the Creative Commons Attribution 4.0 License.
the Creative Commons Attribution 4.0 License.
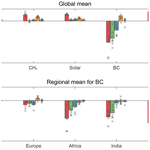
Distinct surface response to black carbon aerosols
Drew Shindell
Yuqiang Zhang
Apostolos Voulgarakis
Jean-Francois Lamarque
Gunnar Myhre
Gregory Faluvegi
Bjørn H. Samset
Timothy Andrews
Dirk Olivié
Toshihiko Takemura
Xuhui Lee
For the radiative impact of individual climate forcings, most previous studies focused on the global mean values at the top of the atmosphere (TOA), and less attention has been paid to surface processes, especially for black carbon (BC) aerosols. In this study, the surface radiative responses to five different forcing agents were analyzed by using idealized model simulations. Our analyses reveal that for greenhouse gases, solar irradiance, and scattering aerosols, the surface temperature changes are mainly dictated by the changes of surface radiative heating, but for BC, surface energy redistribution between different components plays a more crucial role. Globally, when a unit BC forcing is imposed at TOA, the net shortwave radiation at the surface decreases by W m−2 (W m−2)−1 (averaged over global land without Antarctica), which is partially offset by increased downward longwave radiation (2.32±0.38 W m−2 (W m−2)−1 from the warmer atmosphere, causing a net decrease in the incoming downward surface radiation of W m−2 (W m−2)−1. Despite a reduction in the downward radiation energy, the surface air temperature still increases by 0.25±0.08 K because of less efficient energy dissipation, manifested by reduced surface sensible ( W m−2 (W m−2)−1) and latent heat flux ( W m−2 (W m−2)−1), as well as a decrease in Bowen ratio ( (W m−2)−1). Such reductions of turbulent fluxes can be largely explained by enhanced air stability (0.07±0.02 K (W m−2)−1), measured as the difference of the potential temperature between 925 hPa and surface, and reduced surface wind speed ( m s−1 (W m−2)−1). The enhanced stability is due to the faster atmospheric warming relative to the surface, whereas the reduced wind speed can be partially explained by enhanced stability and reduced Equator-to-pole atmospheric temperature gradient. These rapid adjustments under BC forcing occur in the lower atmosphere and propagate downward to influence the surface energy redistribution and thus surface temperature response, which is not observed under greenhouse gases or scattering aerosols. Our study provides new insights into the impact of absorbing aerosols on surface energy balance and surface temperature response.
- Article
(7767 KB) - Full-text XML
-
Supplement
(1428 KB) - BibTeX
- EndNote
Black carbon (BC) aerosols, emitted from diesel engines, biofuels, forest fires, incomplete combustion, and biomass burning, could significantly impact the Earth's climate by changing its radiative balance or by perturbing the hydrological cycle (Ramanathan et al., 2001; Menon et al., 2002). The former is realized via absorbing solar radiation, causing positive effective radiative forcing (ERF) at the top of the atmosphere (TOA) and thus warming the climate (Ramanathan and Carmichael, 2008; Bond et al., 2013; Myhre et al., 2013b), while the latter is partly through modifying the microphysical properties of clouds (e.g., albedo and lifetime) (Koch and Del Genio, 2010; Bond et al., 2013; Boucher et al., 2013), which could further impact ERF. For instance, Menon et al. (2002) attributed the cooling and drying trends in north China in the second half of the 20th century to BC aerosols; Meehl et al. (2008) suggested that BC contributed to the precipitation change in India by altering the meridional temperature gradient.
For radiative impacts, however, most previous studies have only focused on TOA forcing. TOA forcing is useful in understanding the climate feedback, climate sensitivity, and future climate change (Andrews et al., 2012), but it is not necessarily predictive of the spatial pattern of surface temperature response, which is more related to surface radiative changes (Wild et al., 2004). One intriguing phenomenon for BC is that, on the global scale, BC could warm the surface even with reduced solar radiation and net radiation at the surface (Ramanathan and Carmichael, 2008), which is somewhat counterintuitive as higher surface temperature generally requires more incoming radiation at the surface. FAQ 7.2 of Boucher et al. (2013) briefly described the heating process induced by BC. Specifically, BC particles firstly heat the atmosphere and cause surface cooling locally, but then they warm both the surface and the atmosphere due to atmospheric circulation and mixing processes. When it comes to surface response, Ramanathan et al. (2001) suggested that the reduced solar radiation at the surface is possibly counteracted by reduced evaporation, which further perturbs the hydrological cycle. Krishnan and Ramanathan (2002) found that the source regions of haze are subject to cooling due to the absorption of solar radiation, whereas regions outside the source can be warming, thus contributing to overall global warming. Liepert et al. (2004) argued that aerosols and clouds could lead to weakened turbulent flux at the surface. Wilcox et al. (2016) reported a reduction of turbulent flux under BC aerosols at the surface and linked such responses to clouds. Based on model simulations, Myhre et al. (2018) concluded that BC aerosols can change the global hydrological cycle by suppressing sensible heat flux at the surface and attributed this suppression to the changes of air stability.
The published studies cited above provide informative insights into the surface radiative responses to BC aerosols, but our understanding is still incomplete, especially from the perspective of the surface energy balance. In this study, we aim to fill this gap and answer the following scientific questions. (i) How does the surface warming under BC aerosols differ from warming due to greenhouse gases and solar forcing? (ii) What are the specific mechanisms that drive such warming responses? (iii) what are the relative contributions to the surface temperature change from each surface energy budget component?
