the Creative Commons Attribution 4.0 License.
the Creative Commons Attribution 4.0 License.
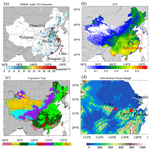
Analysis of CO2 spatio-temporal variations in China using a weather–biosphere online coupled model
Yujun Jiang
Xiao-Ming Hu
Qianli Ma
Jingjiao Pu
Guangqiang Zhou
The dynamics of atmospheric CO2 has received considerable attention in the literature, yet significant uncertainties remain within the estimates of contribution from the terrestrial flux and the influence of atmospheric mixing. In this study we apply the WRF-Chem model configured with the Vegetation Photosynthesis and Respiration Model (VPRM) option for biomass fluxes in China to characterize the dynamics of CO2 in the atmosphere. The online coupled WRF-Chem model is able to simulate biosphere processes (photosynthetic uptake and ecosystem respiration) and meteorology in one coordinate system. We apply WRF-Chem for a multi-year simulation (2016–2018) with integrated data from a satellite product, flask samplings, and tower measurements to diagnose the spatio-temporal variations of CO2 fluxes and concentrations in China. We find that the spatial distribution of CO2 was dominated by anthropogenic emissions, while its seasonality (with maxima in April 15 ppmv higher than minima in August) was dominated by the terrestrial flux and background CO2. Observations and simulations revealed a consistent increasing trend in column-averaged CO2 (XCO2) of 2.46 ppmv (0.6 % yr−1) resulting from anthropogenic emission growth and biosphere uptake. WRF-Chem successfully reproduced ground-based measurements of surface CO2 concentration with a mean bias of −0.79 ppmv and satellite-derived XCO2 with a mean bias of 0.76 ppmv. The model-simulated seasonality was also consistent with observations, with correlation coefficients of 0.90 and 0.89 for ground-based measurements and satellite data, respectively. Tower observations from a background site at Lin'an (30.30∘ N, 119.75∘ E) revealed a strong correlation (−0.98) between vertical CO2 and temperature gradients, suggesting a significant influence of boundary layer thermal structure on the accumulation and depletion of atmospheric CO2.
- Article
(5307 KB) - Full-text XML
-
Supplement
(1128 KB) - BibTeX
- EndNote
Climate research requires accurate characterization of atmospheric CO2, which is closely affected by both atmospheric transport and terrestrial sources and sinks (Bauska et al., 2015; Keenan et al., 2016). Our current knowledge largely comes from interpreting ground- or space-based measurements and model simulations. While observation is limited by spatial and temporal coverage, modelling approaches also suffer from various uncertainties (Shi et al., 2018). Modelling assessment of CO2 is usually conducted through two methods: first, process- or data-driven biosphere models in which terrestrial fluxes are diagnostically calculated with theoretical functions (Tian et al., 2015) or determined through semi-empirical relationships derived from ground measurements and/or satellite products with machine learning techniques (Papale and Valentini, 2003); second, inverse modelling in which prior flux estimates applied in atmospheric transport models are adjusted by observational data and/or satellite products to determine the posterior flux (Peylin et al., 2002; Kountouris et al., 2018). Process-driven biosphere models have difficulties capturing spatial and temporal variabilities at fine resolution because parameters calibrated from a limited number of site observations are applied across a variety of land covers (Todd-Brown et al., 2013). Atmospheric inverse modelling is predominantly affected by the presumed prior flux, and different assimilation techniques can give different and even conflicting results (Peylin et al., 2013). These fundamental features highlight the limits of these approaches for accurately modelling carbon dynamics.
Researchers have attempted to reconcile differences between bottom-up biosphere models and top-down atmospheric inverse models, and recent studies have demonstrated increasing levels of agreement owing to improved understanding of both approaches, such as better parameterization of biosphere processes (Dayalu et al., 2018), more accurately constrained estimates of prior flux (Crowell et al., 2018; Feng et al., 2019), and advanced measurement and satellite instruments that provide high-quality data for assimilation (Gaubert et al., 2019); however, critical model disagreements still persist (Kondo et al., 2020). To bridge the gap between the terrestrial flux and atmospheric mixing, a type of weather–biosphere coupled model (Ahmadov et al., 2007; Mahadevan et al., 2008) has been developed to simulate biosphere processes and meteorology conditions in one coordinate system, allowing their interactions to be properly addressed. Previous modelling studies (Ahmadov et al., 2009; Kretschmer et al., 2012; Park et al., 2018, 2020; Beck et al., 2013; Pillai et al., 2012) have demonstrated the weather–biosphere coupled model can successfully capture the mesoscale CO2 transport at regional and local scales with significant improvements. But whether it can reproduce the long-term variations and subsequently estimate carbon fluxes at regional scales with high confidence remains a crucial issue to be addressed.
Understanding the spatio-temporal characteristics of atmospheric CO2 is a key priority in China because of the central role it plays in regulating the climate and environment. In recent years, tremendous efforts have been made in China to control anthropogenic emissions from fossil fuel combustion for both air quality and climate mitigation purposes (Zheng et al., 2018). While the sources and sinks of air pollutants have been thoroughly examined and well documented (Huang et al., 2020), the dynamics of CO2 at regional to national scales remains poorly understood due to a lack of long-term observations and limited modelling studies (Han et al., 2020). Li et al. (2020) applied a weather–biosphere model with tower observations to analyse CO2 fluxes and concentrations over mixed forest and rice paddy areas in northeast China, but the 1-year simulation limits the attempt to investigate interannual CO2 variation, which is subject to substantial change (Z. Fu et al., 2019). Wang et al. (2019) applied satellite products and in situ observations with inverse modelling to derive posterior carbon fluxes and reported 100 % uncertainty for constraining the global terrestrial flux. Fu et al. (2019) applied the GEOS-Chem simulation with the offline Carbon Tracker (Peters et al., 2007) as input to estimate the impacts of the terrestrial flux and anthropogenic emissions on the annual variation of CO2 concentrations, but regional-scale assessment was limited by the coarse grid resolution (2∘ × 2.5∘). A machine learning technique has also been employed to upscale site observations to the regional scale (Yao et al., 2018; Zhu et al., 2014), but the estimations of carbon budget and dynamics retain large uncertainty due to the diversity of biomass among sites and the coarse grid resolution. These pilot studies have shed light on improving the understanding of spatio-temporal characteristics of CO2 in China with modelling or observational methods, but an integrated investigation with both modelling and observations at a fine scale is urgently needed to expand diagnostic understanding of localized and regional transport, flux, and concentration of CO2 to inform emission management and climate adaption policies (Y. Fu et al., 2019; Niu et al., 2017; Wang et al., 2019).
In this study we use the WRF-Chem model configured with the Vegetation Photosynthesis and Respiration Model (VPRM) option (Hu et al., 2020; Mahadevan et al., 2008) to simulate and characterize the spatio-temporal variation of atmospheric CO2 in China from 2016–2018 and also to validate this weather–biosphere model with recent advanced satellite and tower observations. WRF-Chem has been applied in a few case studies over the United States (Hu et al., 2020), Europe (Kretschmer et al., 2012), northeast China (Li et al., 2020), and South Korea (Park et al., 2020); this study attempts to apply and evaluate it for a multi-year simulation over China. We first describe the modelling methods and data followed by model validation against observations from multiple datasets and then present the spatio-temporal variations and estimates of contributions from anthropogenic emissions, the terrestrial flux, and background concentrations. Finally, we investigate tower data and reveal the boundary layer thermal structure impacts on atmospheric CO2 accumulation and depletion.
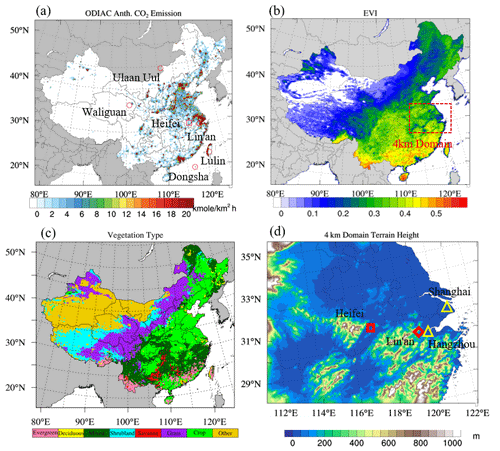
Figure 1Annual averages of (a) ODIAC emission, (b) MODIS EVI, and (c) dominant vegetation type in the 20 km simulation domain and (d) terrain height of the 4 km simulation domain. The locations of the ESRL sites, TCCON Hefei site, and Lin'an tower site are indicated with red circles, rectangles, and diamonds, respectively in (a). The 4 km domain is indicated with the dashed red rectangle in (b), and the locations of Hangzhou and Shanghai are indicated with yellow triangles in (d).
