the Creative Commons Attribution 4.0 License.
the Creative Commons Attribution 4.0 License.
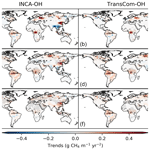
Accelerating methane growth rate from 2010 to 2017: leading contributions from the tropics and East Asia
Frederic Chevallier
Philippe Ciais
Philippe Bousquet
Marielle Saunois
John Worden
A. Anthony Bloom
Robert J. Parker
Daniel J. Jacob
Edward J. Dlugokencky
Christian Frankenberg
After stagnating in the early 2000s, the atmospheric methane growth rate has been positive since 2007 with a significant acceleration starting in 2014. While the causes for previous growth rate variations are still not well determined, this recent increase can be studied with dense surface and satellite observations. Here, we use an ensemble of six multi-species atmospheric inversions that have the capacity to assimilate observations of the main species in the methane oxidation chain – namely, methane, formaldehyde, and carbon monoxide – to simultaneously optimize both the methane sources and sinks at each model grid. We show that the surge of the atmospheric growth rate between 2010–2013 and 2014–2017 is most likely explained by an increase of global CH4 emissions by 17.5±1.5 Tg yr−1 (mean ± 1σ), while variations in the hydroxyl radicals (OH) remained small. The inferred emission increase is consistently supported by both surface and satellite observations, with leading contributions from the tropical wetlands (∼ 35 %) and anthropogenic emissions in China (∼ 20 %). Such a high consecutive atmospheric growth rate has not been observed since the 1980s and corresponds to unprecedented global total CH4 emissions.
- Article
(1945 KB) - Full-text XML
-
Supplement
(4659 KB) - BibTeX
- EndNote
Methane (CH4) is an important greenhouse gas highly relevant to climate mitigation, given its stronger warming potential and shorter lifetime than carbon dioxide (CO2) (IPCC, 2013). Atmospheric levels of methane, usually measured as a dry air mole fraction, have nearly tripled since the industrial revolution according to ice core records (Etheridge et al., 1998; Rubino et al., 2019). This increase is mostly due to increases in anthropogenic emissions from agriculture (ruminant livestock and rice farming), fossil fuel use, and waste processing (Kirschke et al., 2013; Saunois et al., 2016; Schaefer, 2019). A significant portion of methane is also emitted from natural sources, including wetlands, inland freshwaters, geological sources, and biomass burning (although many of the wildfires may have anthropogenic origins) (Saunois et al., 2016). Methane has a lifetime of around 10 years in the atmosphere (Naik et al., 2013), with a dominant sink from oxidation by hydroxyl radicals (OH) in the troposphere (∼ 90 % of the total sink) (Saunois et al., 2019). In addition, its reactions with atomic chlorine (Cl), soil deposition, and stratospheric loss through reaction with a range of reactants (including O(1D), Cl and OH) account for a minor portion of the total methane sink (Saunois et al., 2019).
Since the beginning of the direct measurement period in the early 1980s, the methane growth rate had been gradually declining until it reached a stagnation between the late 1990s and 2006, often referred to as the ”stabilization” period (Dlugokencky et al., 1998, 2003). However, methane has been increasing again since 2007 (Dlugokencky et al., 2009; Nisbet et al., 2014). A sharp increase of the growth rate was observed in 2014 from surface background stations (12.6 ± 0.5 ppb yr−1; mean ± 1σ) (Nisbet et al., 2016; Fletcher and Schaefer, 2019; Nisbet et al., 2019), more than twice the average growth rate of 5.7 ± 1.1 ppb yr−1 during the post stagnation period between 2007 and 2013. Since then, the CH4 growth rate has remained high (8.6 ± 1.6 ppb yr−1 for 2014–2017). Understanding the methane source and sink changes underlying the variations in methane growth rate can help us identify how methane sources respond to human activity, climate, or environmental changes, which are critical to climate mitigation efforts.
The attribution of the plateau and regrowth in atmospheric methane during the 2000s reached conflicting conclusions about the role of fossil fuel emissions (Hausmann et al., 2016; Simpson et al., 2012; Worden et al., 2017), agriculture or wetland emissions (Nisbet et al., 2016; Saunois et al., 2017; Schaefer et al., 2016), OH concentration (Rigby et al., 2017; Turner et al., 2017), and biospheric sinks (Thompson et al., 2018). The range of competing explanations exemplifies the complexity and uncertainty of interpolating limited observations of atmospheric methane and its 13C 12C isotopic ratio (expressed as δ13CH4) to changes in different sectors of methane sources as well as its sinks (Turner et al., 2019; Schaefer, 2019). The situation now is more encouraging than the previous decade as we have continuous global satellite retrievals of the total column CH4 dry air mole fraction (denoted as X) from the Greenhouse Gases Observing Satellite (GOSAT) with better precision and accuracy than previous instruments (Kuze et al., 2009; Parker et al., 2015; Jacob et al., 2016; Buchwitz et al., 2017; Houweling et al., 2017). The combined information from satellite and surface observations provides us a unique opportunity to understand the recent changes in methane growth rate with better spatial coverage.
Atmospheric methane measurements can be linked quantitatively to regional sources and sinks by inverse modeling, where changes in the atmospheric transport are guided by meteorological reanalysis and fluxes are adjusted to match the temporal and spatial variations of the observations given their uncertainties in a Bayesian formalism (Chevallier et al., 2005). A number of inverse studies have explored the surface and GOSAT observations to improve methane emission estimates (Monteil et al., 2013; Cressot et al., 2014; Alexe et al., 2015; Miller et al., 2019; Ganesan et al., 2017; Maasakkers et al., 2019), but the recent acceleration of methane growth since 2014 has not been widely investigated (Nisbet et al., 2019; McNorton et al., 2018; Turner et al., 2019; Zhang et al., 2021). GOSAT satellite X retrievals agree with the surface methane observations on the acceleration of the increase in atmospheric methane burden over the period from mid-2009 to the end of 2017 (Fig. 1). However, the satellite column data show a smoother temporal variation in the global average growth rate. GOSAT X growth rates over different regions show diverse temporal patterns with a higher variability than the global average (Fig. S1 in the Supplement), suggesting that satellite data sampling directly over the source regions could provide valuable information to track regional changes in CH4 fluxes. Furthermore, species in the oxidation chain of methane – namely, methane–formaldehyde–carbon monoxide (CH4–HCHO–CO) – with their reactions to OH as the common sink path, could provide additional constraints on the OH sink of methane. A recent study showed that HCHO levels can provide information about remote tropospheric OH concentrations (Wolfe et al., 2019), and the feedback of CO variations on OH is directly linked to the sink of CH4 (Gaubert et al., 2017; Nguyen et al., 2020). Hence, satellite retrievals of XHCHO from the Ozone Monitoring Instrument (OMI) (González Abad et al., 2016) and XCO from the Measurements of Pollution in the Troposphere (MOPITT) instrument (Deeter et al., 2017) covering the study period could, in theory, provide additional constraints on regional variations of methane sinks.
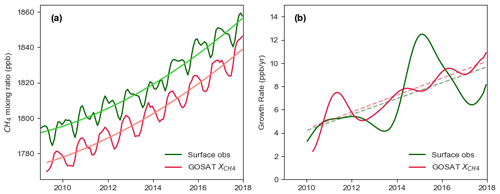
Figure 1Atmospheric methane mixing ratio changes. (a) Monthly time series of the global mean methane mixing ratio from mid-2009 to the end of 2017. The green curve represents methane mixing ratios in the marine boundary layer observed by the NOAA surface network (https://gml.noaa.gov/ccgg/mbl/, last access: 1 May 2020). The red curve represents the total column mixing ratio, X, seen by GOSAT satellite and averaged from all soundings over the land. The smooth curve fit shows a quadratic fit of the trend that accelerates in the latter part of the study period. (b) Smooth methane growth rate derived from the time series shown in (a) following the methods of Thoning et al. (1989).
Hence, we developed a multi-tracer variational inverse system, PYVAR-LMDZ, with the capacity to assimilate observations of the CH4–HCHO–CO oxidation chain to better constrain the sources and sinks of these species at individual model grid cells (Chevallier et al., 2005; Pison et al., 2009; Fortems-Cheiney et al., 2012; Yin et al., 2015; Zheng et al., 2019). Given the observed changes in temporal and spatial variations of all three species, we optimize simultaneously (i) methane emissions, (ii) CO emissions, (iii) HCHO sources (surface emissions + chemical productions from VOC oxidation), and (iv) OH concentrations. These terms are optimized at a weekly temporal resolution and a 1.9∘ by 3.75∘ spatial resolution. In addition, we optimize the initial concentrations of all four species on the individual model grid cell. Here, we performed an ensemble of six inversions using different combinations of observational constraints (surface vs. satellite, single vs. multiple species) and alternative prior estimates of 3-D OH distributions. With the ensemble results we aim to (1) identify key regions that contribute to the methane growth rate acceleration from 2010 to 2017 and (2) evaluate the consistency of results inferred from surface and satellite observations. Inversion methods and observational datasets are documented in Sect. 2. We report estimates of the global methane budget change from 2010 to 2017 in Sect. 3 and discuss regional attributions and sources of uncertainties in Sect. 4. Section 5 summarizes this work and provides some perspectives for future studies.
2.1 Atmospheric observations
We assimilate surface and satellite methane observations in parallel to test the consistency of information brought by these two types of measurements. We also include versions assimilating HCHO and CO along with CH4 to test the impacts of adding chemically related species. In total, there are three groups of observational constraints:
- S1
Methane and CO measurements from surface stations;
- S2
GOSAT X;
- S3
GOSAT X, OMI XHCHO, and MOPITT XCO.
The assimilation is done from April 2009 to February 2018, and we analyze the results of the 8 full years of 2010–2017 with the starting and ending period being spin-up and spin-down phases to avoid the edge effect.
2.1.1 Surface observations
We include surface methane observations from a total of 103 stations (Fig. S2; Table S3), with leading contributions from the following networks: the US National Oceanic and Atmospheric Administration (NOAA, 58 stations), Australia's Commonwealth Scientific and Industrial Research Organisation (CSIRO, nine stations), Environment and Climate Change Canada (ECCC, eight stations), and the Advanced Global Atmospheric Gases Experiment (AGAGE, five stations) (Prinn et al., 2018). Measurements from different networks are calibrated to the WMO scale. Daily afternoon averages between 12:00 and 18:00 local time are used for the assimilation of the continuous in situ measurements to minimize uncertainties associated with boundary layer height modeling. CO observations from those stations are also assimilated in S1.
