the Creative Commons Attribution 4.0 License.
the Creative Commons Attribution 4.0 License.
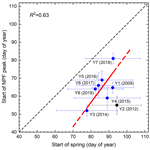
Influence of vegetation on occurrence and time distributions of regional new aerosol particle formation and growth
Wanda Thén
Pasi Aalto
Veli-Matti Kerminen
Anikó Kern
Zoltán Barcza
Tuukka Petäjä
Markku Kulmala
The occurrence frequency of regional atmospheric new aerosol particle formation and consecutive growth events (fNPF) were studied with respect to vegetation activity, aerosol properties, air pollutants and meteorological data in Budapest over the time interval from 2008 to 2018. The data set evaluated contained results of in situ measurements on the land surface that were mostly performed at the Budapest platform for Aerosol Research and Training Laboratory, of satellite-based products recorded by MODIS on Terra and of modelled vegetation emission-related properties from an advanced regional biogeochemical model. The annual mean relative occurrence frequencies were considerable (with an overall mean of 21 %), remained at a constant level (with an overall SD of 5 %) and did not exhibit tendentious change over the years. The shape of the distributions of monthly mean fNPF exhibited large variability from year to year, while the overall average distribution already possessed a characteristic pattern. The structure of the new particle formation (NPF) occurrence distributions was compared to those of environmental variables including concentrations of gas-phase H2SO4, SO2, O3, NO, NO2, CO, PM10 mass and NH3; particle numbers in the size fractions of 6–1000, 6–100 and 100–1000 nm; condensation sink; air temperature (T); relative humidity (RH); wind speed (WS); atmospheric pressure (P); global solar radiation (GRad); gross primary production (GPP) of vegetation; leaf area index (LAI); and stomatal conductance (SCT). There were no evident systematic similarities between fNPF on the one hand and all of the variables studied on the other hand, except for H2SO4 and perhaps NH3. The spring maximum in the NPF occurrence frequency distribution often overlapped with the time intervals of positive T anomaly in vegetated territories. The link between the potential heat stress exerted on plants in sultry summer intervals and the summer fNPF minimum could not be proven. The relevance of environmental variables was assessed by their ratios on NPF event days and on non-event days. The gas-phase H2SO4 concentration showed the largest monthly ratios, followed by O3. The WS, biogenic precursor gases and SO2 can generally favour NPF events, although their influence seemed to be constrained. An association between the fNPF and vegetation growth dynamics was clearly identified.
- Article
(7199 KB) -
Supplement
(432 KB) - BibTeX
- EndNote
New aerosol particle formation (NPF) from atmospheric vapours and consecutive particle diameter growth events (Kulmala et al., 2014) have been observed in all major continental environments in the world (e.g. Kerminen et al., 2018; Nieminen et al., 2018; and references therein). Their relevance for the global aerosol burden, climate system and health risk issues are increasingly recognised (Spracklen et al., 2006; Makkonen et al., 2009, 2012; Merikanto et al., 2009; Yue et al., 2010; Sihto et al., 2011; Kerminen et al., 2012; Carslaw et al., 2013; Braakhuis et al., 2014; Salma et al., 2015; Dunne et al., 2016; Gordon et al., 2016; Ohlwein et al., 2019).
The occurrence of NPF events is one of the fundamental properties of the phenomenon. The main circumstances influencing the regional NPF occurrence involve the atmospheric chemical composition and the concentration and size distribution of aerosol particles, photochemical processes, and meteorological properties (Kulmala et al., 2014). Some precursor compounds and their oxidation products such as SO2 and H2SO4, volatile organic compounds (VOCs) and highly oxygenated molecules (HOMs), NH3 or amines, iodine oxides, and HIO3 were shown to influence NPF events (O'Dowd et al., 2002; Sipilä et al., 2010, 2016; Metzger et al., 2010; Kirkby et al., 2011, 2016; Riipinen et al., 2011; Almeida et al., 2013; Donahue et al., 2013; Schobesberger et al., 2013; Ehn et al., 2014; Riccobono et al., 2014; Jokinen et al., 2015; Bianchi et al., 2016; Tröstl et al., 2016; Yao et al., 2018; Kürten, 2019; He et al., 2021). Further chemical species such as isoprene or NO2 showed inhibiting effects (Kiendler-Scharr et al., 2009; Lehtipalo et al., 2016; Heinritzi et al., 2020; Simon et al., 2020). It was pointed out that NPF can proceed from HOMs alone when it is assisted by air ions (Kirkby et al., 2016). These conclusions were derived mostly from environmental or plant atmosphere chamber experiments. Meteorological parameters such as solar radiation, air temperature (T), water vapour content or relative humidity (RH), wind speed (WS), and boundary mixing layer height can also favour or depress NPF events (Birmili and Wiedensohler, 2000; Hamed et al., 2011; Hirsikko et al., 2012; Jun et al., 2014; Dada et al., 2017). The actual occurrence may also be associated with some limiting or triggering atmospheric processes in the region (Manninen et al., 2010; Dall'Osto et al., 2013). Galactic cosmic rays do not seem to contribute extensively to the overall nucleation under ordinary environmental conditions (e.g. in the presence of atmospheric chemical bases or HOMs) and particularly in the lower troposphere (Kirkby et al., 2011, 2016; Almeida et al., 2013; Riccobono et al., 2014; Dunne et al., 2016). As a consequence of all these factors, NPF events happen with a varying frequency in space and time.
The annual relative occurrence frequency of NPF events is typically between 10 % and 40 % for many geographical regions (Brines et al., 2015; Xiao et al., 2015; Kerminen et al., 2018; Nieminen et al., 2018; Bousiotis et al., 2019; Lee et al., 2019). The frequency changes substantially over a year, as many multifactorial conditions and the complex interplay among the environmental variables influencing it have prominent seasonal variation (Tunved et al., 2006). Many studies have reported a spring or summer maximum (Qian et al., 2007; Wu et al., 2007; Meija and Morawska, 2009; Manninen et al., 2010; Salma et al., 2011; Vakkari et al., 2011; Hirsikko et al., 2012; Nieminen et al., 2014; Dall'Osto et al., 2018). This is, however, not universal, and the order of the seasons can vary among individual territories. Reliable experimental determination of the annual and monthly mean frequencies of regional NPF require long (semi-)continuous measurements, as the occurrence can be influenced by inter-annual differences in chemical, aerosol and meteorological properties and in biogenic cycling.
Studies have also attempted to predict the distributions of the monthly mean occurrence frequency by combining the effects of environmental variables which can be derived from routine atmospheric measurements (e.g. Clement et al., 2001; Boy and Kulmala, 2002; Mikkonen et al., 2006). However, conclusive prognostic or explanative methods could not be achieved (Kerminen et al., 2018). This also means that the driving factors of NPF occurrence and their time variation have remained largely unidentified, poorly understood and unexplained. The lack of this knowledge and experimental information also hinders our understanding of the role of anthropogenic activities in related societal issues of aerosol particles such as their climate and health effects.
A several-year-long, semi-continuous, validated and complex atmospheric data set is available for the Budapest area. It has been studied and evaluated from several aspects. The major objectives of this study are (1) to determine and discuss the annual mean relative occurrence frequency and the distributions of monthly mean frequency of NPF events in Budapest for 7 full measurement years from 2008 to 2018; (2) to investigate and explain the changes in the shape of the distributions and their annual mean with respect to basic meteorological data, criteria air pollutants and other environmental factors, including vegetation-related variables; and (3) to evaluate and interpret the effects of vegetation on NPF occurrence as well as the incidence between them. The involvement of the vegetation-related factors in the ambient NPF and their combination with the environmental influencing properties represent a noteworthy novelty in the research field. The present survey also prepares the full exploitation of the database by advanced multivariate statistical methods.
The following environmental variables were considered in the study: number of NPF event days and non-event days; particle number concentrations in the diameter ranges from 6 to 1000 nm (N), from 6 to 100 nm (N6−100) and from 100 to 1000 nm (N100−1000); concentrations of gas-phase H2SO4, SO2, O3, NO, NOx, NO2, CO, PM10 mass and NH3; condensation sink (CS); T; relative RH; WS; atmospheric pressure (P); global solar radiation (GRad); gross primary production (GPP) of vegetation; leaf area index (LAI); stomatal conductance (SCT); and characteristics of vegetation growth dynamics, such as start of spring (SoS) and green-up duration (GuD). Most variables were determined experimentally, whereas the variables related to vegetation (the last five properties) were derived from an advanced regional biogeochemical model or from satellite-based vegetation products. The data sets actually evaluated in comparisons contained daily median atmospheric concentrations, daily means and standard deviations (SDs) for all variables as well as daily maximum values for GRad (GRadmax). The individual data were averaged over each month, then separately for NPF event days and non-event day in each month, and, finally, over all measurement years in the city centre.
The time intervals investigated comprise 7 full measurement years: (Y1) from 3 November 2008 to 2 November 2009, (Y2) from 19 January 2012 to 18 January 2013, (Y3) from 13 November 2013 to 12 November 2014, (Y4) from 13 November 2014 to 12 November 2015, (Y5) from 13 November 2015 to 12 November 2016, (Y6) from 28 January 2017 to 27 January 2018 and (Y7) from 28 January 2018 to 27 January 2019. In Sect. 3.5, we also included the NPF occurrence data for the last full measurement year completed, i.e. from 28 January 2019 to 27 January 2020 (Y8). Our specific purpose by adding this year was to improve the statistics in studying the effect of vegetation on NPF events. Local time (LT, UTC+1, or daylight saving time, UTC+2) was chosen as the time base of the data because it has been observed that the daily activity time pattern of inhabitants largely influences many atmospheric processes in cities (Salma et al., 2014; Sun et al., 2019; Mikkonen et al., 2020).
2.1 Experimental data and their treatment
The concentrations N, N6−100 and N100−1000 were determined by a flow-switching-type differential mobility particle sizer (DMPS; Salma et al., 2011, 2016b). Its main components include a radioactive (60Ni) bipolar charger, a Nafion semi-permeable membrane dryer, a 28 cm long Vienna-type differential mobility analyser and a condensation particle counter (TSI, model CPC3775). The measurements were performed in a diameter range from 6 to 1000 nm in the dry state of particles. The system was updated twice during the years, in spring 2013 and winter 2016. The major parts of the DMPS system were cleaned and serviced but remained unchanged. Extensive data validation, calibration or comparative exercises were realised in summer 2015 and in autumn 2019, which yielded acceptable results (Salma et al., 2016a; Mikkonen et al., 2020).
The DMPS data were used for identification and classification of the regional NPF events using daily particle number size distribution surface plots (Dal Maso et al., 2005; refined by Kulmala et al., 2012; Németh et al., 2018). The following main classes were separated: event days, non-event days, undefined days and days with missing data (for more than 4 h during the midday). The relative occurrence frequency of NPF events (fNPF) was determined for each month and year as the ratio of the number of event days to the total number of relevant days within the time interval dealt with. In order to evaluate the timing relationships between vegetation growth and NPF events (Sect. 3.5), the start of the NPF occurrence peak in spring (see later Fig. 2) had to be further refined. This was achieved by considering a weekly timescale for determining the occurrence frequency. These data, however, showed larger scatter that was mainly due to the discrete daily character of NPF events. Therefore, the weekly occurrence frequency data sets for winter and spring were subjected to 1 month smoothing to derive less fluctuating time trends. The start of the NPF occurrence spring peak was set at the date (day of year) of the 20 % value of the difference between the maximum smoothed spring peak frequency and the mean winter level of weekly frequencies (on the early side of the peak).
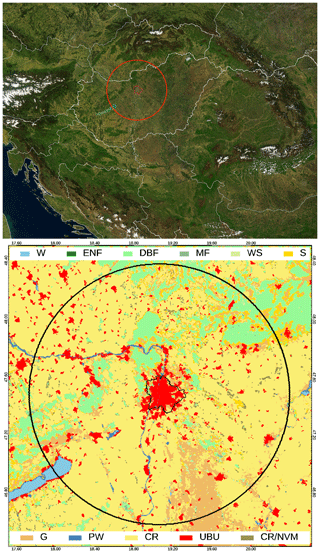
Figure 1Location of Budapest in the Carpathian Basin and the circular geographical area with a radius of 100 km around it, which was considered in modelling calculations (upper panel), and the spatial distribution of land cover types according to the International Geosphere–Biosphere Programme classification (lower panel). Both pictures were derived from satellite-based imagery data recorded by MODIS. W refers to water bodies, ENF refers to evergreen needleleaf forests, DBF refers to deciduous broadleaf forests, MF refers to mixed forests, WS refers to woody savannas, S refers to savannas, G refers to grasslands, PW refers to permanent wetlands, CR refers to croplands, UBU refers to urban and built-up lands, and CR/NVM refers to cropland and natural vegetation mosaics.
The DMPS measurements took place at two urban locations in Budapest, Hungary (Fig. 1). In the measurement year 2012–2013, they were performed in the near-city background, whereas in all other years, they were accomplished in the city centre. The former location is situated at the north-western border of Budapest in a wooded area of the Konkoly Astronomical Observatory (47∘30′00′′ N, 18∘57′47′′ E; 478 , above mean sea level) of the Hungarian Academy of Sciences. This site characterises the air masses which enter the city, as the prevailing wind direction in the area is north-westerly. The measurements in the city centre were conducted at the Budapest platform for Aerosol Research and Training (BpART) Laboratory (47∘28′30′′ N, 19∘3′45′′ E; 115 ) of the Eötvös Loránd University (Salma et al., 2016a). It represents a well-mixed average atmospheric environment for the overall city centre.
The concentrations of SO2; O3; NO, NOx, and NO2; CO; and PM10 mass were measured by UV fluorescence (Ysselbach 43C), UV absorption (Ysselbach 49C), chemiluminescence (Thermo 42C), IR absorption (Thermo 48i) and beta-ray attenuation (Thermo FH62-I-R) methods respectively with a time resolution of 1 h. The data were acquired from the closest measurement stations of the National Air Quality Network in Budapest located 4.5 km from the urban site and 6.9 km from the near-city background site in the prevailing upwind direction (Salma and Németh, 2019).
It was previously shown that the NPF events observed in the Budapest ordinarily happen above a larger territory in the Carpathian Basin (Németh and Salma, 2014) as a spatially coherent regional atmospheric phenomenon (Salma et al., 2016b). Local urban NPF events are superimposed on regional events on several occasions, which result in growth curves with multiple or broad onsets. In these cases, the regional events were included in the evaluations.
