the Creative Commons Attribution 4.0 License.
the Creative Commons Attribution 4.0 License.
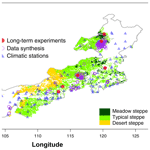
Simulating the spatiotemporal variations in aboveground biomass in Inner Mongolian grasslands under environmental changes
Zhongkui Luo
Yao Huang
Wenjuan Sun
Yurong Wei
Liujun Xiao
Jinhuan Zhu
Tingting Li
Wen Zhang
Grassland aboveground biomass (AGB) is a critical component of the global carbon cycle and reflects ecosystem productivity. Although it is widely acknowledged that dynamics of grassland biomass is significantly regulated by climate change, in situ evidence at meaningfully large spatiotemporal scales is limited. Here, we combine biomass measurements from six long-term (> 30 years) experiments and data in existing literatures to explore the spatiotemporal changes in AGB in Inner Mongolian temperate grasslands. We show that, on average, annual AGB over the past 4 decades is 2561, 1496 and 835 kg ha−1, respectively, in meadow steppe, typical steppe and desert steppe in Inner Mongolia. The spatiotemporal changes of AGB are regulated by interactions of climatic attributes, edaphic properties, grassland type and livestock. Using a machine-learning-based approach, we map annual AGB (from 1981 to 2100) across the Inner Mongolian grasslands at the spatial resolution of 1 km. We find that on the regional scale, meadow steppe has the highest annual AGB, followed by typical and desert steppe. Future climate change characterized mainly by warming could lead to a general decrease in grassland AGB. Under climate change, on average, compared with the historical AGB (i.e. average of 1981–2019), the AGB at the end of this century (i.e. average of 2080–2100) would decrease by 14 % under Representative Concentration Pathway (RCP) 4.5 and 28 % under RCP8.5. If the carbon dioxide (CO2) enrichment effect on AGB is considered, however, the estimated decreases in future AGB can be reversed due to the growing atmospheric CO2 concentrations under both RCP4.5 and RCP8.5. The projected changes in AGB show large spatial and temporal disparities across different grassland types and RCP scenarios. Our study demonstrates the accuracy of predictions in AGB using a modelling approach driven by several readily obtainable environmental variables and provides new data at a large scale and fine resolution extrapolated from field measurements.
- Article
(4428 KB) - Full-text XML
-
Supplement
(920 KB) - BibTeX
- EndNote
Grassland occupies ∼ 40 % of the world land and is an essential component of global terrestrial ecosystems (Hufkens et al., 2016). Grassland provides plenty of ecosystem services such as supplying food to livestock and therefore meat and milk to humans (Sattari et al., 2016) and accumulating carbon from the atmosphere, thus mitigating global warming (O'Mara, 2012). All of these functions are more or less directly dependent on grassland biomass, which has been recognized to be significantly influenced by environmental changes and anthropogenic activities (Hovenden et al., 2019). Thus, quantifying the dynamics of grassland biomass and revealing the underlying mechanisms are of fundamental importance (Andresen et al., 2018).
Dynamics of grassland aboveground biomass (AGB) are driven by complex interactions among a series of environmental attributes such as climate variables (De Boeck et al., 2008; H. Wang et al., 2020). The magnitudes and directions of climate change effects on AGB can vary across different local environments as well. For example, climate warming can either contribute to AGB accumulation through reduction of constraints on low temperature (Gonsamo et al., 2018; Park et al., 2019) or go against AGB formation by aggravating water stress on plant growth (Fan et al., 2009; Hu et al., 2007). In addition, in most existing studies, the mean annual climate attributes (e.g. temperature and precipitation) have widely been treated as potential drivers on spatiotemporal variations in grassland biomass (Fan et al., 2009; Ma et al., 2008). However, growing evidence has demonstrated the importance of seasonality and intra-annual variability of climate in regulating the biomass dynamics (Godde et al., 2020; Grant et al., 2014). For example, Peng et al. (2013) reported that variations in seasonal precipitation significantly alter the annual net primary productivity (NPP) in Inner Mongolian grasslands. To date, climatic seasonality and intra-annual variability have seldom been considered in assessing grassland AGB, particularly at large extents of space and time. Moreover, recent studies have suggested the possible co-regulating effects of soil properties (Bhandari and Zhang, 2019; Jia et al., 2011), grassland type and grazing intensity (Eldridge and Delgado-Baquerizo, 2017) on AGB, which have also seldom been included in exploring the spatiotemporal changes in grassland AGB. Comprehensively considering these covariates, rather than including only a few mean annual climatic attributes, provides an opportunity to more accurately predict grassland AGB dynamics and disentangle the response of AGB to the complex interactions between environmental drivers.
Inner Mongolian grasslands account for more than half of China's northern temperate grassland area and have the nation's largest grassland biomass carbon stock (Piao et al., 2004). The annual productivity in this region tends to vary in response to climate change (Bai et al., 2008). Since the start of the 1980s, warming has been taking place in many parts of Inner Mongolia (Wang et al., 2019). Under this temperature rise, the spatiotemporal variation in grassland AGB, however, is still unclear. Although efforts have been made to quantify AGB dynamics at the regional scale, these studies used mainly remote-sensing approaches and generally showed large disparities (Guo et al., 2016; Long et al., 2010; Ma et al., 2010a). Evidence from datasets independent of remote-sensing products can certainly contribute to the assessments of spatiotemporal dynamics of AGB at the regional scale. In addition, the climate in the future is projected to experience substantial changes (IPCC, 2007) and thus significantly affect grassland AGB dynamics, while little is known about the fate of AGB under future climate changes. Furthermore, it has been reported that carbon dioxide (CO2) enrichment may increase plant productivity through enhancing photosynthetic rates and reducing stomatal conductance, thereby increasing water use efficiency (Fay et al., 2012; Pastore et al., 2019). This might provide an opportunity to mitigate or even reverse the harmful effects of other environmental changes on grassland AGB (Lee et al., 2010), e.g. the enhanced water limitations resulting from climate warming. The actual effects of CO2 enrichment on AGB, however, depend substantially on local environmental factors such as water availability (Brookshire and Weaver, 2015) and soil texture (Polley et al., 2019).
In this study, we collate a comprehensive dataset of in situ measurements on plant biomass and climatic records in Inner Mongolian grasslands from six long-term experiments and those data from existing literature. We calibrate and validate a machine-learning-based model for predicting the aboveground biomass in the study region, by treating tens of environmental covariates (climates, soils, livestock and grassland type) as predicting variables. Then, we map the annual aboveground biomass at a spatial resolution of 1 km over the periods of 1981–2019 (using historical climatic dataset) and 2020–2100 (using climate projections driven by two representative concentration pathways (e.g. Representative Concentration Pathway (RCP) 4.5 and RCP8.5)). We also include the possible effects of atmospheric CO2 enrichment on future grassland AGB dynamics in the study region.
