the Creative Commons Attribution 4.0 License.
the Creative Commons Attribution 4.0 License.
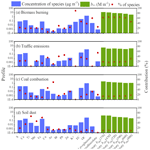
Measurement report: quantifying source contribution of fossil fuels and biomass-burning black carbon aerosol in the southeastern margin of the Tibetan Plateau
Huikun Liu
Qiyuan Wang
Li Xing
Yong Zhang
Ting Zhang
Weikang Ran
Junji Cao
Anthropogenic emissions of black carbon (BC) aerosol are transported from Southeast Asia to the southwestern Tibetan Plateau (TP) during the pre-monsoon; however, the quantities of BC from different anthropogenic sources and the transport mechanisms are still not well constrained because there have been no high-time-resolution BC source apportionments. Intensive measurements were taken in a transport channel for pollutants from Southeast Asia to the southeastern margin of the TP during the pre-monsoon to investigate the influences of fossil fuels and biomass burning on BC. A receptor model that coupled multi-wavelength absorption with aerosol species concentrations was used to retrieve site-specific Ångström exponents (AAEs) and mass absorption cross sections (MACs) for BC. An “aethalometer model” that used those values showed that biomass burning had a larger contribution to BC mass than fossil fuels (BCbiomass=57 % versus BCfossil=43 %). The potential source contribution function indicated that BCbiomass was transported to the site from northeastern India and northern Burma. The Weather Research and Forecasting model coupled with chemistry (WRF-Chem) indicated that 40 % of BCbiomass originated from Southeast Asia, while the high BCfossil was transported from the southwest of the sampling site. A radiative transfer model indicated that the average atmospheric direct radiative effect (DRE) of BC was +4.6 ± 2.4 W m−2, with +2.5 ± 1.8 W m−2 from BCbiomass and +2.1 ± 0.9 W m−2 from BCfossil. The DRE of BCbiomass and BCfossil produced heating rates of 0.07 ± 0.05 and 0.06 ± 0.02 K d−1, respectively. This study provides insights into sources of BC over a transport channel to the southeastern TP and the influence of the cross-border transportation of biomass-burning emissions from Southeast Asia during the pre-monsoon.
- Article
(5087 KB) -
Supplement
(1109 KB) - BibTeX
- EndNote
The Tibetan Plateau (TP) is an important regulator of climate change in the Northern Hemisphere, and it plays a crucial role in the functions of the global ecosystem and climate stability (Liu et al., 2019, 2020a). The TP is covered by one of the largest ice masses on Earth, and it has been called the water tower of Asia (Liu et al., 2020b). The glaciers on the TP are facing rapid retreat, however, and if unchecked, this could result in adverse effects on the Asian hydrological cycle and Asian monsoon (Luo et al., 2020; Hua et al., 2019). In spring, the glaciers on the TP begin to melt as part of the natural hydrological cycle, but the increasing quantities of black carbon (BC) aerosol transported to the TP have accelerated this process (Bond et al., 2013) by causing a warming effect in atmosphere over the TP and enhancing the absorption of radiation on the surface of the glaciers (Ming et al., 2009).
The southern part of the TP is bounded by South Asia, where air pollution often is severe (Chan et al., 2017). Several studies have shown that pollutants (including BC) from South Asia can be transported to the south of the TP along mountain valleys, especially during the pre-monsoon (March–May), when southwesterly winds prevail (e.g., Cao et al., 2010; Xia et al., 2011; Zhu et al., 2017; Niu et al., 2017). For example, Xia et al. (2011) analyzed satellite data and air mass trajectories and found that the TP, particularly the southern TP, was affected by pollutants carried by southwesterly winds from nearby regions in South Asia. In addition, numerous studies have shown that the high bulk BC mass loadings and the associated regional influences on the TP are related to transport from South Asia (Liu et al., 2015; Han et al., 2020; Cong et al., 2015; Wang et al., 2015). Nonetheless, assessments of regional transport of bulk BC aerosol have not fully revealed the impacts of different BC emission sources because the optical properties and radiative effects of BC can not only vary among sources in complex ways but can also be affected by aging during transport (Tian et al., 2019; Zhang et al., 2019). Therefore, quantitative information on the contributions of different sources of BC over the TP is lacking, but it is critically needed for a better understanding of the influence of anthropogenic emissions on its environment and climate.
Several studies have assessed the contributions of different BC sources through model simulations or isotopic methods. For example, Zhang et al. (2015) investigated BC sources for different parts of the TP by using a chemical transport model and a source-tagging approach, and they found that the contributions of BC sources varied among regions and with the seasons. Li et al. (2016) used filter sampling and carbon isotopes (Δ14C and δ13 C) to determine the BC from fossil fuels and biomass burning in several areas of the TP. A major disadvantage of filter-based measurements is that they are constrained by low time resolution, which makes it challenging to capture the detailed evolution of pollution events. On the other hand, the accuracy of model simulations is dependent on many factors, including uncertainties associated with initial particle parameters, aging processes, the accuracy of emission inventory, meteorological fields over the complex terrain, the modules for chemistry and planetary boundary layer (PBL) dynamics, etc. (Koch et al., 2009; Madala et al., 2014; Vignati et al., 2010). Nonetheless, these studies have been helpful for understanding the sources of BC over the TP.
To make up for the deficiencies of filter-based analysis, BC source apportionments based on high-time-resolution online data have been conducted in many locations (e.g., Herich et al., 2011; Zhu et al., 2017; Rajesh and Ramachandran, 2018) but for the TP are limited. An “aethalometer model” based on multi-wavelength absorption data is one efficient approach for distinguishing between BC from fossil fuel and biomass-burning sources (Sandradewi et al., 2008). The accuracy of the aethalometer model relies on the input parameters, including absorption Ångström exponent (AAE) and BC mass absorption cross section (MACBC) of different sources (Zotter et al., 2017). Limited information on site-specific Ångström exponents (AAEs) and MACBCs lead most studies to rely on values taken from measurements made in other locations (e.g., Healy et al., 2017; Zhu et al., 2017). This results in unquantified uncertainties because the AAE and MACBCs can vary with specific fuel subtypes and combustion conditions (Wang et al., 2018; Tian et al., 2019). Therefore site-dependent AAEs and MACBC are essential for improving the reliability of BC source apportionment by the aethalometer model.
In this study, field measurements of BC were taken on the southeastern margin of the TP during the pre-monsoon. This region connects the high-altitude TP with the low-altitude Yungui Plateau and forms a transport channel for pollutants from Southeast Asia (Wang et al., 2019a), and it is an ideal region for investigating the impact of pollutant transport to the southeastern TP. A receptor model combined with multi-wavelength absorption with aerosol species concentrations was used to retrieve site-dependent AAEs and MACBCs. This was done to improve the aethalometer model with the goal of obtaining a more accurate BC source apportionment. The primary objectives of this study were to (1) quantify the mass concentrations of BC from fossil fuel and biomass-burning sources, (2) determine the impact of regional transport on source-specific BC, and (3) assess the radiative effects caused by BC from different sources. This study provides insights into the BC sources on the southeastern TP and an assessment of their radiative effects during the pre-monsoon.
2.1 Sampling site
Intensive field measurements were made at the rooftop of a building (∼ 10 m above the ground) at the Lijiang Astronomical Station, Chinese Academy of Sciences (3260 m above sea level; N, E), Gaomeigu County, Yunnan Province, China (Fig. 1), from 14 March to 13 May 2018. During the campaign, westerly winds created a potential pathway for cross-border transport from Southeast Asia to southwestern China. During the study, the average relative humidity and temperature were 80 % ± 20 % and 7.6 ± 3.2∘, respectively; the mean wind speed near the surface was 5.4 ± 2.1 m s−1, and the winds were mainly from the west and southwest. The sampling site is 3–5 km from Gaomeigu village, which has 27 households and 110 residents. Villagers there rely on farming for their livelihoods, and biomass is the primary residential fuel (Li et al., 2016). There are no large industries near the village, and traffic is light. However, two highways (Hangzhou–Ruili Expressway and Dali–Nujiang Expressway) are located ∼ 5.5 km to the west of the sampling site.
2.2 Online and offline measurements
Aerosol light absorption coefficients at multiple wavelengths (babs(λ), λ=370, 470, 520, 590, 660, 880 and 950 nm) were retrieved with the use of a model AE33 aethalometer (Magee Scientific, Berkeley, CA, USA). The sampled particles were selected by a PM2.5 cuff-off inlet (SCC 1.829, BGI Inc. USA) and dried with a Nafion® dryer (MD-700-24S-3, Perma Pure, Inc., Lakewood, NJ, USA), and the flow rate of the sampler is 5 L min−1. Detailed operating principles of the AE33 aethalometer can be found in Drinovec et al. (2015). Briefly, light at wavelengths (λ) of 370, 470, 520, 590, 660, 880 and 950 nm emitted from diodes is used to irradiate aerosol deposition spots on the filters. The light attenuation produced by the captured particles is measured with optical detectors. Non-linear loading and filter matrix scattering effects are common issues for filter-based absorption measurements (Collaud Coen et al., 2010). A dual-spot technique for the aethalometer measurements was used to compensate for non-linearity, while a factor of 2.14 was used to correct the artifacts caused by quartz filters (Drinovec et al., 2015).
