the Creative Commons Attribution 4.0 License.
the Creative Commons Attribution 4.0 License.
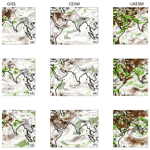
Sensitivity of modeled Indian monsoon to Chinese and Indian aerosol emissions
Peter Sherman
Shaojie Song
Alex T. Archibald
Nathan Luke Abraham
Jean-François Lamarque
Drew Shindell
Gregory Faluvegi
Michael B. McElroy
The South Asian summer monsoon supplies over 80 % of India's precipitation. Industrialization over the past few decades has resulted in severe aerosol pollution in India. Understanding monsoonal sensitivity to aerosol emissions in general circulation models (GCMs) could improve predictability of observed future precipitation changes. The aims here are (1) to assess the role of aerosols in India's monsoon precipitation and (2) to determine the roles of local and regional emissions. For (1), we study the Precipitation Driver Response Model Intercomparison Project experiments. We find that the precipitation response to changes in black carbon is highly uncertain with a large intermodel spread due in part to model differences in simulating changes in cloud vertical profiles. Effects from sulfate are clearer; increased sulfate reduces Indian precipitation, a consistency through all of the models studied here. For (2), we study bespoke simulations, with reduced Chinese and/or Indian emissions in three GCMs. A significant increase in precipitation (up to ∼20 %) is found only when both countries' sulfur emissions are regulated, which has been driven in large part by dynamic shifts in the location of convective regions in India. These changes have the potential to restore a portion of the precipitation losses induced by sulfate forcing over the last few decades.
- Article
(4682 KB) - Full-text XML
-
Supplement
(7215 KB) - BibTeX
- EndNote
The aims here are to assess the role of aerosols in India's monsoon precipitation and to determine the relative contributions from Chinese and Indian emissions using CMIP6 models. We find that increased sulfur emissions reduce precipitation, which is primarily dynamically driven due to spatial shifts in convection over the region. A significant increase in precipitation (up to ∼20 %) is found only when both Indian and Chinese sulfate emissions are regulated.
The South Asian summer monsoon is the dominant weather pattern over India, lasting typically from June to September. Over this period, southwesterly winds transport warm, moist air from the Arabian Sea onto the Indian subcontinent, supplying roughly 80 % of the region's annual rainfall (Turner and Annamalai, 2012). Since the monsoon provides such a significant source for India's water supply, changes in its strength and position would have important socioeconomic implications including though not simply confined to agricultural production (Kumar et al., 2004; Douglas et al., 2009) and drought frequency (Subbiah, 2004). Given the rugged orography of the surrounding region and difficulties in modeling intense precipitation, resolving the future roles of natural variability and the externally forced signal for the monsoon is a fundamentally difficult – but important – problem.
Interannual changes in the monsoon have been linked to internal (natural) variability inherent to the climate system. For instance, numerous studies have found a potential connection between variability in the El Niño–Southern Oscillation and the monsoon (Sikka, 1980; Shukla and Paolino, 1983; Annamalai and Liu, 2005). Such links could be used to improve predictability of Indian rainfall. While internal variability likely plays a non-negligible role in modulating the South Asian summer monsoon – and is expected to continue to do so in the future, even in high-emissions scenarios (Annamalai et al., 2007) – changes in the monsoon's mean state associated with external forcings are also of fundamental importance. Specifically, determining the anthropogenic impacts on monsoonal changes associated with emissions of greenhouse gases (GHGs) and aerosols can provide critical insights that can help better inform policymaking decisions regarding emission regulations.
The steady rise in GHGs over the 20th century has increased the atmosphere's average temperature and water vapor content through the Clausius–Clapeyron relation, and it might be expected as a result to contribute to increased rainfall events over India (Goswami et al., 2006; Turner and Slingo, 2009; Salzmann et al., 2014). CMIP6 models run with just an increase in CO2 forcing generally exhibit such an increase uniformly across India (Fig. S1 in the Supplement). However, in reality the picture is more complex as the literature has indicated no such observed trend for India over the last half century (Ramesh and Goswami, 2014; Saha and Ghosh, 2019). Observed monsoon precipitation aggregated over all of continental India (Fig. 1) actually indicates a slight drying trend over the last few decades. While these trends are not statistically significant at a 95 % confidence level, the purpose of Fig. 1 is to illustrate that the increase in monsoon precipitation expected from the growing greenhouse forcing has certainly not been detected. There may be several mechanisms invoked to explain why Indian monsoon precipitation has not increased. Land use changes over the Indo-Gangetic Plain have been implicated as one of the causes, where decreased evapotranspiration may have limited the amount of available precipitable water in the region (Paul et al., 2016). It has been shown also that aerosol effects have counterbalanced the precipitation changes attributable to the greenhouse warming (Bollasina et al., 2011; Turner and Annamalai, 2012; Westervelt et al., 2020). Ramanathan et al. (2005) found that aerosols over India reduce surface shortwave radiation, which limits the amount of evaporation and thereby reduces monsoon precipitation. Additionally, it has been shown that the atmospheric brown cloud (originally so termed in Ramanathan and Crutzen (2003), referring to the pervasive light-absorbing aerosol layer akin to the stratocumulus cloud decks observed over the oceans) over the northern Indian Ocean is associated with a stable atmosphere that limits convection. Atmospheric brown clouds consist primarily of black and organic carbon, dust, and other anthropogenic aerosols. Sources of aerosols and their precursors in South and East Asia (indicated in Fig. S2) are tied particularly to energy production and biomass combustion, which have grown steadily in response to industrialization in the region, though recent trends in these two regions differ. Meehl et al. (2008) similarly found that an increased aerosol load reduced precipitation over India during the monsoon season but that it also increased rainfall in the pre-monsoon season. Wang et al. (2009) found that absorbing aerosols were particularly important in influencing the summer monsoon system. This has been validated further by a number of studies (highlighted in Li et al., 2016) that found aerosols can influence the atmospheric dynamics and the formation of clouds, with consequent impacts on daily (Singh et al., 2019), seasonal (Lau et al., 2017) and intraseasonal (Hazra et al., 2013) precipitation. The issue with many of these studies is that they focus on individual models. There is a large spread in the precipitation response across models reflecting differing representations of cloud and aerosol processes (e.g., Wilcox et al., 2015), factors that may bias results given the already complex nature of modeling precipitation over India (Ramanathan et al., 2005; Bollasina et al., 2011; Turner and Annamalai, 2012; Ramesh and Goswami, 2014; Paul et al., 2016; Saha and Ghosh, 2019). Multimodel ensembles can improve our understanding and help constrain uncertainty about the impacts of different aerosol constituents on the monsoon.
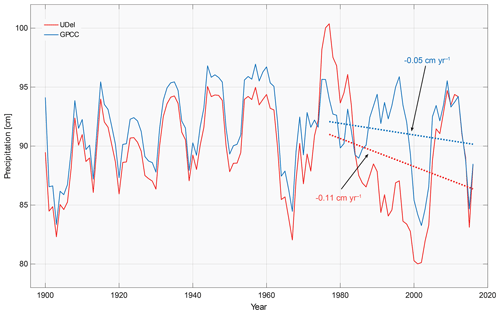
Figure 1Average cumulative summer (JJAS) precipitation [cm] over land in all of India from 1900 to 2016 for two observational datasets: (red) University of Delaware (UDel; Willmott and Matsuura, 2001) and (blue) the Global Precipitation Climatology Center (GPCC; Schneider et al., 2018). Data are smoothed using a moving mean with a window size of 5 years. Linear trend lines are indicated for the last 40 years for each dataset as dashed lines, and the slopes [cm yr−1] are denoted by the arrows.