2.1 Study region and datasets of grassland aboveground biomass
The study region (i.e. Inner Mongolian grasslands) is characterized mainly by a temperate climate (Q. Zhang et al., 2020) and thus is named Inner Mongolian temperate grasslands as well, which can be generally classified into three categories, i.e. meadow steppe, typical steppe and desert steppe (National Research Council, 1992). In brief, meadow steppe is distributed mainly in the eastern areas, typical steppe locates mostly in central Inner Mongolia and desert steppe is found mainly to the west of typical steppe (Fig. 1). In this study, we acquired two datasets of in situ aboveground biomass (AGB) in Inner Mongolian grasslands. First, we obtained the AGB at six long-term (i.e. more than 30 years) experimental sites across the study region (Fig. 1 and Wang, 2020). These six sites were established by the Inner Mongolia Meteorological Bureau of China in the early 1980s; measurements of AGB at each site have been carried out year by year since then. At each site, four fenced plots (i.e. four replicates) were set up to collect plant biomass data during plant growing seasons (e.g. from May to September). For each measurement replicate, the plants within a 1 m2 area were clipped and collected in a cloth bag. The samples were further air-dried to constant weights (weighed once every 3 d until the percent change in two consecutive weights is less than 2 %). It is noted that plant growth rate could peak at different periods across time and space. Following Scurlock et al. (2002), we determined the annual plant biomass as the largest observed monthly biomass during a year (normally at the end of August at Ergün and at the end of September at three other sites). Apart from measurements of these six long-term field experiments, we also retrieved a dataset of grassland AGB from Xu et al. (2018), who recently conducted a thorough literature synthesis and established a comprehensive dataset of plant biomass in the grasslands of northern China. For the dataset constructed by Xu et al. (2018), we used only the observations conducted in Inner Mongolian grasslands and with investigation time and coordinates clearly reported (Fig. 1). In general, the grassland AGB derived from these two different datasets (i.e. long-term experiments and literature synthesis) is comparable (Fig. S1 in the Supplement). In total, we obtained 511 individual measurements across 247 locations in the study region (Fig. 1, Wang, 2020).
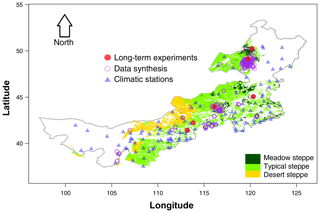
Figure 1Spatial distribution of grassland aboveground biomass observations and climatic stations in Inner Mongolia. The Inner Mongolian grasslands are grouped into three categories (i.e. meadow steppe, typical steppe and desert steppe). Observations of grassland biomass were both derived from the six long-term experimental sites and data synthesis of existing studies. The ground climatic records were obtained from the National Meteorological Information Centre (NMIC) of China.
2.2 Environmental covariates
Environmental covariates including climate, soil, grassland type and livestock were retrieved for both AGB driver assessment and machine-learning-based model fitting. For climatic covariates, we first obtained the daily climatic records of 120 climatic stations established in Inner Mongolia (Fig. 1) from the National Meteorological Information Centre (NMIC) of China. The daily climatic attributes such as minimum, average and maximum temperature and precipitation were transformed into monthly time series data using the daily2monthly function in the R package hydroTSM. Based on these monthly data, we calculated 23 bioclimatic variables (Table 1) with an annual time step over the period of 1981–2019 by using the biovars function in the R package dismo. By doing so, we aim to comprehensively consider the possible effects of seasonality and intra- and inter-annual variability of climates (Fick and Hijmans, 2017) on grassland AGB. By further applying an interpolation algorithm (Thornton et al., 1997) to these 23 bioclimatic variables at the 120 stations, we created the raster layers of the climatic attributes with a spatial resolution of 1 km year by year. For the edaphic covariates, we directly extracted 10 raster soil layers representing key soil physical and chemical properties (Table 1) at a 1 km spatial resolution in the study region from the ISRIC-WISE soil profile database (Batjes, 2016).
The grazing intensity in this study was represented by the quantity of three key livestock (i.e. cattle, goat and sheep; Table 1) because they make up the majority of livestock in the Inner Mongolian grasslands (National Bureau of Statistics of China, 1981–2019). Here, we first derived the regional distribution data for cattle (Fig. S2a), goats (Fig. S2b) and sheep (Fig. S2c) in 2010 in the study region from Gilbert et al. (2018). Then, we obtained the yearly total of each livestock in the study region (Fig. S2d) from the National Bureau of Statistics of China (1981–2019). By assuming a similar spatial distribution of livestock over time, we generated raster layers of each of the three livestock types year by year over the past 4 decades using the above-mentioned two datasets. In addition, a spatial layer of grassland type (i.e. meadow steppe, typical steppe and desert steppe; Fig. 1 and Table 1) at 1 km resolution was derived from the Vegetation Map of China (Zhang, 2007), the digital version of which is publicly obtainable (http://data.casearth.cn/sdo/detail/5c19a5680600cf2a3c557b6b, last access: 29 May 2020).
2.3 Machine learning models to predict grassland AGB
To predict grassland aboveground biomass (AGB) across the region, we generated a suite of machine-learning-based predictive models for AGB treating edaphic and climatic variables, grassland type and livestock (Table 1) as candidate predictors. Here, data from the 511 measurements (Fig. 1 and Wang, 2020) were used to fit the models. For the spatial layers of soil properties and grassland type, which were assumed to be constant over time, we retrieved the associated covariates using the geographical coordinates of the 511 measurements. For those variables varying over time (e.g. climatic variables and livestock), we extracted the associated attributes using both the locations and investigation year of the 511 measurements. In fitting the models, AGB is treated as a dependent variable, and the environmental covariates (Table 1) are treated as independent variables. Before fitting the models, we converted the categorical variables (i.e. grassland type) to dummy variables. This is to avoid simply deducing the dependent variables in a certain category using the independent variables (e.g. climate variables) in other categories in building the models. Then, the function findCorrelation in R package caret was used to exclude the environmental covariates with high multicollinearities. Following Brownlee (2020), the remaining attributes were further adopted in model training (80 % stratified samples) and validation (the remaining 20 % stratified samples). We used a 10-fold cross-validation to train a suite of machine learning models using three algorithms (i.e. random forest (RF), cubist and support vector machines (SVMs)), which are implemented in the R package caret. The amount of variance in AGB explained by each model was assessed by the coefficient of determination (R2). The root-mean-square error (RMSE, kg ha−1) was also calculated (, where n is sample size and Pi and Oi are the ith predicted and observed AGB, respectively) to compare the model simulations and observations. Apart from the three individual machine-learning-based models, we also derived an ensemble model by adopting a principal component analysis (PCA) approach based on the predictions of the above-mentioned three models. In brief, the smaller an individual model's RMSE, the more the model's outputs contribute to the ensemble predictions.