A photoacoustic extinctiometer (PAX, Droplet Measurement Technology, Boulder, CO, USA) was used to determine the aerosol light scattering and absorption coefficient (bscat(532) and babs(532), respectively), which were used to calculate the single scattering albedo (SSA = at λ=532 nm. The bscat was measured using a wide-angle (5–175∘) integrating reciprocal nephelometer in the scattering chamber. The babs(532) was measured simultaneously with an intracavity photoacoustic technique in the acoustic chamber. A detailed description of the PAX can be found in Carrico et al. (2018). During the campaign, the selected concentrations of ammonium sulfate and freshly generated propane soot were used to calibrate the bscat(532) and babs(532) measurements, respectively. Details regarding the calibration procedure are in Wang et al. (2018).
Daily PM2.5 filters were collected for the analysis of selected chemical species. Organic carbon (OC) and elemental carbon (EC) were determined using a thermal and optical carbon analyzer (Atmoslytic Inc., Calabasas, CA, USA). Water-soluble potassium ions (i.e., K+) and levoglucosan were analyzed with the use of an ion chromatograph (Dionex Inc., Sunnyvale, CA, USA). The inorganic elements (i.e., S, Ca, Ti, Mn, Fe, Cu, As, Br, Pb, Zn) were measured using an energy-dispersive X-ray fluorescence spectrometry (Epsilon 5 ED-XRF, PANalytical B.V., Netherlands). Finally, an organic marker of benzothiazolone was determined using a high-performance liquid chromatography (Series 1200, Agilent Technology, Santa Clara, CA). Detailed descriptions of the chemical analyses are given in Text S1 in the Supplement.
2.3 BC source apportionment
The aethalometer model proposed by Sandradewi et al. (2008) was optimized by excluding the babs(370) contributed by the secondary aerosols and soil dust (babs(370)secondary and babs(370)dust, respectively).
The formulae used for the aethalometer model were as follows:
where AAEfossil and AAEbiomass are the AAEs for emissions of fossil fuel contribution and biomass burning. These were retrieved through an optical source apportionment and are discussed in Sect. 3.1; babs(370) and babs(880) are the measured babs at λ=370 and 880 nm, respectively; the absorption coefficients for fossil fuel are babs(370)fossil and babs(880)fossil, while those for biomass-burning sources are babs(370)biomass and babs(880)biomass. A source apportionment of the optical data was used to calculate babs(370)dust as discussed in Sect. 3.1, while babs(370)secondary was estimated using a BC-tracer method combined with a minimum R-squared approach as described by Wang et al. (2019a).
After obtaining babs(880)fossil and babs(880)biomass, the mass concentrations of BC from fossil fuel combustion and biomass burning (BCfossil and BCbiomass, respectively) were estimated as follows:
where MACBC(880)fossil and MACBC(880)biomass are the MACBCs at λ=880 nm generated from fossil fuel combustion and biomass burning, respectively. The MACBC(880)s for the two sources were retrieved from a source apportionment of the optical data discussed in Sect. 3.1.
2.4 Optical source apportionment
Optical source apportionments were obtained using a positive matrix factorization (PMF) model. The fundamental objective of the PMF for applications such as ours is to resolve the chemical mass balance by separating the data matrix into factor contributions and factor profiles as follows:
where Xij represents the input matrix elements, p is the number of sources, gik is the source contribution of the kth factor to the ith sample, fkj is the factor profile of jth species in the kth factor, and eij is representative of the residual. The values gik and fkj are non-negative. The two matrices are resolved by minimizing the sum of squares of the normalized residuals as follows:
where Q represents the object function, and uij denotes the uncertainties in Xij. The PMF version 5.0 (PMF5.0, from the US EPA) was used for the analyses, and the optical parameters (primary babs at different wavelengths) and chemical species concentrations (including carbonaceous aerosols, inorganic elements, K+, levoglucosan and organic markers) were used as model inputs for the optical source apportionment.
2.5 Trajectory-related analysis
To determine the influences of regional transport on BC at Gaomeigu, trajectory clusters were produced from hourly 3 d backward-in-time air mass trajectories at 500 m above the ground level. The trajectories were calculated with the Hybrid Single-Particle Lagrangian Integrated Trajectory model (Draxler and Hess, 1998). The meteorological data were from the Global Data Assimilation System (GDAS; https://www.ready.noaa.gov/gdas1.php, last access: 17 April 2020), which takes into account the influences of terrain. As we focused on differentiating and clustering the main spatial features of the incoming trajectories, an angle-oriented distance definition was adopted in the cluster analysis. Details regarding the trajectory clustering methods can be found in Wang et al. (2018). For the investigations of the effects of transport on the chemical and optical properties of the BC aerosol, trajectories with BC mass concentration greater than the 75th percentile were considered to be polluted.
The potential source contribution function (PSCF) was used to identify the likely pollution regions that influenced BC loadings at Gaomeigu based on the back trajectories. The geographic region covered by the trajectories was overlaid by a 0.5∘ × 0.5∘ (i, j) grid. The PSCF value of each grid was calculated as follows:
where mij is the number of endpoints associated with BC mass concentration higher than the set criterion, and nij is the total endpoints of the ijth cell. To improve the resolution of PSCF source identifications, the 75th percentile of each source's BC mass concentration was set as the criterion for a polluted sample (i.e., 0.6 µg m−3 for BCbiomass and 0.45 µg m−3 for BCfossil) (Cheng and Lin, 2001). Furthermore, arbitrary weighting factors (wij) were applied to different nij ranges to reduce the uncertainty caused by the small nij (Polissar et al., 1999), and they were defined by the following the approach of Polissar et al. (2001):
2.6 Regional chemical dynamical model
The Weather Research and Forecasting model coupled with chemistry (WRF-Chem) was used to quantify the contribution of biomass burning from Southeast Asia to BC mass at Gaomeigu. Detailed descriptions of the model configurations have been described in our previous publication (Xing et al., 2020). Briefly, the model resolution was 3 km × 3 km, and there were 320 grid cells. The domain included the southwest of China and southern and southeastern Asia, with a center at 26.70∘ N, 100.03∘ E. Thirty-five vertical layers have been set in the model from the ground surface to 50 hPa. The BC emission inventory used for the model was based on the Asian anthropogenic emission inventory (that is MIX) for the year 2010 (Li et al., 2017). The inventory has a spatial resolution of 0.25∘ × 0.25∘, and it included industry, power, transportation and residential sources (e.g., fossil fuel and biofuel). The FINN fire inventory (Wiedinmyer et al., 2011) was used for the biomass-burning emission during the simulation.
2.7 Estimations of direct radiative effects and heating rate
The direct radiative effect (DRE) of source-specific BC were estimated with the widely used Santa Barbara DISORT Atmospheric Radiative Transfer (SBDART) model, a detailed description of which may be found in Ricchiazzi and Yang (1998). The important input parameters include aerosol optical depth (AOD), light extinction coefficient (bscat+babs), SSA, asymmetric parameter (ASP) and visibility. Here, the input optical parameters were estimated by the Optical Property of Aerosol and Cloud (OPAC) model using Mie theory (Hess et al., 1998). The measured BC, water-soluble matter (including measured water-soluble inorganic ions and water-soluble organic matter that assumes accounting for 79 % of OC loading; Xu et al., 2015) and water-insoluble matter (calculated as the PM2.5 mass concentration minus that of BC and water-soluble matter) were used in the OPAC model to retrieve the number concentrations of these particles. These were tuned until the modeled bscat(λ), babs(λ) and SSA were within ±5 % (Srivastava et al., 2012) of the values measured by the PAX (see Table S1 in the Supplement) and used to obtain the optical parameters at the nearest observed relative humidity. The underlying assumption was that when the modeled bscat(λ) and babs(λ) were very close to their measured counterparts, the derived optical parameters were a reasonable representation of the measured aerosols. This assumption has been widely used in previous studies (Dumka et al., 2018; Panicker et al., 2010; Rajesh and Ramachandran, 2018). Finally, the DREs attributable to source-specific BC (or PM2.5) at the surface atmosphere (SUF) and the top of the atmosphere (TOA) were estimated as the difference in the net flux with and without BC (or PM2.5) under cloud-free conditions. The solar-heating-rate change induced by atmospheric DRE (DRE at TOA subtracts DRE at SUF) was calculated as follows (Ramachandran and Kedia, 2010):
where DREATM (W m−2) is the atmospheric DRE, DRETOP is the DRE at top atmosphere, DRESUF is the DRE at surface atmosphere, is the heating rate (K d−1), g is the acceleration due to gravity (9.8 m s−2), Cp is the specific heat capacity of air at constant pressure, and ΔP is the difference in atmospheric pressure between the ground and 3 km above.