Here, we analyze results from two climate model intercomparisons to better understand the summer monsoonal impacts from sulfur and black carbon (BC) aerosols, two of the dominant constituents of India's aerosol pollution. First, we study the Precipitation Driver Response Model Intercomparison Project (PDRMIP; Samset et al., 2016) experiments to assess the summer monsoon response to extreme aerosol conditions. The purpose of the PDRMIP experiments here is to determine if a precipitation signal in the South Asian summer monsoon can be detected in scenarios with large emissions perturbations of sulfur and black carbon. Previous analysis of a set of PDRMIP experiments which increase global BC levels 10-fold found a slight enhancement in precipitation minus evaporation during the South Asian summer monsoon, driven by a strengthened land–sea temperature gradient (Xie at al., 2020). We focus the first section of our analysis on Asian perturbation experiments as significant emissions changes are expected over this region in the coming decades (e.g., Samset et al., 2019). We note that these experiments use artificially large emission perturbations to enable isolation of signal detection from climatic variability. Second, we study a set of regional aerosol emissions intercomparison experiments (labeled RAEI experiments for the rest of the paper for convenience) to assess the relative contributions of Indian and Chinese anthropogenic aerosol emissions to the monsoon. Because emissions outside of India may play an important role in its summer monsoon (Bollasina et al., 2014; Shawki et al., 2018), in addition to Indian emissions we choose to study emissions from China because this country is presently the world's leading emitter of BC and SO2, it is in close proximity to India, and its emissions of both pollutants are expected to decline rapidly over the coming decade. Emissions in more remote regions are less likely to change in a major way. A robust analysis of these intercomparisons should refine our understanding of the anthropogenic influence on the South Asian summer monsoon and reduce uncertainty about future changes given that India's anthropogenic emissions are expected to increase at least in the near term, while China's will likely decrease (Rao et al., 2013). We decompose precipitation changes into dynamic (i.e., circulation changes) and thermodynamic (i.e., specific humidity changes) components to assess how aerosols interact with the monsoon. The rest of the paper is structured as follows: Sect. 2 discusses the simulations used in the analysis, Sect. 3 presents and analyzes potential monsoonal impacts associated with sulfur and black carbon emissions, and Sect. 4 summarizes the study and highlights needs for future work.
2.1 PDRMIP intercomparison
We first study the Precipitation Driver Response Model Intercomparison Project experiments. PDRMIP is an idealized set of modeling experiments used to better understand drivers of regional precipitation change. We focus specifically on two experiments that involve perturbations to Asian concentrations or emissions (see Table 1), where Asia is defined by the regional box of 10–50∘ N and 60–140∘ E. The first is BC10xASIA, representing a 10-fold increase in present-day BC concentrations or emissions in Asia at all vertical levels, and the second is SULF10xASIA, which explores a similar 10-fold increase in present-day sulfate concentrations or emissions. The BC10xASIA and SULF10xASIA scenarios are compared with control simulations (henceforth called CTRLPDRMIP) where aerosol concentrations or emissions are maintained at near-current values (either year 2000 or 2005 for each model). We study the six models involved in the PDRMIP experiments that conduct the Asian perturbation experiments (Table 1). These experiments will be used to better constrain uncertainty about the direction of precipitation and circulation changes under anthropogenic aerosol emissions changes. Since these are extreme perturbations to aerosol concentrations, we use these scenarios not as representative of a future emissions trajectory but rather as a way to check if different models with different process representations indicate a consistent response. Due to intermodel differences in spatial resolution, all data are rescaled to the lowest model resolution () when comparing model output. Variations in aerosol schemes and direct and indirect aerosol effects across the six models will affect the spread in predicted precipitation changes associated with the increased aerosol concentrations (Table 1). The different schemes and their effects on precipitation will be discussed further in the Sect. 3.
Table 1Details of the models analyzed in this work. For the models participating in the PDRMIP Asian aerosol perturbation simulations, each simulation lasts 100 years. Cloud scheme refers to the microphysical cloud scheme that describes cloud formation, where a one-moment scheme considers only changes in mass and a two-moment scheme considers changes in mass and number concentration. The first indirect effect refers to the aerosol effect on cloud albedo, and the second indirect effect refers to the aerosol effect on cloud lifetime.
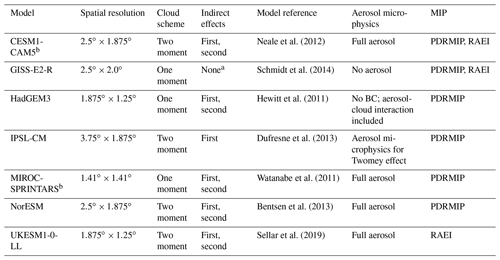
a Indirect effects in the PDRMIP simulations were turned off since these simulations had prescribed aerosol fields and so changes in the hydrologic cycle could not change the aerosols. The first effect was included in the GISS RAEI simulations, however, as those are emissions-driven and hence physically consistent.
b Indicate models that change emissions in the PDRMIP experiments. Rows that do not include this mark indicate models that change concentrations in the PDRMIP experiments.
2.2 RAEI experiments
The purpose of the RAEI experiments is to assess the relative contributions of aerosol emissions from China and India on monsoon precipitation over India. Three general circulation models (GCMs) with coupled chemistry–climate components are used to study the effects of regional perturbations in aerosol emissions on the Indian monsoon: GISS-E2-R (Schmidt et al., 2014), CESM1-CAM5 (Neale et al., 2012) and UKESM1-0-LL (Sellar et al., 2019). Past research has used some of these models to explore the effects of regional aerosol reductions on global precipitation, including emissions changes in the US, Europe, China and India. Some of the experiments from RAEI have been used to study the global effects of US SO2 emissions on global precipitation (Westervelt et al., 2017) as well as local and remote precipitation responses to regional reductions in aerosols (Westervelt et al., 2018). Here, we study the South Asian summer monsoon response to reductions in anthropogenic aerosol emissions in China and India, focusing specifically on a set of three experiments: (1) no SO2 emissions in India (IND NO SO2), (2) 80 % SO2 emissions reduction in China (CHN 20 % SO2), and (3) no SO2 emissions in India and China (IND + CHN NO SO2). We have run additional BC experiments that are included only in the Supplement because we find that changes in BC do not have a clear impact on precipitation in the summer monsoon. The three SO2 experiments will be compared to control simulations (CTRL) with emissions set near present-day values (year 2000 or 2005 depending on the model) to determine the relative importance on summer monsoon precipitation of regional aerosol emissions from India and China. The UKESM experiments were run over a shorter period (40 years) relative to the other models (∼200 years). We found from resampling that 40 years is sufficient to observe the general seasonally aggregated precipitation statistics over India. For climatological variables studied in our PDRMIP and RAEI analysis, we take mean values over the full simulation period, excluding the first 10 years to allow for spin-up.