2.1 Data
This study employs the model output from the Precipitation Driver and Response Model Intercomparison Project (PDRMIP), utilizing simulations examining the climate responses to individual climate drivers (Myhre et al., 2017). The eight models used in this study are CanESM2, GISS-E2R, HadGEM2-ES, HadGEM3, MIROC, CESM-CAM4, CESM-CAM5, and NorESM. The versions of these models are essentially the same as their versions in the 5th Assessment Report of the Intergovernmental Panel on Climate Change (IPCC AR5). The configurations and basic settings are listed in Table 1. In these simulations, five separate perturbations were applied to all the models instantly on a global scale: a doubling of CO2 concentration (CO2×2), a tripling of CH4 concentration (CH4×3), a 2 % increase in solar irradiance (solar + 2 %), a 10-fold increase in present-day black carbon concentration/emission (BC × 10), and a 5-fold increase in present-day SO4 concentration/emission (SO4×5). Each perturbation was run in two parallel configurations, a 15-year fixed sea surface temperature (fsst) simulation and a 100-year coupled simulation. The former is compared with its fsst control simulation to diagnose the ERF at the TOA and fast responses in each model, whereas the latter is used to examine climate responses. One model (CESM-CAM4) used a slab ocean setup for the coupled simulation whereas the others used a full dynamic ocean. For aerosol perturbations, monthly year 2000 concentrations were derived from the AeroCom Phase II initiative (Myhre et al., 2013a) and multiplied by the stated factors in concentration-driven models. Some models were unable to perform simulations with prescribed concentrations. These models multiplied emissions by these factors instead (Table 1). The aerosol loadings in the NorESM model for the two aerosol perturbations are shown in Fig. 1 for an illustrative purpose; the spatial patterns are similar for other models. In the BC experiment, the concentration is highest in east China (E. China), followed by India, tropical Africa and South America (S. America). In the current study, these four regions are referred to as source regions due to their high emissions while the US and Europe are defined as non-source regions due to their relatively low emissions. For the SO4 experiment, the aerosols are mainly restricted to the Northern Hemisphere (NH), with the highest loading observed in E. China, followed by India and Europe. The eastern US also has moderately high concentrations. More detailed descriptions of PDRMIP and some PDRMIP findings are given in Samset et al. (2016), Myhre et al. (2017), and Tang et al. (2018).
2.2 Methods
In this study, we start from the surface energy balance. We restrict our discussions to land grids only because this is where most people live, and thus the temperature response over land is more important to the well-being of humans. The incoming radiative energy (Rin) includes
In Eq. (1), ↓SW represents downward shortwave radiation and ↑SW represents reflected SW radiation. ↓LW denotes the downward LW radiation. The law of energy conservation requires that the Rin should be balanced by the outgoing energy (Eout):
In Eq. (2), ↑LW is the outgoing longwave radiation, which is a function of temperature based on the Stefan–Boltzmann law. H, λE, and G denote sensible heat flux, latent heat flux, and ground heat flux, respectively. For latent heat flux (λE), λ is the specific latent heat of evaporation, and E is the evaporation rate. Rin is defined as surface radiative heating, as it is the radiative input provided to the surface to raise the surface temperature (Wild et al., 2004). The surface responds to the imposed energy by redistributing the energy content through each Eout component. Since Rin is equal to Eout, we have
The changes of each energy component, denoted by Δ, are obtained by subtracting the control simulations from the perturbations using the data of the years 6–15 in each fsst simulation and the years 71–100 in each coupled simulation. The changes are then normalized by the ERF in the corresponding experiments to obtain the changes per unit global forcing. Negative values for the energy component, denoted by a negative sign, represent decreasing trends. The ERF values for each model are obtained from Tang et al. (2019) and are defined as the combination of net SW radiation plus the net LW radiation at the TOA in the fsst simulations (Hansen et al., 2002). The multi-model mean (MMM) ERF values are 3.68±0.09 W m−2 (CO2×2), 1.15±0.09 W m−2 (CH4×3), 4.21±0.05 W m−2 (solar + 2 %), 1.20±0.28 W m−2 (BC ×10), and W m−2 (SO4×5) for indicated experiments (mean ±1 standard error). The MMM changes are estimated by averaging all eight models' results. A two-sided Student t-test is used to examine whether the MMM results are significantly different from zero. The same process was repeated for all variables analyzed in the current study.
3.1 Incoming radiation and surface temperature changes under BC forcing
Figure 2a–c show the MMM changes of Rin and its components for the fsst simulations of the BC experiment. The fsst simulations are analyzed because we mainly focus on the rapid adjustments when the forcing is instantly imposed. Rapid adjustments are generally referred to as the fast responses that affect the components of the climate system and modify the global energy budget indirectly. Unlike feedbacks, rapid adjustments do not operate through changes in the global mean temperature, and most are thought to occur within a few weeks (Boucher et al., 2013). Specifically, when a unit BC forcing is imposed at the TOA, the net surface SW radiation decreases by W m−2 due to the absorption of solar radiation by BC particles, whereas ↓LW radiation shows an increase of 2.32±0.38 W m−2 (Fig. 2a and b), as a result of the warmer atmosphere. When combined, Rin still decreases by W m−2 on the global scale, with some positive changes only in high-latitude regions (Fig. 2c). However, the surface air temperature increases globally by 0.25±0.08 K despite the decreased Rin, except in the source regions where some slight cooling trends occurred (Fig. 2d). It is noted that these results are for land grids only. The pattern of cooling in the source regions and warming elsewhere agrees well with the findings reported by Krishnan and Ramanathan (2002). This type of change persists into the near-equilibrium state, where global mean temperature changes and associated feedbacks are included (Fig. 2e–h). Due to the enhanced warming of the atmosphere and probably water vapor buildup, the ↓LW radiation shows a stronger increase (Fig. 2f), making the Rin mostly positive and therefore the temperature change positive, except for source regions (Fig. 2g and h). An open question is how the temperature increased with a decreasing Rin in the rapid adjustment processes. In order to better understand the mechanisms behind this warming phenomenon, we will explore the Eout components in the next section.
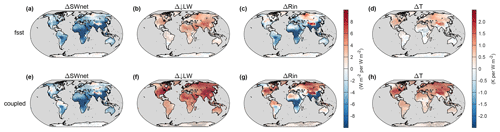
Figure 2MMM changes of surface net SW radiation, downward LW radiation, incoming radiation (Rin), and surface air temperature in the fsst (a–d) and coupled simulations (e–h) for the BC experiment. All changes are normalized to changes per unit of global forcing. Grey dots indicate that the MMM changes are significant at a p value of 0.05.
3.2 Decomposition of outgoing energy
Figure 3 depicts the MMM changes of Rin and all components of Eout in the fsst simulations for all five experiments. The spatial patterns of ΔRin and Δ↑LW for the BC experiment were quite different (Fig. 3d and i). Another notable feature is the significant reductions of sensible and latent heat flux in the BC experiment (Fig. 3n and s), which is in agreement with previous studies (Wilcox et al., 2016; Myhre et al., 2018; Suzuki and Takemura, 2019).
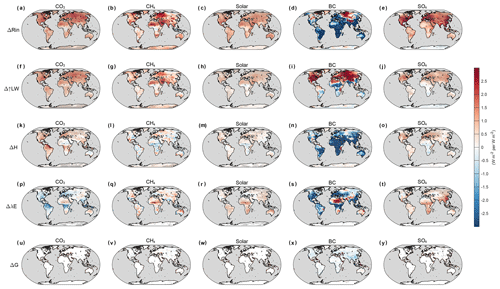
Figure 3MMM changes of Rin and outgoing energy components for all five experiments in the fsst simulations. All changes are normalized to changes per unit of global forcing. Grey dots indicate that the MMM changes are significant at a p value of 0.05.