The connection between the two sites is also one of the main reasons why the data for the city centre were complemented by the near-city background observations. Furthermore, the differences and similarities between the two locations for fNPF and environmental variables (including the vegetation-related properties) could be relevant and of interest in assessing the importance of various urban environmental types in the process.
Considering that NPF events in the Carpathian Basin ordinarily extend over larger horizontal scales, long-term concentrations of NH3, which are available for the K-puszta measurement station, were also accepted in the study. This station (46∘57′56′′ N, 19∘32′42′′ E; 125 ) is situated on the Great Hungarian Plain at a distance of 68 km from the BpART Laboratory, and it is part of the European monitoring and evaluation of the long-range transmission of air pollutants (EMEP) network. The NH3 concentrations were measured in daily air samples collected using the filter pack method on citric-acid-impregnated cellulose filter by UV–Vis spectrophotometry, according to the EMEP protocol (EMEP Manual, 2002; Horváth et al., 2009).
Most meteorological measurements for the city centre took place on-site at a regular station of the Hungarian Meteorological Service (HMS, station no. 12843) at approximately 70 m from the BpART Laboratory. The T, RH, WS and P data were obtained by standardised meteorological methods (Vaisala HMP45D temperature and humidity probe, Vaisala WAV15A anemometer and Vaisala PTB210 digital barometer respectively, all Finland) with a time resolution of 10 min (except for Y1, when it was 1 h). The WS was measured above the rooftop level. The basic meteorological data for the near-city background were derived using a mobile meteorological station installed at the measurement location at a height of ca. 2 m from the ground with a time resolution of 10 min. Global solar radiation was measured by the HMS (station no. 44527; CMP11 pyranometer, Kipp and Zonen, the Netherlands) situated 10 km to the east with a time resolution of 1 h. Since 2018, the GRad has been also measured on-site by the BpART Laboratory on the rooftop of the building complex using an SMP3 pyranometer (Kipp and Zonen, the Netherlands) with a time resolution of 1 min. The annual mean GRad ratio and SD for 1 h mean values at the BpART Laboratory and HMS station in 2018 were 1.03 ± 0.23 for GRad > 100 W m−2.
The Open Database for Climate Change-Related Impact Studies in Central Europe (FORESEE, v. 3.1; Dobor et al., 2014) was utilised to estimate the daily maximum T data for vegetated territories within the modelled area (Fig. 1) in order to study the effect of T on biogenic emissions. The updated database (http://nimbus.elte.hu/FORESEE/, last access: 14 January 2020) contains observation-based spatially interpolated daily meteorological fields on a regular grid with a spatial resolution of ∘ × ∘ for the interval from 1951 to 2019 using the E-OBS 17e data set (Cornes et al., 2018). From the daily T data, 8 d means were calculated at the pixel level on the finer grid of the MODIS products using elevation as supplementary data (Kern et al., 2016). From these T data, area mean values were calculated for those pixels which represent vegetated territories (Sect. 2.2.2). Finally, the difference of the daily maximum T values from its related multi-annual mean in its corresponding 8 d time interval were determined as the anomaly in maximum air temperature. In order to assist the later comparison of this differential effect with that of other environmental properties, the T anomaly was divided by the SD of the overall mean maximum air temperature (thus, it was expressed in units of SD). The resulting quantity is referred to as the standardised T anomaly. The standardised NPF occurrence frequency anomaly – used in Sect. 3.3 – was calculated in an analogous manner to T.
2.2 Modelled data
The condensation sink for vapour molecules (represented by H2SO4) onto the surface of existing aerosol particles was computed for discrete size distributions (Kulmala et al., 2013; Dal Maso et al., 2002, 2005) using computation scripts developed at the University of Helsinki. Dry particle diameters were considered in the calculations. One of the key components in the NPF process is the gas-phase H2SO4 (Sihto et al., 2006; Sipilä et al., 2010; Erupe et al., 2011; Lehtipalo et al., 2018); its long-term atmospheric measurements are demanding and, therefore, rare. The H2SO4 concentrations were determined utilising a recently improved method of Dada et al. (2020) which also involves the dimer formation. The calculations were based on direct on-site H2SO4 measurements performed by chemical ionisation atmospheric-pressure interface time-of-flight (CI-APi-TOF) mass spectrometry (Jokinen et al., 2012) in March–April 2018. Their results were utilised to derive dedicated fitting parameters for Budapest for radiation intensities > 50 W m−2. The H2SO4 concentrations were calculated in a retrospective manner for the other years or intervals as well. The overall data set was also compared to the proxy values derived as [SO2] × GRad/CS (Petäjä et al., 2009), which were used in our earlier studies for many years. There was a reasonable agreement in the relative changes of the two different data sets, for example the overall Pearson's coefficient of correlation was R = 0.85. A rigorous evaluation has been finished and is to be presented in a future article.
2.2.1 Vegetation properties related to emissions
Terrestrial ecosystems emit large amounts of biogenic VOCs (BVOCs) into the atmosphere (Guenther et al., 2012). Of these BVOCs, monoterpenes have been identified as major aerosol precursors, and their low-volatility oxidation products have been shown to play a role in NPF events (Kulmala et al., 2013; Ehn et al., 2014). Their relative importance, for instance, in the growth process increases with particle size (Riipinen et al., 2011). The requested vapour supersaturations are maintained by fast gas-phase auto-oxidation of VOCs (Crounse et al., 2013). Atmospheric concentrations of the monoterpenes can be determined online by proton transfer reaction mass spectrometry (Taipale et al., 2008), and their oxidation products can be measured by CI-APi-TOF mass spectrometry (Jokinen et al., 2015). These data are, however, usually available for shorter time intervals only. As a consequence, concentrations of both the monoterpenes and their oxidation products are estimated by proxies (Kontkanen et al., 2016). The models take into account temperature-controlled emissions from vegetation, dilution caused by mixing within the planetary boundary layer and different oxidation processes (Kontkanen et al., 2016; Lehtipalo et al., 2018). The models are available and advised for forest ecosystems.
In the present study, we derived three compound vegetation properties – GPP, LAI and SCT – to evaluate the impact of BVOC sources on NPF event occurrence in urban environments. These three parameters can indirectly also be associated with vegetation emissions and finally with BVOC concentrations (Hidy et al., 2016). They were computed by the Biome-BGCMuSo biogeochemical model (v6; Thornton and Rosenbloom, 2005; Hidy et al., 2016). This is a widely used, process-based model with a multilayer soil module that simulates the storage and flux of H2O, C and N between terrestrial managed agroecosystems and the atmosphere. The system is driven by daily meteorological data, ecophysiological properties, soil parameters and some optional input data such as CO2 concentration and some site-specific management information to simulate the biogeochemical processes of a biome. It also accounts for fertilisation, harvest and crop rotation. The system utilised here was parameterised specifically to the Carpathian Basin, and its proper functioning and outputs were validated by agriculturally related data products and eddy-covariance measurements (Barcza et al., 2010; Hidy et al., 2016).
Primary production of the vegetation on land depends on many factors, principally on local hydrology, soil fertility, plant species composition, photosynthetically active radiation and disturbance. It is often thought to be proportional to general biogenic activity which involves BVOC emissions as well. In Biome-BGCMuSo, the GPP was calculated using Farquhar's photosynthesis routine (Farquhar et al., 1980). The LAI is a measure of the total area of leaves per unit ground area and is directly related to the amount of light that is intercepted by plants. Virtually, it is considered as a driving parameter for biosphere–atmosphere exchange of CO2 and water vapour (Bonan, 2015). The SCT is a measure of the transport rate of H2O vapour exiting through the stomata of leaves, and it also controls parallel assimilation of CO2. It is a function of the density, aperture and size of stomata, and it is also related to the boundary layer resistance of the leaf and the concentration gradient of H2O vapour between the leaf and the atmosphere. Light is the primary stimulus engaged in this process; the second key factor is the photosynthesis, and plants themselves can also regulate their transpiration rate via their SCT. Other (organic) gases from plants are also emitted through stomata; therefore, the SCT can also be related to the fluxes of BVOCs from vegetation to the atmosphere. The three vegetation-related variables were derived in model calculations for a circular geographical area around Budapest with a radius of 100 km (Fig. 1). Biome-BGCMuSo was run with generic maize, winter wheat, grassland and broadleaf forest parameterisation representing the main plant functional types (PFTs) in the region. The model results were aggregated according to the share of PFTs within the given pixel area.
2.2.2 Vegetation growth dynamics
Two phenological indices which are related to vegetation growth dynamics in springtime were estimated. They are the SoS, which is the onset of vegetation growth after the winter dormancy, and the GuD, which expresses the time period of initial leaf development after SoS. Their determination was accomplished by utilising the satellite-based “normalized difference vegetation index” (NDVI) data sets. The NDVI is a widely used remote-sensing-based measure of the terrestrial vegetation greenness and state, defined as
where RNIR and RRed are the surface reflectances acquired in the near-infrared (NIR, λ = 700–1100 nm) and red regions of the spectra respectively (Rouse et al., 1974). The pigment in live green leaves (chlorophyll) strongly absorbs light in the photosynthetically active range of 400–700 nm (for use in photosynthesis), whereas its cell structure strongly reflects or reemits radiation in the NIR region (and green light) compared with other wavelengths. The NDVI is, therefore, directly related to the photosynthetic capacity of plant canopies. These values were actually derived from the latest version (C006) of the official MOD09A1 atmospherically corrected surface reflectance product (Vermote, 2015). This was generated from the measurements of the MODerate resolution Imaging Spectroradiometer (MODIS) operating on board the satellite EOS AM1, Terra (Justice et al., 1998). The data were downloaded for the h19v04 sinusoidal tile with a spatial resolution of 500 m and a temporal resolution of 8 d (LP DAAC, 2019) in hierarchical data format for the interval from 2009 to 2019.
The land cover data sets for a circular area with a radius of 100 km around Budapest were derived from the official MCD12Q1 land cover product (Sulla-Menashe and Friedl, 2018) with a spatial resolution of 500 m for the years from 2001 to 2018 according to the International Geosphere–Biosphere Programme (IGBP) classification scheme (Fig. 1). In the modelling, the following vegetation types were studied: (1) croplands (58 % of all, 117 817 pixels); (2) grasslands (13 %); (3) deciduous broadleaf, mixed and evergreen needleleaf forests (12 %; of them, 98 % deciduous trees); and (4) all vegetation, i.e. all territory types except for urban and built-up lands (6 %), water bodies (0.9 %) and permanent wetlands (0.6 %).
A daily-resolution data set was calculated after quality filtering and preprocessing, and the characteristics of the spring development were then assessed by the methodology of Kern et al. (2020). From a remote sensing point of view, the green-up dynamics is characterised by a sharp, mostly linear rise in the NDVI curve that represents leaf flushing (Seyednasrollah et al., 2018) and which can be characterised by the date of leaf unfolding (as the SoS). The GuD is the time difference between the date of the end of greening (EoG) and of the SoS. To achieve this, the NDVI span was calculated as the difference between the maximum and the minimum NDVI during spring green-up. The SoS and the EoG were set at the date (day of year) when the NDVI reached the lower and upper 20 % of the NDVI span respectively (e.g. Shen et al., 2015). The applied cut-off method is commonly used to determine the start of the season of a biome. Both the SoS and GuD data were determined at the pixel level for each year. Their SDs were calculated for a given land cover type as characteristics of the spatial variability of the derived metrics. Taking into account that pixels of relatively coarse (500 m × 500 m) spatial resolution can be diverse in vegetation type and species with variable phenological properties and that the processed remote sensing data are affected by noise due mainly to atmospheric, illumination or observation conditions, the derived metrics are subject to relatively large variability (cf. Fig. 7). The vegetation growth for all years and the methodological procedure are summarised in Fig. S1 in the Supplement. The data sets were processed using the Interactive Data Language (v. 8.6, Harris Geospatial Solutions, USA).
Annual averages of the environmental variables over most measurement years have already been summarised in accompanying publications (Salma and Németh, 2019; Mikkonen et al., 2020); therefore, only a brief overview of the new quantities studied is given in Table 1. The data are in line with or comparable to the values ordinarily obtained for the area (Barcza et al., 2010; Salma et al., 2016b).
During the 7 measurement years, the total number of NPF events was 514. The annual means of the relative NPF event occurrence frequency are considerable. The overall 6-year mean and SD for the city centre were (21 ± 5) %. The fNPF in 2015–2016 was unusually low (its value was ca. 13 % – 35 % lower relative to the overall mean), but there is no plausible explanation for this extreme in the present annual data set. The overall mean frequency and its SD imply that the annual fNPF did not change substantially and, more importantly, in a tendentious manner over the decennial interval studied. It is added as background information that there was no significant decreasing trend in major precursors nor in the interacting gaseous chemical species such as SO2 or NO2 in the area over the time interval of interest (Mikkonen et al., 2020, see also Figs. S2 and S6 respectively). Furthermore, the overall annual median formation rate of particles with a diameter of 6 nm was 4.6 , and the median growth rate for 10 nm particles was 7.3 nm h−1 over the years studied; they were without larger fluctuations and both showed a summer maximum (Salma and Németh, 2019).
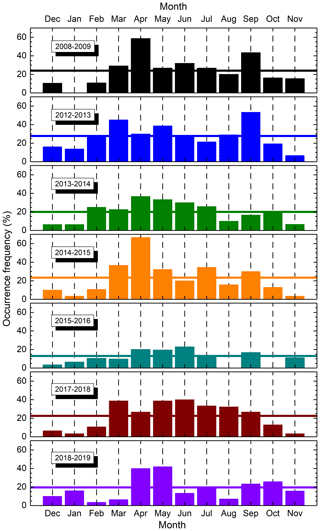
Figure 2Distributions of the monthly mean relative occurrence frequency of NPF event days for the 7 measurement years. The horizontal lines indicate annual means. The value for January 2009 is zero, whereas the values for August and October 2016 are not available. The measurements in 2012–2013 were performed in the near-city background, whereas in the other years, they were accomplished in the city centre.
3.1 Distributions of NPF event occurrence
Distributions of the monthly mean occurrence frequency of event days for each measurement year are shown in Fig. 2. There are obvious similarities and differences among the distributions. The largest diversity was realised in the measurement year 2015–2016 (that also exhibited the smallest annual mean fNPF). Its shape was flattened and featureless. All of the other distributions were much closer to each other in many respects.
