the Creative Commons Attribution 4.0 License.
the Creative Commons Attribution 4.0 License.
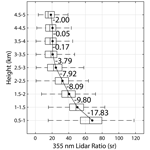
Long-term variation in aerosol lidar ratio in Shanghai based on Raman lidar measurements
Tongqiang Liu
Qianshan He
Yonghang Chen
Jie Liu
Qiong Liu
Wei Gao
Guan Huang
Wenhao Shi
Xiaohong Yu
Accurate lidar ratio (LR) and better understanding of its variation characteristics can not only improve the retrieval accuracy of parameters from elastic lidar, but also play an important role in assessing the impacts of aerosols on climate. Using the observational data of a Raman lidar in Shanghai from 2017 to 2019, LRs at 355 nm were retrieved and their variations and influence factors were analyzed. Within the height range of 0.5–5 km, about 90 % of the LRs were distributed in 10–80 sr with an average value of 41.0 ± 22.5 sr, and the LR decreased with the increase in height. The volume depolarization ratio (δ) was positively correlated with LR, and it also decreased with the increase in height, indicating that the vertical distribution of particle shape was one of the influence factors of the variations in LR with height. LR had a strong dependence on the original source of air masses. Affected by the aerosols transported from the northwest, the average LR was the largest, 44.2 ± 24.7 sr, accompanied by the most irregular particle shape. The vertical distribution of LR was affected by atmospheric turbidity, with the greater gradient of LR under clean conditions. The LR above 1 km could be more than 80 sr, when Shanghai was affected by biomass burning aerosols.
Aerosols in the atmosphere can affect the earth's climate by absorbing and scattering solar radiation (direct effect of aerosols) (Huang et al., 2014; Wang et al., 2013) or acting as cloud condensation nuclei, which can affect cloud physical properties and precipitation (indirect effect of aerosols) (Huang et al., 2006; Liu et al., 2019a, b; Yan and Wang, 2020). In general, the vertical distribution information of aerosols is required to improve our understanding of aerosol climate effects (Ferrare et al., 2001; Sicard et al., 2011). For example, Wang et al. (2020b) found that dust-forced radiative heating decreased significantly as the transport height of dust aerosols decreased. A study by Lu et al. (2020) showed that anomalous elevated aerosol layers above 2 km led to warming in the upper atmosphere (+0.32 K/d) and cooling on the surface (−0.04 K/d). In addition, the results of Liu et al. (2019b) showed that the aerosols mixing with the cloud layer in the vertical direction can significantly reduce the effective radius of ice particles and prolong the life of clouds.
As an active remote-sensing instrument, the elastic scattering lidar can obtain vertical distribution information of aerosols; however, it is necessary to assume an aerosol extinction-to-backscattering ratio (i.e., lidar ratio, LR) in the retrieval process (Fernald, 1984; Welton et al., 2001), which can result in significant errors for the extinction coefficient, followed by aerosol optical depth (AOD). To our knowledge, the LRs at 355, 532, and 1064 nm are usually assumed to be 50 sr in China (Fan et al., 2018; Gong et al., 2015; Lv et al., 2020; Ma et al., 2019). In addition, CALIOP (Cloud-Aerosol Lidar with Orthogonal Polarization) onboard CALIPSO (Cloud-Aerosol Lidar Infrared Pathfinder Satellite Observations) can observe the vertical distribution of global aerosol optical properties, and its data products have been widely used around the world (Kim et al., 2018). The CALIOP algorithm first determines the type of aerosol according to the aerosol classification algorithm and then uses lookup table of multiple types of aerosol to determine the LR (Kim et al., 2018; Omar et al., 2009). Therefore, the quality of the CALIOP aerosol products depends on the accuracy of aerosol type identification and the consistency between actual LR and that in the lookup table (Painemal et al., 2019). LR is a complicated function of time and space, which depends on aerosol size distribution and particle composition (Reagan et al., 1988). LR is also affected by meteorological elements (Salemink et al., 1984), such as relative humidity (RH), which can change the aerosol particle size distribution and refractive index (Young et al., 1993). Therefore, good knowledge of accurate LR and its variation characteristics can not only improve the retrieval accuracy of parameters from elastic lidar, but also obtain information on aerosol types to trace the source of pollutants (Franke et al., 2001).
According to the definition of LR in Müller's (2003) study,
LR was negatively correlated with phase function at 180∘ (P(π)) and single-scattering albedo (ω). P(π) is related to the sphericity of particles, and the sphericity information of particles can be obtained from polarization lidar. ω is indicative of aerosol absorption properties. The aerosol absorption properties and their vertical distributions play a crucial role in evaluating direct radiative forcing of aerosols (McComiskey et al., 2008; Zarzycki and Bond, 2010). Moreover, absorbing aerosols increase atmospheric stability by reducing the solar radiation reaching the surface during the day. By contrast, absorbing aerosols on the near-surface layer heat the surface and increase the atmospheric instability during night (Jacobson, 1998; Jacobson and Kaufman, 2006). The vertical profile of LR can reflect vertical variation in aerosol absorption properties, which can provide a basis for studying aerosol radiative forcing and causes of pollution (Mishchenko et al., 2004).
LR can be obtained by a variety of methods, such as high-spectrum-resolution lidar (HSRL), Raman lidar, and joint retrieval using sun photometer and elastic lidar (Zhao et al., 2018). Raman lidar can independently retrieve the extinction coefficient and backscatter coefficient of aerosols and obtain LR by combining elastic backscatter and Raman backscatter signals (Ansmann et al., 1992), which is the most widely used independent measurement method at present. Moreover, the LR measured by Raman lidar is a useful index to study the variations in aerosol physical properties (Ferrare et al., 2001).
A large number of observations and analysis of LR have been carried out based on Raman lidar all over the world. Since the establishment of the European Aerosol Research Lidar Network (EARLINET) in 2000, long time series observation data of vertical distribution and LR for various types of aerosol have been obtained on the European continent (Müller et al., 2007; Wandinger et al., 2016). In South Korea and Japan, the LR of Asian dust and biomass burning aerosols has also been studied based on Raman lidar (Murayama et al., 2004; Noh et al., 2007, 2008). The LR observed around the world usually shows different values due to different types of aerosols. However, long-term observations and research of LR in China are limited (Wang et al., 2016) due to the limitation of observation instruments. In particular, the observations and studies of LR are still rare in east China; however, range-resolved LR profiles based on independent measurement on a regional scale are very important. On one hand, the range-resolved LR obtained from ground-based Raman lidar can not only be used for comparison with 355 nm LR obtained from ATLID (Atmospheric LIDar) on EarthCARE (Earth Clouds and Radiation Explorer) planned to be launched by ESA (European Space Agency) (Liu et al., 2020a; Nicolae et al., 2018), but can also provide a reliable basis for the inversion hypothesis of elastic lidar in Shanghai and surrounding areas and improve product reliability for elastic lidar networks such as the Asian dust and aerosol lidar observation network. On the other hand, vertical distribution of aerosol absorption properties reflected by LR can be used as an input parameter for regional climate models (Mehta et al., 2018), which can further improve the calculation accuracy of radiative forcing.
In addition, studying the influence factors of LR in Shanghai can be conducive to understanding the LR variation characteristics and determining the source of pollutants. With these motivations, the vertical and temporal variations in LR and its influence factors in Shanghai were analyzed using the results retrieved from Raman lidar, which laid a solid foundation for the quantitative study of pollution and its causes in the future.
2.1 Observation equipment and data
2.1.1 Raman depolarization lidar
The Raman depolarization lidar (Raymetrics S.A., Athens, Greece, model LR231-D300) used in this study is deployed on the roof of a building (31.1916∘ N, 121.4333∘ E; 67 m above sea level, a.s.l.) in downtown Shanghai. The site is 35 km from the East China Sea coastline, surrounded by populated residential and commercial areas. The laser of the lidar system is an Nd: YAG pulse laser equipped with a water cooling device. The system can emit 355, 532, and 1064 nm laser pulses with a pulse width of 5.4 ns and a repetition rate of 20 Hz. The lidar detection system can receive 355, 532, and 1064 nm elastic scattering signals. The 532 nm elastic scattering channel has two polarization channels: 532P (parallel) and 532S (perpendicular). In addition, the lidar can also receive the vibrational Raman scattering signals of nitrogen (387 nm) and water vapor molecules (408 nm) with an incident wavelength of 355 nm. Therefore, 355 nm LRs were obtained and discussed in this study. The transient recorder can detect signals in two modes: analog and photon counting. The analog mode is suitable for detecting strong signals at low altitude, and the photon-counting mode is suitable for detecting weak signals at high altitude. In order to better combine the advantages of the two modes to retrieve aerosol optical properties, it is necessary to glue signals of the two modes together (Newsom et al., 2009; Walker et al., 2014). The range resolution of the raw signal is 7.5 m, and the temporal resolutions are 1 min (before 25 January 2019) and 2 min (after 25 January 2019). Unless otherwise stated, the time in the paper was UTC (universal time coordinated).