These changes are obvious when averaged globally (Fig. 4a and Table 2). The Rin decreases by W m−2, and the H and λE decrease by W m−2 and W m−2, respectively, making the energy partitioned to Δ↑LW positive (0.86±0.36 W m−2). In other words, although the radiative heating (Rin) decreases, convective and evaporative cooling decrease by a greater amount (124 % relative to ΔRin) owing to less efficient energy dissipation, thereby warming the surface and leading to a positive Δ↑LW radiation. The reduction of turbulent fluxes (H and λE) is found for both source regions and non-source regions (Fig. 4b). In the source regions, the reductions of turbulent flux are nearly the same as the reduction of Rin, making the temperature response negligible or only slightly negative. In the non-source regions, the reduction of turbulent fluxes exceeds the reduction of Rin, making the temperature response positive. Another interesting phenomenon is that the reduction of H is greater than the reduction of λE for the BC experiment, both on the global scale and in the source regions (Fig. 4). The greater reduction of H indicates a decrease in Bowen ratio (β), defined as the ratio of sensible heat flux over latent heat flux. Globally, β decreases by in the BC case, and such a drop could reach −0.3 in the source regions. In comparison, under other forcing agents, the changes of β are much smaller (the global MMM changes within ±0.10). The larger change of H is somewhat contradicting to the common sense that λE dominates the turbulent flux on a global mean scale. This is because on a global scale, 85 % of λE is from the ocean (Schmitt, 2008). In this study, we only focus on land grids, in which the λE is largely suppressed.
Table 2Globally averaged multi-model mean (MMM ± 1s.e.) values of changes in surface energy components and temperature per unit of global TOA forcing.
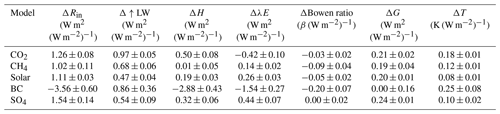
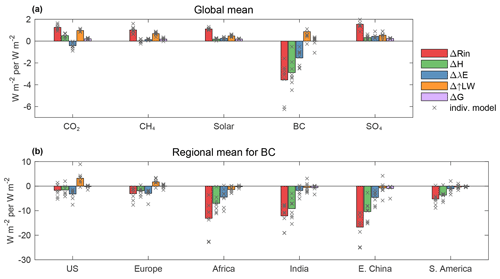
Figure 4Domain-averaged values of Rin and the components of Eout from the fsst simulations for global mean (a) and for selected regions under BC forcing (b). × indicates the values from individual models.
Under other forcing agents, the spatial patterns of Δ↑LW are similar to the patterns of ΔRin. The changes of H and λE are relatively small and sometimes cancel each other out, making little contributions to temperature change compared with BC (Figs. 3 and 4a). Therefore, the temperature change (Δ↑LW) is dominated by the change of radiative heating (ΔRin). It is worth noting that the decrease in λE in the CO2 experiment is due to the physiological effect of vegetation and plantation (Fig. 3p), which is included in the PDRMIP models (Richardson et al., 2018). It is also noted that the stronger responses in the BC scenario (Figs. 3, 4 and Table 2) could be partially related to its larger changes of surface radiative heating (ΔRin) compared with other forcing agents. Taking CO2 as an example, ΔRin is 1.26 W m−2, similar to its TOA forcing (1 W m−2), whereas for BC, ΔRin is roughly 3 times larger (Table 2). Observations show that the surface forcing of BC could be 10 times larger than TOA forcing on regional scales (Magi et al., 2008), indicating that BC could cause stronger changes of surface forcing than TOA forcing relative to other forcing agents. The contributions of G are negligible (Fig. 3u–y) and will not be further discussed.
3.3 Attribution of temperature change
In order to quantify the contributions of each component to ΔT, we applied a multi-linear regression model to the MMM values of ΔT and the energy components in each experiment, as . Here ΔRin represents the changes of radiative heating, and ΔH and ΔλE denote changes in the surface energy redistribution. All grid cells were given equal weight. The results are listed in Table 3. A point-wise comparison of original ΔT and fitted ΔT is shown in Fig. S1. These regressions reproduce the ΔT fairly well, since the correlation coefficients between ΔT and fitted ΔT are all above 0.73, and most of the data points align along the one-to-one line. For CO2, CH4, and solar and sulfate aerosols, the coefficients of ΔRin are 1 order of magnitude larger than the coefficients of the turbulent fluxes, suggesting that ΔRin dominates the temperature change under these forcing agents. When it comes to BC, however, ΔT is more sensitive to ΔH, followed by ΔRin and ΔλE. With the regression coefficients, we estimated the contributions of each energy component to ΔT (Fig. 5). In line with our previous results, ΔT was dominated by surface heating (ΔRin) for most forcing agents with a very limited role from turbulent fluxes. BC, nonetheless, is an exception. For BC aerosols, ΔT is influenced by both surface heating and turbulent fluxes, with the cooling from the former being overwhelmed by the warming from the latter (Fig. 5n, s, and x). The domain-averaged changes for the BC experiment are listed in Table 4. Globally, ΔH produces 0.19 K warming, and ΔλE leads to 0.07 K warming. The combined 0.26 K warming is offset by −0.19 K cooling attributed to reduced ΔRin, producing a net warming of 0.07 K. In terms of percentage, ΔH and ΔλE contribute 73 % and 27 %, respectively, to the total warming. Such patterns are also seen on regional scales. The warming contributions from ΔH were 40 % (US), 47 % (Europe), 76 % (E. China), 87 % (India), 68 % (Africa), and 82 % (S. America), and the remaining part was contributed by ΔλE.
3.4 Mechanisms underlying the reduction of turbulent fluxes
The above analyses show that for most of the forcing agents, ΔRin dominates the surface temperature response, while for BC, the surface energy redistribution also comes into play in modifying temperature response as a result of the significant reductions of turbulent fluxes. The next question is why turbulent fluxes decrease substantially in response to BC particles. According to the bulk parameterization of turbulent fluxes, the sensible heat flux is expressed as ) and latent heat flux as ). In these two equations, ρ is air density, Cp and Lv are air specific heat capacity and latent heat of vaporization, respectively; CH and CE are two exchange coefficients; U denotes surface wind speed; and (Ts−Ta) and (qs−qa) represent temperature gradient and humidity gradient between the surface and air, respectively. In the rapid adjustment stage, wind speed (U) and temperature gradient are the two possible causes for the changes of sensible heat, while wind speed and humidity gradient (qs−qa) are likely to drive the change of latent heat flux.