2.1.2 Satellite observations
The TANSO-FTS instrument onboard the Greenhouse Gases Observing Satellite (GOSAT) was launched by The Japan Aerospace Exploration Agency (JAXA) into a polar sun-synchronous orbit in early 2009. It observes column-averaged dry-air carbon dioxide and methane mixing ratios by solar backscatter in the shortwave infrared (SWIR) with near-unit sensitivity across the air column down to the surface (Butz et al., 2011; Kuze et al., 2016). Observations are made at around 13:00 local time with a circular pixel around 10 km in diameter. The distances between pixels both along and cross track are ∼ 250 km in the default observation mode, and the revisit time for the same observation location is 3 d. Denser observations over particular areas of interest are made in target mode. Here, we use GOSAT X proxy retrievals (OCPR) version 7.2 from the University of Leicester, which has been well documented and evaluated against various observations. The retrieval has a single-observation precision of 14 ppb (∼ 0.7 %) and a regional bias of ∼ 4 ppb compared to TCCON stations (Parker et al., 2015, 2020). This product is also consistent with other GOSAT methane retrievals (Buchwitz et al., 2017). However, we note that there is limited spatial coverage of TCCON stations to fully evaluate GOSAT observations in the high latitudes and the tropics. We only assimilate GOSAT retrievals over land to minimize potential retrieval biases between nadir and glint viewing modes. The same GOSAT data are assimilated in both S2 and S3.
For the multi-tracer inversion, S3, we also include OMI XHCHO retrievals version 3 from the Smithsonian Astrophysical Observatory (SAO) (González Abad et al., 2016) and MOPITT XCO retrievals version 7 from NCAR (Deeter et al., 2017). All satellite retrievals are processed following the recommend quality flags and the application of corresponding prior profiles and retrieval averaging kernels when provided. We exclude data poleward of 60∘. Individual retrievals that are located in the same model grid within 3 h intervals are averaged for further assimilation. The observation uncertainty contains the retrieval errors as reported by the data product plus model errors whose standard deviations are empirically set as 1 % for CH4, 30 % for CO, and 30 % for HCHO based on previous experiments (Fortems-Cheiney et al., 2012; Cressot et al., 2014; Yin et al., 2015).
Satellite retrievals of the three species (CH4, HCHO, and CO) we use here are generally sensitive to the entire vertical column with some differences toward the lower troposphere. GOSAT X retrievals using shortwave infrared (SWIR) radiances have approximately uniform sensitivity to methane at all pressure levels (Parker et al., 2015). OMI HCHO retrievals using ultraviolet (UV) radiance are sensitive to the entire column with some decline in the lowest atmospheric layers (González Abad et al., 2016). For MOPITT, we use the multispectral total column CO retrieval products that combine near-infrared (NIR) and thermal infrared (TIR) radiances and hence have an enhanced sensitivity to the lower troposphere (Deeter et al., 2014). Such subtle differences in the vertical sensitivities of the three retrievals, as well as their different vertical profiles and lifetimes, may influence the ways the observations of the three species provide information about OH, which is another source of uncertainty in addition to the model and observation errors.
2.1.3 Ground-based total column measurements
Ground-based X retrievals from the Total Carbon Column Observing Network (TCCON) from 27 stations are used for an independent evaluation of the posterior model states. TCCON is a network of Fourier transform spectrometers (FTSs) from near-infrared (NIR) solar absorption spectra, designed to retrieve precise total column abundances of CO2, CH4, N2O, and CO to validate satellite observations (Wunch et al., 2011).
2.2 Inverse modeling
2.2.1 Variational inverse system
We use a Bayesian variational inversion system, PYVAR-LMDz, which uses LMDz-INCA as the chemistry transport model (CTM) (Hourdin et al., 2013; Hauglustaine, 2004). This inversion system has been documented and evaluated by a series of studies focusing on tracers including CH4 (Pison et al., 2009; Locatelli et al., 2015; Cressot et al., 2014), HCHO (Fortems-Cheiney et al., 2012), CO (Yin et al., 2015; Zheng et al., 2019), and CO2 (Chevallier et al., 2005, 2010). We use a recently developed version that has the capacity to assimilate observations of the major tracers in the CH4 oxidation chain – namely, CH4–HCHO–CO – with OH being their common sink path, to optimize the sources and sinks for all these species simultaneously. Here, we use a simplified chemistry scheme that assumes methane being oxidized into formaldehyde in a single step. We expect this simplification to have a relatively small impact on the inverse results of methane, given that all pathways of methane oxidation result in formaldehyde as an intermediate product. In addition, HCHO production from non-methane VOC oxidation is simulated upstream with a full-chemistry model, so that the correction on OH from the inversion will not directly feedback to the VOC oxidation. This should not be an issue as we optimize the production of HCHO instead of VOC emissions, but the impact of VOC on OH recycling is not accounted for. Future studies using a full chemistry scheme to optimize methane and OH simultaneously would be helpful to diagnose potential impacts of this simplification on the derived methane lifetime.
The CTM version we use here has a horizontal resolution of 1.875∘ × 3.75∘ (latitude, longitude) and a vertical resolution of 39 eta levels. Atmospheric transport is guided by the ERA-Interim meteorological reanalyses (Dee et al., 2011) to represent changes in the dynamics. Given observational information of the three species, the system optimizes the following quantities at each grid cell at a weekly resolution: (i) surface emissions of CH4, (ii) surface emissions of CO, (iii) scaling factors for the sum of HCHO emissions and its chemical production from hydrocarbon oxidation, (iv) scaling factors of the OH concentration, and (v) the initial state of all the four species CH4, HCHO, CO, and OH. The assimilation is performed continuously for the entire study period to avoid errors in temporal segmentation. The minimization of the cost function is solved iteratively until it reaches a reduction of 99 % in the gradient of the cost function or a minimum of 45 iterations. The reduced chi-squared (J divided by the number of observations) is about 0.5, which is much lower than 1 because of observation error inflation to compensate for the fact that we do not account for observation error correlations, following the findings of Chevallier (2007).
2.2.2 Prior estimates of surface methane fluxes and OH fields
We use prior estimates of climatological methane emissions from various sectors except for biomass burning. This choice is made to avoid prior assumptions about the interannual variations (IAVs) or trends in the surface emissions so that IAVs in the posterior fluxes are primarily driven by assimilated observations. The exception made for fire emissions is due to their non-Gaussian distribution and large variations across different seasons and years where the bottom-up estimates based on satellite-derived burned areas bring valuable prior information to guide the solution. The emission datasets from different sectors are listed in Table S2, and their spatial distributions are shown in Fig. S3. Note that soil deposition is treated as negative fluxes from the land to the atmosphere, and the emissions reported in this study are hence the net methane fluxes from the land to the atmosphere. The Gaussian uncertainty is set as 70 % and 100 %, respectively, for gridded CH4 and CO emissions, whereas 200 % for chemical HCHO productions and 20 % for OH. Those errors are chosen empirically given the spreads across different bottom-up estimates. The a priori spatial error correlations are defined by an e-folding length of 500 km over the land and 1000 km over the ocean. Temporal error correlations are defined by an e-folding length of 2 weeks. We do not account for error correlations across species.
We include two alternative prior estimates for the OH concentration: one based on a full chemistry simulation by the model LMDZ-INCA (Hauglustaine, 2004), noted as INCA-OH hereafter, and one from the TransCom model intercomparison experiment for methane and related species (Patra et al., 2011), noted as TransCom-OH. The two OH fields have contrasting 3-D distributions that could help to evaluate the impact of OH distributions on the resultant methane fluxes (Yin et al., 2015). In particular, the two OH fields have different Northern to Southern hemisphere ratios: ∼ 1.2 for INCA and ∼ 1 for TransCom. Similar to the prior estimates of the emissions, there are no interannual variations in the prior estimates of OH fields. Note that for the case of assimilating surface observations (S1), the spatial error correlation of OH is set to 1 within six latitudinal zones (90–60∘ S, 60–30∘ S, 30–0∘ S, 0–30∘ N, 30–60∘ N, and 60–90∘ N) and 0 across them, i.e., the zonal mean OH is optimized instead of per grid cell given limited observational constraints. In summary, we include six inversions here with three different observational constraints and each pairing with two different prior estimates of global OH distributions (Table S1).
2.2.3 Information content analysis
While the variational inverse system has the advantage of optimizing large state vectors of fluxes for multiple species at high spatial and temporal resolutions, it is computationally too expensive to calculate the error covariances of posterior fluxes. Hence, we perform additional analytical inversions for aggregated source regions to estimate the information content of available methane observations on regional emissions and posterior error covariances. In this configuration, the state vector x becomes monthly regional emissions from 18 regions across the globe (regional mask shown in Fig. 8) plus a background term and the impacts of changes in OH are not accounted for. The transport model and observation operator K, relating each element of x to observable quantities y can be numerically simulated. Using xa to represent the prior and Sa and Sε to represent the error covariance matrices of the state vector x and of the observation vector y, the posterior solution is expressed as
where
Here, G represents the gain matrix that describes the sensitivity of the fluxes to observations, i.e., . The error covariance matrix of can be derived as
The ability of an observational system to constrain the true value of the state vector can be represented by the sensitivity of the posterior solution to the true state x, commonly termed as the averaging kernel matrix , as the product of the gain matrix G and the Jacobian matrix , so that A=GK (Rodgers, 2000). This complementary analysis provides us important estimates of how much information content the surface and satellite methane observations can provide on regional methane emission changes.
3.1 Changes in atmospheric methane growth rate
In general, the observed global average methane growth rate is well captured by the posterior model states both at the surface sites and through the total column, irrespective of which data are being assimilated (Fig. 2b and c and Table S4). Sampled from the same ensemble of posterior model states, the surface growth rates show a sharp increase in 2014 (Fig. 2b), whereas more gradual increase is found in the column average (Fig. 2c). The agreement across different inversions demonstrates that differences in the temporal variations of the growth rates seen by surface and GOSAT observations are primarily due to 3-D sampling differences rather than by some inconsistency between those two types of observations. This contrast suggests that the sharp increase in the surface methane growth rate in 2014 could have been amplified by a sampling effect of the sparse surface network as also shown by a longer record (Pandey et al., 2019). Surface in situ observations with high precision and accuracy provide critical anchor points for monitoring the background methane concentrations in the boundary layer, while satellite retrievals are sensitive to the entire atmospheric column filling in continental gaps that are not effectively covered by surface stations. The consistency between the two observation approaches demonstrates a robust constraint on the acceleration of the atmospheric growth rate on the global scale.
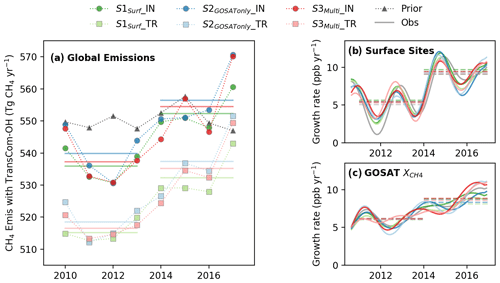
Figure 2Global CH4 emissions and atmospheric growth rates from 2010 to 2017. (a) Surface CH4 fluxes of the prior (black triangles) and posterior estimates (color coded). The circles represent versions using INCA-OH (denoted with IN as suffix), while the squares represent versions using TransCom-OH (denoted with TR as suffix). The horizontal lines mark the average emissions of the two periods, 2010–2013 and 2014–2017. (b) Deseasonalized methane growth rates smoothed for variations shorter than 90 d in the posterior model states sampled at the 103 surface stations included in inversion S1. (c) growth rates in the posterior model states sampled at the measurement time and location of GOSAT retrievals included in inversions S2 and S3.