3.1 Source-dependent AAEs and MACs
Four sources were identified as the main contributors to primary babs(λ) based on the optical source apportionment (Fig. 2). The simulated primary babs(λ) values at different wavelengths all correlated well (r=0.96–0.97, p<0.01; Fig. S1) with the model inputs, indicating that the PMF5.0 performed well. As shown in Fig. 2, the first source factor exhibited high contributions of K+ (90 %), levoglucosan (60 %) and primary babs(λ) (45 %–64 %) as well as moderate loadings of OC (38 %) and EC (47 %). The K+ and levoglucosan are widely used markers for biomass burning (Urban et al., 2012), and these chemical markers are strong indications that this factor resulted from biomass-burning emissions. Furthermore, we note that the presence of BrC in this factor made higher absorption at shorter wavelengths, which is consistent with absorption features of biomass-burning emissions (Forello et al., 2019).
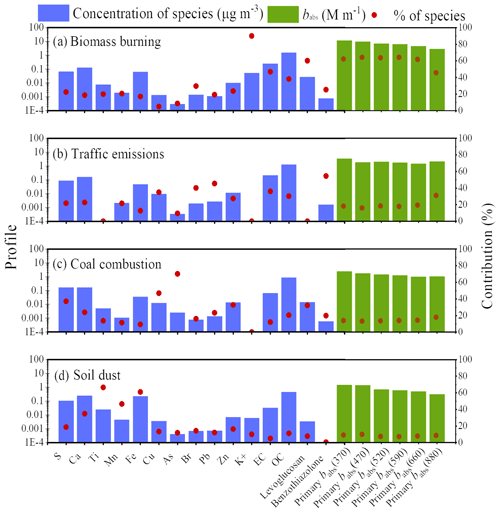
Figure 2Four aerosol light absorption sources identified by a positive matrix factorization (PMF) model. Concentrations (µg m−3) of the chemical species for each source are in purple. Primary babs (λ) at six wavelengths (λ=370, 470, 520, 590, 660 or 880 nm) in each source (M m−1) is in green.
Based on the contributions of biomass burning to babs(370) and babs(880), the AAEbiomass was estimated to be 1.7, which is within a relative boarder range of AAEbiomass (1.2–3.5) determined by other methods (e.g., 14C and organic tracers) in previous studies (Sandradewi et al., 2008; Helin et al., 2018; Harrison et al., 2012; Zotter et al., 2017). The estimated average MACBC(880)biomass was 10.4 m2 g−1; this is more than twice the value for uncoated BC particles suggested by Bond and Bergstrom (2006) (MACBC(880)uncoated=4.7 m2 g−1, extrapolated from 550 to 880 nm by assuming AAEBC = 1.0). The large MACBC(880) indicates that BC particles from biomass burning experienced substantial aging processes during their transport because numerous studies have confirmed that aged BC could result in MAC increases by a factor of 1.5–3.5 relative to uncoated particles (Chen et al., 2017; Ma et al., 2020) due to the “lensing effect” (Lack and Cappa, 2010).
The second source factor was characterized by large loadings of benzothiazolone (54 %), Pb (46 %), Br (40 %), Cu (35 %), Zn (27 %), EC (36 %) and OC (30 %). Benzothiazolone is released from the breakdown of the antioxidant in motor vehicle tires (Cheng et al., 2006), while Br is another tracer of motor vehicle emission (Guo et al., 2009). Similarly, Zn and Cu are associated with the combustion of lubricating fluids and the wear of brakes and tires (Lough et al., 2005; Song et al., 2006). Finally, EC and OC also are components of motor vehicle emissions (Cao et al., 2013). Although unleaded gasoline has been used extensively in China since 2005, a considerable portion of Pb in the environment is still associated with vehicle-related particles, especially from the wear of metal alloys (Hao et al., 2019). Therefore, this second source factor was identified as traffic-related emissions. This source constitutes a moderate percentage of primary babs(λ) (15 %–30 %). The estimated traffic-emission-related AAE (AAEtraffic) was 0.8, consistent with the finding that BC is the dominant light-absorbing carbonaceous aerosol species for traffic emissions (Kirchstetter et al., 2004). The AAEtraffic found here also was close to a value obtained using the 14C approach (Zotter et al., 2017). The estimated MACBC(880) of traffic emissions (MACBC(880)traffic=9.1 m2 g−1) was similar with MACBC(880)biomass, indicating that traffic-emission-related BC particles were also subjected to substantial aging.
The third factor was dominated by high loadings of As (70 %), S (37 %) and Cu (47 %), which are typically associated with coal combustion (Hsu et al., 2016; Kim and Hopke, 2008). Although coal is not used extensively near the site on the TP, emissions from coal combustion may have been transported to the site from surrounding areas (e.g., East Asia; Li et al., 2016). This source contributed 12 %–17 % of primary babs(λ), which is less than that from biomass burning or traffic emissions. The obtained AAE of coal combustion (AAEcoal=1.1) was similar to the AAEtraffic, suggesting that BC was also the dominant light-absorbing carbon species in coal combustion emissions. The AAEcoal was close to the value of chunk coal combustion (1.3) but lower than that for briquettes of coal (2.6) (Sun et al., 2017); this presumably reflects the types of coal transported to Gaomeigu, at least to some degree. The estimated BC MAC(880) of coal combustion (MACBC(880)coal=15.5 m2 g−1) was larger than MACBC(880)biomass and MACBC(880)traffic. The enhancement factor for MACBC(880)coal (3.3) falls near the upper limit of this range noted above, and although this is likely related to the aging of BC particles during transport to Gaomeigu, more work is need to verify this contention in future studies.
The fourth source factor had high loadings of Ca (35 %), Ti (66 %), Mn (47 %) and Fe (61 %), consistent with the characteristics of crustal elements (Guo et al., 2009); thus, it was assigned to soil dust. The light absorption of soil dust is mainly due to the presence of iron oxides and varies with the types and relative concentrations of iron oxide species (Alfaro, 2004; Valenzuela et al., 2015). The mineral dust here contributed a relatively small amount to the primary babs(λ) (6 %–9 %), presumably due to the low levels of iron oxides, and this is consistent with the other results obtained on the southeastern TP (Zhao et al., 2019). The estimated AAE of mineral dust (AAEdust) was 1.5, which is within the range of 1.2–3.0 obtained from multiple non-oceanic sites (Dubovik et al., 2002).
3.2 BC source apportionment
As the results above show that fossil-fuel-related BC aerosol mainly originated from traffic and coal combustion, the AAEfossil (0.9) and MACBC(880)fossil (12.3 m2 g−1) were averaged by the values of AAEtraffic + AAEcoal and MACBC(880)traffic + MACBC(880)coal, respectively, as shown in Table 1. Based on the source-specific AAEs (i.e., AAEfossil and AAEbiomass) and MACBC(880) (i.e., MACBC(880)fossil and MACBC(880)biomass), the mass concentrations of BCfossil and BCbiomass were then estimated using the improved aethalometer model (Eqs. 1–6). As shown in Fig. 3a, no correlation (r=0.01, p=0.02) was found between BCbiomass and BCfossil, implying that BC from these two sources was effectively separated by the improved aethalometer model. The data for the biomass-burning and traffic-related tracers (levoglucosan and benzothiazolone, respectively) further support the results of BC source apportionment. That is, BCbiomass was significantly correlated with levoglucosan (r=0.75, p<0.01; Fig. 3b), and the same was true for BCfossil benzothiazolone (r=0.67, p<0.01; Fig. 3c), respectively. These results indicate that the source-specific AAEs and MACs(880) obtained from optical source apportionment were appropriate
Table 1Derived Ångström absorption exponents (AAEs), mass absorption coefficients (MACs) and percent source contribution of black carbon (BC) from difference sources.
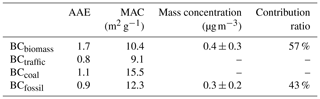
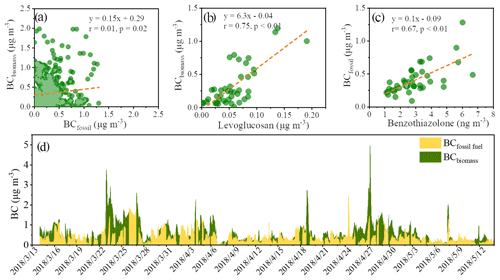
Figure 3Scatterplots of (a) biomass-burning black carbon (BCbiomass) versus fossil fuel combustion BC (BCfossil), (b) BCbiomass versus levoglucosan and (c) BCfossil versus benzothiazolone. (d) Time series of hourly averaged mass concentrations of BCbiomass and BCfossil.
