the Creative Commons Attribution 4.0 License.
the Creative Commons Attribution 4.0 License.
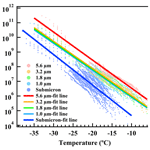
Size-resolved atmospheric ice-nucleating particles during East Asian dust events
Jingchuan Chen
Zhijun Wu
Jie Chen
Naama Reicher
Xin Fang
Yinon Rudich
Asian dust is an important source of atmospheric ice-nucleating particles (INPs). However, the freezing activity of airborne Asian dust, especially its sensitivity to particle size, is poorly understood. In this study we report the first INP measurement of size-resolved airborne mineral dust collected during East Asian dust events. The measured total INP concentrations in the immersion mode ranged from 10−2 to 102 L−1 in dust events at temperatures between −25 and −5 ∘C. The average contributions of heat-sensitive INPs at three temperatures, −10, −15, and −20 ∘C, were 81±12 %, 70±15 %, and 38±21 %, respectively, suggesting that proteinaceous biological materials have a substantial effect on the ice nucleation properties of Asian airborne mineral dust at high temperatures. The dust particles which originated from China's northwest deserts are more efficient INPs compared to those from northern regions. In general, there was no significant difference in the ice nucleation properties between East Asian dust particles and other regions in the world. An explicit size dependence of both INP concentration and surface ice-active-site density was observed. The nucleation efficiency of dust particles increased with increasing particle size, while the INP concentration first increased rapidly and then leveled, due to the significant decrease in the number concentration of larger particles. A new set of parameterizations for INP activity based on size-resolved nucleation properties of Asian mineral dust particles were developed over an extended temperature range (−35 to −6 ∘C). These size-dependent parameterizations require only particle size distribution as input and can be easily applied in models.
- Article
(3852 KB) - Full-text XML
-
Supplement
(513 KB) - BibTeX
- EndNote
Ice formation in tropospheric clouds significantly impacts the microphysical processes and lifetime of clouds, thereby determining radiative forcing, precipitation, and the hydrological cycle (Lohmann and Feichter, 2005; Boucher et al., 2014; Lohmann et al., 2016). Mineral dust particles can act as ice-nucleating particles (INPs) that trigger heterogeneous ice nucleation at relatively high temperatures and low relative humidities by providing nucleation surfaces to efficiently lower the energy barrier of critical ice embryo formation (Pruppacher and Klett, 1997). Up to 2000 Tg of mineral dust is emitted from arid and semiarid areas into the troposphere annually, and its estimated atmospheric loading is the largest among different types of aerosol particles (Prospero, 1999; Textor et al., 2006).
Asian deserts, especially East Asian deserts, are among the major sources (∼800 Tg yr−1) of mineral dust to the global troposphere (Zhang et al., 1997). Asian dust can be transported across the Pacific Ocean, sometimes completing more than one full cycle around the globe (Sun et al., 2001; McKendry et al., 2008; Uno et al., 2009). Ground-based and aircraft cloud measurements have confirmed that dust and adsorbed biological aerosols from regions such as Asia may affect ice formation in mixed-phase clouds (Creamean et al., 2013; Pratt et al., 2009). In addition, modeling studies suggest that Asian dust affects ice particle formation in mixed-phase clouds (Wiacek et al., 2010). However, parameterizations characterizing INP activity are required to predict the occurrence and evolution of clouds, suggesting that there is a need for measurements of ice formation on different INP types (Gultepe et al., 2017; Korolev et al., 2017).
In recent decades, many studies have attempted to quantify the ability of mineral dust particles based on field observations (DeMott et al., 2010; Boose et al., 2016a) and laboratory experiments, investigating worldwide surface-collected dust samples (Niemand et al., 2012; Kaufmann et al., 2016), airborne desert dust (Price et al., 2018; Schrod et al., 2017), reference minerals (Atkinson et al., 2013; Kanji et al., 2008; Knopf et al., 2020), and commercially available dust such as Arizona test dust (Niemand et al., 2012; Kaufmann et al., 2016; Kanji et al., 2008; Niedermeier et al., 2011). Dust particles are mainly composed of clay minerals (including illite, kaolinite, chlorite, etc.), quartz, feldspar, calcite, and other mineral components (Murray et al., 2012; Scanza et al., 2015; Tang et al., 2016). Clay minerals were widely investigated in ice nucleation studies (Mason, 1960; Eastwood et al., 2008; Pinti et al., 2012; Wex et al., 2014; Kumar et al., 2019a) due to their high abundance in mineral dust composition (Murray et al., 2012), especially after long-range transport (Leinen et al., 1994; Uno et al., 2009). However, recent studies have demonstrated that K-feldspars are the most efficient mineral INP (Atkinson et al., 2013; Zolles et al., 2015b; Whale et al., 2017; Harrison et al., 2019; Yakobi-Hancock et al., 2013; Peckhaus et al., 2016; Kiselev et al., 2017; Augustin-Bauditz et al., 2014). As a major component in mineral dust, quartz is important to explain the freezing observed at lower temperatures, although it is less active than feldspars (Zolles et al., 2015a; Boose et al., 2016b; Reicher et al., 2019; Kumar et al., 2019b).
In addition to the active components in mineral dust particles, the effect of particle size on ice nucleation activity, although less explored compared to mineralogical properties, has been investigated in several studies (DeMott et al., 2015; Reicher et al., 2019; Porter et al., 2020). Larger particles often nucleate ice at lower relative humidities (Archuleta et al., 2005; Welti et al., 2009) and higher temperatures (Lüönd et al., 2010). Studying different mineral species (such as montmorillonite, kaolinite, illite, feldspar, and Arizona test dust) at different particle sizes (ranging from 0.1 to 5.6 µm) demonstrated that there is an explicit size dependence in heterogeneous freezing (Welti et al., 2009; Kanji and Abbatt, 2010; Ladino et al., 2011; Hoffmann et al., 2013; Niedermeier et al., 2015; Reicher et al., 2019). Furthermore, biological materials can adhere to the surfaces of larger dust particles during atmospheric lifting and transport processes and effectively extend the ice nucleation ability to higher temperatures (Creamean et al., 2013; O'Sullivan et al., 2016; Augustin-Bauditz et al., 2016; Maki et al., 2018).
Despite their importance, few ice nucleation investigations have focused on collected airborne Asian dust particles, and their nucleation activities and particle properties (Bi et al., 2019; Jiang et al., 2016; Iwata and Matsuki, 2018). In fact, reference single-mineral dust and surface-collected particles do not fully represent the actual dust transport process in the troposphere due to gravitational dust sedimentation, adsorption of biological materials, and other factors. During dust transport, larger particles settle faster due to gravity, while smaller particles can remain lifted for a longer period, thus possibly playing a different role in cloud formation (Kramer et al., 2020; Maki et al., 2019). Moreover, parameterizations are mainly based on the active mineral components of dust, but the effect of particle size is usually not considered. Although the same mineral components are present over a wide range of particle sizes, their concentrations in differently sized particles can vary greatly. Leinen et al. (1994) have demonstrated that quartz and plagioclase exist in significantly higher amounts in the 2 to 20 µm size fraction, while kaolinite is more common in the <2 µm sizes. This variation affects the ice nucleation activity of different particle size fractions. Therefore, a quantitative investigation of size- and temperature-dependent ice nucleation properties of airborne Asian mineral dust is required for a better understanding of the role of Asian dust, especially after long-range transport, in influencing ice formation in clouds and its consequences for climate change.
To further understand the ice nucleation efficiency of Asian dust and its sensitivity to particle size, airborne mineral dust samples were collected during East Asian dust events in the spring of 2018 and 2019. The INP measurements were conducted using a cold-stage technique. We quantified the INP number concentration, surface active-site density, and contribution of heat-sensitive INPs (mostly recognized as proteinaceous biological materials at high temperatures) of mineral dust in different size classes. Furthermore, based on the measurements, a new set of parameterizations were developed, assuming that particle size class can reflect the particle ice nucleation ability. Only particle size distribution is required as input in our parameterizations to predict ice nucleation activity when applied in models.
2.1 Sample collection and analysis
The sampling and measurements were performed at the Peking University Atmosphere Environment Monitoring Station (PKUERS, 39.99∘ N, 116.31∘ E), an integrated observation laboratory in the northwestern urban area of Beijing. A weather station provided minute-level temporal resolution meteorological parameters, including wind speed, wind direction, ambient relative humidity, and temperature.
Aerosol samples were collected on polycarbonate filters (47 mm Nuclepore, Track-Etch Membrane, 0.2 µm pores, Whatman) using the Micro-Orifice Uniform Deposit Impactor (MOUDI, MSP Corporation, USA; Marple et al., 1991). An eight-stage inertia impactor (model 100-R) was used to collect size-resolved aerosol samples during dust events in the spring of 2018 and 2019. We used stages 1 to 8 of the MOUDI with cut points (D50) ranging from 10 to 0.18 µm in aerodynamic diameters at a flow rate of 30 L min−1 in this study. The sizes of collected particles in a given stage are set in a size bin, which is presented as the cut point (D50) in the following. For example, most of the particles with an aerodynamic diameter less than 10 µm and larger than 5.6 µm will be collected on stage D50=5.6 µm; therefore the size range between 5.6 and 10 µm will be presented here as cut point D50=5.6 µm. A total of 14 sets of MOUDI samples were collected: eight in 2018 and six in 2019. The sampling time varied with the duration of the dust events, which ranged from 5 to 24 h. More details of the sampling are available in Table 1.
Particle bounce on the filters is the main cause of interstage losses, especially for larger particles. Due to the limitation of substrates and solid particles, the bounce is unavoidable. However, as many previous studies showed, the losses rapidly decreased as the particles size decreased, and the collection efficiency curves of each stage are sharp, indicating that MOUDI is a high-accuracy cascade impactor for collecting size-resolved particle samples and was widely used in many laboratory experiments and field measurements (Marple et al., 1991; Mason et al., 2015, 2016; Si et al., 2018; Reicher et al., 2019).