Table 4Domain-averaged T and contributions from each radiative component estimated from the linear regression model for the BC experiment (unit: K).
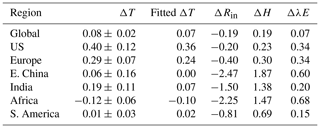
Figure 6a–e show the MMM changes of lower tropospheric stability (LTS), defined as the potential temperature difference between 925 hPa and the surface. An enhanced stability is observed for the BC experiment; ΔLTS is 0.07±0.02 K averaged globally, in contrast to near-zero values from other experiments (−0.01 to 0.01 K). The ΔLTS values for the NH are even larger, with 0.09±0.02 K for BC and −0.01 to 0.01 K for other forcing agents. The enhanced LTS, which can significantly impact the sensible heat flux (Myhre et al., 2018), arises from the fact that the BC layers warm faster relative to the surface due to BC absorption of solar radiation. The changes of LTS patterns are similar for 850 and 700 hPa (Fig. S2).
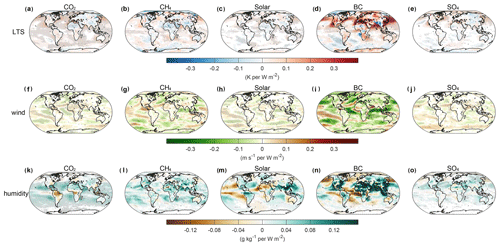
Figure 6MMM changes for lower tropospheric stability (LTS, a–e), surface wind velocity (f–j), and humidity gradient (k–o) per unit of global TOA forcing. For LTS, positive anomalies indicate a more stable atmosphere. The humidity gradient is defined as the specific humidity difference between the surface and 850 hPa. Grey dots indicate that the MMM changes are significant at a p value of 0.05.
Figure 6f–j portray the MMM changes of surface wind speed. BC causes a much larger decrease in wind speed with respect to other forcing agents, 0.05±0.01 m s−1 globally compared with zero from other forcing agents. The reduction in wind speed explains the weakening in both sensible and latent heat fluxes according to the bulk parameterization. Figure 6k–o show the changes of humidity gradient (qs−qa), defined as the specific humidity difference between the surface and 850 hPa. For CH4, solar radiation, and SO4, the gradient increases globally with values of 0.02 g kg−1 (W m−2)−1, 0.01 g kg−1 (W m−2)−1, and 0.01 g kg−1 (W m−2)−1 respectively, causing an increase in λE (Figs. 3 and 4). For CO2, the gradient shows slightly negative values (−0.002 g kg−1 per W m2), corresponding to reduced λE (Figs. 3 and 4). In terms of BC, the humidity gradient increases by 0.06±0.02 g kg−1 (W m−2)−1, but with reduced λE flux, indicating that humidity gradient is not the primary driver of latent heat change. These analyses illustrate that humidity gradient may also influence latent heat flux for CO2, CH4, solar radiation, and scattering aerosols. For BC, on the other hand, change of wind speed should be the primary driver of the reduction of λE, and humidity gradient is of less importance.
Now the last question is why the surface wind speed decreases under BC forcing. The first potential explanation is the abovementioned enhanced LTS. Jacobson and Kaufman (2006) has clearly demonstrated that the enhanced LTS and reduced turbulent exchange can reduce the turbulent kinetic energy and vertical transport of horizonal momentum, thereby reducing surface wind speed. The second possible explanation is that from the dynamical perspective, wind speed is controlled by the pressure gradient force (PGF), the Coriolis force, the gravitational force, and the frictional force. PGF is the driving force for atmospheric motion and is potentially the main driver for the changes of wind speed in the current idealized experiments. On a global scale, the excessive heating in the tropics with respect to middle and high latitudes causes PGF to point toward polar regions. Here we hypothesize that the decrease in temperature gradient between the Equator and poles under BC forcing weakened the PGF and slowed down the wind speed. Evidence in support of this hypothesis is found in Fig. 7a–e showing the zonal mean atmospheric temperature change. Mechanistically, BC caused a larger atmospheric heating in the middle latitudes of NH (30–60∘ N) relative to tropics due to more of the BC forcing being located at middle latitudes of NH (Fig. 7d). The faster warming of middle latitudes weakened the PGF between the Equator and polar regions, as seen from the larger increase in geopotential height of 500 hPa in the middle-latitude regions (Fig. 7i). These patterns are not observed in the other experiments. The changes of geopotential height at other levels show similar results (Fig. S3).
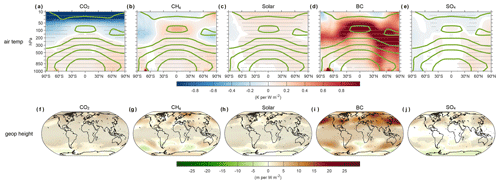
Figure 7MMM changes for zonal atmospheric temperature (a–e) and geopotential height of 500 hPa (f–j) per unit of global TOA forcing. The thick green lines in the upper row are the climatology temperature in the control simulation. Grey dots indicate that the MMM changes are significant at a p value of 0.05.
To further understand the relationship between changes in wind speed and temperature, we defined a temperature gradient index (Allen et al., 2012) as , where ΔT is the mass-weighted (300–850 hPa) temperature response, and subscripts 0–30, 30–60, and 60–90 denote low (0–30∘ N), middle (30–60∘ N), and high (60–90∘ N) latitudinal zones, respectively. When the index becomes more positive, middle latitudes warm faster, and a stronger reduction of wind speed is expected. The results for each individual model and experiment are shown in Fig. 8. A reasonably good correlation is seen in the BC scenario (): a larger change in the temperature gradient index corresponds to a stronger decrease in wind speed. The results for other experiments are mostly scattered around zero.
On regional and local scales, several other factors might also contribute to surface wind change (Wu et al., 2018). For instance, the aerosols in Asia have been reported to modify the land–sea temperature contrast and thus modify monsoon circulation (Xu et al., 2006; Meehl et al., 2008). The different phases of internal variability (e.g., El Niño–Southern Oscillation (ENSO) and North Atlantic Oscillation (NAO)) could modulate the circulations on interannual to multi-decadal timescales (Jerez et al., 2013; Hu and Fedorov, 2018). Bichet et al. (2012) suggested that changes in the surface roughness length may also change the wind speed. These factors are not considered in the present study.