Figure 3d shows a time series plot of hourly averaged mass concentrations of total BC, BCbiomass and BCfossil during the campaign. The hourly total BC mass concentration varied ∼ 50-fold, from 0.1 to 4.9 µg m−3, with an arithmetic mean (± standard deviation) of 0.7 (±0.5) µg m−3, which was lower than what has been reported for the western TP but higher than on the northern TP (Wang et al., 2018, and references therein). The larger BC loading on the western TP can be explained by relatively strong influences from Southeast Asia, where anthropogenic activities are intensive (Kurokawa et al., 2013). With reference to BC sources, the concentrations and contributions as listed in Table 1 show higher mass concentrations of BCbiomass (0.4 ± 0.3 µg m−3, 57 % of total BC) compared with BCfossil (0.3 ± 0.2 µg m−3, 43 % of total BC) on average. The mass fraction of BCbiomass increased with the BC loadings, while BCfossil mass fraction showed an inverse relationship to the loadings (Fig. S2). One can infer from this that biomass-burning emissions were responsible for the high-BC-loading episode during the campaign.
Distinct diurnal variations in the mass concentrations of BCbiomass and BCfossil were observed as shown in Fig. 4a. The BCbiomass started to increase after midnight, reached a small peak at ∼ 05:00 (all times given in local time: UTC+8) and then remained at a constant level before sunrise (∼ 08:00). This may be attributed to effects associated with changes in the height of the planetary boundary layer (PBL) (https://rda.ucar.edu/datasets/ds083.2, last access: 17 April 2020) (Fig. 4b). Thereafter, the BCbiomass increased again and reached the maximum value at midday. This enhancement was accompanied by an increase in PBL height and higher wind speed (https://rda.ucar.edu/datasets/ds083.2) (Fig. 4b). Generally, higher PBLs and stronger wind cause local pollutants to disperse and as a result lower their loadings (Wang et al., 2015). However, the buildup of BCbiomass in daytime at Gaomeigu was more likely influenced by the transport of BCbiomass from regions upwind. After sunrise, the PBL began to deepen, and that was accompanied with west and southwest winds from 08:00 to 12:00 (Fig. S3). These meteorological conditions are favorable for pollutant (including BC) transport from high-density biomass-burning emission areas to the sampling site (Chan et al., 2017). After the midday peak, BCbiomass decreased sharply until midnight. The initial portion of this decrease (13:00–18:00) occurred as the PBL height and wind speed increased, which promoted the dispersion of BCbiomass. Subsequent reduction occurred at night even though the PBL height and wind speed decreased, and that was likely due to the curtailment of local biomass-burning activities.
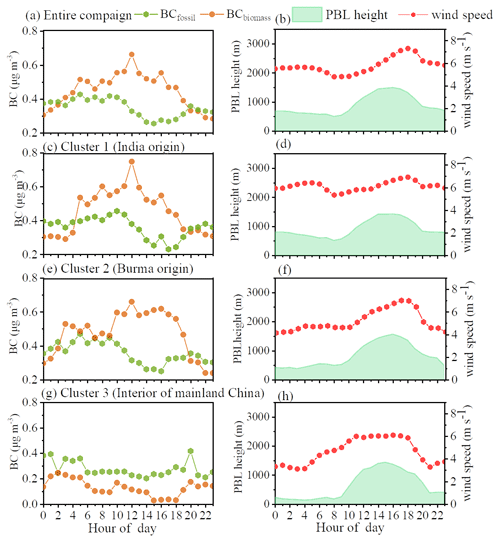
Figure 4Left panels: diurnal variations in hourly averaged black carbon (BC) aerosol from biomass burning (BCbiomass) and fossil fuel sources (BCfossil). Right panels: wind speeds and planetary boundary layer (PBL) heights during the entire campaign and for different air mass directions determined by air mass trajectory clusters 1–3.
As shown in Fig. 4a, the BCfossil showed a diurnal trend that was roughly opposite of that of the unimodal pattern seen for BCbiomass, and that may be explained as follows. Increases in the PBL height and wind speed from 09:00 to 15:00 were associated with a decrease in BCfossil, unlike the increasing trend seen for BCbiomass; this presumably reflects minor effects from the regional transport on BCfossil. Further, because of the small contribution of coal combustion to EC (12 %; Fig. 2c), the BCfossil was best explained by motor vehicle emissions from areas upwind of the site. The subsequent increase in BCfossil from 17:00 to 20:00 was attributed to the reduction in PBL height and as a result the buildup of pollutants in the near-surface air. As there were minimal impacts from traffic at night, the BCfossil loadings remained steady from 21:00 to 08:00. The stable nocturnal BCfossil may reflect the impact of fossil fuel emissions on BC in the southeastern margin of the TP due to the accumulation resulting from the low PBL heights.
3.3 Regional influences of BCbiomass and BCfossil
To investigate the regional impacts on BC, three groups of air masses were identified based on their transport pathways (Fig. 5a). Cluster 1 originated from northeastern India and then passed over Bangladesh before arriving at Gaomeigu. The average BC mass concentration of this cluster was the highest (0.8 ± 0.4 µg m−3) of the three clusters. About 74 % of total trajectories were associated with Cluster 1, of which 22 % were identified as polluted and had an average BC loading of 1.3 ± 0.5 µg m−3. Cluster 2 originated over Burma and had an average BC loading of 0.7 ± 0.7 µg m−3. This cluster accounted for only 24 % of total trajectories, but among them, about 37 % referred to pollution with BC reaching as high as 1.6 ± 0.9 µg m−3. The air masses associated with Cluster 3 originated from the interior of China, and this group had the lowest BC mass concentrations of the three clusters: 0.4 ± 0.1 µg m−3. This third cluster was composed of a small fraction of total trajectories (2 %), and none of them were identified as polluted, suggesting minor influences from mainland China during the campaign.
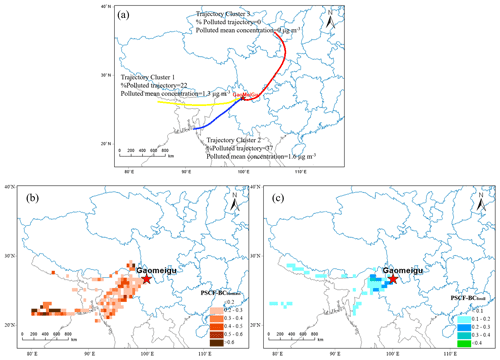
Figure 5Maps of (a) the mean trajectory clusters, (b) the potential source contribution function for black carbon (BC) aerosol from biomass burning (BCbiomass) and (c) from fossil fuel sources (BCfossil).
The diurnal patterns of BCbiomass and BCfossil mass loadings from the three clusters were used to investigate the impacts of regional transport. As shown in Fig. 4c and e, similar diurnal variations in BCbiomass were found for Clusters 1 and 2; they both had larger values during daytime (8:00–12:00) compared with night. This pattern of higher daytime BCbiomass was associated with regional transport from northeastern India (Cluster 1) and Burma (Cluster 2). For Cluster 3, BCbiomass decreased during the day and increased at night (Fig. 4g), and that pattern tracked the daily variations in PBL height. Unlike Clusters 1 and 2, the diurnal variation in BCbiomass for Cluster 3 was more likely due to influences of biomass-burning activities from areas surrounding the sampling site than regional transport. However, it should be noted that these cases were uncommon because only 2 % of air masses were associated with Cluster 3.
For BCfossil, similar diurnal patterns were found for Clusters 1 and 2 (Fig. 4c and e), most likely due to the influences of traffic emissions from surrounding areas as well as daily cycles of PBL height as discussed in Sect. 3.2. The BCfossil loadings of Cluster 3 (Fig. 4g) were relatively stable, showing only sporadic fluctuations. Unlike the declining trend of BCfossil during the daytime found for Clusters 1 and 2, the relatively stable BCfossil loadings in Cluster 3 indicate that there were emissions from fossil fuel sources that offset the effect of the changes in PBL height. The transportation sector has grown rapidly in mainland China (Liu, 2019), and the regional transport of motor vehicle emissions may have been the cause for the observed diurnal variations in BCfossil for Cluster 3.