2.3 Precipitation decomposition
In addition to calculating overall precipitation changes due to sulfur and BC emissions, we seek also to determine the dynamic and thermodynamic components of the changes attributable to these forcing agents. The dynamic component is representative of precipitation changes caused by a change in atmospheric circulation, and the thermodynamic component is representative of variations in precipitation due to changes in moisture under constant circulation. To perform this decomposition, we follow the methodology of Chadwick et al. (2016). The total precipitation change ΔP can be expressed as
where q is the near-surface specific humidity and M* is a proxy for convective mass flux (). The first term on the right-hand side is representative of thermodynamic changes (ΔPtherm), the second dynamic changes (ΔPdyn) and the third the nonlinear interaction of these two components (ΔPcross). ΔPdyn can be further decomposed into shifts in the circulation patterns (ΔPshift) and changes in the mean strength of the tropical circulation (ΔPstrength) as
where (where α= tropical mean ΔM* tropical mean M*). is computed as the residual of ΔM* and . This decomposition follows the methodology in Chadwick et al. (2016) and Monerie et al. (2019).
3.1 PDRMIP analysis: summertime Indian precipitation response to large BC and sulfur perturbations
We start with an evaluation using the PDRMIP experiments (Table 1) of summertime Indian precipitation changes caused by large BC and sulfate concentration increases over all of Asia. The difference in summer precipitation between the BC10xASIA and CTRLPDRMIP experiments provides an estimate for the role of BC in monsoonal changes and is shown in Fig. 2a–g. From the individual models (Fig. 2a–f), there is a noticeably large ensemble spread in the precipitation response over India due to the increase in BC. In north India, for example, HadGEM3 shows a precipitation decrease of up to 70 %, while SPRINTARS exhibits effectively a null response and GISS is identified with a strong precipitation increase of ∼50 %. PDRMIP simulations that globally increase BC 10-fold also do not show a consistent multimodel response over India (Samset et al., 2016). The first regional analysis of the PDRMIP experiments by Liu et al. (2018) found also a weak precipitation response to BC changes, attributed to insignificant circulation changes relative to those induced by the sulfur experiments. While HadGEM3 and GISS generally underestimate precipitation over India (Fig. S3), it does not appear that these biases are manifest in consistent precipitation changes in the BC10xASIA experiments. The weak precipitation over India in HadGEM3 in the CTRL simulation (Fig. S3) also likely explains the large percent changes indicated in the BC and sulfate experiments. Additionally, while two of the six models studied increase BC emissions rather than BC concentrations, this does not appear to alter the BC vertical profile except in the stratosphere (see Fig. S4). It is likely that different aerosol schemes across models (Table 1) may be implicated as one of the dominant sources of the large ensemble spread by altering simulated clouds' radiative properties and lifetimes, as has been shown in previous studies testing different aerosol schemes in the same coupled climate model (Nazarenko et al., 2017). Additionally, both the boundary layer scheme and modeling impacts of absorbing aerosols on cloud formation could play important roles. Specifically, Koch and Del Genio (2010) note that cloud formation is affected significantly by the BC vertical profile; BC within the cloud layer can burn off moisture and reduce cloud cover, BC below the cloud layer can enhance convection and increase cloud cover, and BC above the cloud layer can either increase or decrease cloud cover according to the cloud type. Because of the complexities of the semi-direct effects of absorbing aerosols that are currently not heavily constrained by observations, the role of BC generally has a diverse response across climate models (Koch et al., 2009; Stjern et al., 2017). Large variance in the cloud fraction vertical profile is also apparent in the PDRMIP BC10xASIA simulations (Fig. 3). This large uncertainty does not consistently favor an increase or decrease in cloud fraction across vertical layers except in NorESM and CESM, where a slight increase (on the order of a couple of percent) can be detected across all layers. Variations in the BC vertical profile as well as its lifetime can result in significant changes in cloud cover and precipitation even within an individual model by changing atmospheric stability and humidity (Samset and Myhre, 2015). These effects are manifest in the diverse shortwave responses (Fig. S5), which indicate a large spread between models in magnitude and sign over parts of India. Additionally, changes in the top-of-the-atmosphere net radiative forcing between BC10xASIA and PDRMIPCTRL are generally consistent in magnitude and direction across models over India (Fig. S6a–f). By contrast, the change in cloud radiative effect (Fig. S6g–l) is not consistent in sign across models, suggesting that the models agree on the direct aerosol effects but differ on the aerosol–cloud interactions. While there are more causative factors in precipitation than cloud fraction, the important point is that, because of the large cloud uncertainty that varies in both magnitude and sign, it is difficult to attribute future changes in Indian precipitation to changes in BC concentration. This is reflected in the precipitation change, which fails to demonstrate a clear spatial coherence in the multimodel mean (Fig. 2g).
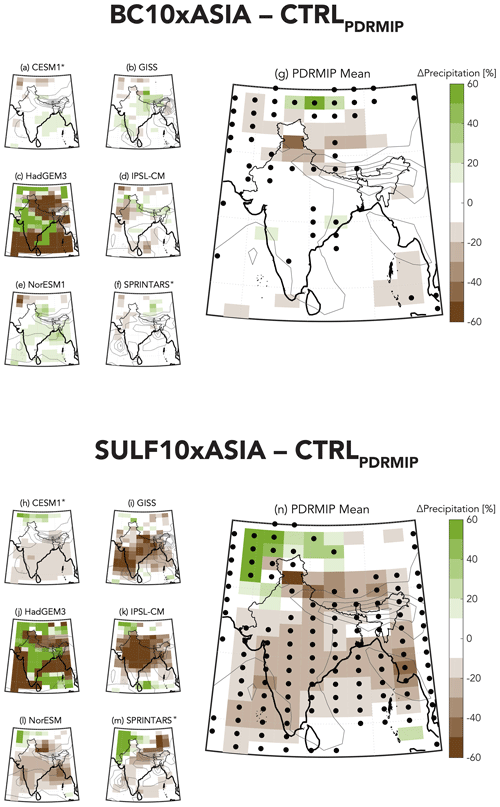
Figure 2Percent change in summertime (JJAS) precipitation between (a–f) the BC10xASIA and the CTRLPDRMIP runs; (g) the multimodel mean of the change. Similarly, panels (h–m) represent the precipitation change in JJAS precipitation between the SULF10xASIA scenarios and the CTRLPDRMIP runs, and panel (n) represents the multimodel mean of the change. Stippled grid cells in panels (g) and (n) denote regions where at least five of the six models agree on the sign of the change. Grey contours indicate mean JJAS precipitation from the control experiment for each model at 5 mm d−1 intervals.
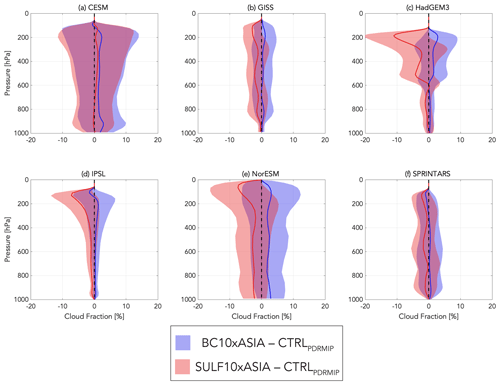
Figure 3JJAS difference in cloud fraction between (blue) the BC10xASIA and the CTRLPDRMIP runs and (red) the SULF10xASIA scenarios and the CTRLPDRMIP runs. The bold lines represent the mean difference, and the shadings represent 25th and 75th percentiles.