2.2 INP measurements
INP measurements in the immersion mode were conducted using the PeKing University Ice Nucleation Array (PKU-INA). PKU-INA is a cold-stage-based ice nucleation array (Chen et al., 2018a). The sampled filter of each stage was completely submerged in 20 mL double-distilled water (resistivity of 18.2 MΩ cm−1 at 25 ∘C). An ultrasonic shaker was used to wash the particles off from the filters. The extraction process lasted 30 min and was kept in an ice-water bath to avoid the effects of ultrasound-induced heating (see Supplement for more details). The ultrasonic shaker is effective for particle removal into the water solution and is widely used (Reicher et al., 2019; Chen et al., 2018b; Ardon-Dryer and Levin, 2014). Note that the ultrasonic waves may influence the properties and function of proteins and change their bioactivity (De Leo et al., 2017).
The particle suspension was immediately measured in PKU-INA. Briefly, 1 µL liquid droplets were pipetted onto a hydrophobic glass slide (40 mm in diameter, supplied by Marienfeld Company, Germany) located on the cold stage (LTS120, Linkam, UK). As many as 90 droplets were pipetted dropwise in an experiment, and another glass slide was placed above to prevent the Wegener–Bergeron–Findeisen process (Jung et al., 2012). Droplets were cooled to 0 ∘C with a cooling rate of 10 ∘C min−1, because freezing is not observed in this temperature range, and then cooled at a rate of 1 ∘C min−1 until all the droplets froze. A charge-coupled device (CCD) camera was used to record the measurements and monitor phase transitions based on the droplets' brightness, with a given time resolution of one frame per 6 s. To avoid frost during cooling, high-purity dry nitrogen was delivered into the cold stage during the measurement. The temperature uncertainty was less than ±0.4 ∘C at the various cooling rates (Chen et al., 2018a).
Ice-active sites are the preferred locations for ice nucleation on an INP, and the cumulative concentration of ice-active sites above T per unit sample volume is given by Vali (1971) and Vali et al. (2015):
where fice(T) is the fraction of droplets frozen above temperature T, and V is the volume of each pipetted droplet (1 µL). Combined with the total sampling volume, the cumulative number concentration of INP (NINP) per unit volume of sampled air is calculated as
where Vair is the total volume of sampled air per droplet converted to standard conditions (0 ∘C and 1013 hPa) during each particle collection period. To quantify and compare the ice nucleation activity of samples with different aerosol particle size, the cumulative ice-nucleation-active-site density ns (Connolly et al., 2009; Hoose and Mohler, 2012; Niemand et al., 2012), i.e., the number of active sites per unit surface area of INPs (Vali et al., 2015), is calculated from the INP concentration as
where A is the total surface area of the particles per unit volume of sampled air, derived from size distribution measurements of the particles (see Sect. 2.3 below). Note that aerodynamic diameter was used in the calculation of ns(T), which is 0.42 to 0.93 times the value of ns(T) determined by the converted geometric diameter (see the Supplement for more details).
The uncertainty of the experimental results arises mainly from the representativeness of testing droplets for the total suspension. The INPs are scarce in the air, thus their number presented in the washing suspension is usually small. These small volume droplets (1 µL) may not contain a representative number of particles, and the number of examined droplets is limited (90 droplets). Therefore, confidence intervals for the number of ice-nucleating particles per unit sample volume (per droplet) should be considered. Following the method of O'Sullivan et al. (2018) and Barker (2002), the confidence intervals are calculated in Eq. (4):
where μ(T) is the number of INPs per droplet, n is the droplet number, and is the standard score at a confidence level , which is 1.96 for a 95 % confidence interval.
The abovementioned parameters (fice(T), K(T), NINP(T), ns(T), and uncertainty) are calculated for each particle size class. We sum size-resolved NINP(T) ranging from 0.18 to 10 µm during each dust event to get the total INP concentration. The total ns(T) is directly derived from total NINP(T) by dividing the total NINP(T) by the total surface area of particles in all size classes.
2.3 Particulate matter measurements
Particle number–size distributions of 3 nm to 10 µm particles were measured during all dust events by a set of two scanning mobility particle sizers (SMPS, TSI Inc., USA) and an aerodynamic particle sizer (APS, model 3321, TSI Inc., USA). The first SMPS, consisting of a short differential mobility analyzer (DMA, model 3085) and an ultrafine condensing particle counter (UCPC, model 3776, with a flow rate of 1.5 L min−1), was used to measure the 3 to 45 nm (stokes diameter) particles. The second SMPS with a long DMA (model 3081) and a CPC (model 3775, with a flow rate of 0.3 L min−1) measured the 45 to 698 nm (stokes diameter) particles. The APS, with a flow rate of 1 L min−1, was used to measure the 0.7–10.0 µm (aerodynamic diameter) particles. The time resolution for both SMPS and APS was 5 min.
Particle surface area distributions were derived from the number–size distributions assuming that the particles were spherical. The Stokes diameter measured by SMPS was transformed to aerodynamic diameter assuming a particle density of 1.5 g cm−3, which is commonly used for small size particles in urban atmospheres (Hu et al., 2012; Qiao et al., 2018). In order to estimate the total surface area sampled on each MOUDI stage, similar to the approach in Reicher et al. (2019), a transformation matrix between the size distribution instruments and the MOUDI stages was applied, which was based on the particle cut-off characteristics of each stage and inter-stage particle losses reported in Marple et al. (1991).
Compared with the transformation matrix used by Reicher et al. (2019), the method adopted in this study has two optimizations. Firstly, the size distribution instruments (SMPS and APS) have more sampling channels (64 channels). The more data points corresponding to each sampling particle size range the instruments measure, the higher the accuracy for total surface area calculated. Secondly, according to the particle collection efficiency curves in Marple et al. (1991), narrowing the intervals of the transformation matrix better reflects the real distribution.
2.4 Air mass back trajectory analysis
Back trajectories of air mass arriving at the sampling site were calculated using the HYSPLIT 4 (Hybrid Single-Particle Lagrangian Integrated Trajectory) model of the NOAA Air Resources Laboratory (Stein et al., 2015). When computing archive trajectories, the GDAS (Global Data Assimilation System, 1∘, global) was selected as meteorological input data. The 72 h back trajectories were initiated at the beginning of each sampling period and started a new trajectory every 1 or 2 h until the end of the sampling period. MeteoInfoMap, a GIS application that enables the user to visualize and analyze the spatial and meteorological data with multiple data formats (Wang, 2014), was used for final analysis and mapping.
3.1 INP concentrations
Detailed sampling and measurement information for the 14 sets of samples collected in 2018 and 2019 are given in Table 1. The total sampled volumes and PM10 mass concentrations during the sampling periods determine the particle collection quantities and affect the INP concentrations. Two weather condition scenarios, dust and non-dust events, were defined, based on PM10 mass concentration (larger than 200 µg m−3 lasting more than 2 h for dust events), the volume concentration of coarse mode particles (mean concentration higher than 75 µm3 cm−3 for dust events; Wu et al., 2009), phenomenological dust storm observations operated by the China Meteorological Administration (CMA, reported as the large-scale dust events), and the concentration of aluminum (Al) element (see Table S1 in the Supplement for more detailed determination criteria). Seven and six dust samples were collected in 2018 (M1, M2, M3, M5, M6, M7, and M8) and in 2019 (D2, D3, D4, D5, D6, and D7), respectively. Sample M4 was sampled from the end of a continuous dust storm period (M1–M3), and its air mass passed through the Bohai Sea before arriving in Beijing (see Fig. S1 in the Supplement). Therefore, sample M4 was not dominated by mineral dust, and it was classified as a non-dust event.
Results of all freezing curves containing size-resolved airborne dust particles are presented in pale yellow in Fig. 1. Each curve corresponds to one sampled filter collected in dust events. Freezing was observed from −5 to −25 ∘C for ambient filters, while blank filters froze between −20 and −30 ∘C. The freezing temperature range of the blank filters is similar to that of distilled water, far lower than that of ambient samples, indicating a low contribution of contaminants from the filters. Frozen fraction curves from sample M1, a set of highly ice-active samples in the collected samples, are also shown in Fig. 1, and each color depicts a different size class ranging from 0.18 to 10.0 µm. Droplets containing different size classes froze at different temperatures. On the whole, large particle samples froze at higher temperatures, while smaller-sized samples froze at lower temperatures, indicating different ice-nucleating abilities. Note that the effect of particle number size distribution on the frozen fraction curves needs to be considered, because fice(T) is sensitive to the number of particles at a given size class.
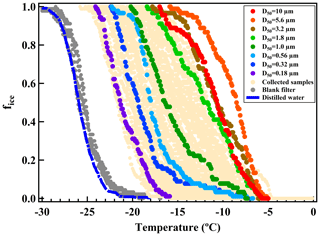
Figure 1Frozen fraction curves of ambient particles during mineral dust events. The collected size-resolved filter measurements are marked in pale yellow (named “Collected samples”), together with background fice curves determined from blank filters (solid grey circles) and distilled water (dashed blue line). As a subset of the collected samples, a set of filters (sample M1) ranging from 0.18 to 10.0 µm are marked with different colors to illustrate the frozen fraction curves for size-resolved particles.
The total concentrations of INPs (NINP) as a function of temperature of all 14 samples, including 13 dust events and 1 non-dust condition (M4), are shown in Fig. 2a, marked by different colors. These results were obtained from the data in Fig. 1 using Eq. (2). In the sampling process, the eight-stage MOUDI collected particles in nine filters simultaneously. Each filter had a corresponding INP concentration, and each line in Fig. 2a represents the sum of INP concentrations from 0.18 to 10 µm in each sample. On the whole, the measured total NINP spanned 4 orders of magnitude from 10−2 to 102 L−1 of standard air, and freezing was measured between −5 and −25 ∘C for dust events and between −15 and −28 ∘C for the non-dust event. The total INP concentrations and freezing temperatures during dust and non-dust periods were significantly different, and the concentration increased by approximately 2 orders of magnitude during dust events at a given temperature, indicating that mineral dust particles are very efficient INPs in the immersion mode. For mineral dust samples, their initial freezing temperatures were similar ( ∘C). However, the variations in concentration between the samples were up to 2 orders of magnitude at a given temperature. The difference in INP concentrations was caused by dust particle properties including particle composition, particle loading, and the sample volume.