2.1.2 HYSPLIT-4
HYSPLIT-4 (Hybrid Single Particle Lagrangian Integrated Trajectory Model, Version 4) is a professional model jointly developed by the National Oceanic and Atmospheric Administration (NOAA) and the Australian Bureau of Meteorology for calculating and analyzing transport and diffusion trajectories of atmospheric pollutants and has been widely used in many studies around the world (Huang et al., 2012; Noh et al., 2007). It supports the input of a variety of meteorological data, and meteorological reanalysis data provided by NOAA were used in this study.
2.1.3 MERRA-2
MERRA-2 (The Modern-Era Retrospective Analysis for Research and Applications, Version 2) is an atmospheric reanalysis dataset provided by the National Aeronautics and Space Administration (NASA) and the Global Modeling and Assimilation Office (GMAO) (Gelaro et al., 2017). The aerosol optical property data used in this study were derived from the 1 h average product of the MERRA-2 tavg1_2d_aer_Nx dataset and CO column concentration from the MERRA-2 tavg1_2d_chm_Nx. The spatial resolution of the two datasets was 0.625∘ × 0.5∘. During data processing, the 24 h data of each day were averaged to obtain daily average data.
2.1.4 ERA5
ERA5 is a global atmospheric reanalysis dataset provided by the European Centre for Medium-range Weather Forecasting (ECMWF) (Zhao et al., 2020). In recent years, some studies have evaluated the accuracy of reanalysis data provided by the ECMWF based on radiosonde data. For example, Luo et al. (2020) found that the average RH discrepancy between the ERA-Interim and radiosonde was within 10 % below 500 hPa. Song et al. (2020) found that the root mean square error (RMSE) of ERA5 RH was 3.85 % compared with the RH profile of the radiosonde. The above results show that RH from reanalysis data has good accuracy, and it has been widely used in various research fields (Sajadi et al., 2020; Tzanis et al., 2019; Xiao et al., 2020). The RH data of ERA5 used in this paper were divided into 37 layers vertically (1–1000 hPa). The temporal resolution was 1 h and the spatial resolution was 0.125∘ × 0.125∘. In order to ensure the spatial consistency, the RH data (31.25∘ N, 121.375∘ E) closest to the location of the Raman lidar were used.
2.2 Lidar data processing
Original signals need to be pre-processed before retrieval, including background subtraction, photon-counting signal dead-time correction, signal gluing, and overlap correction (D'Amico et al., 2016). The calculation of the glue coefficients in this study used the methods proposed by Newsom et al. (2009). In order to reduce the influences of lidar incompletely overlapping detection areas on retrieved results, only signals in the complete overlap area were used for retrieval. In addition, affected by the location altitude of the Raman lidar and the least square method used in the retrieval process, the lowest height of LR obtained by the Raman method was 569.5 m (a.s.l.). Since the Raman lidar used in this study can detect the Raman scattering signal of 387 nm nitrogen and signal-to-noise ratios of Raman signals in daytime are much lower than that in the nighttime, the 355 nm LR at night can be obtained through retrieval. The retrieval results of raw signals were counted by hour, and the hours with more than 15 minutes of retrieval results were regarded as effective observation hours. The retrieval results within the effective observation hours were averaged to obtain hourly average data. During the observation period, data of 667 effective observation hours were obtained through retrieval and statistics. The monthly distribution is shown in Fig. 1.
Previous studies indicated that there are some sources of errors in the retrieval. The relative errors of particle extinction coefficients caused by assumed air density profile are 1.5 % (Masonis, 2002), and the relative errors of particle backscatter coefficients caused by reference height can be 10 % (Ansmann et al., 1992). The mean deviations of particle extinction coefficients caused by signal detection are within 15 % in the 350–2000 m height range and within 20 % in the 3000–4000 m height range (Pappalardo et al., 2004). The difference is caused by different signal-to-noise ratios at low altitude and high altitude. Due to the low signal-to-noise ratio, there were usually more missing values at high altitudes.
3.1 LR temporal and vertical variations
3.1.1 Variation in the averaged LR
Figure 2a shows the averaged LR profile for 667 h. Because of the variability of aerosol particle size and microphysical properties with height (Singh et al., 2005), the averaged LR was characterized by large variability, ranging from 17 to 82 sr. LR reached a maximum at the height of 600 m and decreased with the increase in height. The averaged LR in the height range of 0.5–5 km was 41.0 ± 22.5 sr, and it was relatively small (24.8 ± 13.7 sr) above 2 km. These discrepancies were in good agreement with the results, which were mostly less than 25 sr in the altitude range of 2 km to 3 km, that Hee et al. (2016) found. LR depends on the aerosol size distribution and refractive index (Takamura et al., 1994; Young et al., 1993). The small LR may be caused by the shape effect of aerosol particles (not obviously nonspherical) and relatively low absorption efficiency (Tesche et al., 2007).
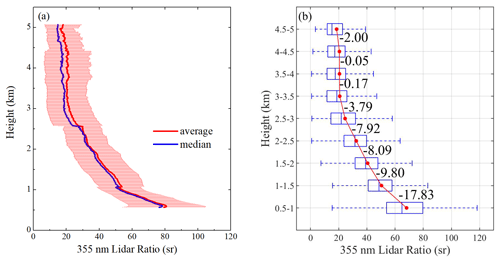
Figure 2General variation in LR. (a) Averaged profile of LR. The red line is the averaged profile, the blue line is the median profile, and the red shadow is the error bar, indicating the standard deviation. (b) Averaged LR in different altitude ranges. The red line is the line of average values at different heights. The numbers between two points are the slopes between the two points.
In order to investigate the variations in LR at different altitude ranges, Fig. 2b presents the averaged LR for different altitude ranges. The averaged LR from 0.5 to 1 km was 68.2 ± 19.5 sr, which was in good agreement with the 355 nm LR in Oklahoma, USA, observed by Ferrare et al. (2001). The mean values of LR were between 40 sr and 50 sr in the altitude range of 1–2 km, and mean values of LR were usually less than 40 sr above 2 km, which was related to low aerosol concentration and the low absorption efficiency of aerosols (Hänel et al., 2012; Hee et al., 2016). Furthermore, the slope of LR for the different height ranges as shown in Fig. 2b also gradually decreased with the increased height. Below 3 km, LR decreased rapidly with the increase in altitude, and the largest slope was −17.83 below 1.5 km. The reason for that was that temperature inversion in the low layer of the planetary boundary layer (PBL) at night weakened the vertical movement of the atmosphere and inhibited the diffusion of pollutants emitted by human activities such as vehicles and fossil fuel combustion. The accumulation of pollutants in the low layer of the PBL resulted in significant differences in aerosol vertical distribution and rapidly decreased aerosol extinction coefficients in the PBL (Liu et al., 2017; Wang et al., 2020a). However, above 3 km, the low aerosol concentration and homogeneous vertical distribution of aerosol led to small differences in LR at different height ranges.
Figure 3 shows the frequency distribution of LR for different altitude ranges. Overall, LRs were widely distributed in the altitude range of 0.5–5 km. In most cases (about 90 %) LR ranged from 10 to 80 sr with the highest frequency of 17.3 % between 40 and 50 sr. It should be noted that the number of observations trailed off at larger LR and the frequency of abnormally large LR (>90 sr) was about 4 %. LR also had a wide distribution range within 0.5–2 km, and the frequency of 40–50 sr was the highest (24.6 %), which was similar to the range of 0.5–5 km. Large LRs (>60 sr) were mainly distributed in the range of 0.5–2.0 km, suggesting that aerosols in this height range had a strong absorbing ability. Although there were a few large LRs (>60 sr) above 2 km, LRs were mainly distributed between 0–40 sr with the highest frequency of 34 % between 10 and 20 sr.
3.1.2 Temporal variations in LR
Figure 4a presents seasonal variation in LR over Shanghai during the observation period. The seasonal average LR was the largest in autumn with 47.6 ± 25.1 sr and the lowest in spring with 39.1 ± 19.6 sr. Generally, the LR of aerosol particles with stronger absorption ability is larger (Müller et al., 2007). Black carbon (BC) has a strong ability to absorb visible light (Chow et al., 2009), and aerosols rich in BC tend to show a larger LR, such as biomass burning aerosols (Giannakaki et al., 2016). Smoke produced by the burning of crop residues during the harvest season in autumn often affects Shanghai (Xu et al., 2018). According to the results found by Wang et al. (2014), the BC concentration in Shanghai was the lowest in spring and higher in autumn and winter. It indicated that the aerosol absorption ability in spring was weaker compared with that in autumn and winter.
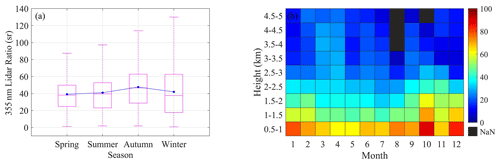
Figure 4LR temporal variations. (a) Seasonal variations in LR. The blue dots are the average for each season. (b) The average of LR at different altitudes in different months. The black areas indicate an invalid value.