2.4 Assessment of drivers on AGB
We used three approaches to explore the effects of environmental covariates on grassland AGB. First, the machine learning models themselves provide assessments of the relative importance (RI) of each independent variable in predicting the dependent variable (e.g. grassland AGB in this study). In general, the greater the RI of a variable, the larger its influence on AGB. Second, we adopted the Mantel test (Mantel, 1967) to assess the relationship between similarity of different grassland types and the similarity of environmental covariates using the R package vegan. Here, the standardized Mantel's r (ranges from 0 to 1) is used to represent the strength of this relationship (the higher the Mantel's r, the stronger the correlation), and the associated significance is indicated by the P value determined from 999 randomizations (Legendre and Fortin, 1989). Third, we conducted a path analysis by using three latent variables, i.e. climate, soil and livestock, to evaluate their regulating effects on AGB. For each latent variable of climate and soil, the specific indicators were pre-identified using the above-mentioned R function findCorrelation to exclude those attributes with high multicollinearities. In constructing the inner model matrix of the path model, we hypothesized that all three latent variables have direct effects on AGB, and climate may also indirectly affect the dependent variable through influencing soil properties (Luo et al., 2019). Here, we adopted the partial least-squares (PLS) approach (Sanchez, 2013) and used the R package plspm to perform the path analysis. In interpreting the path analysis results, it is noted that the loadings of an indicator show the correlations between a latent variable and its indicators. All the indicators were standardized before the path analysis was performed.
2.5 Regional mapping and uncertainty analysis
Using the fitted machine-learning-based ensemble model, we mapped AGB in Inner Mongolian grasslands (at a spatial resolution of 1 km) on an annual time step in the history (1981–2019) and future (2020–2100). In mapping the historical AGB, the model is run using environmental covariates extracted from the regional data layers (see Sect. 2.2). Prediction uncertainty was quantified using a Monte Carlo analysis to develop the probability density functions (PDFs) for each edaphic, climatic and livestock variable within the ranges of mean ±10 %. The ensemble machine learning model was then run for 200 times in each grid with each independent variable assigned from the PDF. The average and coefficient variation (CV, calculated as the standard deviation divided by the average) were then determined in each grid using the 200 model outputs to represent the predicted AGB and the associated uncertainty, respectively.
For predictions of AGB in the future (i.e. 2020–2100), we included the climatic datasets projected by a typical CMIP5 global circulation model, i.e. CESM1-BGC, which was run by the National Center for Atmospheric Research (NCAR). Here, we directly obtained the processed climatic products constructed by Karger et al. (2020), who recently generated downscaled and bias-corrected temperature and precipitation datasets. Specifically, these future climatic datasets were driven by two scenarios of representative concentration pathways (RCP4.5 and RCP8.5) at a monthly step in this century. According to the model projections, mean annual temperature (MAT) under both RCPs will continue to increase in the following decades (Fig. S3a). The extent of climate warming is generally higher under RCP8.5 than that under RCP4.5 (Fig. S3a). The mean annual precipitation under both RCPs shows large inter-annual variabilities (Fig. S3b). After obtaining the future climate datasets, we also use the biovars function in the R environment (see Secxt. 2.2) to calculate the 23 bioclimatic attributes of interest (Table 1) for both RCPs year by year from 2020 to 2100. In projecting the future AGB dynamics using the ensemble machine learning model, we assume that the soil properties will not significantly change over time and current grazing intensity will keep relatively stable (i.e. the average number of livestock during 2014–2019 is used in future predictions). In addition, the uncertainty analysis for future AGB predictions was performed using the same approach as that adopted in mapping the historical AGB. Moreover, the CO2 concentrations have been projected to increase under the two RCPs (i.e. RCP4.5 and RCP8.5) used in this study (Fig. S4a). The growing CO2 concentrations can either increase AGB through enhanced photosynthetic rates (Fay et al., 2012; Lee et al., 2010) or have limited influences because of other environmental constraints on plant growth (Brookshire and Weaver, 2015). In this study, we deduced future AGB dynamics both including and not including the effect of CO2 enrichment on grassland AGB. In including CO2 enrichment effect, we used the relationship between CO2 concentration and aboveground NPP (ANPP) based on long-term experimental data derived from Polley et al. (2019). Here, we assumed a general linear response of AGB to increased CO2 concentrations; i.e. an increase of 100 ppm in CO2 leads to an increase of 850 kg ha−1 in grassland AGB (Fig. S4b). This linearly positive effect of CO2 on AGB is further applied to the model-predicted future AGB (i.e. the AGB not including CO2 enrichment effect). In brief, we used the annual CO2 concentrations under each RCP scenario in the future (Fig. S4a) and the average annual CO2 concentration over 2014–2019 as a baseline, together with the relationship between changes in CO2 and AGB (Fig. S4b), to determine the increment in AGB in each year from 2020 to 2100. All statistical analyses and graphical productions in this study were performed in R v3.6.3 (R Development Core Team, 2020).
The field measurements indicate that, on average, aboveground biomass (AGB) in Inner Mongolian grasslands is 1700 kg ha−1, ranging from 220 kg ha−1 (2.5 % confidence interval (CI)) to 4827 kg ha−1 (97.5 % CI, Fig. 2). Across the three grassland types, meadow steppe has the highest AGB (2561 Mg ha−1 ranging from 736 to 5537 Mg ha−1), followed by typical steppe (1496 Mg ha−1 ranging from 213 to 4418 Mg ha−1), and desert steppe has the lowest AGB (835 Mg ha−1 ranging from 234 to 1928 Mg ha−1, Fig. 2).
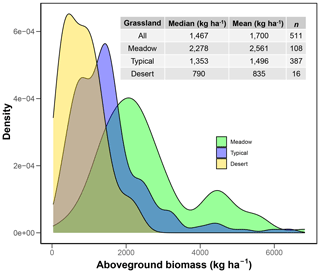
Figure 2Aboveground biomass distribution across different grassland types in Inner Mongolia. See Fig. 1 for the spatial distribution of the three grassland types in Inner Mongolia.
The fitted three individual machine learning algorithms (i.e. RF, cubist and SVM) can explain overall 32 %–48 % of the variance in observed AGB (Fig. 3a, b and c). The ensemble model of the three algorithms can better simulate the observations than any of those individual models (Fig. 3). On average, 52 % of the variance in the observations can be explained by the ensemble model (Fig. 3d). Although the variable importance differed among the three algorithms, climatic and livestock variables seem to substantially regulate the AGB dynamics (Fig. S5). After excluding the covariates with high multilinearities, the remaining 10 climatic attributes, 5 edaphic variables and 3 livestock predictors generally show small autocorrelations (Fig. 4a). The Mantel test suggests that, compared to the edaphic and livestock attributes, the climatic variables are in general stronger correlators of AGB in the three grassland types (Fig. 4a). Furthermore, the path analysis suggests that AGB shows small correlations with climate (using the 10 climatic indicators shown in Fig. 4a) and soil (reflected by the five edaphic properties shown in Fig. 4a) while significantly and positively correlating with livestock (Fig. 4b). We also found that climate can indirectly affect AGB via its influence on soil (Fig. 4b). It should be noticed that the small average magnitude with large variabilities of the loadings for climate (Fig. 4b) suggests the corresponding indicators for climate may distinctly affect AGB dynamics. It should also be noted that the overall performance of the fitted path model (R2=0.22, Fig. 4b) in explaining the variability of AGB is much poorer than that of the machine learning models (Fig. 3). This indicates the complex interactions between the environmental drivers in regulating AGB dynamics.