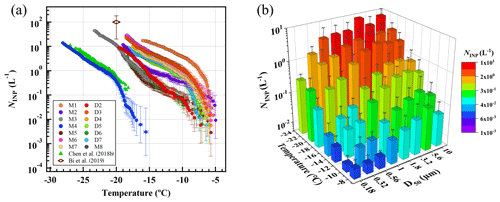
Figure 2INP concentrations (NINP) measured during the different events. (a) NINP of 14 sets of samples are plotted as a function of temperature (solid colored circles). The total INP concentrations in each event are represented by the different colors. NINP values of non-dust days (solid green triangles) measured in Chen et al. (2018b) and the dust event (open brown diamond) measured in Bi et al. (2019) are shown for comparison. Error bars of the INP concentrations indicate the 95 % confidence intervals. (b) Size-resolved NINP versus temperature and particle class. Bars represent the average NINP of 13 dust-dominated samples. Error bars represent the standard deviations in NINP. Uncertainty in temperature values is 0.4 ∘C.
For comparison, two similar observations during dust events (Bi et al., 2019) and non-dust days (Chen et al., 2018b) in Beijing are illustrated in Fig. 2a. Bi et al. (2019) conducted a continuous measurement of INPs using a continuous-flow diffusion chamber–ice activation spectrometer (CFDC–IAS) from 4 May to 4 June 2018, and the NINP at −20 ∘C was 100±80 L−1 during dusty conditions, which agrees with the current study within 1 order of magnitude. For non-dust days, the concentrations in Chen et al. (2018b) are consistent with sample M4 in the present study.
The trend of NINP with temperature and particle size based on 13 mineral dust-dominated samples is depicted in Fig. 2b, where the size-resolved NINP ranged from 10−2 to 101 L−1 of standard air (see Table S2 for more detailed information). For each particle size, INP concentrations increased significantly with decreasing temperature. From another perspective, at a given temperature, NINP increased rapidly and then leveled when the particle size increased from 0.18 to 10 µm. This is explained by the fact that NINP depends not only on the activity of particles in a specific size range, but also on the total number concentration of the same-sized particles.
3.2 Difference of INPs between two transport pathways
In Fig. 3a, two distinct transport pathways, the northwest and north pathways, were identified during the sampling periods, based on air mass back trajectory analysis. These trajectories are consistent with the prevailing mineral dust transport pathways that affect the Beijing region (Huang et al., 2010; Sun et al., 2005). Combined with the geographical distributions of deserts, the northwest pathway passes through the Gobi Desert and Kubuqi sandy desert in northwest China, while the north route passes through Hunshadake, Horqin, and Hulun Buir sandy lands in northeastern China. These two pathways shown in Fig. 3 are based on seven dust events. Four samples (M6, M7, M8, and D7) originated from the northwest region, and three samples (M3, M5, and D6) were from the northern area. Sample D7 is not included in Fig. 3c because the size distribution data are partially missing, and surface area could not be fully derived. The other six samples (M1, M2, D2, D3, D4, and D5) did not follow these two pathways (see Fig. S2) and will not be discussed in this section.
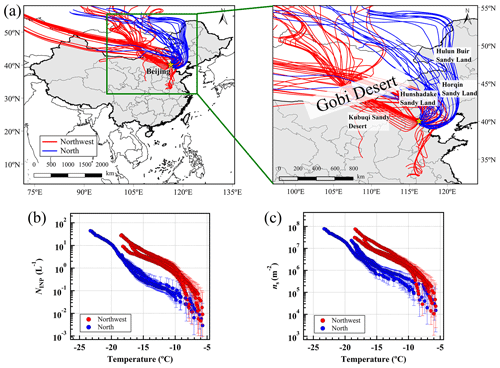
Figure 3Comparison of INPs originating in the northwest and north pathways. (a) Air mass trajectories for the different sampling events based on 72 h back trajectories. (b) INP concentrations (NINP) and (c) ice-nucleation-active-site density (ns(T)) compared for the northwest and north pathways, marked by red and blue, respectively.
It is clearly shown in Fig. 3b that the total INP concentrations of samples that followed the same pathway were very similar in their freezing behavior. Although the concentrations in the two pathways have similar initial and final freezing temperatures, there was a significant difference of an order of magnitude in the intermediate temperatures (−16 to −11 ∘C), indicating that there might be differences in the types of INPs. However, we need to keep in mind that due to the influence of factors such as changing wind speed during the transport process, the atmospheric concentration of dust from the same source region may be different, leading to a varying concentration of atmospheric INPs.
To compare the ice nucleation activity of different samples, ice-active-site density, ns(T), was calculated and is shown in Fig. 3c. Similar to NINP in Fig. 3b, the total ns(T) values were similar in trajectories that followed the same route but differed in trajectories between the two major pathways. This suggests that the particle compositions differed between the different source regions; the ice nucleation activity of particles from the northwest was higher than that from the northern region. The surface area concentrations of the coarse mode particles (Dp≥1.0 µm) were also higher in the northwest pathway (see Fig. S3).
Both NINP and ns(T) in the northwest pathway were higher than those in the north route, suggesting that dust from northwest China deserts has a higher freezing ability. On the one hand, previous studies have shown that Chinese deserts have distinct zoning characteristics; the northwestern deserts are characterized by a relatively higher number of feldspars, while in the northern sandy lands, quartz mineral is more common (Zhao, 2015). The two dust sources in this study are consistent with these two desert regions. On the other hand, the high ice nucleation activity above −15 ∘C may be attributed to the attached biological materials on the dust particles (Tang et al., 2018). The reasons for the different INP activity of two pathway samples are discussed in detail below.
3.3 Surface ice-active-site density of dust particles
Figure 4a and b compare the total and size-resolved (four size classes, D50=5.6, 3.2, 1.8, and 1.0 µm) ns(T) values from this study with other ambient samples dominated by mineral dust, respectively. In the present study, the total ns(T) values (11 dust samples without sample D5 and D7) span 2 orders of magnitude from 105 to 107 m−2 at −15 ∘C. The ns(T) for D50=5.6 µm suggests higher nucleation activity, while the other three size classes show similar freezing performances. The ns(T) values of this study are compared to literature values. Price et al. (2018) reported airborne ice-nucleating particles in the dusty tropical Atlantic, which is near the Sahara desert in Africa (referred to as P18 from here on). Reicher et al. (2019) characterized the properties of size-segregated mineral dust sampled during dust events in the eastern Mediterranean (referred to as R19 from here on) and found that the ns(T) values increased with particle size.
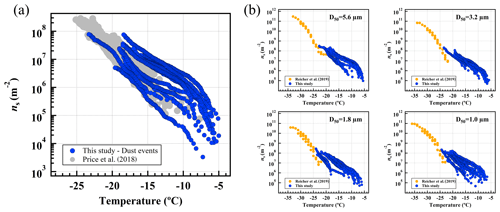
Figure 4Comparison of Chinese mineral dust with African and east Mediterranean mineral dust. (a) Total surface ice-active-site density (ns(T)) from this study compared with aircraft measurements of mineral-dust-dominated samples in the eastern tropical Atlantic (solid grey circles) from Price et al. (2018). (b) Size-resolved ns(T) compared with mineral dust samples collected in the eastern Mediterranean (solid yellow circles) from Reicher et al. (2019).
The total ns(T) values of most samples in this study are in agreement with P18, although a few samples are more active than P18 at higher temperatures (above −15 ∘C). The measured temperature dependency in this study is consistent with that observed in R19 in four size classes. The difference in the temperature range between this study (−25 to −5 ∘C) and R19 (−35 to −20 ∘C) is due to the droplet volume (0.5 nL in R19, in contrast to 1 µL in the present study). Larger droplets tend to freeze at higher temperatures because they contain a broader spectrum of nucleation-active sites (O'Sullivan et al., 2014). Overall, we demonstrate that despite different origins of the dust samples investigated here and in R19, as well as the varying atmospheric transport processes, both the total and size-resolved ice nucleation activity showed similarities (within 1 or 2 orders of magnitude) over a wide range of temperatures (−25 to −5 ∘C), which is consistent with the conclusions of previous studies (Niemand et al., 2012; DeMott et al., 2015; Kaufmann et al., 2016; Price et al., 2018; Reicher et al., 2019).
However, some samples in this study were more active than the measurements in the above two studies. Three hypotheses are proposed to explain the possible reasons. First is the content of feldspar in mineral dust. Nickovic et al. (2012) suggested that Asia has the highest feldspar content (up to 28 %), much higher than that in central Sahara (∼16 %). Although not all feldspars are effective INPs (Harrison et al., 2016), feldspar content is thought to be an important factor. Some efficient samples in this study were mainly from northwest China (see Sect. 3.2), and we cannot exclude the effect of feldspar content on ice nucleation activity when comparing dust particles from different deserts. Note that this is only a possible conjecture based on very limited evidence, and more further studies are needed. Secondly, in light of the difference in the vertical distributions of dust particles, the concentration of larger particles near the ground is higher (Maki et al., 2019), suggesting that the ns(T) values of near-surface-collected samples may be higher; i.e., they may show more efficient INP activity than the aircraft measurements. Finally, another possible cause for the variability in ns(T) values above −15 ∘C may be the contribution of biological materials, which are considered to be more active INPs than dust particles (Murray et al., 2012; Hoose and Mohler, 2012; Kanji et al., 2017). Mineral-dust-dominated particles in our study were collected in the spring, when the plants germinate, grow, and bloom. During the transport process, the air masses passed above grasslands, forests, and cities, so that mineral dust may mix with or serve as a carrier of other more active species, possibly bacteria, fungi, pollen, plant materials, and other microorganisms (Pratt et al., 2009; Tang et al., 2018; Gat et al., 2017). It has been shown that fungal ice-nucleating proteins preferentially bind to and confer their ice-nucleating properties to mineral dust particles (O'Sullivan et al., 2016). In another study, O'Sullivan et al. (2014) found that a major fraction of INPs stemmed from biogenic components in the soil for temperatures above −15 ∘C.