3.2 Changes in global CH4 emissions
Posterior global CH4 emissions derived from all six inversions show similar interannual variations (IAVs) regardless of which observations are assimilated or which prior OH fields are used (Fig. 2a). As stated in the methods, the prior CH4 emission IAVs only account for fire emissions, while the other emission sectors are represented by climatological means; hence, the IAVs of the posterior emissions are primarily driven by methane observations. Surface and satellite observations derive generally consistent IAV results. The choice of the prior OH fields has a notable effect on the magnitude of the optimized global emissions but not on the inferred temporal changes. Inversions using INCA-OH derives on average 20 ± 1.5 Tg yr−1 higher emissions due to a larger OH sink (higher Northern Hemisphere OH concentrations). Therefore, in this study, we focus primarily on the IAV of methane fluxes that are directly relevant to changes in the methane growth rate while avoiding systematic differences across different inversions.
Global CH4 emissions increased by 17.5 ± 1.5 Tg yr−1 between 2010–2013 and 2014–2017 (the uncertainty range represents the standard deviation of the six inversions throughout this study). On average, the increase amounts to a linear trend of 4.1 ± 1.2 Tg yr−2 over the 8 years, corresponding to a nearly 1% increase per year. The lowest annual total emission occurred in 2012 and the highest in 2017. Current global CH4 emissions are thus at a maximum level within the past million years with high growth rates similar to the 1980s, during which the total methane sink was, however, not as high as today due to a lower CH4 burden.
3.3 Variations attributed to OH
The inversion adjusted surface emission levels given the two different prior OH fields (Fig. 2a), indicating that there is not enough information to constrain the magnitudes of the sources and sinks of the three species separately with their atmospheric observations. Changes in the inferred OH concentrations are less than 1 % on the global scale, with a small increase during 2010–2014 followed by a small decline thereafter (Fig. 3). The resulting decrease in OH since 2014, albeit small in magnitude, occurs in both the surface-driven (S1) and satellite-driven (S3) inversions, most notably in the Southern Hemisphere (Fig. S4). Inflating the prior OH uncertainty up to ±50 % at each model grid only results in larger scaling factors on the OH distribution but not higher temporal variations. The resultant small interannual variations in the posterior OH field are in line with a modeling study that showed a high OH recycling probability and hence a weak sensitivity to emission perturbations (Lelieveld et al., 2016). Some atmospheric chemistry models simulate a slightly larger year-to-year variability (1 %–4 %) (Holmes et al., 2013; Turner et al., 2018), while recent data-constrained estimates using observed ozone columns, water vapor, methane, model-simulated NOx, and Hadley cell width suggest a relatively stable OH level over the past several decades (Nicely et al., 2018). In addition, compared to earlier box model studies that infer around 5 % OH IAV from methyl chloroform (MCF) and δ13CH4 observations (Turner et al., 2017; Rigby et al., 2017), a recent box model study that accounts for model biases related to tracer specific dynamics suggest a smaller IAV in OH (Naus et al., 2019).
Recent GOSAT inverse studies explored optimizing gridded annual anthropogenic methane emissions and associated trends, regional monthly wetland emissions, and global (or hemispheric) annual OH concentration with an analytical inversion scheme, where it is possible to compute the full posterior error covariance matrix (Maasakkers et al., 2019; Zhang et al., 2021). The results suggest a strong negative error correlation between global anthropogenic emissions and methane lifetime (), moderate correlations between wetland emissions and methane lifetime (), and moderate correlations between the OH trend and wetland or anthropogenic emission trends () (Zhang et al., 2021). Hence, assimilating GOSAT data alone, the inversion has limited information to separate the sources and the sinks. With our multi-species variational inverse system, it is computationally too costly to estimate the posterior error covariances using a Monte Carlo approach. Given the strong error correlations between the source and sink terms identified by Zhang et al. (2021), we cannot rule out the possibility that numerically it might be easier for the optimization system to adjust surface emissions of the three species to fit the observations rather than modifying OH to adjust the sink terms in the absence of mechanistic chemical feedback in the chemical transport model. The feedback effects are mostly tested using box models at the current stage (Prather, 1994; Nguyen et al., 2020); future studies accounting for these effects in a 3-D inversion would be helpful to diagnose its impacts on estimated changes in methane lifetimes.
4.1 Changes in zonal CH4 emissions
Similar zonal emission increases between 2010–2013 and 2014–2017 are found across the six inversions (Fig. 4a), even though they produce different latitudinal distributions of CH4 fluxes (Fig. 4b). Both satellite and surface data suggest that the largest increase occurred in the southern tropics (0–30∘ S, 7.5 ± 2.1 Tg yr−1) and the northern mid-latitudes (30–60∘ N, 6.5 ± 0.8 Tg yr−1), while a moderate increase is found in the northern high latitudes (60–90∘ N, 1.3 ± 0.5 Tg yr−1). For the northern tropics (0–30∘ N), most versions suggest a small increase, but one version assimilating surface data suggests a small decline. Different versions agree on the overall spatial distribution of the inferred emission trends, with the most significant increase seen in East China, tropical South America, tropical Africa, and Russia (Fig. 5). Opposing trends are noted in former Indochina and Southeast Asia that result in more divergent estimates across the different inversions in the 0–30∘ N zone.
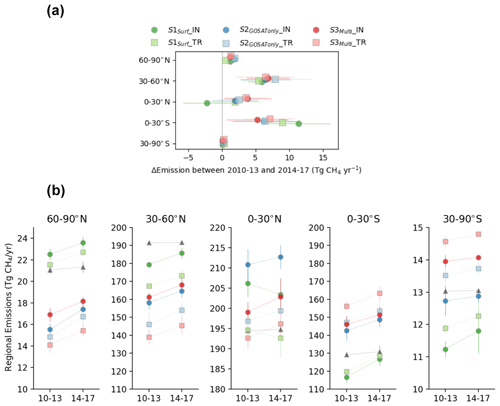
Figure 4(a) Emission change between 2010–2013 and 2014–2017 in the five latitudinal zones. The error bars represent the standard deviation of changes in CH4 fluxes between the two periods. (b) Zonal fluxes estimated by different versions for the period 2010–2013 and 2014–2017. The mean values for each 4-year period are shown and errors bars represent their 1σ standard deviations.
Differences of zonal flux distributions are noted across versions, most notably between surface and satellite data constraints. For the same observational constraints, inversions using INCA OH fields result in higher Northern Hemisphere emissions compared to the cases using TransCom OH fields due to a higher north-to-south hemispheric OH ratio of the former. Compared to the results assimilating surface observations (S1), assimilating GOSAT X retrievals (S2 and S3) allocates smaller emissions in the northern mid- and high latitudes (30–60 and 60–90∘ N) but higher emissions in the tropics and subtropics (0–30∘ N and 0–30∘ S) (Fig. 4b). Such difference is, to a large extent, related to a latitudinal-dependent difference between model states that fit surface data and that fit GOSAT data. Specifically, the posterior model states of S1 that fit surface observations show positive biases against GOSAT X in the northern mid- and high latitudes but negative ones in the tropics (Fig. S5). Symmetrically, the posterior model states of S2 and S3, which fit GOSAT X well, show negative biases in the northern mid- and high latitudes against surface observations (Fig. S7), while the biases turn positive gradually toward the tropics and the Southern Hemisphere (Fig. S16). However, no latitude-dependent biases are found between GOSAT-assimilated posterior model states (S2 and S3) against TCCON total column measurements, and the magnitude of remaining biases are in line with GOSAT data validation (Parker et al., 2015). Yet S1 shows similar model bias structure against TCCON as compared to GOSAT X (Fig. S7), suggesting discrepancies in the vertical distribution of methane concentrations between the model and the satellite retrievals. Such a bias pattern between model and surface or GOSAT data has been identified by previous inverse studies (Alexe et al., 2015; Turner et al., 2015; Miller et al., 2019; Maasakkers et al., 2019), which is likely related to biases in the model representation of the stratosphere. An empirical bias correction on the GOSAT data so that the assimilated model states also agree with surface observations are typically applied by some studies. Here, since we focus on the IAVs of the posterior fluxes where systematic biases do not impact such results, we did not apply an empirical bias correction to the GOSAT data. Future studies to correct those biases with mechanistic understandings will be very valuable.
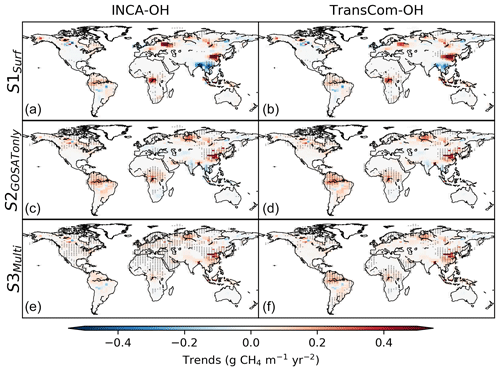
Figure 5Spatial distribution of trends in the posterior CH4 emissions from 2010 to 2017. Panels (a), (c), and (e) show results using INCA-OH and panels ((b), (d), (f) use TransCom-OH. Each row represents one type of observational constraint. The black crosses denote trends that are statistically significant at a 95 % confidence level.
4.2 Information content of observations on regional fluxes
To assess the extent to which the surface and satellite observations can inform us about changes of methane fluxes in distinct regions, we conducted an information content analysis for a total of 18 regions (see Sect. 2.2.3). The regional mask following the convention of the Global Carbon Project (Saunois et al., 2019) is shown in Fig. 8. Note that this analysis assumes all atmospheric methane changes result from surface flux changes and hence does not account for potential contributions from changes in OH or other sink processes. The results suggest that in most cases GOSAT data provide more constraints on regional emissions than the surface observations (Fig. 6). This is particularly obvious in the tropics and subtropics, including the Amazon, eastern Brazil, southern South America, northern Africa, tropical Africa, southern Africa, the Mideast, India, and Southeast Asia. This is because fewer surface sites exist in those regions but satellite data have a better coverage. Consequently, the posterior errors in the optimized emissions constrained by satellite data are less correlated across different regions compared to the case with surface data constraints only (Fig. S8). The error covariances suggest that the surface observations alone, mostly located in the background boundary layer, is insufficient to separate tropical emissions from the three continents – South America, Africa, and Asia. In contrast, the cross-error terms in the GOSAT inversion are much smaller, suggesting that to a large extent emissions from different regions can be individually constrained by these X observations.
4.3 Regional emission changes
Looking into regional emission changes, the differences in the posterior CH4 emissions between the last and the first 4 years of our study period (2014–2017 vs. 2010–2013) are shown in Fig. 7, while regional masks of the 18 sub-regions and regional annual emission anomalies are shown in Fig. 8. The most substantial increases between the two periods occurred in the Amazon, China, and tropical Africa, by 4.2 ± 1.2, 3.7 ± 1.0, and 2.1 ± 0.8 Tg yr−1, respectively (Fig. 7). Changes in the three regions amounts to nearly 60 % of the global emission increase. This increase does not necessarily imply linear trends in emissions as there are considerable interannual variations in the derived emissions (Fig. 8); in particular, the first period includes a La Niña year (2011) during which high wetland emissions are reported (Pandey et al., 2017) and the latter period includes a strong El Niño year (2015) during which large fire emissions are reported (Yin et al., 2016; Worden et al., 2017). While all the six inversions agree on such a regional pattern, the multi-tracer versions (S3) that optimize OH concentrations simultaneously with the surface methane fluxes infer smaller CH4 emission increases compared to the version assimilating GOSAT alone (S2). This difference could stem from adapting the regional mean OH level that converts the same concentration change to different emission changes. In addition, differences between S2 and S3 could result from the variational inversion reaching different approximations of the cost function minimum.