The PSCF model was applied to further investigate the likely spatial distribution of pollution source regions for BCbiomass and BCfossil. As shown in Fig. 5b, a low PSCF value of BCbiomass was found near Gaomeigu, while high values were concentrated in northeastern India and northern Burma, consistent with intensive fire activities in these areas (Fig. S4). This indicates that large BCbiomass loadings at Gaomeigu were more likely influenced by cross-border transport of biomass burning rather than local emissions. For BCfossil (Fig. 5c), the most likely impact region was located to the southwest of Gaomeigu, near where two highways are located (e.g., Hangzhou–Ruili Expressway and Dali–Nujiang Expressway). Owing to the low consumption of coal on the southeastern TP (Li et al., 2016), the high PSCF values of BCfossil were more likely from traffic emissions than coal combustion. Moreover, sporadic high PSCF values of BCfossil were also found in northern Burma, indicating possible influences of fossil fuel emissions here.
To further quantify the contributions of the BC transported from Southeast Asia to Gaomeigu, we studied a high-BC episode (23–27 March 2018) using a simulation with the WRF-Chem model. Two scenarios of emissions were simulated: one involved all BC emission sources, and the other turned off biomass-burning emissions in Southeast Asia. The variation in modeled BC mass concentration shows an acceptable degree of consistency with the measured values (r=0.63, p<0.01; Fig. S5), Furthermore, the index of agreement was estimated to be 0.77, indicating that the development of this BC episode was effectively captured by the WRF-Chem model. Nonetheless, the normalized mean bias between the measured and modeled BC values was estimated to be 24 %, suggesting that simulation was biased towards high values. This discrepancy is best attributed to the uncertainties in the simulation associated with the emission inventory and meteorological conditions. Figure 6a shows the spatial distributions of BC loadings in Gaomeigu and surrounding areas. The mass concentrations of BC at times exceeded 15 µg m−3 over Burma and northern India, and that is more than an order of magnitude higher compared with the southeastern margin of the TP (0.7 µg m−3). After turning off the biomass-burning emission source in Southeast Asia, the BC loading at the southeastern TP dropped over 40 % (Fig. 6b), suggesting a substantial impact of biomass-burning activities in Southeast Asian countries, which is consistent with results of the trajectory cluster analysis and PSCF.
3.4 Radiative effects and heating rate
Figure 7 shows the average atmospheric direct radiative effects of PM2.5 and BC (including BCbiomass and BCfossil) at the TOA and SUF during the campaign. The average PM2.5 DRE at the TOA was +0.03 ± 1.1 W m−2, implying that the positive effect of light-absorbing carbon on the temperature of the atmosphere outweighed the negative effect of scattering aerosols. In fact, BC produced +1.6 ± 0.8 W m−2 at the TOA on average. At the SUF, BC DRE (−3.0 ± 1.5 W m−2) contributed nearly half of the PM2.5 DRE (−6.3 ± 4.5 W m−2). The difference in the DRE between the TOA and SUF was +4.6 ± 2.4 W m−2 for BC aerosol, and that accounted for 73 % of the DRE attributed to PM2.5 (6.3 ± 4 W m−2); this suggests a substantial radiative effect caused by BC over the southeastern margin of the TP even though its mass fraction is small in PM2.5 (3.3 %).
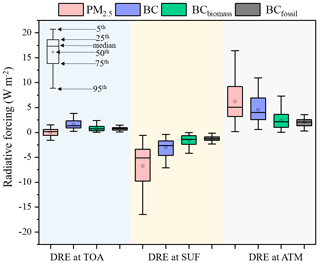
Figure 7The average atmospheric direct radiative effects (DREs) of total black carbon (BC); BC from biomass burning (BCbiomass) and fossil fuel (BCfossil); and PM2.5 at the Earth's surface (SUF), the top of the atmosphere (TOA) and in the atmosphere (ATM = TOA − SUF).
With respect to the BC sources (Fig. 7), the average BCbiomass (BCfossil) DRE was +0.8 ± 0.6 W m−2 (+0.7 ± 0.4 W m−2) at the TOA and −1.7 ± 1.2 W m−2 (−1.4 ± 0.6 W m−2) at the SUF. This is equivalent to an average atmospheric DRE of +2.5 ± 1.8 W m−2 (+2.1 ± 0.9 W m−2). Presumably, the influences of regional transport caused the atmospheric DRE of BCbiomass to be more variable compared with that from BCfossil (Fig. S6). For example, the atmospheric DRE of BCbiomass can be as high as +6.4 W m−2 when the air masses passed over the biomass-burning regions in Southeast Asia, while it was only 1.1 ± 0.2 W m−2 on average when the air masses passed from the mainland of China.
The calculations showing a positive atmospheric DRE imply that energy was trapped in the atmosphere, which would lead to atmospheric heating over the study region. The heating rate calculated for BC varied from 0.02 to 0.3 K d−1 and yielded an average of 0.13 ± 0.07 K d−1. In terms of the DRE efficiency, the heating rate caused by a unit mass concentration of BC in this region was (0.19 (K d−1) (µg m−3)−1), which is roughly comparable with that reported for Qinghai Lake, on the northeastern TP (0.13 (K d−1) (µg m−3)−1) (Wang et al., 2015), but it is generally lower than the values in the southwestern regions of the Himalaya (Fig. S7). Moreover, the heating rate caused by BCbiomass may have been slightly higher (0.07 ± 0.05 K d−1) compared with BCfossil (0.06 ± 0.02 K d−1). Finally, the heating rate of BCbiomass increased to 0.16 K d−1 when the BC mass concentration was heavily influenced by the polluted air from Southeast Asia.
This study quantified the source contributions of BC aerosol from fossil fuel and biomass burning at a site on the southeastern margin of the TP that represents a regional transport channel for air pollution during the pre-monsoon. The study was conducted in the pre-monsoon when the southeastern TP was heavily influenced by the air mass from Southeast Asia. To reduce the uncertainties caused by interferences in absorption measurements (i.e. secondary absorption and dust) and assumptions relative to AAEs and MACBCs, the traditional aethalometer model was optimized in two aspects. First, a BC-tracer method coupled with a minimum R-squared approach was applied to separate secondary absorption from the total absorption, and as a result, the interferences of absorption from secondary aerosols have been eliminated. Then, an optical-source-apportionment model that used primary multi-wavelength absorption and chemical species as inputs was used to derive site-dependent AAE and MACBC values; these minimize the uncertainties associated with prior assumptions on these parameters. The AAE (MACBC) calculated in this way was 0.9 (12.3 m2 g−1) for fossil fuel source and 1.7 (10.4 m2 g−1) for biomass burning. The results of aethalometer model that used these values showed that the average mass concentration of BC was 0.7 ± 0.5 µg m−3, of which 43 % was BC from fossil fuel, and 57 % was from biomass burning. Trajectory analysis showed that the BCbiomass over the site was mainly driven by regional transport from northeastern India and Burma, while BCfossil was primarily influenced by traffic emissions from areas surrounding the sampling site. Moreover, the WRF-Chem model indicates that biomass burning in Southeast Asia contributes 40 % of the BC loading over the southeastern margin of the TP. The SBDART model showed a DRE of +4.6 ± 2.4 W m−2 for the total PM2.5 BC, of which +2.5 ± 1.8 W m−2 was from BCbiomass and +2.1 ± 0.9 W m−2 from BCfossil. The results of this study provide useful information concerning the sources of BC over an atmospheric transport channel to the southeastern TP, and they highlight the importance of the cross-border transport of biomass-burning emissions from Southeast Asia to the region during the pre-monsoon.
The data presented in this study are available at the Zenodo data archive https://doi.org/10.5281/zenodo.4452056 (Liu et al., 2021).
The supplement related to this article is available online at: https://doi.org/10.5194/acp-21-973-2021-supplement.
QW and JC designed the study. WR conducted the field measurements. LX provided the results of the WRF-Chem model. YZ and TZ performed the chemical analysis of filters. HL and QW wrote the article. All the authors reviewed and commented on the paper.
The authors declare that they have no conflict of interest.
The authors are grateful to the staff from Lijiang Astronomical Station for their assistance with field sampling. The authors are also grateful for the helpful comments from the editor and two referees.
This work was supported by the National Natural Science Foundation of China (grant no. 41877391), the Second Tibetan Plateau Scientific Expedition and Research Program (STEP) (grant no. 2019QZKK0602), the Strategic Priority Research Program of Chinese Academy of Sciences (grant no. XDB40000000), and the Youth Innovation Promotion Association of the Chinese Academy of Sciences (grant no. 2019402).
This paper was edited by Jianping Huang and reviewed by Laura Gallardo and one anonymous referee.
Alfaro, S. C.: Iron oxides and light absorption by pure desert dust: An experimental study, J. Geophys. Res.-Atmos., 109, D08208, https://doi.org/10.1029/2003jd004374, 2004.
Bond, T. C. and Bergstrom, R. W.: Light absorption by carbonaceous particles: An investigative review, Aerosol. Sci. Tech., 40, 27–67, https://doi.org/10.1080/02786820500421521, 2006.