The role of sulfate in Indian precipitation is much clearer. The percent change in precipitation between the SULF10xASIA and CTRL PDRMIP experiments is shown in Fig. 2h–n. The sign of the precipitation change is generally consistent across models, with a large decrease in precipitation (∼50 %) over all of India in response to a 10-fold increase in sulfate. There is also large uncertainty in the cloud fraction profile response to sulfate emissions (Fig. 3), similar to the BC PDRMIP experiments. However, five of the six models on average favor a decrease in cloud fraction with increased SO2 emissions, consistent with the precipitation response. So, while there is a comparable measure of intermodel spread for the BC10xASIA and SULF10xASIA cloud responses, the mean change is more consistent in the SULF10xASIA experiments. The results from the PDRMIP experiments, with their higher sulfate concentrations, constrain uncertainty about the sign of precipitation changes and can be used as a frame of reference for the country-specific aerosol experiments described in Sect. 3.2 and beyond.
3.2 RAEI analysis: Indian aerosol burden response to Chinese and Indian aerosol emissions changes
We now consider the RAEI emissions scenarios for China and India. Percent changes in sulfate burden between the sulfate reduction scenarios and control runs are shown in Fig. S7a–i. Indian sulfate emissions play an important role in local sulfate concentrations, contributing up to 60 % of the country's aerosol burden, while China's emissions can contribute up to 60 % over the Himalayas. The change in Indian aerosol burden for sulfate is notably consistent in terms of both the magnitude of the change and the spatial pattern across the three models studied. Since the temperature gradient between the Arabian Sea and Bay of Bengal and the Himalayas has been invoked as a modulator of the South Asian monsoon (e.g., Priya et al., 2017), both Indian and Chinese emissions could influence monsoon precipitation over India by modifying the optical properties of the atmosphere not only over the country but also over surrounding regions.
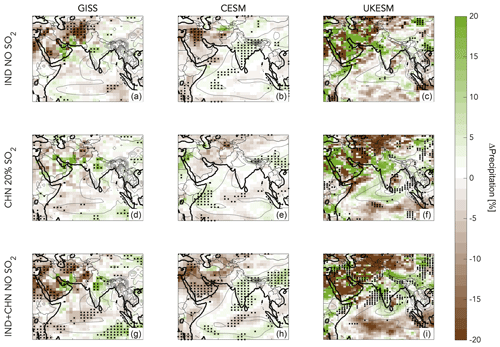
Figure 4JJAS precipitation percentage difference between the SO2 regional emissions scenarios and the CTRL runs. The columns represent the different models, and rows represent the different emissions scenarios. Stippled regions denote areas where the difference is significant at a 90 % confidence level for a two-sample t test. Grey contours indicate mean JJAS precipitation from the control experiment for each model at 5 mm d−1 intervals.
3.3 RAEI analysis: summer monsoon precipitation response to regional SO2 emissions changes
The precipitation response associated with SO2 emissions is significant over parts of India (Fig. 4a–i), in agreement with the PDRMIP results. All scenarios across the multimodel ensemble (with the exception of CESM's CHN 20 % SO2 scenario) show an increase in summer precipitation in India when SO2 emissions in China and/or India are reduced. The strongest response requires reductions from both China and India, with an increase of nearly 20 % in precipitation in some regions of India when SO2 emissions are reduced across the three models studied here. From these results, changes in India's precipitation depend not only on local SO2 emissions but also on regional sources. These emissions can have a measurable impact on India's water availability by altering the underlying statistics in favor of greater precipitation events (e.g., Sillmann et al., 2019). That being said, the spatial patterns associated with these precipitation changes vary to a large degree between models. For instance, precipitation changes in GISS exhibit greater consistency across scenarios than they do with the CESM or UKESM. Additionally, UKESM tends to estimate larger precipitation changes than the other RAEI models, consistent with the HadGEM3 results indicated in Fig. 2, which uses the same physical model. There is, however, general consistency in the increase in precipitation when SO2 emissions are reduced in both China and India. The precipitation responses to lower BC regional emissions are indicated in Fig. S8. BC emissions play a much lesser role in GISS and CESM relative to SO2 emissions and cause an inconsistent response in UKESM across the three regional emissions experiments. For all reduced-BC scenarios (with the exception of two UKESM scenarios), the changes in India's precipitation are generally small (∼5 % locally) and not statistically significant at a 90 % confidence level. The strongest precipitation response occurs when both Chinese and Indian BC emissions are eliminated, but there is a spread in the direction of change across models. This spread in precipitation change is consistent with that of the PDRMIP results in that the intermodel spread in precipitation change due to BC emissions changes tends to be larger than the magnitude of the precipitation response from any individual model. This may highlight large process uncertainty generally. Bond et al. (2013), for example, note that the impact of BC on the cloud radiative forcing in models is highly sensitive to the nucleation regime in the background atmosphere.
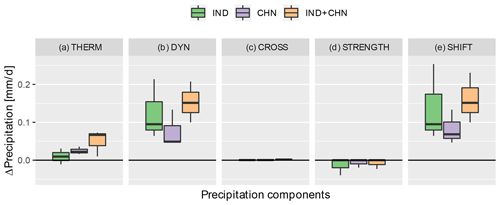
Figure 5Boxplots indicating the decomposition of area-averaged JJAS precipitation anomalies [mm d−1] into (a) ΔPtherm, (b) ΔPdyn, (c) ΔPcross, (d) ΔPstrength and (e) ΔPshift components over India. Different colors represent the three RAEI scenarios relative to the respective CTRL run, with green representing the IND NO SO2 experiment, purple the CHN 20 % SO2 experiment and orange the IND + CHN NO SO2 experiment. The range for each boxplot corresponds to intermodel variability from the three different models studied in the RAEI experiments.
3.4 RAEI analysis: physical understanding of the SO2 precipitation response
Physical explanations for the precipitation changes induced by SO2 emissions changes are explored here. Circulation changes are typically connected to sulfate increases in India; a weakened land–sea temperature gradient associated with SO2 emissions would inhibit monsoonal advection of moisture from the Arabian Sea onto the Indian subcontinent. Warming over the Himalayas can be seen in most of the simulations (Fig. S9), as well as changes in 850 hPa winds, where there is a clear strengthening of the coastal winds when SO2 emissions are reduced (Fig. S10). The fact that the land–sea temperature gradient and 850 hPa winds change suggests that precipitation changes due to SO2 emissions may be dynamically rather than thermodynamically driven, which motivates the precipitation decomposition analysis discussed later. A similar analysis by Shawki et al. (2018) also found that reduced Chinese SO2 emissions strengthened the land–sea temperature contrast and consequently precipitation over India. As shown in Fig. 4, strengthening of the monsoonal winds is largely consistent across models and scenarios, though there are slight differences in the location of the strongest zonal wind increases; in GISS and UKESM, the greatest increase is over India itself for most scenarios, while it is further south in CESM. This suggests that a high sulfate burden reduces the strength of the monsoon winds, consistent with prior studies that connect these changes to the dimming of the downward solar flux (Kim et al., 2007). The relative contributions of thermodynamic (i.e., specific humidity) changes to dynamic (i.e., circulation) changes are indicated in Fig. 5. The thermodynamic precipitation response to sulfur emissions reductions is positive for the three emissions experiments, consistent with the Clausius–Clapeyron relation as less SO2 increases surface temperatures and consequently specific humidity. The interaction of dynamic and thermodynamic components (panel c, ΔPcross) plays a minimal role. The magnitude of the thermodynamic response is on the order of 50 % of that of the dynamic component – i.e., the dynamic component dominates. Figure 5d and e indicate that this effect is driven primarily by shifts in the convective regions, with changes in the tropical mean circulation having a minimal or slightly negative effect. It is of note that the magnitude of each component is consistent across the three models studied here, suggesting consistency in the mechanistic reasons for increased monsoon precipitation over India when sulfur emissions are reduced. Changing circulation patterns are suggested as a consequence of changes in CO2 as well, and potential nonlinear effects of sulfur and greenhouse emissions on monsoon precipitation highlight an important challenge in predicting future changes to the South Asian summer monsoon.