Our analyses demonstrate that under BC forcing surface energy redistribution plays a vital role in modifying the surface temperature due to the changes in turbulent fluxes. The changes of turbulent fluxes are consequences of a downward influence from the atmosphere. The warming of BC layers in the atmosphere enhances air stability and reduces wind speed. As a result, the surface turbulent fluxes are suppressed. This mechanism is not observed for other forcing agents such as greenhouse gases and scattering aerosols. A similar “top-down” mechanism has been previously observed in the solar forcing, in which the stratosphere ozone reacts to the UV part of the solar variability and produces additional heating, leading to changes of circulation in the stratosphere. The changes in the stratosphere modify tropical tropospheric circulation that may impact the surface climate (Haigh, 1996; Gray et al., 2010).
As noted in Sect. 3.1, our above analyses mainly focus on the rapid adjustments, which are part of ERF by definition (Boucher et al., 2013). For the BC experiment, these adjustments drive the surface to respond to the forcing. Most of the changes seen in the rapid adjustment stage extend into the near equilibrium (Figs. S4–S5). For BC forcing, ΔRin in near-equilibrium state is close to zero with large inter-model spread (0.20±1.19 W m−2). The equilibrium turbulent flux H and λE are lowered by and W m−2, respectively, which are comparable in magnitude to changes in the rapid adjustment stage. Such reductions of the equilibrium turbulent fluxes are found in both source regions and non-source regions. Since less energy dissipated away from the surface, more energy (4.34±0.74 W m−2) was partitioned into ΔLW, warming the surface by 0.87±0.13 K. Figure S6 shows the slow responses under each forcing, which are obtained by subtracting the rapid adjustments from the coupled simulations. The slow responses are driven by global mean temperature change alone. Interestingly, the spatial patterns are quite similar across different forcing agents. Our finding further confirms that it is the rapid adjustment that led to the different surface responses to BC.
Two limitations exist in our current study. First, the aerosol–cloud interactions could not be fully represented, because for the models with fixed aerosol concentration, the changes of cloud lifetime do not affect aerosols. Second, for the BC simulations, two models (MIROC and NorESM) include aerosol indirect effects while the remaining ones have only aerosol–radiation interactions included (instantaneous and rapid adjustments). The cloud effects in these two models may slightly modify the SW radiation at the surface (Tang et al., 2020), although the results from these two models do not differ qualitatively from the other models without those effects. We suggest that our conclusions are not sensitive to such cloud effects.
In summary, our study shows that for forcing agents such as greenhouse gas (GHG), solar radiation, and scattering aerosol, ΔRin dominates the surface temperature response. For BC forcing, the surface energy redistribution also plays an important role. Under BC forcing, the energy is dissipated less efficiently from the surface to the lower atmosphere, which causes warming at the surface despite the reduced radiative heating. The reductions of sensible heat flux account for 73 % of the surface warming on a global scale and 40 %–80 % of the warming on regional scales, with the remaining part arising from the reductions of latent heat flux. Such reductions of turbulent fluxes can be explained by enhanced lower tropospheric stability and reduced surface wind speed. The former is attributed to a faster atmospheric warming relative to the surface, whereas the latter is associated with enhanced stability and reduced Equator-to-pole atmospheric temperature gradient. These analyses contribute to our understanding of the impact of absorbing aerosols on surface radiation and climate.
The PDRMIP model output used in this study is available to the public through the Norwegian FEIDE data storage facility. For more information, please see http://cicero.uio.no/en/PDRMIP (Myhre and Forster, 2013). The data shown in the figures are available upon reasonable request.
The supplement related to this article is available online at: https://doi.org/10.5194/acp-21-13797-2021-supplement.
TT designed this study. TT performed the data analysis and wrote the initial manuscript. All authors contributed to scientific discussion, result framing, and manuscript polishing.
The authors declare that they have no conflict of interest.
Publisher's note: Copernicus Publications remains neutral with regard to jurisdictional claims in published maps and institutional affiliations.
We acknowledge the NASA High-End Computing Program through the NASA Center for Climate Simulation at the Goddard Space Flight Center for computational resources to run the GISS-E2R model and support from NASA GISS. PDRMIP is partly funded through the Norwegian Research Council project NAPEX (project number 229778). Apostolos Voulgarakis is partially funded by the Leverhulme Trust, grant RC-2018-023. Computing resources for CESM1-CAM5 (ark:/85065/d7wd3xhc) simulations were provided by the Climate Simulation Laboratory at NCAR Computational and Information System Laboratory, sponsored by the National Science Foundation and other agencies. Xuhui Lee acknowledges support from the US National Science Foundation (grant AGS1933630). Toshihiko Takemura is supported by the Japan Society for the Promotion of Science (JSPS) KAKENHI (grant no. JP19H05669), the Environment Research and Technology Development Fund (S-20) of the Environmental Restoration and Conservation Agency, Japan, and the NEC SX supercomputer system of the National Institute for Environmental Studies, Japan. Timothy Andrews was supported by the Met Office Hadley Centre Climate Programme funded by BEIS and Defra and the Newton Fund through the Met Office Climate Science for Service Partnership Brazil (CSSP Brazil).
The research has been partially supported by the NSF of the United States, with the grant no. 14-19398 and grant AGS1933630.
This paper was edited by Zhanqing Li and reviewed by two anonymous referees.
Allen, R. J., Sherwood, S. C., Norris, J. R., and Zender, C. S.: Recent northern hemisphere tropical expansion primarily driven by black carbon and tropospheric ozone, Nature, 485, 350–354, https://doi.org/10.1038/nature11097, 2012.
Andrews, T., Gregory, J. M., Webb, M. J., and Taylor, K. E.: Forcing, feedbacks and climate sensitivity in CMIP5 coupled atmosphere-ocean climate models, Geophys. Res. Lett., 39, L09712, https://doi.org/10.1029/2012gl051607, 2012.
Arora, V., Scinocca, J., Boer, G., Christian, J., Denman, K., Flato, G., Kharin, V., Lee, W., and Merryfield, W.: Carbon emission limits required to satisfy future Representative Concentration Pathways of greenhouse gases, Geophys. Res. Lett., 38, L05805, https://doi.org/10.1029/2010GL046270, 2011.