Bond, T. C., Doherty, S. J., Fahey, D. W., Forster, P. M., Berntsen, T., DeAngelo, B. J., Flanner, M. G., Ghan, S., Kärcher, B., Koch, D., Kinne, S., Kondo, Y., Quinn, P. K., Sarofim, M. C., Schultz, M. G., Schulz, M., Venkataraman, C., Zhang, H., Zhang, S., Bellouin, N., Guttikunda, S. K., Hopke, P. K., Jacobson, M. Z., Kaiser, J. W., Klimont, Z., Lohmann, U., Schwarz, J. P., Shindell, D., Storelvmo, T., Warren, S. G., and Zender, C. S.: Bounding the role of black carbon in the climate system: A scientific assessment, J. Geophys. Res.-Atmos., 118, 5380–5552, https://doi.org/10.1002/jgrd.50171, 2013.
Cao, J., Tie X., Xu B., Zhao, Z., Zhu, C., Li, G., and Liu, S.: Measuring and modelling black carbon (BC) contamination in the SE Tibetan Plateau, J. Atmos. Chem., 67, 45–60, https://doi.org/10.1007/s10874-011-9202-5, 2010.
Cao, J.-J., Zhu, C.-S., Tie, X.-X., Geng, F.-H., Xu, H.-M., Ho, S. S. H., Wang, G.-H., Han, Y.-M., and Ho, K.-F.: Characteristics and sources of carbonaceous aerosols from Shanghai, China, Atmos. Chem. Phys., 13, 803–817, https://https://doi.org/10.5194/acp-13-803-2013, 2013.
Carrico, C. M., Gomez, S. L., Dubey, M. K., and Aiken, A. C.: Low hygroscopicity of ambient fresh carbonaceous aerosols from pyrotechnics smoke, Atmos. Environ., 178, 101–108, https://doi.org/10.1016/j.atmosenv.2018.01.024, 2018.
Chan, C. Y., Wong, K. H., Li, Y. S., Chan, Y., and Zhang, X. D.: The effects of Southeast Asia fire activities on tropospheric ozone, trace gases and aerosols at a remote site over the Tibetan Plateau of Southwest China, Tellus B, 58B, 310–318, https://doi.org/10.1111/j.1600-0889.2006.00187.x, 2017
Chen, B., Bai, Z., Cui, X., Chen, J., Andersson, A., and Gustafsson, O.: Light absorption enhancement of black carbon from urban haze in Northern China winter, Environ. Pollut., 221, 418–426, https://doi.org/10.1016/j.envpol.2016.12.004, 2017.
Cheng, M.-D. and Lin, C.-J.: Receptor modelling for smoke of 1998 biomass burning in Central America, J. Geophys. Res.-Atmos., 106, 22871–22886, https://doi.org/10.1029/2001jd900024, 2001.
Cheng, Y., Li, S., and Leithead, A.: Chemical characteristics and origins of nitrogen-containing organic compounds in PM2.5 aerosols in the lower fraser valley, Environ. Sci. Technol., 40, 5846–5852, https://doi.org/10.1021/es0603857, 2006.
Collaud Coen, M., Weingartner, E., Apituley, A., Ceburnis, D., Fierz-Schmidhauser, R., Flentje, H., Henzing, J. S., Jennings, S. G., Moerman, M., Petzold, A., Schmid, O., and Baltensperger, U.: Minimizing light absorption measurement artifacts of the Aethalometer: evaluation of five correction algorithms, Atmos. Meas. Tech., 3, 457–474, https://https://doi.org/10.5194/amt-3-457-2010, 2010.
Cong, Z., Kang, S., Kawamura, K., Liu, B., Wan, X., Wang, Z., Gao, S., and Fu, P.: Carbonaceous aerosols on the south edge of the Tibetan Plateau: concentrations, seasonality and sources, Atmos. Chem. Phys., 15, 1573–1584, https://https://doi.org/10.5194/acp-15-1573-2015, 2015.
Draxler, R. and Hess, G.: An overview of the HYSPLIT_4 modelling system for trajectories, Aust. Meteorol. Mag., 47, 295–308, 1998.
Drinovec, L., Močnik, G., Zotter, P., Prévôt, A. S. H., Ruckstuhl, C., Coz, E., Rupakheti, M., Sciare, J., Müller, T., Wiedensohler, A., and Hansen, A. D. A.: The “dual-spot” Aethalometer: an improved measurement of aerosol black carbon with real-time loading compensation, Atmos. Meas. Tech., 8, 1965–1979, https://https://doi.org/10.5194/amt-8-1965-2015, 2015.
Dubovik, O., Holben, B., Eck, T. F., Smirnov, A., Kaufman, Y. J., King, M. D., Tanré, D., and Slutsker, I.: Variability of absorption and optical properties of key aerosol types observed in worldwide locations, J. Atmos. Sci., 59, 590–608, https://doi.org/10.1175/1520-0469(2002)059<0590:VOAAOP>2.0.CO;2, 2002.
Dumka, U. C., Kaskaoutis, D. G., Tiwari, S., Safai, P. D., Attri, S. D., Soni, V. K., Singh, N., and Mihalopoulos, N.: Assessment of biomass burning and fossil fuel contribution to black carbon concentrations in Delhi during winter, Atmos. Environ., 194, 93–109, https://doi.org/10.1016/j.atmosenv.2018.09.033, 2018.
Forello, A. C., Bernardoni, V., Calzolai, G., Lucarelli, F., Massabò, D., Nava, S., Pileci, R. E., Prati, P., Valentini, S., Valli, G., and Vecchi, R.: Exploiting multi-wavelength aerosol absorption coefficients in a multi-time resolution source apportionment study to retrieve source-dependent absorption parameters, Atmos. Chem. Phys., 19, 11235–11252, https://https://doi.org/10.5194/acp-19-11235-2019, 2019.
Guo, H., Ding, A. J., So, K. L., Ayoko, G., Li, Y. S., and Hung, W. T.: Receptor modelling of source apportionment of Hong Kong aerosols and the implication of urban and regional contribution, Atmos. Environ., 43, 1159–1169, https://doi.org/10.1016/j.atmosenv.2008.04.046, 2009.
Han, H., Wu, Y., Liu, J., Zhao, T., Zhuang, B., Wang, H., Li, Y., Chen, H., Zhu, Y., Liu, H., Wang, Q., Li, S., Wang, T., Xie, M., and Li, M.: Impacts of atmospheric transport and biomass burning on the inter-annual variation in black carbon aerosols over the Tibetan Plateau, Atmos. Chem. Phys., 20, 13591–13610, https://doi.org/10.5194/acp-20-13591-2020, 2020.
Hao, Y., Gao, C., Deng, S., Yuan, M., Song, W., Lu, Z., and Qiu, Z.: Chemical characterisation of PM2.5 emitted from motor vehicles powered by diesel, gasoline, natural gas and methanol fuel, Sci. Total Environ., 674, 128–139, https://doi.org/10.1016/j.scitotenv.2019.03.410, 2019.
Harrison, R. M., Beddows, D. C. S., Hu, L., and Yin, J.: Comparison of methods for evaluation of wood smoke and estimation of UK ambient concentrations, Atmos. Chem. Phys., 12, 8271–8283, https://doi.org/10.5194/acp-12-8271-2012, 2012.
Healy, R. M., Sofowote, U., Su, Y., Debosz, J., Noble, M., Jeong, C. H., Wang, J. M., Hilker, N., Evans, G. J., Doerksen, G., Jones, K., and Munoz, A.: Ambient measurements and source apportionment of fossil fuel and biomass burning black carbon in Ontario, Atmos. Environ., 161, 34–47, https://doi.org/10.1016/j.atmosenv.2017.04.034, 2017.
Helin, A., Niemi, J. V., Virkkula, A., Pirjola, L., Teinilä, K., Backman, J., Aurela, M., Saarikoski, S., Rönkkö, T., Asmi, E., and Timonen, H.: Characteristics and source apportionment of black carbon in the Helsinki metropolitan area, Finland, Atmos. Environ., 190, 87–98, https://doi.org/10.1016/j.atmosenv.2018.07.022, 2018.
Herich, H., Hueglin, C., and Burchmann, B.: A 2.5 year’s source apportionment study of black carbon from wood burning and fossil fuel combustion at urban and rural sites in Switzerland, Atmos. Meas. Tech., 4, 1409–1420, https://doi.org/10.5194/amt-4-1409-2011, 2011.
Hess, M., Koepke, P., and Schult, I.: Optical properties of aerosols and clouds: The software package OPAC, B. Am. Meteorol. Soc., 79, 831–844, https://doi.org/10.1175/1520-0477(1998)079<0831:Opoaac>2.0.Co;2, 1998.