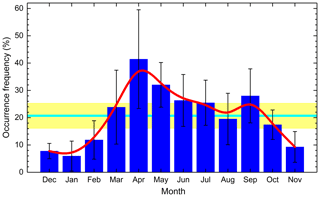
Figure 3Mean distribution of the monthly mean relative occurrence frequency of NPF event days for the joint 6-year-long data set in the city centre. The error bars show ± 1 SD, the horizontal line in cyan indicates the overall mean and the yellow band represents its ± 1 SD. The smooth curve in red serves to guide the eye.
The shapes of the individual distributions also intimated some resemblant components that were repeated over the years. They became more obvious when the overall mean distribution was derived by averaging for all years in the city centre (Fig. 3). The overall distribution exhibits an evident structure which consists of an absolute and a local maximum in April with a monthly mean occurrence frequency of (41 ± 18) % and in September with a mean of (28 ± 10) % respectively and an absolute and a local minimum in January with a mean of (5.9 ± 5.5) % and in August with a mean of (19.5 ± 9.4) % respectively. The relatively large uncertainty intervals of the monthly mean frequencies were caused by inter-annual variability (Fig. 2), and they are also influenced by the absolute number of NPF event days in separate months, which is substantially smaller in winter months than in the other months.
It seems that the overall mean distribution does not show any further extensive change if another new year (for example Y8) is added into the data set. This is also the reason why Fig. 3 and a related plot which was presented earlier (Fig. 1 in Salma and Németh, 2019) are similar. This all implies that the shape of the overall distribution can already be considered to be representative. Moreover, it appears to be characteristic and remarkable. Furthermore, it closely agrees with a multi-year general shape even for some very diverse and detached environments such as boreal forest (Nieminen et al., 2014). Therefore, it seems to be sensible to study the factors that lead to this general structure. We chose to display the up-to-date overall distribution here to foster its comparison to that of environmental variables within the present article.
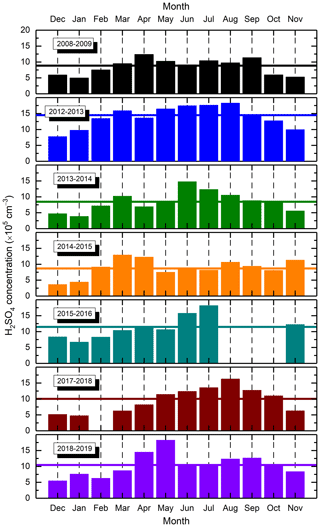
Figure 4Distribution of the monthly median gas-phase H2SO4 concentration for the 7 measurement years. The values for August–October 2016 and February 2018 are not available. The horizontal lines indicate annual medians. The measurements in 2012–2013 were performed in the near-city background, whereas in the other years, they were accomplished in the city centre.
3.2 Distributions of environmental variables
Distributions of the monthly mean values for environmental variables for the 7 measurement years were derived. The plots for the H2SO4 concentration are shown in Fig. 4 as an example. The distributions for some other selected variables, such as SO2, GRadmax, CS, O3, NO2, NH3, RH, WS and T, are presented in Figs. S2–S10 respectively. The monthly averages that are missing from these figures were not available.
By comparing Figs. 2 and 4, we can conclude that there are similar overall tendencies in their shape for several years. The concentration of H2SO4 also tended to have a maximum in spring and another one in August or September. Its seasonal variation could jointly be affected mainly by [SO2], CS and GRad (Petäjä et al., 2009). The concentration of SO2 showed a maximum in winter (Fig. S2), GRadmax had a broad and obvious maximum in summer (Fig. S3), and CS tended to exhibit minimum values in summer (Fig. S4). It seems that in the first approximation, the fNPF distribution is linked to the temporal cycling of the H2SO4 concentration. However, it does not explain the temporal variability alone, and other environmental variables have to play important roles in NPF occurrence.
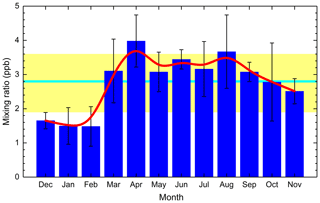
Figure 5Mean distribution of the monthly mean NH3 mixing ratio for the joint 6-year-long data set in the city centre. The error bars show ± 1 SD, the horizontal line in cyan indicates the overall mean and the yellow band represents its ± 1 SD. The smooth curve in red serves to guide the eye.
For most of the other environmental properties, their connections with the occurrence frequency were even weaker or featureless (than for [H2SO4] and fNPF pair) with some similar tendencies reached in sporadic years. The only exception seemed to be the NH3 concentration (Fig. S7). Its overall mean distribution was derived by averaging the data for the corresponding years, and it is shown in Fig. 5. The shape of the resulting distribution resembles the form and structure of the overall fNPF distribution (cf. Fig. 3). It also contains an absolute maximum in April and an absolute minimum in January–February as well as perhaps a local maximum in August. However, the situation is complicated by the dissociation equilibrium in the NH4NO3 (solid or liquid), NH3 (gas) and HNO3 (gas) thermodynamic system, which is rather sensitive to T, RH and particle size, or solution concentration and pH (Mozurkewich, 1993; Nenes et al., 2020). Ambient gas-phase [NH3] is regulated and modified by this equilibrium as well. The similarities in the shapes and the coincidence in the extremes for the two variables may suggest that the availability of NH3 gas – as a base chemical compound in the atmosphere – can enhance the nucleation of H2SO4 molecules in the ambient air and, thus, NPF event occurrence. This is in line with results from chamber experiments (Kirkby et al., 2011), while the involvement of NH3 in NPF under relatively warm ambient conditions (close to the land surface in the atmosphere) has not been completely clarified yet (Kürten, 2019). It also raises a question as to whether other relevant atmospheric chemical bases such as amines have, in general or in synergy with NH3, a similar role in the area.
Pearson's coefficients of correlation between fNPF and the other variables in the joint monthly mean data set were typically |R|<0.50, except for RH, GRadmax, NO, O3 and NH3, which were −0.72, 0.70, −0.61, 0.58 and 0.53 respectively. It is added that the variables influence the occurrence jointly; therefore, the pairwise correlation may not be fully satisfactory for revealing their relationships. These particular results (and the mean event-day-to-non-event-day ratios in Sect. 3.4) prepare further comprehensive multi-statistical analyses.
Another possible explanation of the characteristic structure could be related to the idea that NPF events often occur at elevated heights (as they are favoured at lower Ts), and the nucleated particles are mixed down toward the land surface by general effects of turbulent mixing and air temperature which can have annual cycling. Dedicated measurements on this issue have been in progress to clarify this proposal (Carnerero et al., 2018).
3.3 Temperature anomaly
Possible impacts of T on NPF occurrence exerted indirectly through vegetation was further investigated using anomalies. The anomaly emphasises the deviation of an environmental property (for a given time interval, here for a month or week) from its multi-year trend. The standardised anomaly is expressed in units of SD of the whole data set considered. The anomalies in maximum T and in the NPF occurrence frequency were determined as described in Sect. 2.1, with SDs of 3.1 ∘C and 13 % respectively. Their time distributions (Fig. 6) resembled fluctuations, as expected.
First, the possible impacts of the standardised anomaly in maximum T above vegetated territories on the extreme values of monthly NPF occurrence frequency was examined. This can be achieved by comparing the column plots in Fig. 2 with the T anomaly lines in Fig. 6 for each year. (Their joint graph is shown in Fig. S11.) In many cases (e.g. in spring 2008, 2012, 2017 and 2018), the absolute (spring) maximum of the occurrence frequency overlapped with or followed a substantial positive T anomaly. The exceptions were the years 2014–2015 (Y4) and 2015–2016 (Y5). This suggests that NPF events are generally favoured or are possibly linked to larger Ts in spring. However, the impact of T is part of more comprehensive environmental interactions. No similar observation could be made with respect to the absolute minimum fNPF. This implies that the lowest NPF occurrence in winter is most likely not restricted by T.
The effect of the potential heat stress exerted on plants in sultry summer intervals has become a relevant issue in the Carpathian Basin because of climate change. During these extremely warm intervals, plants could emit less VOCs, as their stomata are more closed to reduce the rate of transpiration (Sect. 2.2.1). However, the coincidence between the positive T anomaly and summer minimum fNPF could not be established in the present data set.
As the next step, the variability of the standardised anomaly in maximum T above vegetated territories and in monthly NPF occurrence frequency was investigated (Fig. 6) to assess the sensitivity of NPF to T. Some temporal tendencies between the two anomalies change concurrently, although their variability seems loose or not coherent in some other intervals. This can partially be explained by multifactorial impacts of environmental variables including vegetation-derived quantities. There could also be some delay in the relationship between T and fNPF. This again emphasises the need for multivariate statistical evaluation methods comprising cross-connections among the variables, which is going to be part of an upcoming dedicated study.
In addition, the monthly or 8 d mean values evaluated so far do not necessarily capture the potential relationships among the variables fully, as they may take effect on shorter (e.g. daily) timescales, which could be of no less importance from the NPF point of view. In order to extend our study to shorter time intervals, we continued investigating the daily mean data.
3.4 Event-day-to-non-event-day ratios
The monthly and annual mean ratios of all environmental variables observed on NPF event days and non-event days in the city centre are summarised in Table 2. The relative occurrence frequencies of event days were also given for comparative purposes. The ratios can be influenced again by the number of event days. The uncertainty of the ratios for modelled variables could be as high as 30 % or even larger if the monthly mean data are relatively small (e.g. for GPP, LAI and SCT in winter). Variables with annual ratios of approximately ran > 1.1 can be considered to favour or to be associated with NPF occurrence in general, variables with approximately ran < 0.9 can be considered to disfavour events, and variables with ran between these two limits possibly have low influence on NPF. The specified values serve only as indicative or guide ratios. The criteria were selected so that the variability of the daily mean values around the monthly mean ordinarily remains between them. This was based upon a simple exercise with non-event time intervals. The procedure represents a pragmatic approach, although alternative limits could also be set.
It is the H2SO4 that shows the largest annual mean ratio. The atmospheric concentration of H2SO4 was larger by a factor of ca. 1.5 on event days than on non-event days. The ratio was even larger in winter months (a mean factor of 1.8) over which the other chemical and meteorological conditions for NPF are less favourable than usual. In winter, NPF events happen if H2SO4 is available in relatively large excess concentrations. For all the other months, the mean ratios were also larger than unity. The smallest monthly mean ratio was obtained in July (and possibly in August and September). This all confirms the primary role of H2SO4 in the phenomenon.
Table 2Overall mean relative occurrence frequency of NPF event days (fNPF, in percent) and overall mean event-day-to-non-event-day ratios for the daily median concentration of gas-phase H2SO4 and O3, daily maximum global solar radiation (GRadmax), N6−100, N, wind speed (WS), gross primary production (GPP) of vegetation, stomatal conductance (SCT), SO2, leaf area index (LAI), NO2, atmospheric pressure (P), NO, NH3, PM10 mass, CO, condensation sink (CS), N100−1000, and relative humidity (RH) for each month and for all data in the city centre. The ratios were organised in descending order of their annual mean values (the first data column).
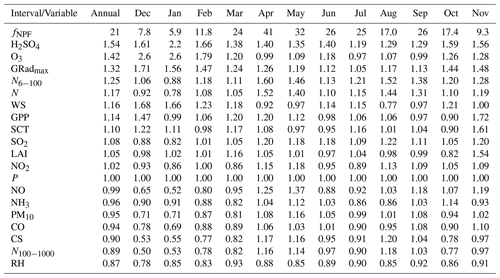
The second largest annual mean ratio was found for O3. Its larger concentrations are often associated with general photochemical activity and secondary organic aerosol (SOA) formation (McFiggans et al., 2019). Its influence was also represented by the ratio of the monthly mean event-day-to-non-event-day ratio to its annual mean ratio. The ratio of ratios for O3 in winter was the largest (1.64) of all cases. This all implies that the photochemical reactivity, involving processes such as the H2SO4 formation in the gas phase and the VOC oxidation, also plays an important role, particularly in those months when the absolute oxidative property is relatively low (Fig. S5 for O3).
The GRadmax exhibited the third largest annual mean ratio, and its monthly mean ratios were also above unity. This property is related to both of the variables discussed above; therefore, the above-mentioned arguments are valid here as well. It has been shown that the presence of clouds decreases the probability of NPF occurrence by attenuating solar radiation intensity below the cloud layer (Baranizadeh et al., 2014; Dada et al., 2017) and that an ongoing event can even be interrupted by the sudden appearance of clouds (Hirsikko et al., 2013; Salma et al., 2016a).
The large annual mean ratios for N and in particular for N6−100 are rather consequences of NPF events than their causes. Large numbers of ultrafine (UF) particles are generated by NPF and growth processes. It is worth noting that the largest ratios of 1.5–1.6 were observed in April, May and August, whereas the smallest ratio, which was below unity (0.88), was realised in January. This can partially be linked to the monthly variation of the particle formation and growth rates in Budapest as well (Salma and Németh, 2019). The interpretations of these ratios are in line with our earlier assessments or findings according to which (1) the concentrations of particles are increased by a factor of 2–3 on event days in central Budapest and (2) the NPF contribution as a single source of UF particles is ca. 13 % as a lower estimate and on a longer run (Salma et al., 2017).
The effect of WS also seems to be noteworthy. On an annual scale, higher WSs can be related to larger event occurrences. The distribution of its monthly mean, however, reveals a more complex relationship. In the months with a large relative occurrence frequency (i.e. in April, May and September), the mean ratios were below unity (0.92 in April), in the winter months, they were extensively above unity (1.52), whereas they were very close to unity in the other months. This behaviour will be explained later in connection with CS and N100−1000.
Precursor BVOC gases – approximated by GPP, LAI and SCT – and SO2 may generally favour NPF occurrence, although their influence could not be quantified and seems to be low. The reason for this could partially be that the oxidation rates of precursors appear to be more important than their atmospheric concentrations (Salma and Németh, 2019) and that the effect of photochemical processes could be delayed in time. The concentrations of BVOCs are expected to be considerable in Budapest in spring. The typical mean contribution of biogenic sources to the total carbon in the PM2.5 size fraction was the second largest with a share of ca. 40 % (Salma et al., 2020). Unfortunately, there is no experimental information available on absolute concentrations or amounts of VOC in the area. The effects of NO2, P and NO seemed to be even more constrained. Concentrations of CO and PM10 mass are often considered as surrogates for urban air quality, and the polluted air seems to suppress NPF occurrence through high CS. Again, the monthly mean event-day-to-non-event-day ratios for NO, PM10 mass and CO were the smallest (typically 0.66, 0.76 and 0.78 respectively) in winter, when the preconditions of events are reached in a more difficult manner.