3.4 Contribution of heat-sensitive INPs
Previous studies have suggested that biological materials may attach to or mix with dust particles and play an important contribution to INP number concentration during dust events (Pratt et al., 2009; Creamean et al., 2013; Boose et al., 2016a; Mazar et al., 2016; Gat et al., 2017). Because biological ice nucleation is mainly induced by proteinaceous components, inactivation by heat treatment is considered to be a common way to identify biological ice nucleation activity (Christner et al., 2008a, b; Garcia et al., 2012; O'Sullivan et al., 2014). In this study, heat-resistant INPs represent those particles that can initiate freezing after heat treatment (heated to 95 ∘C for 30 min). The suspensions of five size classes (D50=10, 5.6, 3.2, 1.8, and 1.0 µm) were measured before and after heat treatment to calculate original and heat-resistant NINP, respectively. We subtracted heat-resistant NINP from the original concentration to evaluate the contribution of heat-sensitive INPs, which are mainly considered to be proteinaceous biological materials at high temperatures.
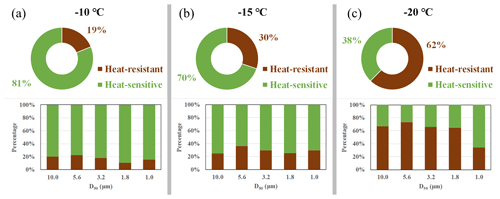
Figure 5A summary of heating test results for various size classes. Characterization of NINP after heat treatment is presented for three freezing temperatures, −10 ∘C (a), −15 ∘C (b), and −20 ∘C (c). The pie charts present the average contribution of heat-resistant and heat-sensitive INPs based on five analyzed size classes (D50≥1.0 µm), while the bar charts present the contribution for each size class (D50=10, 5.6, 3.2, 1.8, and 1.0 µm).
Figure 5 illustrates the average proportion of heat-resistant and heat-sensitive INPs for five different size classes at three temperatures: −10, −15, and −20 ∘C, derived from 12 sets of samples (sample M5 was not included due to experimental preparation). At higher temperatures, heat-sensitive INPs dominated, with 81±12 % at −10 ∘C and 70±15 % at −15 ∘C. At −20 ∘C, and heat-resistant INPs increased to 62±21 %, in comparison with 19±12 % at −10 ∘C (see Table S3). The above uncertainties correspond to the standard deviation of 12 samples at each temperature. In general, the contribution of heat-sensitive INPs is similar for different particle size classes at a given temperature (see Table S4). This proportion is related to the balance of biological materials and active mineral dust. Microorganisms may be more common in larger particles due to larger surface area (Gong et al., 2020). On the other hand, larger particles may contain more efficient mineral species, such as feldspars (Leinen et al., 1994), which are expected to be heat-resistant.
Figure 6 compares the freezing properties before and after heat treatment, based on those samples (with D50≥1.0 µm) originating from northwest and north pathways. Upon heating, the most prominent INP type above −10 ∘C was completely and mostly lost in north and northwest samples, respectively (see Fig. 6a and Table S5). In north samples, these INPs were less common than in northwest samples to begin with, a difference that extended to almost an order of magnitude in some of the cases (e.g., NINP at ∘C). The same conclusion can be derived from Table 2, in which heat-sensitive INPs contributed 88±2 % at −10 ∘C and 79±3 % at −15 ∘C in the northwest samples, while they contributed only 69±19 % at −10 ∘C and 54±20 % at −15 ∘C for north samples. This is probably the reason why samples from the north pathway were less sensitive to heat treatment in general. When comparing ns(T) curves of north and northwest samples in Fig. 6b, they were within an order of magnitude after heating (see Fig. S4). This may suggest that after heat-sensitive INPs were removed the two transport pathways are now dominated by similar material, which is probably mineral dust. However, it should be noted that the nucleation activity of all northwest samples was higher than that of the north samples, suggesting that there might be a difference in mineral composition (e.g., feldspar content), although it was far less important than the contribution of biological materials.
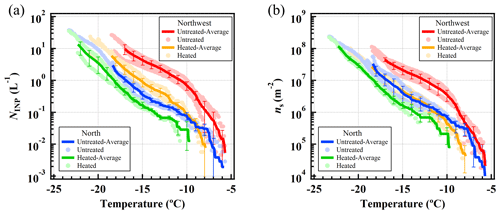
Figure 6Effect of heat treatment on INP concentration and activity. (a) NINP and (b) ns(T) of northwest and north samples before (marked as “Untreated”, solid light red and blue circles) and after (marked as “Heated”, solid light yellow and green circles) heat treatment. The average NINP and ns(T) of samples in the same pathway are presented as solid lines and marked by corresponding colors. Error bars are the standard deviations. The two figures present results with D50≥1.0 µm.
Table 2Average percentage of heat-sensitive INPs of the collected samples (D50≥1.0 µm) at two temperatures.
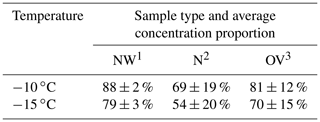
Note: the indicated uncertainties represent the standard deviations. 1 NW: heat-sensitive INPs of the northwest samples (D50≥1.0 µm, samples M6–M8 and D7). 2 N: heat-sensitive INPs of the north samples (D50≥1.0 µm, samples M3 and D6). 3 OV: heat-sensitive INPs of the overall samples (D50≥1.0 µm, 12 dust samples).
Overall, heat-sensitive components dominated the freezing at higher temperatures (above −15 ∘C), and their contribution decreased pronouncedly at lower temperatures. Recently, Harrison et al. (2019) found that some quartz samples are sensitive to aging in aqueous suspension. We cannot rule out the presence of quartz, or determine the fraction of specific quartz in heat-sensitive INPs at −20 ∘C. The higher proportion of heat-sensitive INPs at D50=1.0 µm at −20 ∘C may be attributed to the inactivation of quartz. However, quartz cannot be activated at −10 ∘C, and we can therefore conclude that a great proportion of INPs originated from heat-sensitive sites, mostly attributed to proteinaceous biological materials. This conclusion also confirms the hypotheses in Sect. 3.2 and 3.3; i.e., biological materials made a substantial contribution to ice nucleation activity above −15 ∘C.
3.5 Parameterizations of size-resolved ice-nucleation-active site densities
By combining the observational dataset in our study with those in R19, the new ns(T) parameterizations for size-resolved ice-nucleation-active-site densities of dust particles are calculated by an exponential function:
Here T is the temperature in degrees Celsius, and a and b are coefficients given in Table 3. These new parameterizations broaden the temperature range spanning from −35 to −6 ∘C.
Table 3Parameterization coefficients for different size classes based on the combined dataset in this study and R19 (Reicher et al., 2019).
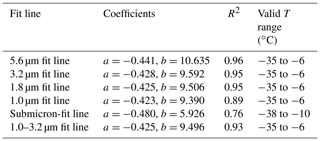
Four sizes, D50=5.6, 3.2, 1.8, and 1.0 µm, measured in both studies were considered for parameterization. The parameterizations for size-resolved dust particles are displayed in Fig. 7a, and their coefficients are given in Table 3. Here, the submicron-fit line, marked in Fig. 7a by the solid blue line, was derived from the dataset of D50=0.6, 0.3µm in R19 and D50=0.56, 0.32, 0.18 µm in this study, representing the ns(T) of submicron desert dust. Note that we only present here the cases dominated by mineral dust rather than all the data.
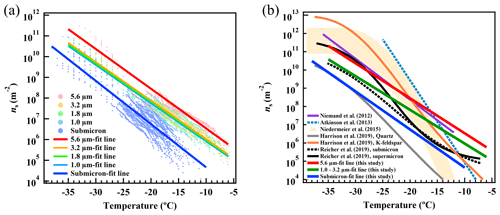
Figure 7Parameterizations of ice-nucleation-active-site densities ns(T) for different size classes. (a) Parameterizations developed from the combined dataset derived from this study and R19 (Reicher et al., 2019). Both the original data (colored solid points) and new fit lines (colored solid lines) are presented. (b) Comparison between the parameterizations derived from this study and those for desert dust (Niemand et al., 2012; Reicher et al., 2019) and single-mineral dust components (Atkinson et al., 2013; Niedermeier et al., 2015; Harrison et al., 2019).
An explicit size-dependent freezing efficiency was observed. The difference between the fit line of D50=5.6 µm and the submicron line was approximately 1 to 2 orders of magnitude, while the three fit lines of D50=3.2, 1.8, and 1.0 µm were almost overlapping and located between the fit lines of D50=5.6 µm and submicron particles. In this case, the D50=3.2, 1.8, and 1.0 µm lines were averaged into one 1.0–3.2 µm fit line, as shown in Fig. 7b.
Figure 7b compares our size-resolved parameterizations with those from previous studies, based on desert dust (Niemand et al., 2012; Reicher et al., 2019) and single-mineral dust components (Atkinson et al., 2013; Niedermeier et al., 2015; Harrison et al., 2019). Niemand et al. (2012) measured surface-collected dust samples (less than 5 µm) to derive the parameterization (solid purple line), which is consistent with our 5.6 µm fit line (solid red line) and is close to the 1.0–3.2 µm fit line (solid dark green line) for temperatures higher than −17 ∘C. For different K-feldspar content predictions, an overlap of the new fit lines with previous studies is observed in the temperature range from −25 to −15 ∘C, particularly for large particles (5.6 and 1.0–3.2 µm). At temperatures below −29 ∘C, the submicron-fit line coincides with the 12 % quartz parameterization by Harrison et al. (2019) but is 1 to 2 orders of magnitude higher above this temperature. The supermicron and submicron parameterizations developed by R19 agree within an order of magnitude with our three parameterizations in the lower temperature range ( ∘C). These two parameterizations underestimate the nucleation activity of large particles (5.6 and 1.0–3.2 µm) and fit for the submicron particles for temperatures higher than −20 ∘C.