The main purpose of this study was to better understand, through the use of several GCM experiments, the sensitivity of the South Asian summer monsoon to regional anthropogenic aerosol emission changes. Given that this is a modeling study, there are a number of caveats that must be acknowledged. There are often questions of how well GCMs can simulate the Indian monsoon since their spatial resolution may be too coarse to resolve the complex orography of India and the surrounding regions (Prell and Kutzbach, 1992). Additionally, representation of cloud microphysical processes is a known limitation of GCMs (e.g., Wilcox et al., 2015). We find a large intermodel spread in cloud profile and precipitation changes in the various BC emissions scenarios studied here. This suggests that discrepancies in representation of cloud processes within GCMs constrain uncertainty in the precipitation response from BC perturbations, which cannot be accounted for simply by differences in the BC vertical profiles (Fig. S4). In contrast, the precipitation responses to SO2 emission changes and the dynamic mechanism for these responses are largely consistent across models, suggesting that there is relative certainty in the models' ability to simulate precipitation changes due to SO2 emissions. So, while it may be difficult to extrapolate on the basis of these simulations from modeled to real-world monsoon precipitation changes induced by anthropogenic aerosols, consistency in the SO2 response across models lends confidence in a potential observed response for future emissions changes.
On investigating the response of the monsoon to a 10-fold increase of Asian BC and sulfate concentrations, we found that the role of BC in Indian precipitation is uncertain but that increased sulfate concentrations over India reduce precipitation across five of the six models studied. Large uncertainty in the precipitation response to changing Asian BC is notably consistent with previous PDRMIP analysis studying monsoon changes to a 10-fold increase in global BC levels (Xie et al., 2020). Consistency between the global and regional PDRMIP simulations in this context suggests further that a BC signal is difficult to detect for the South Asian summer monsoon (a result found also in Liu et al., 2018).
When assessing the relative contributions of Chinese and Indian anthropogenic SO2 emissions to aerosol loading over South Asia (the RAEI emissions experiments), and the consequent precipitation responses, we find that there is only a statistically significant difference in monsoon precipitation when there is reduction of both China and India's SO2 emissions, which leads to a precipitation increase on the order of a 20 % locally. Consistency in the precipitation responses between the increased-sulfate scenario (PDRMIP SULF10xASIA) and the decreased-sulfate scenario (RAEI) suggests that the aerosol–precipitation link may be a reversible process and is attributable in large part to dynamical changes, specifically shifts in convective patterns over the region. Additionally, these results are significant because Chinese emissions of SO2 have declined over the past decade, while Indian emissions have grown steadily. There is also anticipated growth in CO2 emissions and concentrations over the coming decades, and this is expected to result in an increase in the atmospheric water vapor content. These concurrent events will have important implications for policy going forward, as water deficits present a major issue for India that may be exacerbated given the country's exponential population growth. Regions that exhibit large variability in summertime precipitation such as Chennai and Delhi (as indicated in Fig. S11) may be particularly sensitive to future monsoon changes because interannual shifts between wet and dry years at present impose important strains on the available water resource. Moreover, the benefits of policies to control SO2 emissions will have significant impacts not only on mitigating water deficits but also in terms of alleviation of air pollution, estimated to be responsible for hundreds of thousands of premature deaths per year in India (Health Effects Institute, 2019). It is, however, important to bear in mind that SO2 emissions reductions could also increase flooding and extreme precipitation generally (Sillmann et al., 2019).
While China's pollution is expected to decline in most socioeconomic projections, India's is expected to grow except under strong emissions controls (Samset et al., 2019). Regardless of the realism of these scenarios, the results should be seen as further impetus for regional policies to reduce SO2 emissions given that we have found that combined emissions reductions from China and India can increase monsoon precipitation over India by 5 % on average and by up to 20 % locally. This effect, in combination with consequent impacts of continued growth in GHGs (Fig. S1), could result in an overabundance. This calls therefore for careful consideration of implications for both precipitation and health over multiple timescales.
All code and model data to make the figures used in this paper will be made publicly available through Zenodo following acceptance of the paper. The ESRL database makes gridded precipitation data publicly available for both the University of Delaware data (https://www.esrl.noaa.gov/psd/data/gridded/data.UDel_AirT_Precip.html, Willmott and Matsuura, 2001) and the GPCC data (https://www.esrl.noaa.gov/psd/data/gridded/data.gpcc.html, Schneider et al., 2018).
The supplement related to this article is available online at: https://doi.org/10.5194/acp-21-3593-2021-supplement.
ATA, NLA, JFL, DS and GF ran the RAEI experiments for their respective GCMs. PS prepared the manuscript with contributions from all co-authors.
The authors declare that they have no conflict of interest.
This study was supported by the Harvard Global Institute. Alex T. Archibald and Nathan Luke Abraham thank NERC through NCAS for funding for the ACSIS project and NE/P016383/1. The UKESM work used Monsoon2, a collaborative high-performance computing facility funded by the Met Office and the Natural Environment Research Council. This work used JASMIN, the UK collaborative data analysis facility. The NCAR-CESM work is supported by the National Science Foundation and the Office of Science (BER) of the US Department of Energy. NCAR is sponsored by the National Science Foundation. Climate modeling at GISS is supported by the NASA Modeling, Analysis and Prediction program. GISS simulations used resources provided by the NASA High-End Computing (HEC) Program through the NASA Center for Climate Simulation (NCCS) at Goddard Space Flight Center.
This research has been supported by the Harvard Global Institute and the National Centre for Atmospheric Science (grant no. NE/P016383/1).
This paper was edited by Pedro Jimenez-Guerrero and reviewed by Matthew Kasoar and one anonymous referee.
Annamalai, H. and Liu, P.: Response of the Asian Summer Monsoon to changes in El Niño properties, Q. J. Roy. Meteor. Soc., 131, 805–831, https://doi.org/10.1256/qj.04.08, 2005.
Annamalai, H., Hamilton, K., and Sperber, K. R.: South Asian summer monsoon and its relationship with ENSO in the IPCC AR4 simulations, J. Climate, 20, 1071–1083, https://doi.org/10.1175/JCLI4035.1, 2007.