Bellouin, N., Rae, J., Jones, A., Johnson, C., Haywood, J., and Boucher, O.: Aerosol forcing in the Climate Model Intercomparison Project (CMIP5) simulations by HadGEM2-ES and the role of ammonium nitrate, J. Geophys. Res.-Atmos., 116, D20206, https://doi.org/10.1029/2011JD016074, 2011.
Bentsen, M., Bethke, I., Debernard, J. B., Iversen, T., Kirkevåg, A., Seland, Ø., Drange, H., Roelandt, C., Seierstad, I. A., Hoose, C., and Kristjánsson, J. E.: The Norwegian Earth System Model, NorESM1-M – Part 1: Description and basic evaluation of the physical climate, Geosci. Model Dev., 6, 687–720, https://doi.org/10.5194/gmd-6-687-2013, 2013.
Bichet, A., Wild, M., Folini, D., and Schär, C.: Causes for decadal variations of wind speed over land: Sensitivity studies with a global climate model, Geophys. Res. Lett., 39, L11701, https://doi.org/10.1029/2012GL051685, 2012.
Bond, T. C., Doherty, S. J., Fahey, D. W., Forster, P. M., Berntsen, T., DeAngelo, B. J., Flanner, M. G., Ghan, S., Karcher, B., Koch, D., Kinne, S., Kondo, Y., Quinn, P. K., Sarofim, M. C., Schultz, M. G., Schulz, M., Venkataraman, C., Zhang, H., Zhang, S., Bellouin, N., Guttikunda, S. K., Hopke, P. K., Jacobson, M. Z., Kaiser, J. W., Klimont, Z., Lohmann, U., Schwarz, J. P., Shindell, D., Storelvmo, T., Warren, S. G., and Zender, C. S.: Bounding the role of black carbon in the climate system: A scientific assessment, J. Geophys. Res.-Atmos., 118, 5380–5552, https://doi.org/10.1002/jgrd.50171, 2013.
Boucher, O., Randall, D., Artaxo, P., Bretherton, C., Feingold, G., Forster, P., Kerminen, V.-M., Kondo, Y., Liao, H., and Lohmann, U.: Clouds and aerosols, in: Climate change 2013: The physical science basis. Contribution of working group i to the fifth assessment report of the intergovernmental panel on climate change, edited by: Stoker, T. F., Qin, D., Plattner, G.-K., Tignor, M., Allen, S. K., Boschung, J., Nauels, A., Xia, Y., Bex, V., and Midgley, P. M., 571–657, Cambridge University Press, Cambridge, UK and New York, USA, 2013.
Collins, W. J., Bellouin, N., Doutriaux-Boucher, M., Gedney, N., Halloran, P., Hinton, T., Hughes, J., Jones, C. D., Joshi, M., Liddicoat, S., Martin, G., O'Connor, F., Rae, J., Senior, C., Sitch, S., Totterdell, I., Wiltshire, A., and Woodward, S.: Development and evaluation of an Earth-System model – HadGEM2, Geosci. Model Dev., 4, 1051–1075, https://doi.org/10.5194/gmd-4-1051-2011, 2011.
Gent, P. R., Danabasoglu, G., Donner, L. J., Holland, M. M., Hunke, E. C., Jayne, S. R., Lawrence, D. M., Neale, R. B., Rasch, P. J., and Vertenstein, M.: The Community Climate System Model version 4, J. Climate, 24, 4973–4991, https://doi.org/10.1175/2011JCLI4083.1, 2011.
Gray, L. J., Beer, J., Geller, M., Haigh, J. D., Lockwood, M., Matthes, K., Cubasch, U., Fleitmann, D., Harrison, G., Hood, L., Luterbacher, J., Meehl, G. A., Shindell, D., Van Geel, B., and White, W.: Solar influences on climate, Rev. Geophys., 48, RG4001, https://doi.org/10.1029/2009rg000282, 2010.
Haigh, J. D.: The impact of solar variability on climate, Science, 272, 981–984, https://doi.org/10.1126/science.272.5264.981, 1996.
Hansen, J., Sato, M., Nazarenko, L., Ruedy, R., Lacis, A., Koch, D., Tegen, I., Hall, T., Shindell, D., Santer, B., Stone, P., Novakov, T., Thomason, L., Wang, R., Wang, Y., Jacob, D., Hollandsworth, S., Bishop, L., Logan, J., Thompson, A., Stolarski, R., Willson, R., Levitus, S., Antonov, J., Rayner, N., Parker, D., and Christy, J.: Climate forcings in Goddard Institute for Space Studies SI2000 simulations, J. Geophys. Res.-Atmos., 107, 4347, https://doi.org/10.1029/2001JD001143, 2002.
Hu, S. N. and Fedorov, A. V.: Cross-equatorial winds control El Niño diversity and change, Nat. Clim. Change, 8, 798–802, https://doi.org/10.1038/s41558-018-0248-0, 2018.
Hurrell, J. W., Holland, M. M., Gent, P. R., Ghan, S., Kay, J. E., Kushner, P. J., Lamarque, J.-F., Large, W. G., Lawrence, D., and Lindsay, K.: The Community Earth System Model: A framework for collaborative research, B. Am. Meteorol. Soc., 94, 1339–1360, https://doi.org/10.1175/BAMS-D-12-00121.1, 2013.
Iversen, T., Bentsen, M., Bethke, I., Debernard, J. B., Kirkevåg, A., Seland, Ø., Drange, H., Kristjansson, J. E., Medhaug, I., Sand, M., and Seierstad, I. A.: The Norwegian Earth System Model, NorESM1-M – Part 2: Climate response and scenario projections, Geosci. Model Dev., 6, 389–415, https://doi.org/10.5194/gmd-6-389-2013, 2013.
Jacobson, M. Z. and Kaufman, Y. J.: Wind reduction by aerosol particles. Geophys. Res. Lett., 33, L24814, https://doi.org/10.1029/2006gl027838, 2006.
Jerez, S., Trigo, R. M., Vicente-Serrano, S. M., Pozo-Vázquez, D., Lorente-Plazas, R., Lorenzo-Lacruz, J., Santos-Alamillos, F., and Montávez, J. P.: The impact of the North Atlantic Oscillation on renewable energy resources in Southwestern Europe, J. Appl. Meteorol. Clim., 52, 2204–2225, https://doi.org/10.1175/JAMC-D-12-0257.1, 2013.