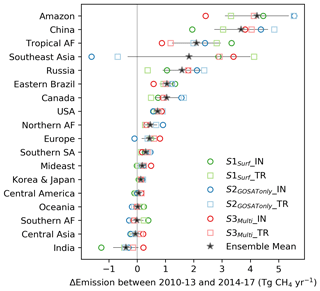
Figure 7Regional emission changes between 2010–2013 and 2014–2017 ranked from the highest to the smallest changes. The color-coded markers represent individual inversions, the gray stars represent the ensemble mean, and the horizontal error bars denote the standard deviation of all versions. The regional mask is shown in Fig. 8.
To gain further understanding of observed changes in regional CH4 emissions, we attribute our inversion emission anomaly estimates into the following categories based on our prior bottom-up emission inventory: fossil fuel (oil, gas, coal mining, industry, residential, transport, and geological), waste (landfills and wastewater), agriculture (enteric fermentation, manure management, and rice cultivation), wetlands (including inland water), and fire (including biofuel). We acknowledge the fact that this prior information has significant uncertainties as evidenced by the large spread across different bottom-up inventories (Saunois et al., 2016). The proportion of the different sectors remains unchanged in each grid cell throughout all years, except for fire, because we use a climatological estimates for prior emissions. Our emission attribution thus reflects a likelihood of contributing processes at a given location and season, which is larger and most useful in regions where emissions are predominately contributed by a specific sector (Figs. S9 and S10).
For the Amazon, wetlands are the major contributor to CH4 emissions according to the bottom-up emission inventories and hence our identified source for the increase, showing an average trend of 0.8 ± 0.1 Tg yr−2 over the 8 study years with shorter-term interannual variations (Fig. 8). Fire emissions from this region were high during the 2010 drought but did not rise significantly in the recent 2015 El Niño, which is in agreement with previous fire emission estimates based on CO and CO2 (Gatti et al., 2014; Liu et al., 2017). No significant trend in the anthropogenic emissions are noted up to 2014 according to the most recent updates from the Community Emissions Data System (Hoesly et al., 2018) (Fig. S11). Our inferred wetland emissions in the 2011 La Niña show the highest positive anomaly in the 2010–2013 period, consistent with previous estimates covering this particular period (Pandey et al., 2017). Wetland methane emissions come from anaerobic degradation of organic matter and hence depend on organic carbon inputs and inundation areas and exponentially on temperature (Whalen, 2005). Consistent behaviors between the time and locations of anomalies in the GOSAT X and changes in wetland extent have been documented with the focus on seasonally flooded wetlands (Parker et al., 2018). An intensification of Amazon flooding extremes has been documented based on water levels in the Amazon river, with anomalously high flood levels and long flood durations since 2012 (Barichivich et al., 2018), which could result in higher wetland CH4 emissions.
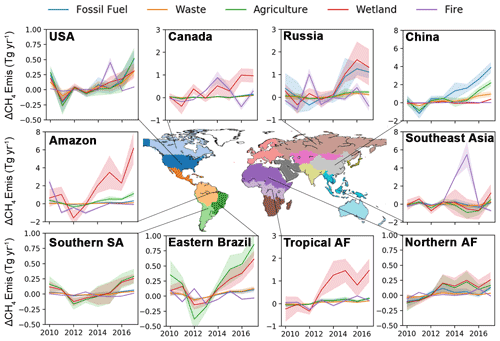
Figure 8Regional emission anomalies relative to the 2010–2013 mean for the sectoral attribution based on prior information. Note the scales on the y axis are different for each subplot.
For the other tropical regions, significant increases are also attributed to wetland emissions, in particular to tropical Africa (1.5 ± 0.7 Tg yr−1; Fig. 8). The increasing tropical Africa wetland emissions are consistent with a recent regional inversion using GOSAT data at a high spatial resolution of 0.5∘ × 0.625∘, which finds a positive trend of 1.5–2.1 Tg yr−2 in the region from 2010 to 2016, mainly from wetlands in the Sudd in South Sudan (Lunt et al., 2019). Smaller wetland emission increases are found in the other tropical regions including eastern Brazil (0.3 ± 0.1 Tg yr−1), northern Africa (0.2 ± 0.1 Tg yr−1), and southern South America (0.1 ± 0.1 Tg yr−1). However, other emission sources also play a significant role in these regions, in particular agricultural emissions (Chang et al., 2019). Thus future studies with additional constraints on wetland emissions are needed to better quantify wetland-related changes. Only in Southeast Asia, is the major contribution to different CH4 emissions between the two periods from fire associated with the strong El Niño in 2015 (Yin et al., 2016; Liu et al., 2017). No significant increases are noted for India, consistent with a previous regional study focusing on the 2010–2015 period (Ganesan et al., 2017).
The sectoral breakdown of emissions from China suggests a substantial increase in anthropogenic sources from fossil fuels, agriculture, and waste, adding up to an overall trend of 1.0 ± 0.2 Tg yr−2 between 2010 and 2017 (Fig. 8). As stated above, this attribution relies on the relative contribution of different sectors from the prior information and does not account for structural changes in time. A recent inverse study focusing on Asian emissions from 2010 to 2015 derived nearly the same magnitude of emission trend for China (Miller et al., 2019); a continued increase is confirmed here beyond 2015 until the end of the record in 2017. In contrast, a global inversion that uses a different prior emissions estimate and separates the mean anthropogenic emissions and trends in the state vector found a smaller trend in anthropogenic emissions over China (0.39 ± 0.27 Tg yr−2) for the period 2010–2018 and a trend of 0.72 ± 0.39 Tg yr−2 focusing on the period 2010–2016 (Zhang et al., 2021). The numbers are comparable given the differences in the inverse setups and the chemical transport models being used.
Russia also contributed significant increase in CH4 emissions by 1.7 ± 0.7 Tg yr−1 between 2010–2013 and 2014–2017 (Fig. 7), possibly from both fossil fuel extraction in northern Russia and extensive peatland areas (Fig. 8). The surface-driven and satellite-driven inversions identify slightly different source regions for the rise (Fig. 5). The surface-driven inversions attribute most of the increases to the European part of Russia where anthropogenic emissions dominate, whereas the satellite-driven inversions attribute more changes to the West Siberian Plain where more wetlands are located (Terentieva et al., 2016). As there are both fossil fuel and wetland sources in the West Siberian Plain (Fig. S10), further information is needed to disentangle relative contributions between anthropogenic and natural wetland sources. For the other extratropical regions showing significant CH4 emission increases, the increase in Canada (1.1 ± 0.4 Tg yr−1) was mostly attributed to wetlands (Fig. 8), with interannual variations consistent with previous regional inversions (Sheng et al., 2018). A relatively small increase in the US is found after 2014 (0.7 ± 0.2 Tg yr−1) with nearly flat emissions before, which is consistent with previous studies finding no trend over the US before 2012 (Saunois et al., 2017; Bruhwiler et al., 2017).
Relying on the prior distribution to approximate possible contributions from wetlands in the mid- and high latitudes, the increase between 2010–2013 and 2014–2017 amounts to 0.9 ± 0.5, 0.6 ± 0.4, and 0.1 ± 0.06 Tg yr−1 for Russia, Canada, and the US. Up to 2012, high-latitude wetland emissions are not identified as significant contributors to increasing atmospheric methane (Saunois et al., 2017). The positive trend in high-latitude wetland emissions found here could be the first sign of the impact of the fast warming observed at these latitudes. Adding up all wetland contributions across the globe, changes in wetland emissions dominate the interannual variations in the emission anomaly (Fig. S12a). The general increase in wetland CH4 fluxes is in line with observed atmospheric δ13CH4 that shows a general negative trend at all latitudes (Fig. S12b), as biogenic sources like wetlands are more δ13CH4 depleted than the other ones (Sherwood et al., 2017). In addition, anthropogenic emissions from agriculture and waste management are also associated with a biogenic δ13CH4 signature.
Our ensemble of inversions assimilating surface or satellite CH4 observations, as well as chemically related species to partly constrain the OH sink, suggests that the recent acceleration in CH4 growth rate from 2010 to 2017 is most likely induced by increases in methane emissions. The derived global emissions point to an unprecedented new maximum in global total methane emissions. The most substantial increases during the 8 study years come from the tropics and East Asia. Given our prior knowledge on the distribution of different CH4 sources, natural wetland emissions show the largest increase with dominant contributions from the tropics. Such an increase would result in potential positive feedback to climate warming (Zhang et al., 2017). The second-largest increase comes from anthropogenic emissions in China. The continuation of existing surface CH4 and δ13CH4 observations and GOSAT/GOSAT-2 X retrievals, the newly available TROPOspheric Monitoring Instrument (TROPOMI) observations with frequent global mapping capability (Hu et al., 2018), and the new methane space missions such as the MEthane Remote sensing Lidar missioN (MERLIN) (Bousquet et al., 2018) will bring further insight into regional methane budget changes and their climate sensitivity. Here, we tested the consistency of using different observational constraints and different prior OH distributions. The sensitivity of prior emission estimates, associated error characteristics, and transport model errors could be further explored by model intercomparison studies through community effort such as Patra et al. (2011). Future studies using spatial–temporal variations in the observed atmospheric δ13CH4 and spatially resolved isotopic source signatures (Ganesan et al., 2018) will provide further constraints on the source attribution. At the same time, a process-based understanding of the wetland CH4 emissions and effective anthropogenic emission regulation measures are urgently needed to meet climate mitigation goals.
LMDz-PYVAR global inversion results presented in this study are available for download from the Caltech Data Center (https://doi.org/10.22002/D1.2079, Yin, 2021).
The supplement related to this article is available online at: https://doi.org/10.5194/acp-21-12631-2021-supplement.
YY and FC designed the study. YY implemented changes to the inverse system and performed the analysis. The manuscript was written by YY and revised and discussed by all coauthors.
The authors declare that they have no conflict of interest.
Publisher’s note: Copernicus Publications remains neutral with regard to jurisdictional claims in published maps and institutional affiliations.