Hsu, C.-Y., Chiang, H.-C., Lin, S.-L., Chen, M.-J., Lin, T.-Y., and Chen, Y.-C.: Elemental characterization and source apportionment of PM10 and PM2.5 in the western coastal area of central Taiwan, Sci. Total Environ., 541, 1139–1150, https://doi.org/10.1016/j.scitotenv.2015.09.122, 2016.
Hua, S., Liu, Y., Luo, R., Shao, T., and Zhu, Q.: Inconsistent aerosol indirect effects on water clouds and ice clouds over the Tibetan Plateau, Int. J. Climatol., 40, 3832–3848, https://doi.org/10.1002/joc.6430, 2019.
Kim, E. and Hopke, P. K.: Source characterization of ambient fine particles at multiple sites in the Seattle area, Atmos. Environ., 42, 6047–6056, https://doi.org/10.1016/j.atmosenv.2008.03.032, 2008.
Kirchstetter, T. W., Novakov, T., and Hobbs, P. V.: Evidence that the spectral dependence of light absorption by aerosols is affected by organic carbon, J. Geophys. Res.-Atmos., 109, D21208, https://doi.org/10.1029/2004jd004999, 2004.
Koch, D., Schulz, M., Kinne, S., McNaughton, C., Spackman, J. R., Balkanski, Y., Bauer, S., Berntsen, T., Bond, T. C., Boucher, O., Chin, M., Clarke, A., De Luca, N., Dentener, F., Diehl, T., Dubovik, O., Easter, R., Fahey, D. W., Feichter, J., Fillmore, D., Freitag, S., Ghan, S., Ginoux, P., Gong, S., Horowitz, L., Iversen, T., Kirkevåg, A., Klimont, Z., Kondo, Y., Krol, M., Liu, X., Miller, R., Montanaro, V., Moteki, N., Myhre, G., Penner, J. E., Perlwitz, J., Pitari, G., Reddy, S., Sahu, L., Sakamoto, H., Schuster, G., Schwarz, J. P., Seland, Ø., Stier, P., Takegawa, N., Takemura, T., Textor, C., van Aardenne, J. A., and Zhao, Y.: Evaluation of black carbon estimations in global aerosol models, Atmos. Chem. Phys., 9, 9001–9026, https://doi.org/10.5194/acp-9-9001-2009, 2009.
Kurokawa, J., Ohara, T., Morikawa, T., Hanayama, S., Janssens-Maenhout, G., Fukui, T., Kawashima, K., and Akimoto, H.: Emissions of air pollutants and greenhouse gases over Asian regions during 2000–2008: Regional Emission inventory in ASia (REAS) version 2, Atmos. Chem. Phys., 13, 11019–11058, https://doi.org/10.5194/acp-13-11019-2013, 2013.
Lack, D. A. and Cappa, C. D.: Impact of brown and clear carbon on light absorption enhancement, single scatter albedo and absorption wavelength dependence of black carbon, Atmos. Chem. Phys., 10, 4207–4220, https://doi.org/10.5194/acp-10-4207-2010, 2010.
Li, C., Bosch, C., Kang, S., Andersson, A. Chen., P. Zhang, Q., Cong., Z. Chen, B., and Gustafsson., Ö.: Sources of black carbon to the Himalayan–Tibetan Plateau glaciers, Nat. Commun., 7, 12574, https://https://doi.org/10.1038/ncomms12574, 2016.
Li, M., Zhang, Q., Kurokawa, J.-I., Woo, J.-H., He, K., Lu, Z., Ohara, T., Song, Y., Streets, D. G., Carmichael, G. R., Cheng, Y., Hong, C., Huo, H., Jiang, X., Kang, S., Liu, F., Su, H., and Zheng, B.: MIX: a mosaic Asian anthropogenic emission inventory under the international collaboration framework of the MICS-Asia and HTAP, Atmos. Chem. Phys., 17, 935–963, https://doi.org/10.5194/acp-17-935-2017, 2017.
Liu, T.-Y.: Spatial structure convergence of China's transportation system, Res. Transp. Econ., 78, 100768, https://doi.org/10.1016/j.retrec.2019.100768, 2019.
Liu, Y., Sato, Y., Jia, R., Xie, Y., Huang, J., and Nakajima, T.: Modeling study on the transport of summer dust and anthropogenic aerosols over the Tibetan Plateau, Atmos. Chem. Phys., 15, 12581–12594, https://doi.org/10.5194/acp-15-12581-2015, 2015.
Liu, Y., Zhu, Q., Huang, J., Hua, S., and Jia, R.: Impact of dust-polluted convective clouds over the Tibetan Plateau on downstream precipitation, Atmos. Environ., 209, 67–77, https://doi.org/10.1016/j.atmosenv.2019.04.001, 2019.
Liu, Y., Zhu, Q., Hua, S., Alam, K., and Cheng, Y.,: Tibetan Plateau driven impact of Taklimakan dust on northern rainfall, Atmos. Environ., 234, 117583, https://doi.org/10.1016/j.atmosenv.2020.117583, 2020a.
Liu, Y., Li, Y., Huang, J., Zhu, Q., and Wang, S.: Attribution of the Tibetan Plateau to Northern Drought, Natl. Sci. Rev., 7, 489–492, https://doi.org/10.1093/nsr/nwz191, 2020b.
Liu, H., Wang, Q., Xing, L., Zhang, Y., Zhang, T., Ran, W., and Cao, J.: Measurement report: quantifying source contribution of fossil fuels and biomass-burning black carbon aerosol in the southeastern margin of the Tibetan Plateau [Data set], Zenodo, https://doi.org/10.5281/zenodo.4452056, 2021.
Luo, M., Liu, Y., Zhu, Q., Tang, Y., and Alam, K.: Role and mechanisms of black carbon affecting water vapor transport to Tibet, Remote Sens., 12, 231, https://doi.org/10.3390/rs12020231, 2020.
Lough, G. C., Schauer, J. J., Park, J. S., Shafer, M. M., and Weinstein, J. P.: Emissions of metals associated with motor vehicle roadways, Environ. Sci. Technol., 39, 826–836, https://doi.org/10.1021/es048715f, 2005.
Ma, Y., Huang, C., Jabbour, H., Zheng, Z., Wang, Y., Jiang, Y., Zhu, W., Ge, X., Collier, S., and Zheng, J.: Mixing state and light absorption enhancement of black carbon aerosols in summertime Nanjing, China, Atmos. Environ., 222, 117141, https://doi.org/10.1016/j.atmosenv.2019.117141, 2020.
Madala, S., Satyanarayana, A. N. V., and Rao, T. N.: Performance evaluation of PBL and cumulus parameterization schemes of WRF ARW model in simulating severe thunderstorm events over Gadanki MST radar facility – Case study, Atmos. Res., 139, 1–17, https://doi.org/10.1016/j.atmosres.2013.12.017, 2014.
Ming, J., Xiao, C., Cachier, H., Qin, D., Qin, X., Li, Z., and Pu, J.: Black Carbon (BC) in the snow of glaciers in west China and its potential effects on albedos, Atmos. Res., 92, 114–123, https://doi.org/10.1016/j.atmosres.2008.09.007, 2009.
Niu, H., Kang, S., and Zhang, Y.: Distribution of light-absorbing impurities in snow of glacier on Mt. Yulong, southeastern Tibetan Plateau, Atmos. Res., 197, 474–484, https://doi.org/10.1016/j.atmosres.2017.07.004, 2017.
Panicker, A. S., Pandithurai, G., Safai, P. D., Dipu, S., and Lee, D.-I.: On the contribution of black carbon to the composite aerosol radiative forcing over an urban environment, Atmos. Environ., 44, 3066–3070, https://doi.org/10.1016/j.atmosenv.2010.04.047, 2010.
Polissar, A. V., Hopke, P. K., Paatero, P., Kaufmann, Y. J., Hall, D. K., Bodhaine, B. A., Dutton, E. G., and Harris, J. M.: The aerosol at Barrow, Alaska: long-term trends and source locations, Atmos. Environ., 33, 2441–2458, https://doi.org/10.1016/S1352-2310(98)00423-3, 1999.
Polissar, A. V., Hopke, P. K., and Harris, J. M.: Source regions for atmospheric aerosol measured at Barrow, Alaska, Environ. Sci. Technol., 35, 4214–4226, https://doi.org/10.1021/es0107529, 2001.
Ramachandran, S. and Kedia, S.: Black carbon aerosols over an urban region: Radiative forcing and climate impact, J. Geophys. Res., 115, D10202, https://doi.org/10.1029/2009jd013560, 2010.