The statistics of the averaged LR for a different height range in each month were shown in Fig. 4b. LR for all months decreased with the increase in altitude. The averaged LR below 2 km was the largest in October, which was attributed to smoke aerosols produced by biomass burning in the surrounding cities and rural areas during the harvest season (Nie et al., 2015). In view of LR vertical variations in different months, aerosols with LR > 40 sr were confined to within 1.5 km from March to August, while in the other months it was 2 km, especially in October and November (within 2.5 km). This revealed the point that the diffusion heights of aerosols with absorbing properties were characterized by strong seasonality. In spring and summer, the diffusion height of absorbing aerosols was lower; by contrast, it was higher in autumn and winter. The discrepancies in the seasonal height distribution of aerosols can be attributed to the monsoon climate (He et al., 2006; Liu et al., 2020b; Wang et al., 2016). Shanghai, in the southeast of China, was affected by the subtropical monsoon climate, and the prevailing winds were southeast and northwest in spring and southeast in summer (Cai et al., 2010). Clean air from the sea could reduce air pollution to a large extent by diluting pollutant concentration (Wang et al., 2014). In particular, in March and April the LR above 2.5 km was higher than that in the other months, which may be due to the influence of dust aerosols brought by the prevailing northwest wind in spring. Liu et al. (2020b) pointed out that dust aerosols from Inner Mongolia and the Gobi desert had a high frequency in spring at the altitude of 3–5 km in Shanghai. The prevailing winds in autumn and winter were northeast and northwest, respectively. Absorbing aerosols originating from north China resulted in relatively large LRs at high altitude in Shanghai.
Liu et al. (2012) reported that vast majority of aerosol particles in the Yangtze Delta region (including Shanghai) were below 2 km. In order to precisely analyze the variation characteristics of the LR in Shanghai, Fig. 5 shows LRs of 667 effective observation hours below 2 km. The abnormally large LRs (>80 sr) were usually distributed in the PBL, meaning that local emissions were the main source of strongly absorbing aerosols. From the figure, one can also conclude that the number of larger LRs within the PBL followed a decreasing trend with the passage of observation time, alluding to gradually reduced emissions of absorbing aerosols in Shanghai. The reduction was in good agreement with the reductions of BC and particulate matter (PM) concentrations caused by a series of energy-saving and emission-reduction measures such as the Shanghai Clean Air Action Plan (2018–2022) implemented by the Shanghai government in recent years (Wei et al., 2020).
3.2 Analysis of influence factors of LR
3.2.1 Reasons for LR variation with height
From Eq. (1), we found that LR was negatively correlated with P(π), and the nonspherical geometry of particles can cause a reduction in the P(π) (Müller, 2003). δ can reflect the regularity of particle shape (Novitsky and Philbrick, 2005) and is defined as the ratio of vertical backscatter coefficient and parallel backscatter coefficient (Behrendt and Nakamura, 2002). The smaller value of δ is, the closer the particle shape is to spherical (Gobbi, 1998). Since the Raman depolarization lidar used in this study can detect the 532 nm polarization signals, 532 nm δ was used to analyze the sphericity of particles. It can be seen from Fig. 6a that LR was proportional to δ with a correlation coefficient of 0.86, which was consistent with Reagan's (1988) study that LR increased with the increase in particle asphericity. LR is the ratio of the extinction coefficient and the backscatter coefficient. Therefore, there are two reasons for the increase in LR with the increasing particle asphericity. On one hand, the backscatter coefficient decreases significantly with the increase in particle asphericity; on the other hand, the extinction coefficient is sensitive to the particle cross section and is less affected by particle shape.
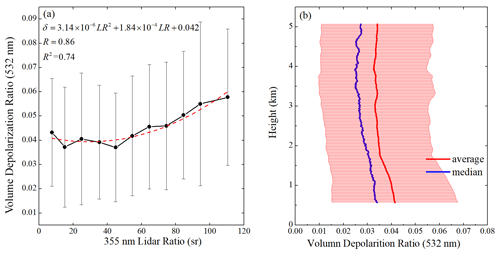
Figure 6Effect of δ on LR. (a) LR was divided into 11 intervals and the mean value of δ in each interval was calculated. The dot and error bar represent the mean and standard deviation of δ in each interval. The red dotted line is the fitting line of LR and δ. (b) The red line is the mean profile of δ, the blue line is the median profile of δ, and the red shadow is the error bar, meaning the standard deviation.
It is worth noting that LR responding to large δ was in the range of 100–120 sr. Generally, the LR of dust aerosols with a large δ was between 40 and 60 sr (Murayama et al., 2004; Noh et al., 2007). Hee et al. (2016) found that the 355 nm LR of aged forest fire aerosols was relatively large, ranging from 80 to 120 sr. And previous studies have found that some aged forest fire aerosols also showed a large depolarization ratio (Hu et al., 2019; Murayama et al., 2004). There might be two reasons for this phenomenon. One is that dust aerosols on the surface are lifted into the biomass burning plume (Müller et al., 2007) and the other is the nonsphericity of particles due to the coagulation of smoke particles during the aging process (Reid et al., 1998). Therefore, the LR in the range of 100–120 sr may correspond to large δ because of aged forest fire aerosols. In order to explore whether the decrease in LR with increasing height is affected by the particle shape effect, Fig. 6b shows the average profile of δ at lidar observation time. The average and median of δ gradually decreased with the increase in height, indicating that the particle shape became more regular with the increase in height. The result evidenced the inference by Tesche et al. (2007) that the regular particle shape was one of the reasons for the smaller LR at high altitudes.
3.2.2 Influences of aerosol sources on LR
In order to further understand the influences of wind directions on LR and its vertical distribution, a cluster analysis of back trajectories was used to study the transport of atmospheric aerosols. Based on the HYSPLIT-4 model (Franke et al., 2001; Noh et al., 2007), the 72 h backward trajectories at the height of 1000 m were shown in Fig. 7a. The cluster analysis resulted in four main air mass directions (Hänel et al., 2012; Pietruczuk and Podgorski, 2009). Backward trajectory cluster analysis based on the HYSPLIT model is widely used in atmospheric aerosol research (Wang et al., 2020a; Xu et al., 2018; Zhang et al., 2020). We performed a significance test on the cluster analysis results, and the one-way ANOVA showed that P<0.05, indicating that the LR of the four clusters referred to as 1, 2, 3, and 4 was significantly different. Similarly, there were significant differences in δ among the four clusters.
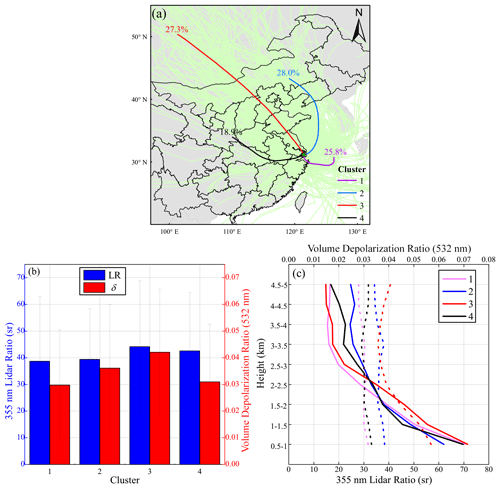
Figure 7(a) Seventy-two-hour back trajectory cluster analysis. (b) Mean values of LR and δ corresponding to different air masses. (c) The average of LR and δ at different heights corresponding to different air masses; the solid line is LR, and the dotted line is δ.
The averaged LR and δ between 0.5 and 5 km for the four clusters are shown in Fig. 7b, and Fig. 7c presents the distribution of LR and δ at different heights for the four clusters. The averaged LR (38.7 ± 24.2 sr) and δ (0.030 ± 0.021) of air mass 1 were the lowest in all clusters. As air mass 1 came from the western Pacific Ocean, it could bring abundant marine aerosols. Sea salt particles are characterized by a coarse mode and are spherical in wet conditions. In addition, marine aerosols have lower LRs than dust aerosols because of their weak absorption ability (Papagiannopoulos et al., 2018). Interestingly, the 355 nm LR of clean marine aerosols was usually between 20 and 35 sr (Alexander and Protat, 2019; Hee et al., 2016), which was smaller than that of aerosols brought by air mass 1 in the study. It was observed that the averaged LR in the range of 0.5–1 km was more than 60 sr, which suggested that clean marine aerosols from the sea might be mixed with local absorbing aerosols in Shanghai (Franke et al., 2001; Müller et al., 2007).
The averaged LR affected by the aerosols brought by air mass 2 was approximately equivalent to the LR affected by air mass 1, with an averaged value of 39.4 ± 19 sr at 0.5–5 km altitude. The source region of air mass 2 was located in Inner Mongolia, which could bring dust aerosols. During the transport over the ocean, clean marine aerosols mixed with dust aerosols led to LRs larger than that of the clean marine aerosols.