We conduct nested WRF-Chem (Version 3.9.1.1) simulations over China (domain shown in Fig. 1a) and the Yangtze River Delta (YRD) region (domain shown in Fig. 1d) at 20 and 4 km grid resolution, respectively. Both simulations were configured with 47 vertical layers with model tops at 10 hPa. Model configuration in this study followed the work by Hu et al. (2020) and Li et al. (2020). We applied the YSU planetary boundary layer (PBL) scheme (Hong et al., 2006), Morrison microphysics (Morrison et al., 2009), Duhia short-wave radiation (Dudhia, 1989), RRTM long-wave radiation (Mlawer et al., 1997), Grell-3 cumulus scheme (Grell and Devenyi, 2002), and Noah land-surface scheme (Chen and Dudhia, 2001), with more details summarized in Table S1 in the Supplement. In general, the 4 km grid simulation showed no significant difference as compared to the 20 km grid simulation (demonstrated in Figs. S1 and S2 in the Supplement); thus the 20 km grid simulation was used to characterize the spatio-temporal distributions of CO2 over China, and the 4 km grid simulation was only used for comparison with tower data collected at a background site in YRD. Discussions in the next section will mostly refer to the 20 km grid simulation unless otherwise specified. Initial and lateral boundary conditions for the 20 km grid simulations were derived from the mole fraction product of CarbonTracker (Peters et al., 2007) with 3∘ × 2∘ resolution. The latest update of column-averaged CO2 (XCO2) concentration assimilation product from CarbonTracker (CT2019) with 1∘ × 1∘ resolution (Jacobson, 2020) was also employed for comparison with the WRF-Chem simulation. The anthropogenic emission inventory is the Open-Data Inventory for Anthropogenic Carbon dioxide (ODIAC) with 0.1∘ × 0.1∘ resolution (Oda et al., 2018), shown in Fig. 1a. ODIAC has been widely applied in recent modelling studies and demonstrated good agreement with other global inventories (Hedelius et al., 2017; Hu et al., 2020). The ocean flux is from climatology estimation (Takahashi et al., 2009); and vegetation fractions and enhanced vegetation index (EVI; shown in Fig. 1b) are from MODIS (Huete et al., 2002). CO2 from initial and boundary conditions, anthropogenic emission, and the terrestrial biogenic flux was tagged as BCG, ANT, and BIO, respectively, to allow the contributions from each process to be identified and quantified through one simulation.
WRF-Chem calculates ecosystem respiration (ER) and gross ecosystem exchange (GEE) with the following functions as
where T is the air temperature at 2 (T2); α, β, and λ are vegetation-type-dependent parameters; PAR0 is the vegetation-type-dependent half-saturation value of photosynthetically active radiation (PAR); and Tscale, Wscale, and Pscale are scaling factors for temperature, water stress, and phenology, respectively. In this study we take the atmosphere as a reference; thus GEE has a negative sign and ER has a positive sign. The current version of WRF-Chem is parameterized (α, β, λ) for seven vegetation types (Fig. 1c): crops, mixed forest, evergreen forest, deciduous forest, shrub, savanna, and grass. For each modelling grid, ER and GEE are calculated as the weighted averages of each vegetation type based on their fractional abundance. Recent studies (Hu et al., 2020; Li et al., 2020) have investigated the uncertainty associated with this parameterization through sensitivity simulations and suggested the crops can be further divided into subcategories based on eddy-covariance (EC) tower measurements to improve the model. In this study we used the default parameterization (values presented in Table S2), which has been demonstrated to successfully reproduce the terrestrial flux over northeast China (Li et al., 2020). In contrast, CT2019 applies a process-based biosphere model, the Carnegie-Ames-Stanford Approach (CASA; Zhou et al., 2020), driven by year-specific weather and satellite data to simulate terrestrial fluxes (Peters et al., 2007). CASA also estimates photosynthetic uptake based on solar radiation and plant phenology and estimates respiration as a function of T2. CASA directly simulates monthly means of net primary production (NPP) and heterotrophic respiration (RH). NPP is the difference between photosynthetic uptake (equivalent to GEE) and autotrophic respiration (RA). The summary of RH and RA is equivalent to ER. Thus, WRF-Chem and CASA are essentially very similar in terms of considering methodology impact; however, it should be noted that to resolve CASA simulated NPP into GEE and RA, CT2019 applies the assumption that GEE is twice that of NPP, which implies that for the same plants the photosynthetic carbon uptake is double the magnitude of autotrophic respiration (but of opposite sign). This assumption is applicable at monthly scale but may have difficulty to reproduce the rapid changes at hourly and daily scales due to impact from weather systems, which will be demonstrated with more details in Sect. 3.2.
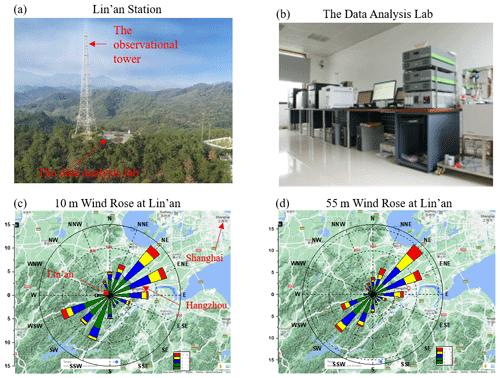
Figure 2Photos of the (a) Lin'an regional atmospheric background station and (b) the data analysis lab. Wind rose map at Lin'an derived from wind speed and wind direction observations for 2016–2018 at (c) 10 m and (d) 50 m.
Hourly measurements of CO2 concentrations were collected at the Lin'an Regional Atmospheric Background Station (30.30∘ N, 119.75∘ E, surroundings shown in Fig. 2a) with Picarro G1301 and G1302 trace gas analysers mounted on an observation tower at 21 and 55 (above ground level), respectively, and analysed online (data analysis lab shown in Fig. 2b). The station is located in the remote area of Hangzhou 138.6 in the middle of a hilly area covered by mixed forest. The observation tower is 60 km to the west of the downtown centre of Hangzhou and 195 km to the southwest of Shanghai. Figure 2c and d present the wind rose map at Lin'an derived from hourly observations of 10 and 55 m wind, respectively, which clearly shows the northeast and southwest as prevailing wind directions. The station can properly represent the background atmospheric environment in YRD as demonstrated in previous studies (Deng et al., 2018; Pu et al., 2020). The tower data provide a representative sampling of CO2 gradients resulting from the exchange between atmosphere mixing and the terrestrial flux.
Atmospheric samples near the surface were collected at monthly intervals and analysed for CO2 through the National Oceanic and Atmospheric Administration (NOAA) Earth System Research Laboratory (ESRL) at four sites (locations shown in Fig. 1a) within our study domain, including Dongsha Island (DSI; 20.69∘ N, 116.73∘ E), Lulin (LLN; 23.47∘ N, 120.87∘ E), Ulaan Uul (UUM; 44.45∘ N, 111.09∘ E), and Mt. Waliguan (WLG; 36.29∘ N, 100.89∘ E). The Orbiting Carbon Observatory-2 (OCO-2) satellite product (Kiel et al., 2019) with daily intervals was employed to validate simulation of column-averaged CO2 (XCO2) concentrations. A total of 204 940 OCO-2 version 9 swath data covering the simulation period were used in this study. Daily ground-based Fourier transform spectrometer (FTS)-measured XCO2 data at Hefei site (31.90∘ N, 117.17∘ E) were also collected through the Total Carbon Column Observing Network (TCCON) for the year 2016 (Wang et al., 2017). The TCCON Hefei site was located in the northwestern rural area of the city of Hefei, and measurements were conducted from September 2015 to December 2016 (Wang et al., 2017). WRF has been evaluated extensively and consistently performs well for reproducing the meteorology fields and the transport of atmospheric tracers in China (Gao et al., 2015; Tang et al., 2016; Wang et al., 2017; Yang et al., 2019), so this study will only present the simulation performance for CO2, which has not been thoroughly discussed in the literature.
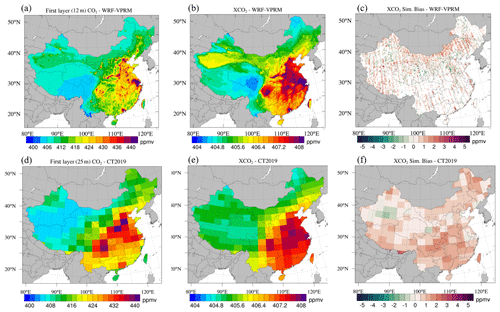
Figure 32016–2018 averages of WRF-Chem simulations of (a) first-layer (mid-level height is 12 km) CO2 concentration and (b) XCO2 concentration. (c) WRF-Chem-simulated XCO2 bias against OCO-2. Panels (d)–(f) are the same as (a)–(c) but for CT2019 (first-layer mid-level height is 25 m).