Rajesh, T. A. and Ramachandran, S.: Black carbon aerosols over urban and high altitude remote regions: Characteristics and radiative implications, Atmos. Environ., 194, 110–122, https://doi.org/10.1016/j.atmosenv.2018.09.023, 2018.
Ricchiazzi, P., Yang, S., Gautier, C., and Sowle, D.: SBDART: A research and teaching software tool for plane-parallel radiative transfer in the earth's atmosphere, B. Am. Meteorol. Soc., 79, 2101–2114, 1998.
Sandradewi, J., Prévôt, A. S. H., Weingartner, E., Schmidhauser, R., Gysel, M., and Baltensperger, U.: A study of wood burning and traffic aerosols in an Alpine valley using a multi-wavelength Aethalometer, Atmos. Environ., 42, 101–112, https://doi.org/10.1016/j.atmosenv.2007.09.034, 2008.
Song, Y., Zhang, Y., Xie, S., Zeng, L., Zheng, M., Salmon, L. G., Shao, M., and Slanina, S.: Source apportionment of PM2.5 in Beijing by positive matrix factorization, Atmos. Environ., 40, 1526–1537, https://doi.org/10.1016/j.atmosenv.2005.10.039, 2006.
Srivastava, A. K., Ram, K., Pant, P., Hedge, P., and Hema, J.: Black carbon aerosols over Manora Peak in the Indian Himalayan foothills: implications for climate forcing, Environ. Res. Lett., 7, 014002, https://doi.org/10.1088/1748-9326/7/1/014002, 2011.
Sun, J., Zhi, G., Hitzenberger, R., Chen, Y., Tian, C., Zhang, Y., Feng, Y., Cheng, M., Zhang, Y., Cai, J., Chen, F., Qiu, Y., Jiang, Z., Li, J., Zhang, G., and Mo, Y.: Emission factors and light absorption properties of brown carbon from household coal combustion in China, Atmos. Chem. Phys., 17, 4769–4780, https://doi.org/10.5194/acp-17-4769-2017, 2017.
Tian, J., Wang, Q., Ni, H., Wang, M., Zhou, Y., Han, Y., Shen, Z., Pongpiachan, S., Zhang, N., Zhao, Z., Zhang, Q., Zhang, Y., Long, X., and Cao, J.: Emission characteristics of primary brown carbon absorption from biomass and coal burning: Development of an optical emission inventory for China, J. Geophys. Res.-Atmos., 124, 1879–1893, https://doi.org/10.1029/2018jd029352, 2019.
Urban, R. C., Lima-Souza, M., Caetano-Silva, L., Queiroz, M. E. C., Nogueira, R. F. P., Allen, A. G., Cardoso, A. A., Held, G., and Campos, M. L. A. M.: Use of levoglucosan, potassium, and water-soluble organic carbon to characterize the origins of biomass-burning aerosols, Atmos. Environ., 61, 562–569, https://doi.org/10.1016/j.atmosenv.2012.07.082, 2012.
Valenzuela, A., Olmo, F. J., Lyamani, H., Antón, M., Titos, G., Cazorla, A., and Alados-Arboledas, L.: Aerosol scattering and absorption Angström exponents as indicators of dust and dust-free days over Granada (Spain), Atmos. Res., 154, 1–13, https://doi.org/10.1016/j.atmosres.2014.10.015, 2015.
Vignati, E., Karl, M., Krol, M., Wilson, J., Stier, P., and Cavalli, F.: Sources of uncertainties in modelling black carbon at the global scale, Atmos. Chem. Phys., 10, 2595–2611, https://doi.org/10.5194/acp-10-2595-2010, 2010.
Wang, Q., Cao, J., Han, Y., Tian, J., Zhu, C., Zhang, Y., Zhang, N., Shen, Z., Ni, H., Zhao, S., and Wu, J.: Sources and physicochemical characteristics of black carbon aerosol from the southeastern Tibetan Plateau: internal mixing enhances light absorption, Atmos. Chem. Phys., 18, 4639–4656, https://doi.org/10.5194/acp-18-4639-2018, 2018.
Wang, Q., Han, Y., Ye, J., Liu, S., Pongpiachan, S., Zhang, N., Han, Y., Tian, J., Wu, C., Long, X., Zhang, Q., Zhang, W., Zhao, Z., and Cao, J.: High contribution of secondary brown carbon to aerosol light absorption in the southeastern margin of Tibetan Plateau, Geophys. Res. Lett., 46, 4962–4970, https://doi.org/10.1029/2019gl082731, 2019a.
Wang, Q. Y., Huang, R.-J., Cao, J. J., Tie, X. X., Ni, H. Y., Zhou, Y. Q., Han, Y. M., Hu, T. F., Zhu, C. S., Feng, T., Li, N., and Li, J. D.: Black carbon aerosol in winter northeastern Qinghai–Tibetan Plateau, China: the source, mixing state and optical property, Atmos. Chem. Phys., 15, 13059–13069, https://doi.org/10.5194/acp-15-13059-2015, 2015.
Wiedinmyer, C., Akagi, S. K., Yokelson, R. J., Emmons, L. K., Al-Saadi, J. A., Orlando, J. J., and Soja, A. J.: The Fire INventory from NCAR (FINN): a high resolution global model to estimate the emissions from open burning, Geosci. Model Dev., 4, 625–641, https://doi.org/10.5194/gmd-4-625-2011, 2011.
Xing, L., Li, G., Pongpiachan, S., Wang, Q., Han, Y., Cao, J., Tipmanee, D., Palakun, J., Aukkaravittayapun, S., Surapipith, V., and Poshyachinda, S.: Quantifying the contributions of local emissions and regional transport to elemental carbon in Thailand, Environ. Pollut., 262, 114272, https://doi.org/10.1016/j.envpol.2020.114272, 2020.
Xia, X., Zong, X, Cong, Z., Chen, H., Kang, S., and Wang, P.: Baseline continental aerosol over the central Tibetan plateau and a case study of aerosol transport from South Asia, Atmos. Environ., 45, 7370–7378, https://doi.org/10.1016/j.atmosenv.2011.07.067, 2011.
Xu, J. Z., Zhang, Q., Wang, Z. B., Yu, G. M., Ge, X. L., and Qin, X.: Chemical composition and size distribution of summertime PM2.5 at a high altitude remote location in the northeast of the Qinghai–Xizang (Tibet) Plateau: insights into aerosol sources and processing in free troposphere, Atmos. Chem. Phys., 15, 5069–5081, https://doi.org/10.5194/acp-15-5069-2015, 2015.
Zhang, R., Wang, H., Qian, Y., Rasch, P. J., Easter, R. C., Ma, P.-L., Singh, B., Huang, J., and Fu, Q.: Quantifying sources, transport, deposition, and radiative forcing of black carbon over the Himalayas and Tibetan Plateau, Atmos. Chem. Phys., 15, 6205–6223, https://doi.org/10.5194/acp-15-6205-2015, 2015.
Zhang, Y., Li, M., Cheng, Y., Geng, G., Hong, C., Li, H., Li, X., Tong, D., Wu, N., Zhang, X., Zheng, B., Zheng, Y., Bo, Y., Su, H., and Zhang, Q.: Modeling the aging process of black carbon during atmospheric transport using a new approach: a case study in Beijing, Atmos. Chem. Phys., 19, 9663–9680, https://doi.org/10.5194/acp-19-9663-2019, 2019.
Zhao, Z., Cao, J., Chow, J. C., Watson, J. G., Chen, A. L. W., Wang, X., Wang, Q., Tian, J., Shen, Z., Zhu, C., Liu, S., Tao, J., Ye, Z., Zhang, T., Zhou, J., and Tian, R.: Multi-wavelength light absorption of black and brown carbon at a high-altitude site on the Southeastern margin of the Tibetan Plateau, China, Atmos. Environ., 212, 54–64, https://doi.org/10.1016/j.atmosenv.2019.05.035, 2019.
Zhu, C. S., Cao, J. J., Hu, T. F., Shen, Z. X., Tie, X. X., Huang, H., Wang, Q. Y., Huang, R. J., Zhao, Z. Z., Mocnik, G., and Hansen, A. D. A.: Spectral dependence of aerosol light absorption at an urban and a remote site over the Tibetan Plateau, Sci. Total Environ., 590-591, 14–21, https://doi.org/10.1016/j.scitotenv.2017.03.057, 2017.
Zotter, P., Herich, H., Gysel, M., El-Haddad, I., Zhang, Y., Močnik, G., Hüglin, C., Baltensperger, U., Szidat, S., and Prévôt, A. S. H.: Evaluation of the absorption Ångström exponents for traffic and wood burning in the Aethalometer-based source apportionment using radiocarbon measurements of ambient aerosol, Atmos. Chem. Phys., 17, 4229–4249, https://doi.org/10.5194/acp-17-4229-2017, 2017.