Part of this research was carried out at the Jet Propulsion Laboratory, California Institute of Technology, under a contract with the National Aeronautics and Space Administration. We acknowledge the University of Leicester for the GOSAT X retrievals, the NCAR MOPITT group for the CO retrievals, and the Goddard Earth Sciences Data and Information Services Center for the SAO OMI HCHO retrievals. We thank the WDCGG, NOAA, AGAGE, and TCCON archives for publishing the ground-based observations and we are very grateful to all the people involved in maintaining the networks and archiving the data. Specifically, we acknowledge the following networks for making the measurements available: NOAA, CSIRO, ECCC, AGAGE, JMA, UBAG, NIWA, LSCE, MGO, DMC, Empa, FMI, KMA, RSE, SAWS, UMLT, UNIURB, and VNMHA. The Mace Head, Trinidad Head, Ragged Point, Cape Matatula, and Cape Grim AGAGE stations are supported by the National Aeronautics and Space Administration (NASA) (grants NNX16AC98G to MIT, and NNX16AC97G and NNX16AC96G to SIO). Support also comes from the UK Department for Business, Energy & Industrial Strategy (BEIS) for Mace Head, the National Oceanic and Atmospheric Administration (NOAA) for Barbados, and the Commonwealth Scientific and Industrial Research Organisation (CSIRO) and the Bureau of Meteorology (Australia) for Cape Grim. RJP is funded via the UK National Centre for Earth Observation (NE/N018079/1). This research used the ALICE high-performance computing facility at the University of Leicester for the GOSAT retrievals. We thank the Japanese Aerospace Exploration Agency, National Institute for Environmental Studies, and the Ministry of the Environment for the GOSAT data and their continuous support as part of the joint research agreement. We also thank François Marabelle and the LSCE IT team for computing support at LSCE.
This research is supported by NASA ROSES IDS (grant no. 80NM0018F0583), the NASA Carbon Monitoring System (grant no. 80NM0018F0583), and a grant from the Grantham Foundation. This work also benefited from HPC resources from GENCI-TGCC (grant no. 2018-A0050102201).
This paper was edited by Bryan N. Duncan and reviewed by two anonymous referees.
Alexe, M., Bergamaschi, P., Segers, A., Detmers, R., Butz, A., Hasekamp, O., Guerlet, S., Parker, R., Boesch, H., Frankenberg, C., Scheepmaker, R. A., Dlugokencky, E., Sweeney, C., Wofsy, S. C., and Kort, E. A.: Inverse modelling of CH4 emissions for 2010–2011 using different satellite retrieval products from GOSAT and SCIAMACHY, Atmos. Chem. Phys., 15, 113–133, https://doi.org/10.5194/acp-15-113-2015, 2015. a, b
Barichivich, J., Gloor, E., Peylin, P., Brienen, R. J. W., Schöngart, J., Espinoza, J. C., and Pattnayak, K. C.: Recent intensification of Amazon flooding extremes driven by strengthened Walker circulation, Science Advances, 4, eaat8785, https://doi.org/10.1126/sciadv.aat8785, 2018. a
Bousquet, P., Pierangelo, C., Bacour, C., Marshall, J., Peylin, P., Ayar, P. V., Ehret, G., Bréon, F.-M., Chevallier, F., Crevoisier, C., Gibert, F., Rairoux, P., Kiemle, C., Armante, R., Bès, C., Cassé, V., Chinaud, J., Chomette, O., Delahaye, T., Edouart, D., Estève, F., Fix, A., Friker, A., Klonecki, A., Wirth, M., Alpers, M., and Millet, B.: Error Budget of the MEthane Remote LIdar missioN and Its Impact on the Uncertainties of the Global Methane Budget, J. Geophys. Res.-Atmos., 123, 11766–11785, https://doi.org/10.1029/2018JD028907, 2018. a
Bruhwiler, L. M., Basu, S., Bergamaschi, P., Bousquet, P., Dlugokencky, E., Houweling, S., Ishizawa, M., Kim, H.-S., Locatelli, R., Maksyutov, S., Montzka, S., Pandey, S., Patra, P. K., Petron, G., Saunois, M., Sweeney, C., Schwietzke, S., Tans, P., and Weatherhead, E. C.: U.S. CH4 emissions from oil and gas production: Have recent large increases been detected?, J. Geophys. Res.-Atmos., 122, 4070–4083, https://doi.org/10.1002/2016JD026157, 2017. a
Buchwitz, M., Reuter, M., Schneising, O., Hewson, W., Detmers, R., Boesch, H., Hasekamp, O., Aben, I., Bovensmann, H., Burrows, J., Butz, A., Chevallier, F., Dils, B., Frankenberg, C., Heymann, J., Lichtenberg, G., De Mazière, M., Notholt, J., Parker, R., Warneke, T., Zehner, C., Griffith, D., Deutscher, N., Kuze, A., Suto, H., and Wunch, D.: Global satellite observations of column-averaged carbon dioxide and methane: The GHG-CCI XCO2 and XCH4 CRDP3 data set, Remote Sens. Environ., 203, 276–295, https://doi.org/10.1016/J.RSE.2016.12.027, 2017. a, b
Butz, A., Guerlet, S., Hasekamp, O., Schepers, D., Galli, A., Aben, I., Frankenberg, C., Hartmann, J.-M., Tran, H., Kuze, A., Keppel-Aleks, G., Toon, G., Wunch, D., Wennberg, P., Deutscher, N., Griffith, D., Macatangay, R., Messerschmidt, J., Notholt, J., and Warneke, T.: Toward accurate CO2 and CH4 observations from GOSAT, Geophys. Res. Lett., 38, L14812, https://doi.org/10.1029/2011GL047888, 2011. a
Chang, J., Peng, S., Ciais, P., Saunois, M., Dangal, S. R., Herrero, M., Havlík, P., Tian, H., and Bousquet, P.: Revisiting enteric methane emissions from domestic ruminants and their δ13C source signature, Nat. Commun., 10, 1–14, https://doi.org/10.1038/s41467-019-11066-3, 2019. a
Chevallier, F.: Impact of correlated observation errors on inverted CO2 surface fluxes from OCO measurements, Geophys. Res. Lett., 34, L24804, https://doi.org/10.1029/2007GL030463, 2007. a
Chevallier, F., Fisher, M., Peylin, P., Serrar, S., Bousquet, P., Bréon, F. M., Chédin, A., and Ciais, P.: Inferring CO2 sources and sinks from satellite observations: Method and application to TOVS data, J. Geophys. Res., 110, D24309, https://doi.org/10.1029/2005JD006390, 2005. a, b, c
Chevallier, F., Ciais, P., Conway, T. J., Aalto, T., Anderson, B. E., Bousquet, P., Brunke, E. G., Ciattaglia, L., Esaki, Y., Fröhlich, M., Gomez, A., Gomez-Pelaez, A. J., Haszpra, L., Krummel, P. B., Langenfelds, R. L., Leuenberger, M., Machida, T., Maignan, F., Matsueda, H., Morguí, J. A., Mukai, H., Nakazawa, T., Peylin, P., Ramonet, M., Rivier, L., Sawa, Y., Schmidt, M., Steele, L. P., Vay, S. A., Vermeulen, A. T., Wofsy, S., and Worthy, D.: CO2 surface fluxes at grid point scale estimated from a global 21 year reanalysis of atmospheric measurements, J. Geophys. Res., 115, D21307, https://doi.org/10.1029/2010JD013887, 2010. a
Cressot, C., Chevallier, F., Bousquet, P., Crevoisier, C., Dlugokencky, E. J., Fortems-Cheiney, A., Frankenberg, C., Parker, R., Pison, I., Scheepmaker, R. A., Montzka, S. A., Krummel, P. B., Steele, L. P., and Langenfelds, R. L.: On the consistency between global and regional methane emissions inferred from SCIAMACHY, TANSO-FTS, IASI and surface measurements, Atmos. Chem. Phys., 14, 577–592, https://doi.org/10.5194/acp-14-577-2014, 2014. a, b, c
Dee, D. P., Uppala, S. M., Simmons, A. J., Berrisford, P., Poli, P., Kobayashi, S., Andrae, U., Balmaseda, M. A., Balsamo, G., Bauer, P., Bechtold, P., Beljaars, A. C. M., van de Berg, L., Bidlot, J., Bormann, N., Delsol, C., Dragani, R., Fuentes, M., Geer, A. J., Haimberger, L., Healy, S. B., Hersbach, H., Hólm, E. V., Isaksen, L., Kållberg, P., Köhler, M., Matricardi, M., McNally, A. P., Monge-Sanz, B. M., Morcrette, J.-J., Park, B.-K., Peubey, C., de Rosnay, P., Tavolato, C., Thépaut, J.-N., and Vitart, F.: The ERA-Interim reanalysis: configuration and performance of the data assimilation system, Q. J. Roy. Meteor. Soc., 137, 553–597, https://doi.org/10.1002/qj.828, 2011. a
Deeter, M. N., Martínez-Alonso, S., Edwards, D. P., Emmons, L. K., Gille, J. C., Worden, H. M., Sweeney, C., Pittman, J. V., Daube, B. C., and Wofsy, S. C.: The MOPITT Version 6 product: algorithm enhancements and validation, Atmos. Meas. Tech., 7, 3623–3632, https://doi.org/10.5194/amt-7-3623-2014, 2014. a
Deeter, M. N., Edwards, D. P., Francis, G. L., Gille, J. C., Martínez-Alonso, S., Worden, H. M., and Sweeney, C.: A climate-scale satellite record for carbon monoxide: the MOPITT Version 7 product, Atmos. Meas. Tech., 10, 2533–2555, https://doi.org/10.5194/amt-10-2533-2017, 2017. a, b
Dlugokencky, E. J., Masarie, K. A., Lang, P. M., and Tans, P. P.: Continuing decline in the growth rate of the atmospheric methane burden, Nature, 393, 447–450, https://doi.org/10.1038/30934, 1998. a
Dlugokencky, E. J., Houweling, S., Bruhwiler, L., Masarie, K. A., Lang, P. M., Miller, J. B., and Tans, P. P.: Atmospheric methane levels off: Temporary pause or a new steady-state?, Geophys. Res. Lett., 30, 1992, https://doi.org/10.1029/2003GL018126, 2003. a
Dlugokencky, E. J., Bruhwiler, L., White, J. W. C., Emmons, L. K., Novelli, P. C., Montzka, S. A., Masarie, K. A., Lang, P. M., Crotwell, A. M., Miller, J. B., and Gatti, L. V.: Observational constraints on recent increases in the atmospheric CH4 burden, Geophys. Res. Lett., 36, L18803, https://doi.org/10.1029/2009GL039780, 2009. a