3.1 Model evaluation
We first evaluate the capability of WRF-Chem to reproduce concentrations of surface CO2 and XCO2, and we find fairly good model performance through the comparison with satellite and ground-based observations. The WRF-Chem-simulated surface layer (mid-level height a.g.l. is 12 m) CO2 and XCO2 averages between 2016–2018 are demonstrated in Fig. 3a and b, respectively. High concentrations were found over industrial areas such as the North China Plain (NCP), Pearl River Delta (PRD), and Yangtze River Delta (YRD), where the surface CO2 and XCO2 were above 440 and 408 ppmv, respectively; the domain averages were 411 and 406 ppmv, respectively. While most climate models assume evenly distributed CO2 (Fung et al., 1983; Kiehl and Ramanathan, 1983), our data demonstrate a prominent gradient between industrial and remote areas (e.g., Tibetan Plateau, Mongolia), especially for surface CO2, which could be an important source of uncertainty for estimating the long-wave radiation effect (Xie et al., 2018). Spatial patterns of CO2 and XCO2 were in close agreement with ODIAC, indicating the dominant impact of anthropogenic emission in determining the CO2 distribution. WRF-Chem-simulated CO2 was generally consistent with CT2019 (Fig. 3c), but CT2019 estimated near-surface CO2 (mid-level height a.g.l. is 25 m) over the coastal industrial areas YRD and PRD because the ocean module used in CT2019 estimated stronger air–sea exchange than the ocean flux by Takahashi et al. (2009) used in WRF-Chem. The two models showed better agreement for XCO2 (Fig. 3b and e) but also differed by ∼ 1 ppmv over Taklamakan Desert and along the eastern side of the Tibetan Plateau. The OCO-2 swath data were integrated into the corresponding horizontal grids of WRF-Chem and CT2019, respectively, to validate XCO2. Biases of WRF-Chem and CT2019 both fall into the range of ± 3 ppmv as shown in Fig. 3c and f, respectively, but WRF-Chem evidently provided more details of the spatial gradient. WRF-Chem showed well-mixed underestimations and overestimations along neighbouring satellite tracks, while CT2019 tended to overestimate (underestimate) over the Tibetan Plateau (Taklamakan Desert) where WRF-Chem gave slightly smaller biases. Figure 4a and b present the raw data pairs between models and OCO-2 with daily intervals for WRF-Chem and CT2019, respectively. In general, the WRF-Chem model reproduced OCO-2 well, with a mean bias (MB) of 0.76 ppmv, and CT2019 showed a MB of 0.54 ppmv, suggesting an overall acceptable performance of the weather–biosphere model to simulate the spatial distribution pattern of XCO2 in China.
We further analyse WRF-Chem validation against OCO-2 for the seven vegetation types in each season and find no prominent difference (evaluation statistics summarized in Table 1). Regarding vegetation type, the model showed the largest MB over deciduous forest of −1.01 and 1.27 ppmv in summer and winter, respectively, which only covered a very small portion in northeast China. The three most abundant coverage vegetation types in China are grass, crops, and mixed forest. XCO2 simulated by WRF-Chem over grass areas was slightly overestimated by 0.31 ∼ 0.68 ppmv throughout the year, and the MB over mixed forest was −0.43 ∼ 0.59 ppmv, indicating a good performance of the model over the vast majority of areas of China. Performance over crops generally showed a larger discrepancy than other vegetation types, with the MB ranging from 0.66 ppmv in summer to 1.19 ppmv in winter, suggesting the model tends to slightly overestimate column concentration of CO2 over cropland. Li et al. (2020) reported that WRF-Chem underestimated biosphere carbon over rice paddy sites (by ∼ 3 %) in northeast China and suggested the parameterization of α, β, and λ as the most important cause. Cropland differs significantly across China, with various types of species such as rice, wheat, and corn, for which literature reported substantially different rates of ecosystem respiration and photolysis uptake (Gao et al., 2018; Yang et al., 2016; Zhu et al., 2020). Thus, applying one set of parameters to represent all crops may be responsible for the lingering uncertainty of simulated XCO2. In terms of seasonal difference, WRF-VPRM showed a slightly smaller bias in summer and a larger bias in winter, and the correlation coefficients were all ∼ 0.8, consistent with application over the United States (Hu et al., 2020), which also reported slightly better performance in summer than other seasons.
Figure 4 also presents the overall simulation bias against ground-based observations at their raw temporal intervals (monthly for data at ESRL sites, hourly for tower data at Lin'an, and daily for TCCON at Hefei). At the ESRL sites (Fig. 4c), surface CO2 concentrations were simulated well with minor overestimation by 0.69 ppmv. Evaluation at the Lin'an station was performed with the 4 km grid simulation. The mid-level heights of WRF-Chem's first, second, and third layers were 12.3, 36.9, and 61.6 m, respectively, and simulations were linearly interpolated to 21 and 55 m for comparison with the tower data. The evaluation at 21 (Fig. 4d) shows slight overestimation by 0.02 ppmv, but the evaluation at 55 m height (Fig. 4e) shows relatively large overestimations by 1.06 ppmv. The discrepancy is likely due to the combined effect of vertical allocation of anthropogenic emission (Brunner et al., 2019) and parameterization of VPRM. Tracer transport models (such as WRF-Chem and CASA) and inverse modelling methods allocate anthropogenic CO2 emission to the near-surface layer due to lack of injection height information, which may subsequently lead to systematic overestimation of surface CO2 concentration in industrial areas. Through a regional-scale (750 km × 650 km) modelling study around the city of Berlin, Brunner et al. (2019) reported that distributing anthropogenic emission into the surface layer caused near-surface CO2 concentration to be overestimated by 14 % in summer and 43 % in winter as compared with considering the vertical profiles of local anthropogenic sources. Lin'an observation tower is located in a densely vegetated area. Validation against OCO-2 suggested that WRF-Chem did not show significantly different performance over different vegetation types as shown in Table 1. As compared to the ESRL background sites which were located in more remote areas with little anthropogenic emission (Fig. 1a), Lin'an was more frequently affected by regional anthropogenic emissions, which were transported from Shanghai and Hangzhou due to the prevailing northeast wind (Pu et al., 2014), indicating that the emission allocation discrepancy may induce a more prominent error at Lin'an. In fact, the 20 km grid WRF-Chem simulation bias at Lin'an was 5.34 and 5.41 ppmv at 21 and at 55 m, respectively (Fig. S2), significantly larger than the bias at ESRL sites. In addition, both the 20 km grid and 4 km grid simulations showed relatively larger bias at 55 m than 21 m due to smaller topography roughness and higher wind speed, which increases with height according to observations (Fig. S3). CT2019 also substantially overestimated at Lin'an, but the first, second, and third layers' mid-level heights are 25, 103, and 247 m, respectively, so we do not present a direct comparison with the tower data. Simulated XCO2 from both WRF-Chem and CT2019 was consistent with the TCCON Hefei site observations as shown in Fig. 4f, with a MB of −0.79 and −0.78 ppmv, respectively, and a normalized mean bias (NMB) of −0.20 % and −0.19 %, respectively. The 4 km grid simulation showed a very similar result to the 20 km grid simulation for XCO2 (Figs. S1 and S2). Recent atmospheric inverse modelling studies (Y. Fu et al., 2019; Wang et al., 2019; Xie et al., 2018) reported the simulation bias of XCO2 as 0.5–2 ppmv with posterior flux inputs. The WRF-Chem model applied in this study has demonstrated good agreement with the observations though our evaluation.
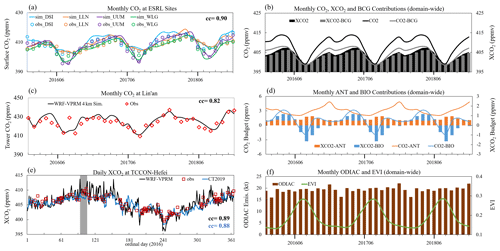
Figure 5Monthly variations of (a) CO2 at ESRL sites, (b) total (black) and background (BCG, grey) CO2 (line) and XCO2 (area and bar), (c) CO2 at Lin'an station (averaged for daytime 21 and 55 m data). (d) Contributions from anthropogenic (ANT, orange) and biogenic (BIO, blue) for CO2 (lines) and XCO2 (bars); (f) ODIAC emission and MODIS EVI; and (e) daily variation of XCO2 at the TCCON Hefei site.
3.2 CO2 seasonal variation and trend in China
We next analyse the seasonality of CO2 and XCO2 and find that the terrestrial flux played a more influential role than anthropogenic emission. WRF-Chem successfully reproduced seasonal variations of CO2 at ESRL sites, with a correlation coefficient of 0.90 (Fig. 5a). The WRF-Chem 4 km grid simulation showed a correlation coefficient of 0.82 with the Lin'an tower observation (averaged for daytime 21 and 55 m data). Both the model and measurements showed prominent seasonal cycles for surface CO2 concentrations. The WRF-Chem simulation showed maxima in April (413–419 ppmv) and minima in August (399–404 ppmv) as presented in Fig. 5b. The model suggested that the anthropogenic CO2 contribution was 2.6 ppmv in both months, while the biogenic contributions were 3.1 and −1.2 ppmv in April and August, respectively (Fig. 5d). Anthropogenic emission (Fig. 5f) showed a flat curve with relatively higher values in December due to fuel combustion for heating (Zheng et al., 2018). EVI showed maxima in July and August (Fig. 5f). During summer, photosynthetic uptake almost completely compensated for anthropogenic emission, causing the minimum CO2 concentration to be observed in August, while the higher anthropogenic emission in December and respiration flux in April led to the two corresponding peaks. The anthropogenic XCO2 contributions were 0.5 and 0.6 ppmv in April and August, respectively, and the biogenic contributions were 0.8 and −1.5 ppmv, respectively, suggesting that the seasonality of XCO2 was also primarily dominated by the terrestrial flux. Furthermore, the seasonality at high-latitude ESRL sites (UUM and WLG) was stronger than at Lin'an and low-latitude sites (DSI and LLN) because of the larger temperature and photosynthetically active radiation (PAR) gradients. Annual average anthropogenic and biogenic XCO2 contributions were 7.1 and −1.9 ppmv, respectively, indicating that biosphere uptake was an important carbon sink offsetting 27 % of anthropogenic emission and slowing the growth of atmospheric CO2.