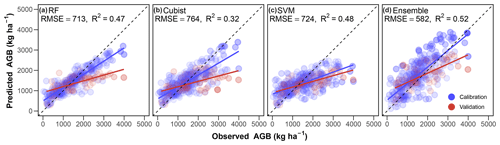
Figure 3Performances of models to predict grassland aboveground biomass (AGB). (a) Random forest (RF); (b) cubist; (c) support vector machines (SVM); (d), the ensemble model of (a)–(c). For each individual model, 80 % of the stratified samples of observations were used for model calibration, with the other 20 % used for validation. R2 and RMSE show the coefficient of determination and root-mean-square error of model validations. In model calibrations, the R2 is 0.82, 0.66 and 0.43 for RF, cubist and SVM, respectively, and RMSE is 359, 460 and 579 kg ha−1 for RF, cubist and SVM, respectively.
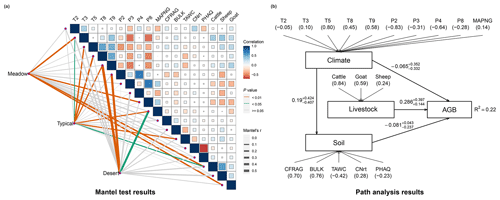
Figure 4Environmental drivers of aboveground grassland biomass (AGB). (a) The correlation matrix of environmental drivers and Mantel test results. The upper triangle shows the pairwise comparisons of predicting variables, with a colour gradient denoting Spearman's correlation coefficient. Taxonomic grassland type (i.e. meadow, typical and desert steppe) was related to each environmental factor by a partial (geographic-distance-corrected) Mantel test. Line colour represents the statistical significance, and line width denotes Mantel's r statistic for the corresponding distance correlations. (b) The path analysis results of the direction and magnitude of the effects of latent variable climate (reflected by T2, T3, T5, T8, T9, P2, P3, P4, P8 and MAPNG), soil (using CFRAG, BULK TAWC, CNrt and PHAQ as indicators) and livestock (using cattle, goats and sheep as indicators) on AGB. Numbers in parentheses represent the loadings (correlation coefficients) of the indicators to the latent variables. See Table 1 for descriptions of each variable, and see details in the Materials and methods section for the statistical analysis.
The model-simulated average AGB during 1981–2019 (Fig. 5a) and under RCP4.5 (Fig. 5b) and RCP8.5 (Fig. 5c) in the future shows large spatial variations. On average, the regional AGB during the past 4 decades is 1438 kg ha−1, and the corresponding lower and upper limits of the 95 % CI are 479 and 2284 kg ha−1, respectively (Fig. 5a). Across grassland types, meadow steppe has the highest average AGB (2194 Mg ha−1 ranging from 1153 to 2631 Mg ha−1), followed by typical steppe (1552 Mg ha−1 ranging from 539 to 2200 Mg ha−1) and desert steppe (893 Mg ha−1 ranging from 405 to 1341 Mg ha−1, Fig. 5a). Spatially, the average coefficient of variation (CV) in the predictions is lowest in meadow steppe (10.5 %), followed by desert steppe (14.6 %) and typical steppe (21.8 %, Fig. 5d). Over 1981–2019, the regional average AGB displayed a decreasing trend (Fig. 6a). Among the three grassland types, the historical changes in AGB (Fig. 6b, c and d) are in general consistent with that of the total Inner Mongolian grassland AGB (Fig. 6a). Moreover, the long-term field observations also show large inter-annual variabilities in the grassland biomass (Fig. 7) and can support our predicted temporal biomass dynamics at the regional scale (Fig. 6). For example, at four of the six sites, AGB showed a general decreasing trend (Fig. 7).
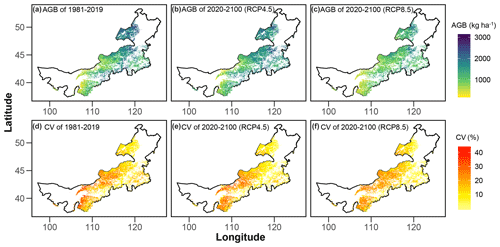
Figure 5Spatial patterns of Inner Mongolian grassland aboveground biomass (AGB) and the uncertainties in terms of coefficient of variations (CV). The upper panels show the average gridded AGB over 1981–2019 (a) and under two climate change scenarios (RCP4.5 b and RCP8.5 c) over 2020–2100. The lower panels (d, e, f) exhibit the associated CV of the upper panels. Please note that these estimations were derived from simulations without considering the atmospheric CO2 enrichment effects on AGB.
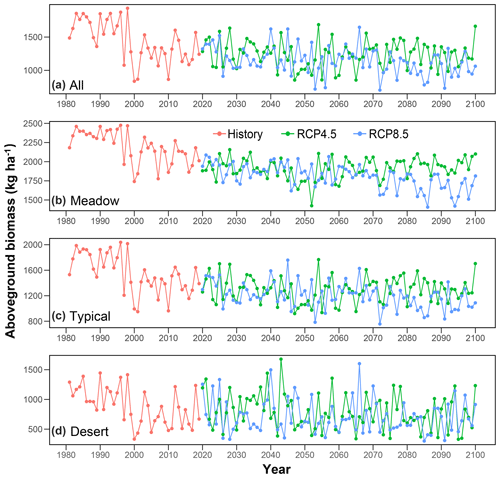
Figure 6Temporal variations in the predicted average aboveground biomass (AGB) in Inner Mongolian grasslands. Each year, data are averages of all the 1 km × 1 km grids (a) and across a certain grassland type at the regional scale (b, c, d). It should be noticed that these estimations were derived from simulations without considering the atmospheric CO2 effects on AGB.
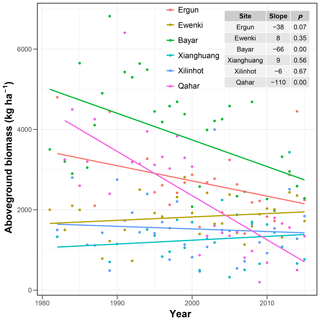
Figure 7Temporal changes in aboveground biomass (AGB) in the six long-term filed experiments in Inner Mongolian grasslands. The table inside shows the linear trends (slope, kg ha−1 yr−1) in AGB and the significance (reflected by P value).