Bentsen, M., Bethke, I., Debernard, J. B., Iversen, T., Kirkevåg, A., Seland, Ø., Drange, H., Roelandt, C., Seierstad, I. A., Hoose, C., and Kristjánsson, J. E.: The Norwegian Earth System Model, NorESM1-M – Part 1: Description and basic evaluation of the physical climate, Geosci. Model Dev., 6, 687–720, https://doi.org/10.5194/gmd-6-687-2013, 2013.
Bollasina, M. A., Ming, Y., and Ramaswamy, V.: Anthropogenic aerosols and the weakening of the South Asian Summer Monsoon, Science, 6055, 502–505, https://doi.org/10.1126/science.1204994, 2011.
Bollasina, M. A., Ming, Y., Ramaswamy, V., Schwarzkopf, M. D., and Naik, V.: Contribution of local and remote anthropogenic aerosols to the twentieth century weakening of the South Asian Monsoon, Geophys. Res. Lett., 41, 680–687, https://doi.org/10.1002/2013GL058183, 2014.
Bond, T. C, Doherty, S. J., Fahey, D. W., Forster, P. M., Berntsen, T., DeAngelo, B. J., Flanner, M. G., Ghan, S., Kärcher, B., Koch, D., Kinne, S., Kondo, Y., Quinn, P. K., Sarofim, M. C., Schultz, M. G., Schulz, M., Venkataraman, C., Zhang, H., Zhang, S., Bellouin, N., Guttikunda, S. K., Hopke, P. K., Jacobson, M. Z., Kaiser, J. W., Klimont, Z., Lohmann, U., Schwarz, J. P., Shindell, D., Storelvmo, T., Warren, S. G., and Zender, C. S.: Bounding the role of black carbon in the climate system: A scientific assessment, J. Geophys. Res.-Atmos., 118, 5380–5552, https://doi.org/10.1002/jgrd.50171, 2013.
Chadwick, R., Good, P., and Willett, K. M.: A simple moisture advection model of specific humidity change over land in response to SST warming, J. Climate, 29, 7613–7632, https://doi.org/10.1175/JCLI-D-16-0241.1, 2016.
Douglas, E. M., Beltrán-Przekurat, A., Niyogi, D., Pielke, R. A., and Vörösmarty, C. J.: The impact of agricultural intensification and irrigation on land-atmosphere interactions and Indian monsoon precipitation – a mesoscale modeling perspective, Glob. Planet. Change, 67, 117–128, https://doi.org/10.1016/j.gloplacha.2008.12.007, 2009.
Dufresne, J.-L., Foujols, M.-A., Denvil, S., Caubel, A., Marti, O., Aumont, O., Balkanski, Y., Bekki, S., Bellenger, H., Benshila, R., Bony, S., Bopp, L., Braconnot, P., Brockmann, P., Cadule, P., Cheruy, F., Codron, F., Cozic, A., Cugnet, D., de Noblet, N., Duvel, J.-P., Ethé, C., Fairhead, L., Fichefet, T., Flavoni, S., Friedlingstein, P., Grandpeix, J.-Y., Guez, L., Guilyardi, E., Hauglustaine, D., Hourdin, F., Idelkadi, A., Ghattas, J., Joussaume, S., Kageyama, M., Krinner, G., Labetoulle, S., Lahellec, A., Lefebvre, M.-P., Lefevre, F., Levy, C., Li, Z. X., Lloyd, J., Lott, F., Madec, G., Mancip, M., Marchand, M., Masson, S., Meurdesoif, Y., Mignot, J., Musat, I., Parouty, S., Polcher, J., Rio, C., Schulz, M., Swingedouw, D., Szopa, S., Talandier, C., Terray, P., Viovy, N., and Vuichard, N.: Climate change projections using the IPSL-CM5 Earth System Model: from CMIP3 to CMIP5, Clim. Dynam., 10, 2123–2165, https://doi.org/10.1007/s00382-012-1636-1, 2013.
Goswami, B. N., Venugopal, V., Sengupta, D., Madhusoodanan, M. S., and Xavier, P. K.: Increasing trend of extreme rain events over India in a warming environment, Science, 314, 1442–1445, https://doi.org/10.1126/science.1132027, 2006.
Hazra, A., Goswami, B. N., and Chen, J.-P.: Role of Interactions between Aerosol Radiative Effect, Dynamics, and Cloud Microphysics on Transitions of Monsoon Intraseasonal Oscillations, J. Atmos. Sci., 70, 2073–2087, https://doi.org/10.1175/JAS-D-12-0179.1, 2013.
Health Effects Institute: State of Global Air 2019, Special Report, Boston, MA, Health Effects Institute, 2019.
Hewitt, H. T., Copsey, D., Culverwell, I. D., Harris, C. M., Hill, R. S. R., Keen, A. B., McLaren, A. J., and Hunke, E. C.: Design and implementation of the infrastructure of HadGEM3: the next-generation Met Office climate modelling system, Geosci. Model Dev., 4, 223–253, https://doi.org/10.5194/gmd-4-223-2011, 2011.
Kim, M.-K., Lau, W. K. M., Kim, K.-M., and Lee, W.-S.: A GCM study of effects of radiative forcing of sulfate aerosol on large scale circulation and rainfall in East Asia during boreal spring, Geophys. Res. Lett., 34, L24701, https://doi.org/10.1029/2007GL031683, 2007.
Koch, D. and Del Genio, A. D.: Black carbon semi-direct effects on cloud cover: review and synthesis, Atmos. Chem. Phys., 10, 7685–7696, https://doi.org/10.5194/acp-10-7685-2010, 2010.
Koch, D., Schulz, M., Kinne, S., McNaughton, C., Spackman, J. R., Balkanski, Y., Bauer, S., Berntsen, T., Bond, T. C., Boucher, O., Chin, M., Clarke, A., De Luca, N., Dentener, F., Diehl, T., Dubovik, O., Easter, R., Fahey, D. W., Feichter, J., Fillmore, D., Freitag, S., Ghan, S., Ginoux, P., Gong, S., Horowitz, L., Iversen, T., Kirkevåg, A., Klimont, Z., Kondo, Y., Krol, M., Liu, X., Miller, R., Montanaro, V., Moteki, N., Myhre, G., Penner, J. E., Perlwitz, J., Pitari, G., Reddy, S., Sahu, L., Sakamoto, H., Schuster, G., Schwarz, J. P., Seland, Ø., Stier, P., Takegawa, N., Takemura, T., Textor, C., van Aardenne, J. A., and Zhao, Y.: Evaluation of black carbon estimations in global aerosol models, Atmos. Chem. Phys., 9, 9001–9026, https://doi.org/10.5194/acp-9-9001-2009, 2009.
Kumar, K. K., Kumar, R. K., Ashrit, R. G., Deshpande, N. R., and Hansen, J. W.: Climate impacts on Indian agriculture, Int. J. Clim., 24, 1375–1393, https://doi.org/10.1002/joc.1081, 2004.
Lau, W. K. M., Kim, K.-M., Shi, J.-J., Matsui, T., Chin, M., Tan, Q., Peters-Lidard, C., and Tao, W. K.: Impacts of aerosol-monsoon interaction on rainfall and circulation over Northern India and the Himalaya Foothills, Clim. Dynam., 49, 1945–1960, https://doi.org/10.1007/s00382-016-3430-y, 2017.