The averaged LR of air mass 3 was 44.2 ± 24.7 sr, the largest in the four clusters. As air mass 3 passed through the northern Chinese region where pollution levels and pollutant amounts were relatively high, aerosol particles brought by the air mass had a strong absorbing ability. In addition, it was evident that δ corresponding to air mass 3 below 2.5 km was larger than that of the other three air masses in Fig. 7c. The larger δ hinted at a high contribution of irregularly shaped aerosol particles. Air mass 3 passing through dust source areas of Mongolia and Inner Mongolia could bring abundant dust aerosols to Shanghai (Huang et al., 2012). Dust aerosols usually show larger particle depolarization ratios than other types of aerosols due to their irregular particle shape (Fu et al., 2009; Kai et al., 2008; Murayama et al., 1999). For example, Huang et al. (2012) found that the aerosol 532 nm depolarization ratio in the case of dust pollution was significantly greater than that in the cases of secondary inorganic pollution and biomass burning pollution. As a consequence, the larger δ corresponding to air mass 3 was attributed to dust aerosols, and long-range-transported dust aerosols could reach a height of about 2.5 km.
The averaged LR affected by aerosols from air mass 4 was 42.6 ± 21.8 sr. It should be noted that the averaged δ affected by aerosols brought by air mass 4 was small, which was comparable to that of air mass 1, indicating a high contribution of spherical aerosol particles, but LR was larger. By observing the trajectory of air mass 4, we found that it passed through Hubei Province with a high industrial level (Wang et al., 2016) and Anhui Province with heavy pollution from biomass burning (Wu et al., 2020). The industrial and smoke aerosols accompanying air mass 4 were approximately spherical (Giannakaki et al., 2016; Müller et al., 2007) and could be responsible for the smaller δ.
In summary, the variations and vertical distributions of LR and δ in Shanghai were caused by the synthetic impacts of long-range transport from different source areas and local emissions. Large LR and δ were the results of smoke and dust aerosols from the northwest. And the mixing of aerosols from the sea and locally emitted absorbing aerosols resulted in a smaller δ and slightly larger LR than that of clean marine aerosols.
3.2.3 Influence of atmospheric turbidity on LR
As mentioned previously, the vertical variations in absorbing aerosols and their influence factors played an important role in evaluating the aerosol radiation effect and studying the cause of pollution (Mishchenko et al., 2004). The LR vertical variation under different atmospheric turbidity has rarely been discussed; however, previous studies have analyzed the vertical profiles of LR in different pollution degree cases and their main concern was the averaged LR of the aerosol layer (Chen et al., 2014; Wang et al., 2016). AOD is an important parameter to characterize aerosol optical properties, which can reflect aerosol content in the atmosphere and is also an important index to evaluate atmospheric quality and visibility (Cheng et al., 2015; Hess et al., 1998; Qi et al., 2013). Previous studies have shown a positive correlation between AOD and LR by analyzing averaged LR for different AOD ranges (Ferrare et al., 2001; He et al., 2006) due to the increase in aerosol absorption and extinction caused by the increase in small particles (Takamura et al., 1994).
AOD was obtained by integrating 355 nm extinction coefficients in the range of 0.5–2 km. The averaged profile of LR below 2 km in different AOD ranges was drawn as shown in Fig. 8. Under clean conditions, LR decreased more dramatically with the increase in height. By contrast, the lack of significant vertical variability of LR under high atmospheric-turbidity conditions reflected the homogeneous vertical distribution of absorbing aerosols. The result that the vertical slope of LR presented a decreasing trend with increasing atmospheric turbidity can be explained by aerosol radiative effects on thermal structure and atmospheric stability. Under high atmospheric-turbidity conditions, aerosol particles that absorb a large amount of solar radiation during the day radiatively warm the surface at night but radiatively cool the air above the surface (Jacobson and Kaufman, 2006; Ramanathan et al., 2005). The decrease in the atmosphere stability due to the temperature difference increases vertical turbulence and results in the homogeneous vertical distribution of aerosols. By contrast, in the clear and pollution-free nights, the surface radiation cooling results in temperature inversion near the ground. The stable atmosphere is not conducive to the lifting of absorbing aerosols, resulting in a significant vertical variation in LR.
3.3 The main aerosol types causing the abnormal variation in LR
As shown in Fig. 5, abnormally large LR occurred occasionally in relatively high locations approximately above the top of PBL in spite of a usual decay trend in LR with height. To investigate the reasons, we selected 5 d with LR > 80 sr over 1 km. According to previous reports, biomass burning aerosols are relatively small and spherical, and their strong absorption ability makes them have a large LR (Papagiannopoulos et al., 2018). For example, Amiridis et al. (2009) observed smoke plumes from biomass burning over Greece and found that the 355 nm LR ranged from 40 to 100 sr. Giannakaki et al. (2016) used a Raman lidar in South Africa and found that the 355 nm LR of biomass burning aerosols was 92 ± 10 sr. Generally, the determination of aerosol type with a large LR observed by Raman lidar was based on fire data and backward trajectory models such as HYSPLIT (Hee et al., 2016; Noh et al., 2008). In our study, the spatial distribution of biomass burning tracers was used to determine whether the abnormally large LRs were related to biomass burning aerosols (Huang et al., 2012), which could lay the foundation for future research on aerosol three-dimensional spatial distribution and pollution causes.
Biomass burning is one of the important sources of PM, organic carbon (OC) and BC in the atmosphere (Wu et al., 2020). It also emits pollutant gases such as CO, SO2, NOx, and HCN (Andreae and Merlet, 2001; Kalluri et al., 2020; Randel et al., 2010). CO can be used as a tracer for biomass burning. For example, Huang et al. (2012) found that CO column concentration in the biomass burning zone was significantly different from that in the non-biomass burning zone. In addition, biomass burning would also result in high AOD and AAOD (absorbing aerosol optical depth) in the region due to strong absorption of biomass burning aerosols (Shaik et al., 2019). For example, He et al. (2015) found that 500 nm AOD increased from 0.73 to 1.00 when analyzing the smoke plume of biomass burning in Shanghai. Similarly, Vadrevu et al. (2011) found that the AOD of the wheat stalks burning season and the rice stalk burning season were both high by using satellite data in India: 0.598 and 0.58, respectively. CAOD is the optical depth of black carbon aerosols and organic carbon aerosols. Hence, 550 nm AOD, AAOD, CAOD, and CO column concentrations were used as tracers to determine whether these five cases were affected by biomass burning. Figure 9 depicts the spatial distribution of four tracers for the five cases. AOD in Shanghai during these 5 d ranged from 0.45 to 1.05, pointing to heavy aerosol pollution. The average value of AAOD was between 0.0375 and 0.1, and CAOD was between 0.12 and 0.24, which reasonably demonstrated the presence of absorbing aerosols and carbon aerosols in Shanghai (Shaik et al., 2019). Additionally, the CO column concentrations in Shanghai for these 5 d were relatively high, all more than 9 × 10−4 kg/m2, which reflected significant possibility of smoke advection. Therefore, it could be inferred that Shanghai was affected by biomass burning aerosols from local rural areas or surrounding provinces in the five cases.
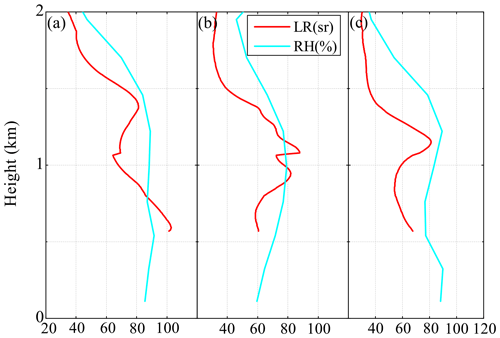
Figure 10RH and LR profiles in three cases: (a) 8 December 2017, 20:00; (b) 17 March 2018, 16:00; (c) 23 September 2018, 20:00.
Although the abnormally large LR above 1 km was mainly relevant for the advection of biomass burning aerosols, it should be noted that the increasing aerosol extinction caused by the increase in RH could also result in large LR (Salemink et al., 1984). For example, Ackerman (1998) found that the LR of continental aerosols increased from 40 to 80 sr with RH. Figure 10 presents LR and RH profiles for three cases and shows that LR was a function of RH. The abnormally large LRs above 1 km had a good corresponding relationship with high RH, which demonstrated that the abnormally larger LRs were also related to high RH.
For the first time, a long-term (2017–2019) observation based on Raman lidar was carried out in Shanghai. The aerosol 355 nm LR was retrieved, and the variations in LR and their influence factors were analyzed. In the height range of 0.5–5 km, about 90 % of LRs were distributed in 10–80 sr, with an average of 41.0 ± 22.5 sr, and LR decreased with the increase in height. The averaged LR in autumn was the largest, which was 47.6 ± 25.1 sr. The averaged LRs in summer and winter were close (41.0 ± 21.6 and 42.0 ± 27.3 sr, respectively), and the averaged LR in spring was the smallest. The seasonal variation in LR was closely related to the seasonal variations in BC concentration. In the height range of 0.5–2 km, the monthly average LR was the largest in October, which was related to biomass burning aerosols produced by burning straw in the surrounding areas during the harvest season. In addition, affected by the prevailing winds in spring and summer, the aerosols with LRs > 40 sr were confined within 1.5 km from March to August.