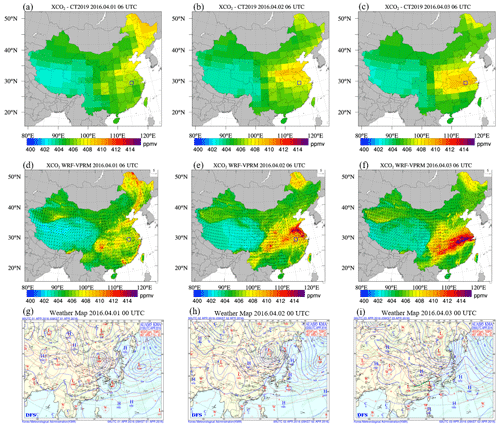
Figure 6Daily XCO2 from CT2019 (a–c) and WRF-Chem (d–f). Weather map from the Korea Meteorological Administration (g–i). The blue box represents the location of Hefei.
Table 1Evaluation statisticsa for WRF-Chem 20 km grid simulation against the OCO-2 satellite product at daily intervals.
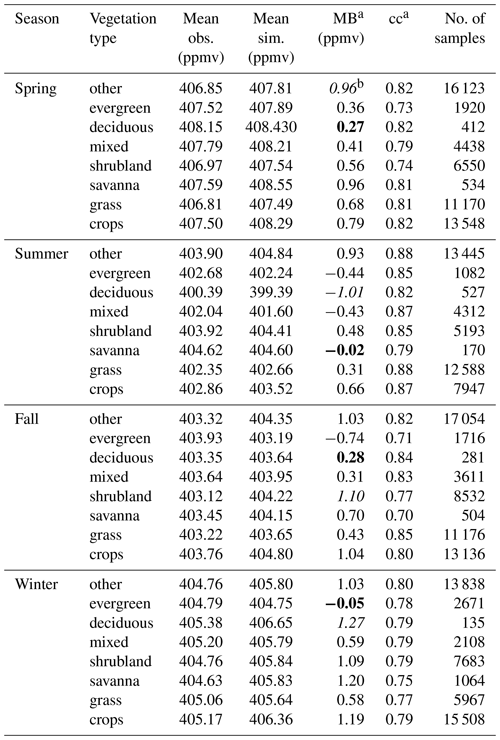
a Mean bias was calculated as , and correlation coefficient was calculated as , where is the average of simulations, and is the average of observations. b For each season, the evaluation statistic with the worst performance (largest absolute value of MB) is highlighted in italics, and the one with the best performance (smallest absolute value of MB) is highlighted in bold.
XCO2 showed similar seasonality, with minima in August and maxima in April and December (Fig. 5b). Both WRF-Chem and CT2019 showed good agreement with TCCON Hefei observations, with correlations of 0.89 and 0.88, respectively (Fig. 5e). However, we note that WRF-Chem simulated drastic changes (e.g., the grey shaded period in Fig. 5e) that were not reproduced by CT2019. Figure 6 shows the daily concentrations of XCO2 overlaid with horizontal wind speed at 10 from WRF-Chem and CT2019 and highlights large discrepancies over Hefei (Fig. S4 shows the same comparison but using WRF-Chem 4 km grid simulation data). Between 1 and 3 April 2016, an 850 hPa trough associated with a surface cold front moved southeastward from Mongolia to the North China Plain (NCP) (weather maps shown in Fig. 6g–i). At the leading edge of the front, a convergence zone associated with a low-pressure centre formed, which led to significant cloud formation and subsequently reduced short-wave radiation. As a result, photosynthetic carbon uptake was reduced, leading to enhancement of atmospheric CO2. Meanwhile, the cold front transported anthropogenic CO2 from NCP to YRD, and the convergence zone along YRD ahead of the front facilitated the accumulation of air pollutants and CO2 from anthropogenic emissions. With its coarse spatio-temporal resolution, CT2019 had difficulty reproducing such regional weather systems that can lead to rapid and localized changes in CO2 concentration and the terrestrial flux, indicating the importance of fine-resolution modelling to better represent the small spatial-scale and rapid temporal-scale variations of CO2 (Agusti-Panareda et al., 2019).
We also find a notable increasing trend for the 3-year study period. Observed CO2 annual enhancement was 2.2 ppmv yr−1 (0.56 % yr−1) at the ESRL sites and 2.3 ppmv yr−1 (0.54 % yr−1) at Lin'an. The observed average CO2 concentrations at Lin'an (428 ppmv) were substantially higher than those at ESRL sites (407–410 ppmv). The prominent higher levels of CO2 and slightly higher absolute growth rate at Lin'an can be attributed to the influence of the transport regional anthropogenic emission, which is growing at a rate of 0.82 % yr−1 as suggested by ODIAC. Domain-wide XCO2 was also found to increase by 2.3 ppmv yr−1 (0.57 % yr−1) as suggested by OCO-2 and 2.5 ppmv yr−1 (0.61 % yr−1) as suggested by the simulation. WRF-Chem reproduced the trends in good agreement with ground and satellite observations. Model-simulated budgets suggested that the increasing trends for anthropogenic, biogenic, and background XCO2 were 0.81, −9.17, and 0.59 % yr−1, respectively; the trends for anthropogenic, biogenic, and background CO2 were 4.95, −0.73, and 0.59 % yr−1, respectively. Our findings are consistent with recent measurements and inverse modelling studies but provide process-based estimates for anthropogenic emission and the terrestrial flux. Wu et al. (2012) reported that measured CO2 concentration at the Changbai Mountain forest site in northeast China increased by 1.76 ppmv yr−1 between 2003 and 2010. With the atmospheric inversion modelling method, Z. Fu et al. (2019) estimated that surface CO2 in East Asia increased by 2–3 ppmv yr−1 between 2004 and 2012. These trends suggest that although anthropogenic emission increases at a steady rate in East Asia, photosynthetic uptake also serves as an increasing carbon sink due to enhanced EVI (0.29 % yr−1). However, as the interannual variability (IAV) of the terrestrial flux is usually critically large and is affected by both vegetation itself and climate conditions (Z. Fu et al., 2019; Niu et al., 2017), simulation over longer time periods is necessary in future studies to conclusively comment on the changing trend of biosphere CO2 in China.
3.3 Diurnal variation of near-surface CO2 and influence factors
Finally, we examine the diurnal variation of CO2 data at Lin'an station as shown in Fig. 7 to reveal the temporal dynamics and atmospheric mixing of CO2 at a local scale. While both 21 m (Fig. 7a) and 55 m (Fig. 7b) CO2 show prominent diurnal changes, the variations were larger in summer (JJA) than winter (DJF) and were larger at 21 m than 55 m, indicating the dominant influence of the terrestrial flux over anthropogenic emission in determining the near-surface CO2 concentration. Figure 7c and d present the WRF-Chem simulation bias at 21 and 55 m, respectively, and Fig. 7e and f present the bias of CT2019 at 21 and 55 m, respectively. We find that both models prominently overestimated during night-time, which shall be attributed to the bias in simulating NEE. Li et al. (2020) reported that the model overestimated night-time NEE at a mixed forest site, Wuying (47.15∘ N, 131.94∘ E), by 34 % during the growing season (May–September) according to eddy-covariance tower measurements. Figure 7g and h present the simulated NEE by WRF-Chem and CT2019, respectively, which show close correlations with the CO2 simulation biases. While Lin'an is also covered by mixed forest, our evaluation suggests that WRF-Chem may also overestimate night-time ecosystem respiration at Lin'an as it has warmer climate conditions than Wuying (Fig. S5), and CT2019 has an even greater bias for presenting the diurnal cycles of the terrestrial flux.
We also find that planetary boundary layer height (PBLH) significantly affects diurnal accumulation and depletion of atmospheric CO2 as shown in Fig. 8a. During daytime in the growing season, photosynthetic uptake results in lower CO2 concentration; meanwhile, PBLH is also high and allows rapid vertical mixing between near-surface and upper air. During night-time when photosynthesis stops, CO2 from ecosystem respiration starts to accumulate in the shallow stable boundary layer, while the residual layer remains largely decoupled. Thus, atmospheric constituents with surface sources normally exhibit a vertical profile in which concentrations decrease with height in the stable boundary layer (Hu et al., 2020, 2012). Such boundary layer characteristics are confirmed by CO2 vertical gradients at Lin'an in this study. CO2 at 55 m height was consistently lower than the near-surface air at 21 m during night-time due to accumulation of respired CO2 in the stable boundary layer. As photosynthetic uptake depleted the near-surface CO2, and daytime boundary layer convection developed, the CO2 gradient was gradually weakened from 06:00 to 11:00 LT and remained minimal through the rest of the day; at midday when photosynthesis reaches maximum intensity, CO2 at 21 m was even lower than at 55 m. WRF-Chem roughly reproduced the diurnal profile but noticeably underestimated the intensity of night-time CO2 difference (ΔCO2), likely due to the bias for simulating the night-time terrestrial flux as discussed above or the underestimation of night-time boundary layer stability by the PBL scheme (Hu et al., 2012).