The mean ratios of CS and N100−1000 were close to each other and mostly below unity. Their lowest values of around 0.53 were reached in December and January. This implies that the NPF events preferably took place in winter on those days when the concentrations of pre-existing particles were relatively small. The whole issue can be explained if considering that the basic preconditions of NPF events are realised by competing sources and sinks for condensing vapours. The source strength in winter is generally low due to lower solar radiation intensities and less biogenic precursor gases in the air. New particle formation events can occur at these low source intensities if the condensation and scavenging sinks – which are related to low particle number concentrations – are even smaller (Lehtinen et al., 2007). This can happen, for instance, due to a stronger wind (Fig. S9) that brings low concentrations of regional and chemically aged particles (N100−1000) into city centres. The reasoning above is in line with and confirms our earlier findings related to diurnal and seasonal variations of UF particles (Salma et al., 2014, 2017).
The smallest annual mean event-day-to-non-event-day ratio was obtained for RH. All monthly mean ratios were also below unity and were similar to each other with an annual mean and SD of 0.87 ± 0.04 (cf. Fig. S8). This unambiguously indicates that RH counteracts NPF occurrence. It can serve as a scavenger for the OH radical (Petäjä et al., 2009). This dependency has already been observed in earlier studies on continental NPF processes (Hamed et al., 2011).
Table 3Start of spring (SoS) with its SD (SDSoS) and the green-up duration (GuD) with its SD (SDGuD) for all vegetation within a 100 km diameter circular area of Budapest for all measurement years. The years in parentheses indicate the calendar year of the spring.
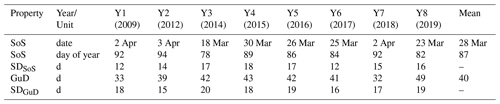
It is noted for completeness that the mean event day minus non-event day T difference in the city centre for various months was 1.2 (December), 0.4 (January), −0.8 (February), 0.4 (March), 1.5 (April), 1.9 (May), 0.1 (June), −0.8 (July), 0.1 (August), −0.5 (September), 1.8 (October) and 1.4 ∘C (November). (The mean event-day-to-non-event-day ratios for T, in Kelvin, were all 1.00.) The monthly mean air temperature data do not indicate obvious relationships with fNPF (cf. Fig. S10). This is contrasting with its effect on NPF dynamic properties, for which the T causes summer maxima (Lee et al., 2019; Salma and Németh, 2019). The latter effect can be facilitated, for instance, through gas-phase auto-oxidation reactions involved in HOMs formation (Frege et al., 2018). The monthly and annual mean ratios of [NH3]/CS, GPP/CS, LAI/CS and SCT/CS on NPF event days and on non-event days in the city centre were also derived considering that NH3 and BVOCs could, in principle, play a driving role in the events. The monthly ratios did not exhibit tendentious variation and did not resemble the distribution of the occurrence frequency (Fig. 3). This and the concentration ratios for NH3 do not explicitly support the indications on its possible outstanding role (Sect. 3.2); therefore, further dedicated systematic studies are required in the area to arrive a conclusive overall interpretation of NH3. The plans should preferably comprise other chemical species as well, such as BVOCs or anthropogenic organics.
It is added that the effect of an environmental variable can also depend on its absolute value. This can exhibit seasonal or other variability in time (Kerminen et al., 2018). The absolute values can also change from site to site; furthermore, there can be different interactions or biases among some variables at different sites. Moreover, even dominant nucleation or growth mechanisms can vary at a fixed location depending on the availability of precursors or of different types of oxidation agents (e.g. OH, O3 or NO3; Bianchi et al., 2016). All of these factors can modify the effect of a variable. Therefore, strictly speaking, the interpretations above are related to the region investigated. These aspects likely explain why the effects of some variables were interpreted inconclusively. For instance, both higher (Birmili and Wiedensohler, 2000; Zhao et al., 2015) and lower (Wu et al., 2007) SO2 concentrations on event days relative to non-event days were reported at diverse locations.
3.5 Vegetation growth
The SoS and the GuD data are summarised in Table 3 for all vegetation. It is seen that spring typically starts in the Budapest area around 28 March and that the green-up of vegetation normally takes 40 d. However, these characteristics were diverse when different vegetation types were considered. The SoS increased monotonically in the order of croplands, grasslands and forests. The spring started 2–3 d earlier for cultivated crops than for all vegetation; the start was almost identical for grass and all vegetation, whereas it was delayed by ca. 9 d for forest with respect to all vegetation. At the same time, the GuD values for grasslands and croplands were identical (42–43 d), whereas the green-up was 32 % faster for forests (27 d) than for all vegetation. This can all likely be explained by phytophysiological properties of the different plants, seeding routine of cultivated crops and increasing intensity of solar radiation (and T) over the course of springtime.
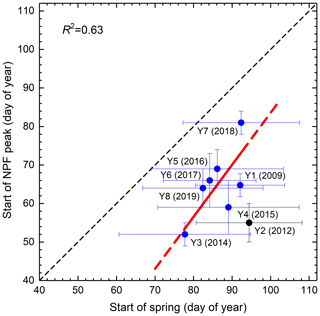
Figure 7Scatter plot of the starting date of spring considering all vegetation and the start of the spring NPF occurrence season (peak). Labels for the measurement years Y1–Y8 and the calendar year of their spring (in parentheses) are also shown. The error bars indicate ± 1 SD. The solid red line represents the linear fit, whereas its dashed parts were obtained by extrapolation. The data point for Y2 (2012), in black, represents a forested environment; therefore, it was excluded from the fitting. The coefficient of determination (R2) for the fit is also given. The line of equality is displayed in black for orientation purposes.
The scatter plot of the SoS date for all vegetation and the start of NPF event occurrence spring peak is shown in Fig. 7. It is recalled that the measurements in 2012–2013 (Y2) were conducted in a forest clearing in the near-city background (Sect. 2.1, description of the measurement sites) and that the growth characteristics are different for various vegetation types, as just concluded in the paragraph above. For this reason, the data point for year Y2 was excluded from further evaluations. We display it in Fig. 7, but it is shown in a different (black) colour from the other points to emphasise this. It is seen that the NPF spring occurrence reacted more sensitively than the visible vegetation spring or green-up in general. This outcome agrees with our long-term sensing perceptions. More importantly, a clear relationship between the NPF and SoS timing could be identified. The Pearson's coefficient of correlation of the data set was R = 0.80. Their link was expressed by a linear fit utilising the weighted least-squares method. The goodness of the fit was quantified by the coefficient of determination, which was R2 = 0.63. The statistical quantities above support that the association between vegetation dynamics and NPF occurrence is significant. We are aware that the two properties are likely biased by other variables, such as GRad; therefore, the interpretation of their causal relationship or direct links is subject to further dedicated investigations.
The relationships of GuD with all vegetation and the total number of NPF events in spring, the maximum monthly occurrence frequency in spring, and the monthly mean occurrence frequency in spring are shown in Fig. S12a–c respectively. The data points imply that the vegetation growth rate does not affect the NPF spring characteristics. This could suggest that a faster vegetation green-up, which is also expected to be connected with generally larger concentrations of BVOCs, does not appear to influence the NPF occurrence.
The annual mean NPF occurrence frequencies in a continental central European area were considerable (with an overall mean of 21 %), remained at a constant level (with an overall SD of 5 %) and did not exhibit tendentious change from 2008 to 2018. The shapes of the distributions of monthly mean occurrence frequency for the years varied substantially. The overall mean distribution, however, possessed a pattern. Its structure was likely caused by the multifactorial influences of environmental properties. The most important components quantified in this ambient study included gas-phase H2SO4, O3, GRad, WS, CS and RH. The factors also involved precursor gases of vapours and their photochemical transformation processes.
A large fraction of chemical compounds contributing to NPF events in cities is expected to originate from anthropogenic precursors. Their emissions may peak any time of year depending on urban activities and human habits. Nevertheless, the fNPF distributions seem to follow a general spring maximum and winter minimum behaviour. This could be associated with a very universal and widespread phenomenon. Emissions from vegetation or the availability of (biogenic) atmospheric chemical bases can be involved. Here, we investigated the role of some vegetation-related factors in combination with influential environmental properties in the ambient NPF process. This approach represents a noteworthy novelty. We showed that there are several important links between the plant phenology in the area and event occurrence in spring as far as both their timing properties and some absolute measures and magnitudes are concerned. However, tight pairwise relationships between fNPF on the one hand and a large variety of environmental variables on the other hand could not be proven. This suggests that the environmental players comprising vegetation exert their impact in a joint manner as a sensitive outcome of interacting components.
The relationships between vegetation and NPF can further be investigated at a molecular level utilising long-term advanced sophisticated online mass spectrometry of organic chemical species of vegetation origin among precursors, nucleating vapours and in molecular clusters. These measurements could also uncover additional links between vegetation and NPF events in summer and winter, which remained open questions in the present work. An understanding of these very complex and internally interacting multicomponent atmospheric chemical mixtures also requires complementing field and laboratory studies with modelling.
The observational data are available from the corresponding author upon reasonable request.
The supplement related to this article is available online at: https://doi.org/10.5194/acp-21-2861-2021-supplement.
IS designed and organised the research study. WT, PA, ZB and IS performed and assisted with most aerosol and meteorological measurements. WT accomplished most of the data treatment and prepared most figures. AK derived and evaluated the products from MODIS and the temperature anomaly data and created the maps in Fig. 1. ZB calculated the Biome-BGCMuSo results. IS, MK, VMK, AK and ZB interpreted the results. IS wrote the paper with input from all co-authors.
The authors declare that they have no conflict of interest.
The authors thank Zoltán Németh and Tamás Weidinger, both of the Eötvös Loránd University, for their assistance. We are grateful to László Horváth and Krisztina Labacz of the Hungarian Meteorological Service for providing the NH3 concentrations.
This research has been supported by the Hungarian Research, Development and Innovation Office (grant nos. K116788, FK128709 and K132254), the European Regional Development Fund and the Hungarian Government (grant no. GINOP-2.3.2-15-2016-00028), the “Advanced research supporting the forestry and wood-processing sector's adaptation to global change” project (grant no. CZ.02.1.01/0.0/0.0/16_019/0000803), the Academy of Finland (grant no. 4100104, Center of Excellence in Atmospheric Sciences) and an ERC Advanced Grant (grant no. 742206).
This paper was edited by Kari Lehtinen and reviewed by two anonymous referees.
Almeida, J., Schobesberger, S., Kurten, A., Ortega, I. K., Kupiainen-Maatta, O., Praplan, A. P., Adamov, A., Amorim, A., Bianchi, F., Breitenlechner, M., David, A., Dommen, J., Donahue, N. M., Downard, A., Dunne, E., Duplissy, J., Ehrhart, S., Flagan, R. C., Franchin, A., Guida, R., Hakala, J., Hansel, A., Heinritzi, M., Henschel, H., Jokinen, T., Junninen, H., Kajos, M., Kangasluoma, J., Keskinen, H., Kupc, A., Kurten, T., Kvashin, A. N., Laaksonen, A., Lehtipalo, K., Leiminger, M., Leppa, J., Loukonen, V., Makhmutov, V., Mathot, S., McGrath, M. J., Nieminen, T., Olenius, T., Onnela, A., Petäjä, T., Riccobono, F., Riipinen, I., Rissanen, M., Rondo, L., Ruuskanen, T., Santos, F. D., Sarnela, N., Schallhart, S., Schnitzhofer, R., Seinfeld, J. H., Simon, M., Sipilä, M., Stozhkov, Y., Stratmann, F., Tome, A., Tröstl, J., Tsagkogeorgas, G., Vaattovaara, P., Viisanen, Y., Virtanen, A., Vrtala, A., Wagner, P. E., Weingartner, E., Wex, H., Williamson, C., Wimmer, D., Ye, P. L., Yli-Juuti, T., Carslaw, K. S., Kulmala, M., Curtius, J., Baltensperger, U., Worsnop, D. R., Vehkamäki, H., and Kirkby, J.: Molecular understanding of sulphuric acid–amine particle nucleation in the atmosphere, Nature, 502, 359–363, https://doi.org/10.1038/nature12663, 2013.
Baranizadeh, E., Arola, A., Hamed, A., Nieminen, T., Mikkonen, S., Virtanen, A., Kulmala, M., Lehtinen, K., and Laaksonen, A.: The effect of cloudiness on new-particle formation: investigation of radiation levels, Boreal Environ. Res., 19, 343–354, 2014.
Barcza, Z., Bondeau, A., Churkina, G., Ciais, P., Czóbel, S., Gelybó, G., Grosz, B., Haszpra, L., Hidy, D., Horváth, L., Machon, A., Pásztor, L., Somogyi, Z., and Van Oost, K.: Modeling of biosphere-atmosphere exchange of greenhouse gases – Model based biospheric greenhouse gas balance of Hungary, in: Atmospheric Greenhouse Gases: The Hungarian Perspective, edited by: Haszpra, L., Springer, Dordrecht, 295–330, https://doi.org/10.1007/978-90-481-9950-1_13, 2010.
Bianchi, F., Tröstl, J., Junninen, H., Frege, C., Henne, S., Hoyle, C. R., Molteni, U., Herrmann, E., Adamov, A., Bukowiecki, N., Chen, X., Duplissy, J., Gysel, M., Hutterli, M. Kangasluoma, J., Kontkanen, J., Kürten, A. Manninen, H. E., Münch, S., Peräkylä, O., Petäjä, T., Rondo, L., Williamson, C., Weingartner, E. Curtius, J., Worsnop, D. R., Kulmala, M., Dommen, J., and Baltensperger, U.: New particle formation in the free troposphere: A question of chemistry and timing, Science, 352, 1109–1112, https://doi.org/10.1126/science.aad5456, 2016.
Birmili, W. and Wiedensohler, A.: New particle formation in the continental boundary layer: meteorological and gas phase parameter influence, Geophys. Res. Lett., 27, 3325–3328, https://doi.org/10.1029/1999GL011221, 2000.
Bonan, G.: Ecological Climatology: concepts and applications, Cambridge University Press, Cambridge, https://doi.org/10.1017/CBO9781107339200, 2015.