We note that the quantitative mineralogical composition was not investigated in this study, so that we cannot explain the discrepancy accurately in terms of mineral composition. On the other hand, while relatively minor, measurement and calculation uncertainties should be borne in mind when comparing our parameterizations with other curves as well. First, different experimental methods introduce measurement errors. A cold-stage-based technique was applied in this study, while cloud simulation chamber (Niemand et al., 2012), laminar flow tube (Niedermeier et al., 2015), and many other cold-stage instruments (with varying size–volume droplets; Atkinson et al., 2013; Harrison et al., 2019; Reicher et al., 2019) were used to measure the activated fractions of tested particles/droplets at a given temperature. Then, the investigated particles came from various sources and underwent different processing, including airborne-collected and surface-collected (sieved or milled) samples and single-mineral dust components. Next, the calculation of ns(T) depends on a key parameter, particle surface area, which refers to the surface area of dust particles in laboratory studies, while referring to the surface area of total aerosol particles in this study and in R19. Furthermore, we adopted aerodynamic diameter to obtain ns(T), which underestimated the result (0.42 to 0.93 times) compared with that determined by the converted geometric diameter.
These airborne dust particles are composed of a complex mixture of various mineral components (e.g., feldspar, quartz, clay, and calcite), varying particle sizes, biological materials, and anthropogenic fine particulate matter. Its ice nucleation activity was determined by all factors and dominated by the most active substance. Despite the uncertainties, it is certain that there is an explicit size-dependent freezing efficiency over a large temperature range, and the contribution of biological materials to nucleation activity at ∘C is highlighted. Compared with mineral-composition-based parameterizations, the advantage of particle-size-based curves is that we do not need to know the complex mineralogical composition of dust. Only the particle size distribution, a widely monitored parameter, is required in size-based prediction. Furthermore, there is a vertical distribution of mineral dust in the atmosphere (Maki et al., 2019), implying potentially different contributions of these various size particles in cloud formation. Our size-dependent parameterizations can provide more refined simulation and prediction in theory, which needs to be confirmed in further model studies.
The ice nucleation activities of size-resolved airborne East Asian dust particles in the immersion mode were investigated for the first time. Compared to the non-dust event, the total INP concentrations during the East Asian dust events increased by approximately 2 orders of magnitude and ranged between 10−2 and 102 L−1 of standard air at temperatures between −25 and −5 ∘C. The total surface ice-active-site density, ns(T), spanned 2 orders of magnitude from 105 to 107 m−2 at −15 ∘C. Based on air mass back trajectory analysis, dust particles transported from China's northwest and northern deserts have different INP concentrations and ice nucleation efficiencies.
An explicit size dependence of both INP concentration and surface ice-active density was observed for Asian dust samples. The nucleation efficiency of dust particles increased with increasing particle size, while the concentration first increased rapidly with particle size and then leveled. Although larger particles had higher ns(T), their atmospheric number concentration was much less than that of fine particles, i.e., INP concentrations depend on the combined effect of both individual particle activity and the particle number at a given size. We also demonstrated that the differences of both the total and size-resolved ns(T) values of natural mineral dust particles from East Asia, North Africa, and eastern Mediterranean are within 1 to 2 orders of magnitude, suggesting similarities in ice nucleation activity.
During the all East Asian dust events, the average contributions of heat-sensitive INPs at three temperatures, −10, −15, and −20 ∘C, were 81±12 %, 70±15 %, and 38±21 %, respectively. And the average concentration proportion of heat-sensitive INPs was higher in the northwest than in the north pathway, indicating that the highest discrepancy in nucleation activity between the two pathways was attributed to the abundance of heat-sensitive INPs, although the presence of different mineral fractions cannot be excluded. These results not only explain the higher nucleation activity exhibited by our samples at relatively high temperatures (above −15 ∘C) but also emphasize the important role of biological materials during the seasonal Asian dust transport process.
A new set of size-resolved parameterizations based on the field observations in R19 and this study were developed, which are valid in an extended temperature range spanning from −35 to −6 ∘C, characterizing the ice nucleation properties of size-resolved mineral dust particles. The size of the particles controls their atmospheric lifetime, transport distance, and interactions with clouds, as larger particles sediment more quickly. Supermicron particles detected at high altitudes are more abundant than expected by sedimentation theory alone (Ryder et al., 2018), potentially suggesting the importance of larger particles for cloud formation. Larger particles are normally more active INPs, as they have a higher ns(T) or a larger surface area. In both field observations and laboratory experiments, it is easier to obtain particle size distributions than mineralogical compositions. Since only particle size distribution is required as an input without particle mineralogical compositions, the new particle-size-based parametrizations can be widely applied in models and help better characterize and predict INP concentrations related to natural mineral dust, especially related to long-range transport.
The data presented in this article can be accessed through the corresponding author Zhijun Wu (zhijunwu@pku.edu.cn).
The supplement related to this article is available online at: https://doi.org/10.5194/acp-21-3491-2021-supplement.
ZW designed and led the experiments. JCC and JC collected the samples during dust events. JCC, JC, XF, and MH maintained the apparatuses and collected the dataset. The measurements and analysis of field samples were performed by JCC under the guidance of ZW and with the help of JC. All authors discussed the results and contributed to the writing of this paper. JCC prepared the manuscript with contributions from all co-authors. ZW, JC, NR, YR, and MH proofread and helped improve earlier versions of the paper.
The authors declare that they have no conflict of interest.
We sincerely thank our two referees for their valuable comments and constructive suggestions to improve the scientific and rigorous nature of our paper. We gratefully acknowledge the NOAA Air Resources Laboratory (ARL) for the provision of the HYSPLIT transport and dispersion model and MeteoInfoMap for the visualization and analysis used in this paper.
This research has been supported by the National Natural Science Foundation of China (grant nos. 41875149, 42011530121, and 91844301) and by the Israel Science Foundation (grant no. 236/16).
This paper was edited by Ottmar Möhler and reviewed by two anonymous referees.
Archuleta, C. M., DeMott, P. J., and Kreidenweis, S. M.: Ice nucleation by surrogates for atmospheric mineral dust and mineral dust/sulfate particles at cirrus temperatures, Atmos. Chem. Phys., 5, 2617–2634, https://doi.org/10.5194/acp-5-2617-2005, 2005.
Ardon-Dryer, K. and Levin, Z.: Ground-based measurements of immersion freezing in the eastern Mediterranean, Atmos. Chem. Phys., 14, 5217–5231, https://doi.org/10.5194/acp-14-5217-2014, 2014.
Atkinson, J. D., Murray, B. J., Woodhouse, M. T., Whale, T. F., Baustian, K. J., Carslaw, K. S., Dobbie, S., O'Sullivan, D., and Malkin, T. L.: The importance of feldspar for ice nucleation by mineral dust in mixed-phase clouds, Nature, 498, 355–358, https://doi.org/10.1038/nature12278, 2013.
Augustin-Bauditz, S., Wex, H., Kanter, S., Ebert, M., Niedermeier, D., Stolz, F., Prager, A., and Stratmann, F.: The immersion mode ice nucleation behavior of mineral dusts: A comparison of different pure and surface modified dusts, Geophys. Res. Lett., 41, 7375–7382, https://doi.org/10.1002/2014gl061317, 2014.
Augustin-Bauditz, S., Wex, H., Denjean, C., Hartmann, S., Schneider, J., Schmidt, S., Ebert, M., and Stratmann, F.: Laboratory-generated mixtures of mineral dust particles with biological substances: characterization of the particle mixing state and immersion freezing behavior, Atmos. Chem. Phys., 16, 5531–5543, https://doi.org/10.5194/acp-16-5531-2016, 2016.
Barker, L.: A Comparison of Nine Confidence Intervals for a Poisson Parameter When the Expected Number of Events is = 5, Am. Statist., 56, 85–89, https://doi.org/10.1198/000313002317572736, 2002.
Bi, K., McMeeking, G. R., Ding, D. P., Levin, E. J. T., DeMott, P. J., Zhao, D. L., Wang, F., Liu, Q., Tian, P., Ma, X. C., Chen, Y. B., Huang, M. Y., Zhang, H. L., Gordon, T. D., and Chen, P.: Measurements of Ice Nucleating Particles in Beijing, China, J. Geophys. Res.-Atmos., 124, 8065–8075, https://doi.org/10.1029/2019jd030609, 2019.
Boose, Y., Sierau, B., García, M. I., Rodríguez, S., Alastuey, A., Linke, C., Schnaiter, M., Kupiszewski, P., Kanji, Z. A., and Lohmann, U.: Ice nucleating particles in the Saharan Air Layer, Atmos. Chem. Phys., 16, 9067–9087, https://doi.org/10.5194/acp-16-9067-2016, 2016a.
Boose, Y., Welti, A., Atkinson, J., Ramelli, F., Danielczok, A., Bingemer, H. G., Plötze, M., Sierau, B., Kanji, Z. A., and Lohmann, U.: Heterogeneous ice nucleation on dust particles sourced from nine deserts worldwide – Part 1: Immersion freezing, Atmos. Chem. Phys., 16, 15075–15095, https://doi.org/10.5194/acp-16-15075-2016, 2016b.
Boucher, O., D. , Randall, P., Artaxo, C., Bretherton, G., Feingold, P., Forster, V.-M., Kerminen, Y., Kondo, H., Liao, U., Lohmann, P., Rasch, S. K., Satheesh, S., Sherwood, B., Stevens, and Zhang, X. Y.: Clouds and Aerosols, in: Climate Change 2013 – The Physical Science Basis: Working Group I Contribution to the Fifth Assessment Report of the Intergovernmental Panel on Climate Change, Intergovernmental Panel on Climate, Cambridge University Press, Cambridge, 571–658, 2014.
Chen, J., Pei, X. Y., Wang, H., Chen, J. C., Zhu, Y. S., Tang, M. J., and Wu, Z. J.: Development, Characterization, and Validation of a Cold Stage-Based Ice Nucleation Array (PKU-INA), Atmosphere, 9, 13, https://doi.org/10.3390/atmos9090357, 2018a.
Chen, J., Wu, Z. J., Augustin-Bauditz, S., Grawe, S., Hartmann, M., Pei, X. Y., Liu, Z. R., Ji, D. S., and Wex, H. K.: Ice-nucleating particle concentrations unaffected by urban air pollution in Beijing, China, Atmos. Chem. Phys., 18, 3523–3539, https://doi.org/10.5194/acp-18-3523-2018, 2018b.
