., Delgadillo, R., Zhang, J., Aiken, A. C., Koontz, A., and Muradyan, P.: The Ascension Island Boundary Layer in the Remote Southeast Atlantic is Often Smoky, Geophys. Res. Lett., 45, 4456–4465, https://doi.org/10.1002/2017gl076926, 2018.