If the CO2 enrichment effect on AGB is not considered, our predicting results show that future AGB in general decreases under both RCPs (i.e. RCP4.5 and RCP8.5, Fig. 6a and Table 2). Compared with the historical AGB (i.e. average AGB during 1981–2019, hereafter the same), on average, AGB at the end of this century (i.e. average of 2080–2100, hereafter the same) would decrease by 14 % under RCP4.5 and 28 % under RCP8.5, respectively (Table 2). The decreases in AGB under future climate change show large disparities across different grassland types and climate change scenarios. Compared with the historical average AGB, AGB at the end of this century under RCP4.5 is estimated to decrease by a smaller extent (i.e. 10 %) in meadow steppe than that in typical (16 %) and desert steppe (21 %, Table 2). In general, AGB under RCP8.5 would decrease by larger extents compared with those under RCP4.5. Under RCP8.5, the average AGB at the end of this century is estimated to experience 24 % (in meadow steppe), 30 % (in typical steppe) and 25 % (in desert steppe) reductions, compared with the averages over 1981–2019 (Table 2). The magnitudes and spatial patterns of CV in the simulations under both RCP4.5 (Fig. 5e) and RCP8.5 (Fig. 5f) are comparable with those during the period of 1981–2019 (Fig. 5d).
If the CO2 enrichment effect on AGB is included, the predicted losses in future AGB can be reversed under both RCP scenarios and over different grassland types (Fig. 8). By the end of this century, the regional average AGB is increased by 63 % under RCP4.5 and 232 % under RCP8.5 compared with the average AGB during 1981–2019 (Fig. 8a, Table 2). The magnitudes of increases in future AGB differ across different grassland types. For example for RCP4.5, the average AGB at the end of this century is estimated to increase by 40 % in meadow steppe, 55 % in typical steppe and 102 % in desert steppe compared with their counterparts during 1981–2019 (Fig. 8b, c and d, Table 2). The increases in AGB are much larger under RCP8.5 than those under RCP4.5. On average, under RCP8.5, the AGB at the end of this century is projected to enhance by 147 %, 212 % and 394 % in meadow, typical and desert steppe, respectively, compared with those over 1981–2019 (Fig. 8b, c and d, Table 2).
Our results, based on AGB observations derived from six long-term field experiments and literature synthesis, indicate the large spatial disparities in aboveground biomass across different grassland types (Fig. 2). This gradient spatial pattern in AGB is comparable with that of Ma et al. (2008), who carried out comprehensive field measurements and investigated 113 locations in Inner Mongolian temperate grasslands during 2002–2005. On the regional scale, we mapped grassland AGB at high spatial resolution, which shows that AGB generally decreases from north-eastern to south-western areas in the study region (Fig. 5a). Such a spatial pattern is also consistent with the maps generated from remote sensing derivations (Fig. S6). This demonstrates the accuracy of our data-driven predictions of AGB. It should be noted that existing mapping products of grassland AGB use mainly remote sensing approaches requiring inputs from satellite-based datasets (Guo et al., 2016; Jiao et al., 2019; Ma et al., 2010a). Our fitted machine learning model, however, uses only several readily obtainable environmental covariates (Fig. 4 and Table 1). Our results demonstrate the ability of machine learning approaches to effectively extrapolate grassland AGB to much larger spatiotemporal extents (e.g. Figs. 5 and 6).
Our simulation results show that, under the climate warming over the past 4 decades (Fig. S3), the average AGB generally experienced a declining trend in the study region (Fig. 6). This may partly support the possible negative effects of temperature rise on AGB that have been widely reported (De Boeck et al., 2008; H. Wang et al., 2020), particularly in arid and semi-arid ecosystems (Ma et al., 2010b). This harmful influence of warming on AGB is explainable. For example, in a system restrained by water availability (e.g. temperate grassland), warming can not only inhibit plant photosynthesis (Xu and Zhou, 2005) but also enhance evaporation and further intensify water stress (De Boeck et al., 2006), thereby decreasing grassland biomass. Precipitation has generally been recognized to have positive effects on AGB in the temperate grasslands (Hovenden et al., 2019; Ma et al., 2010a), which supports our findings in this study. For example, the simulated average AGB is relatively higher in the years with higher mean annual precipitation (e.g. 1998 and 2012) than that in other years (Fig. 6a). The importance of precipitation on AGB can be more reflected by the spatial patterns of these two attributes; e.g. AGB is much lower in the more arid regions (Fig. 5a) where soils suffer more severe water deficiencies. Apart from climatic factors, our results also demonstrate the co-regulating effects of soil conditions and livestock on the dynamics of grassland AGB as indicated by the machine learning models (Fig. S5) and the path analysis model (Fig. 4b). For example, the increasing trend in livestock over the past 4 decades (Fig. S2d) is generally in line with the overall decreasing trend in the contemporary AGB (Fig. 6a). It should be noted that the major drivers of the simulated temporal changes in AGB (Fig. 6) can vary during different periods in this study due to data unavailability, particularly for livestock. Specifically, AGB dynamics over 1981–2019 is co-regulated by changes in both climate and livestock (Figs. S2, S3 and S5). In future scenario simulations (e.g. 2020–2100, Fig. 6); however, AGB variations are predominantly controlled by climate since a constant grazing intensity was adopted over time in future predictions (see Materials and methods). We admit that the actual grazing intensity can vary over time in the future under different RCP scenarios, and simply assuming a stable grazing intensity over time can lead to substantial biases in AGB estimations. We need novel approaches to derive the temporal variations in grazing intensity at larger temporal extents.
Our estimations indicate that AGB can be substantially increased under future CO2 enrichment (Fig. 8). Here, several uncertainties and limitations should be noticed in interpreting our results. First, the gradient of CO2 concentrations in Polley et al. (2019), which is used to derive the effect of CO2 enrichment on AGB, has a smaller range (i.e. 250 to 500 ppm) than those under RCP8.5 (i.e. around 900 ppm by the end of this century, Fig. S4a). Here, extrapolations of such a relationship between CO2 concentration and AGB to larger extents of CO2 concentrations can lead to substantial uncertainties in estimations of AGB. Second, the local soil (Fay et al., 2012) and climatic (Brookshire and Weaver, 2015) factors can modify the actual CO2 enrichment effect on AGB, which may also result in large uncertainties in the quantified AGB. For example, any stimulation in plant growth is constrained by the availability of other resources required by plant growth (Reyes-Fox et al., 2014) such as soil water availability (Brookshire and Weaver, 2015). Consequently, the magnitude of the increases in AGB induced by CO2 enrichment estimated in this study, particularly under RCP8.5, can be largely overestimated due to possible deficiencies of either nutrients or water required by plant growth (S. Wang et al., 2020).