Bousiotis, D., Dall'Osto, M., Beddows, D. C. S., Pope, F. D., and Harrison, R. M.: Analysis of new particle formation (NPF) events at nearby rural, urban background and urban roadside sites, Atmos. Chem. Phys., 19, 5679–5694, https://doi.org/10.5194/acp-19-5679-2019, 2019.
Boy, M. and Kulmala, M.: Nucleation events in the continental boundary layer: Influence of physical and meteorological parameters, Atmos. Chem. Phys., 2, 1–16, https://doi.org/10.5194/acp-2-1-2002, 2002.
Braakhuis, H. M., Park, M. V., Gosens, I., De Jong, W. H., and Cassee, F. R.: Physicochemical characteristics of nanomaterials that affect pulmonary inflammation, Part. Fibre Toxicol., 11, 18, https://doi.org/10.1186/1743-8977-11-18, 2014.
Brines, M., Dall'Osto, M., Beddows, D. C. S., Harrison, R. M., Gómez-Moreno, F., Núñez, L., Artíñano, B., Costabile, F., Gobbi, G. P., Salimi, F., Morawska, L., Sioutas, C., and Querol, X.: Traffic and nucleation events as main sources of ultrafine particles in high-insolation developed world cities, Atmos. Chem. Phys., 15, 5929–5945, https://doi.org/10.5194/acp-15-5929-2015, 2015.
Carnerero, C., Pérez, N., Reche, C., Ealo, M., Titos, G., Lee, H.-K., Eun, H.-R., Park, Y.-H., Dada, L., Paasonen, P., Kerminen, V.-M., Mantilla, E., Escudero, M., Gómez-Moreno, F. J., Alonso-Blanco, E., Coz, E., Saiz-Lopez, A., Temime-Roussel, B., Marchand, N., Beddows, D. C. S., Harrison, R. M., Petäjä, T., Kulmala, M., Ahn, K.-H., Alastuey, A., and Querol, X.: Vertical and horizontal distribution of regional new particle formation events in Madrid, Atmos. Chem. Phys., 18, 16601–16618, https://doi.org/10.5194/acp-18-16601-2018, 2018.
Carslaw, K. S., Lee, L. A., Reddington, C. L., Pringle, K. J., Rap, A., Forster, P. M., Mann, G. W., Spracklen, D. V., Woodhouse, M. T., Regayre, L. A., and Pierce, J. R: Large contribution of natural aerosols to uncertainty in indirect forcing, Nature, 503, 67–71, https://doi.org/10.1038/nature12674, 2013.
Clement, C. F., Pirjola, L., Dal Maso, M., Mäkelä, J., and Kulmala, M.: Analysis of particle formation bursts observed in Finland, J. Aerosol Sci., 32, 217–36, https://doi.org/10.1016/S0021-8502(00)00059-8, 2001.
Cornes, R., Van Der Schrier, G., Van Den Besselaar, E. J. M., and Jones, P. D.: An ensemble version of the E OBS temperature and precipitation datasets, J. Geophys. Res.-Atmos., 123, 9391–9409, https://doi.org/10.1029/2017JD028200, 2018.
Crounse, J. D., Nielsen, L. B., Jørgensen, S., Kjaergaard, H. G., and Wennberg, P. O.: Autoxidation of organic compounds in the atmosphere, J. Phys. Chem. Lett., 4, 3513–3520, https://doi.org/10.1021/jz4019207, 2013.
Dada, L., Paasonen, P., Nieminen, T., Buenrostro Mazon, S., Kontkanen, J., Peräkylä, O., Lehtipalo, K., Hussein, T., Petäjä, T., Kerminen, V.-M., Bäck, J., and Kulmala, M.: Long-term analysis of clear-sky new particle formation events and nonevents in Hyytiälä, Atmos. Chem. Phys., 17, 6227–6241, https://doi.org/10.5194/acp-17-6227-2017, 2017.
Dada, L., Ylivinkka, I., Baalbaki, R., Li, C., Guo, Y., Yan, C., Yao, L., Sarnela, N., Jokinen, T., Daellenbach, K. R., Yin, R., Deng, C., Chu, B., Nieminen, T., Wang, Y., Lin, Z., Thakur, R. C., Kontkanen, J., Stolzenburg, D., Sipilä, M., Hussein, T., Paasonen, P., Bianchi, F., Salma, I., Weidinger, T., Pikridas, M., Sciare, J., Jiang, J., Liu, Y., Petäjä, T., Kerminen, V.-M., and Kulmala, M.: Sources and sinks driving sulfuric acid concentrations in contrasting environments: implications on proxy calculations, Atmos. Chem. Phys., 20, 11747–11766, https://doi.org/10.5194/acp-20-11747-2020, 2020.
Dall'Osto, M., Querol, X., Alastuey, A., O'Dowd, C., Harrison, R. M., Wenger, J., and Gómez-Moreno, F. J.: On the spatial distribution and evolution of ultrafine particles in Barcelona, Atmos. Chem. Phys., 13, 741–759, https://doi.org/10.5194/acp-13-741-2013, 2013.
Dall'Osto, M., Beddows, D. C. S., Asmi, A., Poulain, L., Hao, L., Freney, E., Allan, J. D., Canagaratna, M., Crippa, M., Bianchi, F., De Leeuw, G., Eriksson, A., Swietlicki, E., Hansson, H. C., Henzing, J. S., Granier, C., Zemankova, K., Laj, P., Onasch, T., Prevot, A., Putaud, J. P., Sellegri, K., Vidal, M., Virtanen, A., Simo, R., Worsnop, D., O'Dowd, C., Kulmala, M., and Harrison, R. M.: Novel insights on new particle formation derived from a pan-European observing system, Sci. Rep.-UK, 8, 1482, https://doi.org/10.1038/s41598-017-17343-9, 2018.
Dal Maso, M., Kulmala, M., Lehtinen, K. E. J., Mäkelä, J. M., Aalto, P. P., and O'Dowd, C.: Condensation and coagulation sinks and formation of nucleation mode particles in coastal and boreal forest boundary layers, J. Geophys. Res., 107, 8097, https://doi.org/10.1029/2001jd001053, 2002.
Dal Maso, M., Kulmala, M., Riipinen, I., Wagner, R., Hussein, T., Aalto, P. P., and Lehtinen, K. E. J.: Formation and growth of fresh atmospheric aerosols: eight years of aerosol size distribution data from SMEAR II, Hyytiälä, Finland, Boreal Environ. Res., 10, 323–336, 2005.
Dobor, L., Barcza, Z., Hlásny, T., Havasi, Á., Horváth, F., Ittzés, P., and Bartholy, J.: Bridging the gap between climate models and impact studies: the FORESEE Database, Geosci. Data J., 2, 1–11, https://doi.org/10.1002/gdj3.22, 2014.
Donahue, N. M., Ortega, I. K., Chuang, W., Riipinen, I., Riccobono, F., Schobesberger, S., Dommen, J., Baltensperger, U., Kulmala, M., and Worsnop, D. R.: How do organic vapors contribute to new-particle formation?, Faraday Discuss., 165, 91–104, https://doi.org/10.1039/c3fd00046j, 2013.
Dunne, E., Gordon, H., Kürten, A., Almeida, J., Duplissy, J., Williamson, C., Ortega, I., Pringle, K., Adamov, A., Baltensperger, U., Barmet, P., Benduhn, F., Bianchi, F., Breitenlechner, M., Clarke, A., Curtius, J., Dommen, J., Donahue, N., Ehrhart, S., and Carslaw, K.: Global atmospheric particle formation from CERN CLOUD measurements, Science, 354, 1119–1124, https://doi.org/10.1126/science.aaf2649, 2016.
Ehn, M., Thornton, J. A., Kleist, E., Sipilä, M., Junninen, H., Pullinen, I., Springer, M., Rubach, F., Tillmann, R., Lee, B., Lopez-Hilfiker, F., Andres, S., Acir, I. H., Rissanen, M., Jokinen, T., Schobesberger, S., Kangasluoma, J., Kontkanen, J., Nieminen, T., Kurten, T., Nielsen, L. B., Jorgensen, S., Kjaergaard, H. G., Canagaratna, M., Dal Maso, M., Berndt, T., Petäjä, T., Wahner, A., Kerminen, V. M., Kulmala, M., Worsnop, D. R., Wildt, J., and Mentel, T. F.: A large source of low-volatility secondary organic aerosol, Nature, 506, 476–479, https://doi.org/10.1038/nature13032, 2014.
EMEP: Co-operative Programme for Monitoring and Evaluation of the Long-range Transmission of Air Pollutants in Europe, Manual, Chemical Co-ordination Centre Report 1/2002, Norwegian Institute for Air Research, Kjeller, 2002.
Erupe, M. E., Viggiano, A. A., and Lee, S.-H.: The effect of trimethylamine on atmospheric nucleation involving H2SO4, Atmos. Chem. Phys., 11, 4767–4775, https://doi.org/10.5194/acp-11-4767-2011, 2011.
Farquhar, G. D., von Caemmerer, S., and Berry, J. A.: A bio-chemical model of photosynthetic CO2 assimilation in leaves of C3 species, Planta, 149, 78–90, https://doi.org/10.1007/BF00386231, 1980.
Frege, C., Ortega, I. K., Rissanen, M. P., Praplan, A. P., Steiner, G., Heinritzi, M., Ahonen, L., Amorim, A., Bernhammer, A.-K., Bianchi, F., Brilke, S., Breitenlechner, M., Dada, L., Dias, A., Duplissy, J., Ehrhart, S., El-Haddad, I., Fischer, L., Fuchs, C., Garmash, O., Gonin, M., Hansel, A., Hoyle, C. R., Jokinen, T., Junninen, H., Kirkby, J., Kürten, A., Lehtipalo, K., Leiminger, M., Mauldin, R. L., Molteni, U., Nichman, L., Petäjä, T., Sarnela, N., Schobesberger, S., Simon, M., Sipilä, M., Stolzenburg, D., Tomé, A., Vogel, A. L., Wagner, A. C., Wagner, R., Xiao, M., Yan, C., Ye, P., Curtius, J., Donahue, N. M., Flagan, R. C., Kulmala, M., Worsnop, D. R., Winkler, P. M., Dommen, J., and Baltensperger, U.: Influence of temperature on the molecular composition of ions and charged clusters during pure biogenic nucleation, Atmos. Chem. Phys., 18, 65–79, https://doi.org/10.5194/acp-18-65-2018, 2018.
Gordon, H., Sengupta, K., Rap, A., Duplissy, J., Frege, C., Williamson, C., Heinritzi, M., Simon, M., Yan, C., Almeida, J., Tröstl, J., Nieminen, T., Ortega, I. K., Wagner, R., Dunne, E. M., Adamov, A., Amorim, A., Bernhammer, A. K., Bianchi, F., Breitenlechner, M., Brilke, S., Chen, X., Craven, J. S., Dias, A., Ehrhart, S., Fischer, L., Flagan, R. C., Franchin, A., Fuchs, C., Guida, R., Hakala, J., Hoyle, C. R., Jokinen, T., Junninen, H., Kangasluoma, J., Kim, J., Kirkby, J., Krapf, M., Kürten, A., Laaksonen, A., Lehtipalo, K., Makhmutov, V., Mathot, S., Molteni, U., Monks, S. A., Onnela, A., Peräkylä, O., Piel, F., Petäjä, T., Praplan, A. P., Pringle, K. J., Richards, N. A. D., Rissanen, M. P., Rondo, L., Sarnela, N., Schobesberger, S., Scott, C. E., Seinfeld, J. H., Sharma, S., Sipilä, M., Steiner, G., Stozhkov, Y., Stratmann, F., Tomé, A., Virtanen, A., Vogel, A. L., Wagner, A. C., Wagner, P. E., Weingartner, E., Wimmer, D., Winkler, P. M., Ye, P., Zhang, X., Hansel, A., Dommen, J., Donahue, N. M., Worsnop, D. R., Baltensperger, U., Kulmala, M., Curtius, J., and Carslaw, K. S.: Reduced anthropogenic aerosol radiative forcing caused by biogenic new particle formation, P. Natl. Acad. Sci. USA, 113, 12053–12058, https://doi.org/10.1073/pnas.1602360113, 2016.
Guenther, A. B., Jiang, X., Heald, C. L., Sakulyanontvittaya, T., Duhl, T., Emmons, L. K., and Wang, X.: The Model of Emissions of Gases and Aerosols from Nature version 2.1 (MEGAN2.1): an extended and updated framework for modeling biogenic emissions, Geosci. Model Dev., 5, 1471–1492, https://doi.org/10.5194/gmd-5-1471-2012, 2012.
Hamed, A., Korhonen, H., Sihto, S.-L., Joutsensaari, J., Järvinen, H., Petäjä, T., Arnold, F., Nieminen, T., Kulmala, M., Smith, J. N., Lehtinen, K. E. J., and Laaksonen, A.: The role of relative humidity in continental new particle formation. J. Geophys. Res., 116, D03202, https://doi.org/10.1029/2010JD014186, 2011.
He, X.-C., Tham, Y. J., Dada, L., Wang, M., Finkenzeller, H., Stolzenburg, D., Iyer, S., Simon, M., Shen, J., Rörup, B., Rissanen, M., Schobesberger, S., Baalbaki, R., Wang, D. S., Koenig, T. K., Jokinen, T., Sarnela, N., Beck, L., Almeida, J., Kürten, A., Amanatidis, S., Amorim, A., Ataei, F., Baccarini, A., Bertozzi, B., Bianchi, F., Brilke, S., Caudillo, L., Chen, D., Chiu, R., Chu, B., Dias, A., Ding, A., Dommen, J., Duplissy, J., El Haddad, I., Carracedo, L. G., Granzin, M., Hansel, A., Heinritzi, M., Hofbauer, V., Junninen, H., Kangasluoma, J., Kemppainen, D., Kim, C., Kong, W., Krechmer, J. E., Kvashnin, A., Laitinen, T., Lamkaddam, H., Lee, C. P., Lehtipalo, K., Leiminger, M., Li, Z., Makhmutov, V., Manninen, H. E., Marie, G., Marten, R., Mauldin, R. L., Mentler, B., Möhler, O., Müller, T., Nie, W., Onnela, A., Petäjä, T., Pfeifer, J., Philippov, M., Ranjithkumar, A., Saiz-López, A., Salma, I., Scholz, W., Schuchmann, S., Schulze, B., Steiner, G., Stozhkov, Y., Tauber, C., Tomé, A., Thakur, R. C., Väisänen, O., Vazquez-Pufleau, M., Wagner, A. C., Wang, Y., Weber, S. K., Winkler, P. M., Wu, Y., Xiao, M., Yan, C., Ye, Q., Ylisirniö, A., Zauner-Wieczorek, M., Zha, Q., Zhou, P., Flagan, R. C., Curtius, J., Baltensperger, U., Kulmala, M., Kerminen, V.-M., Kurtén, T., Donahue, N. M., Volkamer, R., Kirkby, J., Worsnop, D. R., and Sipilä, M.: Role of iodine oxoacids in atmospheric aerosol nucleation, Science, 371, 589–595, https://doi.org/10.1126/science.abe0298, 2021.