Christner, B., Cai, R., Morris, C., McCarter, K., Foreman, C., Skidmore, M., Montross, S., and Sands, D.: Geographic, seasonal, and precipitation chemistry influence on the abundance and activity of biological ice nucleators in rain and snow, P. Natl. Acad. Sci. USA, 105, 18854–18859, https://doi.org/10.1073/pnas.0809816105, 2008a.
Christner, B. C., Morris, C. E., Foreman, C. M., Cai, R., and Sands, D. C.: Ubiquity of Biological Ice Nucleators in Snowfall, Science, 319, 1214, https://doi.org/10.1126/science.1149757, 2008b.
Connolly, P. J., Möhler, O., Field, P. R., Saathoff, H., Burgess, R., Choularton, T., and Gallagher, M.: Studies of heterogeneous freezing by three different desert dust samples, Atmos. Chem. Phys., 9, 2805–2824, https://doi.org/10.5194/acp-9-2805-2009, 2009.
Creamean, J. M., Suski, K. J., Rosenfeld, D., Cazorla, A., DeMott, P. J., Sullivan, R. C., White, A. B., Ralph, F. M., Minnis, P., Comstock, J. M., Tomlinson, J. M., and Prather, K. A.: Dust and Biological Aerosols from the Sahara and Asia Influence Precipitation in the Western U.S., Science, 339, 1572, https://doi.org/10.1126/science.1227279, 2013.
De Leo, V., Catucci, L., Di Mauro, A. E., Agostiano, A., Giotta, L., Trotta, M., and Milano, F.: Effect of ultrasound on the function and structure of a membrane protein: The case study of photosynthetic Reaction Center from Rhodobacter sphaeroides, Ultrason. Sonochem., 35, 103–111, https://doi.org/10.1016/j.ultsonch.2016.09.007, 2017.
DeMott, P. J., Prenni, A. J., Liu, X., Kreidenweis, S. M., Petters, M. D., Twohy, C. H., Richardson, M. S., Eidhammer, T., and Rogers, D. C.: Predicting global atmospheric ice nuclei distributions and their impacts on climate, P. Natl. Acad. Sci. USA, 107, 11217–11222, https://doi.org/10.1073/pnas.0910818107, 2010.
DeMott, P. J., Prenni, A. J., McMeeking, G. R., Sullivan, R. C., Petters, M. D., Tobo, Y., Niemand, M., Möhler, O., Snider, J. R., Wang, Z., and Kreidenweis, S. M.: Integrating laboratory and field data to quantify the immersion freezing ice nucleation activity of mineral dust particles, Atmos. Chem. Phys., 15, 393–409, https://doi.org/10.5194/acp-15-393-2015, 2015.
Eastwood, M. L., Cremel, S., Gehrke, C., Girard, E., and Bertram, A. K.: Ice nucleation on mineral dust particles: Onset conditions, nucleation rates and contact angles, J. Geophys. Res.-Atmos., 113, D22203, https://doi.org/10.1029/2008JD010639, 2008.
Garcia, E., Hill, T. C. J., Prenni, A. J., DeMott, P. J., Franc, G. D., and Kreidenweis, S. M.: Biogenic ice nuclei in boundary layer air over two U.S. High Plains agricultural regions, J. Geophys. Res.-Atmos., 117, D18209, https://doi.org/10.1029/2012JD018343, 2012.
Gat, D., Mazar, Y., Cytryn, E., and Rudich, Y.: Origin-Dependent Variations in the Atmospheric Microbiome Community in Eastern Mediterranean Dust Storms, Environ. Sci. Technol., 51, 6709–6718, https://doi.org/10.1021/acs.est.7b00362, 2017.
Gong, X., Wex, H., van Pinxteren, M., Triesch, N., Fomba, K. W., Lubitz, J., Stolle, C., Robinson, T. B., Müller, T., Herrmann, H., and Stratmann, F.: Characterization of aerosol particles at Cabo Verde close to sea level and at the cloud level – Part 2: Ice-nucleating particles in air, cloud and seawater, Atmos. Chem. Phys., 20, 1451–1468, https://doi.org/10.5194/acp-20-1451-2020, 2020.
Gultepe, I., Heymsfield, A. J., Gallagher, M., Ickes, L., and Baumgardner, D.: Ice Fog: The Current State of Knowledge and Future Challenges, Meteorol. Monogr., 58, 4.1–4.24, https://doi.org/10.1175/AMSMONOGRAPHS-D-17-0002.1, 2017.
Harrison, A. D., Whale, T. F., Carpenter, M. A., Holden, M. A., Neve, L., O'Sullivan, D., Vergara Temprado, J., and Murray, B. J.: Not all feldspars are equal: a survey of ice nucleating properties across the feldspar group of minerals, Atmos. Chem. Phys., 16, 10927–10940, https://doi.org/10.5194/acp-16-10927-2016, 2016.
Harrison, A. D., Lever, K., Sanchez-Marroquin, A., Holden, M. A., Whale, T. F., Tarn, M. D., McQuaid, J. B., and Murray, B. J.: The ice-nucleating ability of quartz immersed in water and its atmospheric importance compared to K-feldspar, Atmos. Chem. Phys., 19, 11343–11361, https://doi.org/10.5194/acp-19-11343-2019, 2019.
Hoffmann, N., Duft, D., Kiselev, A., and Leisner, T.: Contact freezing efficiency of mineral dust aerosols studied in an electrodynamic balance: quantitative size and temperature dependence for illite particles, Faraday Discuss., 165, 383–390, https://doi.org/10.1039/C3FD00033H, 2013.
Hoose, C. and Mohler, O.: Heterogeneous ice nucleation on atmospheric aerosols: a review of results from laboratory experiments, Atmos. Chem. Phys., 12, 9817–9854, https://doi.org/10.5194/acp-12-9817-2012, 2012.
Hu, M., Peng, J., Sun, K., Yue, D., Guo, S., Wiedensohler, A., and Wu, Z.: Estimation of Size-Resolved Ambient Particle Density Based on the Measurement of Aerosol Number, Mass, and Chemical Size Distributions in the Winter in Beijing, Environ. Sci. Technol., 46, 9941–9947, https://doi.org/10.1021/es204073t, 2012.
Huang, K., Zhuang, G., Li, J., Wang, Q., Sun, Y., Lin, Y., and Fu, J. S.: Mixing of Asian dust with pollution aerosol and the transformation of aerosol components during the dust storm over China in spring 2007, J. Geophys. Res.-Atmos., 115, D00K13, https://doi.org/10.1029/2009JD013145, 2010.
Iwata, A. and Matsuki, A.: Characterization of individual ice residual particles by the single droplet freezing method: a case study in the Asian dust outflow region, Atmos. Chem. Phys., 18, 1785–1804, https://doi.org/10.5194/acp-18-1785-2018, 2018.
Jiang, H., Yin, Y., Wang, X., Gao, R., Yuan, L., Chen, K., and Shan, Y.: The measurement and parameterization of ice nucleating particles in different backgrounds of China, Atmos. Res., 181, 72–80, https://doi.org/10.1016/j.atmosres.2016.06.013, 2016.
Jung, S., Tiwari, M. K., and Poulikakos, D.: Frost halos from supercooled water droplets, P. Natl. Acad. Sci. USA, 109, 16073–16078, https://doi.org/10.1073/pnas.1206121109, 2012.
Kanji, Z., Ladino, L., Wex, H., Boose, Y., Burkert-Kohn, M., Cziczo, D., and Krämer, M.: Ice Formation and Evolution in Clouds and Precipitation: Measurement and Modeling Challenges. Chapter 1: Overview of Ice Nucleating Particles, Meteorol. Monogr., 58, 1–25, https://doi.org/10.1175/AMSMONOGRAPHS-D-0006.1, 2017.
Kanji, Z. A. and Abbatt, J. P. D.: Ice Nucleation onto Arizona Test Dust at Cirrus Temperatures: Effect of Temperature and Aerosol Size on Onset Relative Humidity, J. Phys. Chem. A, 114, 935–941, https://doi.org/10.1021/jp908661m, 2010.
Kanji, Z. A., Florea, O., and Abbatt, J. P. D.: Ice formation via deposition nucleation on mineral dust and organics: dependence of onset relative humidity on total particulate surface area, Environ. Res. Lett., 3, 025004, https://doi.org/10.1088/1748-9326/3/2/025004, 2008.
Kaufmann, L., Marcolli, C., Hofer, J., Pinti, V., Hoyle, C. R., and Peter, T.: Ice nucleation efficiency of natural dust samples in the immersion mode, Atmos. Chem. Phys., 16, 11177–11206, https://doi.org/10.5194/acp-16-11177-2016, 2016.
Kiselev, A., Bachmann, F., Pedevilla, P., Cox, S. J., Michaelides, A., Gerthsen, D., and Leisner, T.: Active sites in heterogeneous ice nucleation – the example of K-rich feldspars, Science, 355, 367–371, https://doi.org/10.1126/science.aai8034, 2017.
Knopf, D. A., Alpert, P. A., Zipori, A., Reicher, N., and Rudich, Y.: Stochastic nucleation processes and substrate abundance explain time-dependent freezing in supercooled droplets, npj Clim. Atmos. Sci., 3, 2, https://doi.org/10.1038/s41612-020-0106-4, 2020.
Korolev, A., McFarquhar, G., Field, P. R., Franklin, C., Lawson, P., Wang, Z., Williams, E., Abel, S. J., Axisa, D., Borrmann, S., Crosier, J., Fugal, J., Krämer, M., Lohmann, U., Schlenczek, O., Schnaiter, M., and Wendisch, M.: Mixed-Phase Clouds: Progress and Challenges, Meteorol. Monog., 58, 5.1–5.50, https://doi.org/10.1175/AMSMONOGRAPHS-D-17-0001.1, 2017.
Kramer, S. J., Alvarez, C., Barkley, A. E., Colarco, P. R., Custals, L., Delgadillo, R., Gaston, C. J., Govindaraju, R., and Zuidema, P.: Apparent dust size discrepancy in aerosol reanalysis in north African dust after long-range transport, Atmos. Chem. Phys., 20, 10047–10062, https://doi.org/10.5194/acp-20-10047-2020, 2020.
Kumar, A., Marcolli, C., and Peter, T.: Ice nucleation activity of silicates and aluminosilicates in pure water and aqueous solutions – Part 3: Aluminosilicates, Atmos. Chem. Phys., 19, 6059–6084, https://doi.org/10.5194/acp-19-6059-2019, 2019a.