Etheridge, D. M., Steele, L. P., Francey, R. J., and Langenfelds, R. L.: Atmospheric methane between 1000 A.D. and present: Evidence of anthropogenic emissions and climatic variability, J. Geophys. Res.-Atmos., 103, 15979–15993, https://doi.org/10.1029/98JD00923, 1998. a
Fletcher, S. E. M. and Schaefer, H.: Rising methane: A new climate challenge, Science, 364, 932–933, https://doi.org/10.1126/science.aax1828, 2019. a
Fortems-Cheiney, A., Chevallier, F., Pison, I., Bousquet, P., Saunois, M., Szopa, S., Cressot, C., Kurosu, T. P., Chance, K., and Fried, A.: The formaldehyde budget as seen by a global-scale multi-constraint and multi-species inversion system, Atmos. Chem. Phys., 12, 6699–6721, https://doi.org/10.5194/acp-12-6699-2012, 2012. a, b, c
Ganesan, A. L., Rigby, M., Lunt, M. F., Parker, R. J., Boesch, H., Goulding, N., Umezawa, T., Zahn, A., Chatterjee, A., Prinn, R. G., Tiwari, Y. K., van der Schoot, M., and Krummel, P. B.: Atmospheric observations show accurate reporting and little growth in India's methane emissions, Nat. Commun., 8, 836, https://doi.org/10.1038/s41467-017-00994-7, 2017. a, b
Ganesan, A. L., Stell, A. C., Gedney, N., Comyn-Platt, E., Hayman, G., Rigby, M., Poulter, B., and Hornibrook, E. R. C.: Spatially Resolved Isotopic Source Signatures of Wetland Methane Emissions, Geophys. Res. Lett., 45, 3737–3745, https://doi.org/10.1002/2018GL077536, 2018. a
Gatti, L. V., Gloor, M., Miller, J. B., Doughty, C. E., Malhi, Y., Domingues, L. G., Basso, L. S., Martinewski, A., Correia, C. S. C., Borges, V. F., Freitas, S., Braz, R., Anderson, L. O., Rocha, H., Grace, J., Phillips, O. L., and Lloyd, J.: Drought sensitivity of Amazonian carbon balance revealed by atmospheric measurements, Nature, 506, 76–80, https://doi.org/10.1038/nature12957, 2014. a
Gaubert, B., Worden, H. M., Arellano, A. F. J., Emmons, L. K., Tilmes, S., Barré, J., Martinez Alonso, S., Vitt, F., Anderson, J. L., Alkemade, F., Houweling, S., and Edwards, D. P.: Chemical Feedback From Decreasing Carbon Monoxide Emissions, Geophys. Res. Lett., 44, 9985–9995, https://doi.org/10.1002/2017GL074987, 2017. a
González Abad, G., Vasilkov, A., Seftor, C., Liu, X., and Chance, K.: Smithsonian Astrophysical Observatory Ozone Mapping and Profiler Suite (SAO OMPS) formaldehyde retrieval, Atmos. Meas. Tech., 9, 2797–2812, https://doi.org/10.5194/amt-9-2797-2016, 2016. a, b, c
Hauglustaine, D. A.: Interactive chemistry in the Laboratoire de Météorologie Dynamique general circulation model: Description and background tropospheric chemistry evaluation, J. Geophys. Res., 109, D04314, https://doi.org/10.1029/2003JD003957, 2004. a, b
Hausmann, P., Sussmann, R., and Smale, D.: Contribution of oil and natural gas production to renewed increase in atmospheric methane (2007–2014): top–down estimate from ethane and methane column observations, Atmos. Chem. Phys., 16, 3227–3244, https://doi.org/10.5194/acp-16-3227-2016, 2016. a
Hoesly, R. M., Smith, S. J., Feng, L., Klimont, Z., Janssens-Maenhout, G., Pitkanen, T., Seibert, J. J., Vu, L., Andres, R. J., Bolt, R. M., Bond, T. C., Dawidowski, L., Kholod, N., Kurokawa, J.-I., Li, M., Liu, L., Lu, Z., Moura, M. C. P., O'Rourke, P. R., and Zhang, Q.: Historical (1750–2014) anthropogenic emissions of reactive gases and aerosols from the Community Emissions Data System (CEDS), Geosci. Model Dev., 11, 369–408, https://doi.org/10.5194/gmd-11-369-2018, 2018. a
Holmes, C. D., Prather, M. J., Søvde, O. A., and Myhre, G.: Future methane, hydroxyl, and their uncertainties: key climate and emission parameters for future predictions, Atmos. Chem. Phys., 13, 285–302, https://doi.org/10.5194/acp-13-285-2013, 2013. a
Hourdin, F., Grandpeix, J.-Y., Rio, C., Bony, S., Jam, A., Cheruy, F., Rochetin, N., Fairhead, L., Idelkadi, A., Musat, I., Dufresne, J.-L., Lahellec, A., Lefebvre, M.-P., and Roehrig, R.: LMDZ5B: the atmospheric component of the IPSL climate model with revisited parameterizations for clouds and convection, Clim. Dynam., 40, 2193–2222, https://doi.org/10.1007/s00382-012-1343-y, 2013. a
Houweling, S., Bergamaschi, P., Chevallier, F., Heimann, M., Kaminski, T., Krol, M., Michalak, A. M., and Patra, P.: Global inverse modeling of CH4 sources and sinks: an overview of methods, Atmos. Chem. Phys., 17, 235–256, https://doi.org/10.5194/acp-17-235-2017, 2017. a
Hu, H., Landgraf, J., Detmers, R., Borsdorff, T., Aan de Brugh, J., Aben, I., Butz, A., and Hasekamp, O.: Toward Global Mapping of Methane With TROPOMI: First Results and Intersatellite Comparison to GOSAT, Geophys. Res. Lett., 45, 3682–3689, https://doi.org/10.1002/2018GL077259, 2018. a
IPCC: Climate Change 2013. The Physical Science Basis, Tech. rep., Intergovernmental Panel on Climate Change-IPCC, 2013. a
Jacob, D. J., Turner, A. J., Maasakkers, J. D., Sheng, J., Sun, K., Liu, X., Chance, K., Aben, I., McKeever, J., and Frankenberg, C.: Satellite observations of atmospheric methane and their value for quantifying methane emissions, Atmos. Chem. Phys., 16, 14371–14396, https://doi.org/10.5194/acp-16-14371-2016, 2016. a
Kirschke, S., Bousquet, P., Ciais, P., Saunois, M., Canadell, J. G., Dlugokencky, E. J., Bergamaschi, P., Bergmann, D., Blake, D. R., Bruhwiler, L., Cameron-Smith, P., Castaldi, S., Chevallier, F., Feng, L., Fraser, A., Heimann, M., Hodson, E. L., Houweling, S., Josse, B., Fraser, P. J., Krummel, P. B., Lamarque, J.-F., Langenfelds, R. L., Le Quéré, C., Naik, V., O'Doherty, S., Palmer, P. I., Pison, I., Plummer, D., Poulter, B., Prinn, R. G., Rigby, M., Ringeval, B., Santini, M., Schmidt, M., Shindell, D. T., Simpson, I. J., Spahni, R., Steele, L. P., Strode, S. A., Sudo, K., Szopa, S., van der Werf, G. R., Voulgarakis, A., van Weele, M., Weiss, R. F., Williams, J. E., and Zeng, G.: Three decades of global methane sources and sinks, Nature Publishing Group, 6, 813–823, https://doi.org/10.1038/ngeo1955, 2013. a
Kuze, A., Suto, H., Nakajima, M., and Hamazaki, T.: Thermal and near infrared sensor for carbon observation Fourier-transform spectrometer on the Greenhouse Gases Observing Satellite for greenhouse gases monitoring, Appl. Opt., 48, 6716–6733, https://doi.org/10.1364/AO.48.006716, 2009. a
Kuze, A., Suto, H., Shiomi, K., Kawakami, S., Tanaka, M., Ueda, Y., Deguchi, A., Yoshida, J., Yamamoto, Y., Kataoka, F., Taylor, T. E., and Buijs, H. L.: Update on GOSAT TANSO-FTS performance, operations, and data products after more than 6 years in space, Atmos. Meas. Tech., 9, 2445–2461, https://doi.org/10.5194/amt-9-2445-2016, 2016. a
Lelieveld, J., Gromov, S., Pozzer, A., and Taraborrelli, D.: Global tropospheric hydroxyl distribution, budget and reactivity, Atmos. Chem. Phys., 16, 12477–12493, https://doi.org/10.5194/acp-16-12477-2016, 2016. a
Liu, J., Bowman, K. W., Schimel, D. S., Parazoo, N. C., Jiang, Z., Lee, M., Bloom, A. A., Wunch, D., Frankenberg, C., Sun, Y., O'Dell, C. W., Gurney, K. R., Menemenlis, D., Gierach, M., Crisp, D., and Eldering, A.: Contrasting carbon cycle responses of the tropical continents to the 2015–2016 El Niño, Science, 358, eaam5690, https://doi.org/10.1126/science.aam5690, 2017. a, b
Locatelli, R., Bousquet, P., Saunois, M., Chevallier, F., and Cressot, C.: Sensitivity of the recent methane budget to LMDz sub-grid-scale physical parameterizations, Atmos. Chem. Phys., 15, 9765–9780, https://doi.org/10.5194/acp-15-9765-2015, 2015. a
Lunt, M. F., Palmer, P. I., Feng, L., Taylor, C. M., Boesch, H., and Parker, R. J.: An increase in methane emissions from tropical Africa between 2010 and 2016 inferred from satellite data, Atmos. Chem. Phys., 19, 14721–14740, https://doi.org/10.5194/acp-19-14721-2019, 2019. a
Maasakkers, J. D., Jacob, D. J., Sulprizio, M. P., Scarpelli, T. R., Nesser, H., Sheng, J.-X., Zhang, Y., Hersher, M., Bloom, A. A., Bowman, K. W., Worden, J. R., Janssens-Maenhout, G., and Parker, R. J.: Global distribution of methane emissions, emission trends, and OH concentrations and trends inferred from an inversion of GOSAT satellite data for 2010–2015, Atmos. Chem. Phys., 19, 7859–7881, https://doi.org/10.5194/acp-19-7859-2019, 2019. a, b, c
McNorton, J., Wilson, C., Gloor, M., Parker, R. J., Boesch, H., Feng, W., Hossaini, R., and Chipperfield, M. P.: Attribution of recent increases in atmospheric methane through 3-D inverse modelling, Atmos. Chem. Phys., 18, 18149–18168, https://doi.org/10.5194/acp-18-18149-2018, 2018. a
Miller, S. M., Michalak, A. M., Detmers, R. G., Hasekamp, O. P., Bruhwiler, L. M. P., and Schwietzke, S.: China's coal mine methane regulations have not curbed growing emissions, Nat. Commun., 10, 303, https://doi.org/10.1038/s41467-018-07891-7, 2019. a, b, c
Monteil, G., Houweling, S., Butz, A., Guerlet, S., Schepers, D., Hasekamp, O., Frankenberg, C., Scheepmaker, R., Aben, I., and Röckmann, T.: Comparison of CH4 inversions based on 15 months of GOSAT and SCIAMACHY observations, J. Geophys. Res.-Atmos., 118, 11807–11823, https://doi.org/10.1002/2013JD019760, 2013. a