Heinritzi, M., Dada, L., Simon, M., Stolzenburg, D., Wagner, A. C., Fischer, L., Ahonen, L. R., Amanatidis, S., Baalbaki, R., Baccarini, A., Bauer, P. S., Baumgartner, B., Bianchi, F., Brilke, S., Chen, D., Chiu, R., Dias, A., Dommen, J., Duplissy, J., Finkenzeller, H., Frege, C., Fuchs, C., Garmash, O., Gordon, H., Granzin, M., El Haddad, I., He, X., Helm, J., Hofbauer, V., Hoyle, C. R., Kangasluoma, J., Keber, T., Kim, C., Kürten, A., Lamkaddam, H., Laurila, T. M., Lampilahti, J., Lee, C. P., Lehtipalo, K., Leiminger, M., Mai, H., Makhmutov, V., Manninen, H. E., Marten, R., Mathot, S., Mauldin, R. L., Mentler, B., Molteni, U., Müller, T., Nie, W., Nieminen, T., Onnela, A., Partoll, E., Passananti, M., Petäjä, T., Pfeifer, J., Pospisilova, V., Quéléver, L. L. J., Rissanen, M. P., Rose, C., Schobesberger, S., Scholz, W., Scholze, K., Sipilä, M., Steiner, G., Stozhkov, Y., Tauber, C., Tham, Y. J., Vazquez-Pufleau, M., Virtanen, A., Vogel, A. L., Volkamer, R., Wagner, R., Wang, M., Weitz, L., Wimmer, D., Xiao, M., Yan, C., Ye, P., Zha, Q., Zhou, X., Amorim, A., Baltensperger, U., Hansel, A., Kulmala, M., Tomé, A., Winkler, P. M., Worsnop, D. R., Donahue, N. M., Kirkby, J., and Curtius, J.: Molecular understanding of the suppression of new-particle formation by isoprene, Atmos. Chem. Phys., 20, 11809–11821, https://doi.org/10.5194/acp-20-11809-2020, 2020.
Hidy, D., Barcza, Z., Marjanović, H., Ostrogović Sever, M. Z., Dobor, L., Gelybó, G., Fodor, N., Pintér, K., Churkina, G., Running, S., Thornton, P., Bellocchi, G., Haszpra, L., Horváth, F., Suyker, A., and Nagy, Z.: Terrestrial ecosystem process model Biome-BGCMuSo v4.0: summary of improvements and new modeling possibilities, Geosci. Model Dev., 9, 4405–4437, https://doi.org/10.5194/gmd-9-4405-2016, 2016.
Hirsikko, A., Vakkari, V., Tiitta, P., Manninen, H. E., Gagné, S., Laakso, H., Kulmala, M., Mirme, A., Mirme, S., Mabaso, D., Beukes, J. P., and Laakso, L.: Characterisation of sub-micron particle number concentrations and formation events in the western Bushveld Igneous Complex, South Africa, Atmos. Chem. Phys., 12, 3951–3967, https://doi.org/10.5194/acp-12-3951-2012, 2012.
Hirsikko, A., Vakkari, V., Tiitta, P., Hatakka, J., Kerminen, V.-M., Sundström, A.-M., Beukes, J. P., Manninen, H. E., Kulmala, M., and Laakso, L.: Multiple daytime nucleation events in semi-clean savannah and industrial environments in South Africa: analysis based on observations, Atmos. Chem. Phys., 13, 5523–5532, https://doi.org/10.5194/acp-13-5523-2013, 2013.
Horváth, L., Fagerli, H., and Sutton, M. A.: Long-term record (1981–2005) of ammonia and ammonium concentrations at K-puszta, Hungary and the effect of sulphur dioxide emission change on measured and modelled concentrations, in: Atmospheric ammonia, edited by: Sutton, M. A., Reis, S., and Baker, S. M., Springer, Dordrecht, https://doi.org/10.1007/978-1-4020-9121-6_12, 2009.
Jokinen, T., Sipilä, M., Junninen, H., Ehn, M., Lönn, G., Hakala, J., Petäjä, T., Mauldin III, R. L., Kulmala, M., and Worsnop, D. R.: Atmospheric sulphuric acid and neutral cluster measurements using CI-APi-TOF, Atmos. Chem. Phys., 12, 4117–4125, https://doi.org/10.5194/acp-12-4117-2012, 2012.
Jokinen, T., Berndt, T., Makkonen, R., Kerminen, V.-M., Junninen, H., Paasonen, P., Stratmann, F., Herrmann, H., Guenther, A. B., Worsnop, D. R., Kulmala, M., Ehn, M., and Sipilä, M.: Production of extremely low volatile organic compounds from biogenic emissions: Measured yields and atmospheric implications, P. Natl. Acad. Sci. USA, 112, 7123–7128, https://doi.org/10.1073/pnas.1423977112, 2015.
Jun, Y.-S., Jeong, C.-H., Sabaliauskas, K., Leaitch, W. R., and Evans, G. J.: A year-long comparison of particle formation events at paired urban and rural locations, Atmos. Pollut. Res., 5, 447–454, https://doi.org/10.5094/APR.2014.052, 2014.
Justice, C. O., Vermote, E., Townshend, J. R. G., Defries, R., Roy, D. P., Hall, D. K., Salomonson, V. V., Privette, J. L., Riggs, G., Strahler, A., Lucht, W., Myneni, R., Knjazihhin, Y., Running, S., Nemani, R., Wan, Z., Huete, A., van Leeuwen, W., Wolfe, R., Giglio, L., Muller, J.-P., Lewis, P., and Barnsley, M.: The Moderate Resolution Imaging Spectroradiometer (MODIS): land remote sensing for global change research, IEEE T. Geosci. Remote, 36, 1228–1249, https://doi.org/10.1109/36.701075, 1998.
Kerminen, V.-M., Paramonov, M., Anttila, T., Riipinen, I., Fountoukis, C., Korhonen, H., Asmi, E., Laakso, L., Lihavainen, H., Swietlicki, E., Svenningsson, B., Asmi, A., Pandis, S. N., Kulmala, M., and Petäjä, T.: Cloud condensation nuclei production associated with atmospheric nucleation: a synthesis based on existing literature and new results, Atmos. Chem. Phys., 12, 12037–12059, https://doi.org/10.5194/acp-12-12037-2012, 2012.
Kerminen, V.-M., Chen, X., Vakkari, V., Petäjä, T., Kulmala, M., and Bianchi, F.: Atmospheric new particle formation and growth: review of field observations, Environ. Res. Lett., 13, 103003, https://doi.org/10.1088/1748-9326/aadf3c, 2018.
Kern, A., Marjanović, H., and Barcza, Z.: Evaluation of the quality of NDVI3g dataset against Collection 6 MODIS NDVI in Central-Europe between 2000 and 2013, Remote Sens.-Basel, 8, 955, https://doi.org/10.3390/rs8110955, 2016.
Kern, A., Marjanović, H., and Barcza, Z.: Spring vegetation green-up dynamics in Central Europe based on 20-year long MODIS NDVI data, Agr. Forest Meteorol., 287, 107969, https://doi.org/10.1016/j.agrformet.2020.107969, 2020.
Kiendler-Scharr, A., Wildt, J., Dal Maso, M., Hohaus, T., Kleist, E., Mentel, T. F., Tillmann, R., Uerlings, R., Schurr, U., and Wahner, A.: New particle formation in forests inhibited by isoprene emissions, Nature, 461, 381–384, https://doi.org/10.1038/nature08292, 2009.
Kirkby, J., Curtius, J., Almeida, J., Dunne, E., Duplissy, J., Ehrhart, S., Franchin, A., Gagné, S., Ickes, L., Kürten, A., Kupc, A., Metzger, A., Riccobono, F., Rondo, L., Schobesberger, S., Tsagkogeorgas, G., Wimmer, D., Amorim, A., Bianchi, F., Breitenlechner, M., David, A., Dommen, J., Downard, A., Ehn, M., Flagan, R. C., Haider, S., Hansel, A., Hauser, D., Jud, W., Junninen, H., Kreissl, F., Kvashin, A., Laaksonen, A., Lehtipalo, K., Lima, J., Lovejoy, E. R., Makhutov, V., Mathot, S., Mikkilä, J., Minginette, P., Mogo, S., Nieminen, T., Onnela, A., Pereira, A., Petäjä, T., Schnitzhofer, R., Seinfeld, J. H., Sipilä, M., Stozhkov, Y., Stratmann, F., Tome, A., Vanhanen, J., Viisanen Y., Vrtala, A., Wagner, P. E., Walther, H., Weingartner, E., Wex, H., Winkler, P. M., Carslaw, K. S., Worsnop, D. R., Baltensperger, U., and Kulmala, M.: Role of sulfuric acid, ammonia and galactic cosmic rays in atmospheric aerosol nucleation, Nature, 476, 429–433, https://doi.org/10.1038/nature10343, 2011.
Kirkby, J., Duplissy, J., Sengupta, K., Frege, C., Gordon, H., Williamson, C., Heinritzi, M., Simon, M., Yan, C., Almeida, J., Tröstl, J., Nieminen, T., Ortega, I. K., Wagner, R., Adamov, A., Amorim, A., Bernhammer, A.-K., Bianchi, F., Breitenlechner, M., Brilke, S., Chen, X., Craven, J., Dias, A., Ehrhart, S., Flagan, R. C., Franchin, A., Fuchs, C., Guida, R., Hakala, J., Hoyle, C. R., Jokinen, T., Junninen, H., Kangasluoma, J., Kim, J., Krapf, M., Kürten, A., Laaksonen, A., Lehtipalo, K., Makhmutov, V., Mathot, S., Molteni, U., Onnela, A., Peräkylä, O., Piel, F., Petäjä, T., Praplan, A. P., Pringle, K., Rap, A., Richards, N. A. D., Riipinen, I., Rissanen, M. P., Rondo, L., Sarnela, N., Schobesberger, S., Scott, C. E., Seinfeld, J. H., Sipilä, M., Steiner, G., Stozhkov, Y., Stratmann, F., Tomé, A., Virtanen, A., Vogel, A. L., Wagner, A., Wagner, P. E., Weingartner, E., Wimmer, D., Winkler, P. M., Ye, P., Zhang, X., Hansel, A., Dommen, J., Donahue, N. M., Worsnop, D. R., Baltensperger, U., Kulmala, M., Carslaw, K. S., and Curtius, J.: Ion-induced nucleation of pure biogenic particles, Nature, 533, 521–526, https://doi.org/10.1038/nature17953, 2016.
Kontkanen, J., Paasonen, P., Aalto, J., Bäck, J., Rantala, P., Petäjä, T., and Kulmala, M.: Simple proxies for estimating the concentrations of monoterpenes and their oxidation products at a boreal forest site, Atmos. Chem. Phys., 16, 13291–13307, https://doi.org/10.5194/acp-16-13291-2016, 2016.
Kulmala, M., Petäjä, T., Nieminen, T., Sipilä, M., Manninen, H. E., Lehtipalo, K., Dal Maso, M., Aalto, P. P., Junninen, H., Paasonen, P., Riipinen, I., Lehtinen, K. E. J., Laaksonen, A., and Kerminen, V.-M.: Measurement of the nucleation of atmospheric aerosol particles, Nat. Protoc., 7, 1651–1667, https://doi.org/10.1038/nprot.2012.091, 2012.
Kulmala, M., Kontkanen, J., Junninen, H., Lehtipalo, K., Manninen, H. E., Nieminen, T., Petäjä, T., Sipilä, M., Schobesberger, S., Rantala, P., Franchin, A., Jokinen, T., Järvinen, E., Äijälä, M., Kangasluoma, J., Hakala, J., Aalto, P. P., Paasonen, P., Mikkilä, J., Vanhanen, J., Aalto, J., Hakola, H., Makkonen, U., Ruuskanen, T., Mauldin III, R. L., Duplissy, J., Vehkamäki, H., Bäck, J., Kortelainen, A., Riipinen, I., Kurtén, T., Johnston, M. V., Smith, J. N., Ehn, M., Mentel, T. F., Lehtinen, K. E. J., Laaksonen, A., Kerminen, V.-M., and Worsnop, D. R.: Direct observations of atmospheric aerosol nucleation, Science, 339, 943–946, https://doi.org/10.1126/science.1227385, 2013.
Kulmala, M., Petäjä, T., Ehn, M., Thornton, J., Sipilä, M., Worsnop, D. R., and Kerminen, V.-M.: Chemistry of atmospheric nucleation: On the recent advances on precursor characterization and atmospheric cluster composition in connection with atmospheric new particle formation, Annu. Rev. Phys. Chem., 65, 21–37, https://doi.org/10.1146/annurev-physchem-040412-110014, 2014.
Kürten, A.: New particle formation from sulfuric acid and ammonia: nucleation and growth model based on thermodynamics derived from CLOUD measurements for a wide range of conditions, Atmos. Chem. Phys., 19, 5033–5050, https://doi.org/10.5194/acp-19-5033-2019, 2019.
Lee, S.-H., Gordon, H., Yu, H., Lehtipalo, K., Haley, R., Li, Y., and Zhang, R.: New particle formation in the atmosphere: from molecular clusters to global climate, J. Geophys. Res.-Atmos., 124, 7098–7146, https://doi.org/10.1029/2018JD029356, 2019.
Lehtinen, K. E. J., Dal Maso, M., Kulmala, M., and Kerminen, V.-M.:. Estimating nucleation rates from apparent particle formation rates and vice versa: Revised formulation of the Kerminen–Kulmala equation, J. Aerosol Sci., 38, 988–994, https://doi.org/10.1016/j.jaerosci.2007.06.009, 2007.