LR and δ were positively correlated, meaning that the more regular the particle shape is, the smaller the LR is. δ decreased with the increase in height, which proved that particle shape was one of the factors affecting the vertical distribution of LR. LR had a strong dependence on the source directions of air masses. Large LR coincided with the air masses from the northwest, while the air masses from the east led to small LR. In addition, the shape of aerosol particles was the most irregular due to aerosols brought by air masses from the northwest. The vertical distribution of LR was affected by atmospheric turbidity, and the slope of LR vertical variation decreased with the increase in AOD.
We analyzed the spatial distribution of 500 nm AOD, AAOD, CAOD, and CO column concentrations of five cases with LRs > 80 sr and found that large LRs above 1 km in Shanghai were related to biomass burning aerosols. In addition, the large LRs above 1 km could be also related to high RH.
The data presented in this paper are available from the corresponding authors upon request.
TL retrieved the data and wrote the paper. QH and YC formulated the project goals and edited and reviewed the paper. JL, QL, and WG downloaded and analyzed the reanalysis data. GH, WS, and XY revised the paper.
The authors declare that they have no conflict of interest.
We are grateful to NASA for providing MERRA-2 data and the NOAA Air Resources Laboratory (ARL) for the provision of the HYSPLIT transport and dispersion model. We also gratefully acknowledge the ECMWF for the provision of the ERA5 dataset.
This research has been supported by the National Key R&D Program of China (grant no. 2016YFC0201900), the National Natural Science Foundation of China (grant nos. 41975029, 91637101, and 91644211), the Science Research Project of Shanghai Meteorological Service (grant no. MS202016), the Chinese Ministry of Science and Technology (grant no. 2018YFC1506305), and the Fundamental Research Funds for the Central Universities (grant no. 2232019D3-27).
This paper was edited by Jianping Huang and reviewed by two anonymous referees.
Ackermann, J.: The extinction-to-backscatter ratio of tropospheric aerosol: A numerical study, J. Atmos. Ocean. Tech., 15, 1043–1050, https://doi.org/10.1175/1520-0426(1998)015<1043:Tetbro>2.0.Co;2, 1998.
Alexander, S. P. and Protat, A.: Vertical Profiling of Aerosols With a Combined Raman-Elastic Backscatter Lidar in the Remote Southern Ocean Marine Boundary Layer (43–66∘ S, 132–150∘ E), J. Geophys. Res.-Atmos., 124, 12107–12125, https://doi.org/10.1029/2019jd030628, 2019.
Amiridis, V., Balis, D. S., Giannakaki, E., Stohl, A., Kazadzis, S., Koukouli, M. E., and Zanis, P.: Optical characteristics of biomass burning aerosols over Southeastern Europe determined from UV-Raman lidar measurements, Atmos. Chem. Phys., 9, 2431–2440, https://doi.org/10.5194/acp-9-2431-2009, 2009.
Andreae, M. O. and Merlet, P.: Emission of trace gases and aerosols from biomass burning, Global Biogeochem. Cy., 15, 955–966, https://doi.org/10.1029/2000gb001382, 2001.
Ansmann, A., Riebesell, M., Wandinger, U., Weitkamp, C., Voss, E., Lahmann, W., and Michaelis, W.: Combined Raman Elastic-Backscatter Lidar for Vertical Profiling of Moisture, Aerosol Extinction, Backscatter, and Lidar Ratio, Appl. Phys. B, 55, 18–28, https://doi.org/10.1007/Bf00348608, 1992.
Behrendt, A. and Nakamura, T.: Calculation of the calibration constant of polarization lidar and its dependency on atmospheric temperature, Opt. Express, 10, 805–817, https://doi.org/10.1364/oe.10.000805, 2002.
Cai, C., Geng, F., Tie, X., Yu, Q., and An, J.: Characteristics and source apportionment of VOCs measured in Shanghai, China, Atmos. Environ., 44, 5005–5014, https://doi.org/10.1016/j.atmosenv.2010.07.059, 2010.
Chen, Z., Liu, W., Heese, B., Althausen, D., Baars, H., Cheng, T., Shu, X., and Zhang, T.: Aerosol optical properties observed by combined Raman-elastic backscatter lidar in winter 2009 in Pearl River Delta, south China, J. Geophys. Res.-Atmos., 119, 2496–2510, https://doi.org/10.1002/2013jd020200, 2014.
Cheng, T., Xu, C., Duan, J., Wang, Y., Leng, C., Tao, J., Che, H., He, Q., Wu, Y., Zhang, R., Li, X., Chen, J., Kong, L., and Yu, X.: Seasonal variation and difference of aerosol optical properties in columnar and surface atmospheres over Shanghai, Atmos. Environ., 123, 315–326, https://doi.org/10.1016/j.atmosenv.2015.05.029, 2015.
Chow, J. C., Watson, J. G., Doraiswamy, P., Chen, L.-W. A., Sodeman, D. A., Lowenthal, D. H., Park, K., Arnott, W. P., and Motallebi, N.: Aerosol light absorption, black carbon, and elemental carbon at the Fresno Supersite, California, Atmos. Res., 93, 874–887, https://doi.org/10.1016/j.atmosres.2009.04.010, 2009.
D'Amico, G., Amodeo, A., Mattis, I., Freudenthaler, V., and Pappalardo, G.: EARLINET Single Calculus Chain – technical – Part 1: Pre-processing of raw lidar data, Atmos. Meas. Tech., 9, 491–507, https://doi.org/10.5194/amt-9-491-2016, 2016.
Fan, S., Liu, C., Xie, Z., Dong, Y., Hu, Q., Fan, G., Chen, Z., Zhang, T., Duan, J., Zhang, P., and Liu, J.: Scanning vertical distributions of typical aerosols along the Yangtze River using elastic lidar, Sci. Total Environ., 628/629, 631–641, https://doi.org/10.1016/j.scitotenv.2018.02.099, 2018.
Fernald, F. G.: Analysis of atmospheric lidar observations: some comments, OSA Proc., 23, 652–653, 1984.
Ferrare, R. A., Turner, D. D., Brasseur, L. H., Feltz, W. F., Dubovik, O., and Tooman, T. P.: Raman lidar measurements of the aerosol extinction-to-backscatter ratio over the Southern Great Plains, J. Geophys. Res.-Atmos., 106, 20333–20347, https://doi.org/10.1029/2000jd000144, 2001.
Franke, K., Ansmann, A., Muller, D., Althausen, D., Wagner, A., and Scheele, R.: One-year observations of particle lidar ratio over the tropical Indian Ocean with Raman lidar, Geophys. Res. Lett., 28, 4559–4562, https://doi.org/10.1029/2001gl013671, 2001.
Fu, Q., Thorsen, T. J., Su, J., Ge, J. M., and Huang, J. P.: Test of Mie-based single-scattering properties of non-spherical dust aerosols in radiative flux calculations, J. Quant. Spectrosc. Ra., 110, 1640–1653, https://doi.org/10.1016/j.jqsrt.2009.03.010, 2009.
Gelaro, R., McCarty, W., Suarez, M. J., Todling, R., Molod, A., Takacs, L., Randles, C., Darmenov, A., Bosilovich, M. G., Reichle, R., Wargan, K., Coy, L., Cullather, R., Draper, C., Akella, S., Buchard, V., Conaty, A., da Silva, A., Gu, W., Kim, G. K., Koster, R., Lucchesi, R., Merkova, D., Nielsen, J. E., Partyka, G., Pawson, S., Putman, W., Rienecker, M., Schubert, S. D., Sienkiewicz, M., and Zhao, B.: The Modern-Era Retrospective Analysis for Research and Applications, Version 2 (MERRA-2), J. Climate, 30, 5419–5454, https://doi.org/10.1175/JCLI-D-16-0758.1, 2017.
Giannakaki, E., van Zyl, P. G., Müller, D., Balis, D., and Komppula, M.: Optical and microphysical characterization of aerosol layers over South Africa by means of multi-wavelength depolarization and Raman lidar measurements, Atmos. Chem. Phys., 16, 8109–8123, https://doi.org/10.5194/acp-16-8109-2016, 2016.
Gobbi, G. P.: Polarization lidar returns from aerosols and thin clouds: a framework for the analysis, Appl. Opt., 37, 5505–5508, https://doi.org/10.1364/ao.37.005505, 1998.
Gong, W., Liu, B., Ma, Y., and Zhang, M.: Mie LIDAR Observations of Tropospheric Aerosol over Wuhan, Atmosphere, 6, 1129–1140, https://doi.org/10.3390/atmos6081129, 2015.
Hänel, A., Baars, H., Althausen, D., Ansmann, A., Engelmann, R., and Sun, J. Y.: One-year aerosol profiling with EUCAARI Raman lidar at Shangdianzi GAW station: Beijing plume and seasonal variations, J. Geophys. Res.-Atmos., 117, D13201, https://doi.org/10.1029/2012jd017577, 2012.