Kay, J., Deser, C., Phillips, A., Mai, A., Hannay, C., Strand, G., Arblaster, J., Bates, S., Danabasoglu, G., and Edwards, J.: The Community Earth System Model (CESM) large ensemble project: A community resource for studying climate change in the presence of internal climate variability, B. Am. Meteorol. Soc., 96, 1333–1349, https://doi.org/10.1175/BAMS-D-13-00255.1, 2015.
Kirkevåg, A., Iversen, T., Seland, Ø., Hoose, C., Kristjánsson, J. E., Struthers, H., Ekman, A. M. L., Ghan, S., Griesfeller, J., Nilsson, E. D., and Schulz, M.: Aerosol–climate interactions in the Norwegian Earth System Model – NorESM1-M, Geosci. Model Dev., 6, 207–244, https://doi.org/10.5194/gmd-6-207-2013, 2013.
Koch, D. and Del Genio, A. D.: Black carbon semi-direct effects on cloud cover: review and synthesis, Atmos. Chem. Phys., 10, 7685–7696, https://doi.org/10.5194/acp-10-7685-2010, 2010.
Krishnan, R., and Ramanathan, V.: Evidence of surface cooling from absorbing aerosols, Geophys. Res. Lett., 29, https://doi.org/10.1029/2002GL014687, 2002.
Liepert, B. G., Feichter, J., Lohmann, U., and Roeckner, E.: Can aerosols spin down the water cycle in a warmer and moister world?, Geophys. Res. Lett., 31, L06207, https://doi.org/10.1029/2003GL019060, 2004.
Magi, B. I., Fu, Q., Redemann, J., and Schmid, B.: Using aircraft measurements to estimate the magnitude and uncertainty of the shortwave direct radiative forcing of southern african biomass burning aerosol, J. Geophys. Res.-Atmos., 113, D05213, https://doi.org/10.1029/2007JD009258, 2008.
Meehl, G. A., Arblaster, J. M., and Collins, W. D.: Effects of black carbon aerosols on the Indian monsoon, J. Climate, 21, 2869–2882, https://doi.org/10.1175/2007jcli1777.1, 2008.
Menon, S., Hansen, J., Nazarenko, L., and Luo, Y.: Climate effects of black carbon aerosols in China and India, Science, 297, 2250–2253, https://doi.org/10.1126/science.1075159, 2002.
Myhre, G. and Forster, P.: Precipitation driver response model intercomparison project, available at: https://cicero.oslo.no/en/PDRMIP (last access: 14 September 2021), 2013.
Myhre, G., Forster, P., Samset, B., Hodnebrog, Ø., Sillmann, J., Aalbergsjø, S., Andrews, T., Boucher, O., Faluvegi, G., Fläschner, D., Iversen, T., Kasoar, M., Kharin, V., Kirkeväg, A., Lamarque, J.-F., Olivié, D., Richardson, T., Shindell, D., Shine, K., Stjern, C., Takemura, T., Voulgarakis, A., and Zwiers, F.: PDRMIP: A Precipitation Driver and Response Model Intercomparison Project, protocol and preliminary results, B. Am. Meteorol. Soc., 98, 1185–1198, https://doi.org/10.1175/BAMS-D-16-0019.1, 2017.
Myhre, G., Samset, B. H., Schulz, M., Balkanski, Y., Bauer, S., Berntsen, T. K., Bian, H., Bellouin, N., Chin, M., Diehl, T., Easter, R. C., Feichter, J., Ghan, S. J., Hauglustaine, D., Iversen, T., Kinne, S., Kirkevåg, A., Lamarque, J.-F., Lin, G., Liu, X., Lund, M. T., Luo, G., Ma, X., van Noije, T., Penner, J. E., Rasch, P. J., Ruiz, A., Seland, Ø., Skeie, R. B., Stier, P., Takemura, T., Tsigaridis, K., Wang, P., Wang, Z., Xu, L., Yu, H., Yu, F., Yoon, J.-H., Zhang, K., Zhang, H., and Zhou, C.: Radiative forcing of the direct aerosol effect from AeroCom Phase II simulations, Atmos. Chem. Phys., 13, 1853–1877, https://doi.org/10.5194/acp-13-1853-2013, 2013a.
Myhre, G., Shindell, D., Bréon, F.-M., Collins, W., Fuglestvedt, J., Huang, J., Koch, D., Lamarque, J.-F., Lee, D., Mendoza, B., Nakajima, T., Robock, A., Stephens, G., Takemura, T., and Zhang, H.: Anthropogenic and natural radiative forcing, in: Climate change 2013: The physical science basis. Contribution of working group I to the fifth assessment report of the intergovernmental panel on climate change, Cambridge University Press, Cambridge, UK and New York, USA, 2013b.
Myhre, G., Samset, B. H., Hodnebrog, O., Andrews, T., Boucher, O., Faluvegi, G., Flaschner, D., Forster, P. M., Kasoar, M., Kharin, V., Kirkevag, A., Lamarque, J. F., Olivie, D., Richardson, T. B., Shawki, D., Shindell, D., Shine, K. P., Stjern, C. W., Takemura, T., and Voulgarakis, A.: Sensible heat has significantly affected the global hydrological cycle over the historical period, Nat. Commun., 9, 1922, https://doi.org/10.1038/s41467-018-04307-4, 2018.
Neale, R. B., Richter, J. H., Conley, A. J., Park, S., Lauritzen, P. H., Gettelman, A., Williamson, D. L., Rasch, P. J., Vavrus, S. J., Taylor, M. A., Collins, W. D., Zhang, M., and Lin, S.-J.: Description of the NCAR Community Atmosphere Model (CAM 4.0), Boulder, CO, USA, available at: https://www.ccsm.ucar.edu/models/ccsm4.0/cam/docs/description/cam4_desc.pdf (last access: 14 September 2021), 2010.
Otto-Bliesner, B. L., Brady, E. C., Fasullo, J., Jahn, A., Landrum, L., Stevenson, S., Rosenbloom, N., Mai, A., and Strand, G.: Climate variability and change since 850 ce: An ensemble approach with the Community Earth System Model, B. Am. Meteorol. Soc., 97, 735–754, https://doi.org/10.1175/BAMS-D-14-00233.1, 2016.
Ramanathan, V. and Carmichael, G.: Global and regional climate changes due to black carbon, Nat. Geosci., 1, 221–227, https://doi.org/10.1038/ngeo156, 2008.
Ramanathan, V., Crutzen, P. J., Kiehl, J. T., and Rosenfeld, D.: Aerosols, climate, and the hydrological cycle, Science, 294, 2119–2124, https://doi.org/10.1126/science.1064034, 2001.