Lehtipalo, K., Rondo, L., Kontkanen, J., Schobesberger, S., Jokinen, T., Sarnela, N., Kürten, A., Ehrhart, S., Franchin, A., Nieminen, T., Riccobono, F., Sipilä, M., Yli-Juuti, T., Duplissy, J., Adamov, A., Ahlm, L., Almeida, J., Amorim, A., Bianchi, F., Breitenlechner, M., Dommen, J., Downard, A. J., Dunne, E. M., Flagan, R. C., Guida, R., Hakala, J., Hansel, A., Jud, W., Kangasluoma, J., Kerminen, V.-M., Keskinen, H., Kim, J., Kirkby, J., Kupc, A., Kupiainen-Määttä, O., Laaksonen, A., Lawler, M. J., Leiminger, M., Mathot, S., Olenius, T., Ortega, I. K., Onnela, A., Petäjä, T., Praplan, A., Rissanen, M. P., Ruuskanen, T., Santos, F. D., Schallhart, S., Schnitzhofer, R., Simon, M., Smith, J. N., Tröstl, J., Tsagkogeorgas, G., Tomé, A., Vaattovaara, P., Vehkamäki, H., Vrtala, A. E., Wagner, P. E., Williamson, C., Wimmer, D., Winkler, P. M., Virtanen, A., Donahue, N. M., Carslaw, K. S., Baltensperger, U., Riipinen, I., Curtius, J., Worsnop, D. R., and Kulmala, M.: The effect of acid–base clustering and ions on the growth of atmospheric nano-particles, Nat. Commun., 7, 11594, https://doi.org/10.1038/ncomms11594, 2016.
Lehtipalo, K., Yan, C., Dada, L., Bianchi, F., Xiao, M., Wagner, R., Stolzenburg, D., Ahonen, L. R., Amorim, A., Baccarini, A., Bauer, P. S., Baumgartner, B., Bergen, A., Bernhammer, A.-K., Breitenlechner, M., Brilke, S., Buchholz, A., Mazon, S. B., Chen, D., Chen, X., Dias, A., Dommen, J., Draper, D. C., Duplissy, J., Ehn, M., Finkenzeller, H., Fischer, L., Frege, C., Fuchs, C., Garmash, O., Gordon, H., Hakala, J., He, X., Heikkinen, L., Heinritzi, M., Helm, J. C., Hofbauer, V., Hoyle, C. R., Jokinen, T., Kangasluoma, J., Kerminen, V.-M., Kim, C., Kirkby, J., Kontkanen, J., Kürten, A., Lawler, M. J., Mai, H., Mathot, S., Mauldin, R. L., Molteni, U., Nichman, L., Nie, W., Nieminen, T., Ojdanic, A., Onnela, A., Passananti, M., Petäjä, T., Piel, F., Pospisilova, V., Quéléver, L. L. J., Rissanen, M. P., Rose, C., Sarnela, N., Schallhart, S., Schuchmann, S., Sengupta, K., Simon, M., Sipilä, M., Tauber, C., Tomé, A., Tröstl, J., Väisänen, O., Vogel, A. L., Volkamer, R., Wagner, A. C., Wang, M., Weitz, L., Wimmer, D., Ye, P., Ylisirniö, A., Zha, Q., Carslaw, K. S., Curtius, J., Donahue, N. M., Flagan, R. C., Hansel, A., Riipinen, I., Virtanen, A., Winkler, P. M., Baltensperger, U., Kulmala, M., and Worsnop, D. R.: Multicomponent new particle formation from sulfuric acid, ammonia, and biogenic vapors, Sci. Adv., 4, eaau5363, https://doi.org/10.1126/sciadv.aau5363, 2018.
LP DAAC – Land Processes Distributed Active Archive Center: MOD09A1, Collection 6, NASA EOSDIS Land Processes DAAC, USGS Earth Resources Observation and Science (EROS) Center, Sioux Falls, South Dakota, available at: https://lpdaac.usgs.gov (last access: 14 January 2020), 2019.
Makkonen, R., Asmi, A., Korhonen, H., Kokkola, H., Järvenoja, S., Räisänen, P., Lehtinen, K. E. J., Laaksonen, A., Kerminen, V.-M., Järvinen, H., Lohmann, U., Bennartz, R., Feichter, J., and Kulmala, M.: Sensitivity of aerosol concentrations and cloud properties to nucleation and secondary organic distribution in ECHAM5-HAM global circulation model, Atmos. Chem. Phys., 9, 1747–1766, https://doi.org/10.5194/acp-9-1747-2009, 2009.
Makkonen, R., Asmi, A., Kerminen, V.-M., Boy, M., Arneth, A., Hari, P., and Kulmala, M.: Air pollution control and decreasing new particle formation lead to strong climate warming, Atmos. Chem. Phys., 12, 1515–1524, https://doi.org/10.5194/acp-12-1515-2012, 2012.
Manninen, H. E., Nieminen, T., Asmi, E., Gagné, S., Häkkinen, S., Lehtipalo, K., Aalto, P., Vana, M., Mirme, A., Mirme, S., Hőrrak, U., Plass-Dülmer, C., Stange, G., Kiss, G., Hoffer, A., Törő, N., Moerman, M., Henzing, B., de Leeuw, G., Brinkenberg, M., Kouvarakis, G. N., Bougiatioti, A., Mihalopoulos, N., O'Dowd, C., Ceburnis, D., Arneth, A., Svenningsson, B., Swietlicki, E., Tarozzi, L., Decesari, S., Facchini, M. C., Birmili, W., Sonntag, A., Wiedensohler, A., Boulon, J., Sellegri, K., Laj, P., Gysel, M., Bukowiecki, N., Weingartner, E., Wehrle, G., Laaksonen, A., Hamed, A., Joutsensaari, J., Petäjä, T., Kerminen, V.-M., and Kulmala, M.: EUCAARI ion spectrometer measurements at 12 European sites – analysis of new particle formation events, Atmos. Chem. Phys., 10, 7907–7927, https://doi.org/10.5194/acp-10-7907-2010, 2010.
McFiggans, G., Mentel, T. F., Wildt, J., Pullinen, I., Kang, S., Kleist, E., Schmitt, S., Springer, M., Tillmann, R., Wu, C., Zhao, D., Hallquist, M., Faxon, C., Le Breton, M., Hallquist, A. M., Simpson, D., Bergstroem, R., Jenkin, M. E., Ehn, M., Thornton, J. A., Alfarra, M. R., Bannan, T. J., Percival, C. J., Priestley, M., Topping, D., and Kiendler-Scharr, A.: Secondary organic aerosol reduced by mixture of atmospheric vapours, Nature, 565, 587–593, https://doi.org/10.1038/s41586-018-0871-y, 2019.
Mejía, J. F. and Morawska, L.: An investigation of nucleation events in a coastal urban environment in the Southern Hemisphere, Atmos. Chem. Phys., 9, 7877–7888, https://doi.org/10.5194/acp-9-7877-2009, 2009.
Merikanto, J., Spracklen, D. V., Mann, G. W., Pickering, S. J., and Carslaw, K. S.: Impact of nucleation on global CCN, Atmos. Chem. Phys., 9, 8601–8616, https://doi.org/10.5194/acp-9-8601-2009, 2009.
Metzger, A., Verheggen, B., Dommen, J., Duplissy, J., Prévôt, A. S. H., Weingartner, E., Riipinen, I., Kulmala, M., Spracklen, D. V., Carslaw, K. S., and Baltensperger, U.: Evidence for the role of organics in aerosol particle formation under atmospheric conditions, P. Natl. Acad. Sci. USA, 107, 6646–6651, https://doi.org/10.1073/pnas.0911330107, 2010.
Mikkonen, S., Lehtinen, K. E. J., Hamed, A., Joutsensaari, J., Facchini, M. C., and Laaksonen, A.: Using discriminant analysis as a nucleation event classification method, Atmos. Chem. Phys., 6, 5549–5557, https://doi.org/10.5194/acp-6-5549-2006, 2006.
Mikkonen, S., Németh, Z., Varga, V., Weidinger, T., Leinonen, V., Yli-Juuti, T., and Salma, I.: Decennial time trends and diurnal patterns of particle number concentrations in a central European city between 2008 and 2018, Atmos. Chem. Phys., 20, 12247–12263, https://doi.org/10.5194/acp-20-12247-2020, 2020.
Mozurkewich, M.: The dissociation constant of ammonium nitrate and its dependence on temperature, relative humidity and particle size, Atmos. Environ. A, 27, 261–270, https://doi.org/10.1016/0960-1686(93)90356-4, 1993.
Németh, Z. and Salma, I.: Spatial extension of nucleating air masses in the Carpathian Basin, Atmos. Chem. Phys., 14, 8841–8848, https://doi.org/10.5194/acp-14-8841-2014, 2014.
Németh, Z., Rosati, B., Zíková, N., Salma, I., Bozó, L., Dameto de España, C., Schwarz, J., Ždímal, V., and Wonaschütz, A.: Comparison of atmospheric new particle formation and growth events in three Central European cities, Atmos. Environ., 178, 191–197, https://doi.org/10.1016/j.atmosenv.2018.01.035, 2018.
Nenes, A., Pandis, S. N., Weber, R. J., and Russell, A.: Aerosol pH and liquid water content determine when particulate matter is sensitive to ammonia and nitrate availability, Atmos. Chem. Phys., 20, 3249–3258, https://doi.org/10.5194/acp-20-3249-2020, 2020.
Nieminen, T., Asmi, A., Dal Maso, M., P. Aalto, P., Keronen, P., Petäjä, T., Kulmala, M., and Kerminen, V.-M.: Trends in atmospheric new-particle formation: 16 years of observations in a boreal-forest environment, Boreal Environ. Res., 19, 191–214, 2014.
Nieminen, T., Kerminen, V.-M., Petäjä, T., Aalto, P. P., Arshinov, M., Asmi, E., Baltensperger, U., Beddows, D. C. S., Beukes, J. P., Collins, D., Ding, A., Harrison, R. M., Henzing, B., Hooda, R., Hu, M., Hőrrak, U., Kivekäs, N., Komsaare, K., Krejci, R., Kristensson, A., Laakso, L., Laaksonen, A., Leaitch, W. R., Lihavainen, H., Mihalopoulos, N., Németh, Z., Nie, W., O'Dowd, C., Salma, I., Sellegri, K., Svenningsson, B., Swietlicki, E., Tunved, P., Ulevicius, V., Vakkari, V., Vana, M., Wiedensohler, A., Wu, Z., Virtanen, A., and Kulmala, M.: Global analysis of continental boundary layer new particle formation based on long-term measurements, Atmos. Chem. Phys., 18, 14737–14756, https://doi.org/10.5194/acp-18-14737-2018, 2018.
O'Dowd, C. D., Jimenez, J. L., Bahreini, R. Flagan, R. C., Seinfeld, J. H., Hämeri, K., Pirjola, L., Kulmala, M., Jennings, S. G., and Hoffmann, T.: Marine aerosol formation from biogenic iodine emissions, Nature, 417, 632–636, https://doi.org/10.1038/nature00775, 2002.
Ohlwein, S., Kappeler, R., Joss, M. K., Künzli, N., and Hoffmann, B.: Health effects of ultrafine particles: a systematic literature review update of epidemiological evidence, Int. J. Publ. Health, 685, 547–559, https://doi.org/10.1007/s00038-019-01202-7, 2019.
Petäjä, T., Mauldin III, R. L., Kosciuch, E., McGrath, J., Nieminen, T., Paasonen, P., Boy, M., Adamov, A., Kotiaho, T., and Kulmala, M.: Sulfuric acid and OH concentrations in a boreal forest site, Atmos. Chem. Phys., 9, 7435–7448, https://doi.org/10.5194/acp-9-7435-2009, 2009.
Qian, S., Sakurai, H., and McMurry, P. H.: Characteristics of regional nucleation events in urban East St. Louis, Atmos. Environ. 41 4119–4127, https://doi.org/10.1016/j.atmosenv.2007.01.011, 2007.
Riccobono, F., Schobesberger, S., Scott, C., Dommen, J., Ortega, I., Rondo, L., Almeida, J., Amorim, A., Bianchi, F., Breitenlechner, M., David, A., Downard, A., Dunne, E., Duplissy, J., Ehrhart, S., Flagan, R., Franchin, A., Hansel, A., Junninen, H., Kajos, M., Keskinen, H., Kupc, A., Kurten, A., Kvashin, A., Laaksonen, A., Lehtipalo, K., Makhmutov, V., Mathot, S., Nieminen, T., Onnela, A., Petäjä, T., Praplan, A., Santos, F., Schallhart, S., Seinfeld, J., Sipilä, M., Spracklen, D., Stozhkov, Y., Stratmann, F., Tome, A., Tsagkogeorgas, G., Vaattovaara, P., Viisanen, Y., Vrtala, A., Wagner, P., Weingartner, E., Wex, H., Wimmer, D., Carslaw, K., Curtius, J., Donahue, N., Kirkby, J., Kulmala, M., Worsnop, D., and Baltensperger, U.: Oxidation products of biogenic emissions contribute to nucleation of atmospheric particles, Science, 344, 717–721, https://doi.org/10.1126/science.1243527, 2014.
Riipinen, I., Pierce, J. R., Yli-Juuti, T., Nieminen, T., Häkkinen, S., Ehn, M., Junninen, H., Lehtipalo, K., Petäjä, T., Slowik, J., Chang, R., Shantz, N. C., Abbatt, J., Leaitch, W. R., Kerminen, V.-M., Worsnop, D. R., Pandis, S. N., Donahue, N. M., and Kulmala, M.: Organic condensation: a vital link connecting aerosol formation to cloud condensation nuclei (CCN) concentrations, Atmos. Chem. Phys., 11, 3865–3878, https://doi.org/10.5194/acp-11-3865-2011, 2011.
Rouse, J. W., Haas, R. H., Deering, D. W., Schell, J. A., and Harlan, J. C.: Monitoring the vernal advancement and retrogradation (green wave effect) of natural vegetation, NASA/GSFC Type III Final Report, NASA, Greenbelt, MD, 371 pp., 1974.
Salma, I. and Németh, Z.: Dynamic and timing properties of new aerosol particle formation and consecutive growth events, Atmos. Chem. Phys., 19, 5835–5852, https://doi.org/10.5194/acp-19-5835-2019, 2019.