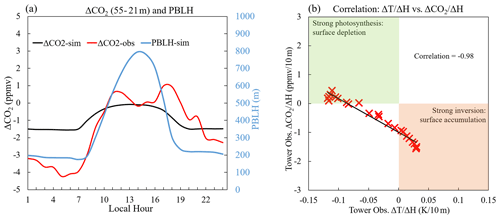
Figure 8(a) Average (2016–2018) diurnal variations of simulated (black line) and observed (red line) ΔCO2 and simulated (blue line) PBLH at Lin'an station and (b) correlation between CO2 gradient between 55 and 21 m () and temperature gradient () at Lin'an station.
The relationship between the near-surface CO2 profile and boundary layer stability is statistically examined further. Figure 8b presents the correlation between air temperature gradient () and CO2 concentration gradient () calculated with diurnal profiles of tower observations averaged for 2016–2018, where ΔT, ΔH, and ΔCO2 are the differences of temperature, height, and CO2 concentration between the two tower levels, respectively. Figure 8b clearly demonstrates the influence of boundary layer stability on the CO2 vertical profile, as the correlation between and reaches −0.98. On the one hand, a more stable PBL with a strongly positive temperature gradient would promote surface CO2 accumulation and lead to a strongly negative CO2 gradient, especially under inversion conditions when upper air has higher temperature (orange area in Fig. 8b). Conversely, a strongly negative temperature gradient indicates stronger radiation and subsequently greater photosynthesis and CO2 depletion in the near-surface layer, which would result in a positive CO2 gradient (green area in Fig. 8b), implying a lower CO2 concentration at the surface. While the diurnal variations of ΔCO2 were primarily dictated by local biogenic CO2 fluxes and boundary layer dynamics, the two minor daytime peaks of ΔCO2 at Lin'an, at 10:00 and 18:00 LT (Fig. 8a), likely suggest influence of transport of CO2 from urban plumes in the region, for example, from Hangzhou which is 60 km away from the tower. Due to rush-hour anthropogenic emissions, CO2 enhancement at Hangzhou relative to a background site exhibited a prominent bimodal curve with two peaks during early morning and early evening (Pu et al., 2018). Depending on meteorological conditions, particularly wind fields, urban CO2 plumes from cities such as Hangzhou may be transported to the Lin'an site. The influence of boundary layer conditions on CO2 variability has been discussed in several studies through the analysis of mountain site ground-based observations (Arrillaga et al., 2019; Esteki et al., 2017; Li et al., 2014), but our study applied tower data as direct evidence to demonstrate the significant impact of PBL thermal structure, which has rarely been documented. More importantly, although WRF-Chem failed to capture the bimodal ΔCO2 peaks at rush hour because monthly ODIAC data lacked an hourly profile, our analysis reveals the critical importance of careful configuration of the PBL scheme and spatio-temporal distribution of anthropogenic emission for weather–biosphere modelling of atmospheric CO2.
In this study, the spatio-temporal variations of CO2 in China are investigated with measurements from multiple datasets and a weather–biosphere coupled model simulation for 2016–2018. We find consistent higher concentrations over industrial areas with excessive anthropogenic emission and lower concentrations over densely vegetated areas. Observed CO2 concentrations at Lin'an (427 ppmv) are significantly higher than remote ESRL sites (408 ppmv), although they are all established as “background” stations, indicating the dominant influence of anthropogenic emission at regional scales. The Lin'an tower data show a large negative correlation (−0.98) between vertical CO2 concentration and air temperature gradients, suggesting the significant influence of boundary layer stability on CO2 accumulation and depletion. The online coupled weather–biosphere model WRF-Chem enables process-based estimations of contributions from anthropogenic emission (0.59 ppmv, 0.15 %), the terrestrial flux (0.16 ppmv, −0.04 %), and background concentration (405.70 ppmv, 99.89 %) to average total XCO2. Respective simulation biases of surface CO2 and XCO2 are 0.69 and 0.76 ppmv against ESRL site observations and the OCO-2 satellite product, with correlations of 0.87 and 0.90, indicating overall good performance of the WRF-Chem model. Maximum CO2 concentrations are found in April, and minima are found in August for all 3 years, and the seasonality is reproduced well by the model, which also reveals that the terrestrial flux and background concentration dominated the seasonality rather than anthropogenic emission.
A steadily increasing trend in XCO2 by 2.46 ppmv (∼ 0.6 % yr−1) during the study period is demonstrated consistently by both model simulation and the satellite product. Budget analysis suggests that anthropogenic emission increased by 0.83 % yr−1, contributing to the 0.81 % yr−1 growth rate of anthropogenic XCO2 enhancement, 27 % of which was offset by biosphere uptake. It is noted that the terrestrial flux has significant inter-annual variability; thus a more robust estimation of the terrestrial flux trend should be obtained through a long-term study in the future. The background XCO2, representing contributions from global circulation, increased by 2.37 ppm (0.59 % yr−1), suggesting that the CO2 level in China was growing at the same rate as the rest of the world.
The most significant modelling bias is identified from validation against the Lin'an tower 55 m observations, which WRF-Chem 4 km grid simulation overestimated by about 1.06 ppmv, with a correlation coefficient of 0.82. The allocation of anthropogenic emission into the surface layer is partially responsible for this modelling bias because Lin'an is closely affected by upwind industrial mega cities in YRD, suggesting the need to include vertical profiles of fossil fuel combustion to properly redistribute the ODIAC for modelling purposes. In addition, diurnal variations of the bias suggest that the modelling discrepancy is also induced by large uncertainty associated with simulating night-time ecosystem respiration. Representation and parameterization of photosynthetic carbon uptake in VPRM have been continuously improved during the past 10 years since its first release (Hu et al., 2020), but ecosystem respiration parameterization is still too simplified to fully represent the autotrophic and heterotrophic respiration of biomass (Hu et al., 2021). Li et al. (2020) and our study both reveal the urgent need to better calibrate VPRM parameterization over different vegetation types in China, and other methods such as inverse modelling are necessary to further validate the anthropogenic fluxes from ODIAC. Nevertheless, WRF-Chem is demonstrated to be a reliable tool to model the dynamics of CO2 and exchange between the atmosphere and the terrestrial flux. Most importantly, as the online coupled modelling system is able to simulate meteorology and biosphere processes simultaneously, it promotes the opportunity to investigate the interactions between atmospheric mixing and the terrestrial flux (Carvalhais et al., 2014; Schimel et al., 2015) while comprehensively considering various factors from both sides that affect CO2 in one coordinate frame, which could be a very helpful tool to support policymakers for balancing short-term carbon cycles at regional scales.
The modelling output is accessible by contacting the corresponding author (yjjiang@pku.org.cn, xhu@ou.edu).
The supplement related to this article is available online at: https://doi.org/10.5194/acp-21-7217-2021-supplement.
The concept and ideas to design the integrated simulation and observation analysis were devised by YJ, XMH, and XD. Simulation was performed by XMH. The OCO-2 satellite product was collected and processed by XMH. CT2019 assimilation data and ground-based observations were collected by XD. Tower measurements were conducted, processed, and analysed by QM, JP, and YJ. Model evaluation was performed by MY. The paper was prepared by XD and XMH with input and feedback from YJ, MY, QM, JP, and GZ.
The authors declare that they have no conflict of interest.
This work is supported by the Fundamental Research Funds for the Central Universities (14380049) and the National Key Research and Development Program of China (2016YFC0201900). We thank NASA and NOAA ESRL for providing the public accessible satellite products and observations used in this study. OCO-2 data were collected through https://co2.jpl.nasa.gov/#mission=OCO-2 (last access: 30 October 2020). We thank Tomohiro Oda for providing Open-Data Inventory for Anthropogenic Carbon dioxide (ODIAC) emissions. ESRL surface flask CO2 data were downloaded from https://www.esrl.noaa.gov/gmd/dv/data.html (last access: 15 August 2020). TCCON data were downloaded from https://data.caltech.edu/records/1092 last access: 15 August 2020). CT2019B results were provided by NOAA ESRL, Boulder, Colorado, USA, on the website at http://carbontracker.noaa.gov (last access: 15 August 2020).
This work is supported by the Fundamental Research Funds for the Central Universities (14380049) and the National Key Research and Development Program of China (2016YFC0201900).
This paper was edited by Alex B. Guenther and reviewed by three anonymous referees.
Agustí-Panareda, A., Diamantakis, M., Massart, S., Chevallier, F., Muñoz-Sabater, J., Barré, J., Curcoll, R., Engelen, R., Langerock, B., Law, R. M., Loh, Z., Morguí, J. A., Parrington, M., Peuch, V.-H., Ramonet, M., Roehl, C., Vermeulen, A. T., Warneke, T., and Wunch, D.: Modelling CO2 weather – why horizontal resolution matters, Atmos. Chem. Phys., 19, 7347–7376, https://doi.org/10.5194/acp-19-7347-2019, 2019.
Ahmadov, R., Gerbig, C., Kretschmer, R., Koerner, S., Neininger, B., Dolman, A. J., and Sarrat, C.: Mesoscale covariance of transport and CO2 fluxes: Evidence from observations and simulations using the WRF-VPRM coupled atmosphere-biosphere model, J. Geophys. Res.-Atmos., 112, D22107, https://doi.org/10.1029/2007JD008552, 2007.