He, Q., Zhao, X., Lu, J., Zhou, G., Yang, H., Gao, W., Yu, W., and Cheng, T.: Impacts of biomass-burning on aerosol properties of a severe haze event over Shanghai, Particuology, 20, 52–60, https://doi.org/10.1016/j.partic.2014.11.004, 2015.
He, Q. S., Li, C. C., Mao, J. T., Lau, A. K. H., and Li, P. R.: A study on the aerosol extinction-to-backscatter ratio with combination of micro-pulse LIDAR and MODIS over Hong Kong, Atmos. Chem. Phys., 6, 3243–3256, https://doi.org/10.5194/acp-6-3243-2006, 2006.
Hee, W. S., Lim, H. S., Jafri, M. Z. M., Lolli, S., and Ying, K. W.: Vertical Profiling of Aerosol Types Observed across Monsoon Seasons with a Raman Lidar in Penang Island, Malaysia, Aerosol Air Qual. Res., 16, 2843–2854, https://doi.org/10.4209/aaqr.2015.07.0450, 2016.
Hess, M., Koepke, P., and Schult, I.: Optical properties of aerosols and clouds: The software package OPAC, B. Am. Meteorol. Soc., 79, 831–844, https://doi.org/10.1175/1520-0477(1998)079<0831:Opoaac>2.0.Co;2, 1998.
Hu, Q., Goloub, P., Veselovskii, I., Bravo-Aranda, J.-A., Popovici, I. E., Podvin, T., Haeffelin, M., Lopatin, A., Dubovik, O., Pietras, C., Huang, X., Torres, B., and Chen, C.: Long-range-transported Canadian smoke plumes in the lower stratosphere over northern France, Atmos. Chem. Phys., 19, 1173–1193, https://doi.org/10.5194/acp-19-1173-2019, 2019.
Huang, J., Lin, B., Minnis, P., Wang, T., Wang, X., Hu, Y., Yi, Y., and Ayers, J. K.: Satellite-based assessment of possible dust aerosols semi-direct effect on cloud water path over East Asia, Geophys. Res. Lett., 33, L19802, https://doi.org/10.1029/2006gl026561, 2006.
Huang, J. P., Wang, T. H., Wang, W. C., Li, Z. Q., and Yan, H. R.: Climate effects of dust aerosols over East Asian arid and semiarid regions, J. Geophys. Res.-Atmos., 119, 11398–11416, https://doi.org/10.1002/2014jd021796, 2014.
Huang, K., Zhuang, G., Lin, Y., Fu, J. S., Wang, Q., Liu, T., Zhang, R., Jiang, Y., Deng, C., Fu, Q., Hsu, N. C., and Cao, B.: Typical types and formation mechanisms of haze in an Eastern Asia megacity, Shanghai, Atmos. Chem. Phys., 12, 105–124, https://doi.org/10.5194/acp-12-105-2012, 2012.
Jacobson, M. Z.: Studying the effects of aerosols on vertical photolysis rate coefficient and temperature profiles over an urban airshed, J. Geophys. Res.-Atmos., 103, 10593–10604, https://doi.org/10.1029/98jd00287, 1998.
Jacobson, M. Z. and Kaufman, Y. J.: Wind reduction by aerosol particles, Geophys. Res. Lett., 33, L24814, https://doi.org/10.1029/2006gl027838, 2006.
Kai, K., Nagata, Y., Tsunematsu, N., Matsumura, T., Kim, H.-S., Matsumoto, T., Hu, S., Zhou, H., Abo, M., and Nagai, T.: The Structure of the Dust Layer over the Taklimakan Deser during the Dust Storm in April 2002 as Observed Using a Depolarization Lidar, J. Meteorol. Soc. Jpn., 86, 1–16, https://doi.org/10.2151/jmsj.86.1, 2008.
Kalluri, R. O. R., Zhang, X., Bi, L., Zhao, J., Yu, L., and Kotalo, R. G.: Carbonaceous aerosol emission reduction over Shandong province and the impact of air pollution control as observed from synthetic satellite data, Atmos. Environ., 222, 117150, https://doi.org/10.1016/j.atmosenv.2019.117150, 2020.
Kim, M.-H., Omar, A. H., Tackett, J. L., Vaughan, M. A., Winker, D. M., Trepte, C. R., Hu, Y., Liu, Z., Poole, L. R., Pitts, M. C., Kar, J., and Magill, B. E.: The CALIPSO version 4 automated aerosol classification and lidar ratio selection algorithm, Atmos. Meas. Tech., 11, 6107–6135, https://doi.org/10.5194/amt-11-6107-2018, 2018.
Liu, D., Kanitz, T., Ciapponi, A., Mondello, A., D'Ottavi, A., Mateo, A. B., Straume, A.-G., Voland, C., Bon, D., Checa, E., Alvarez, E., Bellucci, I., Do Carmo, J. P., Brewster, J., Marshall, J., Schillinger, M., Hannington, M., Rennie, M., Reitebuch, O., Lecrenier, O., Bravetti, P., Sacchieri, V., De Sanctis, V., Lefebvre, A., Parrinello, T., Wernham, D., Wang, Y., Wu, Y., Gross, B., and Moshary, F.: ESA’s Lidar Missions Aeolus and EarthCARE, The 29th International Laser Radar Conference (ILRC 29), 24-28 June 2019, Hefei, Peoples R China, 237, 01006, https://doi.org/10.1051/epjconf/202023701006, 2020a.
Liu, J., Zheng, Y., Li, Z., Flynn, C., and Cribb, M.: Seasonal variations of aerosol optical properties, vertical distribution and associated radiative effects in the Yangtze Delta region of China, J. Geophys. Res.-Atmos., 117, D00K38, https://doi.org/10.1029/2011jd016490, 2012.
Liu, Q., He, Q., Fang, S., Guang, Y., Ma, C., Chen, Y., Kang, Y., Pan, H., Zhang, H., and Yao, Y.: Vertical distribution of ambient aerosol extinctive properties during haze and haze-free periods based on the Micro-Pulse Lidar observation in Shanghai, Sci. Total Environ., 574, 1502–1511, https://doi.org/10.1016/j.scitotenv.2016.08.152, 2017.
Liu, Q., Liu, X., Liu, T., Kang, Y., Chen, Y., Li, J., and Zhang, H.: Seasonal variation in particle contribution and aerosol types in Shanghai based on satellite data from MODIS and CALIOP, Particuology, 51, 18–25, https://doi.org/10.1016/j.partic.2019.10.001, 2020b.
Liu, Y., Zhu, Q., Huang, J., Hua, S., and Jia, R.: Impact of dust-polluted convective clouds over the Tibetan Plateau on downstream precipitation, Atmos. Environ., 209, 67–77, https://doi.org/10.1016/j.atmosenv.2019.04.001, 2019a.
Liu, Y., Hua, S., Jia, R., and Huang, J. P.: Effect of Aerosols on the Ice Cloud Properties Over the Tibetan Plateau, J. Geophys. Res.-Atmos., 124, 9594–9608, https://doi.org/10.1029/2019jd030463, 2019b.
Lu, X., Mao, F., Pan, Z., Gong, W., Zhu, Y., and Yang, J.: Enhancement of Atmospheric Stability by Anomalous Elevated Aerosols During Winter in China, J. Geophys. Res.-Atmos., 125, e2019JD031734, https://doi.org/10.1029/2019jd031734, 2020.
Luo, B., Minnett, P. J., Szczodrak, M., Nalli, N. R., and Morris, V. R.: Accuracy Assessment of MERRA-2 and ERA-Interim Sea Surface Temperature, Air Temperature, and Humidity Profiles over the Atlantic Ocean Using AEROSE Measurements, J. Climate, 33, 6889–6909, https://doi.org/10.1175/jcli-d-19-0955.1, 2020.
Lv, L., Xiang, Y., Zhang, T., Chai, W., and Liu, W.: Comprehensive study of regional haze in the North China Plain with synergistic measurement from multiple mobile vehicle-based lidars and a lidar network, Sci. Total Environ., 721, 137773, https://doi.org/10.1016/j.scitotenv.2020.137773, 2020.
Ma, X., Wang, C., Han, G., Ma, Y., Li, S., Gong, W., and Chen, J.: Regional Atmospheric Aerosol Pollution Detection Based on LiDAR Remote Sensing, Remote Sens., 11, 2339, https://doi.org/10.3390/rs11202339, 2019.
Masonis, S. J.: An intercomparison of aerosol light extinction and 180∘ backscatter as derived using in situ instruments and Raman lidar during the INDOEX field campaign, J. Geophys. Res., 107, 8014, https://doi.org/10.1029/2000jd000035, 2002.
McComiskey, A., Schwartz, S. E., Schmid, B., Guan, H., Lewis, E. R., Ricchiazzi, P., and Ogren, J. A.: Direct aerosol forcing: Calculation from observables and sensitivities to inputs, J. Geophys. Res., 113, D09202, https://doi.org/10.1029/2007jd009170, 2008.