Salma, I., Borsós, T., Weidinger, T., Aalto, P., Hussein, T., Dal Maso, M., and Kulmala, M.: Production, growth and properties of ultrafine atmospheric aerosol particles in an urban environment, Atmos. Chem. Phys., 11, 1339–1353, https://doi.org/10.5194/acp-11-1339-2011, 2011.
Salma, I., Borsós, T., Németh, Z., Weidinger, T., Aalto, T., and Kulmala, M.: Comparative study of ultrafine atmospheric aerosol within a city, Atmos. Environ., 92, 154–161, https://doi.org/10.1016/j.atmosenv.2014.04.020, 2014.
Salma, I., Füri, P., Németh, Z., Farkas, Á., Balásházy, I., Hofmann, W., and Farkas, Á.: Lung burden and deposition distribution of inhaled atmospheric urban ultrafine particles as the first step in their health risk assessment, Atmos. Environ., 104, 39–49, https://doi.org/10.1016/j.atmosenv.2014.12.060, 2015.
Salma, I., Németh, Z., Weidinger, T., Kovács, B., and Kristóf, G.: Measurement, growth types and shrinkage of newly formed aerosol particles at an urban research platform, Atmos. Chem. Phys., 16, 7837–7851, https://doi.org/10.5194/acp-16-7837-2016, 2016a.
Salma, I., Németh, Z., Kerminen, V.-M., Aalto, P., Nieminen, T., Weidinger, T., Molnár, Á., Imre, K., and Kulmala, M.: Regional effect on urban atmospheric nucleation, Atmos. Chem. Phys., 16, 8715–8728, https://doi.org/10.5194/acp-16-8715-2016, 2016b.
Salma, I., Varga, V., and Németh, Z.: Quantification of an atmospheric nucleation and growth process as a single source of aerosol particles in a city, Atmos. Chem. Phys., 17, 15007–15017, https://doi.org/10.5194/acp-17-15007-2017, 2017.
Salma, I., Vasanits-Zsigrai, A., Machon, A., Varga, T., Major, I., Gergely, V., and Molnár, M.: Fossil fuel combustion, biomass burning and biogenic sources of fine carbonaceous aerosol in the Carpathian Basin, Atmos. Chem. Phys., 20, 4295–4312, https://doi.org/10.5194/acp-20-4295-2020, 2020.
Schobesberger, S., Junninen, H., Bianchi, F., Lonn, G., Ehn, M., Lehtipalo, K., Dommen, J., Ehrhart, S., Ortega, I. K., Franchin, A., Nieminen, T., Riccobono, F., Hutterli, M., Duplissy, J., Almeida, J., Amorim, A., Breitenlechner, M., Downard, A. J., Dunne, E. M., Flagan, R. C., Kajos, M., Keskinen, H., Kirkby, J., Kupc, A., Kurten, A., Kurten, T., Laaksonen, A., Mathot, S., Onnela, A., Praplan, A. P., Rondo, L., Santos, F. D., Schallhart, S., Schnitzhofer, R., Sipilä, M., Tome, A., Tsagkogeorgas, G., Vehkamäki, H., Wimmer, D., Baltensperger, U., Carslaw, K. S., Curtius, J., Hansel, A., Petäjä, T., Kulmala, M., Donahue, N. M., and Worsnop, D. R.: Molecular understanding of atmospheric particle formation from sulfuric acid and large oxidized organic molecules, P. Natl. Acad. Sci. USA, 110, 17223–17228, https://doi.org/10.1073/pnas.1306973110, 2013.
Shen, M., Piao, S., Cong, N., Zhang, G., and Jassens, I. A.: Precipitation impacts on vegetation spring phenology on the Tibetan Plateau, Global Change Biol., 21, 3647–3656, https://doi.org/10.1111/gcb.12961, 2015.
Seyednasrollah, B., Swenson, J. J., Domec, J. C., and Clark, J. S.: Leaf phenology paradox: Why warming matters most where it is already warm, Remote Sens. Environ., 209, 446–455, https://doi.org/10.1016/j.rse.2018.02.059, 2018.
Sihto, S.-L., Kulmala, M., Kerminen, V.-M., Dal Maso, M., Petäjä, T., Riipinen, I., Korhonen, H., Arnold, F., Janson, R., Boy, M., Laaksonen, A., and Lehtinen, K. E. J.: Atmospheric sulphuric acid and aerosol formation: implications from atmospheric measurements for nucleation and early growth mechanisms, Atmos. Chem. Phys., 6, 4079–4091, https://doi.org/10.5194/acp-6-4079-2006, 2006.
Sihto, S.-L., Mikkilä, J., Vanhanen, J., Ehn, M., Liao, L., Lehtipalo, K., Aalto, P. P., Duplissy, J., Petäjä, T., Kerminen, V.-M., Boy, M., and Kulmala, M.: Seasonal variation of CCN concentrations and aerosol activation properties in boreal forest, Atmos. Chem. Phys., 11, 13269–13285, https://doi.org/10.5194/acp-11-13269-2011, 2011.
Simon, M., Dada, L., Heinritzi, M., Scholz, W., Stolzenburg, D., Fischer, L., Wagner, A. C., Kürten, A., Rörup, B., He, X.-C., Almeida, J., Baalbaki, R., Baccarini, A., Bauer, P. S., Beck, L., Bergen, A., Bianchi, F., Bräkling, S., Brilke, S., Caudillo, L., Chen, D., Chu, B., Dias, A., Draper, D. C., Duplissy, J., El-Haddad, I., Finkenzeller, H., Frege, C., Gonzalez-Carracedo, L., Gordon, H., Granzin, M., Hakala, J., Hofbauer, V., Hoyle, C. R., Kim, C., Kong, W., Lamkaddam, H., Lee, C. P., Lehtipalo, K., Leiminger, M., Mai, H., Manninen, H. E., Marie, G., Marten, R., Mentler, B., Molteni, U., Nichman, L., Nie, W., Ojdanic, A., Onnela, A., Partoll, E., Petäjä, T., Pfeifer, J., Philippov, M., Quéléver, L. L. J., Ranjithkumar, A., Rissanen, M. P., Schallhart, S., Schobesberger, S., Schuchmann, S., Shen, J., Sipilä, M., Steiner, G., Stozhkov, Y., Tauber, C., Tham, Y. J., Tomé, A. R., Vazquez-Pufleau, M., Vogel, A. L., Wagner, R., Wang, M., Wang, D. S., Wang, Y., Weber, S. K., Wu, Y., Xiao, M., Yan, C., Ye, P., Ye, Q., Zauner-Wieczorek, M., Zhou, X., Baltensperger, U., Dommen, J., Flagan, R. C., Hansel, A., Kulmala, M., Volkamer, R., Winkler, P. M., Worsnop, D. R., Donahue, N. M., Kirkby, J., and Curtius, J.: Molecular understanding of new-particle formation from α-pinene between −50 and +25 ∘C, Atmos. Chem. Phys., 20, 9183–9207, https://doi.org/10.5194/acp-20-9183-2020, 2020.
Sipilä, M., Berndt, T., Petäjä, T., Brus, D., Vanhanen, J., Stratmann, F., Patokoski, J., Mauldin 3rd, R. L., Hyvärinen, A. P., Lihavainen, H., and Kulmala, M.: The role of sulfuric acid in atmospheric nucleation, Science, 327, 1243–1246, https://doi.org/10.1126/science.1180315, 2010.
Sipilä, M., Sarnela, N., Jokinen, T., Henschel, H., Junninen, H., Kontkanen, J., Richters, S., Kangasluoma, J., Franchin, A., Peräkylä, O., Rissanen, M. P., Ehn, M., Vehkamäki, H., Kurten, T., Berndt, T., Petäjä, T., Worsnop, D., Ceburnis, D., Kerminen, V.-M., Kulmala, M., and O'Dowd, C.: Molecular-scale evidence of aerosol particle formation via sequential addition of HIO3, Nature, 537, 532–534, https://doi.org/10.1038/nature19314, 2016.
Spracklen, D. V., Carslaw, K. S., Kulmala, M., Kerminen, V.-M., Mann, G. W., and Sihto, S.-L.: The contribution of boundary layer nucleation events to total particle concentrations on regional and global scales, Atmos. Chem. Phys., 6, 5631–5648, https://doi.org/10.5194/acp-6-5631-2006, 2006.
Sulla-Menashe, D. and Frield, M. A.: User guide to collection 6 MODIS land cover (MCD12Q1 and MCD12C1) product, available at: https://icdc.cen.uni-hamburg.de/fileadmin/user_upload/icdc_Dokumente/MODIS/mcd12_user_guide_v6.pdf (last access: 14 January 2020), 2018.
Sun, J., Birmili, W., Hermann, M., Tuch, T., Weinhold, K., Spindler, G., Schladitz, A., Bastian, S., Löschau, G., Cyrys, J., Gu, J., Flentje, H., Briel, B., Asbach, C., Kaminski, H., Ries, L., Sohmer, R., Gerwig, H., Wirtz, K., Meinhardt, F., Schwerin, A., Bath, O., Ma, N., and Wiedensohler, A.: Variability of Black Carbon mass concentrations, sub-micrometer particle number concentrations and size distributions: Results of the German Ultrafine Aerosol Network ranging from city street to high Alpine locations, Atmos. Environ., 202, 256–268, https://doi.org/10.1016/j.atmosenv.2018.12.029, 2019.
Taipale, R., Ruuskanen, T. M., Rinne, J., Kajos, M. K., Hakola, H., Pohja, T., and Kulmala, M.: Technical Note: Quantitative long-term measurements of VOC concentrations by PTR-MS – measurement, calibration, and volume mixing ratio calculation methods, Atmos. Chem. Phys., 8, 6681–6698, https://doi.org/10.5194/acp-8-6681-2008, 2008.
Thornton, P. E. and Rosenbloom, N. A.: Ecosystem model spin-up: Estimating steady state conditions in a coupled terrestrial carbon and nitrogen cycle model, Ecol. Model., 189, 25–48, https://doi.org/10.1016/j.ecolmodel.2005.04.008, 2005.
Tröstl, J., Chuang, W. K., Gordon, H., Heinritzi, M., Yan, C., Molteni, U., Ahlm, L., Frege, C., Bianchi, F., Wagner, R., Simon, M., Lehtipalo, K., Williamson, C., Craven, J. S., Duplissy, J., Adamov, A., Almeida, J., Bernhammer, A. K., Breitenlechner, M., Brilke, S., Dias, A., Ehrhart, S., Flagan, R. C., Franchin, A., Fuchs, C., Guida, R., Gysel, M., Hansel, A., Hoyle, C. R., Jokinen, T., Junninen, H., Kangasluoma, J., Keskinen, H., Kim, J., Krapf, M., Kürten, A., Laaksonen, A., Lawler, M., Leiminger, M., Mathot, S., Möhler, O., Nieminen, T., Onnela, A., Petäjä, T., Piel, F. M., Miettinen, P., Rissanen, M. P., Rondo, L., Sarnela, N., Schobesberger, S., Sengupta, K., Sipilä, M., Smith, J. N., Steiner, G., Tomè, A., Virtanen, A., Wagner, A. C., Weingartner, E., Wimmer, D., Winkler, P. M., Ye, P. L., Carslaw, K. S., Curtius, J., Dommen, J., Kirkby, J., Kulmala, M., Riipinen, I., Worsnop, D. R., Donahue, N. M., and Baltensperger, U.: The role of low-volatility organic compounds in initial particle growth in the atmosphere, Nature, 533, 527–531, https://doi.org/10.1038/nature18271, 2016.
Tunved, P., Hansson, H.-C., Kerminen, V.-M., Ström, J., Dal Maso, M., Lihavainen, H., Viisanen, Y., Aalto, P. P., Komppula, M., and Kulmala, M.: High natural aerosol loading over boreal forest, Science, 312, 261–263, https://doi.org/10.1126/science.1123052, 2006.
Vakkari, V., Laakso, H., Kulmala, M., Laaksonen, A., Mabaso, D., Molefe, M., Kgabi, N., and Laakso, L.: New particle formation events in semi-clean South African savannah, Atmos. Chem. Phys., 11, 3333–3346, https://doi.org/10.5194/acp-11-3333-2011, 2011.
Vermote, E.: MOD09A1 MODIS/Terra Surface Reflectance 8-Day L3 Global 500 m SIN Grid V006, NASA EOSDIS Land Processes DAAC, NASA, https://doi.org/10.5067/MODIS/MOD09A1.006, 2015.
Wu, Z., Hu, M., Liu, S., Wehner, B., Bauer, S., Massling, A., Wiedensohler, A., Petäjä, T., Dal Maso, M., and Kulmala, M.: New particle formation in Beijing, China: statistical analysis of a 1 year data set, J. Geophys. Res., 112, D09209, https://doi.org/10.1029/2006JD007406, 2007.
Xiao, S., Wang, M. Y., Yao, L., Kulmala, M., Zhou, B., Yang, X., Chen, J. M., Wang, D. F., Fu, Q. Y., Worsnop, D. R., and Wang, L.: Strong atmospheric new particle formation in winter in urban Shanghai, China, Atmos. Chem. Phys., 15, 1769–1781, https://doi.org/10.5194/acp-15-1769-2015, 2015.
Yao, L., Garmash, O., Bianchi, F., Zheng, J., Yan, C., Kontkanen, J., Junninen, H., Mazon, S. B., Ehn, M., Paasonen, P., Sipilä, M., Wang, M., Wang, X., Xiao, S., Chen, H., Lu, Y., Zhang, B., Wang, D., Fu, Q., Geng, F., Li, L., Wang, H., Qiao, L., Yang, X., Chen, J., Kerminen, V.-M., Petäjä, T., Worsnop, D. R., Kulmala, M., and Wang, L.: Atmospheric new particle formation from sulfuric acid and amines in a Chinese megacity, Science, 361, 278–281, https://doi.org/10.1126/science.aao4839, 2018.
Yue, D. L., Hu, M., Zhang, R. Y., Wang, Z. B., Zheng, J., Wu, Z. J., Wiedensohler, A., He, L. Y., Huang, X. F., and Zhu, T.: The roles of sulfuric acid in new particle formation and growth in the mega-city of Beijing, Atmos. Chem. Phys., 10, 4953–4960, https://doi.org/10.5194/acp-10-4953-2010, 2010.
Zhao, S., Yu, Y., Yin, D., and He, J.: Meteorological dependence of particle number concentrations in an urban area of complex terrain, Atmos. Res., 164–165, 304–305, https://doi.org/10.1016/j.atmosres.2015.06.001, 2015.