Naik, V., Voulgarakis, A., Fiore, A. M., Horowitz, L. W., Lamarque, J.-F., Lin, M., Prather, M. J., Young, P. J., Bergmann, D., Cameron-Smith, P. J., Cionni, I., Collins, W. J., Dalsøren, S. B., Doherty, R., Eyring, V., Faluvegi, G., Folberth, G. A., Josse, B., Lee, Y. H., MacKenzie, I. A., Nagashima, T., van Noije, T. P. C., Plummer, D. A., Righi, M., Rumbold, S. T., Skeie, R., Shindell, D. T., Stevenson, D. S., Strode, S., Sudo, K., Szopa, S., and Zeng, G.: Preindustrial to present-day changes in tropospheric hydroxyl radical and methane lifetime from the Atmospheric Chemistry and Climate Model Intercomparison Project (ACCMIP), Atmos. Chem. Phys., 13, 5277–5298, https://doi.org/10.5194/acp-13-5277-2013, 2013. a
Naus, S., Montzka, S. A., Pandey, S., Basu, S., Dlugokencky, E. J., and Krol, M.: Constraints and biases in a tropospheric two-box model of OH, Atmos. Chem. Phys., 19, 407–424, https://doi.org/10.5194/acp-19-407-2019, 2019. a
Nguyen, N. H., Turner, A. J., Yin, Y., Prather, M. J., and Frankenberg, C.: Effects of Chemical Feedbacks on Decadal Methane Emissions Estimates, Geophys. Res. Lett., 47, e2019GL085706, https://doi.org/10.1029/2019GL085706, 2020. a, b
Nicely, J. M., Canty, T. P., Manyin, M., Oman, L. D., Salawitch, R. J., Steenrod, S. D., Strahan, S. E., and Strode, S. A.: Changes in Global Tropospheric OH Expected as a Result of Climate Change Over the Last Several Decades, J. Geophys. Res.-Atmos., 123, 10774–10795, https://doi.org/10.1029/2018JD028388, 2018. a
Nisbet, E. G., Dlugokencky, E. J., and Bousquet, P.: Methane on the Rise–Again, Science, 343, 493–495, https://doi.org/10.1126/science.1247828, 2014. a
Nisbet, E. G., Dlugokencky, E. J., Manning, M. R., Lowry, D., Fisher, R. E., France, J. L., Michel, S. E., Miller, J. B., White, J. W. C., Vaughn, B., Bousquet, P., Pyle, J. A., Warwick, N. J., Cain, M., Brownlow, R., Zazzeri, G., Lanoisellé, M., Manning, A. C., Gloor, E., Worthy, D. E. J., Brunke, E.-G., Labuschagne, C., Wolff, E. W., and Ganesan, A. L.: Rising atmospheric methane: 2007–2014 growth and isotopic shift, Global Biogeochem. Cy., 30, 1356–1370, https://doi.org/10.1002/2016GB005406, 2016. a, b
Nisbet, E. G., Manning, M. R., Dlugokencky, E. J., Fisher, R. E., Lowry, D., Michel, S. E., Myhre, C. L., Platt, S. M., Allen, G., Bousquet, P., Brownlow, R., Cain, M., France, J. L., Hermansen, O., Hossaini, R., Jones, A. E., Levin, I., Manning, A. C., Myhre, G., Pyle, J. A., Vaughn, B., Warwick, N. J., and White, J. W. C.: Very strong atmospheric methane growth in the four years 2014-2017: Implications for the Paris Agreement, Global Biogeochem. Cy., 33, 318–342, https://doi.org/10.1029/2018GB006009, 2019. a, b
Pandey, S., Houweling, S., Krol, M., Aben, I., Monteil, G., Nechita-Banda, N., Dlugokencky, E. J., Detmers, R., Hasekamp, O., Xu, X., Riley, W. J., Poulter, B., Zhang, Z., McDonald, K. C., White, J. W. C., Bousquet, P., and Röckmann, T.: Enhanced methane emissions from tropical wetlands during the 2011 La Niña, Sci. Rep.-UK, 7, 45759, https://doi.org/10.1038/srep45759, 2017. a, b
Pandey, S., Houweling, S., Krol, M., Aben, I., Nechita-Banda, N., Thoning, K., Röckmann, T., Yin, Y., Segers, A., and Dlugokencky, E. J.: Influence of Atmospheric Transport on Estimates of Variability in the Global Methane Burden, Geophys. Res. Lett., 46, 2302– 2311, https://doi.org/10.1029/2018GL081092, 2019. a
Parker, R. J., Boesch, H., Byckling, K., Webb, A. J., Palmer, P. I., Feng, L., Bergamaschi, P., Chevallier, F., Notholt, J., Deutscher, N., Warneke, T., Hase, F., Sussmann, R., Kawakami, S., Kivi, R., Griffith, D. W. T., and Velazco, V.: Assessing 5 years of GOSAT Proxy XCH4 data and associated uncertainties, Atmos. Meas. Tech., 8, 4785–4801, https://doi.org/10.5194/amt-8-4785-2015, 2015. a, b, c, d
Parker, R. J., Boesch, H., McNorton, J., Comyn-Platt, E., Gloor, M., Wilson, C., Chipperfield, M. P., Hayman, G. D., and Bloom, A. A.: Evaluating year-to-year anomalies in tropical wetland methane emissions using satellite CH4 observations, Remote Sens. Environ., 211, 261–275, https://doi.org/10.1016/J.RSE.2018.02.011, 2018. a
Parker, R. J., Webb, A., Boesch, H., Somkuti, P., Barrio Guillo, R., Di Noia, A., Kalaitzi, N., Anand, J. S., Bergamaschi, P., Chevallier, F., Palmer, P. I., Feng, L., Deutscher, N. M., Feist, D. G., Griffith, D. W. T., Hase, F., Kivi, R., Morino, I., Notholt, J., Oh, Y.-S., Ohyama, H., Petri, C., Pollard, D. F., Roehl, C., Sha, M. K., Shiomi, K., Strong, K., Sussmann, R., Té, Y., Velazco, V. A., Warneke, T., Wennberg, P. O., and Wunch, D.: A decade of GOSAT Proxy satellite CH4 observations, Earth Syst. Sci. Data, 12, 3383–3412, https://doi.org/10.5194/essd-12-3383-2020, 2020. a
Patra, P. K., Houweling, S., Krol, M., Bousquet, P., Belikov, D., Bergmann, D., Bian, H., Cameron-Smith, P., Chipperfield, M. P., Corbin, K., Fortems-Cheiney, A., Fraser, A., Gloor, E., Hess, P., Ito, A., Kawa, S. R., Law, R. M., Loh, Z., Maksyutov, S., Meng, L., Palmer, P. I., Prinn, R. G., Rigby, M., Saito, R., and Wilson, C.: TransCom model simulations of CH4 and related species: linking transport, surface flux and chemical loss with CH4 variability in the troposphere and lower stratosphere, Atmos. Chem. Phys., 11, 12813–12837, https://doi.org/10.5194/acp-11-12813-2011, 2011. a, b
Pison, I., Bousquet, P., Chevallier, F., Szopa, S., and Hauglustaine, D.: Multi-species inversion of CH4, CO and H2 emissions from surface measurements, Atmos. Chem. Phys., 9, 5281–5297, https://doi.org/10.5194/acp-9-5281-2009, 2009. a, b
Prather, M. J.: Lifetimes and eigenstates in atmospheric chemistry, Geophys. Res. Lett., 21, 801–804, https://doi.org/10.1029/94GL00840, 1994. a
Prinn, R. G., Weiss, R. F., Arduini, J., Arnold, T., DeWitt, H. L., Fraser, P. J., Ganesan, A. L., Gasore, J., Harth, C. M., Hermansen, O., Kim, J., Krummel, P. B., Li, S., Loh, Z. M., Lunder, C. R., Maione, M., Manning, A. J., Miller, B. R., Mitrevski, B., Mühle, J., O'Doherty, S., Park, S., Reimann, S., Rigby, M., Saito, T., Salameh, P. K., Schmidt, R., Simmonds, P. G., Steele, L. P., Vollmer, M. K., Wang, R. H., Yao, B., Yokouchi, Y., Young, D., and Zhou, L.: History of chemically and radiatively important atmospheric gases from the Advanced Global Atmospheric Gases Experiment (AGAGE), Earth Syst. Sci. Data, 10, 985–1018, https://doi.org/10.5194/essd-10-985-2018, 2018. a
Rigby, M., Montzka, S. A., Prinn, R. G., White, J. W. C., Young, D., O'Doherty, S., Lunt, M. F., Ganesan, A. L., Manning, A. J., Simmonds, P. G., Salameh, P. K., Harth, C. M., Mühle, J., Weiss, R. F., Fraser, P. J., Steele, L. P., Krummel, P. B., McCulloch, A., and Park, S.: Role of atmospheric oxidation in recent methane growth, P. Natl. Acad. Sci. USA, 114, 5373–5377, https://doi.org/10.1073/pnas.1616426114, 2017. a, b
Rodgers, C. D.: Inverse Methods for Atmospheric Sounding: Theory and Practice, World Scientific, River Edge, USA, https://doi.org/10.1142/3171, 2000. a
Rubino, M., Etheridge, D. M., Thornton, D. P., Howden, R., Allison, C. E., Francey, R. J., Langenfelds, R. L., Steele, L. P., Trudinger, C. M., Spencer, D. A., Curran, M. A. J., van Ommen, T. D., and Smith, A. M.: Revised records of atmospheric trace gases CO2, CH4, N2O, and δ13C-CO2 over the last 2000 years from Law Dome, Antarctica, Earth Syst. Sci. Data, 11, 473–492, https://doi.org/10.5194/essd-11-473-2019, 2019. a
Saunois, M., Bousquet, P., Poulter, B., Peregon, A., Ciais, P., Canadell, J. G., Dlugokencky, E. J., Etiope, G., Bastviken, D., Houweling, S., Janssens-Maenhout, G., Tubiello, F. N., Castaldi, S., Jackson, R. B., Alexe, M., Arora, V. K., Beerling, D. J., Bergamaschi, P., Blake, D. R., Brailsford, G., Brovkin, V., Bruhwiler, L., Crevoisier, C., Crill, P., Covey, K., Curry, C., Frankenberg, C., Gedney, N., Höglund-Isaksson, L., Ishizawa, M., Ito, A., Joos, F., Kim, H.-S., Kleinen, T., Krummel, P., Lamarque, J.-F., Langenfelds, R., Locatelli, R., Machida, T., Maksyutov, S., McDonald, K. C., Marshall, J., Melton, J. R., Morino, I., Naik, V., O'Doherty, S., Parmentier, F.-J. W., Patra, P. K., Peng, C., Peng, S., Peters, G. P., Pison, I., Prigent, C., Prinn, R., Ramonet, M., Riley, W. J., Saito, M., Santini, M., Schroeder, R., Simpson, I. J., Spahni, R., Steele, P., Takizawa, A., Thornton, B. F., Tian, H., Tohjima, Y., Viovy, N., Voulgarakis, A., van Weele, M., van der Werf, G. R., Weiss, R., Wiedinmyer, C., Wilton, D. J., Wiltshire, A., Worthy, D., Wunch, D., Xu, X., Yoshida, Y., Zhang, B., Zhang, Z., and Zhu, Q.: The global methane budget 2000–2012, Earth Syst. Sci. Data, 8, 697–751, https://doi.org/10.5194/essd-8-697-2016, 2016. a, b, c