Richardson, T. B., Forster, P. M., Andrews, T., Boucher, O., Faluvegi, G., Flaschner, D., Kasoar, M., Kirkevag, A., Lamarque, J. F., Myhre, G., Olivie, D., Samset, B. H., Shawki, D., Shindell, D., Takemura, T., and Voulgarakis, A.: Carbon dioxide physiological forcing dominates projected Eastern Amazonian drying, Geophys. Res. Lett., 45, 2815–2825, https://doi.org/10.1002/2017gl076520, 2018.
Samset, B., Myhre, G., Forster, P., Hodnebrog, Ø., Andrews, T., Faluvegi, G., Flaeschner, D., Kasoar, M., Kharin, V., and Kirkevåg, A.: Fast and slow precipitation responses to individual climate forcers: A PDRMIP multimodel study, Geophys. Res. Lett., 43, 2782–2791, https://doi.org/10.1002/2016GL068064, 2016.
Schmidt, G. A., Kelley, M., Nazarenko, L., Ruedy, R., Russell, G. L., Aleinov, I., Bauer, M., Bauer, S. E., Bhat, M. K., and Bleck, R.: Configuration and assessment of the GISS model E2 contributions to the CMIP5 archive, J. Adv. Model. Earth Sy., 6, 141–184, https://doi.org/10.1002/2013MS000265, 2014.
Schmitt, R. W.: Salinity and the global water cycle, Oceanography, 21, 12–19, 2008.
Suzuki, K. and Takemura, T.: Perturbations to global energy budget due to absorbing and scattering aerosols, J. Geophys. Res.-Atmos., 124, 2194–2209, https://doi.org/10.1029/2018JD029808, 2019.
Takemura, T., Egashira, M., Matsuzawa, K., Ichijo, H., O'ishi, R., and Abe-Ouchi, A.: A simulation of the global distribution and radiative forcing of soil dust aerosols at the Last Glacial Maximum, Atmos. Chem. Phys., 9, 3061–3073, https://doi.org/10.5194/acp-9-3061-2009, 2009.
Takemura, T., Nozawa, T., Emori, S., Nakajima, T. Y., and Nakajima, T.: Simulation of climate response to aerosol direct and indirect effects with aerosol transport-radiation model, J. Geophys. Res.-Atmos., 110, D02202, https://doi.org/10.1029/2004JD005029, 2005.
Tang, T., Shindell, D., Faluvegi, G., Myhre, G., Olivié, D., Voulgarakis, A., Kasoar, M., Andrews, T., Boucher, O., Forster, P. M., Hodnebrog, Ø., Iversen, T., Kirkevåg, A., Lamarque, J. F., Richardson, T., Samset, B. H., Stjern, C. W., Takemura, T., and Smith, C.: Comparison of effective radiative forcing calculations using multiple methods, drivers, and models, J. Geophys. Res.-Atmos., 124, 4382–4394, https://doi.org/10.1029/2018JD030188, 2019.
Tang, T., Shindell, D., Samset, B. H., Boucher, O., Forster, P. M., Hodnebrog, Ø., Myhre, G., Sillmann, J., Voulgarakis, A., Andrews, T., Faluvegi, G., Fläschner, D., Iversen, T., Kasoar, M., Kharin, V., Kirkevåg, A., Lamarque, J.-F., Olivié, D., Richardson, T., Stjern, C. W., and Takemura, T.: Dynamical response of Mediterranean precipitation to greenhouse gases and aerosols, Atmos. Chem. Phys., 18, 8439–8452, https://doi.org/10.5194/acp-18-8439-2018, 2018.
Tang, T., Shindell, D., Zhang, Y., Voulgarakis, A., Lamarque, J.-F., Myhre, G., Stjern, C. W., Faluvegi, G., and Samset, B. H.: Response of surface shortwave cloud radiative effect to greenhouse gases and aerosols and its impact on summer maximum temperature, Atmos. Chem. Phys., 20, 8251–8266, https://doi.org/10.5194/acp-20-8251-2020, 2020.
Walters, D. N., Williams, K. D., Boutle, I. A., Bushell, A. C., Edwards, J. M., Field, P. R., Lock, A. P., Morcrette, C. J., Stratton, R. A., Wilkinson, J. M., Willett, M. R., Bellouin, N., Bodas-Salcedo, A., Brooks, M. E., Copsey, D., Earnshaw, P. D., Hardiman, S. C., Harris, C. M., Levine, R. C., MacLachlan, C., Manners, J. C., Martin, G. M., Milton, S. F., Palmer, M. D., Roberts, M. J., Rodríguez, J. M., Tennant, W. J., and Vidale, P. L.: The Met Office Unified Model Global Atmosphere 4.0 and JULES Global Land 4.0 configurations, Geosci. Model Dev., 7, 361–386, https://doi.org/10.5194/gmd-7-361-2014, 2014.
Watanabe, M., Suzuki, T., O'ishi, R., Komuro, Y., Watanabe, S., Emori, S., Takemura, T., Chikira, M., Ogura, T., and Sekiguchi, M.: Improved climate simulation by MIROC5: Mean states, variability, and climate sensitivity, J. Climate, 23, 6312–6335, https://doi.org/10.1175/2010JCLI3679.1, 2010.
Wilcox, E. M., Thomas, R. M., Praveen, P. S., Pistone, K., Bender, F. A. M., and Ramanathan, V.: Black carbon solar absorption suppresses turbulence in the atmospheric boundary layer, P. Natl. Acad. Sci. USA, 113, 11794, https://doi.org/10.1073/pnas.1525746113, 2016.
Wild, M., Ohmura, A., Gilgen, H., and Rosenfeld, D.: On the consistency of trends in radiation and temperature records and implications for the global hydrological cycle, Geophys. Res. Lett., 31, L11201, https://doi.org/10.1029/2003GL019188, 2004.
Wu, J., Zha, J., Zhao, D., and Yang, Q.: Changes in terrestrial near-surface wind speed and their possible causes: An overview, Clim. Dynam., 51, 2039–2078, https://doi.org/10.1007/s00382-017-3997-y, 2018.
Xu, M., Chang, C.-P., Fu, C., Qi, Y., Robock, A., Robinson, D., and Zhang, H.: Steady decline of east Asian monsoon winds, 1969–2000: Evidence from direct ground measurements of wind speed, J. Geophys. Res.-Atmos., 111, D24111, https://doi.org/10.1029/2006JD007337, 2006.