Mehta, M., Singh, N., and Anshumali: Global trends of columnar and vertically distributed properties of aerosols with emphasis on dust, polluted dust and smoke – inferences from 10-year long CALIOP observations, Remote Sens. Environ., 208, 120–132, https://doi.org/10.1016/j.rse.2018.02.017, 2018.
Mishchenko, M. I., Cairns, B., Hansen, J. E., Travis, L. D., Burg, R., Kaufman, Y. J., Vanderlei Martins, J., and Shettle, E. P.: Monitoring of aerosol forcing of climate from space: analysis of measurement requirements, J. Quant. Spectrosc. Ra., 88, 149–161, https://doi.org/10.1016/j.jqsrt.2004.03.030, 2004.
Müller, D.: Saharan dust over a central European EARLINET-AERONET site: Combined observations with Raman lidar and Sun photometer, J. Geophys. Res., 108, 4345, https://doi.org/10.1029/2002jd002918, 2003.
Müller, D., Ansmann, A., Mattis, I., Tesche, M., Wandinger, U., Althausen, D., and Pisani, G.: Aerosol-type-dependent lidar ratios observed with Raman lidar, J. Geophys. Res., 112, D16202, https://doi.org/10.1029/2006jd008292, 2007.
Murayama, T., Okamoto, H., Kaneyasu, N., Kamataki, H., and Miura, K.: Application of lidar depolarization measurement in the atmospheric boundary layer: Effects of dust and sea-salt particles, J. Geophys. Res.-Atmos., 104, 31781–31792, https://doi.org/10.1029/1999jd900503, 1999.
Murayama, T., Müller, D., Wada, K., Shimizu, A., Sekiguchi, M., and Tsukamoto, T.: Characterization of Asian dust and Siberian smoke with multi-wavelength Raman lidar over Tokyo, Japan in spring 2003, Geophys. Res. Lett., 31, L23103, https://doi.org/10.1029/2004gl021105, 2004.
Newsom, R. K., Turner, D. D., Mielke, B., Clayton, M., Ferrare, R., and Sivaraman, C.: Simultaneous analog and photon counting detection for Raman lidar, Appl. Opt., 48, 3903–3914, https://doi.org/10.1364/ao.48.003903, 2009.
Nicolae, D., Donovan, D., Zadelhoff, G.-J., Daou, D., Wandinger, U., Makoto, A., Vassilis, A., Balis, D., Behrendt, A., Comeron, A., Gibert, F., Landulfo, E., McCormick, M. P., Senff, C., Veselovskii, I., and Wandinger, U.: Earthcare atlid extinction and backscatter retrieval algorithms, The 28th International Laser Radar Conference, 25–30 June 2017, Politehnica Univ Bucharest, Bucharest, ROMANIA, 176, 02022, https://doi.org/10.1051/epjconf/201817602022, 2018.
Nie, W., Ding, A. J., Xie, Y. N., Xu, Z., Mao, H., Kerminen, V.-M., Zheng, L. F., Qi, X. M., Huang, X., Yang, X.-Q., Sun, J. N., Herrmann, E., Petäjä, T., Kulmala, M., and Fu, C. B.: Influence of biomass burning plumes on HONO chemistry in eastern China, Atmos. Chem. Phys., 15, 1147–1159, https://doi.org/10.5194/acp-15-1147-2015, 2015.
Noh, Y. M., Kim, Y. J., Choi, B. C., and Murayama, T.: Aerosol lidar ratio characteristics measured by a multi-wavelength Raman lidar system at Anmyeon Island, Korea, Atmos. Res., 86, 76–87, https://doi.org/10.1016/j.atmosres.2007.03.006, 2007.
Noh, Y. M., Kim, Y. J., and Müller, D.: Seasonal characteristics of lidar ratios measured with a Raman lidar at Gwangju, Korea in spring and autumn, Atmos. Environ., 42, 2208–2224, https://doi.org/10.1016/j.atmosenv.2007.11.045, 2008.
Novitsky, E. J. and Philbrick, C. R.: Multistatic lidar profiling of urban atmospheric aerosols, J. Geophys. Res.-Atmos., 110, D07S11, https://doi.org/10.1029/2004jd004723, 2005.
Omar, A. H., Winker, D. M., Vaughan, M. A., Hu, Y., Trepte, C. R., Ferrare, R. A., Lee, K.-P., Hostetler, C. A., Kittaka, C., Rogers, R. R., Kuehn, R. E., and Liu, Z.: The CALIPSO Automated Aerosol Classification and Lidar Ratio Selection Algorithm, J. Atmos. Ocean. Tech., 26, 1994–2014, https://doi.org/10.1175/2009jtecha1231.1, 2009.
Painemal, D., Clayton, M., Ferrare, R., Burton, S., Josset, D., and Vaughan, M.: Novel aerosol extinction coefficients and lidar ratios over the ocean from CALIPSO–CloudSat: evaluation and global statistics, Atmos. Meas. Tech., 12, 2201–2217, https://doi.org/10.5194/amt-12-2201-2019, 2019.
Papagiannopoulos, N., Mona, L., Amodeo, A., D'Amico, G., Gumà Claramunt, P., Pappalardo, G., Alados-Arboledas, L., Guerrero-Rascado, J. L., Amiridis, V., Kokkalis, P., Apituley, A., Baars, H., Schwarz, A., Wandinger, U., Binietoglou, I., Nicolae, D., Bortoli, D., Comerón, A., Rodríguez-Gómez, A., Sicard, M., Papayannis, A., and Wiegner, M.: An automatic observation-based aerosol typing method for EARLINET, Atmos. Chem. Phys., 18, 15879–15901, https://doi.org/10.5194/acp-18-15879-2018, 2018.
Pappalardo, G., Amodeo, A., Pandolfi, M., Wandinger, U., Ansmann, A., Bosenberg, J., Matthias, V., Amiridis, V., De Tomasi, F., Frioud, M., Larlori, M., Komguem, L., Papayannis, A., Rocadenbosch, F., and Wang, X.: Aerosol lidar intercomparison in the framework of the EARLINET project. 3. Raman lidar algorithm for aerosol extinction, backscatter, and lidar ratio, Appl. Opt., 43, 5370–5385, https://doi.org/10.1364/ao.43.005370, 2004.
Pietruczuk, A. and Podgorski, J.: The lidar ratio derived from sun-photometer measurements at Belsk Geophysical Observatory, Acta Geophys., 57, 476–493, https://doi.org/10.2478/s11600-009-0006-9, 2009.
Qi, Y., Ge, J., and Huang, J.: Spatial and temporal distribution of MODIS and MISR aerosol optical depth over northern China and comparison with AERONET, Chinese Sci. Bull., 58, 2497–2506, https://doi.org/10.1007/s11434-013-5678-5, 2013.
Ramanathan, V., Chung, C., Kim, D., Bettge, T., Buja, L., Kiehl, J. T., Washington, W. M., Fu, Q., Sikka, D. R., and Wild, M.: Atmospheric brown clouds: impacts on South Asian climate and hydrological cycle, P. Natl. Acad. Sci. USA, 102, 5326–5333, https://doi.org/10.1073/pnas.0500656102, 2005.
Randel, W. J., Park, M., Emmons, L., Kinnison, D., Bernath, P., Walker, K. A., Boone, C., and Pumphrey, H.: Asian monsoon transport of pollution to the stratosphere, Science, 328, 611–613, https://doi.org/10.1126/science.1182274, 2010.
Reagan, J. A., Apte, M. V., Ben-David, A., and Herman, B. M.: Assessment of Aerosol Extinction to Backscatter Ratio Measurements Made at 694.3 Nm in Tucson, Arizona, Aerosol Sci. Tech., 8, 215–226, https://doi.org/10.1080/02786828808959184, 1988.
Reid, J. S., Hobbs, P. V., Ferek, R. J., Blake, D. R., Martins, J. V., Dunlap, M. R., and Liousse, C.: Physical, chemical, and optical properties of regional hazes dominated by smoke in Brazil, J. Geophys. Res.-Atmos., 103, 32059–32080, https://doi.org/10.1029/98jd00458, 1998.
Sajadi, M. M., Habibzadeh, P., Vintzileos, A., Shokouhi, S., Miralles-Wilhelm, F., and Amoroso, A.: Temperature, Humidity, and Latitude Analysis to Estimate Potential Spread and Seasonality of Coronavirus Disease 2019 (COVID-19), JAMA Netw. Open., 3, e2011834, https://doi.org/10.1001/jamanetworkopen.2020.11834, 2020.
Salemink, H. W. M., Schotanus, P., and Bergwerff, J. B.: Quantitative Lidar at 532nm for Vertical Extinction Profiles and the Effect of Relative Humidity, Appl. Phys. B, 34, 187–189, https://doi.org/10.1007/BF00697633, 1984.
Shaik, D. S., Kant, Y., Mitra, D., Singh, A., Chandola, H. C., Sateesh, M., Babu, S. S., and Chauhan, P.: Impact of biomass burning on regional aerosol optical properties: A case study over northern India, J. Environ. Manage., 244, 328–343, https://doi.org/10.1016/j.jenvman.2019.04.025, 2019.