Ahmadov, R., Gerbig, C., Kretschmer, R., Körner, S., Rödenbeck, C., Bousquet, P., and Ramonet, M.: Comparing high resolution WRF-VPRM simulations and two global CO2 transport models with coastal tower measurements of CO2, Biogeosciences, 6, 807–817, https://doi.org/10.5194/bg-6-807-2009, 2009.
Arrillaga, J. A., Yagüe, C., Román-Cascón, C., Sastre, M., Jiménez, M. A., Maqueda, G., and Vilà-Guerau de Arellano, J.: From weak to intense downslope winds: origin, interaction with boundary-layer turbulence and impact on CO2 variability, Atmos. Chem. Phys., 19, 4615–4635, https://doi.org/10.5194/acp-19-4615-2019, 2019.
Bauska, T. K., Joos, F., Mix, A. C., Roth, R., Ahn, J., and Brook, E. J.: Links between atmospheric carbon dioxide, the land carbon reservoir and climate over the past millennium, Nat. Geosci., 8, 574–574, 2015.
Beck, V., Gerbig, C., Koch, T., Bela, M. M., Longo, K. M., Freitas, S. R., Kaplan, J. O., Prigent, C., Bergamaschi, P., and Heimann, M.: WRF-Chem simulations in the Amazon region during wet and dry season transitions: evaluation of methane models and wetland inundation maps, Atmos. Chem. Phys., 13, 7961–7982, https://doi.org/10.5194/acp-13-7961-2013, 2013.
Brunner, D., Kuhlmann, G., Marshall, J., Clément, V., Fuhrer, O., Broquet, G., Löscher, A., and Meijer, Y.: Accounting for the vertical distribution of emissions in atmospheric CO2 simulations, Atmos. Chem. Phys., 19, 4541–4559, https://doi.org/10.5194/acp-19-4541-2019, 2019.
Carvalhais, N., Forkel, M., Khomik, M., Bellarby, J., Jung, M., Migliavacca, M., Mu, M. Q., Saatchi, S., Santoro, M., Thurner, M., Weber, U., Ahrens, B., Beer, C., Cescatti, A., Randerson, J. T., and Reichstein, M.: Global covariation of carbon turnover times with climate in terrestrial ecosystems, Nature, 514, 213–217, 2014.
Chen, F. and Dudhia, J.: Coupling an advanced land surface-hydrology model with the Penn State-NCAR MM5 modeling system. Part I: Model implementation and sensitivity, Mon. Weather Rev., 129, 569–585, 2001.
Crowell, S. M. R., Kawa, S. R., Browell, E. V., Hammerling, D. M., Moore, B., Schaefer, K., and Doney, S. C.: On the Ability of Space-Based Passive and Active Remote Sensing Observations of CO2 to Detect Flux Perturbations to the Carbon Cycle, J. Geophys. Res.-Atmos., 123, 1460–1477, 2018.
Dayalu, A., Munger, J. W., Wofsy, S. C., Wang, Y., Nehrkorn, T., Zhao, Y., McElroy, M. B., Nielsen, C. P., and Luus, K.: Assessing biotic contributions to CO2 fluxes in northern China using the Vegetation, Photosynthesis and Respiration Model (VPRM-CHINA) and observations from 2005 to 2009, Biogeosciences, 15, 6713–6729, https://doi.org/10.5194/bg-15-6713-2018, 2018.
Deng, J. J., Zhang, Y. R., Qiu, Y. Q., Zhang, H. L., Du, W. J., Xu, L. L., Hong, Y. W., Chen, Y. T., and Chen, J. S.: Source apportionment of PM2.5 at the Lin'an regional background site in China with three receptor models, Atmos. Res., 202, 23–32, 2018.
Dudhia, J.: Numerical Study of Convection Observed during the Winter Monsoon Experiment Using a Mesoscale Two-Dimensional Model, J. Atmos. Sci., 46, 3077–3107, 1989.
Esteki, K., Prakash, N., Li, Y. L., Mu, C., and Du, K.: Seasonal Variation of CO2 Vertical Distribution in the Atmospheric Boundary Layer and Impact of Meteorological Parameters, Int. J. Environ. Res., 11, 707–721, 2017.
Feng, S., Lauvaux, T., Keller, K., Davis, K. J., Rayner, P., Oda, T., and Gurney, K. R.: A Road Map for Improving the Treatment of Uncertainties in High-Resolution Regional Carbon Flux Inverse Estimates, Geophys. Res. Lett., 46, 13461–13469, 2019.
Fu, Y., Liao, H., Tian, X. J., Gao, H., Cai, Z. N., and Han, R.: Sensitivity of the simulated CO2 concentration to inter-annual variations of its sources and sinks over East Asia, Adv. Clim. Chang. Res., 10, 250–263, 2019.
Fu, Z., Stoy, P. C., Poulter, B., Gerken, T., Zhang, Z., Wakbulcho, G., and Niu, S. L.: Maximum carbon uptake rate dominates the interannual variability of global net ecosystem exchange, Glob. Change Biol., 25, 3381–3394, 2019.
Fung, I., Prentice, K., Matthews, E., Lerner, J., and Russell, G.: 3-Dimensional Tracer Model Study of Atmospheric CO2 – Response to Seasonal Exchanges with the Terrestrial Biosphere, J. Geophys. Res.-Oceans, 88, 1281–1294, 1983.
Gao, Y. H., Xu, J. W., and Chen, D. L.: Evaluation of WRF Mesoscale Climate Simulations over the Tibetan Plateau during 1979–2011, J. Climate, 28, 2823–2841, 2015.
Gao, Y. Q., Lee, X. H., Liu, S. D., Hu, N., Hu, C., Liu, C., Zhang, Z., and Yang, Y. C.: Spatiotemporal variability of the near-surface CO2 concentration across an industrial-urban-rural transect, Nanjing, China, Sci. Total Environ., 631–632, 1192–1200, 2018.
Gaubert, B., Stephens, B. B., Basu, S., Chevallier, F., Deng, F., Kort, E. A., Patra, P. K., Peters, W., Rödenbeck, C., Saeki, T., Schimel, D., Van der Laan-Luijkx, I., Wofsy, S., and Yin, Y.: Global atmospheric CO2 inverse models converging on neutral tropical land exchange, but disagreeing on fossil fuel and atmospheric growth rate, Biogeosciences, 16, 117–134, https://doi.org/10.5194/bg-16-117-2019, 2019.
Grell, G. A. and Devenyi, D.: A generalized approach to parameterizing convection combining ensemble and data assimilation techniques, Geophys. Res. Lett., 29, 1693, https://doi.org/10.1029/2002GL015311, 2002.
Han, P. F., Zeng, N., Yao, B., Zhou, W. J., Chen, L. Q., Wang, S. Q., Lv, H. G., Xiao, W., Zhu, L. Y., and Xu, J. P.: Preface to Special Topic on Atmospheric Greenhouse Gas Measurement and Application in China, Adv. Atmos. Sci., 37, 555–556, 2020.
Hedelius, J. K., Feng, S., Roehl, C. M., Wunch, D., Hillyard, P., Podolske, J. R., Iraci, L. T., Patarasuk, R., Rao, P., O'Keeffe, D., Gurney, K. R., Lauvaux, T., and Wennberg, P. O.: Emissions and topographic effects on column CO2 (X-CO2) variations, with a focus on the Southern California Megacity, J. Geophys. Res.-Atmos., 122, 7200–7215, 2017.
Hong, S. Y., Noh, Y., and Dudhia, J.: A new vertical diffusion package with an explicit treatment of entrainment processes, Mon. Weather Rev., 134, 2318–2341, 2006.
Hu, X. M., Doughty, D. C., Sanchez, K. J., Joseph, E., and Fuentes, J. D.: Ozone variability in the atmospheric boundary layer in Maryland and its implications for vertical transport model, Atmos. Environ., 46, 354–364, 2012.
Hu, X. M., Crowell, S., Wang, Q. Y., Zhang, Y., Davis, K. J., Xue, M., Xiao, X. M., Moore, B., Wu, X. C., Choi, Y., and DiGangi, J. P.: Dynamical Downscaling of CO2 in 2016 Over the Contiguous United States Using WRF-VPRM, a Weather-Biosphere-Online-Coupled Model, J. Adv. Model. Earth Sy., 12, e2019MS001875, https://doi.org/10.1029/2019MS001875, 2020.
Hu, X. M., Gourdji, S. M., Davis, K. J., Wang, Q., Zhang, Y., Xue, M., Feng, S., Moore, B., and Crowell, S. M. R.: Implementation of improved parameterization of terrestrial flux in WRF-VPRM improves the simulation of nighttime CO2 peaks and a daytime CO2 band ahead of a cold front, J. Geophy. Res.-Atmos., https://doi.org/10.1029/2020JD034362, in press, 2021.
Huang, X., Ding, A. J., Wang, Z. L., Ding, K., Gao, J., Chai, F. H., and Fu, C. B.: Amplified transboundary transport of haze by aerosol-boundary layer interaction in China, Nat. Geosci., 13, 428–434, 2020.
Huete, A., Didan, K., Miura, T., Rodriguez, E. P., Gao, X., and Ferreira, L. G.: Overview of the radiometric and biophysical performance of the MODIS vegetation indices, Remote Sens. Environ., 83, 195–213, 2002.