Kumar, A., Marcolli, C., and Peter, T.: Ice nucleation activity of silicates and aluminosilicates in pure water and aqueous solutions – Part 2: Quartz and amorphous silica, Atmos. Chem. Phys., 19, 6035–6058, https://doi.org/10.5194/acp-19-6035-2019, 2019b.
Ladino, L., Stetzer, O., Lüönd, F., Welti, A., and Lohmann, U.: Contact freezing experiments of kaolinite particles with cloud droplets, J. Geophys. Res.-Atmos., 116, D22202, https://doi.org/10.1029/2011JD015727, 2011.
Leinen, M., Prospero, M. J., Arnold, E., and Blank, M.: Mineralogy of aeolian dust reaching the North Pacific Ocean: 1. Sampling and analysis, J. Geophys. Res.-Atmos., 99, 21017–21023, https://doi.org/10.1029/94JD01735, 1994.
Lohmann, U. and Feichter, J.: Global indirect aerosol effects: a review, Atmos. Chem. Phys., 5, 715–737, https://doi.org/10.5194/acp-5-715-2005, 2005.
Lohmann, U., Lüönd, F., and Mahrt, F.: An Introduction to Clouds: From the Microscale to Climate, Cambridge University Press, Cambridge, 2016.
Lüönd, F., Stetzer, O., Welti, A., and Lohmann, U.: Experimental study on the ice nucleation ability of size-selected kaolinite particles in the immersion mode, J. Geophys. Res.-Atmos., 115, D14201, https://doi.org/10.1029/2009JD012959, 2010.
Maki, T., Furumoto, S., Asahi, Y., Lee, K. C., Watanabe, K., Aoki, K., Murakami, M., Tajiri, T., Hasegawa, H., Mashio, A., and Iwasaka, Y.: Long-range-transported bioaerosols captured in snow cover on Mount Tateyama, Japan: impacts of Asian-dust events on airborne bacterial dynamics relating to ice-nucleation activities, Atmos. Chem. Phys., 18, 8155–8171, https://doi.org/10.5194/acp-18-8155-2018, 2018.
Maki, T., Bin, C., Kai, K., Kawai, K., Fujita, K., Ohara, K., Kobayashi, F., Davaanyam, E., Noda, J., Minamoto, Y., Shi, G., Hasegawa, H., and Iwasaka, Y.: Vertical distributions of airborne microorganisms over Asian dust source region of Taklimakan and Gobi Desert, Atmos. Environ., 214, 116848, https://doi.org/10.1016/j.atmosenv.2019.116848, 2019.
Marple, V. A., Rubow, K. L., and Behm, S. M.: A Microorifice Uniform Deposit Impactor (MOUDI): Description, Calibration, and Use, Aerosol Sc. Tech., 14, 434–446, https://doi.org/10.1080/02786829108959504, 1991.
Mason, B. J.: Ice-nucleating properties of clay minerals and stony meteorites, Q. J. Roy. Meteorol. Soc., 86, 552–556, https://doi.org/10.1002/qj.49708637014, 1960.
Mason, R. H., Chou, C., McCluskey, C. S., Levin, E. J. T., Schiller, C. L., Hill, T. C. J., Huffman, J. A., DeMott, P. J., and Bertram, A. K.: The micro-orifice uniform deposit impactor–droplet freezing technique (MOUDI-DFT) for measuring concentrations of ice nucleating particles as a function of size: improvements and initial validation, Atmos. Meas. Tech., 8, 2449–2462, https://doi.org/10.5194/amt-8-2449-2015, 2015.
Mason, R. H., Si, M., Chou, C., Irish, V. E., Dickie, R., Elizondo, P., Wong, R., Brintnell, M., Elsasser, M., Lassar, W. M., Pierce, K. M., Leaitch, W. R., MacDonald, A. M., Platt, A., Toom-Sauntry, D., Sarda-Estève, R., Schiller, C. L., Suski, K. J., Hill, T. C. J., Abbatt, J. P. D., Huffman, J. A., DeMott, P. J., and Bertram, A. K.: Size-resolved measurements of ice-nucleating particles at six locations in North America and one in Europe, Atmosp. Chem. Phys., 16, 1637–1651, https://doi.org/10.5194/acp-16-1637-2016, 2016.
Mazar, Y., Cytryn, E., Erel, Y., and Rudich, Y.: Effect of Dust Storms on the Atmospheric Microbiome in the Eastern Mediterranean, Environ. Sci. Technol., 50, 4194–4202, https://doi.org/10.1021/acs.est.5b06348, 2016.
McKendry, I. G., Macdonald, A. M., Leaitch, W. R., van Donkelaar, A., Zhang, Q., Duck, T., and Martin, R. V.: Trans-Pacific dust events observed at Whistler, British Columbia during INTEX-B, Atmos. Chem. Phys., 8, 6297–6307, https://doi.org/10.5194/acp-8-6297-2008, 2008.
Murray, B. J., O'Sullivan, D., Atkinson, J. D., and Webb, M. E.: Ice nucleation by particles immersed in supercooled cloud droplets, Chem. Soc. Rev., 41, 6519–6554, https://doi.org/10.1039/c2cs35200a, 2012.
Nickovic, S., Vukovic, A., Vujadinovic, M., Djurdjevic, V., and Pejanovic, G.: Technical Note: High-resolution mineralogical database of dust-productive soils for atmospheric dust modeling, Atmos. Chem. Phys., 12, 845–855, https://doi.org/10.5194/acp-12-845-2012, 2012.
Niedermeier, D., Hartmann, S., Clauss, T., Wex, H., Kiselev, A., Sullivan, R. C., DeMott, P. J., Petters, M. D., Reitz, P., Schneider, J., Mikhailov, E., Sierau, B., Stetzer, O., Reimann, B., Bundke, U., Shaw, R. A., Buchholz, A., Mentel, T. F., and Stratmann, F.: Experimental study of the role of physicochemical surface processing on the IN ability of mineral dust particles, Atmos. Chem. Phys., 11, 11131–11144, https://doi.org/10.5194/acp-11-11131-2011, 2011.
Niedermeier, D., Augustin-Bauditz, S., Hartmann, S., Wex, H., Ignatius, K., and Stratmann, F.: Can we define an asymptotic value for the ice active surface site density for heterogeneous ice nucleation?, J. Geophys. Res.-Atmos., 120, 5036–5046, https://doi.org/10.1002/2014jd022814, 2015.
Niemand, M., Möhler, O., Vogel, B., Vogel, H., Hoose, C., Connolly, P., Klein, H., Bingemer, H., DeMott, P., Skrotzki, J., and Leisner, T.: A Particle-Surface-Area-Based Parameterization of Immersion Freezing on Desert Dust Particles, J. Atmos. Sci., 69, 3077–3092, https://doi.org/10.1175/jas-d-11-0249.1, 2012.
O'Sullivan, D., Murray, B. J., Malkin, T. L., Whale, T. F., Umo, N. S., Atkinson, J. D., Price, H. C., Baustian, K. J., Browse, J., and Webb, M. E.: Ice nucleation by fertile soil dusts: relative importance of mineral and biogenic components, Atmos. Chem. Phys., 14, 1853–1867, https://doi.org/10.5194/acp-14-1853-2014, 2014.
O'Sullivan, D., Murray, B. J., Ross, J. F., and Webb, M. E.: The adsorption of fungal ice-nucleating proteins on mineral dusts: a terrestrial reservoir of atmospheric ice-nucleating particles, Atmos. Chem. Phys., 16, 7879–7887, https://doi.org/10.5194/acp-16-7879-2016, 2016.
O'Sullivan, D., Adams, M. P., Tarn, M. D., Harrison, A. D., Vergara-Temprado, J., Porter, G. C. E., Holden, M. A., Sanchez-Marroquin, A., Carotenuto, F., Whale, T. F., McQuaid, J. B., Walshaw, R., Hedges, D. H. P., Burke, I. T., Cui, Z., and Murray, B. J.: Contributions of biogenic material to the atmospheric ice-nucleating particle population in North Western Europe, Sci. Rep., 8, 13821, https://doi.org/10.1038/s41598-018-31981-7, 2018.
Peckhaus, A., Kiselev, A., Hiron, T., Ebert, M., and Leisner, T.: A comparative study of K-rich and Na/Ca-rich feldspar ice-nucleating particles in a nanoliter droplet freezing assay, Atmos. Chem. Phys., 16, 11477–11496, https://doi.org/10.5194/acp-16-11477-2016, 2016.
Pinti, V., Marcolli, C., Zobrist, B., Hoyle, C. R., and Peter, T.: Ice nucleation efficiency of clay minerals in the immersion mode, Atmos. Chem. Phys., 12, 5859–5878, https://doi.org/10.5194/acp-12-5859-2012, 2012.
Porter, G. C. E., Sikora, S. N. F., Adams, M. P., Proske, U., Harrison, A. D., Tarn, M. D., Brooks, I. M., and Murray, B. J.: Resolving the size of ice-nucleating particles with a balloon deployable aerosol sampler: the SHARK, Atmos. Meas. Tech., 13, 2905–2921, https://doi.org/10.5194/amt-13-2905-2020, 2020.
Pratt, K. A., DeMott, P. J., French, J. R., Wang, Z., Westphal, D. L., Heymsfield, A. J., Twohy, C. H., Prenni, A. J., and Prather, K. A.: In situ detection of biological particles in cloud ice-crystals, Nat. Geosci., 2, 398–401, https://doi.org/10.1038/ngeo521, 2009.
Price, H. C., Baustian, K. J., McQuaid, J. B., Blyth, A., Bower, K. N., Choularton, T., Cotton, R. J., Cui, Z., Field, P. R., Gallagher, M., Hawker, R., Merrington, A., Miltenberger, A., Neely Iii, R. R., Parker, S. T., Rosenberg, P. D., Taylor, J. W., Trembath, J., Vergara-Temprado, J., Whale, T. F., Wilson, T. W., Young, G., and Murray, B. J.: Atmospheric Ice-Nucleating Particles in the Dusty Tropical Atlantic, J. Geophys. Res.-Atmos., 123, 2175–2193, https://doi.org/10.1002/2017jd027560, 2018.