Sicard, M., Rocadenbosch, F., Reba, M. N. M., Comerón, A., Tomás, S., García-Vízcaino, D., Batet, O., Barrios, R., Kumar, D., and Baldasano, J. M.: Seasonal variability of aerosol optical properties observed by means of a Raman lidar at an EARLINET site over Northeastern Spain, Atmos. Chem. Phys., 11, 175–190, https://doi.org/10.5194/acp-11-175-2011, 2011.
Singh, U. N., Pappalardo, G., Mizutani, K., Amodeo, A., Mona, L., and Pandolfi, M.: Systematic measurements of the aerosol extinction-to-backscatter ratio, P. Soc. Photo-Opt. Ins., 5653, 77–87, 2005.
Song, H.-J., Kim, S., Lee, H., and Kim, K.-H.: Climatology of Tropospheric Relative Humidity over the Korean Peninsula from Radiosonde and ECMWF Reanalysis, Atmosphere, 11, 704, https://doi.org/10.3390/atmos11070704, 2020.
Takamura, T., Sasano, Y., and Hayasaka, T.: Tropospheric aerosol optical properties derived from lidar, sun photometer, and optical particle counter measurements, Appl. Opt., 33, 7132–7140, https://doi.org/10.1364/AO.33.007132, 1994.
Tesche, M., Ansmann, A., Muller, D., Althausen, D., Engelmann, R., Hu, M., and Zhang, Y.: Particle backscatter, extinction, and lidar ratio profiling with Raman lidar in south and north China, Appl. Opt., 46, 6302–6308, https://doi.org/10.1364/ao.46.006302, 2007.
Tzanis, C. G., Koutsogiannis, I., Philippopoulos, K., and Deligiorgi, D.: Recent climate trends over Greece, Atmos. Res., 230, 104623, https://doi.org/10.1016/j.atmosres.2019.104623, 2019.
Vadrevu, K. P., Ellicott, E., Badarinath, K. V., and Vermote, E.: MODIS derived fire characteristics and aerosol optical depth variations during the agricultural residue burning season, north India, Environ. Pollut., 159, 1560–1569, https://doi.org/10.1016/j.envpol.2011.03.001, 2011.
Walker, M., Venable, D., and Whiteman, D. N.: Gluing for Raman lidar systems using the lamp mapping technique, Appl. Opt., 53, 8535–8543, https://doi.org/10.1364/AO.53.008535, 2014.
Wandinger, U., Freudenthaler, V., Baars, H., Amodeo, A., Engelmann, R., Mattis, I., Groß, S., Pappalardo, G., Giunta, A., D'Amico, G., Chaikovsky, A., Osipenko, F., Slesar, A., Nicolae, D., Belegante, L., Talianu, C., Serikov, I., Linné, H., Jansen, F., Apituley, A., Wilson, K. M., de Graaf, M., Trickl, T., Giehl, H., Adam, M., Comerón, A., Muñoz-Porcar, C., Rocadenbosch, F., Sicard, M., Tomás, S., Lange, D., Kumar, D., Pujadas, M., Molero, F., Fernández, A. J., Alados-Arboledas, L., Bravo-Aranda, J. A., Navas-Guzmán, F., Guerrero-Rascado, J. L., Granados-Muñoz, M. J., Preißler, J., Wagner, F., Gausa, M., Grigorov, I., Stoyanov, D., Iarlori, M., Rizi, V., Spinelli, N., Boselli, A., Wang, X., Lo Feudo, T., Perrone, M. R., De Tomasi, F., and Burlizzi, P.: EARLINET instrument intercomparison campaigns: overview on strategy and results, Atmos. Meas. Tech., 9, 1001–1023, https://doi.org/10.5194/amt-9-1001-2016, 2016.
Wang, H., He, Q., Chen, Y., and Kang, Y.: Characterization of black carbon concentrations of haze with different intensities in Shanghai by a three-year field measurement, Atmos. Environ., 99, 536–545, https://doi.org/10.1016/j.atmosenv.2014.10.025, 2014.
Wang, L., Lyu, B., and Bai, Y.: Aerosol vertical profile variations with seasons, air mass movements and local PM2.5 levels in three large China cities, Atmos. Environ., 224, 117329, https://doi.org/10.1016/j.atmosenv.2020.117329, 2020a.
Wang, T., Han, Y., Huang, J., Sun, M., Jian, B., Huang, Z., and Yan, H.: Climatology of Dust-Forced Radiative Heating Over the Tibetan Plateau and Its Surroundings, J. Geophys. Res.-Atmos., 125, e2020JD032942, https://doi.org/10.1029/2020jd032942, 2020b.
Wang, W., Huang, J., Zhou, T., Bi, J., Lin, L., Chen, Y., Huang, Z., and Su, J.: Estimation of radiative effect of a heavy dust storm over northwest China using Fu-Liou model and ground measurements, J. Quant. Spectrosc. Ra., 122, 114–126, https://doi.org/10.1016/j.jqsrt.2012.10.018, 2013.
Wang, W., Gong, W., Mao, F., Pan, Z., and Liu, B.: Measurement and Study of Lidar Ratio by Using a Raman Lidar in Central China, Int. J. Environ. Res. Pu., 13, 508, https://doi.org/10.3390/ijerph13050508, 2016.
Wei, C., Wang, M. H., Fu, Q. Y., Dai, C., Huang, R., and Bao, Q.: Temporal Characteristics and Potential Sources of Black Carbon in Megacity Shanghai, China, J. Geophys. Res.-Atmos., 125, e2019JD031827, https://doi.org/10.1029/2019jd031827, 2020.
Welton, E. J., Campbell, J. R., Spinhirne, J. D., and Scott, V. S.: Global monitoring of clouds and aerosols using a network of micro-pulse lidar systems, Proc. Spie., 4153, 151–158, https://doi.org/10.1117/12.417040, 2001.
Wu, J., Kong, S., Wu, F., Cheng, Y., Zheng, S., Qin, S., Liu, X., Yan, Q., Zheng, H., Zheng, M., Yan, Y., Liu, D., Ding, S., Zhao, D., Shen, G., Zhao, T., and Qi, S.: The moving of high emission for biomass burning in China: View from multi-year emission estimation and human-driven forces, Environ. Int., 142, 105812, https://doi.org/10.1016/j.envint.2020.105812, 2020.
Xiao, M., Yu, Z., Kong, D., Gu, X., Mammarella, I., Montagnani, L., Arain, M. A., Merbold, L., Magliulo, V., Lohila, A., Buchmann, N., Wolf, S., Gharun, M., Hörtnagl, L., Beringer, J., and Gioli, B.: Stomatal response to decreased relative humidity constrains the acceleration of terrestrial evapotranspiration, Environ. Res. Lett., 15, 094066, https://doi.org/10.1088/1748-9326/ab9967, 2020.
Xu, J., Wang, Q., Deng, C., McNeill, V. F., Fankhauser, A., Wang, F., Zheng, X., Shen, J., Huang, K., and Zhuang, G.: Insights into the characteristics and sources of primary and secondary organic carbon: High time resolution observation in urban Shanghai, Environ. Pollut., 233, 1177–1187, https://doi.org/10.1016/j.envpol.2017.10.003, 2018.
Yan, H. and Wang, T.: Ten Years of Aerosol Effects on Single-Layer Overcast Clouds over the US Southern Great Plains and the China Loess Plateau, Adv. Meteorol., 2020, 1–15, https://doi.org/10.1155/2020/6719160, 2020.
Young, S. A., Cutten, D. R., Lynch, M. J., and Davies, J. E.: Lidar-Derived Variations in the Backscatter-to-Extinction Ratio in Southern-Hemisphere Coastal Maritime Aerosols, Atmos. Environ., 27, 1541–1551, https://doi.org/10.1016/0960-1686(93)90154-Q, 1993.
Zarzycki, C. M. and Bond, T. C.: How much can the vertical distribution of black carbon affect its global direct radiative forcing?, Geophys. Res. Lett., 37, L20807, https://doi.org/10.1029/2010gl044555, 2010.
Zhang, L., Qiao, L., Lan, J., Yan, Y., and Wang, L.: Three-years monitoring of PM2.5 and scattering coefficients in Shanghai, China, Chemosphere, 253, 126613, https://doi.org/10.1016/j.chemosphere.2020.126613, 2020.
Zhao, H., Mao, J. D., Zhou, C. Y., and Gong, X.: A method of determining multi-wavelength lidar ratios combining aerodynamic particle sizer spectrometer and sun-photometer, J. Quant. Spectrosc. Ra., 217, 224–228, https://doi.org/10.1016/j.jqsrt.2018.05.030, 2018.
Zhao, L., Wang, W., Hao, T., Qu, W., Sheng, L., Luo, C., An, X., and Zhou, Y.: The autumn haze-fog episode enhanced by the transport of dust aerosols in the Tianjin area, Atmos. Environ., 237, 117669, https://doi.org/10.1016/j.atmosenv.2020.117669, 2020.