Jacobson, A. R., Schuldt, K. N., Miller, J. B., Oda, T., Tans, P., Andrews, A., Mund, J., Ott, L., Collatz,G. J., Aalto, T., Afshar, S., Aikin, K., Aoki, S., Apadula, F., Baier, B., Bergamaschi, P., Beyersdorf, A., Biraud, S. C., Bollenbacher, A., Bowling, D., Brailsford, G., Abshire, J. B., Chen, G., Chen, H., Chmura, L., Colomb, A., Conil, S., Cox, A., Cristofanelli, P., Cuevas, E., Curcoll, R., Sloop, C. D., Davis, K., Wekker, S. D., Delmotte, M., DiGangi, J. P., Dlugokencky, E., Ehleringer, J., Elkins, J. W., Emmenegger, L., Fischer, M. L., Forster, G., Frumau, A., Galkowski, M., Gatti, L. V., Gloor, E., Griffis, T., Hammer, S., Haszpra, L., Hatakka, J., Heliasz, M., Hensen, A., Hermanssen, O., Hintsa, E., Holst, J., Jaffe, D., Karion, A., Kawa, S. R., Keeling, R., Keronen, P., Kolari, P., Kominkova, K., Kort, E., Krummel, P., Kubistin, D., Labuschagne, C., Langenfelds, R., Laurent, O., Laurila, T., Lauvaux, T., Law, B., Lee, J., Lehner, I., Leuenberger, M., Levin, I., Levula, J., Lin, J., Lindauer, M., Loh, Z., Lopez, M., Luijkx, I. T., Lund Myhre, C., Machida, T., Mammarella, I., Manca, G., Manning, A., Marek, M. V., Marklund, P., Martin, M. Y., Matsueda, H., McKain, K., Meijer, H., Meinhardt, F., Miles, N., Miller, C. E., Molder, M., Montzka, S., Moore, F., Morgui, J.-A., Morimoto, S., Munger, B., Necki, J., Newman, S., Nichol, S., Niwa, Y., ODoherty, S., Ottosson-Lofvenius, M., Paplawsky, B., Peischl, J., Peltola, O., Pichon, J.-M., Piper, S., Plass-Dolmer, C., Ramonet, M., Reyes-Sanchez, E., Richardson, S., Riris, H., Ryerson, T., Saito, K., Sargent, M., Sasakawa, M., Sawa, Y., Say, D., Scheeren, B., Schmidt, M., Schmidt, A., Schumacher, M., Shepson, P., Shook, M., Stanley, K., Steinbacher, M., Stephens, B., Sweeney, C., Thoning, K., Torn, M., Turnbull, J., Tørseth, K., Bulk, P. V. D., Dinther, D. V., Vermeulen, A., Viner, B., Vitkova, G., Walker, S., Weyrauch, D., Wofsy, S., Worthy, D., Young, D., and Zimnoch, M.: CarbonTracker CT2019B, NOAA – National Oceanic and Atmospheric Administration's and ESRL – Earth System Research Laboratories, https://doi.org/10.25925/20201008, 2020.
Keenan, T. F., Prentice, I. C., Canadell, J. G., Williams, C. A., Wang, H., Raupach, M., and Collatz, G. J.: Recent pause in the growth rate of atmospheric CO2 due to enhanced terrestrial carbon uptake, Nat. Commun., 8, 16137, https://doi.org/10.1038/ncomms16137, 2017.
Kiehl, J. T. and Ramanathan, V.: CO2 Radiative Parameterization Used in Climate Models - Comparison with Narrow-Band Models and with Laboratory Data, J. Geophys. Res.-Oceans, 88, 5191–5202, 1983.
Kiel, M., O'Dell, C. W., Fisher, B., Eldering, A., Nassar, R., MacDonald, C. G., and Wennberg, P. O.: How bias correction goes wrong: measurement of affected by erroneous surface pressure estimates, Atmos. Meas. Tech., 12, 2241–2259, https://doi.org/10.5194/amt-12-2241-2019, 2019.
Kondo, M., Patra, P. K., Sitch, S., Friedlingstein, P., Poulter, B., Chevallier, F., Ciais, P., Canadell, J. G., Bastos, A., Lauerwald, R., Calle, L., Ichii, K., Anthoni, P., Arneth, A., Haverd, V., Jain, A. K., Kato, E., Kautz, M., Law, R. M., Lienert, S., Lombardozzi, D., Maki, T., Nakamura, T., Peylin, P., Rodenbeck, C., Zhuravlev, R., Saeki, T., Tian, H. Q., Zhu, D., and Ziehn, T.: State of the science in reconciling top-down and bottom-up approaches for terrestrial CO2 budget, Glob. Change Biol., 26, 1068–1084, 2020.
Kountouris, P., Gerbig, C., Rödenbeck, C., Karstens, U., Koch, T. F., and Heimann, M.: Atmospheric CO2 inversions on the mesoscale using data-driven prior uncertainties: quantification of the European terrestrial CO2 fluxes, Atmos. Chem. Phys., 18, 3047–3064, https://doi.org/10.5194/acp-18-3047-2018, 2018.
Kretschmer, R., Gerbig, C., Karstens, U., and Koch, F.-T.: Error characterization of CO2 vertical mixing in the atmospheric transport model WRF-VPRM, Atmos. Chem. Phys., 12, 2441–2458, https://doi.org/10.5194/acp-12-2441-2012, 2012.
Li, X. L., Hu, X. M., Cai, C. J., Jia, Q. Y., Zhang, Y., Liu, J. M., Xue, M., Xu, J. M., Wen, R. H., and Crowell, S. M. R.: Terrestrial CO2 Fluxes, Concentrations, Sources and Budget in Northeast China: Observational and Modeling Studies, J. Geophys. Res.-Atmos., 125, 2020.
Li, Y., Deng, J., Mu, C., Xing, Z., and Du, K.: Vertical distribution of CO2 in the atmospheric boundary layer: Characteristics and impact of meteorological variables, Atmos. Environ., 91, 110–117, 2014.
Mahadevan, P., Wofsy, S. C., Matross, D. M., Xiao, X. M., Dunn, A. L., Lin, J. C., Gerbig, C., Munger, J. W., Chow, V. Y., and Gottlieb, E. W.: A satellite-based biosphere parameterization for net ecosystem CO2 exchange: Vegetation Photosynthesis and Respiration Model (VPRM), Global Biogeochem. Cy., 22, GB2005, https://doi.org/10.1029/2006GB002735, 2008.
Mlawer, E. J., Taubman, S. J., Brown, P. D., Iacono, M. J., and Clough, S. A.: Radiative transfer for inhomogeneous atmospheres: RRTM, a validated correlated-k model for the longwave, J. Geophys. Res.-Atmos., 102, 16663–16682, 1997.
Morrison, H., Thompson, G., and Tatarskii, V.: Impact of Cloud Microphysics on the Development of Trailing Stratiform Precipitation in a Simulated Squall Line: Comparison of One- and Two-Moment Schemes, Mon. Weather Rev., 137, 991–1007, 2009.
Niu, S. L., Fu, Z., Luo, Y. Q., Stoy, P. C., Keenan, T. F., Poulter, B., Zhang, L. M., Piao, S. L., Zhou, X. H., Zheng, H., Han, J. Y., Wang, Q. F., and Yu, G. R.: Interannual variability of ecosystem carbon exchange: From observation to prediction, Global Ecol. Biogeogr., 26, 1225–1237, 2017.
Oda, T., Maksyutov, S., and Andres, R. J.: The Open-source Data Inventory for Anthropogenic CO2, version 2016 (ODIAC2016): a global monthly fossil fuel CO2 gridded emissions data product for tracer transport simulations and surface flux inversions, Earth Syst. Sci. Data, 10, 87–107, https://doi.org/10.5194/essd-10-87-2018, 2018.
Papale, D. and Valentini, A.: A new assessment of European forests carbon exchanges by eddy fluxes and artificial neural network spatialization, Glob. Change Biol., 9, 525–535, 2003.
Park, C., Gerbig, C., Newman, S., Ahmadov, R., Feng, S., Gurney, K. R., Carmichael, G. R., Park, S. Y., Lee, H. W., Goulden, M., Stutz, J., Peischl, J., and Ryerson, T.: CO2 Transport, Variability, and Budget over the Southern California Air Basin Using the High-Resolution WRF-VPRM Model during the CalNex 2010 Campaign, J. Appl. Meteorol. Clim., 57, 1337–1352, 2018.
Park, C., Park, S. Y., Gurney, K. R., Gerbig, C., DiGangi, J. P., Choi, Y., and Lee, H. W.: Numerical simulation of atmospheric CO2 concentration and flux over the Korean Peninsula using WRF-VPRM model during Korus-AQ 2016 campaign, Plos One, 15, e0228106, https://doi.org/10.1371/journal.pone.0228106, 2020.
Peters, W., Jacobson, A. R., Sweeney, C., Andrews, A. E., Conway, T. J., Masarie, K., Miller, J. B., Bruhwiler, L. M. P., Petron, G., Hirsch, A. I., Worthy, D. E. J., van der Werf, G. R., Randerson, J. T., Wennberg, P. O., Krol, M. C., and Tans, P. P.: An atmospheric perspective on North American carbon dioxide exchange: CarbonTracker, P. Natl. Acad. Sci. USA, 104, 18925–18930, https://doi.org/10.1073/pnas.0708986104, 2007.