We also notice that our model predictions show larger inter-annual variations in AGB (Fig. 6a) than those in the estimations based on remote-sensing approaches (Fig. S6). In fact, the remote-sensing-derived AGB has also been bias-corrected by the field measurements (Jiao et al., 2019). Consequently, this disparity could be related to the difference of observed AGB datasets used in different studies. Specifically, the measurements of biomass used to calibrate remote sensing data (normalized difference vegetation index (NDVI)) in Jiao et al. (2019) were generally conducted during 2001–2015. Extrapolations of these observations from the short term (e.g. 2001–2015) to the much longer term (e.g. 1982–2015) might lead to underestimations in the long-term interannual variabilities. Our study, however, integrates the in-situ-observed data from six long-term (1982–2015) field experiments (Fig. 1), which can potentially better represent the AGB over larger temporal scales. It is noteworthy that the accuracy of our predictions on future grassland AGB relies substantially on the robustness of future climate change projections simulated by the global circulation models (GCMs) (e.g. CESM1-BGC). However, although CESM1-BGC (like all the other CMIP5 models) can simulate changes in temperature reasonably well, it may not predict precipitation well, particularly for eastern China which is affected by large-scale atmospheric circulations (Huang et al., 2013). In addition, the effects of solar radiation (Yu et al., 2021; J. Zhang et al., 2020) and its complex responses to dust aerosol (Fu et al., 2009; Qi et al., 2013; Wang et al., 2013) on plant photosynthesis and biomass formation were not considered in this study, which can be another source of uncertainties in the estimated AGB under future climate change. Last but not least, the assumption of space-for-time substitution has been widely debated and challenged (Johnson and Miyanishi, 2008; Walker et al., 2010). Although grassland type across space is treated as an independent predictor of AGB in this study, we admit that using the spatial gradients of observations to predict AGB backward or forward in time may still lead to large uncertainties. Consequently, caution should be exercised in interpreting the modelled future grassland AGB in this study.
Our results demonstrate that the aboveground biomass in Inner Mongolian grasslands shows large spatial and temporal variations during the past 4 decades, which is driven by a series of environmental covariates. Particularly, current climate change characterized mainly by warming together with an increased grazing intensity can have negative effects on grassland AGB. The decreases in AGB, however, can potentially be reversed by the positive effects of atmospheric CO2 enrichment. In addition, our results demonstrate that adopting a machine learning model approach with only a few readily obtainable environmental predictors can accurately capture AGB dynamics, which enables extrapolations of AGB across larger spatiotemporal extents. Moreover, our study provides new data on annual AGB in Inner Mongolian grasslands at fine spatial (1 km) and temporal (yearly) resolutions at large temporal scales (1981–2100).
The data that support the findings of this study are openly available at https://doi.org/10.6084/m9.figshare.13108430 (Wang, 2020).
The supplement related to this article is available online at: https://doi.org/10.5194/acp-21-3059-2021-supplement.
GW and YH conceived this study. GW conducted the data analysis with interpretations from ZL and YH. GW and ZL prepared the article with contributions from all authors.
The authors declare that they have no conflict of interest.
The authors acknowledge the people who conducted the filed long-term experiments and collected the observed data.
This study was financially supported by the National Natural Science Foundation of China (grant nos. 41775156 and 41590875) and the Strategic Priority Research Program of the Chinese Academy of Sciences (grant no. XDA26010103).
This paper was edited by Jianping Huang and reviewed by Tao Wang and one anonymous referee.
Andresen, L. C., Yuan, N., Seibert, R., Moser, G., Kammann, C. I., Luterbacher, J., Erbs, M., and Müller, C.: Biomass responses in a temperate European grassland through 17 years of elevated CO2, Global Change Biol., 24, 3875–3885, 2018.
Bai, Y., Wu, J., Xing, Q., Pan, Q., Huang, J., Yang, D., and Han, X.: Primary production and rain use efficiency across a precipitation gradient on the Mongolia plateau, Ecology, 89, 2140–2153, 2008.
Batjes, N. H.: Harmonized soil property values for broad-scale modelling (WISE30sec) with estimates of global soil carbon stocks, Geoderma, 269, 61–68, https://doi.org/10.1016/j.geoderma.2016.01.034, 2016.
Bhandari, J. and Zhang, Y.: Effect of altitude and soil properties on biomass and plant richness in the grasslands of Tibet, China, and Manang District, Nepal, Ecosphere, 10, e02915, https://doi.org/10.1002/ecs2.2915, 2019.
Brookshire, E. N. J. and Weaver, T.: Long-term decline in grassland productivity driven by increasing dryness, Nat. Commun., 6, 7148, https://doi.org/10.1038/ncomms8148, 2015.
Brownlee, J.: Machine Learning Mastery, Train-Test Split for Evaluating Machine Learning Algorithms, available at: https://machinelearningmastery.com/train-test-split-for-evaluating-machinelearning-algorithms/, last access: 1 July 2020.
De Boeck, H. J., Lemmens, C. M., Bossuyt, H., Malchair, S., Carnol, M., Merckx, R., Nijs, I., and Ceulemans, R.: How do climate warming and plant species richness affect water use in experimental grasslands?, Plant Soil, 288, 249–261, 2006.
De Boeck, H. J., Lemmens, C. M. H. M., Zavalloni, C., Gielen, B., Malchair, S., Carnol, M., Merckx, R., Van den Berge, J., Ceulemans, R., and Nijs, I.: Biomass production in experimental grasslands of different species richness during three years of climate warming, Biogeosciences, 5, 585–594, https://doi.org/10.5194/bg-5-585-2008, 2008.
Eldridge, D. J. and Delgado-Baquerizo, M.: Continental-scale impacts of livestock grazing on ecosystem supporting and regulating services, Land Degrad. Dev., 28, 1473–1481, 2017.
Fan, J., Wang, K., Harris, W., Zhong, H., Hu, Z., Han, B., Zhang, W., and Wang, J.: Allocation of vegetation biomass across a climate-related gradient in the grasslands of Inner Mongolia, J. Arid Environ., 73, 521–528, 2009.
Fay, P. A., Jin, V. L., Way, D. A., Potter, K. N., Gill, R. A., Jackson, R. B., and Polley, H. W.: Soil-mediated effects of subambient to increased carbon dioxide on grassland productivity, Nat. Clim. Change, 2, 742–746, https://doi.org/10.1038/nclimate1573, 2012.
Fick, S. E. and Hijmans, R. J.: WorldClim 2: new 1-km spatial resolution climate surfaces for global land areas, Int. J. Climatol., 37, 4302–4315, https://doi.org/10.1002/joc.5086, 2017.
Fu, Q., Thorsen, T., Su, J., Ge, J., and Huang, J.: Test of Mie-based single-scattering properties of non-spherical dust aerosols in radiative flux calculations, J. Quant. Spectrosc. Ra., 110, 1640–1653, 2009.