Li, Z., Lau, W. K.-M., Ramanathan, V., et al: Aerosol and monsoon climate interactions over Asia, Rev. Geophys. 54, 866–929, https://doi.org/10.1002/2015RG000500, 2016.
Liu, L., Shawki, D., Voulgarakis, A., Kasoar, M., Samset, B. H., Myhre, G., Forster, P. M., Hodnebrog, Ø., Sillman, J., Aalbergsjø, S. G., Boucher, O., Faluvegi, G., Iversen, T., Kirkevåg, A., Lamarque, J.-F., Olivié, D., Richadson, T., Shindell, D., and Takemura, T.: A PDRMIP multimodel study on the impacts of regional aerosol forcings on global and regional precipitation, J. of Climate, 31, 4429–4447, https://doi.org/10.1175/JCLI-D-17-0439.1, 2018.
Meehl, G. A., Arblaster, J. M., and Collins, W. D.: Effects of black carbon aerosols on the Indian Monsoon, J. Climate, 21, 2869–2882, https://doi.org/10.1175/2007JCLI1777.1, 2008.
Monerie, P.-A., Robson, J., Dong, B., Hodson, D. L. R., and Klingaman, N. P.: Effect of the Atlantic multidecadal variability on the global monsoon, Geophys. Res. Lett., 46, 1765–1775, https://doi.org/10.1029/2018GL080903, 2019.
Nazarenko, L., Rind, D., Tsigaridis, K., Genio, A. D., Kelley, M., and Tausnev, N.: Interactive nature of climate change and aerosol forcing, J. Geophys. Res.-Atmos., 122, 3457–3480, https://doi.org/10.1002/2016JD025809, 2017.
Neale, R. B., Gettelman, A., Park, S., Conley, A. J., Kinnison, D., Marsh, D., Smith, A. K., Vitt, F., Morrison, H., Cameron-Smith, P., Collins, W. D., Iacono, M. J., Easter, R. C., Liu, X., Taylor, M. A., Chen, C.-C., Lauritzen, P. H., Williamson, D. L., Garcia, R., Lamarque, J.-F., Mills, M., Tilmes, S., Ghan, S. J., and Rasch, P. J.: Description of the NCAR Community Atmosphere Model (CAM 5.0), NCAR Technical Note TN-486, Boulder, CO, USA, 274 pp., 2012.
Paul, S., Ghosh, S., Oglesby, R., Pathak, A., Chandrasekharan, A., and Ramasankaran, R.: Weakening of Indian summer monsoon rainfall due to changes in land use land cover, Sci. Rep., 6, 32177, https://doi.org/10.1038/srep32177, 2016.
Prell, W. L. and Kutzbach, J. E.: Sensitivity of the Indian monsoon to forcing parameters and implications for its evolution, Nature, 360, 647–652, https://doi.org/10.1038/360647a0, 1992.
Priya, P., Krishnan, R., Mujumdar, M., and Houze Jr., R. A.: Changing monsoon and midlatitude circulation interactions over the Western Himalayas and possible links to occurrences of extreme precipitation, Clim. Dynam., 49, 2351–2364, https://doi.org/10.1007/s00382-016-3458-z, 2017.
Ramanathan, V. and Crutzen, P.: New directions: Atmospheric brown “clouds”, Atmos. Env., 37, 4033–4035, https://doi.org/10.1016/S1352-2310(03)00536-3, 2003.
Ramanathan, V., Chung, C., Kim, D., Bettge, T., Buja, L., Kiehl, J. T., Washington, W. M., Fu, Q., Sikka, D. R., and Wild, M.: Atmospheric brown clouds: Impacts on South Asian climate and hydrological cycle, P. Natl. Acad. Sci. USA, 102, 5326–5333, https://doi.org/10.1073/pnas.0500656102, 2005.
Ramesh, K. V. and Goswami, P.: Assessing reliability of climate projections: the case of Indian monsoon, Sci. Rep., 4, 161–174, https://doi.org/10.1038/srep04071, 2014.
Rao, S., Pachauri, S., Dentener, F., Kinney, P., Klimont, Z., Riahi, K., and Schöpp, W.: Better air for better health: Forging synergies in policies for energy access, climate change and air pollution, Global Environ. Chang., 23, 1122–1130, https://doi.org/10.1016/j.gloenvcha.2013.05.003, 2013.
Saha, A. and Ghosh, S.: Can the weakening of Indian Monsoon be attributed to anthropogenic aerosols?, Environ. Res. Commun., 1, 061006, https://doi.org/10.1088/2515-7620/ab2c65, 2019.
Salzmann, M., Weser, H., and Cherian, R.: Robust response of Asian summer monsoon to anthropogenic aerosols in CMIP5 models, J. Geophys. Res., 119, 11321–11337, https://doi.org/10.1002/2014JD021783, 2014.
Samset, B. H. and Myhre, G.: Climate response to externally mixed black carbon as a function of altitude, J. Geophys. Res., 120, 2913–2927, https://doi.org/10.1002/2014JD022849, 2015.
Samset, B. H., Myhre, G., Forster, P. M., Hodnebrog, Ø., Andrews, T., Faluvegi, G., Fläschner, D., Kasoar, M., Kharin, V., Kirkevåg, A., Lamarque, J.-F., Olivié, D., Richardson, T., Shindell, D., Shine, K. P., Takemura, T., and Voulgarakis, A.: Fast and slow precipitation responses to individual climate forcers: A PDRMIP multimodel study, Geophys. Res. Lett., 43, 2782–2691, https://doi.org/10.1002/2016GL068064, 2016.
Samset, B. H., Lund, M. T., Bollasina, M., Myhre, G., and Wilcox, L.: Emerging Asian aerosol patterns, Nat. Geosci., 12, 582–584, https://doi.org/10.1038/s41561-019-0424-5, 2019.
Schmidt, G. A., Kelley, M., Nazarenko, L., Ruedy, R., Russell, G. L., Aleinov, I., Bauer, M., Bauer, S. E., Bhat, M. K., Bleck, R., Canuto, V., Chen, Y., Cheng, Y., Clune, T. L., Del Genio, A., de Fainchtein, R., Faluvegi, G., Hansen, J. E., Healy, R. J., Kiang, N. Y., Koch, D., Lacis, A. A., LeGrande, A. N., Lerner, J., Lo, K. K., Matthews, E. E., Menon, S., Miller, R. L., Oinas, V., Oloso, A. O., Perlwitz, J. P., Puma, M. J., Putman, W. M., Rind, D., Romanou, A., Sato, M., Shindell, D. T., Sun, S., Syed, R. A., Tausnev, N., Tsigaridis, K., Unger, N., Voulgarakis, A., Yao, M.-S., and Zhang, J.: Configuration and assessment of the GISS ModelE2 contributions to the CMIP5 archive, J. Adv. Model. Earth Syst., 1, 141–184, https://doi.org/10.1002/2013MS000265, 2014.