Saunois, M., Bousquet, P., Poulter, B., Peregon, A., Ciais, P., Canadell, J. G., Dlugokencky, E. J., Etiope, G., Bastviken, D., Houweling, S., Janssens-Maenhout, G., Tubiello, F. N., Castaldi, S., Jackson, R. B., Alexe, M., Arora, V. K., Beerling, D. J., Bergamaschi, P., Blake, D. R., Brailsford, G., Bruhwiler, L., Crevoisier, C., Crill, P., Covey, K., Frankenberg, C., Gedney, N., Höglund-Isaksson, L., Ishizawa, M., Ito, A., Joos, F., Kim, H.-S., Kleinen, T., Krummel, P., Lamarque, J.-F., Langenfelds, R., Locatelli, R., Machida, T., Maksyutov, S., Melton, J. R., Morino, I., Naik, V., O'Doherty, S., Parmentier, F.-J. W., Patra, P. K., Peng, C., Peng, S., Peters, G. P., Pison, I., Prinn, R., Ramonet, M., Riley, W. J., Saito, M., Santini, M., Schroeder, R., Simpson, I. J., Spahni, R., Takizawa, A., Thornton, B. F., Tian, H., Tohjima, Y., Viovy, N., Voulgarakis, A., Weiss, R., Wilton, D. J., Wiltshire, A., Worthy, D., Wunch, D., Xu, X., Yoshida, Y., Zhang, B., Zhang, Z., and Zhu, Q.: Variability and quasi-decadal changes in the methane budget over the period 2000–2012, Atmos. Chem. Phys., 17, 11135–11161, https://doi.org/10.5194/acp-17-11135-2017, 2017. a, b, c
Saunois, M., Stavert, A. R., Poulter, B., Bousquet, P., Canadell, J. G., Jackson, R. B., Raymond, P. A., Dlugokencky, E. J., Houweling, S., Patra, P. K., Ciais, P., Arora, V. K., Bastviken, D., Bergamaschi, P., Blake, D. R., Brailsford, G., Bruhwiler, L., Carlson, K. M., Carrol, M., Castaldi, S., Chandra, N., Crevoisier, C., Crill, P. M., Covey, K., Curry, C. L., Etiope, G., Frankenberg, C., Gedney, N., Hegglin, M. I., Höglund-Isaksson, L., Hugelius, G., Ishizawa, M., Ito, A., Janssens-Maenhout, G., Jensen, K. M., Joos, F., Kleinen, T., Krummel, P. B., Langenfelds, R. L., Laruelle, G. G., Liu, L., Machida, T., Maksyutov, S., McDonald, K. C., McNorton, J., Miller, P. A., Melton, J. R., Morino, I., Müller, J., Murguia-Flores, F., Naik, V., Niwa, Y., Noce, S., O'Doherty, S., Parker, R. J., Peng, C., Peng, S., Peters, G. P., Prigent, C., Prinn, R., Ramonet, M., Regnier, P., Riley, W. J., Rosentreter, J. A., Segers, A., Simpson, I. J., Shi, H., Smith, S. J., Steele, L. P., Thornton, B. F., Tian, H., Tohjima, Y., Tubiello, F. N., Tsuruta, A., Viovy, N., Voulgarakis, A., Weber, T. S., van Weele, M., van der Werf, G. R., Weiss, R. F., Worthy, D., Wunch, D., Yin, Y., Yoshida, Y., Zhang, W., Zhang, Z., Zhao, Y., Zheng, B., Zhu, Q., Zhu, Q., and Zhuang, Q.: The Global Methane Budget 2000–2017, Earth Syst. Sci. Data, 12, 1561–1623, https://doi.org/10.5194/essd-12-1561-2020, 2020. a, b, c
Schaefer, H.: On the Causes and Consequences of Recent Trends in Atmospheric Methane, Curr. Clim. Change Rep., 5, 259–274, https://doi.org/10.1007/s40641-019-00140-z, 2019. a, b
Schaefer, H., Fletcher, S. E. M., Veidt, C., Lassey, K. R., Brailsford, G. W., Bromley, T. M., Dlugokencky, E. J., Michel, S. E., Miller, J. B., Levin, I., Lowe, D. C., Martin, R. J., Vaughn, B. H., and White, J. W. C.: A 21st century shift from fossil-fuel to biogenic methane emissions indicated by 13CH4, Science, 352, 80–84, https://doi.org/10.1126/science.aad2705, 2016. a
Sheng, J.-X., Jacob, D. J., Turner, A. J., Maasakkers, J. D., Sulprizio, M. P., Bloom, A. A., Andrews, A. E., and Wunch, D.: High-resolution inversion of methane emissions in the Southeast US using SEAC4RS aircraft observations of atmospheric methane: anthropogenic and wetland sources, Atmos. Chem. Phys., 18, 6483–6491, https://doi.org/10.5194/acp-18-6483-2018, 2018. a
Sherwood, O. A., Schwietzke, S., Arling, V. A., and Etiope, G.: Global Inventory of Gas Geochemistry Data from Fossil Fuel, Microbial and Burning Sources, version 2017, Earth Syst. Sci. Data, 9, 639–656, https://doi.org/10.5194/essd-9-639-2017, 2017. a
Simpson, I. J., Sulbaek Andersen, M. P., Meinardi, S., Bruhwiler, L., Blake, N. J., Helmig, D., Rowland, F. S., and Blake, D. R.: Long-term decline of global atmospheric ethane concentrations and implications for methane, Nature, 488, 490–494, https://doi.org/10.1038/nature11342, 2012. a
Terentieva, I. E., Glagolev, M. V., Lapshina, E. D., Sabrekov, A. F., and Maksyutov, S.: Mapping of West Siberian taiga wetland complexes using Landsat imagery: implications for methane emissions, Biogeosciences, 13, 4615–4626, https://doi.org/10.5194/bg-13-4615-2016, 2016. a
Thompson, R. L., Nisbet, E. G., Pisso, I., Stohl, A., Blake, D., Dlugokencky, E. J., Helmig, D., and White, J. W. C.: Variability in Atmospheric Methane From Fossil Fuel and Microbial Sources Over the Last Three Decades, Geophys. Res. Lett., 45, 11499–11508, https://doi.org/10.1029/2018GL078127, 2018. a
Thoning, K. W., Tans, P. P., and Komhyr, W. D.: Atmospheric carbon dioxide at Mauna Loa Observatory: 2. Analysis of the NOAA GMCC data, 1974–1985, J. Geophys. Res.-Atmos., 94, 8549–8565, https://doi.org/10.1029/JD094iD06p08549, 1989. a
Turner, A. J., Jacob, D. J., Wecht, K. J., Maasakkers, J. D., Lundgren, E., Andrews, A. E., Biraud, S. C., Boesch, H., Bowman, K. W., Deutscher, N. M., Dubey, M. K., Griffith, D. W. T., Hase, F., Kuze, A., Notholt, J., Ohyama, H., Parker, R., Payne, V. H., Sussmann, R., Sweeney, C., Velazco, V. A., Warneke, T., Wennberg, P. O., and Wunch, D.: Estimating global and North American methane emissions with high spatial resolution using GOSAT satellite data, Atmos. Chem. Phys., 15, 7049–7069, https://doi.org/10.5194/acp-15-7049-2015, 2015. a
Turner, A. J., Frankenberg, C., Wennberg, P. O., and Jacob, D. J.: Ambiguity in the causes for decadal trends in atmospheric methane and hydroxyl, P. Natl. Acad. Sci. USA, 114, 5367–5372, https://doi.org/10.1073/pnas.1616020114, 2017. a, b
Turner, A. J., Fung, I., Naik, V., Horowitz, L. W., and Cohen, R. C.: Modulation of hydroxyl variability by ENSO in the absence of external forcing, P. Natl. Acad. Sci. USA, 115, 8931–8936, https://doi.org/10.1073/pnas.1807532115, 2018. a
Turner, A. J., Frankenberg, C., and Kort, E. A.: Interpreting contemporary trends in atmospheric methane, P. Natl. Acad. Sci. USA, 116, 2805–2813, https://doi.org/10.1073/PNAS.1814297116, 2019. a, b
Whalen, S.: Biogeochemistry of Methane Exchange between Natural Wetlands and the Atmosphere, Environ. Eng. Sci., 22, 73–94, https://doi.org/10.1089/ees.2005.22.73, 2005. a
Wolfe, G. M., Nicely, J. M., St. Clair, J. M., Hanisco, T. F., Liao, J., Oman, L. D., Brune, W. B., Miller, D., Thames, A., González Abad, G., Ryerson, T. B., Thompson, C. R., Peischl, J., McKain, K., Sweeney, C., Wennberg, P. O., Kim, M., Crounse, J. D., Hall, S. R., Ullmann, K., Diskin, G., Bui, P., Chang, C., and Dean-Day, J.: Mapping hydroxyl variability throughout the global remote troposphere via synthesis of airborne and satellite formaldehyde observations, P. Natl. Acad. Sci. USA, 116, 11171–11180, https://doi.org/10.1073/pnas.1821661116, 2019. a
Worden, J. R., Bloom, A. A., Pandey, S., Jiang, Z., Worden, H. M., Walker, T. W., Houweling, S., and Röckmann, T.: Reduced biomass burning emissions reconcile conflicting estimates of the post-2006 atmospheric methane budget, Nat. Commun., 8, 2227, https://doi.org/10.1038/s41467-017-02246-0, 2017. a, b
Wunch, D., Toon, G. C., Blavier, J.-F. L., Washenfelder, R. A., Notholt, J., Connor, B. J., Griffith, D. W. T., Sherlock, V., and Wennberg, P. O.: The total carbon column observing network, Philos. T. R. Soc. A, 369, 2087–112, https://doi.org/10.1098/rsta.2010.0240, 2011. a
Yin, Y.: Global methane inversion ensemble using LMDzPYVAR for 2010–2017, Caltech Data Center [data set], https://doi.org/10.22002/D1.2079, 2021. a
Yin, Y., Chevallier, F., Ciais, P., Broquet, G., Fortems-Cheiney, A., Pison, I., and Saunois, M.: Decadal trends in global CO emissions as seen by MOPITT, Atmos. Chem. Phys., 15, 13433–13451, https://doi.org/10.5194/acp-15-13433-2015, 2015. a, b, c, d
Yin, Y., Ciais, P., Chevallier, F., van der Werf, G. R., Fanin, T., Broquet, G., Boesch, H., Cozic, A., Hauglustaine, D., Szopa, S., and Wang, Y.: Variability of fire carbon emissions in Equatorial Asia and its non-linear sensitivity to El Niño, Geophys. Res. Lett., 43, 10472–10479, https://doi.org/10.1002/2016GL070971, 2016. a, b
Zhang, Y., Jacob, D. J., Lu, X., Maasakkers, J. D., Scarpelli, T. R., Sheng, J.-X., Shen, L., Qu, Z., Sulprizio, M. P., Chang, J., Bloom, A. A., Ma, S., Worden, J., Parker, R. J., and Boesch, H.: Attribution of the accelerating increase in atmospheric methane during 2010–2018 by inverse analysis of GOSAT observations, Atmos. Chem. Phys., 21, 3643–3666, https://doi.org/10.5194/acp-21-3643-2021, 2021. a, b, c, d
Zhang, Z., Zimmermann, N. E., Stenke, A., Li, X., Hodson, E. L., Zhu, G., Huang, C., and Poulter, B.: Emerging role of wetland methane emissions in driving 21st century climate change, P. Natl. Acad. Sci. USA, 114, 9647–9652, https://doi.org/10.1073/pnas.1618765114, 2017. a
Zheng, B., Chevallier, F., Yin, Y., Ciais, P., Fortems-Cheiney, A., Deeter, M. N., Parker, R. J., Wang, Y., Worden, H. M., and Zhao, Y.: Global atmospheric carbon monoxide budget 2000–2017 inferred from multi-species atmospheric inversions, Earth Syst. Sci. Data, 11, 1411–1436, https://doi.org/10.5194/essd-11-1411-2019, 2019. a, b