Gilbert, M., Nicolas, G., Cinardi, G., Van Boeckel, T. P., Vanwambeke, S. O., Wint, G. W., and Robinson, T. P.: Global distribution data for cattle, buffaloes, horses, sheep, goats, pigs, chickens and ducks in 2010, Sci. Data, 5, 1–11, 2018.
Godde, C. M., Boone, R., Ash, A. J., Waha, K., Sloat, L., Thornton, P. K., and Herrero, M.: Global rangeland production systems and livelihoods at threat under climate change and variability, Environ. Res. Lett., 15, 044021, https://doi.org/10.1088/1748-9326/ab7395, 2020.
Gonsamo, A., Chen, J. M., and Ooi, Y. W.: Peak season plant activity shift towards spring is reflected by increasing carbon uptake by extratropical ecosystems, Global Change Biol., 24, 2117–2128, 2018.
Grant, K., Kreyling, J., Dienstbach, L. F., Beierkuhnlein, C., and Jentsch, A.: Water stress due to increased intra-annual precipitation variability reduced forage yield but raised forage quality of a temperate grassland, Agr. Ecosyst. Environ., 186, 11–22, 2014.
Guo, L. H., Hao, C. Y., Wu, S. H., Zhao, D. S., and Gao, J. B.: Analysis of changes in net primary productivity and its susceptibility to climate change of Inner Mongolian grasslands using the CENTURY model, Geogr. Res., 35, 271–284, 2016 (in Chinese with English abstract).
Hovenden, M. J., Leuzinger, S., Newton, P. C., Fletcher, A., Fatichi, S., Lüscher, A., Reich, P. B., Andresen, L. C., Beier, C., and Blumenthal, D. M.: Globally consistent influences of seasonal precipitation limit grassland biomass response to elevated CO2, Nat. Plants, 5, 167–173, 2019.
Hu, Z., Fan, J., Zhong, H., and Yu, G.: Spatiotemporal dynamics of aboveground primary productivity along a precipitation gradient in Chinese temperate grassland, Sci. China Ser. D, 50, 754–764, https://doi.org/10.1007/s11430-007-0010-3, 2007.
Huang, D.-Q., Zhu, J., Zhang, Y.-C., and Huang, A.-N.: Uncertainties on the simulated summer precipitation over Eastern China from the CMIP5 models, J. Geophys. Res.-Atmos., 118, 9035–9047, https://doi.org/10.1002/jgrd.50695, 2013.
Hufkens, K., Keenan, T. F., Flanagan, L. B., Scott, R. L., Bernacchi, C. J., Joo, E., Brunsell, N. A., Verfaillie, J., and Richardson, A. D.: Productivity of North American grasslands is increased under future climate scenarios despite rising aridity, Nat. Clim. Change, 6, 710–714, 2016.
IPCC: Climate change 2007: impacts, adaptation and vulnerability, Contribution of working group II to the fourth assessment report of the intergovernmental panel on climate change, Cambridge University Press, Cambridge, UK, 2007.
Jia, X., Shao, M., Wei, X., Horton, R., and Li, X.: Estimating total net primary productivity of managed grasslands by a state-space modeling approach in a small catchment on the Loess Plateau, China, Geoderma, 160, 281–291, https://doi.org/10.1016/j.geoderma.2010.09.016, 2011.
Jiao, C. C., Yu, G. R., Chen, Z., and He, N. P.: A dataset for aboveground biomass of the northern temperate and Tibetan Plateau alpine grasslands in China, based on field investigation and remote sensing inversion (1982–2015), China Sci. Data, 4, 63–75, https://doi.org/10.11922/csdata.2018.0029.zh, 2019.
Johnson, E. A. and Miyanishi, K.: Testing the assumptions of chronosequences in succession, Ecol. Lett., 11, 419–431, https://doi.org/10.1111/j.1461-0248.2008.01173.x, 2008.
Karger, D. N., Schmatz, D. R., Dettling, G., and Zimmermann, N. E.: High-resolution monthly precipitation and temperature time series from 2006 to 2100, Sci. Data, 7, 248, https://doi.org/10.1038/s41597-020-00587-y, 2020.
Lee, M., Manning, P., Rist, J., Power, S. A., and Marsh, C.: A global comparison of grassland biomass responses to CO2 and nitrogen enrichment, Philos. T. R. Soc. B, 365, 2047–2056, https://doi.org/10.1098/rstb.2010.0028, 2010.
Legendre, P. and Fortin, M. J.: Spatial pattern and ecological analysis, Vegetatio, 80, 107–138, 1989.
Long, L. H., Li, X. B., Wang, H., Wei, D. D., and Zhang, C.: Net primary productivity (NPP) of grassland ecosystem and its relationship with climate in Inner Mongolia, Acta Ecologica Sinica, 30, 1367–1378, 2010 (in Chinese with English abstract).
Luo, Z., Wang, G., and Wang, E.: Global subsoil organic carbon turnover times dominantly controlled by soil properties rather than climate, Nat. Commun., 10, 3688, https://doi.org/10.1038/s41467-019-11597-9, 2019.
Ma, W., Yang, Y., He, J., Zeng, H., and Fang, J.: Above-and belowground biomass in relation to environmental factors in temperate grasslands, Inner Mongolia, Sci. China Ser. C, 51, 263–270, 2008.
Ma, W., Fang, J., Yang, Y., and Mohammat, A.: Biomass carbon stocks and their changes in northern China's grasslands during 1982–2006, Sci. China Life Sci., 53, 841–850, 2010a.
Ma, W., Liu, Z., Wang, Z., Wang, W., Liang, C., Tang, Y., He, J.-S., and Fang, J.: Climate change alters interannual variation of grassland aboveground productivity: evidence from a 22-year measurement series in the Inner Mongolian grassland, J. Plant Res., 123, 509–517, https://doi.org/10.1007/s10265-009-0302-0, 2010b.
Mantel, N.: The detection of disease clustering and a generalized regression approach, Cancer research, 27, 209–220, 1967.
National Bureau of Statistics of China: China Statistical Yearbook (various issues 1981–2019), China Statistics Press, Beijing, available at: https://data.stats.gov.cn/easyquery.htm?cn=E0103 (last access: 1 June 2020), 1981–2019 (in Chinese).
National Research Council: Grasslands and Grassland Sciences in Northern China, The National Academies Press, Washington, DC, USA, 1992.
O'Mara, F. P.: The role of grasslands in food security and climate change, Ann. Bot., 110, 1263–1270, 2012.
Park, T., Chen, C., Macias-Fauria, M., Tømmervik, H., Choi, S., Winkler, A., Bhatt, U. S., Walker, D. A., Piao, S., and Brovkin, V.: Changes in timing of seasonal peak photosynthetic activity in northern ecosystems, Global Change Biol., 25, 2382–2395, 2019.