Schneider, U., Becker, A., Finger, P., Meyer-Christoffer, A., and Ziese, M.: GPCC Full Data Monthly Product Version 2018 at 0.5∘: Monthly Land-Surface Precipitation from Rain-Gauges built on GTS-based and Historical Data, https://doi.org/10.5676/DWD_GPCC/FD_M_V2018_050, 2018.
Sellar, A. A., Jones, C. G., Mulcahy, J., Tang, Y., Yool, A., Wiltshire, A., O'Connor, F. M., Stringer, M., Hill, R., Palmieri, J., Woodward, S., Mora, L., Kuhlbrodt, T., Rumbold, S., Kelley, D. I., Ellis, R., Johnson, C. E., Walton, J., Abraham, N. L., Andrews, M. B., Andrews, T., Archibald, A. T., Berthou, S., Burke, E., Blockley, E., Carslaw, K., Dalvi, M., Edwards, J., Folberth, G. A., Gedney, N., Griffiths, P. T., Harper, A. B., Hendry, M. A., Hewitt, A. J., Johnson, B., Jones, A., Jones, C. D., Keeble, J., Liddicoat, S., Morgenstern, O., Parker, R. J., Predoi, V., Robertson, E., Siahaan, A., Smith, R. S., Swaminathan, R., Woodhouse, M. T., Zeng, G., and Zerroukat, M.: UKESM1: Description and evaluation of the UK Earth System Model, J. Adv. Model. Earth Syst., 11, 4513–4558, https://doi.org/10.1029/2019MS001739, 2019.
Shawki, D., Voulgarakis, A., Chakraborty, A., Kasoar, M., and Srinivasan, J.: The South Asian Monsoon response to remote aerosols: global and regional mechanisms, J. Geophys. Res., 123, 11585–11601, https://doi.org/10.1029/2018JD028623, 2018.
Shukla, J. and Paolino, D. A.: The Southern Oscillation and long-range forecasting of the summer monsoon rainfall over India, Mon. Weather Rev., 111, 1830–1837, https://doi.org/10.1175/1520-0493(1983)111<1830:TSOALR>2.0.CO;2, 1983.
Sikka, D. R.: Some aspects of the large-scale fluctuations of summer monsoon rainfall over India in relation to fluctuations in the planetary and regional scale circulation parameters, Proc. Indian Natl. Acad. Sci., 89, 179–195, https://doi.org/10.1007/BF02913749, 1980.
Sillmann, J., Stjern, C. W., Myhre, G., Samset, B. H., Hodnebrog, Ø., Andrews, T., Boucher, O., Faluvegi, G., Forster, P., Kasoar, M. R., Kharin, V. V., Kirkevåg, A., Lamarque, J.-F., Olivié, D. J. L., Richardson, T. B., Shinndell, D., Takemura, T., Voulgarakis, A., and Zwiers, F. W.: Extreme wet and dry conditions affected differently by greenhouse gases and aerosols, NPJ Clim. Atmos. Sci., 2, 24, https://doi.org/10.1038/s41612-019-0079-3, 2019.
Singh, D., Bollasina, M., Ting, M., and Diffenbaugh, N. S.: Disentangling the influence of local and remote anthropogenic aerosols on South Asian monsoon daily rainfall characteristics, Clim. Dynam., 52, 6301–6320, https://doi.org/10.1007/s00382-018-4512-9, 2019.
Stjern, C. W., Samset, B. H., Myhre, G., Forster, P. M., Hodnebrog, Ø., Andrews, T., Boucher, O., Faluvegi, G., Iversen, T., Kasoar, M., Kharin, V., Kirkevåg, A., Lamarque, J.-F., Olivié, D., Richardson, T., Shawki, D., Shindell, D., Smith, C., Takemura, T., and Voulgarakis, A.: Rapid adjustments cause weak surface temperature response to increased black carbon concentrations, J. Geophys. Res., 122, 11462–11481, https://doi.org/10.1002/2017JD027326, 2017.
Subbiah, A.: Initial report on the Indian monsoon drought of 2002, Asian Disaster Preparedness Center, Bangkok, Thailand, 29 pp., 2004.
Turner, A. G. and Annamalai, H.: Climate change and the South Asian Summer Monsoon, Nat. Clim. Change, 2, 1–9, https://doi.org/10.1038/nclimate1495, 2012.
Turner, A. G. and Slingo, J. M.: Subseasonal extremes of precipitation and active-break cycles of the Indian summer monsoon in a climate change scenario, Q. J. Roy. Meteor. Soc., 135, 549–567, https://doi.org/10.1002/qj.401, 2009.
Wang, C., Kim, D., Ekman, A. M. L., Barth, M. C., and Rasch, P. J.: Impact of anthropogenic aerosols on Indian summer monsoon, Geophys. Res. Lett., 36, L21704, https://doi.org/10.1029/2009GL040114, 2009.
Watanabe, S., Hajima, T., Sudo, K., Nagashima, T., Takemura, T., Okajima, H., Nozawa, T., Kawase, H., Abe, M., Yokohata, T., Ise, T., Sato, H., Kato, E., Takata, K., Emori, S., and Kawamiya, M.: MIROC-ESM 2010: model description and basic results of CMIP5-20c3m experiments, Geosci. Model Dev., 4, 845–872, https://doi.org/10.5194/gmd-4-845-2011, 2011.
Westervelt, D. M., Conley, A. J., Fiore, A. M., Lamarque, J.-F., Shindell, D., Previdi, M., Faluvegi, G., Correa, G., and Horowitz, L. W.: Multimodel precipitation responses to removal of U.S. sulfur dioxide emissions, J. Geophys. Res., 122, 5024–5038, https://doi.org/10.1002/2017JD026756, 2017.
Westervelt, D. M., Conley, A. J., Fiore, A. M., Lamarque, J.-F., Shindell, D. T., Previdi, M., Mascioli, N. R., Faluvegi, G., Correa, G., and Horowitz, L. W.: Connecting regional aerosol emissions reductions to local and remote precipitation responses, Atmos. Chem. Phys., 18, 12461–12475, https://doi.org/10.5194/acp-18-12461-2018, 2018.
Westervelt, D. M., You, Y., Li, X., Ting, M., Lee, D. E., and Ming, Y.: Relative importance of greenhouse gases, sulfate, organic carbon, and black carbon aerosol for South Asian monsoon rainfall changes, Geophys. Res. Lett., 47, 13, https://doi.org/10.1029/2020GL088363, 2020.
Wilcox, L. J., Highwood, E. J., Booth, B. B. B., and Carslaw, K. S.: Quantifying sources of inter-model diversity in the cloud albedo effect, Geophys. Res. Lett., 42, 1568–1575, https://doi.org/10.1002/2015GL063301, 2015.
Willmott, C. J. and Matsuura, K.: Terrestrial Air Temperature and Precipitation: Monthly and Annual Time Series (1950–1999), available at: http://climate.geog.udel.edu/~climate/html_pages/README.ghcn_ts2.html (last access: 8 March 2021), 2001.
Xie, X., Myhre, G., Liu, X., Li, X., Shi, Z., Wang, H., Kirkevåg, A., Lamarque, J.-F., Shindell, D., Takemura, T., and Liu, Y.: Distinct responses of Asian summer monsoon to black carbon aerosols and greenhouse gases, Atmos. Chem. Phys., 20, 11823–11839, https://doi.org/10.5194/acp-20-11823-2020, 2020.