Prospero, J. M.: Long-range transport of mineral dust in the global atmosphere: Impact of African dust on the environment of the southeastern United States, P. Natl. Acad. Sci. USA, 96, 3396, https://doi.org/10.1073/pnas.96.7.3396, 1999.
Pruppacher, H. R. and Klett, J. D.: Microphysics of Clouds and Precipitation: Second Revised and Enlarged Edition with an Introduction to Cloud Chemistry and Cloud Electricity, Kluwer Academic Publishers, Dordrecht, the Netherlands, 1997.
Qiao, K., Wu, Z. J., Pei, X. Y., Liu, Q. Y., Shang, D. J., Zheng, J., Du, Z. F., Zhu, W. F., Wu, Y. S., Lou, S. R., Guo, S., Chan, C. K., Pathak, R. K., Hallquist, M., and Hu, M.: Size-resolved effective density of submicron particles during summertime in the rural atmosphere of Beijing, China, J. Environ. Sci., 73, 69–77, https://doi.org/10.1016/j.jes.2018.01.012, 2018.
Reicher, N., Budke, C., Eickhoff, L., Raveh-Rubin, S., Kaplan-Ashiri, I., Koop, T., and Rudich, Y.: Size-dependent ice nucleation by airborne particles during dust events in the eastern Mediterranean, Atmos. Chem. Phys., 19, 11143–11158, https://doi.org/10.5194/acp-19-11143-2019, 2019.
Ryder, C. L., Marenco, F., Brooke, J. K., Estelles, V., Cotton, R., Formenti, P., McQuaid, J. B., Price, H. C., Liu, D., Ausset, P., Rosenberg, P. D., Taylor, J. W., Choularton, T., Bower, K., Coe, H., Gallagher, M., Crosier, J., Lloyd, G., Highwood, E. J., and Murray, B. J.: Coarse-mode mineral dust size distributions, composition and optical properties from AER-D aircraft measurements over the tropical eastern Atlantic, Atmos. Chem. Phys., 18, 17225–17257, https://doi.org/10.5194/acp-18-17225-2018, 2018.
Scanza, R., Mahowald, N., Ghan, S., Zender, C., Kok, J., Liu, X., Zhang, Y., and Albani, S.: Modeling dust as component minerals in the Community Atmosphere Model: Development of framework and impact on radiative forcing, Atmos. Chem. Phys., 15, 537–561, https://doi.org/10.5194/acp-15-537-2015, 2015.
Schrod, J., Weber, D., Drücke, J., Keleshis, C., Pikridas, M., Ebert, M., Cvetković, B., Nickovic, S., Marinou, E., Baars, H., Ansmann, A., Vrekoussis, M., Mihalopoulos, N., Sciare, J., Curtius, J., and Bingemer, H. G.: Ice nucleating particles over the Eastern Mediterranean measured by unmanned aircraft systems, Atmos. Chem. Phys., 17, 4817–4835, https://doi.org/10.5194/acp-17-4817-2017, 2017.
Si, M., Irish, V. E., Mason, R. H., Vergara-Temprado, J., Hanna, S. J., Ladino, L. A., Yakobi-Hancock, J. D., Schiller, C. L., Wentzell, J. J. B., Abbatt, J. P. D., Carslaw, K. S., Murray, B. J., and Bertram, A. K.: Ice-nucleating ability of aerosol particles and possible sources at three coastal marine sites, Atmos. Chem. Phys., 18, 15669–15685, https://doi.org/10.5194/acp-18-15669-2018, 2018.
Stein, A. F., Draxler, R. R., Rolph, G. D., Stunder, B. J. B., Cohen, M. D., and Ngan, F.: NOAA's HYSPLIT Atmospheric Transport and Dispersion Modeling System, B. Am. Meteorol. Soc., 96, 2059–2077, https://doi.org/10.1175/BAMS-D-14-00110.1, 2015.
Sun, J., Zhang, M., and Liu, T.: Spatial and temporal characteristics of dust storms in China and its surrounding regions, 1960–1999: Relations to source area and climate, J. Geophys. Res.-Atmos., 106, 10325–10333, https://doi.org/10.1029/2000JD900665, 2001.
Sun, Y., Zhuang, G., Wang, Y., Zhao, X., Li, J., Wang, Z., and An, Z.: Chemical composition of dust storms in Beijing and implications for the mixing of mineral aerosol with pollution aerosol on the pathway, J. Geophys. Res.-Atmos., 110, D24209, https://doi.org/10.1029/2005JD006054, 2005.
Tang, K., Huang, Z., Huang, J., Maki, T., Zhang, S., Shimizu, A., Ma, X., Shi, J., Bi, J., Zhou, T., Wang, G., and Zhang, L.: Characterization of atmospheric bioaerosols along the transport pathway of Asian dust during the Dust-Bioaerosol 2016 Campaign, Atmos. Chem. Phys., 18, 7131–7148, https://doi.org/10.5194/acp-18-7131-2018, 2018.
Tang, M., Cziczo, D. J., and Grassian, V. H.: Interactions of Water with Mineral Dust Aerosol: Water Adsorption, Hygroscopicity, Cloud Condensation, and Ice Nucleation, Chem. Rev., 116, 4205–4259, https://doi.org/10.1021/acs.chemrev.5b00529, 2016.
Textor, C., Schulz, M., Guibert, S., Kinne, S., Balkanski, Y., Bauer, S., Berntsen, T., Berglen, T., Boucher, O., Chin, M., Dentener, F., Diehl, T., Easter, R., Feichter, H., Fillmore, D., Ghan, S., Ginoux, P., Gong, S., Grini, A., Hendricks, J., Horowitz, L., Huang, P., Isaksen, I., Iversen, I., Kloster, S., Koch, D., Kirkevåg, A., Kristjansson, J. E., Krol, M., Lauer, A., Lamarque, J. F., Liu, X., Montanaro, V., Myhre, G., Penner, J., Pitari, G., Reddy, S., Seland, Ø., Stier, P., Takemura, T., and Tie, X.: Analysis and quantification of the diversities of aerosol life cycles within AeroCom, Atmos. Chem. Phys., 6, 1777–1813, https://doi.org/10.5194/acp-6-1777-2006, 2006.
Uno, I., Eguchi, K., Yumimoto, K., Takemura, T., Shimizu, A., Uematsu, M., Liu, Z., Wang, Z., Hara, Y., and Sugimoto, N.: Asian dust transported one full circuit around the globe, Nat. Geosci., 2, 557–560, https://doi.org/10.1038/ngeo583, 2009.
Vali, G.: Quantitative evaluation of experimental results an the heterogeneous freezing nucleation of supercooled liquids, J. Atmos. Sci., 28, 402–409, 1971.
Vali, G., DeMott, P. J., Möhler, O., and Whale, T. F.: Technical Note: A proposal for ice nucleation terminology, Atmos. Chem. Phys., 15, 10263–10270, https://doi.org/10.5194/acp-15-10263-2015, 2015.
Wang, Y. Q.: MeteoInfo: GIS software for meteorological data visualization and analysis, Meteorol. Appl., 21, 360–368, https://doi.org/10.1002/met.1345, 2014.
Welti, A., Lüönd, F., Stetzer, O., and Lohmann, U.: Influence of particle size on the ice nucleating ability of mineral dusts, Atmos. Chem. Phys., 9, 6705–6715, https://doi.org/10.5194/acp-9-6705-2009, 2009.
Wex, H., DeMott, P. J., Tobo, Y., Hartmann, S., Rösch, M., Clauss, T., Tomsche, L., Niedermeier, D., and Stratmann, F.: Kaolinite particles as ice nuclei: learning from the use of different kaolinite samples and different coatings, Atmos. Chem. Phys., 14, 5529–5546, https://doi.org/10.5194/acp-14-5529-2014, 2014.
Whale, T. F., Holden, M. A., Kulak, A. N., Kim, Y.-Y., Meldrum, F. C., Christenson, H. K., and Murray, B. J.: The role of phase separation and related topography in the exceptional ice-nucleating ability of alkali feldspars, Phys. Chem. Chem. Phys., 19, 31186–31193, https://doi.org/10.1039/C7CP04898J, 2017.
Wiacek, A., Peter, T., and Lohmann, U.: The potential influence of Asian and African mineral dust on ice, mixed-phase and liquid water clouds, Atmos. Chem. Phys., 10, 8649–8667, https://doi.org/10.5194/acp-10-8649-2010, 2010.
Wu, Z. J., Cheng, Y. F., Hu, M., Wehner, B., Sugimoto, N., and Wiedensohler, A.: Dust events in Beijing, China (2004–2006): comparison of ground-based measurements with columnar integrated observations, Atmos. Chem. Phys., 9, 6915–6932, https://doi.org/10.5194/acp-9-6915-2009, 2009.
Yakobi-Hancock, J. D., Ladino, L. A., and Abbatt, J. P. D.: Feldspar minerals as efficient deposition ice nuclei, Atmos. Chem. Phys., 13, 11175–11185, https://doi.org/10.5194/acp-13-11175-2013, 2013.
Zhang, X. Y., Arimoto, R., and An, Z. S.: Dust emission from Chinese desert sources linked to variations in atmospheric circulation, J. Geophys. Res.-Atmos., 102, 28041–28047, https://doi.org/10.1029/97jd02300, 1997.
Zhao, W.: Geochemistry characteristics of clay-sized fractions from East Asian deserts-mineral dust provenance, transport and tracer, Nanjing University, Nanjing, 2015.
Zolles, T., Burkart, J., Hausler, T., Pummer, B., Hitzenberger, R., and Grothe, H.: Identification of ice nucleation active sites on feldspar dust particles, J. Phys. Chem. A, 119, 2692–2700, https://doi.org/10.1021/jp509839x, 2015a.
Zolles, T., Burkart, J., Häusler, T., Pummer, B., Hitzenberger, R., and Grothe, H.: Identification of Ice Nucleation Active Sites on Feldspar Dust Particles, J. Phys. Chem. A, 119, 2692–2700, https://doi.org/10.1021/jp509839x, 2015b.