Pastore, M. A., Lee, T. D., Hobbie, S. E., and Reich, P. B.: Strong photosynthetic acclimation and enhanced water-use efficiency in grassland functional groups persist over 21 years of CO2 enrichment, independent of nitrogen supply, Global Change Biol., 25, 3031–3044, https://doi.org/10.1111/gcb.14714, 2019.
Peng, S., Piao, S., Shen, Z., Ciais, P., Sun, Z., Chen, S., Bacour, C., Peylin, P., and Chen, A.: Precipitation amount, seasonality and frequency regulate carbon cycling of a semi-arid grassland ecosystem in Inner Mongolia, China: A modeling analysis, Agr. Forest Meteorol., 178/179, 46–55, https://doi.org/10.1016/j.agrformet.2013.02.002, 2013.
Piao, S., Fang, J., He, J., and Xiao, Y.: Spatial distribution of grassland biomass in China, Acta Phytoecologica Sinica, 28, 491–498, 2004 (in Chinese with English Abstract).
Polley, H. W., Aspinwall, M. J., Collins, H. P., Gibson, A. E., Gill, R. A., Jackson, R. B., Jin, V. L., Khasanova, A. R., Reichmann, L. G., and Fay, P. A.: CO2 enrichment and soil type additively regulate grassland productivity, New Phytol., 222, 183–192, https://doi.org/10.1111/nph.15562, 2019.
Qi, Y., Ge, J., and Huang, J.: Spatial and temporal distribution of MODIS and MISR aerosol optical depth over northern China and comparison with AERONET, Chinese Sci. Bull., 58, 2497–2506, 2013.
R Development Core Team: R: A language and environment for statistical computing. R Foundation for Statistical Computing, Vienna, Austria, 2020.
Reyes-Fox, M., Steltzer, H., Trlica, M. J., McMaster, G. S., Andales, A. A., LeCain, D. R., and Morgan, J. A.: Elevated CO2 further lengthens growing season under warming conditions, Nature, 510, 259–262, https://doi.org/10.1038/nature13207, 2014.
Sanchez, G.: PLS path modeling with R, Trowchez Editions, Berkeley, CL, USA, 2013.
Sattari, S., Bouwman, A., Rodriguez, R. M., Beusen, A., and Van Ittersum, M.: Negative global phosphorus budgets challenge sustainable intensification of grasslands, Nat. Commun., 7, 1–12, 2016.
Scurlock, J. M., Johnson, K., and Olson, R. J.: Estimating net primary productivity from grassland biomass dynamics measurements, Global Change Biol., 8, 736–753, 2002.
Thornton, P. E., Running, S. W., and White, M. A.: Generating surfaces of daily meteorological variables over large regions of complex terrain, J. Hydrol., 190, 214–251, 1997.
Walker, L. R., Wardle, D. A., Bardgett, R. D., and Clarkson, B. D.: The use of chronosequences in studies of ecological succession and soil development, J. Ecol., 98, 725–736, https://doi.org/10.1111/j.1365-2745.2010.01664.x, 2010.
Wang, G.: Inner Mongolian grassland aboveground biomass measurements, figshare, Figshare, https://doi.org/10.6084/m9.figshare.13108430.v1, 2020.
Wang, G., Huang, Y., Wei, Y., Zhang, W., Li, T., and Zhang, Q.: Climate Warming Does Not Always Extend the Plant Growing Season in Inner Mongolian Grasslands: Evidence From a 30-Year In Situ Observations at Eight Experimental Sites, J. Geophys. Res.-Biogeosci., 124, 2364–2378, https://doi.org/10.1029/2019jg005137, 2019.
Wang, H., Liu, H., Cao, G., Ma, Z., Li, Y., Zhang, F., Zhao, X., Zhao, X., Jiang, L., and Sanders, N. J.: Alpine grassland plants grow earlier and faster but biomass remains unchanged over 35 years of climate change, Ecol. Lett., 23, 701–710, https://doi.org/10.1111/ele.13474, 2020.
Wang, S., Zhang, Y., Ju, W., Chen, J. M., Ciais, P., Cescatti, A., Sardans, J., Janssens, I. A., Wu, M., Berry, J. A., Campbell, E., Fernández-Martínez, M., Alkama, R., Sitch, S., Friedlingstein, P., Smith, W. K., Yuan, W., He, W., Lombardozzi, D., Kautz, M., Zhu, D., Lienert, S., Kato, E., Poulter, B., Sanders, T. G. M., Krüger, I., Wang, R., Zeng, N., Tian, H., Vuichard, N., Jain, A. K., Wiltshire, A., Haverd, V., Goll, D. S., and Peñuelas, J.: Recent global decline of CO2 fertilization effects on vegetation photosynthesis, Science, 370, 1295–1300, https://doi.org/10.1126/science.abb7772, 2020.
Wang, W., Huang, J., Zhou, T., Bi, J., Lin, L., Chen, Y., Huang, Z., and Su, J.: Estimation of radiative effect of a heavy dust storm over northwest China using Fu-Liou model and ground measurements, J. Quant. Spectrosc. Ra., 122, 114–126, 2013.
Xu, L., Yu, G., He, N., Wang, Q., Gao, Y., Wen, D., Li, S., Niu, S., and Ge, J.: Carbon storage in China's terrestrial ecosystems: A synthesis, Sci. Rep., 8, 1–13, 2018.
Xu, Z. and Zhou, G.: Effects of water stress and high nocturnal temperature on photosynthesis and nitrogen level of a perennial grass Leymus chinensis, Plant Soil, 269, 131–139, 2005.
Yu, L., Zhang, M., Wang, L., Lu, Y., and Li, J.: Effects of aerosols and water vapour on spatial-temporal variations of the clear-sky surface solar radiation in China, Atmos. Res., 248, 105162, https://doi.org/10.1016/j.atmosres.2020.105162, 2021.
Zhang, J., Ding, J., Zhang, J., Yuan, M., Li, P., Xiao, Z., Peng, C., Chen, H., Wang, M., and Zhu, Q.: Effects of increasing aerosol optical depth on the gross primary productivity in China during 2000–2014, Ecol. Indic., 108, 105761, https://doi.org/10.1016/j.ecolind.2019.105761, 2020.
Zhang, Q., Buyantuev, A., Fang, X., Han, P., Li, A., Li, F. Y., Liang, C., Liu, Q., Ma, Q., Niu, J., Shang, C., Yan, Y., and Zhang, J.: Ecology and sustainability of the Inner Mongolian Grassland: Looking back and moving forward, Landscape Ecol., 35, 2413–2432, https://doi.org/10.1007/s10980-020-01083-9, 2020.
Zhang, X.: Vegetation Map of China and Its Geographic Pattern: Illustration of the Vegetation Map of the People's Republic China (1:10,000,000), Geological Press, Beijing, China, 296–326, 2007.