Peylin, P., Baker, D., Sarmiento, J., Ciais, P., and Bousquet, P.: Influence of transport uncertainty on annual mean and seasonal inversions of atmospheric CO2 data, J. Geophys. Res.-Atmos., 107, 4385, https://doi.org/10.1029/2001JD000857, 2002.
Peylin, P., Law, R. M., Gurney, K. R., Chevallier, F., Jacobson, A. R., Maki, T., Niwa, Y., Patra, P. K., Peters, W., Rayner, P. J., Rödenbeck, C., van der Laan-Luijkx, I. T., and Zhang, X.: Global atmospheric carbon budget: results from an ensemble of atmospheric CO2 inversions, Biogeosciences, 10, 6699–6720, https://doi.org/10.5194/bg-10-6699-2013, 2013.
Pillai, D., Gerbig, C., Kretschmer, R., Beck, V., Karstens, U., Neininger, B., and Heimann, M.: Comparing Lagrangian and Eulerian models for CO2 transport – a step towards Bayesian inverse modeling using WRF/STILT-VPRM, Atmos. Chem. Phys., 12, 8979–8991, https://doi.org/10.5194/acp-12-8979-2012, 2012.
Pu, J. J., Xu, H. H., He, J., Fang, S. X., and Zhou, L. X.: Estimation of regional background concentration of CO2 at Lin'an Station in Yangtze River Delta, China, Atmos. Environ., 94, 402–408, 2014.
Pu, J. J., Hu, H. H., Jiang, Y. J., Du, R. G., and Qi, B.: Characteristics of and factors affecting atmospheric CO2 concentration in Hangzhou, Environ. Sci., 39, 3082–3089, https://doi.org/10.13227/j.hjkx.201708258, 2018.
Pu, J. J., Xu, H. H., Yao, B., Yu, Y., Jiang, Y. J., Ma, Q. L., and Chen, L. Q.: Estimate of Hydrofluorocarbon Emissions for 2012–16 in the Yangtze River Delta, China, Adv. Atmos. Sci., 37, 925–925, 2020.
Schimel, D., Stephens, B. B., and Fisher, J. B.: Effect of increasing CO2 on the terrestrial carbon cycle, P. Natl. Acad. Sci. USA, 112, 436–441, 2015.
Shi, Z., Crowell, S., Luo, Y. Q., and Moore, B.: Model structures amplify uncertainty in predicted soil carbon responses to climate change, Nat. Commun., 9, 2171, https://doi.org/10.1038/s41467-018-04526-9, 2018.
Takahashi, T., Sutherland, S. C., Wanninkhof, R., Sweeney, C., Feely, R. A., Chipman, D. W., Hales, B., Friederich, G., Chavez, F., Sabine, C., Watson, A., Bakker, D. C. E., Schuster, U., Metzl, N., Yoshikawa-Inoue, H., Ishii, M., Midorikawa, T., Nojiri, Y., Kortzinger, A., Steinhoff, T., Hoppema, M., Olafsson, J., Arnarson, T. S., Tilbrook, B., Johannessen, T., Olsen, A., Bellerby, R., Wong, C. S., Delille, B., Bates, N. R., and de Baar, H. J. W.: Climatological mean and decadal change in surface ocean pCO2, and net sea–air CO2 flux over the global oceans, Deep-Sea Res. Pt. I, 56, 2075–2076, 2009.
Tang, J. P., Niu, X. R., Wang, S. Y., Gao, H. X., Wang, X. Y., and Wu, J.: Statistical downscaling and dynamical downscaling of regional climate in China: Present climate evaluations and future climate projections, J. Geophys. Res.-Atmos., 121, 2110–2129, 2016.
Tian, H. Q., Lu, C. Q., Yang, J., Banger, K., Huntzinger, D. N., Schwalm, C. R., Michalak, A. M., Cook, R., Ciais, P., Hayes, D., Huang, M. Y., Ito, A., Jain, A. K., Lei, H. M., Mao, J. F., Pan, S. F., Post, W. M., Peng, S. S., Poulter, B., Ren, W., Ricciuto, D., Schaefer, K., Shi, X. Y., Tao, B., Wang, W. L., Wei, Y. X., Yang, Q. C., Zhang, B. W., and Zeng, N.: Global patterns and controls of soil organic carbon dynamics as simulated by multiple terrestrial biosphere models: Current status and future directions, Global Biogeochem. Cy., 29, 775–792, 2015.
Todd-Brown, K. E. O., Randerson, J. T., Post, W. M., Hoffman, F. M., Tarnocai, C., Schuur, E. A. G., and Allison, S. D.: Causes of variation in soil carbon simulations from CMIP5 Earth system models and comparison with observations, Biogeosciences, 10, 1717–1736, https://doi.org/10.5194/bg-10-1717-2013, 2013.
Wang, H., Jiang, F., Wang, J., Ju, W., and Chen, J. M.: Terrestrial ecosystem carbon flux estimated using GOSAT and OCO-2 XCO2 retrievals, Atmos. Chem. Phys., 19, 12067–12082, https://doi.org/10.5194/acp-19-12067-2019, 2019.
Wang, W., Tian, Y., Liu, C., Sun, Y., Liu, W., Xie, P., Liu, J., Xu, J., Morino, I., Velazco, V. A., Griffith, D. W. T., Notholt, J., and Warneke, T.: Investigating the performance of a greenhouse gas observatory in Hefei, China, Atmos. Meas. Tech., 10, 2627–2643, https://doi.org/10.5194/amt-10-2627-2017, 2017.
Wu, J. B., Guan, D. X., Yuan, F. H., Yang, H., Wang, A. Z., and Jin, C. J.: Evolution of atmospheric carbon dioxide concentration at different temporal scales recorded in a tall forest, Atmos. Environ., 61, 9–14, 2012.
Xie, X. D., Huang, X. X., Wang, T. J., Li, M. M., Li, S., and Chen, P. L.: Simulation of Non-Homogeneous CO2 and Its Impact on Regional Temperature in East Asia, J. Meteorol. Res.-PRC, 32, 456–468, 2018.
Yang, S. H., Xu, J. Z., Liu, X. Y., Zhang, J. G., and Wang, Y. J.: Variations of carbon dioxide exchange in paddy field ecosystem under water-saving irrigation in Southeast China, Agr. Water Manage., 166, 42–52, 2016.
Yang, Y., Hu, X. M., Gao, S. H., and Wang, Y. M.: Sensitivity of WRF simulations with the YSU PBL scheme to the lowest model level height for a sea fog event over the Yellow Sea, Atmos. Res., 215, 253–267, 2019.
Yao, Y. T., Li, Z. J., Wang, T., Chen, A. P., Wang, X. H., Du, M. Y., Jia, G. S., Li, Y. N., Li, H. Q., Luo, W. J., Ma, Y. M., Tang, Y. H., Wang, H. M., Wu, Z. X., Yan, J. H., Zhang, X. Z., Zhang, Y. P., Zhang, Y., Zhou, G. S., and Piao, S. L.: A new estimation of China's net ecosystem productivity based on eddy covariance measurements and. a model tree ensemble approach, Agr. Forest Meteorol., 253, 84–93, 2018.
Zheng, B., Tong, D., Li, M., Liu, F., Hong, C., Geng, G., Li, H., Li, X., Peng, L., Qi, J., Yan, L., Zhang, Y., Zhao, H., Zheng, Y., He, K., and Zhang, Q.: Trends in China's anthropogenic emissions since 2010 as the consequence of clean air actions, Atmos. Chem. Phys., 18, 14095–14111, https://doi.org/10.5194/acp-18-14095-2018, 2018.
Zhou, Y., Williams, C. A., Lauvaux, T., Davis, K. J., Feng, S., Baker, I., Denning, S., and Wei, Y.: A Multiyear Gridded Data Ensemble of Surface Biogenic Carbon Fluxes for North America: Evaluation and Analysis of Results, J. Geophys. Res.-Biogeo., 125, e2019JG005314, https://doi.org/10.1029/2019JG005314, 2020.
Zhu, X. J., Yu, G. R., He, H. L., Wang, Q. F., Chen, Z., Gao, Y. N., Zhang, Y. P., Zhang, J. H., Yan, J. H., Wang, H. M., Zhou, G. S., Jia, B. R., Xiang, W. H., Li, Y. N., Zhao, L., Wang, Y. F., Shi, P. L., Chen, S. P., Xin, X. P., Zhao, F. H., Wang, Y. Y., Tong, C. L., Fu, Y. L., Wen, X. F., Liu, Y. C., Zhang, L. M., Zhang, L., Su, W., Li, S. G., and Sun, X. M.: Geographical statistical assessments of carbon fluxes in terrestrial ecosystems of China: Results from upscaling network observations, Global Planet. Change, 118, 52–61, 2014.
Zhu, Z. L., Tang, X. Z., and Zhao, F. H.: Comparison of Ozone Fluxes over a Maize Field Measured with Gradient Methods and the Eddy Covariance Technique, Adv. Atmos. Sci., 37, 586–596, 2020.